Month: September 2024
Presentation: Are You Done Yet? Mastering Long-running Processes in Modern Architectures
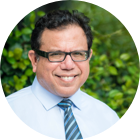
MMS • Bernd Ruecker
Article originally posted on InfoQ. Visit InfoQ
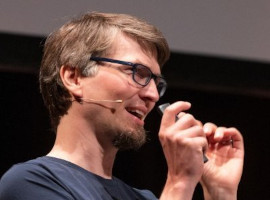
Transcript
Ruecker: I talk about long running, not so much about exercise, actually. We want to start talking about food first, probably more enjoyable. If you want to order pizza, there are a couple of ways of ordering pizza. You probably have ordered a pizza in the past. If you live in a very small city like I do, if you order pizza, what you do is actually you call the pizza place. That’s a phone call. If I do a phone call, that’s synchronous blocking communication. Because I’m blocked, I pick up the phone, I have to wait for the other person to answer it. I’m blocked until I got my message and whatever, whatnot.
If then the person answers me, I get a direct feedback loop. Normally, that person either tells me they make my pizza or they don’t. They can reject it. I get a direct feedback. I’m also temporarily coupled to the availability of the other side. If the person is currently not available to pick up the phone, if they’re already talking on another line, they might not be able to take my call. Then it’s on me to fix that. I have to probably call them again in 5 minutes, or really stay on the line to do that. Synchronous blocking communication. What would be an alternative? I know you could probably use the app. Again, I can’t do that where I live. You could send an email. An email puts basically a queue in between. It’s asynchronous non-blocking communication, and there’s no temporal coupling.
I can send the email, even if the peer is not available, even if they take other orders. How does it make you feel if you send an email to your pizza place? Exactly that, because there is no feedback loop at all. Do they read my email? I pick up the phone to call them. It could be. It’s not a technical restriction that there is no feedback loop. They could simply answer the email saying, we got your order, and you get your pizza within whatever, 30 minutes. You can do a feedback loop again, asynchronously. It’s not really the focus of today. I have another talk also talking about that this is not the same. You can have those interaction patterns decoupled basically from the technology you’re using for doing that. Synchronous blocking communication, asynchronous non-blocking.
The most important thing is on the next slide. Even if I do that independent of email, or phone, it’s important to distinguish that the feedback loop is not the result. I’m still hungry. They told me they send a pizza, I’m probably even more hungry than before the result of the pizza. The task of pizza making is long running, so it probably goes into a queue for being baked. It goes into the oven. They hopefully take the right time to do that.
They can’t do that in a rush. Then the pizza is ready, and it needs to be delivered to me. It’s always long running, it takes time. It’s inherently there. That’s actually a pattern we see in a lot of interactions, not only for pizza, but for a lot of other things. We have a first step, that synchronous blocking, but we have an asynchronous result later on.
Could you do synchronous blocking behavior for the result, in that case? Probably not such a good idea. If you take the example not of pizza but of coffee. If you go to a small bakery and order coffee, what happens is that the person behind the counter takes your offer, takes your money, basically turns around, going for the coffee machine, presses a button, waits for the coffee to come out of that. Going back to you, give you the cup. It’s synchronous blocking. They can’t do anything else. I can’t do anything else.
We’re waiting for the coffee to get ready. If you have a queue behind you, and if you’re in a good mood to make friends, you probably order 10 coffees. It takes a while. It’s not a good idea. It’s not a good user experience here and it doesn’t scale very well. The coffee making is relatively quick compared to the pizza making and other things. It doesn’t have to be that way. There’s a great article from Gregor Hohpe. He called it, “Starbucks Doesn’t Use Two-Phase Commit.” He talked about scalable coffee making at Starbucks, where you also separate the two things. The first step is the synchronous blocking thing. I go to the counter, order, pay. Then they basically ask for my names or correlation identifier. Then they put me in a queue, saying, to the baristas, make that coffee for Bernd. Then these baristas are scaled independently.
There might be more than one, for example, doing the coffee, and then I get the coffee later on. That scales much better. That’s another thing you can recognize here, it also makes it easier to change the experience of the whole process. A lot of the fast-food chains have started to replace, not fully replaced, but replace some of the counters or the humans behind the counter with simply ordering by the app. Because that’s very easy for the first step, but not so easy for the coffee making. There’s robotics also for that. There are videos on the internet, how you can do that, but it’s not on a big scale. Normally, the baristas are still there, the coffee making itself. We want to distribute those two steps.
With that in mind, if I come back to long running, when I say long running, I don’t refer to any AI algorithm that runs for ages until I get a result. No, I’m basically simply referring to waiting. Long running for me is waiting because I have to wait for certain things, that could be human work, the human in the loop, like we just heard, because somebody has to prove something. Somebody has to decide something that are typically things, or somebody has to do something. Waiting for a response, I sent whatever inquiry to the customer, and they have to give me certain data.
They have to make their decision. They have to sign the document, whatever it is, so I have to wait for that. Both of those things are not within seconds, they can be within hours, days, or even weeks, sometimes even longer. Or I simply want to let some time pass. The pizza baking is one example, but I had a lot of other examples in the past. One of my favorites was a startup. They did a manufactured service, which was completely automated, but they wanted to make the impression to the customer that it’s like a human does it. They waited for a random time, between 10 and 50 minutes, for example, to process a response. There are also more serious examples.
Why Is Waiting a Pain?
Why is waiting a pain? It basically boils down to because we have to remember that we are waiting. It’s important to not forget about waiting. That involves persistent state. Because if I have to wait not only for seconds, but minutes, hours, days, or weeks, or a month, I have to persist it somewhere to still remember it when somebody comes back. Persistent state. Is that a problem? We have databases? We do. There are a lot of subsequent requirements, if you look at that.
For example, you have to have an understanding what you’re waiting for. You probably have to escalate if you’re waiting for too long. You have versioning problems, like if I have a process that runs for a month, and I start at like every day a couple of times, I always have processes in flux. If I want to change the process, I have to think about already running ones, and probably do something different for them than for newer ones, for example. I have to run that at scale. I want to see where I’m at, and a lot of those things.
