Month: June 2024
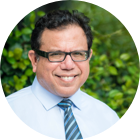
MMS • RSS
Posted on mongodb google news. Visit mongodb google news
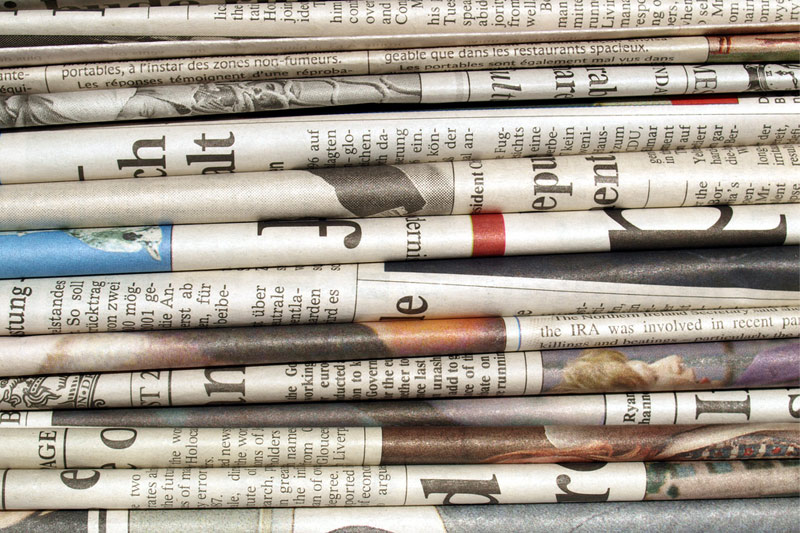
MongoDB has reportedly experienced a slower operational start due to changes in its go-to-market strategy and a more moderate level of activity from end-users than was anticipated. This slowdown is notable as it contrasts with other companies in the consumption business sector, such as Elastic (NYSE:ESTC), Snowflake (NYSE:SNOW), and Datadog (NASDAQ:DDOG), which have not reported similar challenges in the broader macroeconomic environment.
Scotiabank’s outlook for MongoDB has remained consistent since the firm began covering the stock. The bank does not foresee accelerated consolidation among database vendors impacting MongoDB in 2024. Additionally, while generative AI workloads are expected to provide tailwinds, these are not predicted to become a significant catalyst until 2025.
Furthermore, a rapid resurgence in demand for MongoDB’s services is deemed unlikely as the software is integral to core operational workloads, which were less subject to optimization in the previous year.
Despite these challenges, Scotiabank acknowledges MongoDB as a high-quality business. However, the firm advises investors to adopt a “wait and see” approach for the upcoming fiscal year, suggesting caution amidst the current market conditions and company-specific factors impacting MongoDB’s performance.
In light of Scotiabank’s recent price target revision for MongoDB (NASDAQ:MDB), it’s valuable to consider additional insights from InvestingPro. Notably, MongoDB holds more cash than debt on its balance sheet, which is a positive indicator of financial stability. Additionally, analysts forecast that the company’s net income is expected to grow this year, offering a glimpse of potential recovery and growth prospects despite the current headwinds.
From a data standpoint, MongoDB’s market capitalization stands at $17.32 billion, with a high revenue growth rate of over 31% over the last twelve months as of Q4 2024. However, the company is trading near its 52-week low, reflecting the recent price pressure. The price, at the previous close, was $236.06, which is significantly lower than the fair value estimates by analysts at $320, suggesting a potential undervaluation according to market experts.
For investors looking for a deeper dive into MongoDB’s financial health and future prospects, InvestingPro offers additional InvestingPro Tips to help make informed decisions. Use coupon code PRONEWS24 to get an additional 10% off a yearly or biyearly Pro and Pro+ subscription, unlocking access to a suite of professional-grade tools and analytics. With 15 additional tips available on InvestingPro, subscribers can gain a more comprehensive understanding of MongoDB’s position in the market.
This article was generated with the support of AI and reviewed by an editor. For more information see our T&C.
Article originally posted on mongodb google news. Visit mongodb google news
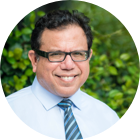
MMS • RSS
Posted on nosqlgooglealerts. Visit nosqlgooglealerts
The capacity to quickly store and analyze highly related data has led to graph databases’ meteoric popularity in the past few years. Applications like social networks, recommendation engines, and fraud detection benefit greatly from graph databases, which differ from conventional relational databases’ ability to depict complicated relationships between elements.
What are Graph Databases?
Graph databases are a subset of NoSQL databases that store and display data using graph structures. They include “nodes,” which stand in for things, and “edges,” which show their connections. Using a graph-based method, queries of highly connected data may be efficiently and flexibly executed, facilitating the discovery of patterns and insights.
Advantages
There are three main benefits to using a graph database instead of a relational one.
- High Flexibility: The graph database’s schema can be changed to suit different applications without impacting current functions, making it very flexible.
- High Performance: A graph database maintains superior performance even when dealing with intricate transactions. Deep analytics work in a similar manner.
- Graph databases are more efficient than relational databases due to the shortness of graph queries and the speed with which relationships can be traversed.
Everyone can view and contribute to the source code of an open-source graph database. The code is published on GitHub. In 2024, companies could benefit from the following open-source graph databases:
As a native graph database incorporating a graph model down to the storage level, Neo4j is among the most well-known and long-running open-source graph databases, boasting over 12,000 stars on GitHub. It offers ACID transactions, cluster support, and runtime failover.
WhiteDB is a C-based NoSQL database package that runs entirely in main memory and is very lightweight. No server process is available. There is no need for sockets because WhiteDB and the application program read and write data directly from and to shared memory.
ArangoDB is a free and open-source graph database that prioritizes speed and scalability. More than 13,000 people have given it a star on GitHub. It combines an integrated search engine, native graphing, JSON compatibility, and various data access patterns with a single query language.
Stardog is a platform for knowledge graphs that combines features of graph databases and semantic reasoning. It has sophisticated tools for creating and querying knowledge graphs and supports SPARQL.
A native graph database with over 19,000 stars on GitHub, Dgraph supports native GraphQL. This open-source database is known for its speed, scalability, distributed nature, and high availability. Dgraph instantly resolves queries through graph navigation and is designed to handle big data volumes.
Memgraph is an open-source, in-memory graph database with over 2000 stars on GitHub. It may be used either on-premises or in the cloud. Memgraph Platform is an on-premises option for businesses, while Memgraph Cloud is a completely managed service that eliminates the need for an administrator.
Orly is a non-relational database designed to handle massive user loads quickly and efficiently. Because of its speed and high concurrency, we won’t need memcache anymore; it gives a single path to data.
Graph Engine (GE) is a distributed graph processing engine that runs in memory. It is supported by a general compute engine and strongly typed RAM storage. Over a network of computers, the distributed RAM store offers a high-performance key-value store that can be accessed from anywhere in the world. Using the RAM store, GE makes it possible to access massive dispersed data sets quickly and arbitrarily.
Aerospike Graph, a distributed graph database built on top of the Aerospike Database, enables users to store and query massive volumes of data without sacrificing performance. This open-source database employs Apache TinkerPop, a graph computing platform, to perform online transactions and analytical graph queries.
With over 4,500 stars on GitHub, OrientDB is a dependable graph database that is quick, adaptable, and extensible. This multi-model database supports graph, document, full-text, and geographic models and doesn’t necessitate costly runtime JOINs. Additionally, this database supports ACID and SQL transactions.
One free RDF triplestore that supports desktop computers is GraphDB Lite, which can hold up to 100 million triples. The JAVA platform makes it easy to deploy this GraphDB version. Instead of relying on indexes stored in files, SPARQL 1.1 queries are executed entirely in memory. Inferencing reasoning operations are GraphDB Lite-compatible.
Cayley, an open-source graph database inspired by Google’s Knowledge Graph, has received over 14,000 stars on GitHub. The Go-based database has RDF support and can be used on top of any pre-existing SQL or NoSQL database. The user can add new query languages or custom logic to Cayley, and the database can be connected to any programming language. This makes Cayley highly modular. Multiple query languages, including GraphQL and MQL, are built into it.
The MapGraph API simplifies the process of creating GPU-based graph analytics with great performance. The API’s basis is the Gather-Apply-Scatter (GAS) model, which is employed in GraphLab. The CUDA kernels in MapGraph employ various advanced techniques, including vertex-degree-dependent dynamic parallelism granularity and frontier compaction, to execute high-performance computations and effectively use GPUs’ high memory bandwidth.
The distributed graph store Weaver features horizontal scalability, excellent performance, and good consistency. Through a straightforward Python API, Weaver allows users to perform transactional graph modifications and searches.
Virtuoso, an open-source graph database developed by OpenLink, has over 800 stars on GitHub. A data virtualization platform and a DBMS supporting many models are part of its functionality. This database is highly available, fast, and scalable; it doesn’t rely on complicated queries or heavy usage to do so.
