Month: August 2024
2024-08-11 | MongoDB, Inc. Sued for Securities Law Violations – MDB | Press Release – Stockhouse
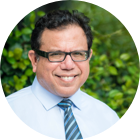
MMS • RSS
Posted on mongodb google news. Visit mongodb google news
NEW YORK, Aug. 11, 2024 /PRNewswire/ —
Why: Rosen Law Firm, a global investor rights law firm, reminds purchasers of securities of MongoDB, Inc. (NASDAQ: MDB) between August 31, 2023 and May 30, 2024, both dates inclusive (the “Class Period”), of the important September 9, 2024 lead plaintiff deadline.
So What: If you purchased MongoDB securities during the Class Period you may be entitled to compensation without payment of any out of pocket fees or costs through a contingency fee arrangement.
What to do Next: To join the MongoDB class action, go to https://rosenlegal.com/submit-form/?case_id=27182 or call Phillip Kim, Esq. toll-free at 866-767-3653 or email case@rosenlegal.com for information on the class action. A class action lawsuit has already been filed. If you wish to serve as lead plaintiff, you must move the Court no later than September 9, 2024. A lead plaintiff is a representative party acting on behalf of other class members in directing the litigation.
Details of the case: According to the lawsuit, throughout the Class Period, defendants created the false impression that they possessed reliable information pertaining to the Company’s projected revenue outlook and anticipated growth while also minimizing risk from seasonality and macroeconomic fluctuations. In truth, MongoDB’s sales force restructure, which prioritized reducing friction in the enrollment process, had resulted in complete loss of upfront commitments; a significant reduction in the information gathered by their sales force as to the trajectory for the new MongoDB Atlas enrollments; and reduced pressure on new enrollments to grow. Defendants misled investors by providing the public with materially flawed statements of confidence and growth projections which did not account for these variables. When the true details entered the market, the lawsuit claims that investors suffered damages.
To join the MongoDB class action, go to https://rosenlegal.com/submit-form/?case_id=27182 or call Phillip Kim, Esq. toll-free at 866-767-3653 or email case@rosenlegal.com for information on the class action.
No Class Has Been Certified. Until a class is certified, you are not represented by counsel unless you retain one. You may select counsel of your choice. You may also remain an absent class member and do nothing at this point. An investor’s ability to share in any potential future recovery is not dependent upon serving as lead plaintiff.
Follow us for updates on LinkedIn: https://www.linkedin.com/company/the-rosen-law-firm, on Twitter: https://twitter.com/rosen_firm or on Facebook: https://www.facebook.com/rosenlawfirm/.
Attorney Advertising. Prior results do not guarantee a similar outcome.
Contact Information:
Laurence Rosen, Esq.
Phillip Kim, Esq.
The Rosen Law Firm, P.A.
275 Madison Avenue, 40th Floor
New York, NY 10016
Tel: (212) 686-1060
Toll Free: (866) 767-3653
Fax: (212) 202-3827
case@rosenlegal.com
www.rosenlegal.com
View original content to download multimedia:https://www.prnewswire.com/news-releases/mongodb-inc-sued-for-securities-law-violations–contact-the-rosen-law-firm-before-september-9-2024-to-discuss-your-rights—mdb-302219284.html
SOURCE THE ROSEN LAW FIRM, P. A.
Article originally posted on mongodb google news. Visit mongodb google news
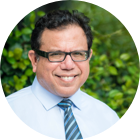
MMS • RSS
Posted on mongodb google news. Visit mongodb google news
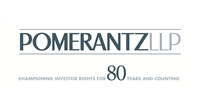
NEW YORK, NY / ACCESSWIRE / August 11, 2024 / Pomerantz LLP announces that a class action lawsuit has been filed against MongoDB, Inc. (“MongoDB” or the “Company”) (NASDAQ:MDB). Such investors are advised to contact Danielle Peyton at [email protected] or 646-581-9980, (or 888.4-POMLAW), toll-free, Ext. 7980. Those who inquire by e-mail are encouraged to include their mailing address, telephone number, and the number of shares purchased.
The class action concerns whether MongoDB and certain of its officers and/or directors have engaged in securities fraud or other unlawful business practices.
You have until September 9, 2024, to ask the Court to appoint you as Lead Plaintiff for the class if you are a shareholder who purchased or otherwise acquired MongoDB securities during the Class Period. A copy of the Complaint can be obtained at www.pomerantzlaw.com.
[Click here for information about joining the class action]
On March 7, 2024, MongoDB issued a press release announcing its fiscal year 2024 earnings and held a related earnings call that same day. Among other items, MongoDB announced an anticipated near-zero revenue from unused commitments for the Company’s MongoDB Atlas platform in fiscal year 2025, a decrease of approximately $40 million in revenue, attributed to the Company’s decision to change their sales incentive structure to reduce enrollment. Additionally, MongoDB projected only 14% growth for fiscal year 2025, compared to projected 16% growth for the previous year, which had resulted in an actualized 31% growth.
On this news, MongoDB’s stock price fell $28.59 per share, or 6.94%, to close at $383.42 per share on March 8, 2024.
Then, on May 30, 2024, MongoDB again announced significantly reduced growth projections for fiscal year 2025. The Company attributed the reduction to its decision to change its sales incentive structure to reduce enrollment frictions, as well as purportedly unanticipated macroeconomic headwinds.
On this news, Mongo’s stock price fell $73.94 per share, or 23.85%, to close at $236.06 per share on May 31, 2024.
Pomerantz LLP, with offices in New York, Chicago, Los Angeles, London, Paris, and Tel Aviv, is acknowledged as one of the premier firms in the areas of corporate, securities, and antitrust class litigation. Founded by the late Abraham L. Pomerantz, known as the dean of the class action bar, Pomerantz pioneered the field of securities class actions. Today, more than 85 years later, Pomerantz continues in the tradition he established, fighting for the rights of the victims of securities fraud, breaches of fiduciary duty, and corporate misconduct. The Firm has recovered billions of dollars in damages awards on behalf of class members. See www.pomlaw.com.
Attorney advertising. Prior results do not guarantee similar outcomes.
SOURCE: Pomerantz LLP
Article originally posted on mongodb google news. Visit mongodb google news
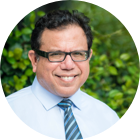
MMS • RSS
Posted on mongodb google news. Visit mongodb google news
New York, New York–(Newsfile Corp. – August 10, 2024) – WHY: Rosen Law Firm, a global investor rights law firm, reminds purchasers of securities of MongoDB, Inc. (NASDAQ: MDB) between August 31, 2023 and May 30, 2024, both dates inclusive (the “Class Period”), of the important September 9, 2024 lead plaintiff deadline.
SO WHAT: If you purchased MongoDB securities during the Class Period you may be entitled to compensation without payment of any out of pocket fees or costs through a contingency fee arrangement.
WHAT TO DO NEXT: To join the MongoDB class action, go to https://rosenlegal.com/submit-form/?case_id=27182 or call Phillip Kim, Esq. toll-free at 866-767-3653 or email case@rosenlegal.com for information on the class action. A class action lawsuit has already been filed. If you wish to serve as lead plaintiff, you must move the Court no later than September 9, 2024. A lead plaintiff is a representative party acting on behalf of other class members in directing the litigation.
WHY ROSEN LAW: We encourage investors to select qualified counsel with a track record of success in leadership roles. Often, firms issuing notices do not have comparable experience, resources or any meaningful peer recognition. Many of these firms do not actually litigate securities class actions, but are merely middlemen that refer clients or partner with law firms that actually litigate the cases. Be wise in selecting counsel. The Rosen Law Firm represents investors throughout the globe, concentrating its practice in securities class actions and shareholder derivative litigation. Rosen Law Firm has achieved the largest ever securities class action settlement against a Chinese Company. Rosen Law Firm was Ranked No. 1 by ISS Securities Class Action Services for number of securities class action settlements in 2017. The firm has been ranked in the top 4 each year since 2013 and has recovered hundreds of millions of dollars for investors. In 2019 alone the firm secured over $438 million for investors. In 2020, founding partner Laurence Rosen was named by law360 as a Titan of Plaintiffs’ Bar. Many of the firm’s attorneys have been recognized by Lawdragon and Super Lawyers.
DETAILS OF THE CASE: According to the lawsuit, throughout the Class Period, defendants created the false impression that they possessed reliable information pertaining to the Company’s projected revenue outlook and anticipated growth while also minimizing risk from seasonality and macroeconomic fluctuations. In truth, MongoDB’s sales force restructure, which prioritized reducing friction in the enrollment process, had resulted in complete loss of upfront commitments; a significant reduction in the information gathered by their sales force as to the trajectory for the new MongoDB Atlas enrollments; and reduced pressure on new enrollments to grow. Defendants misled investors by providing the public with materially flawed statements of confidence and growth projections which did not account for these variables. When the true details entered the market, the lawsuit claims that investors suffered damages.
To join the MongoDB class action, go to https://rosenlegal.com/submit-form/?case_id=27182 or call Phillip Kim, Esq. toll-free at 866-767-3653 or email case@rosenlegal.com for information on the class action.
No Class Has Been Certified. Until a class is certified, you are not represented by counsel unless you retain one. You may select counsel of your choice. You may also remain an absent class member and do nothing at this point. An investor’s ability to share in any potential future recovery is not dependent upon serving as lead plaintiff.
Follow us for updates on LinkedIn: https://www.linkedin.com/company/the-rosen-law-firm, on Twitter: https://twitter.com/rosen_firm or on Facebook: https://www.facebook.com/rosenlawfirm/.
Attorney Advertising. Prior results do not guarantee a similar outcome.