The big question is, how do I do that? How do I solve those technical challenges without adding accidental complexity? That’s what I’m seeing, actually, quite often. I wrote a blog post, I think, more than 10 years ago, where I said, I don’t want to see any more homegrown workflow engines. Because people stumble into that, like we simply have to write a status flag in the database. Then we wait, that’s fine. Then they start, “We have to remember that we have to have a scheduler. We have to have an escalation. People want to see that.” They start adding stuff. That’s not a good idea to do.
Background
I’m working on workflow engines, process engines, orchestration engines, however you want to call them, for almost all my life, at least my professional life. I co-founded Camunda, a process orchestration company, and wrote a lot of things in the past about it. I’ve worked on a couple of different open source workflow engines as well in the past.
Workflow Engine (Customer Onboarding)
One of the components that can solve these long running issues is a workflow engine. We’re currently more going towards naming it an orchestration engine, some call it a process engine. It’s all fine. The idea is that you define workflows, which you can run instances off, and then you have all these requirements being settled. I wanted to give you a 2-minutes demo, not because I want to show the tool, that’s a nice side effect. There are other tools doing the same thing. I want to get everybody to the same page of, what is that? What’s a workflow engine? If you want to play around with that yourself, there’s a link.
It’s all on GitHub, so you can just run it yourself. What I use as an example is an onboarding process. We see that in basically every company to some extent. You want to open up a new bank account, you go through an onboarding process, as a bank. You want to have a new mobile phone contract, you go through onboarding. If you want to have new insurance contract, onboarding. It’s always the same. This is how it could look like. What I’m using here, it’s called BPMN, it’s an ISO standard, how to define those processes.
You do that graphically. In the background, it’s simply an XML file basically describing that. It’s standardized, ISO standard. That’s not a proprietary thing here. Then you can do things like, I score the customer, then I approve the order. That’s a manual thing. I always like adding things live with the risk of breaking down. We could say, that takes too long. We want to basically escalate that. Let’s just say, escalate. Yes, we keep it like that. We have to say what too long is. That’s a duration with a period, time, 10 seconds should be enough for a person to review it. I just save that.
What I have in the background is a Java application, in this case. It doesn’t have to be Java, but I’m a Java person. It’s a Java Spring Boot application basically that connects to the workflow engine, in this case also deploys the process. Then also provides a small web UI. I can open a new bank account. I don’t even have to type in data because it does know everything. I submit the application. It triggers a REST call basically. The REST call goes into the Spring Boot application. That kicks off a process instance within the workflow engine. I’m using our SaaS service, so you have tools like Operate, where it can look into what’s going on.
There it can see that I have processes running. You see the versioning. I have a new version. I have that instance running. If I kick off another one, I get a second one in a second. I’m currently waiting for approval. I also already have escalated it, at the same time. Then you have tasks list, because I’m now waiting for a human, for example. I have UI stuff. I could also do that via chatbot or teams’ integration, for example. Yes, to automatic processing, please. Complete the task. Then this moves on. I’m seeing that here as well. I’m seeing that this moves on, and also sends an email. I have that one.
Process instance finish, for example. It runs through a couple of steps. Those couple of steps then basically connect to either the last two things I want to show, for example, create customer in CRM system, is, in this case, tied to a bit of Java code where it can do whatever you want to. That’s custom glue code, you simply can program it. Or if you want to send a welcome email, you already see that. That’s a pre-built connector. For example, for SendGrid, I can simply configure. That means in the background, also, my email was sent, which I can also show you hopefully here. Proof done, “Hello, QCon,” in email. We’re good.
That’s a workflow engine running in the background. We are having a workflow model. We have instances running through. We have code attached, or UIs attached to either connect to systems or to the human. Technically, I was using Camunda as a SaaS service here, and I had a Spring Boot application. Sometimes I’m being asked, ok, workflow, isn’t that for these like, I do 10 approvals a day things? No. We’re having customers running that at a huge scale. There’s a link for a blog post where we go into the thousands of process instances per second.
We run that in geographically distributed data centers in the U.S. and UK, for example, and this adds latency, but it doesn’t bring throughput down, for example. We are also working to reduce the latency of certain steps. What I’m trying to say is that that’s not only for I run five workflows a day, you can run that at a huge scale for core things.
When Do Services Need to Wait? (Technical Reasons)
So far, I looked at some business reasons why we want to wait. There are also a lot of technical reasons why you want to wait for things, why things get long running. That could be, first of all, asynchronous communication. If you send a message, you might not know when you get a message back. It might be within seconds in the happy case or milliseconds. What if not, then you have to do something. If you have a failure scenario, you don’t get a message back, you want to probably just stop where you are, and then wait for it to happen.
Then probably you can also notify an operator to resolve that. Or the peer service is not available, so especially if you go into microservices, or generally distributed systems, the peer might not be available, so you probably have to do something about it. You have to wait for that peer to become available. That’s a problem you should solve. Because otherwise, yes, you get to that. You get chain reactions, basically.
The example I always like to use is this one. If you use an airplane, you get an email invitation to check in a day before, 24 hours before that normally. Then you click a link, and you should check in. I did that for a flight actually to London. I think that was pre-pandemic, 2019, or something like that. I flew to London with Eurowings. I wanted to check in, and what it said to me was, “There was an error while sending you your boarding pass.” I couldn’t check in. That’s it. What would you do? Try it again. Yes, of course. I try it again. That’s what I did. Didn’t work. I tried it again 5 minutes later, didn’t work.
What was the next thing I did? I made a calendar entry in my Outlook, to remind me of trying it again in a couple of hours. Because there was still time. It wasn’t the next day. I just wanted to make sure not to forget to check in. That’s what I call a stateful retry. I want to retry but in a long running form, like 4 hours from now because it actually doesn’t work. It doesn’t matter because I don’t need it yet now.