Filament is a navigational query style-based graph persistence framework with accompanying toolkits. The storage model can be customized, but it stores graph objects and their properties in basic relational databases by default.
The Linux Foundation is behind the JanusGraph project, which has over 5,000 stars on GitHub. With its multi-machine cluster and highly scalable graph database, it can manage massive graphs with billions of nodes and edges, handle graph queries, and perform complicated traversals.
Titan is a transaction database for graphs that can scale to store and query data from distributed graphs with hundreds of billions of edges and vertices. Titan can handle hundreds of users running complicated graph traversals in real-time.
Directed hypergraphs are the basis of HyperGraphDB, an open-source database that may be expanded upon. It has robust data modeling, knowledge representation capabilities, and customizable indexing. It can read and write data concurrently without blocking and is completely transactional and multi-threaded. This database has more than 190 stars on GitHub.
Storage for massive amounts of dispersed, highly connected, semi-structured data is made easy with Sones GraphDB, an object-oriented graph database.
Dhanshree Shenwai is a Computer Science Engineer and has a good experience in FinTech companies covering Financial, Cards & Payments and Banking domain with keen interest in applications of AI. She is enthusiastic about exploring new technologies and advancements in today’s evolving world making everyone’s life easy.
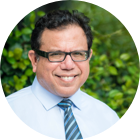
MMS • RSS
Posted on mongodb google news. Visit mongodb google news
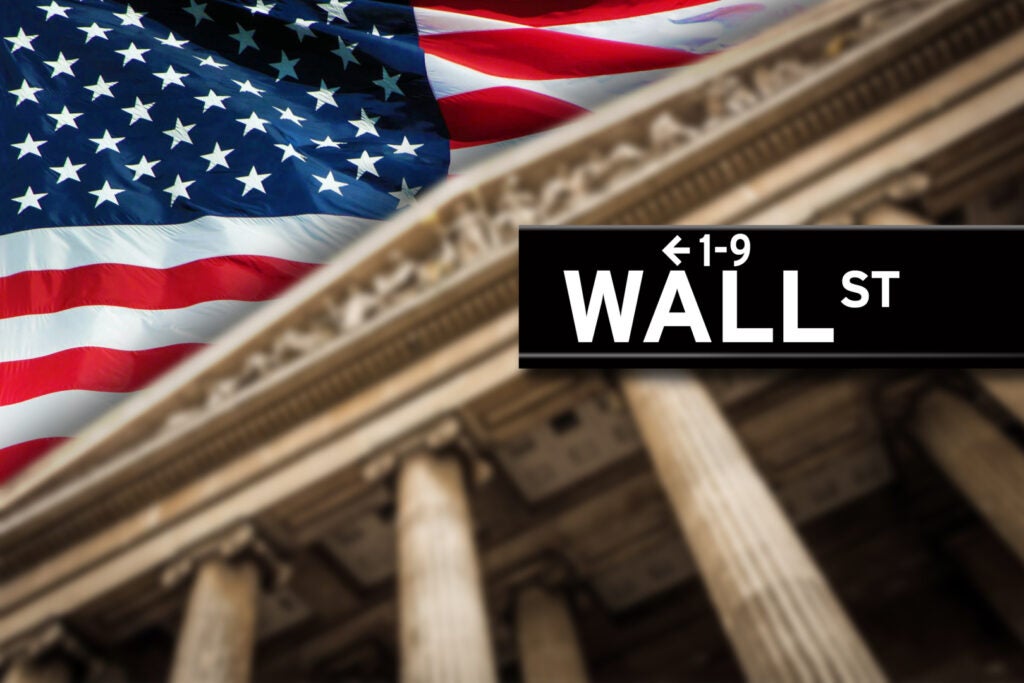
Top Wall Street analysts changed their outlook on these top names. For a complete view of all analyst rating changes, including upgrades and downgrades, please see our analyst ratings page.
- Guggenheim analyst Howard Ma upgraded the rating for MongoDB, Inc. MDB from Sell to Neutral. MongoDB shares fell 23.9% to close at $236.06 on Friday. See how other analysts view this stock.
- Keefe, Bruyette & Woods analyst Bose George upgraded Radian Group Inc. RDN from Market Perform to Outperform, while raising the price target from $35 to $36. Independent Bank shares rose 0.9% to settle at $31.24 on Friday. See how other analysts view this stock.
- RBC Capital analyst Nik Modi upgraded the rating for Kimberly-Clark Corporation KMB from Sector Perform to Outperform and boosted the price target from $126 to $165. Kimberly-Clark shares rose 2.8% to settle at $133.30 on Friday. See how other analysts view this stock. See how other analysts view this stock.
- Keefe, Bruyette & Woods analyst Bose George upgraded MGIC Investment Corporation MTG from Market Perform to Outperform, while increasing the price target from $24 to $25. MGIC Investment shares rose 1.6% to close at $21.00 on Friday. See how other analysts view this stock.
- HSBC analyst Stephen Bersey upgraded the rating for Asana, Inc. ASAN from Reduce to Hold. Asana shares fell 0.6% to settle at $13.05 on Friday. See how other analysts view this stock.
Check This Out: Top 3 Energy Stocks That Could Blast Off This Month
Market News and Data brought to you by Benzinga APIs
© 2024 Benzinga.com. Benzinga does not provide investment advice. All rights reserved.
Article originally posted on mongodb google news. Visit mongodb google news
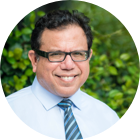
MMS • RSS
Posted on mongodb google news. Visit mongodb google news
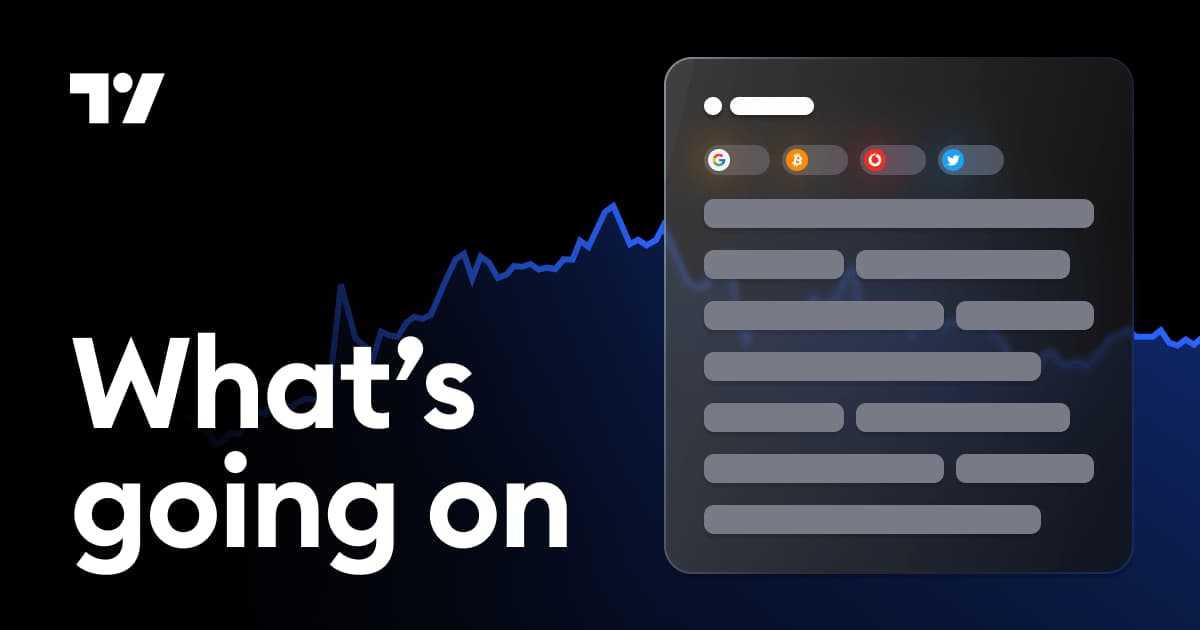
Top Wall Street analysts changed their outlook on these top names. For a complete view of all analyst rating changes, including upgrades and downgrades, please see our analyst ratings page.
Check This Out: Top 3 Energy Stocks That Could Blast Off This Month
Latest Ratings for MDB
Date | Firm | Action | From | To |
---|---|---|---|---|
Mar 2022 | Needham | Maintains | Buy | |
Mar 2022 | Mizuho | Maintains | Neutral | |
Mar 2022 | Barclays | Maintains | Overweight |
View More Analyst Ratings for MDB
View the Latest Analyst Ratings
© 2024 Benzinga.com. Benzinga does not provide investment advice. All rights reserved.
Article originally posted on mongodb google news. Visit mongodb google news
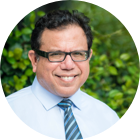
MMS • RSS
Posted on mongodb google news. Visit mongodb google news
U.S. stocks settled mostly higher on Friday, with the Dow Jones index recording its best session of the year.