——————————-
To view the source version of this press release, please visit https://www.newsfilecorp.com/release/219467
Article originally posted on mongodb google news. Visit mongodb google news
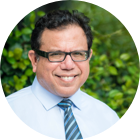
MMS • Daniel Dominguez
Article originally posted on InfoQ. Visit InfoQ
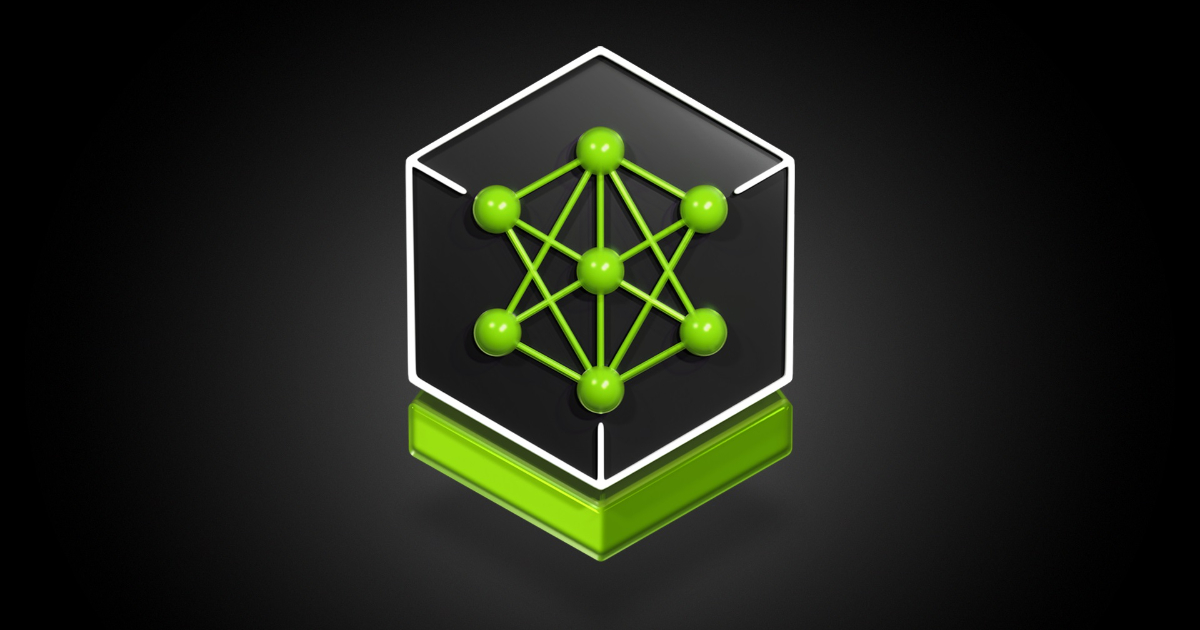
Hugging Face has announced the launch of an inference-as-a-service capability powered by NVIDIA NIM. This new service will provide developers easy access to NVIDIA-accelerated inference for popular AI models.
The new service allows developers to rapidly deploy leading large language models such as the Llama 3 family and Mistral AI models with optimization from NVIDIA NIM microservices running on NVIDIA DGX Cloud. This will help developers quickly prototype with open-source AI models hosted on the Hugging Face Hub and deploy them in production.
The Hugging Face inference-as-a-service on NVIDIA DGX Cloud powered by NIM microservices offers easy access to compute resources that are optimized for AI deployment. The NVIDIA DGX Cloud platform is purpose-built for generative AI and provides scalable GPU resources that support every step of AI development, from prototype to production.
To use the service, users must have access to an Enterprise Hub organization and a fine-grained token for authentication. The NVIDIA NIM Endpoints for supported Generative AI models can be found on the model page of the Hugging Face Hub.
Currently, the service only supports the chat.completions.create
and models.list
APIs, but Hugging Face is working on extending this while adding more models. Usage of Hugging Face Inference-as-a-Service on DGX Cloud is billed based on the compute time spent per request, using NVIDIA H100 Tensor Core GPUs.
Hugging Face is also working with NVIDIA to integrate the NVIDIA TensorRT-LLM library into Hugging Face’s Text Generation Inference (TGI) framework to improve AI inference performance and accessibility. In addition to the new Inference-as-a-Service, Hugging Face also offers Train on DGX Cloud, an AI training service.
Clem Delangue, CEO at Hugging Face, posted on his X account:
Very excited to see that Hugging Face is becoming the gateway for AI compute!
And Kaggle Master Rohan Paul shared a post on X saying:
So, we can use open models with the accelerated compute platform of NVIDIA DGX Cloud for inference serving. Code is fully compatible with OpenAI API, allowing you to use the openai’s sdk for inference.
At SIGGRAPH, NVIDIA also introduced generative AI models and NIM microservices for the OpenUSD framework to accelerate developers’ abilities to build highly accurate virtual worlds for the next evolution of AI.
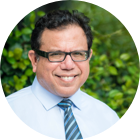
MMS • RSS
Posted on mongodb google news. Visit mongodb google news
NEW YORK, NY / ACCESSWIRE / August 11, 2024 / Leading securities law firm Bleichmar Fonti & Auld LLP announces a lawsuit has been filed against MongoDB, Inc. (Nasdaq:MDB) and certain of the Company’s senior executives.
If you suffered losses on your MongoDB investment, you are encouraged to submit your information at https://www.bfalaw.com/cases-investigations/mongodb-inc.
Investors have until September 9, 2024 to ask the Court to be appointed to lead the case. The complaint asserts claims under Sections 10(b) and 20(a) of the Securities Exchange Act of 1934 on behalf of investors in MongoDB securities between August 31, 2023 and May 30, 2024, inclusive. The case is pending in the U.S. District Court for the Southern District of New York and is captioned John Baxter v. MongoDB, Inc., et al., No. 1:24-cv-05191.
What is the Lawsuit About?
The complaint alleges that the Company misrepresented the purported benefits stemming from the restructuring of its sales force. This includes how the restructuring helped reduce friction in acquiring new customers and increased new workload acquisition among existing customers.
These statements were allegedly materially false and misleading. In truth, MongoDB’s sales force restructuring resulted in a near total loss of upfront customer commitments, a significant reduction in actionable information gathered by the sales force, and hindered enrollment and revenue growth.
On March 7, 2024, the Company allegedly announced that due to the sales restructuring, it experienced an annual decrease of approximately $40 million in multiyear license revenue, anticipated near zero revenue from unused Atlas commitments (one of its core offerings) in fiscal year 2025, and provided a disappointing revenue growth forecast that trailed that of the prior year. This news caused the price of MongoDB stock to decline $28.59 per share, or about 7%, from $412.01 per share on March 7, 2024, to $383.42 per share on March 8, 2024.
Then, on May 30, 2024, the Company again announced significantly reduced growth expectations, this time cutting fiscal year 2025 growth projections further, again attributing the losses to the sales force restructuring. On this news, the price of MongoDB stock declined $73.94 per share, or nearly 24%, from $310.00 per share on May 30, 2024, to $236.06 per share on May 31, 2024.
Click here if you suffered losses: https://www.bfalaw.com/cases-investigations/mongodb-inc.
What Can You Do?
If you invested in MongoDB, Inc. you have rights and are encouraged to submit your information to speak with an attorney.
All representation is on a contingency fee basis, there is no cost to you. Shareholders are not responsible for any court costs or expenses of litigation. The Firm will seek court approval for any potential fees and expenses. Submit your information:
https://www.bfalaw.com/cases-investigations/mongodb-inc
Or contact us at:
Ross Shikowitz
ross@bfalaw.com
212-789-3619
Why Bleichmar Fonti & Auld LLP?
Bleichmar Fonti & Auld LLP is a leading international law firm representing plaintiffs in securities class actions and shareholder litigation. It was named among the Top 5 plaintiff law firms by ISS SCAS in 2023 and its attorneys have been named Titans of the Plaintiffs’ Bar by Law360 and SuperLawyers by Thompson Reuters. Among its recent notable successes, BFA recovered over $900 million in value from Tesla, Inc.’s Board of Directors (pending court approval), as well as $420 million from Teva Pharmaceutical Ind. Ltd.
For more information about BFA and its attorneys, please visit https://www.bfalaw.com.
https://www.bfalaw.com/cases-investigations/mongodb-inc
Attorney advertising. Past results do not guarantee future outcomes.
SOURCE: Bleichmar, Fonti, & Auld LLP
View the original press release on accesswire.com
Article originally posted on mongodb google news. Visit mongodb google news
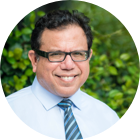
MMS • RSS
Posted on mongodb google news. Visit mongodb google news
Faruqi & Faruqi, LLP Securities Litigation Partner James (Josh) Wilson Encourages Investors Who Suffered Losses Exceeding $100,000 In MongoDB To Contact Him Directly To Discuss Their Options
If you suffered losses exceeding $100,000 investing in MongoDB stock or options between August 31, 2023 and May 30, 2024 and would like to discuss your legal rights, call Faruqi & Faruqi partner Josh Wilson directly at 877-247-4292 or 212-983-9330 (Ext. 1310). You may also click here for additional information: www.faruqilaw.com/MDB.
New York, New York–(Newsfile Corp. – August 10, 2024) – Faruqi & Faruqi, LLP, a leading national securities law firm, is investigating potential claims against MongoDB, Inc. (“MongoDB” or the “Company”) (NASDAQ: MDB) and reminds investors of the September 9, 2024 deadline to seek the role of lead plaintiff in a federal securities class action that has been filed against the Company.