The situation I envision is that, in the background, they had their web interface, they probably had a check-in microservice. They probably had some components downstream required for that to work, for example, the barcode generation, or document output management, or whatever. One of those components did fail. The barcode generation, for example, didn’t work, so they couldn’t check me in. The thing is that the more we distribute our systems into a lot of smaller services, the more we have to accept that certain parts are always broken, or that network to certain parts are always broken.
That’s the whole resiliency thing we’re discussing about. The only thing that we have to make sure, which is really important, that it doesn’t bring down our whole system. In other words, just that the 3D barcode generation, which is probably needed for my PDF boarding pass, I need to print out later, is not working, shouldn’t prevent my check-in. That’s a bad design. That’s not resilient. Because then you get a chain reaction here. The barcode generation is not working, probably not a big deal. It gets to a big deal because nobody can check in anymore. They make it my problem.
They transport the error all the way up to me, for me to resolve because I’m the last one in the chain. Everybody throws the hot potato ones further, I’m the last part in the chain as a user. That makes me responsible for the Outlook entry. The funny part about that story was really, the onwards flight, same trip from London, easyJet, “We are sorry.” Same problem, I couldn’t check in, but they give you the work instruction. They are better with that. “We’re having some technical difficulties, log on again, retry. If that doesn’t work, please try again in 5 minutes.” I like that, increase the interval. That makes a lot of sense. You could automate that probably.
The next thing, and I love that, “We do actively monitor our site. We’ll be working to resolve the issue. There’s no need to call.” It’s your problem, leave us alone. In this case, it’s very obvious because it’s facing the user. It’s an attitude I’m seeing in a lot of organizations, even internally to other services, their problem, which is, throw an error, we’re good.
The much better situation would be the check-in should probably handle that. They should check me in. They could say, you’re checked in, but we can’t issue the boarding pass right now, we’re sorry, but we send it on time. Or, you get it in the app anyway. I don’t want to print it out, don’t need a PDF. They could handle it in a much more local scope. That’s a better design. It gives you a better designed system. The responsibilities are much cleaner defined, but the thing is now you need long running capabilities within the check-in service. If you don’t have them, that’s why a lot of teams are rethrowing the error.
Otherwise, we have to keep state, we want to be stateless. That’s the other part, which I was discussing with a lot of customers over the last 5 years. The customer wants a synchronous response. They want to see a response in the website where it says you’re checked in, here’s your boarding pass, here’s the PDF, and whatever. We need that. People are used to that experience. I wouldn’t say so. If my decision as the customer is either I get a synchronous error message and have to retry myself or I get some result later on. I know what I’d pick. It’s still a better customer experience. It needs a little bit of rethink, but I find it important.
Let’s extend the example a little bit and add a bit more flavor on a couple of those things. Let’s say you’re still doing flight bookings, but maybe you also want to collect payments for it. That would make sense as a company. The payments might need credit card handling, so they want to take money from the credit card. Let’s look at that. The same thing could happen. You want to charge the credit card. The credit card service at least internally but maybe also on that level will use some SaaS whatever service in the internet. You will probably not do credit card handling yourself unless you’re very big, but normally, you use some Stripe-like mechanism to do that.
You will call an external API, REST, typically, to make the credit card charge. Then you have that availability thing. That service might not be available when you want to charge a credit card. You probably also then have the same thing, you want to charge it and want to probably wait for availability of the credit card service, because you don’t want to tell your customers, we can’t book your flight because our credit card service is currently not available. You probably want to find other ways. That’s not where it stops. It normally then goes beyond that, which is very interesting if you look into all the corner cases.
Let’s say you give up after some time, which makes sense. You don’t want to try to book the flight for tomorrow, for the next 48 hours. It does make sense. You give up at some point in time. You probably say the payment failed, and we probably can’t book your flight, or whatever it is that you do. There’s one interesting thing about distributed systems, if you do a remote call, and you get an exception out of that, you can’t differentiate those three situations. Probably the network was broken, you have not reached the service provider.
Maybe the network was great but the service provider, the thread exploded while you were doing it. It didn’t process it. Did it commit its transaction or not? You have no idea. Or everything worked fine and the response got lost in the network. You can’t know what just happened. That makes it hard in that scenario, because even if you get an exception, you might have charged the credit card, actually.
It might be a corner case, but it’s possible. Depending on what you do, you might not want to ignore it. Maybe you can. If that’s a conscious decision, that’s fine. Maybe you can’t, then you have to do something about that. You also can do that in a workflow way. You could also run monthly conciliation jobs, probably also a good solution. It always depends. If you want to do it in a workflow way, you might even have to check if it was charged and refunded, so it gets more complicated. That’s what I’m trying to say.
In order to do these kinds of things, again, embrace asynchronous thinking. Make an API that’s ready to probably not deliver a synchronous result. That’s saying, we try our best, maybe you get something in a good case, but maybe you don’t. Then, that’s HTTP codes. I like to think in HTTP codes, like 202 means we got your request, that’s the feedback loop, we got it, but the result will be later. Now you can make it long running, and that extends your options, what it can do. Speaking of that, one of the core thoughts there is also, if you make APIs like that, make it asynchronous, make it be able to handle long running.
Within your services, you’re more free to implement requirements the way you want. Let’s say you extend the whole payment thing, not only to credit cards, but probably to also have customer credits on their account. Some companies allow that. If you return goods, for example, you get credits on your account, which you can use for other things, or PayPal has that. If you get money sent via PayPal, it’s on your PayPal account, you can use that first before they deduct it from your bank account, for example. Then you could add that where you say, I first deduct credit and then I charge the credit card, and you get more options of doing that also long running. That poses interesting new problems around really consistency. For example, now we have a situation where we talk to different services, probably for credit handling, or for credit card charging.