However, U.S. stocks recorded losses last week, with the S&P 500 falling 0.51% and the Dow Jones index declining 0.98% during the week.
Wall Street analysts make new stock picks on a daily basis. Unfortunately for investors, not all analysts have particularly impressive track records at predicting market movements. Even when it comes to one single stock, analyst ratings and price targets can vary widely, leaving investors confused about which analyst’s opinion to trust.
Benzinga’s Analyst Ratings API is a collection of the highest-quality stock ratings curated by the Benzinga news desk via direct partnerships with major sell-side banks. Benzinga displays overnight ratings changes on a daily basis three hours prior to the U.S. equity market opening. Data specialists at investment dashboard provider Toggle.ai recently uncovered that the analyst insights Benzinga Pro subscribers and Benzinga readers regularly receive can successfully be used as trading indicators to outperform the stock market.
Top Analyst Picks: Fortunately, any Benzinga reader can access the latest analyst ratings on the Analyst Stock Ratings page. One of the ways traders can sort through Benzinga’s extensive database of analyst ratings is by analyst accuracy. Here’s a look at the most recent stock picks from each of the five most accurate Wall Street analysts, according to Benzinga Analyst Stock Ratings.
Analyst: Matt Bryson
- Analyst Firm: Wedbush
- Ratings Accuracy: 87%
- Latest Rating: Maintained a Neutral rating on NetApp, Inc. (NASDAQ:NTAP) and raised the price target from $100 to $120 on May 28. This analysts sees around 1% downside in the stock.
- Recent News: On May 30, NetApp posted better-than-expected quarterly earnings.
Analyst: Aaron Rakers
- Analyst Firm: Wells Fargo
- Ratings Accuracy: 87%
- Latest Rating: Maintained an Overweight rating on Pure Storage, Inc. (NYSE:PSTG) and increased the price target from $70 to $75 on May 30. This analyst sees around 23% upside in the stock.
- Recent News: On May 29, Pure Storage posted better-than-expected first-quarter financial results.
Analyst: Howard Rubel
- Analyst Firm: Jefferies
- Ratings Accuracy: 86%
- Latest Rating: Maintained a Buy rating on Booz Allen Hamilton Holding Corporation (NYSE:BAH) and raised the price target from $180 to $185 on May 29. This analyst sees around 22% upside in the stock.
- Recent News: On May 24, Booz Allen Hamilton posted better-than-expected fourth-quarter earnings.
Analyst: Alex Rygiel
- Analyst Firm: B. Riley Securities
- Ratings Accuracy: 86%
- Latest Rating: Maintained a Buy rating on Dycom Industries, Inc. (NYSE:DY) and boosted the price target from $172 to $205 on May 31. This analyst sees about 14% gain in the stock.
- Recent News: On May 22, Dycom Industries reported better-than-expected first-quarter financial results.
Analyst: William Power
- Analyst Firm: Baird
- Ratings Accuracy: 85%
- Latest Rating: Maintained an Outperform rating on MongoDB, Inc. (NASDAQ:MDB) and cut the price target from $450 to $305 on May 31. This analyst sees over 29% upside in the stock.
- Recent News: On May 30, MongoDB issued weak FY25 guidance.
Read More: Jim Cramer: Beyond Meat Is ‘Way Too Risky,’ Warby Parker Is ‘Alright To Buy‘
Article originally posted on mongodb google news. Visit mongodb google news
Java News Roundup: JEPs Targeted for JDK 23, JHipster 8.5, Gradle 8.8, Spring AI 1.0-M1
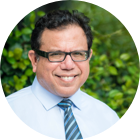
MMS • Michael Redlich
Article originally posted on InfoQ. Visit InfoQ
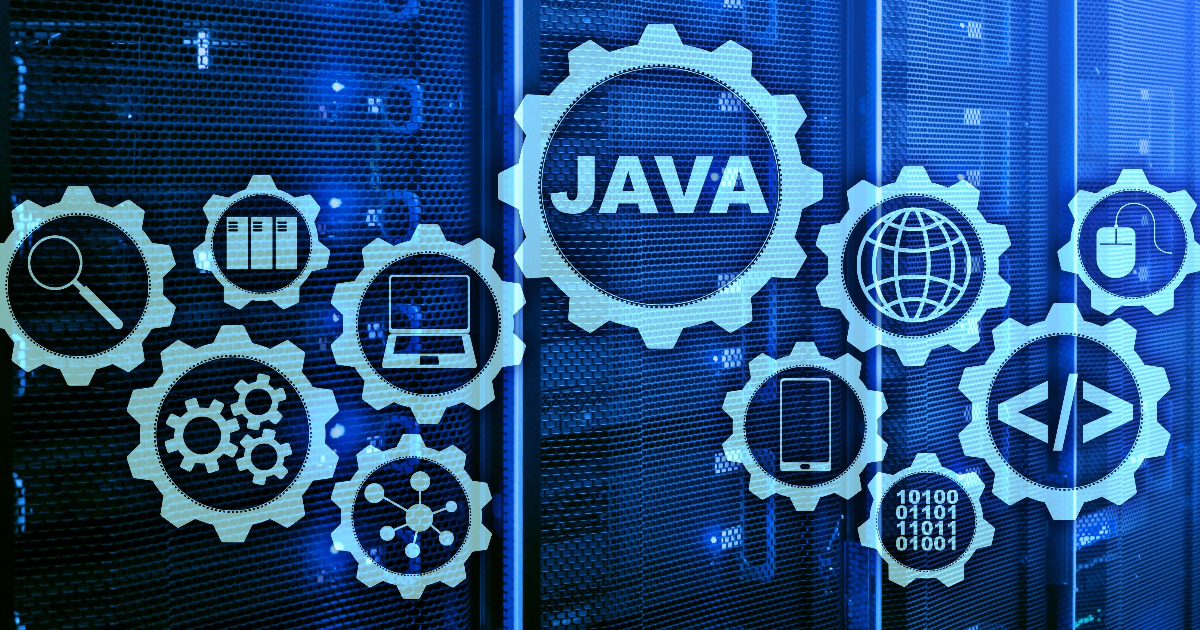
This week’s Java roundup for May 27th, 2024 features news highlighting: four JEPs targeted for JDK 23, namely: JEP 482, Flexible Constructor Bodies (Second Preview), JEP 481, Scoped Values (Third Preview), JEP 480, Structured Concurrency (Third Preview) and JEP 471, Deprecate the Memory-Access Methods in Unsafe for Removal; and the releases of JHipster 8.5, Gradle 8.8 and Spring AI 1.0-M1.
OpenJDK
After its review has concluded, JEP 482, Flexible Constructor Bodies (Second Preview), has been promoted from Proposed to Target to Targeted for JDK 23. This JEP proposes a second round of preview and a name change to obtain feedback from the previous round of preview, namely JEP 447, Statements before super(…) (Preview), delivered in JDK 22. This feature allows statements that do not reference an instance being created to appear before the this()
or super()
calls in a constructor; and preserve existing safety and initialization guarantees for constructors. Changes in this JEP include: a treatment of local classes; and a relaxation of the restriction that fields can not be accessed before an explicit constructor invocation to a requirement that fields can not be read before an explicit constructor invocation. Gavin Bierman, Consulting Member of Technical Staff at Oracle, has provided an initial specification of this JEP for the Java community to review and provide feedback.
After its review has concluded, JEP 481, Scoped Values (Third Preview), has been promoted from Proposed to Target to Targeted for JDK 23. Formerly known as Extent-Local Variables (Incubator), this JEP proposes a third preview, with one change, in order to gain additional experience and feedback from one round of incubation and two rounds of preview, namely: JEP 464, Scoped Values (Second Preview), delivered in JDK 22; JEP 446, Scoped Values (Preview), delivered in JDK 21; and JEP 429, Scoped Values (Incubator), delivered in JDK 20. This feature enables sharing of immutable data within and across threads. This is preferred to thread-local variables, especially when using large numbers of virtual threads. The change in this feature is related to the operation parameter of the callWhere()
method, defined in the ScopedValue
class, is now a functional interface which allows the Java compiler to infer whether a checked exception might be thrown. With this change, the getWhere()
method is no longer needed and has been removed.
After its review has concluded, JEP 480, Structured Concurrency (Third Preview), has been promoted from Proposed to Target to Targeted for JDK 23. This JEP proposes a third preview, without change, in order to gain more feedback from the previous two rounds of preview, namely: JEP 462, Structured Concurrency (Second Preview), delivered in JDK 22; and JEP 453, Structured Concurrency (Preview), delivered in JDK 21. This feature simplifies concurrent programming by introducing structured concurrency to “treat groups of related tasks running in different threads as a single unit of work, thereby streamlining error handling and cancellation, improving reliability, and enhancing observability.”