Faruqi & Faruqi is a leading national securities law firm with offices in New York, Pennsylvania, California and Georgia. The firm has recovered hundreds of millions of dollars for investors since its founding in 1995. See www.faruqilaw.com.
The complaint alleges that on March 7, 2024, MongoDB reported strong Q4 2024 results and then announced lower than expected full-year guidance for 2025. MongoDB attributed it to the Company’s change in its “sales incentive structure” which led to a decrease in revenue related to “unused commitments and multi-year licensing deals.”
Following this news, MongoDB’s stock price fell by $28.59 per share to close at $383.42 per share.
Later, on May 30, 2024, MongoDB further lowered its guidance for the full year 2025 attributing it to “macro impacting consumption growth.” Analysts commenting on the reduced guidance questioned if changes made to the Company’s marketing strategy “led to change in customer behavior and usage patterns.”
Following this news, MongoDB’s stock price fell by $73.94 per share to close at $236.06 per share.
The court-appointed lead plaintiff is the investor with the largest financial interest in the relief sought by the class who is adequate and typical of class members who directs and oversees the litigation on behalf of the putative class. Any member of the putative class may move the Court to serve as lead plaintiff through counsel of their choice, or may choose to do nothing and remain an absent class member. Your ability to share in any recovery is not affected by the decision to serve as a lead plaintiff or not.
Faruqi & Faruqi, LLP also encourages anyone with information regarding MongoDB’s conduct to contact the firm, including whistleblowers, former employees, shareholders and others.
Follow us for updates on LinkedIn, on X, or on Facebook.
Attorney Advertising. The law firm responsible for this advertisement is Faruqi & Faruqi, LLP (www.faruqilaw.com). Prior results do not guarantee or predict a similar outcome with respect to any future matter. We welcome the opportunity to discuss your particular case. All communications will be treated in a confidential manner.
To view the source version of this press release, please visit https://www.newsfilecorp.com/release/219459
Article originally posted on mongodb google news. Visit mongodb google news
Podcast: Enhancing Team Dynamics and Psychological Safety in DevOps with Brittany Woods
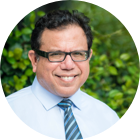
MMS • Brittany Woods
Article originally posted on InfoQ. Visit InfoQ
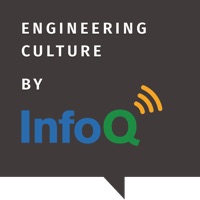
Subscribe on:
Transcript
Shane Hastie: Good day, folks. This is Shane Hastie for the InfoQ, Engineering Culture Podcast. Today, I’m sitting down with Brittany Woods. Brittany, welcome. Thanks for taking the time to talk to us today.
Brittany Woods: Thank you for having me. I’m excited to be here.
Shane Hastie: My normal starting point for these conversations is who’s Brittany?
Introductions [00:40]
Brittany Woods: By way of introduction, I’ve worked in several industries. Well, I guess I’ve been in the tech industry, but I’ve worked across several verticals within it, so retail, finance, the weight industry. So I have sort of a broad experience and understanding across what different companies go through.
I started out as an engineer in the infrastructure space. I did server builds when we were still building servers by hand. And it was from there that I got into automation, and then, automation sort of led me into cloud. And then from being a cloud engineer, I moved into the leadership space. And I’ve been a leader probably the last four and a half years, leading everything from configuration management, configuration automation, infrastructure as code to development teams, very DevOps-centric teams to not-DevOps-centric teams, you name it.
Shane Hastie: I came across you because of a talk at QCon London, Bits, Bots, and Banter: How Tech Teams Work in a DevOps World. What is different about the way teams work in a DevOps world?
What is different about the way teams work in a DevOps world? [01:56]
Brittany Woods: I think the biggest thing is that the way teams work was obviously changing for years, and teams were adopting more agile mindsets, et cetera. But then, as teams made this shift to DevOps model, they were being asked really to do a lot more than they had done previously. And some companies understood the assignment. Some companies looked at that and said, “You know, we have to enable these teams in a different way than we historically did. We can’t just say we want you to do things you didn’t do before.” But other companies maybe didn’t see that part. So the way that they adopted DevOps was different.
And DevOps itself is not really a vague methodology, but it’s so broad that companies can adopt it in the way that makes sense for them. And because of that, there’s this growing number of organizations that have gotten multiple years on their DevOps journey that are taking a step back and looking and saying, “I have teams that I was expecting to be able to deliver faster and do all of these things because that’s what DevOps promised me. Why am I not really seeing this golden path,” so to speak, “that was supposed to make itself present?”
And so this talk that I gave at QCon was really about what I’ve seen work in enabling teams, whether that’s development teams that you’re now asking to do different things, or whether that’s the historical operations teams that handled a lot of the stuff developers are now being asked to do, and how their role changes. So it was about giving insights to that, giving insights to team structure, how you can build psychological safety within these new structured teams, and so on.
Shane Hastie: So let’s dig into psychological safety. It’s almost a buzzword today. You can’t open a culture book, a management book without the importance of psychological safety. But what does it really mean?
What psychological safety means in practice [03:56]
Brittany Woods: I think for me, at least in the positions that I’ve been in and from the level that I’m at, realistically, psychological safety means that your engineers feel safe to be able to experiment. They feel safe to be able to do the things that you’re asking of them without repercussion from either you or the business. And that experimentation is key to being able to deliver innovation and to deliver solutions and things on the edge.
So from my level, it’s very tactical psychological safety. I think broadly, though, it’s really important, and it’s become such a buzzword because, particularly in DevOps, when you’re asking teams to do things they didn’t historically do, you’re asking them to step out of their comfort zone. And realistically, you’re asking them to trust. So without trust and without that psychological safety, your teams aren’t going to be successful.
So I guess, on the broader level, that’s probably what I would say it means to me. And if I were speculating, that’s why I feel like it’s become so important and such a buzzword because a lot of companies, again, went down this DevOps path with a clear instruction book, but it was just clear enough. And so they’re getting to this place where they’re like, the landscape is changing, the engineers are changing. How can I enable teams to move forward?
Shane Hastie: So how can we?
Walking the talk around trust and safety [05:24]
Brittany Woods: I mentioned trust is really key to all of that. So as a leader, for me, it’s about walking the walk and talking the talk. It’s about when I say that I want to build trust with my team, actually building that trust, actually coming to bat for them, I guess, like if there is an issue. We all get sort of bogged down when there’s an issue, like making sure to cover for ourselves or cover for our team, it’s about more than that. It’s about showing the team. When things get hairy because of an experiment that failed or something, I’m willing to come forward and say, “Hey, we were learning. We were doing that thing. This is what we learned from it. This is what we took from it,” and move forward without yelling, or getting angry, or somebody getting in trouble, or fired, or whatever the case may be.
So it’s about coming to the table for your team. It’s about building that trust between you as engineer and a leader, but then also facilitating that trust across the team as well. So making sure that a more junior engineer feels safe to experiment among more senior engineers and that they feel like their contributions are just as valid as that senior engineer’s contribution.
So as a leader, that’s sort of my perspective at this point. Facilitating that growth for the engineers and facilitating that space for them to be able to build trust with each other. And that sort of lays the foundation for building psychological safety. And then, as you move forward, continuing to show them that there’s time to experiment, that there’s time to do the right hard thing. That even though we have a deadline, there’s space and ability for them to be able to do the things they need to do and to use their engineering backgrounds, and brains, and experience, and all of that to come up with the right solution and not sort of have the right solution for them prescribed before they even start working.
And then, I would say, the other thing is making sure that you share with them and show them that there’s always space to learn. So giving them time to learn a new concept or thing, giving them time to explore a piece or an area that they’ve not explored before. All of these things also build trust, but it builds this environment where engineers feel like they have the space to breathe.
I used to tell my team, use their big brains to come up with a solution to a big problem. And they have the space to take in the information that they need to take in while not being overloaded cognitively, because that’s the final point that I’d make. Making sure that, again, as a leader, you’re not contributing to cognitive overload, because if a team is being constantly bogged down by multiple things during the day, all at the same time, they’re not going to be able to maintain that single thread of thought.
So doing what you can to reduce that cognitive load of having to context switch and all the things that a team may have to do in a day and finding ways that you can either solve for that or finding ways that you can better prioritize and better set the working agreements for your team to make that not the case.
Shane Hastie: Stereotypically, senior engineers are not the most patient people when dealing with more novices. How do we help that senior engineer become more empathic?
Senior engineers need to be mentors [08:57]
Brittany Woods: Yes, that’s a really good question. I think, first of all, one of the things that I’ve always shared with my team is when we talk about their career growth and what direction they want to go, I’ve always discussed with them that the more senior you become, the more you’re looked to as sort of a mentor in that capacity. So sure, you have more experience, and you have more technical solutions under your belt, but you’re also looked to help build up those engineers around you and that come after you.
So as you excel as an engineer, that expectation grows. And then you can either decide at this fork in the road, do you want to go into leadership? Do you get value yourself? Does it build you up to be able to build up others, or do you still get a lot of joy and meaning out of solving those hard technical problems? Because the answer to that question will very much show you which direction on that path that you should go.
That being said, though, I think showing them that important part that they play in the careers of the engineers that come after them has helped me in the past. And just making that point of, you may want to be in the technical weeds and that’s awesome. But try to take somebody with you, because somebody took you with them. And that’s how you were able to get there.
Obviously, all engineers got to where they are, because they have those big brains, and they got to where they are because they can solve the big problems. But there was always a senior engineer that was before them whenever they first started their career, maybe that answered a question for them, or kicked down a door for them, or gave space for them to solve a challenge that built their confidence. So just making that point and trying to get them to understand that it’s not without somebody else opening the door, I guess, that you’re able to grow. And so try to be that door for somebody else.