All of them have their transactions internally, probably, but you don’t have a technical transaction spawning all of those steps. Where you say, if the credit card charging fails, I also didn’t deduct the customer credit, I just say payment failed. I need to think about these scenarios where a deducted customer credit card charge doesn’t work. I want to fail the payment. Then I have to basically rebook the customer credit. That’s, for example, also what you can do with these kinds of workflows. That’s called compensation. Where you say, I have compensating, like undo activities for activities if something failed. The only thing I’m trying to say here is, it gets more complex very quickly if you think about all the implications of distributed systems here.
Long Running Capabilities (Example)
Going back to the long running capabilities. My view on that is, you need long running capabilities to design good services, good service boundaries. That’s a technical capability you should have in your architecture. I made another example to probably also make it easier to grasp. Let’s say the booking service basically tells the payment service via method via REST call, saying, retrieve payment. I won’t discuss orchestration versus choreography, because that could be something you’re also interested in. Why doesn’t it just emit an event? Booking says, payment, retrieve payment for that flight, for example. Payment chose the credit card. Now let’s say the credit card is rejected. Service is available, but the credit card is rejected. That very often happens in scenarios where I store the credit card in my profile, it’s expired, and then it gets rejected.
Now the next question is what to do with that. Typically, a requirement could be, if the credit card is rejected, the customer can provide new details. They hopefully still book their flight. We want them to do that. They need to provide new credit card details. You can also think about other scenarios. Somewhere I have the example of GitHub subscriptions, because there, it’s a fully automated process that renews my subscription, uses my credit card. It doesn’t work, they send you an email, “Update your credit card.”
The question is where to implement that requirement. One of the typical reactions I’m seeing in a lot of scenarios is that, as a payment, we’re stateless again. We want to be simple. We can’t do that, because then we have to send the customer an email. We have to wait for the customer to update the credit card details. We have to control that whole process. It gets long running.
They understand it adds complexity, they don’t want to do that. Just hot potato forward to the booking, because the booking is long running anyway, for a couple of reasons. They also have that. They can handle that requirement better, so let’s just throw it over the fence over there. I’m seeing that very often, actually. If you make the same example with order fulfillment, or other things where it’s very clear that that component, like booking, order fulfillment has a workflow mechanism, then this happens. The problem is now you’re leaking a lot of domain concepts, out of payment into booking, because booking shouldn’t know about credit card at all. They want to get money. They want to have the payment. They shouldn’t care about the way of payment. Because that probably also changes over time, and you don’t always want to adjust the booking, just because there’s a new payment method.
It’s a better design to separate that. That’s questionable. If you go into DDD, for example, it also leaks domain language, like, credit card rejected. I don’t care, I wanted to retrieve payment. Either you got my payment or you didn’t. That’s the two results I care about as booking. You want to really put it into the payment service. That makes more sense. Then, get a proper response, like the final thing. In order to do that, you have to deal with long running requirements within payment. That’s the thing. You should make that easy for the teams to do that.
I added potentially on the slide. In such a situation, payment in 99% of the cases might be really super-fast, and could be synchronous. Then there are all these edge cases where it might not be and it’s good to be able to handle that. Then you can still design, for example, an API versus say, in the happy case I get a synchronous result. It’s not an exceptional case. It’s just one case. The other case could be, I don’t get that. I get an HTTP 202, and an asynchronous response. Make your architecture ready for that. Then you could use probably also workflows for implementing that.
Just because there’s a workflow orchestration doesn’t mean it’s a monolithic thing. I would even say, the other way round, if you have long running capabilities available in the different services you might want to do, it gets easier to put the right parts of the process in the right microservices, for example, and it’s not monolithic at all. It gets monolithic if, for example, payment doesn’t have long running capabilities, and you move that logic into the booking service, just because that booking service has the possibility to do long running. I find that important. It’s not that having orchestration, or long running capabilities adds the monolithic thing. It’s the other way round, because not all the services have them at their disposal. Normally, what they do is they push all the long running stuff towards that one service that does, and then this gets monolithic. From my perspective, having long running at the disposal for everybody avoids these, what Sam Newman once called, god services.
Successful Process Orchestration (Centers of Excellence)
Long running capabilities are essential. It makes it easier to distribute all the responsibilities correctly. Also, it makes it easier to embrace asynchronous, non-blocking stuff. You need a process orchestration capability. That’s what I’m convinced of. Otherwise, probably, I wouldn’t do it for all my life. That’s also easy to get as a team. Nowadays, that means as a service, either internally or probably also externally, to create a good architecture. I’m really convinced by that. Looking into that, how can I do that? How can I get that into the organization better? What we’re seeing very successful, all organizations I talk with that use process orchestration to a bigger extent, very successfully, they have some Center of Excellence, organizationally. They not always call it Center of Excellence. Sometimes it’s a digital enabler, or even process ninjas. It might be named very differently. That depends a little bit on company culture and things.
It’s a dedicated team within the organization that cares about long running, if you phrase it more technically, or process orchestration, process automation, these kinds of things. This is the link, https://camunda.com/process-orchestration/automation-center-of-excellence/, for a 40-page article where we collected a lot of the information about Center of Excellence: how to build them, what are best practices to design them, and so on. One of the core ideas there is, a Center of Excellence should focus on enablement, and probably providing a platform.
They should not create solutions. Because sometimes people ask me, but we did that BPM, where we had these central teams doing an ESB and very complicated technology and didn’t work. It didn’t work, because at that time, a lot of those central teams had to be involved in the solution creation. They had to build workflows. It was not possible without them. That’s a very different model nowadays. You normally have a central team that focuses on enabling others that then build the things. Enabling means probably consulting, helping them, building a community, but also providing technology where they can do that.
What I’m discussing very often within the last two or three years is, but we stopped doing central things. We want to be more autonomous. We have the teams, they should be free in their decisions. We don’t want to put too much guardrails on them. Isn’t a central CoE the path? Why do you do that? I discuss that with a lot of organizations actually. I was so happy about the Team Topologies book. That’s definitely a recommendation to look into. The core ideas are very crisp, actually. In order to be very efficient in your development, you have different types of teams. That’s the stream-aligned team that does business logic, that implements business logic, basically. They provide value. That’s very often also value streams and whatever. You want to make them as productive as possible to remove as much friction as possible so they can really provide value, provide features. In order to do that you have other types of teams.