After its review has concluded, JEP 471, Deprecate the Memory-Access Methods in sun.misc.Unsafe for Removal, has been promoted from Proposed to Target to Targeted for JDK 23. This JEP proposes to deprecate the memory access methods in the Unsafe
class for removal in a future release. These unsupported methods have been superseded by standard APIs, namely; JEP 193, Variable Handles, delivered in JDK 9; and JEP 454, Foreign Function & Memory API, delivered in JDK 22.
Build 23-loom+4-102 of the Project Loom early-access builds are based on JDK 23 Build 25 and improves the implementation of Java monitors (synchronized methods) to work better with virtual threads.
Build 22-jextract+5-33 of the Project Jextract early-access builds, also known as Project Panama, are based on JDK 22 and provides a recommendation in which the quarantine attribute from the bits may need to be removed before using the jextract
binaries on macOS Catalina or later.
JDK 23
Build 25 of the JDK 23 early-access builds was made available this past week featuring updates from Build 24 that include fixes for various issues. Further details on this release may be found in the release notes.
Jakarta EE
In his weekly Hashtag Jakarta EE blog, Ivar Grimstad, Jakarta EE Developer Advocate at the Eclipse Foundation, has provided an additional update on the upcoming GA release of Jakarta EE 11.
Nine (9) specifications, namely – Jakarta Annotations 3.0, Jakarta Authorization 3.0, Jakarta Contexts and Dependency Injection 4.1, Jakarta Expression Language 6.0, Jakarta Interceptors 2.2, Jakarta RESTful Web Services 4.0, Jakarta Persistence 3.2, Jakarta Validation 3.1 and Jakarta WebSocket 2.2 – have been finalized for Jakarta EE 11.
Five (5) specifications, namely – Jakarta Concurrency 3.1, Jakarta Data 1.0, Jakarta Faces 4.1, Jakarta Pages 4.0 and Jakarta Servlet 6.1 – should have their respective reviews completed during the week of June 3, 2024.
And reviews for the remaining two (2) specifications, Jakarta Authentication 3.0 and Jakarta Security 4.0, should start during the week of June 10, 2024.
Discussing the remaining work required for the GA release, Grimstad stated:
There is some work to be done on the TCK and the specification documents before the release reviews of the Jakarta EE 11 Platform, Jakarta EE 11 Web Profile, and Jakarta EE 11 Core Profile specifications can start. This will likely happen in late June or early July, so we are on track according to the release plan.
GlassFish
GlassFish 7.0.15, fifteenth maintenance release in the 7.0.0 release train, provides improvements in documentation, dependency upgrades and notable resolutions to issues such as: a ClassCastException
due to improper classloader matching in the Weld BeanDeploymentArchive
interface; cluster monitoring data not being displayed in the Admin Console; and an IllegalArgumentException
in the toString()
method, defined in the Application
class, due to a bundle without descriptor being valid. More details on this release may be found in the release notes.
TornadoVM
TornadoVM 1.0.5, the fifth maintenance release, ships with bug fixes and improvements such as: support for Vector types at the API level in the variants of the Floatx
class with variants of Intx
class; an improved OpenCL build log; an improvements in the TornadoOptions
and TornadoLogger
classes where all options (debug, events, etc.) have been moved to the former and all debugging has been moved to the latter.
Spring Framework
It was a very quiet week at Spring compared to the release activity during the week of May 20, 2024.
Following the release of Spring Boot 3.3.0, 3.2.6 and 3.1.12, versions 3.0.16 and 2.7.21 were released this past week featuring many dependency upgrades and notable bug fixes: when graceful shutdown of Tomcat is aborted, it may report that it completed successfully; and resolving an instance of the BuildpackReference
class, created from a URL-like String, can fail on Windows. Further details on these releases may be found in the release notes for version 3.0.16 and version 2.7.21.
Spring Cloud 2023.0.2, codenamed Leyton, and 2022.0.7 Enterprise Edition have been released featuring featuring bug fixes and notable updates to sub-projects: Spring Cloud Kubernetes 3.1.2; Spring Cloud Function 4.1.2; Spring Cloud OpenFeign 4.1.2; Spring Cloud Stream 4.1.2; and Spring Cloud Gateway 4.1.4. This release is based on Spring Boot 3.3.0. More details on this release may be found in the release notes.
The first milestone release of Spring AI 1.0.0 delivers new features such as: a new ChatClient fluent API, composed of the ChatClient
interface and Builder
inner class, providing methods to construct an instance of a Prompt
class, which is then passed as input to an AI model; eight (8) new AI models; updated existing models; and support for Testcontainers. Developers interested in learning more can follow this example application.
Versions 3.3.0-RC1, 3.2.5 and 3.1.12 of Spring Shell have been released featuring a breaking change that replaces the use of the Spring Boot ApplicationArguments
interface with a plain String
array in the ShellRunner
interface and its implementations. Default methods to bridge new methods were created and old methods were deprecated with a plan to remove them in Spring Shell 3.4.x. This is part of on-going work to remove all Spring Boot classes from the Spring Shell core package. These versions build upon Spring Boot 3.3.0, 3.2.5 and 3.1.12, respectively, and JLine 3.26.1. Further details on these releases may be found in the release notes for version 3.3.0-RC1, version 3.2.5 and version 3.1.12.
Helidon
The release of Helidon 4.0.9 ships with notable changes such as: a resolution to an IndexOutOfBoundsException
generated from the first(String)
method, defined in the Parameters
interface, due to index 0 being out of bounds for an array of length 0; a refactor of the OCI metrics library code is organized so other implementations can extend common types without having to also include the OCI metrics library CDI module; and improvements to the WebClientSecurity
class against absent or disabled tracing. More details on this release may be found in the release notes.
Quarkus
The release of Quarkus 3.11 delivers notable changes such as: a new OidcRedirectFilter
interface to dynamically customize OIDC redirects; initial support of security integration for the WebSockets Next extension; and a new Infinispan Cache extension that will replace the now-deprecated @CacheResult
annotation. Further details on this release may be found in the release notes. InfoQ will follow up with a more detailed news story.
Micronaut
The Micronaut Foundation has released version 4.4.3 of the Micronaut Framework featuring Micronaut Core 4.4.10, bug fixes, improvements in documentation and updates to modules: Micronaut Data and Micronaut Test Resources. More details on this release may be found in the release notes.
WildFly
WildFly 32.0.1, first maintenance release, delivers component upgrades and notable resolutions to issues such as: a ClassCastException
when running live-only High Availability policy in the messaging-activemq
subsystem; a possible NoSuchElementException
upon deploying multiple OpenAPI endpoints; and restoration of the io.smallrye.opentelemetry
module to the OpenTelemetryDependencyProcessor
class as this module is required on the deployment classpath since that contains the CDI provider method providing the implementation of the OpenTelemetry Tracer
interface. Further details on this release may be found in the release notes.
Hibernate
The release of Hibernate Reactive 2.3.1.Final ships with dependency upgrades and a resolution to a NullPointerException
due to a method that converts the generated ID from the database to a ResultSet
was not implemented. This release is compatible with Hibernate ORM 6.5.2.Final. More details on this release may be found in the release notes.
Apache Software Foundation
Apache Maven 4.0.0-beta-3 provides bug fixes, dependency upgrades and improvements such as: initial support for Maven 4.0 API to be usable outside of the runtime; changes in the PathType
interface to control the type of path where each dependency can be placed; and support for the fatjar
, a dependency type to be used when dependency is considered self contained. Further details on this release may be found in the release notes.
Similarly, Apache Maven 3.9.7 ships with bug fixes, dependency upgrades and new features: support for wildcards in an OS version to specify wildcard in the OS version tag; and the ability to ignore transitive dependency repositories using the mvn
-itr
command. More details on this release may be found in the release notes.
Maintaining alignment with Quarkus, the release of Camel Quarkus 3.11.0, composed of Camel 4.6.0 and Quarkus 3.11.0, provides resolutions to notable issues such as: Quarkus Infinispan not compatible with Camel Infinispan due to differences in Infinispan version support; and improved auto-configuration by moving the options for the SupervisingRouteController
interface into its own group. Further details on this release may be found in the release notes.
JobRunr
Version 7.2.0 of JobRunr, a library for background processing in Java that is distributed and backed by persistent storage, has been released featuring bug fixes, dependency upgrades and new features such as: support for Spring Boot 3.3.0 where JobRunr now declares the spring-boot-starter
dependency with a provided
scope; support for Kotlin 2.0.0 (support for Kotlin 1.7.0 ahs been dropped); and deleting a job using the JobScheduler
or JobRequestScheduler
classes will be retried if a ConcurrentJobModificationException
is thrown. More details on this release may be found in the release notes.
JHipster
The release of JHipster 8.5.0 delivers: support for Spring Boot 3.3.0; a resolution to issues with OIDC claims in the SecurityUtils
class when the syncUserWithIdp
boolean is set to false
; and resolutions to multiple issues with SonarCloud, used by JHipster for code quality. Further details on this release may be found in the release notes. InfoQ will follow up with a more detailed news story.