Shane Hastie: The unit of value delivery today for most organizations is the team. And you’ve given us some good advice about psychological safety and trust in a team, but let’s dig into what does that team look like and how do they work together today.
Teams need diverse viewpoints and shared goals [11:21]
Brittany Woods: I guess that it kind of depends on the type of team. Historically, you would see teams that comprised of similar experience similar backgrounds, all working toward the same problem and goal. And then, with the influx of DevOps, you saw something similar, but you would often have more DevOps-centered squads. So squads with diversity of thought, diversity of experience, all working toward the same goals, sure, but able to sort of take a different lens to make things.
And then you also had this influx of site reliability engineering teams and platform engineering teams. And so fundamentally, the way they work also changed. So I guess, from the perspective of a platform engineering team, it’s all a team with a diverse background. You’ve got software engineers. You’ve got infrastructure engineers. You have people that specialize in automation, for example.
But instead of building a thing for them or solving a problem for them, they’re now focused on solving problems for others and how they can facilitate others solving those problems in the future. So are they building a platform to enable development teams to build their infrastructure? For example, are they building a platform to enable development teams to visualize their deployments to be able to deploy faster? Maybe, but they’re always doing things with another team in mind. How can they do the thing that you historically achieved?
So one of the things I’ve called this before to my teams is codifying your brain is essentially what a platform engineering team does. They’re trying to codify their brain, so other teams are able to leverage it without having to rely on them directly. But then you have the development teams, who, again, are now more than likely made up of a diversity of thought, engineers from different backgrounds. Maybe those engineers used to be a platform engineer because software development is a key facet of that now. Maybe they used to be a tester, so a QA analyst.
Having all of that within the same team and having that expertise allows them to deliver faster. So maybe you have people with development backgrounds that are primarily focused on delivery and code, but then you also have those people with backgrounds and testing that are able to help with driving quality within the code that’s being released within that team.
So I think they’re all still working the same, and that they’re delivering an outcome, but the makeup and the composition of that team has drastically changed in recent years.
Shane Hastie: With that composition shift, what has shifted in the ways of working?
Ways of working need to be clear and explicit [14:04]
Brittany Woods: I think, fundamentally, you have to be more upfront about having these ways of working conversations. Something that I’ve found really important with my teams is going through and having the conversation and revisiting it frequently on what your expectations as an engineer are for yourself and how you plan to work with the team and other teams around you, but then also what those teams around you should expect from you in return. That can be done for the engineers external to a team and for the engineers within a team.
I think another thing, as you’re going through those ways of working conversations, we talked about psychological safety. We talked about trust. That helps to build trust, because it’s essentially engineers coming to the table and saying, “This is who I am. This is how it’s easiest to work with me. This is my experience. This is the things that I’ve either built my career on or that I have the most experience with. This is what you can rely on me for.”
So you’re giving that space for all of the engineers to sort of lay that groundwork for that relationship and set those expectations early so there’s no surprises later.
So maybe somebody has a background in software development and is part of a development squad, and then somebody else has a background in QA analysis or something. And so instead of them coming to the table feeling inferior, which sometimes happens within a team because they don’t have that same experience, they know that about each other.
And they know I may not have the same code experience as this person, this more senior engineer, but I’m bringing this thing to the table, and they know that. So they may not come to me with questions about it, but they’ll be understanding when I come to them with questions about it. So it’s sort of just like an expectation level set from the start.
Shane Hastie: And what about the bigger ecosystem, stepping outside of the team and helping our team become more effective in that ecosystem?
Communicating beyond the team into the larger organisation [16:08]
Brittany Woods: I think these ways of working also extends beyond just that makeup of the team as well, and just setting expectations of this is what my team does. This is how we work. This is how we function. This is who we are. And these are the things that you can come to us for and rely on us for. And these are the ways in which you can do that. But also, I think organizationally, it’s really important to tie all of these things back to the bigger picture.
So I have a mentor, and one of the things her and I have talked about at length is tying teams to the bigger picture. So that’s all of those strategic imperatives, or all of those things that your organization is working toward, showing your team, “This is the part that you’re playing in it. This is how these smaller things that we’re doing tie back to it.”
But then, as you start to get beyond just the team, tying that back to a group. So tying that back to maybe a director. And then they’re able to talk to their peers about, like, “This is the piece that we’re playing. This is how we work. And this is what you can rely on us for.” So they’re spreading that message, and then that sort of just spreads on and on and upward and upward. So you’re setting those expectations all the way up, but you’re also showing this is the value that my team is bringing, and this is what you can rely on us for. So people know this is where you stand.
Shane Hastie: One of the hardest areas for many organizations is getting the metrics right. What are the things that we can realistically measure from our teams?
Finding useful metrics [17:45]
Brittany Woods: I think there’s many sets of measurements. So part of my talk that I gave at QCon talked about the different things that you can measure, and it’s different with different teams. There’s all kinds of metrics that exist and frameworks that exist for measuring DevOps. I guess adoption, for lack of better phrasing.
You’ve got metrics that you can measure teams on to understand what their productivity is. You’ve got things that you can measure, like from a community perspective, to measure that the programs, and the things that you’re building, and the relationships that you’re building across the organization are functional. So I think it just depends on the team.
From a platform team perspective, which is arguably where I’ve had most of my experience working is within a platform team, I think the most important thing you can measure above anything else is adoption. Because if you’re building a thing and nobody’s using it, or nobody’s adopting it, you’ve built the wrong thing, or you’ve built a thing that might have seemed right and might be right still, but you’re missing something that would make it valuable for teams to use. So that’s the key thing you would measure there.
From a development team perspective, with the teams that I’ve led and the teams that I’ve helped support measuring things like build times, measuring things like time to delivery, those things are really important because it shows where you have a bottleneck. It shows where you might be missing a platform or where somebody’s having to reach out to somebody else, and something sits in a queue for a week. So understanding where those points of friction are, I think, the most critical metrics.
Then you get into measuring things, like doing team health checks. I talked pretty at length about health checks in my talk. That’s another unit of measure. How healthy your team feels from a psychological safety perspective, from a delivery perspective? Are they hitting their goals? And if they’re not, why? Are they feeling like they can work together and that they’re in a safe environment to do so? And if they’re not, why? So understanding all of those things are also important.
Shane Hastie: And how do you create opportunities for people to learn and grow?
Creating opportunities for people to grow [20:01]
Brittany Woods: So I think, first and foremost, it’s making space for them. So making space for people that may not try to make space for themselves. So there’s that part. There’s making space for them to learn.
So as a leader, saying something as simple as I’ve talked with product and every Friday afternoon is learning. So whatever it is that you feel like you want to brush up on or learn, try to wrap up your things by lunchtime, and then after lunch, come back renewed, and focused on learning a thing. And showing them that you’re willing to negotiate on their behalf and give them that space is again important for trust. But that’s one way to help promote learning and growing.
There’s other things that I’ve done with my platform teams in the past to make sure that we’re not building silos within the team, and that’s just having knowledge share sessions, so a weekly knowledge share where you’re giving them space to build a new skill, which is presenting to each other, presenting to other engineers, but you’re also showing them the value of that mentorship we talked about earlier, showing them the value of teaching the people around them.
But in doing that, you’re building the team up, first of all, but you’re also making sure that, again, you’re not building these tiny little silos within your team where you’ve got a whole team that’s supporting the platform, but only this one person is able to help with this specific question because they’re the only person that knows how it works. So being very careful and being very practiced about how you spread your knowledge, I think, is really important too.
Shane Hastie: What’s the future direction of teamwork?
The future of teamwork? [21:46]
Brittany Woods: I think that’s a really hard one. So when I look at where we were, DevOps was the biggest thing that teams and companies were pursuing to where we are. And you see things like SRE and you see things like platform engineering, I feel like the next iteration of that is just honing where we were, which is DevOps. You’ll see blogs, and you’ll see things about is DevOps dead and all of that. The argument that I’ve always tried to make is DevOps was like this umbrella methodology, if you will.
And from that, we got crisper, and we said, “Okay, in order to do these things, in order to follow this methodology, we need to break that down further, and we need to enable these teams.” And that’s where you started to see SREs and platform engineers come into play.
So I think we’ll continue to break that down, and we’ll say, “Okay, we’ve broken down SREs and platform engineers and dev teams into these functions, and this is how they’re working. This is how we can make it even better. And this is how we can facilitate those things. That overarching goal that we’ve been trying for for years.”
I don’t know necessarily what that looks like. It’s all driven by these points of friction, but I think we’re just going to continue further breaking that down and further figuring out this is how we get to that nirvana, so to speak, that we’ve all been trying to achieve for all of these years.
Shane Hastie: And where does generative AI fit in those?
Brittany Woods: There’s always the AI question.
Shane Hastie: There’s got to be the AI question.
Where does generative AI fit in the future of teamwork? [23:22]
Brittany Woods: There’s got to be. I think it’s interesting. I’ve been to a couple conferences now that took their entire agenda and sort of geared it toward AI. And I think the interesting thing is people think of AI as this big, overarching overlord. And it doesn’t always have to be that way.
Generative AI and AI in general, but specifically generative AI, it’s all about that help task. It’s all about those helper things that make my job, and your job, and everybody’s job easier. So I think as you start to see companies start adopting things like all of the Microsoft suite of helper tools, the Copilots, every software I think at this point has one. As you start to see engineers adopting those frameworks, I think you’ll start to see growth when it comes to engineering quality, more focus on the client and the end-user experience.