The two important ones are the enabling team, a consulting function, like hopping through the different projects, and the platform team, providing all the technology they need, so they don’t have to figure out everything themselves. The complicated subsystem team is something we don’t focus on too much. It can be some fraud check AI thing somebody does, and then provides an internal as a service thing. You can map that very well. Our customers do that actually very well to having a Center of Excellence around process orchestration, automation, for example.
Where you say they provide the technology. In our case, that’s very often Camunda, but it could be something else. Very often, they also own adjacent tools like RPA tools, robotic process automation, and others. They provide the technology and also the enablement: project templates, and whatnot. That’s very efficient, actually. It frees the teams of figuring out that themselves, because that’s so hard. As a team, if you don’t have an idea how you build your stack, you can go into evaluation mode for two or three months, and you don’t deliver any business value there. That’s actually not new. There are a couple of recommendable blog posts out there also talking about that. One is the thing from Spotify. Spotify published about Golden Path, 2020, where they basically said, we want to have certain defined ways of building a certain solution type. If we build a customer facing web app, this is normally how we do it.
If we build a long running workflow, this is how we do it. They have these kinds of solution templates. The name is good, actually, they name it Golden Path, because it’s golden. They make it so easy to be used. They don’t force teams to use it. That’s the autonomy thing. They don’t force it upon people. They make it desirable to be used. They make it easy. It’s not your fault if it’s not working. Then it’s golden. I like the blog post, actually, I love that quote, because they found that rumor-driven development simply wasn’t scalable. “I heard they do it like that, probably you should do that as well.” Then you end up with quite a slew of technology that doesn’t work. I find this really important that you want to consolidate on certain technologies. You want to make it easy to use them across the whole organization. That makes you efficient. Don’t force it upon the people.
They also have a tool. That’s a big company, they do open source on the side. They made backstage.io. I have no idea if the tool is good. I have not used it at all. I love the starting page of their website, The Speed Paradox, where they said, “At Spotify, we’ve always believed in the speed and ingenuity that comes from having autonomous development teams, but as we learn firsthand, the faster you grow, the more fragmented and complex your software ecosystems become, and then everything slows down again.” The Standards Paradox, “By centralizing services and standardizing your tooling, Backstage streamlines your development environment.
Instead of restricting autonomy, standardization frees your engineers from infrastructure complexity.” I think that’s an important thought. They’re not alone. If you search the internet, you find a couple of other places, for example, Twilio, but also others. Same thing. We’re offering paved path, mature services, pull off the shelf, get up and running super quickly. What you do is create the incentive structure for teams to take the paved path, because it’s a lot easier. If they really have to go a different route, you make it possible. It’s not restricting autonomy, simply helping them. That’s important. I think it’s also important to discuss that internally.
Graphical Models
Last thing, graphical models. That’s the other thing I discuss regularly. Center of Excellence, yes, probably makes sense. Process orchestration, yes, I understand why we have to do that. Graphical models? We’re developers. We write code. Thing is, BPMN, that’s what I showed. It’s an ISO standard. It’s worldwide adopted. It can do pretty complex things. I just scratched the surface. It can express a lot of complex things in relatively simple model, so it’s powerful. It’s living documentation. It’s not a picture that’s requirement, but it’s running code. That’s the model you put into production. It’s running code. That’s so powerful.
This is an example where it’s used for test cases. That’s what the test case tests, for example. You can leverage that as a visual. Or it can use it in operations like, where is it stuck, or what is the typical way it’s going through, or where are typical bottlenecks, and so on? You can use that to discuss that also with different kinds of stakeholders, not only developers, but all of them.
If you discuss a complex algorithm, like a longer process or workflow, you normally go to the whiteboard and sketch it because we’re visual as a human. Just because I’m a programmer doesn’t make me less visual. I want to see it. Very powerful. It’s even more important, because I think a lot of the decisions about long running behavior needs to be elevated to the business level.
They need to understand, why we want to get asynchronous. Why this might take longer. Why we need to change, also customer experience to leverage the architecture. The only way of doing that is to really make it transparent, to make it visual. I think it was a former marketing colleague that worked with me, phrased it like that. What you’re trying to say is that in order to leverage your hipster architecture, you need to redesign the customer journey. That’s exactly that. That’s important to keep in mind.
Example (Customer Experience)
I want to quickly close that with another flight story. The first thing it’s happening, so you get everything asynchronous. They did change the customer experience a lot. Now I’m working on train companies. That’s the same thing. Mobile. You get automatically checked in for flights. You don’t even have to do that. Why should I do that? My flight to London was delayed by an hour. Ok, that’s delayed. That was canceled. That’s not so nice. Then I got a relatively quickly and automated email, that’s the only one in German, which I don’t get why. Did I get that one in German? It wasn’t German.
I got the link to book my hotel at Frankfurt airport. Why? I don’t want to get a hotel in Frankfurt, I want to get to London. Everything automated, everything pushed. Nice. Then I got, via the app not via email, a link to a chatbot where I should chat about my flight. It says, we rebooked you for tomorrow morning. It didn’t do that completely because it’s not Lufthansa, so you have to see a human colleague. I don’t want to get to London tomorrow, I want to get there today. I basically visit a counter.
The end of the story is they could rebook me to a very late flight to London, Heathrow, which was very late. I hated that. What I still like, everything was asynchronously. I got notification of everything in the app via email. I think there’s some good things on the horizon there. The customer experience for airlines at least changed quite a bit over the last 5 years. Funny enough, last anecdote, I read an article about the bad NPS score of Lufthansa, and I probably understand why.
Recap
You need long running capabilities for a lot of reasons. Process orchestration platforms, workflow engines, great technology. You should definitely use that for those, because it allows you to design better service boundaries, implement quicker, less accidental complexity. You can embrace asynchronicity better. Provide a better customer experience. We haven’t even talked about the other stuff like increased operational efficiency, automation, reduce risk, be more compliant, document the process, and so on. In order to do that successfully across the organization, you should organize some central enablement. I’m a big advocate for that, to really adopt that at scale.