JDKUpdater
Versions 14.0.53+75 and 14.0.51+73 of JDKUpdater, a new utility that provides developers the ability to keep track of updates related to builds of OpenJDK and GraalVM. Introduced in mid-March by Gerrit Grunwald, principal engineer at Azul, these releases include: an update in the base URL for CVE details; and modifications related to menu items. More details on this release may be found in the release notes for version 14.0.53+75 and version 14.0.51+73.
Gradle
The release of Gradle 8.8 delivers: full support for JDK 22; a preview feature to configure the Gradle daemon JVM using toolchains; improved IDE performance with large projects; and improvements to build authoring, error and warning messages, the build cache, and the configuration cache. Further details on this release may be found in the release notes.
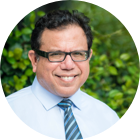
MMS • RSS
Posted on mongodb google news. Visit mongodb google news
MongoDB, Inc. (NASDAQ:MDB – Free Report) – Equities research analysts at DA Davidson issued their Q2 2025 earnings per share (EPS) estimates for MongoDB in a research report issued to clients and investors on Friday, May 31st. DA Davidson analyst R. Kessinger forecasts that the company will post earnings per share of ($0.73) for the quarter. DA Davidson currently has a “Strong-Buy” rating on the stock. The consensus estimate for MongoDB’s current full-year earnings is ($2.53) per share.
MongoDB (NASDAQ:MDB – Get Free Report) last posted its quarterly earnings data on Thursday, March 7th. The company reported ($1.03) EPS for the quarter, missing analysts’ consensus estimates of ($0.71) by ($0.32). The company had revenue of $458.00 million for the quarter, compared to analyst estimates of $431.99 million. MongoDB had a negative net margin of 11.50% and a negative return on equity of 16.00%.
MDB has been the topic of a number of other reports. Barclays lowered their price objective on MongoDB from $458.00 to $290.00 and set an “overweight” rating on the stock in a research report on Friday. Citigroup increased their price target on MongoDB from $515.00 to $550.00 and gave the stock a “buy” rating in a report on Wednesday, March 6th. JMP Securities reduced their price objective on MongoDB from $440.00 to $380.00 and set a “market outperform” rating for the company in a research report on Friday. Morgan Stanley cut their price objective on MongoDB from $455.00 to $320.00 and set an “overweight” rating for the company in a research report on Friday. Finally, Robert W. Baird lowered their price objective on MongoDB from $450.00 to $305.00 and set an “outperform” rating on the stock in a research note on Friday. Two investment analysts have rated the stock with a sell rating, four have issued a hold rating, nineteen have issued a buy rating and one has given a strong buy rating to the company’s stock. According to data from MarketBeat.com, the stock currently has an average rating of “Moderate Buy” and an average price target of $374.29.
View Our Latest Stock Analysis on MongoDB
MongoDB Trading Down 23.9 %
Shares of NASDAQ:MDB opened at $236.06 on Monday. The company has a current ratio of 4.40, a quick ratio of 4.40 and a debt-to-equity ratio of 1.07. MongoDB has a 1 year low of $225.25 and a 1 year high of $509.62. The firm has a fifty day moving average price of $353.32 and a 200 day moving average price of $390.71. The stock has a market capitalization of $17.19 billion, a PE ratio of -84.01 and a beta of 1.19.
Insider Activity
In other MongoDB news, CEO Dev Ittycheria sold 17,160 shares of the firm’s stock in a transaction that occurred on Tuesday, April 2nd. The shares were sold at an average price of $348.11, for a total value of $5,973,567.60. Following the transaction, the chief executive officer now directly owns 226,073 shares of the company’s stock, valued at approximately $78,698,272.03. The transaction was disclosed in a filing with the Securities & Exchange Commission, which is available at the SEC website. In other news, CEO Dev Ittycheria sold 17,160 shares of the firm’s stock in a transaction that occurred on Tuesday, April 2nd. The shares were sold at an average price of $348.11, for a total transaction of $5,973,567.60. Following the completion of the sale, the chief executive officer now owns 226,073 shares in the company, valued at approximately $78,698,272.03. The sale was disclosed in a legal filing with the Securities & Exchange Commission, which can be accessed through this link. Also, Director Dwight A. Merriman sold 6,000 shares of MongoDB stock in a transaction that occurred on Friday, May 3rd. The shares were sold at an average price of $374.95, for a total transaction of $2,249,700.00. Following the completion of the sale, the director now owns 1,148,784 shares of the company’s stock, valued at approximately $430,736,560.80. The disclosure for this sale can be found here. Insiders sold 46,802 shares of company stock worth $16,514,071 over the last three months. 3.60% of the stock is currently owned by corporate insiders.
Institutional Investors Weigh In On MongoDB
A number of institutional investors and hedge funds have recently bought and sold shares of the stock. Raymond James & Associates grew its position in MongoDB by 14.2% during the fourth quarter. Raymond James & Associates now owns 60,557 shares of the company’s stock worth $24,759,000 after buying an additional 7,510 shares during the period. Nordea Investment Management AB increased its position in MongoDB by 298.2% during the fourth quarter. Nordea Investment Management AB now owns 18,657 shares of the company’s stock worth $7,735,000 after purchasing an additional 13,972 shares during the last quarter. Assenagon Asset Management S.A. increased its position in shares of MongoDB by 1,196.1% in the fourth quarter. Assenagon Asset Management S.A. now owns 29,215 shares of the company’s stock worth $11,945,000 after acquiring an additional 26,961 shares in the last quarter. Realta Investment Advisors acquired a new stake in shares of MongoDB in the fourth quarter worth $212,000. Finally, Blueshift Asset Management LLC acquired a new stake in MongoDB during the 3rd quarter valued at $902,000. 89.29% of the stock is owned by hedge funds and other institutional investors.
MongoDB Company Profile
MongoDB, Inc, together with its subsidiaries, provides general purpose database platform worldwide. The company provides MongoDB Atlas, a hosted multi-cloud database-as-a-service solution; MongoDB Enterprise Advanced, a commercial database server for enterprise customers to run in the cloud, on-premises, or in a hybrid environment; and Community Server, a free-to-download version of its database, which includes the functionality that developers need to get started with MongoDB.
Read More
Receive News & Ratings for MongoDB Daily – Enter your email address below to receive a concise daily summary of the latest news and analysts’ ratings for MongoDB and related companies with MarketBeat.com’s FREE daily email newsletter.
Article originally posted on mongodb google news. Visit mongodb google news
Podcast: Edo Liberty on Vector Databases for Successful Adoption of Generative AI and LLM based Applications
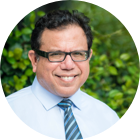
MMS • Edo Liberty
Article originally posted on InfoQ. Visit InfoQ
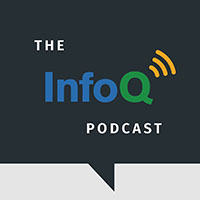
Subscribe on:
Transcript
Srini Penchikala: Hi everyone. My name is Srini Penchikala. I am the Lead Editor for AI/ML and Data Engineering community at the InfoQ website, and I’m also a podcast host.
In this episode, I will be speaking with Edo Liberty, founder and the CEO of Pinecone, the company behind the vector database product. We will be discussing the topic of vector databases, which has gotten a lot of attention recently, and also the critical role these types of databases play in the generative AI or GenAI space.
Introductions [01:24]
Hi Edo. Thank you for joining me today. First, can you introduce yourself and tell our listeners about your career and what areas have you been focusing on recently?
Edo Liberty: Hi Srini. Hello all InfoQ listeners. So my career has been a mix of science and engineering for pretty much the entire time. I did my undergraduate in physics and computer science, my PhD and postdoc in computer science, and then. So my PhD in computer science, my postdoc in applied math, focusing on, back then it was called big data, algorithms, theory of machine learning, theoretical computer science and so on.
Interestingly, I ended up with my PhD thesis and so my postdoc work on something called dimensional reduction, which has to deal with very large data sets that are represented as vectors in high-dimensional spaces, which will become very useful later in my career.
Then I started my first company, moved to Yahoo to be a scientist and an adjunct professor at Tel Aviv University. I stayed there for a long time as director, eventually leading the research out of the New York team, focusing on ML infrastructure for Yahoo.
In 2016 moved to AWS to build AI services and platforms, including a well-known platform called SageMaker, but really plugging in many different pieces of infrastructure from AWS and learning how to build managed services and managed platforms in the cloud. And in 2019 opened Pinecone to build what is now widely known as a vector database, but back then nobody really understood what it is and what it’s supposed to do, and frankly it took almost two years for people to even know what the hell I’m talking about. But we’ve been added ever since, trying to make AI more knowledgeable and less elucidatory and more useful.