I think you’ll start to see those things come into play. So it doesn’t have to be this big AI-driven world where everything is done automatically. And you, and I, and everybody else doesn’t have a job anymore. It can just be these things that are making it easier for me to be an engineer and making it easier for me to test the code that I’ve just written, like Copilot, can help me do that and help me understand test cases that maybe I, as a human, didn’t think about, but it could see because it’s processing tons of things.
I mean, I think that’s where we are, at least in the short term. I’m not an AI expert by any means, but I think it’s just seeing engineers getting used to and embracing things that help make their life easier and help reduce that cognitive load that we talked about earlier, in favor of them using their human brain to be able to develop these solutions because it takes us teaching for AI to be successful.
Shane Hastie: Let’s use some really useful points and lots of food for thought here. If people want to continue the conversation, where do they find you?
Brittany Woods: I am on Bluesky, which I don’t know if a lot of people have adopted that yet, but that’s sort of where I’m at in terms of social media. I’m always on LinkedIn and available to chat. And then I have and maintain just a technical blog/personal page. Any of those areas, you can reach out to me. I’m always happy to have a chat really on anything. I’d love to meet new people.
Shane Hastie: I’ll make sure we include those links in the show notes. Brittany, thank you so much for taking time to talk to us today.
Brittany Woods: Thank you for having me.
Mentioned:
.
From this page you also have access to our recorded show notes. They all have clickable links that will take you directly to that part of the audio.
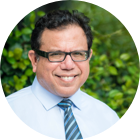
MMS • Nikhil Simha
Article originally posted on InfoQ. Visit InfoQ
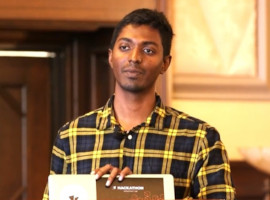
Transcript
Simha: We’ll start with a story. The story is of a civilization. A civilization that was really good at building things. It was expanding. It found this piece of land where they said, let’s build a huge tower that stretched into the sky. They started building, and it looks like they’re about to finish it, they’re about to reach the sky. The gods in the sky said, “We don’t like this.
We’re going to prevent these people from reaching the sky.” This is where it gets interesting. The gods say, the way to prevent them from doing this is by confusing their language. If we destroy the unity of their language, we will prevent them from building large complicated things. This story is 2000 years old. There is a variant of this story that is 2000 more years old, so 4000 years ago from a Syrian myth. The lesson here remains the same, if you want to build large complex things, and run civilizations that span the globe, you need to have linguistic unity. A similar way of communicating with systems and people.
ML’s Direction (Supervised Learning)
Let me address the elephant in the room first. There is a lot of talk about generative AI. This is a slide that I borrowed from Andrew Ng’s presentation in Stanford, called, “Opportunities in AI.” If you see, there is a prediction of where different kinds of machine learning will go. There is generative AI, your ChatGPT type stuff. There is the traditional machine learning called supervised learning, the big green blob, and unsupervised and reinforcement learning.
The prediction says that supervised learning is going to be pretty big, and is going to grow. Why is that? Why would supervised learning have such staying power? There are two reasons. This is the very first artifact on which records were kept. It has a tally of some sort, we don’t know of what, on a bone, found in France, which dates back to about 17,000 to 12,000 years ago. The point of this is that since we know counting, we have been counting and keeping records of it. It is as primitive as language itself.
Since then, we have been counting by different names. We have been keeping records by different names. Today we call it data warehousing. As long as we count and we speak, we’re going to keep recording data, maybe in different forms. This is very fundamental to our civilization. This is why supervised learning has that very high staying power, because supervised learning allows us to incorporate labels and make predictions on numerical data. This is why feature engineering is going to be relevant for time to come.
There are certain things that make feature engineering hard. First one is that features can be in different forms. The raw data that powers these features could be in a data stream, or in a data lake, which is data at rest, or from services. Usually, there is hundreds of features per model, that means to power this model, what ends up happening is we end up creating pipeline jungles. There is way more pipelines to power a model that create features, than there are pipelines to train the model or serve the model. This is what makes iterating on a model hard. Whenever you need to add new sets of features, you need to create many more pipelines that span the data stream, the data lake, or the microservice.
Essentially, features are the bottlenecks. There is a survey at Airbnb and at different companies, which says, 80% of the time is being spent doing feature engineering and doing data pipeline. What adds one more dimension of difficulty is the high fanout. There is one prediction but hundreds of features, that means we need to fetch them very quickly and the latency budgets are pretty tight usually.
Chronon – Feature Platform
This is where Chronon comes in. Chronon is a feature platform that addresses all of these problems. We’ll start with an example. It’s a scenario where we’re trying to predict if a transaction is fraudulent. You’re a bank and you’re trying to identify if a particular transaction of a user is unusual. We’re going to use only one feature. The feature is essentially measuring how far away from the mean is the current transaction amount. This is called a z-score. You take the current amount, subtract from the mean, and divide by variance. It tells you how far away from the distribution is the current transaction. Here, user is Leela. We have timestamps of transactions of this user. Then there is an amount. What the z-score will tell us is how far out of the distribution.
A negative number will tell us how unusually low it is, and a positive number will tell us how unusually high it is. The higher the number, the more fraudulent it probably is. A model will take in the z-score and tell us whether it’s a fraud or not. This is an example. In the real world, there will be hundreds of such features. Assuming the distribution is a normal distribution that looks like this, or a bell curve, the distance from the mean, measured as variances from the mean, will tell us how much out of the distribution it is. If it is three standard deviations away, that means it’s less than 0.3% likely that it’s unusual transaction. How do we keep this up to date in real time? How to measure the z-score in real time.
We talked about two terms, mean and variance. Mean is sum by count. To update the mean in real time, you need to maintain sum and count. Similarly, to update variance, you need to keep track of three things: count, sum, and square-sum. Whenever a new transaction comes in, you bump the count, you bump the sum, and you square and bump the square-sum. There is a problem with this approach. The issue is that squared-sum is very prone to overflowing. There are various ways of dealing with this, called Welford’s algorithm. The point is, we manage this in the framework for you. Meaning, Chronon figures out what is the state that needs to be maintained, and it’ll update it in real time, and compute the means and variances for you. When you need the z-score for an amount, you can run this formula.
Just to put this in familiar terms, I’m going to show a pseudo SQL. Pseudo because this kind of SQL is not very easy to write. If you look at this thing, we are maintaining mean and variance in real time, it’s really simple. It’s a GroupBy in SQL. You maintain mean and variance of a particular user. The data source is what makes it interesting. The data source is going to be the transaction history table. The current transaction stream is grouped by the user.
When you need to compute the z-score, we read the amount, subtract the mean, and normalize it by the variance, and return that as the z-score for a particular user. Because it’s grouped by user, it’s going to be fast when you query with where user equal to Leela. Behind the scenes, what we do is we look at the transaction history source, which is data at rest, a table in the warehouse, so Hive, or Redshift, or BigQuery. Turns that would be a warehouse table. We bootstrap the triple that we need to maintain, or the state that we need to maintain, and update this in the key-value store. Then, when new transactions come in, we update the triple again, and increment the count, increment the sum, increment the squared-sum.
This is not exactly what happens. We talked about Welford’s algo. This is just to give you an intuition. When a z-score is requested, we pull mean and variance, and normalize the amount based on that, and compute the z-score, basically. If you see, we are splitting the computation across three systems, three systems with different ways of interacting with them. The first system is batch processing. The second one is stream processing. The third one is serving. This is where most of the power of Chronon comes from. People are able to write these kinds of statements, and we behind the scenes will create the pipelines that are necessary to maintain these features and serve them in low latency.
First, we will talk about the inputs to the code example. We are going to say the user, merchant, amount in dollars, and status of the transaction, and timestamp. This is the schema of the transaction. We talked about only one z-score of the user distribution. To make the example a bit more interesting, we are going to compute three z-scores. The model is going to look at three distribution distances instead of one. It tells us what’s the distribution distance for the user, how far it is away from the usual transactions of the merchant, and how far it is away for the user-merchant pair. You spend $5 at Starbucks, probably, and $100 at Costco. Knowing how much you spend typically at a merchant makes more sense.
We’re going to filter the data out. We’re going to filter out and only select the successful transaction, but not the cancelled, or refunded, or pending transactions. This is what a Chronon GroupBy looks like. This is called a GroupBy. I’ll walk you through these things slowly. The first thing to note are the key columns. This is a Python function that takes arguments which are the key columns, and groups and computes mean and variance by those key columns. If you look below, the keys are user, merchant, and user-merchant pair. The other interesting aspect of it is the source.
If you look at the source, the source is composite, which is the main deviation from SQL. We allow you to specify Hive tables, or the mutation streams, or the mutation logs of this table. Transactions can mutate. We allow you to specify all three. We will see what exactly is an entity source in the coming slides. The idea is that a source is composite. It’s not just its manifestation in the warehouse. The interesting thing is we run this computation across these sources, and ship the computation to stream processing pipelines/pipelines of services behind the scenes.
Usually, there is hundreds of such features. The way to put them together is by using a join. In this join, we are putting together those three features that we created. Usually, there might be other features coming from other sources for the merchant, or for the user, or for that interaction. We express the z-score as a derivation. We’ll unpack all of this in the coming slides. The idea is that we GroupBy, and then we use a join to get all the features together in one place, and then apply derivations which compute the z-score.
The interesting thing about Python, where it adds power compared to SQL, is that, in SQL you would have had to repeat a lot of queries again and again, for each of the key pairs. In Python, you can essentially use the framework to write functions and abstract away, and reuse code better. There are other reasons why we use Python, because we want to extend what’s possible in SQL in a large way. How do you use this? You define that join. Let’s call it payments, and you’re trying to detect transaction fraud, and it’s the first version. You query. You say for Leela and for this merchant, Chewy, and this amount, 35, what are the z-scores? The response would be the user_z_score, the merchant_z_score, and the interaction_z_score. Model would look at these things and tell us if it’s fraudulent or not.