See more presentations with transcripts
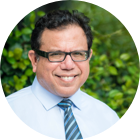
MMS • Anthony Alford
Article originally posted on InfoQ. Visit InfoQ
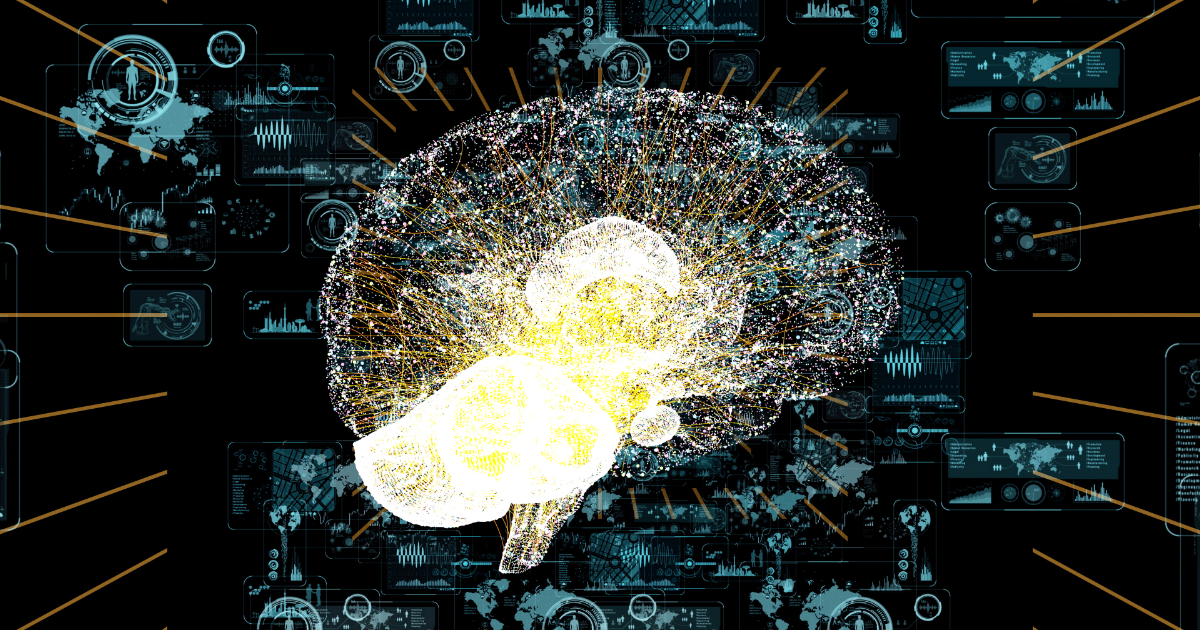
Researchers at Apple and the Swiss Federal Institute of Technology Lausanne (EPFL) have open-sourced 4M-21, a single any-to-any AI model that can handle 21 input and output modalities. 4M-21 performs well “out of the box” on several vision benchmarks and is available under the Apache 2.0 license.
4M-21 is a 3B-parameter Transformer-based encoder-decoder model. All 21 input modalities are mapped to discrete tokens using modality-specific tokenizers, and the model can generate any output modality given any input modality. The model was trained on around 500 million samples of multimodal data, including COYO and C4. Out of the box, 4M-21 can perform a wide range of tasks, including steerable image generation and image retrieval. On vision benchmarks including semantic segmentation and depth estimation, it outperformed comparable baseline models. According to Apple:
The resulting model demonstrates the possibility of training a single model on a large number of diverse modalities/tasks without any degradation in performance and significantly expands the out-of- the-box capabilities compared to existing models. Adding all these modalities enables new potential for multimodal interaction, such as retrieval from and across multiple modalities, or highly steerable generation of any of the training modalities, all by a single model.
4M-21 builds on Apple’s earlier model, Massively Multimodal Masked Modeling (4M), which handled only seven modalities. The new model triples the modalities, which include text and pixel data, as well as “multiple types of image, semantic and geometric metadata.” Each modality has a dedicated tokenizer; text modalities use a WordPiece tokenizer, while image modalities use variational auto-encoders (VAE). The model is trained using a single objective: “a per-token classification problem using the cross-entropy loss.”
By allowing inputs with multiple modalities and chaining operations, 4M-21 supports fine-grained image editing and generation. For example, providing a text caption input will prompt the model to generate the described image. Users can control details about the generated image by including geometric input such as bounding boxes, segmentation maps, or human poses along with the caption. The model can also perform image retrieval based on different inputs; for example, by finding images given a caption or a semantic segmentation map.
Research team member Amir Zamir posted about the work in a thread on X. One user asked Zamir why the model does not support audio modalities. Zamir replied that “It’s a matter of data,” and suggested their method should work with audio. He also wrote:
IMO, the multitask learning aspect of multimodal models has really taken a step forward. We can train a single model on many diverse tasks with ~SOTA accuracy. But a long way to go in terms of transfer/emergence.
Andrew Ng’s AI newsletter The Batch also covered 4M-21, saying:
The limits of this capability aren’t clear, but it opens the door to fine control over the model’s output. The authors explain how they extracted the various modalities; presumably users can do the same to prompt the model for the output they desire. For instance, a user could request an image by entering not only a prompt but also a color palette, edges, depth map extracted from another image, and receive output that integrates those elements.
The code and model weights for 4M-21 are available on GitHub.
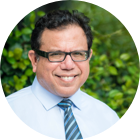
MMS • RSS
Posted on mongodb google news. Visit mongodb google news
We recently compiled a list titled Jim Cramer’s Top 10 Stocks to Track for Potential Growth. In this article, we will look at where MongoDB, Inc. (NASDAQ:MDB) ranks among Jim Cramer’s top stocks to track for potential growth.