Srini Penchikala: Yes, definitely. Vector databases is one of the, I don’t want to call it byproducts, but a nice development out of the LLM adoption. I know these databases have been around, but since the ChatGPT and the large language models have been getting a lot of attention, vector databases are also getting a lot of attention.
What are Vector Databases [03:51]
For our listeners who are new to vector database concept, can you please define what a vector database is and how is it different from traditional databases?
Edo Liberty: They’re very different. I mean, in fact, one of the most exciting things for me with creating Pinecone was the fact that it is such a completely new kind of infrastructure that people use. It’s a database but used predominantly like a search engine. It deals with these types of data that are called vectors, what’s called vector embeddings. They are the output of machine learning models and the way that generative AI models or foundational models, language models represent anything really, whether it’s text or images or what have you.
And those internal representations tend to be very semantically rich are much more actionable in some sense than the raw object that you started with. And if you’re doing something that looks like semantic search or RAG or other use cases we can talk about later, then you really have to retrieve the right context for you on the lens. You really need to bring in relevant information at the right time and the objects that you work with are complex. They’re not rows in a table, right? They’re not rows in a database. They’re all these PDFs or images or JIRA tickets or what have you.
And so a vector database is really highly specialized to work with vectors with those very specialized queries that search and find things by relevance, by similarity, by alignment in this numerical representation. And maybe it’s too early to dive deep at this point, but this seemingly simple change of, oh, I’ll just change the data type and the kind of how I query ends up having far-reaching implications to what architectures, what cloud architectures actually makes sense, how you reduce cost, how you increase efficiency, and it ends up that you really need a completely new kind of database to do this well. Very exciting.
Srini Penchikala: Thank you. Yes. Can you talk about some background of vector databases? Like you mentioned the concept probably is older than LLMs, but how have they evolved in the last three to four or five years?
Edo Liberty: Yes. The idea of vector search is actually not new. Okay. I’ve been working on some of these algorithms even in my PhD, which was, I’m dating myself, but that’s like 20 years ago now. These algorithms have been around, many of them. They present really interesting mathematical challenges, engineering challenges and so on, and they’ve been used internally at big companies for many years at Facebook for fin ranking, at Google for like adserving, at Amazon for shopping recommendation, and in many other places. Again, I’m just listing a few.
Because those hyperscalers already had capacity to train what’s called these embeddings and were able to really benefit from those solutions, with AI and foundational models becoming commonplace enough that most engineers need to deal with it, that need to ingest, manage, and search over a very large number of these embeddings became commonplace. And at the same time, the demands from those systems became a lot higher.
First, they needed to be a lot easier to use. Your consumer is now somebody developing an application and not a systems engineer at Google. Second, they needed to be a lot cheaper and a lot more cost-effective because you’re not running Google Ads, which is maybe the biggest cash cow in tech history. You might be running something that’s a lot less lucrative or frankly that you don’t know the ROI on yet, and you’re just trying to make sure that you build responsibly.
And the third is that just the scale and the performance requirements have gotten a lot more demanding. When we started Pinecone, having a few million or 10 million vectors in an index was considered fairly large. That was a large index. Today we have customers with tens of billions of embeddings in one index, right? Sometimes broken to namespaces, sometimes not. Latency requirements became stricter throughput, SLAs on variance and latency. So even if you’re P90, P90 is good, the people really care about P99s and so on.
So with the more use cases we see, the more demands are put on the system to be extremely performant at many different operating points, which when we built those systems internally in big companies, you were really building for one type of application so you really didn’t have to care about other operating points. Those are the main three differences in how these platforms have had to evolve. And frankly, we had the privilege to be grappling with a lot of those engineering and then science issues for almost five years now, and just see them maybe a year or two before the rest of the market all the time and start working on them before people understand it’s actually a problem.
Use Cases [09:34]
Srini Penchikala: You mentioned a few use cases earlier. So are there any other specific use cases that are good candidates for a vector DB? What are some popular use cases or applications you see using the vector database solutions?
Edo Liberty: Vector databases really excel at retrieving complex objects. It usually takes, to be honest, but also images and others based on similarity and meaning. And as a result, they really excel at partnering in some sense, like being paired up with large language models in what’s something called RAG, retrieval augmented generation. That’s been a very, very strong pattern because it’s a very successful pairing. People create embeddings and push data into a vector database, use that basically as the search engine to create the context for the LLM, and that ends up being, even if you do something fairly basic, it already outperforms most systems out there and then if you spend a week or two more playing with it and improving it here and there, you end up getting really impressive results.
But people are doing all sorts of really amazing things with vector databases now and in general, this idea of semantics or similarity search at scale. People do drug design and chemical compound search and people do security and abuse prevention online. People do cyber prevention and people do support chats for call centers and you name it because use cases are really limitless. And of course all the original use cases we started thinking about when we created the company, including recommendation engines and others are still there as well.
Vector Database Terminology [11:24]
Srini Penchikala: Sounds good. Before we get into the technical details of the vector databases, I would like to ask a question about the terminology. There is the vector embedding and there is the vector index and of course the vector database. So can you talk about what these terms are and then how they are used in these databases?
Edo Liberty: Yes. So all three are parts of what it means to run this, for example, RAG introduction. The vector embedding is just a numerical representation of an item in your system. Say it’s like a document or a part of a text document. You would push that the way an embedding model. This is a foundational model that takes this input and outputs a numerical presentation, so let’s say a thousand-dimensional float array, like a thousand long float array. That array is the embedding.
By the way, the word embedding is just a mathematical term of flow. Taking an item from one space and lodging it into a different space flow, some different properties are preserved. That’s how the word embedding came to be. Got nothing to do with embedded hardware, stuff like that, or embedded software and that people sometimes think. It’s not. It’s a mathematical term. So that is the embedding. The embedding is just measuring that set of numbers. Okay?
A vector index is a piece of code, it’s an algorithm, it’s a data structure that takes a set of vectors of this embedding, say it’s 1,000 or 10,000 or 100,000 or a million, and recomputes all sorts of statistics, data structures, pointers, organizations like clustering and so on, organizes them such that given a query, you can pinpoint the most similar or otherwise best matching in that set. This really is an almost always in-memory algorithms focused, high-performance computing type of effort. There are many competitions on running those indexes faster and so on.
We ourselves sponsored and ran one of those called BigANN. We ran it with Facebook and Microsoft and others and AWS. So this is not our competition. It’s an open thing. People can contribute to it. There’s a running leading board and that is the vector index.
A vector database is a much more complicated object. First of all, it’s at least Pinecone, and I think most systems today are cloud-native systems that separate write paths and read paths and procuring hardware and so on, but also really have to do with how you organize very large indexes in disk or on blob storage efficiently, how you access them efficiently, how you distribute loads. And be able to do way more complicated kinds of questions. This is not just vector search finding the most similar thing, but also filter net data. So I want to find the most recommended item in my shop, but I want it to be in stock or I want the most similar document, but I want it to have been written in the last month and so on. So hard filters.
You need to do what’s called boosting or it’s called sparse boosting or sparse search, which is basically something that looks like keyword search. It’s much more general, but you can bake in something like keyword search into the same kind of index and so on, allow for fresh updates, allow for deletes, really build a whole distributed system around it. So that is a vector that can, all three of course are objects that we deal with on a regular basis.
Vector Algorithms [15:23]
Srini Penchikala: So you mentioned about some algorithms to facilitate these vector database management, right? So can you talk about more of these algorithms, which use cases use which type of algorithms?
Edo Liberty: Yes. So first I’ll just say this is one of my favorite topics to speak about. I just gave a whole semester course at Princeton on exactly this topic, focusing specifically on the algorithms in the core of vector database system. So I’ll just say that this is potentially a very large topic.
I’ll say that there are two kinds of optimizations that are somewhat orthogonal or whatever like this, somewhat distinct. First, it’s how you organize the data and what fraction of data you need to look at when you get a query. In Pinecone, we do this in blob storage, so we can do this incredibly efficiently. But you can organize your data in these small clumps called clusters that at query time you can intelligently figure out, oh, out of my maybe 10,000 files, I can only look at 1,000 and see, get the right answers, almost always, and if not, get a good approximation for them.
And how you do that is a whole science. There’s randomized algorithms to do that, for doing this with random projections and random hashing and what’s called making semantic hashing. There are clustering algorithms and so on. We internally also devised more complex versions of those that lend themselves well, not only high quality, but also for very efficient query routing.
The second type of optimization is now that you’ve reduced the number of vectors that you look at, how do you now compute very efficiently the top matches? How do you now scan them in the most efficient way? And that goes back to the vector indexing. Again, there are very, very interesting trade-offs with how much memory you compute, how much disk you compute, and you can see how much are you willing to spend in latency versus memory consumption versus what kind of instruction sets that you have on your machine and so on.