The next thing to talk about is what makes the aggregation engine in Chronon very powerful. In Chronon, time is a first-class citizen. If you make time a first-class citizen, you are able to express time windows. What’s the point of time windowing? What is time windowing? Instead of saying the transaction z-score of Leela, for the last 20 years, you’re able to say, compute the distribution z-scores for the last year. Because maybe, if you get promoted or if you start your first job, your distribution and transactions will shift, and if you lose money, it will shift down. This allows us to account for such changes. This allows us to separate natural patterns. Maybe every week or every month or every year, you have a period of time where you spend a lot more money.
Like I said, recent data is the most important data when it comes to fraud detection or for personalization. The last, but not the least, is whenever a new user registers in your bank or in your platform, you want to be able to do fraud detection for them without having to wait for a day. Again, why windows? What does this mean? If you have just lifetime aggregates, just sums and counts, they are monotonically increasing. The issue with that is that the distribution of features are shifting, and you will need to retrain the model. What windows do is they make these values stable. Stable distributions mean stable predictions.
Those are the benefits of windows. Windows are not easy or cheap to build. What makes these windows hard? The first thing is tail eviction. You are going to remove events from the tail of the window. That’s a very unusual thing. Whenever a new event comes in, you can trigger some computation. How do you trigger a computation for eviction? We’re going to see how Chronon manages this.
Take a minute to think about how would you remove old events from a window. Because you need to keep a set of events in memory all the time to slide the window forward, it makes the windows very memory hungry. In Chronon, we use a variant of these windows called sawtooth windows. This is a timeline, and e1, e2, e3, up to e6 are the transactions. We are computing some aggregations in a 1-hour window. If a query comes in at 2:27, we go back to 1:27, look at all the events in that time, and do the aggregation. This is what’s called a sliding window where the scanning is exact. The problem with this is that sliding window is very memory hungry, you will need to store all the raw events. If the windows are large, like 14 days or a year, it will become more expensive.
There is another kind of window called the hopping window, where instead of being very precise about the time, if you’re allowed to be a bit stale, you can basically partially aggregate all the data into hops. Hops, in this example, are the 10-minute buckets in the 1-hour window. What we do is called a sawtooth window, which is a variant of these two windows. We use the hops and we also get the most recent data. Essentially, the timeline goes from 2:27 to 1:20. It’s slightly more than an hour, and that’s the tradeoff. We get freshness and cheapness.
We take into account most recent data, but at the same time, we are able to compress old data into hops. Just to summarize what I said, why sawtooth? Hopping windows are stale, so they’re not good for fraud detection, or personalization. Sliding windows are memory hungry, so they’re really hard to scale. Sawtooth windows get the benefit of both worlds, but it sacrifices the tail precision, meaning it’s not exactly 1 hour, but it’s going to be 1 hour plus up to 10 minutes. The advantage is that it’s going to be fresh, most recent data is accounted for, and it’s cheap to compute.
How do we maintain this window in Chronon? The red boxes are all the raw events. We break the window into three parts, the head of the window, it’s the most recent events, the mid portion of the window, and the tail portion of the window which is the oldest events. If we are computing a 7-day window, we are going to first compress the tail into hops. We talked about 10-minute hops in a 1-hour window. This is something like that. We compress the tail events into these green boxes. Fewer boxes mean less data to fetch and less data to store. We compress all or aggregate all the data in the mid portion of the window into a single value, or into a single aggregate. We use batch processing, or Spark, in particular, to maintain these aggregates.
Instead of storing all the raw events or all the red boxes, we store fewer boxes, so the green boxes. The head events are maintained using stream processing. The idea is that instead of fetching and storing a lot of data, we fetch fewer data or fewer boxes. The reader is going to read and aggregate this into a result. This is a Chronon client, which does this aggregation behind the scenes. We partially aggregate first, and then merge all the partial aggregates later on read. Whenever a mean or a variance is requested, we look at all these tiles or hops behind the scenes, or all the raw events, pull them and reaggregate for you.
Next thing about GroupBys, or what makes them a bit more interesting compared to the SQL version of the GroupBy is bucketing. Usually, depending on the category of the transaction, the z-score varies, or the distribution varies. Maybe you want to bucket by the category of the transaction. If you spend a couple thousand dollars on groceries, it’s fraudulent, but it’s not going to be fraudulent if you probably buy home supplies. What we are doing here is we are bucketing by the category, so mean by category. For groceries and utilities, we have different means. The next thing I’m going to talk about is called auto-explode. A lot of times you have itemized bills.
There are different scenarios where you get these lists of things in the column, and you want to still be able to do your aggregation or mean and variance over these lists. In SQL, you would write what’s called an explode statement and then do GroupBy. In Chronon, this happens automatically. You just specify mean. We unpack this list and compute the mean. The other thing is that you can use bucketing along with explode. Explode is implicit, but you can still bucket over nested data or lists of data. In fact, you can specify windows, buckets, and whether you want to explode or not, in any combination you want to. You can have windows, buckets, and explode, or you can follow any of them.
We talked about GroupBy, which is an aggregation primitive. The point of this is that it allows you to specify feature views that are maintained by Chronon in real time. We talked about windowing, and sawtooth windows in particular, and why they’re more powerful than other kinds of windows, and more specific to machine learning. We talked about bucketing and auto-explode.
These GroupBys also map to feature groups, and they are a unit of reuse. These transaction features get reused across many different models. The other thing is that, because these are reused, they are immutable ones, they are online. Once you say this thing is online, and many models are using it, the only way to change or add a new version of this is to create a duplicate.
Sources
The next and probably the more important thing that we are going to talk about is sources, or data sources. We talked about how data is available in different kinds of sources, so like data streams, or data at rest, or data in warehouse and in microservices. There are many patterns in which this data is stored. If you have heard about terms like CDC, or events, or fact tables, or dims tables, or star schema, that all falls into this pattern of representing data. All of this can be very complex to deal with and create feature pipelines out of. Moreover, you are taking what’s a simple computation and splitting across these different patterns and different sources. Because we want to unify compute, we need to have a good representation of these diverse sources of data. Just to take a very short detour, and talk about the high-level view of data sources. In a web company, or in a digital company, usually you have a service that is serving users, which is backed usually by a production database that’s serving data to these services.
Then, you collect the data from these two sources into your data streams and into the data lake. Streams are more real time and data lake is historical. Whenever events happen, like clicks, or impressions, usually they go into an event stream. They end up in an event log to store historical versions of these events. These production databases also get snapshotted. Every day or every hour, you take snapshots of this database, and put them in your data lake. The point of doing all of this is you’re recording history. You’re recording history, and you’re trying to compute features at historical points in time, or use this data for other kinds of analyses other than machine learning.
The other thing this database has produced is changes. If you’re changing rows in the database, they create mutations. This conventionally is called change capture. You have a change capture stream and a change capture log. We will see examples of each of these things in the subsequent slides. Then, there is derived data. People might be taking all of this data or some external data and deriving or doing computation over this. This is roughly the land. I simplified it significantly. There is other variations on top of this. This is what at a high level the data landscape looks like.
If you look at this event stream and log, they represent the same data. Similarly, the change capture data and the database snapshot, it’s the same data. We’re just storing it in different ways. This is where Chronon unifies the idea of a source. You are able to specify all of these things as a single source. The first sources are events. These are your clicks and streams. In the warehouse, they are stored as partitions of events. Every day, we store only the events that happened during the day, in that partition or in that day’s partition. The other name for these in star schema terms is fact sources. The important thing is that with these sources, if you have the timestamp, you can reconstruct what a feature value would have been in history.
We’ll see why that is important. We’ll see why deconstructing feature values is very important. The other thing that you can specify as part of the source is the data stream. This is used to update and maintain features for feature serving. The next one, this is just the representation of the data we saw earlier as event tables. I removed the user merchant columns for simplicity. These are transactions with their IDs and with the amount at different timestamp of a given day. In the next day, you will have a new transaction. The issue with modeling transactions as events is that it does not allow for mutations. Let’s say you go to a restaurant and you add a tip, it registers in your bank as a mutation to a previous transaction, where the tip gets added. We’ll talk about entities.
Entities allow us to model these mutations to transactions. In the example you saw an entity source, and this is what that looks like in reality. Each partition contains data for all transactions. It could be a bit repetitive. We also have table snapshots, so the transaction table is snapshotted every day or every hour. We have mutations which tell us about the changes to the rows in these tables.
Just to show the example in terms of data, let’s say you have a transaction table in a particular day, and in the next snapshot some of the rows have mutated. If you see, the transaction ID 3 is missing, 4 is new. Transaction 2 somehow went from 40 to 400. There is another table or another stream called mutation stream or change data stream or BinLog. There are many names to it.
What essentially it says is it tells us how these rows have changed at what times. If you see, this represents that 3 is deleted. There is only a before value, but no after value for the transaction ID 3. Transaction ID 4 is inserted, so there is only an after value. Transaction ID 2 is updated, so it went from 40 to 400. There is a before value, which is 40, after value, which is 400. Then there is a new updated row in the new partition. Some of this is not yet an industry standard, but systems like Debezium and Kafka, they are making this easier. What Chronon is able to do is use these set of data sources and compute features at millisecond precise timestamps.