In a recent episode of Mad Money, Jim Cramer points out the surprising strength in the market, noting that many companies are performing better than Wall Street recognizes. He argues that people should stop doubting these companies every time there’s a negative data point. Cramer highlights the impressive management and execution by CEOs, which often goes unnoticed.
“Suddenly, all is forgiven, or if not all, then at least most. I’m talking about the incredible resilience in this market, buoyed by a recognition that many companies are simply better than Wall Street gives them credit for. We need to stop turning against them every time there’s a seemingly bad data point. Every day I come to work, I’m dazzled by the resourcefulness of executives who do their best to create value for you, the shareholder. Lots of stocks went up on days like today when the Dow advanced 335 points, the S&P gained 75%, and the NASDAQ jumped 1.0%, all thanks to good management and excellent execution that often goes unnoticed.”
While Cramer acknowledges that some CEOs deserve skepticism, he emphasizes that many are outstanding and deserve recognition for their hard work. He criticizes the focus on short-term economic indicators and emphasizes that great companies aren’t distracted by minor fluctuations.
“Listen, I’m not a pushover. I can hit CEOs with tough questions when needed, some of them deserve skepticism and scorn. But there are also plenty of brilliant, hardworking CEOs with incredible teams, and you ignore their hustle at your own peril. This often gets lost in the shuffle when we’re focused on the parlor game of guessing the Fed’s next move—a quarter point, half a point, quarter, half. You know what I say? Let’s get serious. Terrific companies don’t get caught up in that quarter-half shuffle.”
Cramer explains how Kroger CEO Rodney McMullen has led the supermarket chain to success despite challenges, including resistance to its acquisition of Albertsons and a tough economic environment. McMullen has managed to keep food costs down and deliver strong results through effective strategies like a superior loyalty program and regional store improvements. Despite high food prices, the company’s stock rose more than 7% following a positive earnings report, showcasing the company’s successful turnaround.
“CEO Rodney McMullen has managed to keep food costs down and deliver fantastic numbers, all while maintaining an expensive, unionized labor force in a very uncertain commodity environment. How? The company confounded critics by developing a superior loyalty program, regionalizing their stores, and creating some of the best private-label products out there, second only to Costco. Food is still expensive, but cooking at home is far cheaper than dining out. McMullen tells us that consumers are no longer flush with cash, especially his most budget-conscious clientele. He notes, “Budget-conscious customers are buying more at the beginning of the month to stock up on essentials, and as the month progresses, they become more cautious with their spending.”
Wow, that’s a tough environment. When I heard this, I thought back to the old company, the one that used to miss its numbers whenever the environment got a little tough. Everybody else remembers the old company too, which is why the stock was just sitting there waiting to be picked up, until this quarter’s report, after which it soared more than 7% in response to the fabulous results. Everyone thought the company would drop the ball, as they used to, but McMullen has finally whipped his supermarket into shape.”
Cramer contrasts this with the tech industry, where complex details often lead Wall Street to misunderstand a company’s true potential. He believes that in tech, analysts frequently overlook the expertise and capabilities of CEOs who have a deep understanding of their businesses.
“We all need to eat, so it’s not hard to understand the grocery business. But it’s quite different when it comes to tech, where analysts constantly doubt the resolve and expertise of CEOs who simply know more about their businesses than the critics. In tech, the complexity often leads Wall Street to conclusions that have little to do with reality.”
Our Methodology
This article reviews a recent episode of Jim Cramer’s Mad Money, where he discussed several stocks. We selected and analyzed ten companies from that episode and ranked them by the level of hedge fund ownership, from the least to the most owned.
At Insider Monkey we are obsessed with the stocks that hedge funds pile into. The reason is simple: our research has shown that we can outperform the market by imitating the top stock picks of the best hedge funds. Our quarterly newsletter’s strategy selects 14 small-cap and large-cap stocks every quarter and has returned 275% since May 2014, beating its benchmark by 150 percentage points (see more details here).
A software engineer hosting a remote video training session on a multi-cloud database-as-a-service solution.
MongoDB Inc.(NASDAQ:MDB)
Number of Hedge Fund Investors: 54
Jim Cramer believes MongoDB, Inc. (NASDAQ:MDB) is an enterprise software company delivering excellent results, but it isn’t receiving the same level of recognition as competitors like Salesforce.com (NYSE:CRM). He notes that investors generally seem to shy away from enterprise software companies, with the exception of Salesforce.com (NYSE:CRM). However, Cramer feels that MongoDB, Inc. (NASDAQ:MDB) is currently at a good price, suggesting it may be undervalued despite its strong performance. Cramer sees potential in MongoDB, Inc. (NASDAQ:MDB) and implies it deserves more attention in the enterprise software space.
“You know, MongoDB, Inc.(NASDAQ:MDB) is an enterprise software company that put up terrific numbers and isn’t getting credit in the same way Salesforce.com, inc. (NYSE:CRM) and others are. People tend to dislike enterprise software, except for ServiceNow. I think MongoDB, Inc.(NASDAQ:MDB) is at the right price.”
MongoDB, Inc. (NASDAQ:MDB) offers a strong case for long-term growth, driven by its outstanding financial performance and strategic advancements. In Q2 2024, MongoDB, Inc. (NASDAQ:MDB) reported a 40% jump in revenue, reaching $423.8 million, with its cloud-based Atlas platform accounting for 65% of total revenue. This growth exceeded market expectations and demonstrates the growing demand for its flexible database solutions. MongoDB, Inc. (NASDAQ:MDB) also turned its operating loss from the previous year into a profit of $53.6 million, reflecting its ability to grow while controlling costs.
Analysts are optimistic about MongoDB, Inc. (NASDAQ:MDB), with KeyBanc raising its price target to $543, citing MongoDB’s dominant position in the NoSQL database market and its potential to capitalize on rising demand from cloud and AI-driven applications. MongoDB, Inc. (NASDAQ:MDB)’s educational initiatives, such as partnering with India’s Ministry of Education to train 500,000 students, further strengthen its developer community and support future growth.