But once you scan in basically or computing the best results within, get one of those shards, and again, a whole world of innovation with what’s called quantization and compression and dimension reduction and very deep accelerations of compute with the vectorized instruction cells like CND instructions and so on. And again, there are whole competitions dedicated to running this more efficiently. So there’s an infinite amount of literature in order to go dive into if one is really into that kind of stuff.
Retrieval Augmented Generation (RAG) [18:10]
Srini Penchikala: Thank you. You also mentioned about the RAG, Retrieval Augmented Generation. Definitely I see this is being one of the main developments this year. I know a lot of people are using ChatGPT and Copilot, but I think the real value of these large language models is where the companies can train the models using their own data, their own proprietary data and ask questions about their own business domain related requirements.
So how do you see vector databases kind of helping with this RAG based applications development?
Edo Liberty: So RAG is one of the most common use cases of vector database nowadays. We see that people are able to get better context for the LLMs and get better results without retraining or fine-tuning their models. They’re able to securely and in a data governed way, which they couldn’t do before. And that became a very common use case for us. And so I agree with you a million percent that these general pre-trained LLMs are great at what they do, but for me as a business, if they can’t interact with my data somehow, then they become somewhat useless, or at least I can’t apply them to most of the problems that I care about. I shouldn’t say useless.
Security [19:39]
Srini Penchikala: Okay. We can switch gears a little bit Edo and then talk about security. So how do you see with the AI and all this power, so we definitely need to balance the security concerns. So how do you see if somebody is using vector databases? How can they make sure that their solutions are still secure and there are no other concerns?
Edo Liberty: I think we should separate two different kinds of securing. One of them has to do with cyber, and then one of them has to do with data governance. I want to address both.
First in terms of not shipping data where you shouldn’t ship data and so on, it’s critical. I mean, this is why we invest so much in security features and being HIPAA-compliant and SOX-compliant and so on. Every other managed service has to put a significant amount of effort and make sure that this is one of the core operating tenets like with any data infrastructure by the way. Any infrastructure that touches your data needs to be, you have to have a high level of confidence it does the right thing. You have to have a high level of trust in the vendor and their ability to actually deliver.
There is a data governance issue, which I think people have to think about very seriously. When you fine tune a model with data, you can’t later “delete” that data from the model. And so now you upfront commit to a future of very complex operation that if you want to be, say, GDPR-compliant, you really can do it. The only solution is keep retraining your models, keep fine-tuning them, so on, based on whatever it is you’re allowed to do and so on. And we see that with basically putting the data adjacent to the foundation model.
In a vector database, you can now decide dynamically what information is available to the model. You can keep it fresh, and then if a data was added literally five seconds ago, it is now available to your system. If you had to delete some portion of the data because of GDPR compliance, five seconds later that data is gone and your model never knows about it anymore and so on. So we find that to be very, very convenient, one of the main reasons why people choose this paradigm versus fine-tuning or retraining.
Serverless Data [22:12]
Srini Penchikala: One of the new features in the vector databases is the serverless architecture where the developers don’t need to worry about managing or provisioning the infrastructure. Can you talk about this and how these serverless architectures are enabling the vector databases even more and maybe give some examples of how this contributes to faster market entry for Gen-AI applications?
Edo Liberty: Yes. So first of all, different people call serverless different things, and so we want to make sure that we understand what serverless data is to begin with. What I mean by serverless and what we ship is a complete disassociation between your workload and the hardware that it is used to run it. It’s our job to figure out hardware to run where to answer your queries well, and in some sense we want to make all of that completely none of your problem. You want to know what data you have and what queries you run, and that’s it. And if you have some amount of data today and tomorrow that amount of data doubles or cuts in half, that’s great. You don’t need to worry about rescaling, resharding, moving data around, any of that stuff. If you cut the amount of data in half, you pay half as much and that’s it. You don’t need to worry about anything else.
And so that’s solved two main problems for us and for our customers at the same time. One is planning. We have a lot of users who are customers who build very aggressive and very ambitious projects, and they hope that the projects are going to be adopted by say 5% of the user base. But they say, do we need to provision for 20% of volumes base to adopt this thing or maybe 50%? We don’t know. And so they would go into this really complex decision-making that they just shouldn’t be worried about. They should just use as much as they need, and our system’s job is to scale to meet that demand.
By the way, we’ve had issues in the past where somebody tried to, and I’m not going to name the customer, but they thought that if 10% of the user base would adopt the feature, that would be a stellar success. Within a week, 90% of the use case will be the user base adopted because it was so novel that was so exciting. But also those went through the roof and suddenly they had scaling issues and so on. So we really had to go in and help them fix that.
The second thing is just overall cost reduction. When you procure hardware, you are buying a certain mix of memory, disk, network and so on. You just buy a pre-packaged ratio between different kinds of resources on your machine. And if your workload doesn’t fit exactly that, that machine is severely underutilized. And so anything that operates on a node basis, on a machine basis is inherently in various amount. What we were able to do with serverless is reduce the total application cost for some of our users by 50x or many, many of our users by 5 to 10x. That’s a massive savings. We were able to do that just because of that move.
Our ability to manage hardware a lot more automatically in a lot more sophisticated ways allows us to actually ship something that is way more cost-effective, and frankly, based on our analysis, that’s the only way to get the win.
Responsible AI [26:07]
Srini Penchikala: I want to talk about the social side of these amazing technologies. So I heard this somewhere, and I kind of like this statement. Responsible AI starts with responsible data. Can you talk about what the application developers should consider to create responsible solutions when they use vector databases?
Edo Liberty: I don’t think this has to do with vector databases at all. I think you’re right. I think this is the tension that AI and machine learning developers, engineers in general actually face all the time, balance between how fast you want to move and ship and how much you want to hold back and be responsible and be thoughtful and be careful.
And today, with incredibly fast moving market in this field, I think that tension has never been higher and companies are under a tremendous amount of pressure to ship something, and they’re oftentimes very concerned that what they’re going to put out there is going to backfire in some spectacular way. And it could be in some sense the least of their problem is if it’s just a marketing debacle. Sometimes they produce actual harm and that’s dangerous.
So I would say that being thoughtful and careful with what data you use for what, what guardrails you put in place, in general and specifically with AI is incredibly important.
That said, people still ship amazing things and companies should not be paralyzed with fear that something will potentially go wrong. And what I’ve seen happen is that people take on the slightly less risky parts of the stack or the applications that require slightly less access to very, very governed data or touch some really high stakes decision-making and go and ship those products first. And as a result, not only are they able to progress and get some value out of it, they actually get to learn and build talent in-house and build know-how, and then they’re able to get a lot more confidence that they can go and execute on the more complex or the most somehow potentially risky use cases.
Srini Penchikala: I heard this prediction at a recent Microsoft conference. So what they said was in no more than three years, anything that is not connected to AI will be considered broken or invisible. So that kind of tells the presence of AI in our daily lives. So how do you see AI and maybe even vector databases as part of the main part of this overall evolution playing a larger role in our work and daily lives in the future?
Edo Liberty: Yes, I mean, I agree with that statement and I think it’s much more sort of a mundane and practical statement than people make it to be. It sounds like some grandiose like prediction about general intelligence. I view this as something a lot more practical and obvious.
I have young kids. I have a nine-year-old and I have six-year-old twins, and they touch every screen and talk to every speaker. The idea that the speaker can’t hear them and talk back is weird to them, or the fact that some screen doesn’t react to being touched. That’s just the interface they grew up with. Things that don’t behave that way, are just like, whatever, old timing if you know. Whatever, it just don’t work in their opinion. For us, taking a flip phone, it’s just like, oh yes, this is a thing. It used to be a thing.
Interfacing with technology through language has become such an expected interface that people will just come to expect it. I think that’s the statement. It just became an interface that every product needs to have in some capacity. In that vein, I 100% agree, right?
What part we play in this? We play the part of the vector database, we play the part of knowledge and retrieval and managing data. We play a part of the ecosystem with models, with application, with evaluations, with monitoring, with ETLs, with ML hosting, with a few other technologies that are required to do top-grade AR platforms. And every company is going to have to invest in many different products because again, that’s an interface that people expect.
Online Resources [30:59]
Srini Penchikala: Thank you, Edo. So one last question. Do you have any recommendations on what resources our listeners can check out to learn more about vector databases, RAG or any other related technologies?
Edo Liberty: Yes. So we have put up a ton of material at Pinecone on how to do RAG, how to use LangChain, how to use open models from all of our partners, from Anthropic and Cohere and Hugging Face and OpenAI and so on. We have taken a conscious decision very early on to not evangelize our product, but rather to teach people how to build stuff. And so as a result, there’s a lot of notebooks and examples and integrations and so on.