Imagine if you didn’t have the mutations, and just had these two tables, you will only be able to compute features as of midnight of 9:20, and midnight of 9:21, but not in between. Having these allows us to recreate the state of the table at any point in time. Other related thing is to use service as sources of features. This is an extremely bad idea. We’ll see why that is. A model is requesting features from a service and service reaches back into a database, and does probably a range scan or an aggregation. This requires significant disk bandwidth, and can slow down other queries to the database and slow down your feature request. Amplified across hundreds of features, this is going to make the read latency very bad.
The other interesting thing is that the model owner is usually not the service owner. There is a payments team that is maintaining this table, and then there is a payments risk team that is trying to predict the validity of a transaction. These two are different teams. You need to go and convince someone in the payments team to do the wrong thing. That’s usually impossible. This is where entities really help. Instead of talking to the table directly or talking to the service directly, you can look at the change data stream and the snapshot. You can use it to compute features before the query even arrives, and maintain a feature index. The model simply fetches these features from the feature index. If you see, we are no longer required to talk to the service owner, we no longer need to hit the transaction table directly, have slow scans, and risk other data flows that update the table. We have our own isolated system that is maintaining features now. This is what makes entities extremely powerful.
Just to recap what I said, change data gives us agility. You don’t need to cut across organizational boundaries and convince other people to update their live systems with range scans. It will also create isolation because we’re not impacting the producer of that data anymore. Again, efficiency. Because we are not doing range scans and most of it is pre-computed, the features are going to be fetched in much lower latency. Last thing is scalability. Because they’re pre-aggregated, you can look at much larger ranges of times to construct your features instead of limiting yourself to smaller ranges. It comes with some limitations. There are two classes of aggregations, the first class, of which the examples are sum, count, mean, variance, can be subtracted.
When there is an update or a delete, you can remove aggregations or subtract events from the aggregation. That’s not the case for other aggregations, like first, last, min, max. These aggregations don’t allow you to subtract. In Chronon, the batch job, we talked about the breaking of windows into different pieces, and batch job is updating the mid and the tail, that corrects away the inconsistencies that are created by these subtractions. To step back, the variation between the offline and the online system is a huge reason why a lot of ML use cases don’t succeed when they’re online. Having this batch job that corrects away inconsistencies regularly, helps us to keep the features consistent.
The last source is what’s called a cumulative source. These are insert only tables, which means you don’t need to look at mutations at all, you know that they are all insert only. The snapshot itself is enough. Each new partition is a superset of any old partition, and latest partition is enough, and there is no deletes and updates. Just to show the example, this is what you would represent transactions as, if you’re using slowly changing dimensions or cumulative events. You would have the same data in the next partition with a few additional rows. If you see, you really don’t need the previous partition, this is enough to recompute a partition at any data or any particular point in time. This is enough. You don’t need mutation tables separately to understand what was the feature value at historical points in time.
We simply do what’s called a surrogate column where we say, between what times was this row valid. Whenever there is a new row, we just don’t have an end time, that means this is current. The benefit of this is, if you store only the most recent partition, you are reducing repetition. You don’t need to store this transaction ID 1, again and again. You can simply delete this partition and just keep this. This also makes computing over this data very efficient.
Those are the three sources. We talked about events, which can be real time, using entity streams and logs, or fact tables. We have entities which are table snapshots or dim tables. We have cumulative, which is slowly changing dimensions that we talked about. In Chronon, users just tell us what is the type of the source. They don’t tell us what are the ranges that we need to scan, and they don’t tell us how to optimize the query. They just tell us what is the data model or the pattern of the data. We figure out, behind scenes, what is the right partition range to scan, what is the minimum required partition range to scan. This was added as part of a rewrite.
It was added because we realize that manually specifying these ranges is a huge source of errors in feature computation. If people just tell us what the pattern of the data is, and if they don’t specify these ranges, it’s much easier to do the right thing and to avoid errors. We talked about different source modes as well, stream and batch, talked about services. The interesting thing about services is that you cannot backfill or compute features as historical points in time. This is also slow, very high effort, and very hard to isolate, and very hard to convince people to pull features from services. We recommend that we should use CDC instead of directly talking to services.
Join
Next, we’ll talk about join. Join is a primitive that allows you to put together hundreds of features into a feature vector. It allows you to also define labels. It allows you to define external services to create that feature vector. Before we talk about the details behind the join, we should talk about this thing called log-and-wait. When there is a real time feature, and when we need to serve these features, what we do is we take this raw data, convert them into a serving system, and begin logging, and wait for these logs to be enough to train a model.
Then, we know if this particular feature is useful. To tell if your idea is going to be useful, you need to first build the serving layer, then wait until you have enough logs, and then you will be able to tell if it’s useful or not. This process takes months. What you can do instead is simply backfill, or compute features at historical points in time, and simulate those features.
Going back to our example, we have this table of queries. These are points in time at which we need to produce features. We are producing the z-score feature. We talked about how we would do this using a serving system. What Chronon can do is backfill them without having to create the streaming pipeline or keeping an index. It will just look at the raw historical logs and create these z-scores for you. You can train a model and immediately know if the z-score is useful or not, without having to wait for 6 months and without having to do all that additional work. This speeds up the iteration time or your experimentation time considerably. This is just the same example that we showed before but with the source.
Join is an enrichment primitive. We are taking this transaction table which has all the chargebacks or fraudulent transactions, and we are enriching it with new features. If you have another feature idea, you would add a line here, basically. We are able to compute the entire feature training set and train a model, and be able to judge if this feature is useful or not.
Just to visualize, a join allows you to put together features from different feature groups or GroupBys, and from external services, also incorporate contextual features. Amount, for example, was a contextual feature on which we computed z-score. You can also specify labels. This allows you to create this wide feature vector, or wide training dataset. The interesting thing about GroupBys is that they can be both online and backfill. If you use services, you have to rely on logs, and log-and-wait. Labels are only used offline to generate training sets. This is what a Chronon join does. It maintains all of these things, all of these moving parts, and evolves the training set as you update it. It’s also used for serving.
Just to summarize, it’s an enrichment primitive. It can enrich tables with features. It can reply to requests with features. It can enrich topics or streams with features. The interesting thing is that each of the join part has an implicit key. We looked at different keys, user, merchant, and user-merchant pair. Each of these join parts is already keyed, and the keys are implicit. The join knows how to fetch the right data and fan out to the right data source. We also generate the names of these features automatically for you. It is a variation based on a couple of things. It’s based on the name of the feature group, name of the input column, name of the operation, mean or variance, name of the bucket.
If you’re bucketing by groceries, or the category itself, the bucket would be category. The window is the amount of time that is used for aggregating features. We have names that look like this. These are transaction features where we’re computing the mean of the amount, bucketed by the category in the last 7 days. It’s long, but the idea is that reading this will give you a quick sense of what this feature is doing, and where to go look if there is an issue with this feature, or which files to look at if you have an issue with any of these features. One thing we did not talk about here is, how do we actually scale the backfills? That requires custom optimizations. There are other talks that we have given in the past that talk about optimizing these backfills and computing features in history.
Recap
We talked about GroupBy, which is the aggregation primitive, and the unit of reuse. We talked about source, many different kinds of sources, can be batch or streaming, they can be events, entities, or cumulative. Users just tell us what the type of the source is, and we take care of doing the right thing for aggregations. We talked about join, which is an enrichment primitive that produces training data and that serves features. This is mostly what users interact with. They run a join to generate training data, and they prepare this join to serve features. We didn’t talk about a lot of things.
We didn’t talk about algorithms to scale backfills, or observability, or metadata, which is also autogenerated based on the joins. We generate dashboards and alerts. We also generate logs and maintain the schema of these logs as joins evolve. We didn’t talk about how to compose these different things, so create chains of GroupBys that feed into a join that feed into another GroupBy. These are things that are all possible with Chronon.
Questions and Answers
Participant 1: You mentioned that if you gave Chronon the entities, it will derive the data. If you gave it an incremental event stream, it can construct the point in time?
Simha: Exactly.
Participant 1: It does feature generation, if you have the primitives of aggregations, and all that stuff. What about feature selection and elimination after that?
Simha: This is what users want to do. They want to test their ideas and want to remove ideas that don’t work. This is something users do. The framework helps you evolve your data when you try to do it. It’s like removing feature groups from a join or adding new feature groups, essentially.
Luu: Is this open source or about to be? What’s the current state?
Simha: The current state is that it’s in a closed beta. We are working with a few other companies, and deploy it there and see what happens, and incorporate some early feedback before open sourcing.
Luu: At some point in the near future looks like this framework is going to be open source. I think that’s going to be very useful for a lot of companies that are doing feature engineering at scale and such, all these very interesting features.