For other sources, I find documentation and blog posts from different technology evangelists could be a lot more useful than say, GDOs or Docs. I personally love to follow examples and to learn by doing rather than go read the manual. And frankly, I mean just extrapolating from what I see, people who are successful in building with AI are people who just start doing it. And still by far the most common mode of failure is people get either so afraid or so whatever, concerned, they don’t start at all, or go into this analysis-paralysis mode where they start serving 7,000 technologies. If you’re using the wrong thing, you’ll figure it out mid-way. Just start building. And people who’ve done that have by and large been successful eventually.
Srini Penchikala: Thank you. Do you have any additional comments before we wrap up today’s discussion?
Edo Liberty: No, just to say thank you again, it was lovely and I’m glad I get to tell your listeners that building with AI it’s not as hard as it potentially used to be, and if they get started, they can start getting something done within probably a day or two and put everything aside. It’s fun. It’s really exciting new technology, and when things start to work, it’s like an aha moment. It’s like, “Holy shit. I just can do that. I can build a lot of things with this thing.” So highly recommend it and I’m glad I could encourage people to start whatever it is they already planned to do.
Srini Penchikala: Yes, AI and GenAI definitely bring different perspectives, different dimensions to problem solving and the multiple dimensions to solve a problem which humans cannot do as fast as machines, right? So thank you Edo, thank you very much for joining this podcast. It’s been great to discuss one of the very important topics in the AI space, the vector databases, which I see as the foundation of the GenAI and LLM evolution.
And to our InfoQ listeners, thank you for listening to this podcast. If you would like to learn more about the AI/ML topics, please check out the AI/ML and Data Engineering community on infoq.com website. I also encourage you to listen to the other podcasts and also check out the articles and news items on the website. Thank you.
Mentioned:
- SageMaker
- Pinecone
- vector embeddings
- Retrieval Augmented Generation (RAG)
- LangChain
- Anthropic
- Cohere
- Hugging Face
.
From this page you also have access to our recorded show notes. They all have clickable links that will take you directly to that part of the audio.
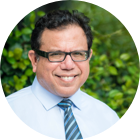
MMS • RSS
Posted on mongodb google news. Visit mongodb google news
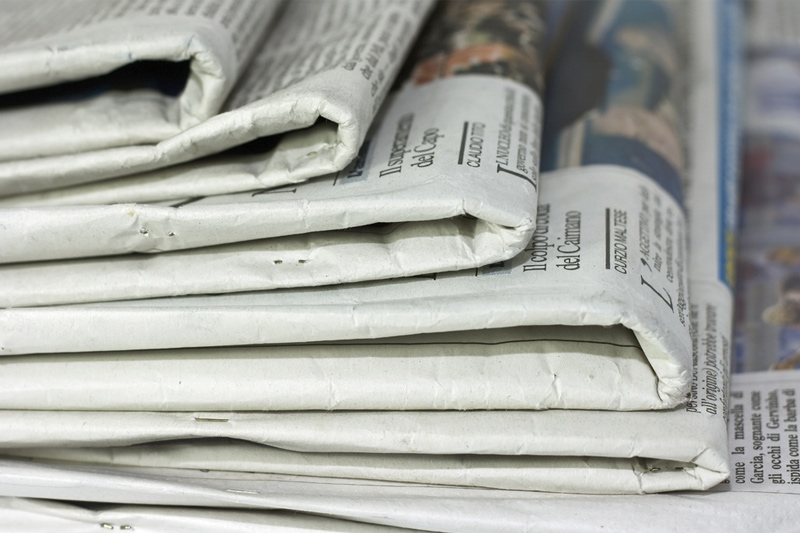
MongoDB’s shares experienced a sharp drop, closing down 24% after the release of its first-quarter fiscal year 2025 earnings on May 30th, underperforming against the S&P 500’s 1% gain and the iShares Expanded Tech-Software Sector ETF’s (IGV) similar increase.
The downgrade in MongoDB’s stock occurred amidst a 54% fall from its 52-week high, a contrast to the S&P 500’s 1% dip and IGV’s 12% decrease.
The current discussions surrounding the company focus on its ability to achieve a 20% growth target for the year, which contrasts with its recently reduced guidance of 12% at the midpoint.
Despite the lower guidance and management’s cautious commentary on macroeconomic conditions, the analyst suggested that MongoDB’s situation might not be as dire as it appears.
MongoDB was the only consumption-based vendor to report a deteriorating macroeconomic environment this quarter, which was at odds with peers’ indications of stabilizing or improving trends.
The analyst pointed to the deceleration in Atlas (NYSE:ATCO) New Annual Recurring Revenue (ARR) growth in the first quarter of fiscal 2025 as a potential leading indicator, but noted that it was not as significant as the lowered guidance might suggest.
The downgrade in guidance and the company’s performance could be attributed to temporary go-to-market (GTM) headwinds that MongoDB is facing, rather than broader macroeconomic issues.
In light of Guggenheim’s recent upgrade of MongoDB, Inc. (NASDAQ:MDB) from Sell to Neutral, it’s valuable to consider additional insights from InvestingPro. MongoDB holds more cash than debt on its balance sheet, which can provide a buffer in volatile market conditions. Moreover, the company’s net income is expected to grow this year, offering a potential upside despite the recent decline in share price.
The company’s financial health is further underscored by a robust gross profit margin of 74.78% for the last twelve months as of Q4 2024, indicating strong operational efficiency. Additionally, MongoDB’s revenue growth remains solid, with a 31.07% increase over the same period. However, the stock is currently trading near its 52-week low, which may attract investors looking for entry points in a fundamentally sound company.
For those looking to delve deeper into MongoDB’s financials and future outlook, InvestingPro offers a range of additional tips. There are 15 more InvestingPro Tips available that could provide valuable guidance for investors considering MongoDB. To explore these insights, visit: https://www.investing.com/pro/MDB and remember to use coupon code PRONEWS24 to get an additional 10% off a yearly or biyearly Pro and Pro+ subscription.
This article was generated with the support of AI and reviewed by an editor. For more information see our T&C.
Article originally posted on mongodb google news. Visit mongodb google news
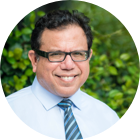
MMS • RSS
Posted on mongodb google news. Visit mongodb google news
The CE 100 Index slipped 1.1%, as AI names were – to put it mildly – volatile in the wake of earnings reports.
MongoDB Leads to the Downside
MongoDB shares sank 32.5% and led the Enablers segment 2.6% lower in the holiday shortened trading week.
The shares plummeted after the company reported earnings that detailed first-quarter revenue growth of 22% year over year to $451 million. But that growth rate marked a significant slowdown from the roughly 57% top line increase that had been seen only a few quarters ago. Net losses expanded to more than $80 million from a bit more than $54 million last year.
“We had a slower than expected start to the year for both Atlas consumption growth and new workload wins, which will have a downstream impact for the remainder of fiscal 2025,” MongoDB’s president and CEO Dev Ittycheria said in the most recent earnings release.
Salesforce shares gave up 13.9% as the Work segment lost 4.6%. The company reported earnings this past week that saw revenues increase by 11% to $9.1 billion, while subscription revenues were up a bit more than that, at 12%, to $8.6 billion. Forward-looking guidance disappointed investors, as management said that the current fiscal year’s second quarter growth will come in at between 7-8% and full year subscription and and support revenue growth will come in at roughly 10%.
DraftKings Sinks on Tax-Related News
DraftKings lost 13.9% as the Have Fun segment slipped 1.4%. As widely reported, the Illinois Senate passed legislation this past week boosting taxes on sports betting, where the tax rates could be as high as 40% from a current 15% level.
Elsewhere, and turning attention to positive performers, while demonstrating the volatility in the AI space, C3.ai soared 23.1%.
As reported this past week, the enterprise-focused AI software firm posted strong growth in its subscription business, which accounted for 92% of the consolidated top line. Total revenues gained 20% year over year to $86.6 million, while subscription revenues surged 41%.
The federal defense and aerospace sector proved to be a significant growth driver for C3.ai, accounting for approximately 50% of its bookings in the fourth quarter, as we reported. Over the past year, the company closed 65 agreements with federal agencies and made inroads with ten new federal organizations in Q4.
Looking ahead, C3.ai expects revenue growth to accelerate to approximately 23% in fiscal year 2025.
Sezzle gained 15.8%, continuing to ride a wave of momentum in the wake of its announcement of an expanded relationship with Celerant Technology. Celerant now enables the users of its eCommerce platform to offer their customers buy now, pay later (BNPL) options when checking out in-store as well as online.
The company has implemented additional integration functionalities with Sezzle to allow these options at the point of sale, in-store, Celerant said earlier in the month. The two companies had already partnered to offer BNPL options online, having launched that collaboration in April 2023.
Peloton shares gathered 15.6%, retracing at least some of the company’s recent stock slides. The company announced that it completed a “holistic refinancing that reduced overall debt, extended debt maturities and achieved more flexible loan terms.” The refinancing included a new $1 billion five-year term loan facility.
Article originally posted on mongodb google news. Visit mongodb google news