See more presentations with transcripts
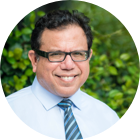
MMS • Sergio De Simone
Article originally posted on InfoQ. Visit InfoQ
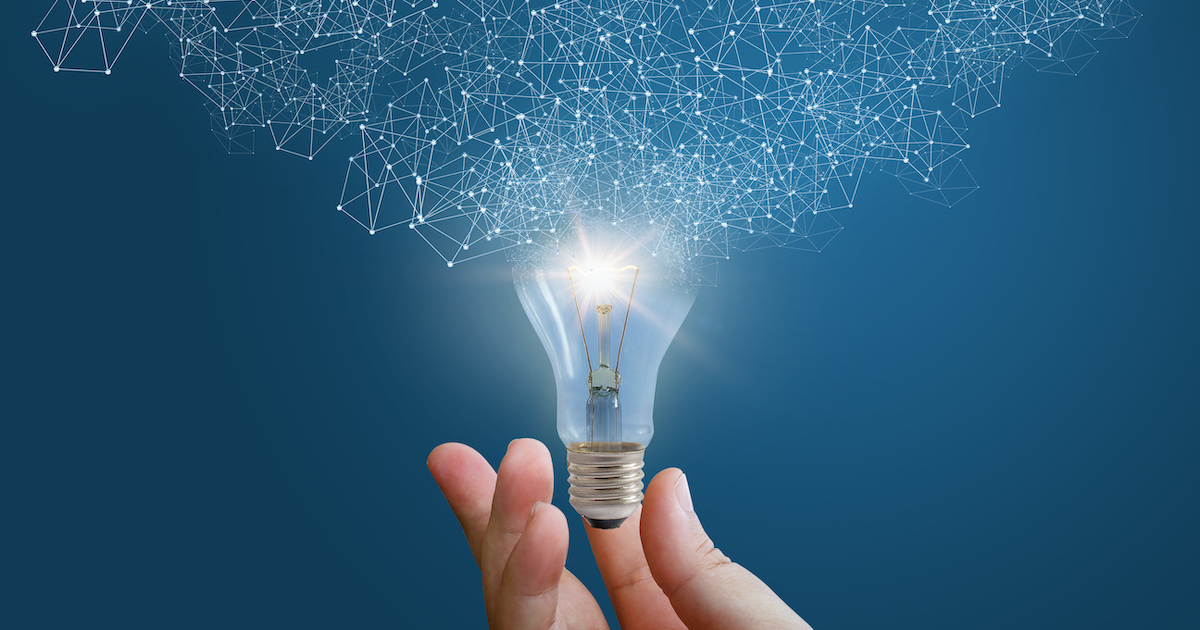
GitHub has launched GitHub Models, a free capability aimed at letting developers explore various AI models from within the GitHub tool ecosystem and make it easier to deploy AI-based services using Azure AI. GitHub Models includes both private and public models and is currently in closed preview.
GitHub Models provides a playground that lets developers test prompts and fine-tune model parameters. In order to use a model, you go to the GitHub Marketplace and select the one you want to work with, for example Meta-Llama-3-70B-Instruct. From the model page, you can get further details about how to get started, as well as inspect rate limits associated to that model. The playground also makes it easy to switch from one model to another and compare their behavior. For example, GitHub describes a scenario where a developer defines their prompts using GPT4o, then switches to a lighter-weight model like Phi-3 mini to see how it performs with those same prompts. Finally, when they are satisfied with the results, the model can be deployed directly to Azure AI by replacing GitHub tokens with Azure credentials.
The GitHub Models playground is also integrated with GitHub Codespaces, allowing developers to spin a new pre-configured Codespace environment directly from the playground once they are satisfied with the results. The integration with Codespaces appears to rely on existing SDKs that are already available for Azure AI with support for several languages. The generated codespace uses the entitlements that come with a GitHub account, which means you do not need to add an API key or sign up for any other services, making it straightforward to start coding with any of the supported AI models.
The new capability is also integrated with the GitHub CLI, allowing users to run
a given prompt against one of the supported models and see the results in the terminal. The run
command can be combined with other git commands, for example, to summarize the last commits from a repo, you could use:
git log -n 10 | gh models run phi-3-mini-4k-instruct "Summarize this commit history. Use max 1 line per commit"
A few commenters on Hacker News pointed out that while closely related to Azure AI Studio, GitHub Models has the advantage of being free from the constraints deriving from Azure AI Studio enterprise-level security features, which makes the latter cumbersome to use when it comes to authentication and lagging behind competitor playgrounds in terms of supported models versions. On the other hand, some criticize that the only paid tier available is through upgrading to Azure AI.
At the time of the announcements, GitHub Models supports Llama 3.1, GPT-4o and GPT-4o mini, Phi 3, Mistral Large 2, and others. Not supported is the possibility for users to upload and run their custom models. As a final note, according to GitHub, no prompts or generated outputs are shared with model providers, nor used to train or improve the models.
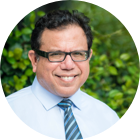
MMS • Ben Linders
Article originally posted on InfoQ. Visit InfoQ
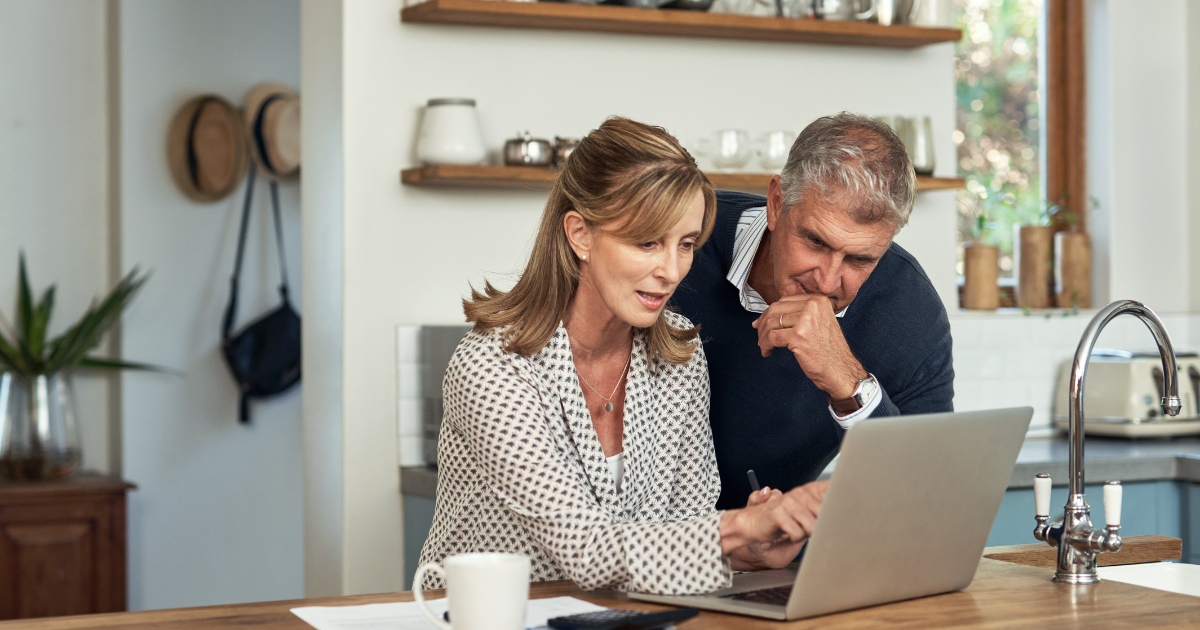
Public software platforms should be inclusive and accessible to everyone, where people feel comfortable using them. Aleksandra Osińska and Natalia Sidor gave a talk about crafting public platforms at ACE Conference.
It’s a big challenge to design a platform for everyone, from digital newbies to natives, with varying knowledge and experience of administrative processes, Osińska said. Users can have diverse needs and life situations, for example, a teenager applying for their first ID has different needs than someone doing it for the second or third time.
We should understand that while we can design anything we want and the wireframes can handle our ideas, the actual government platforms cannot. We must explore what’s possible, Sidor said. She mentioned data connection as an example:
Depending on the country’s government system, connecting data from different platforms may be easier or more complex, which affects design and development.
Osińska suggested providing services categorisation by life events with easy navigation. When designing government platforms, we should focus on a content-first approach, hence designing them as an open knowledge hub is crucial, she added.
Government platforms must ensure their users’ trust so that they feel safe using government services online, Osińska stated. More and more public platforms offer a convenient single sign-in. At the same time, they enforce multi-step verification during the logging-in process, such as confirming the user identity through a bank application, SMS, or email.
In terms of public platforms, inclusive design means ensuring everyone feels comfortable using them. The interface should adapt to accommodate all users, regardless of their circumstances, Sidor mentioned.
Accessibility is important for everyone, not only people with disabilities, Sidor said. Everyone benefits from fully accessible products:
For example, we can all experience temporary difficulties, such as a broken arm, conjunctivitis, or simply entering a website on our phone in a crowded bus with our other hand busy. Accessible products mean that all of us can use them, regardless of the situation we find ourselves in.
When creating a public platform, Sidor advised prioritising simple design and interface. There’s no such thing as making the interface too easy, she said; everything should be well-explained and easy to access.
InfoQ interviewed Aleksandra Osińska and Natalia Sidor about designing inclusive platforms.
InfoQ: What can be done to create an inclusive online experience?
Aleksandra Osińska: An inclusive platform should encourage users to find information about services and perform the necessary actions online. You can use a life-event-centric approach to address different needs and situations. Aside from standard categories (e.g. service names), we should also have categories that correspond to life events, like getting married or looking for a job. This way, we meet everyone’s needs, even those who do not know legal or administrative procedures.
Natalia Sidor: For example, when creating a form, we must consider various life situations, e.g., including the possibility of selecting a gender identity in our forms. There are also local and foreign users who have different experiences and legal documentation. We can’t exclude people who, for example, don’t have a PESEL number (an identification number in Poland). Instead, we must address their situation, explain what they can do, and provide alternative identification methods.
InfoQ: What’s your advice for increasing accessibility?
Osińska: I’d advise everyone to test and validate designs and flows with users who have diverse needs to see whether they can easily find needed information and complete a required process successfully. This is the best way to ensure our platform is accessible and user-friendly.
If possible, we should test the product on ourselves, using various methods known to people with disabilities. If we want to test whether a product is accessible to visually impaired people, let’s test it with a screen reader and see if we can use it. This approach validates the designs and empowers us to better cater to the users’ needs.
Sidor: Certain rules ensure a great user experience: appropriate contrast, font sizes, and responsive design elements. But we shouldn’t simply follow the rules; we must empathise with our users and consider their different needs. Accessibility should come first and visual aspects second.