ClearBridge All Cap Growth Strategy stated the following regarding MongoDB, Inc. (NASDAQ:MDB) in its first quarter 2024 investor letter:
“During the first quarter, we initiated a new position in MongoDB, Inc. (NASDAQ:MDB), in the IT sector. The company offers a leading modern database platform that handles all data types and is geared toward modern Internet applications, which constitute the bulk of new workloads. Database is one of the largest and fastest-growing software segments, and we believe it is early innings in the company’s ability to penetrate this market. MongoDB is actively expanding its potential market by adding ancillary capabilities like vector search for AI applications, streaming and real-time data analytics. The company reached non-GAAP profitability in 2022, and we see significant room for improved margins as revenue scales.”
Overall MDB ranks 6th on the list of Jim Cramer’s top stocks to track for potential growth. While we acknowledge the potential of MDB as an investment, our conviction lies in the belief that under the radar AI stocks hold greater promise for delivering higher returns, and doing so within a shorter timeframe. If you are looking for an AI stock that is more promising than MDB but that trades at less than 5 times its earnings, check out our report about the cheapest AI stock.
READ NEXT: $30 Trillion Opportunity: 15 Best Humanoid Robot Stocks to Buy According to Morgan Stanley and Jim Cramer Says NVIDIA ‘Has Become A Wasteland’.
Disclosure: None. This article was originally published on Insider Monkey.
Article originally posted on mongodb google news. Visit mongodb google news
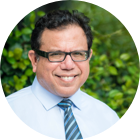
MMS • RSS
Posted on nosqlgooglealerts. Visit nosqlgooglealerts
By Ben Paul, Solutions Engineer – SingleStore
By Aman Tiwari, Solutions Architect – AWS
By Saurabh Shanbhag, Sr. Partner Solutions Architect – AWS
By Srikar Kasireddy, Database Specialist Solutions Architect – AWS
![]() |
SingleStore |
![]() |
The fast pace of business today often demands the ability to reliably handle an immense number of requests daily with millisecond response times. Such a requirement calls for a high-performance, non-schematic database like Amazon DynamoDB. DynamoDB is a serverless NoSQL database that supports key-value and
document data models that offers consistent single-digit millisecond performance at any scale. DynamoDB enables customers to offload the administrative burdens of operating and scaling distributed databases to AWS cloud so that they don’t have to worry about hardware provisioning, setup and configuration, throughput capacity planning, replication, software patching, or cluster scaling.
Perform near real-time analytics on DynamoDB data allows customers to quickly respond to changing market conditions, customer behavior, or operational trends. With near real-time data processing, you can identify patterns, detect anomalies, and make timely adjustments to your strategies or operations. This can help you stay ahead of the competition, improve customer satisfaction, and optimize your business processes.
SingleStore is an AWS Data and Analytics Competency Partner and AWS Marketplace Seller. It’s SingleStore Helios is a fully managed, cloud-native database that powers real-time workloads needing transactional and analytical capabilities.
By combining DynamoDB with SingleStore, organizations can efficiently capture, process, and analyze DynamoDB data at scale. SingleStore has high-throughput data ingestion and near-real time analytical query capability for both relational and JSON data. This integration empowers businesses to derive actionable insights from their data in near real time, enabling faster decision-making and improved operational efficiency.
SingleStore has the ability to stream Change Data Capture (CDC) data from DynamoDB and serve up fast analytics on top of its Patented Universal Storage. SingleStore has native support for JSON so DynamoDB items can be stored directly in their own JSON column. Alternatively, key-value pairs from DynamoDB can be stored as own column in SingleStore.
In this blog post we will cover two architectural patterns to integrate DynamoDB with Singlestore in order to perform near real-time analytics on your DynamoDB data –
- Using DynamoDB Stream and AWS Lambda
- Using Amazon Kinesis Data Streams connector with Amazon MSK
Using DynamoDB Stream and AWS Lambda
Figure 1 – Architecture pattern for Amazon DynamoDB CDC to SingleStore using DynamoDB Stream and AWS Lambda
The design pattern described leverages the power of DynamoDB Stream and AWS Lambda to enable near real-time data processing and integration. The following is the detail workflow for the architecture:
1. Client applications interact with DynamoDB using the DynamoDB API, performing operations such as inserting, updating, deleting, and reading items from DynamoDB tables at scale.
2. DynamoDB Stream is a feature that captures a time-ordered sequence of item-level modifications in a DynamoDB table and durably stores this information for up to 24 hours. This allows applications to access a series of stream records containing item changes in near real-time.
3. The AWS Lambda service polls the stream for new records four times per second. When new stream records are available, your Lambda function is synchronously invoked. You can subscribe up to two Lambda functions to the same DynamoDB stream.
4. Within the Lambda function, you can implement custom logic to handle the changes from the DynamoDB table, such as pushing the updates to a SingleStore table. If your Lambda function requires any additional libraries or dependencies, you can create a Lambda Layer to manage them.
5. The Lambda function needs IAM execution role with appropriate permissions to manage resources related to your DynamoDB stream.
This design pattern allows for an event-driven architecture, where changes in the DynamoDB table can trigger immediate actions and updates in other systems. It’s a common approach for building real-time data pipelines and integrating DynamoDB with other AWS services or external data stores.
This pattern is suitable for most DynamoDB customers, but is subject to throughput quotas for DynamoDB table and AWS Region. For higher throughput limit you can consider provisioned throughput or the following design pattern with Amazon Kinesis Data Streams connector.
Using Amazon Kinesis Data Streams connector with Amazon MSK
Figure 2 – Architecture pattern for Amazon DynamoDB CDC to SingleStore using Amazon Kinesis Data Streams
This design pattern leverages Amazon Kinesis Data Streams and Amazon MSK to enable more flexible data processing and integration. The following is the detail workflow for the architecture:
1. Client applications interact with DynamoDB using the DynamoDB API, performing operations such as inserting, updating, deleting, and reading items from DynamoDB tables at scale.
2. Amazon Kinesis Data Streams captures changes from DynamoDB table asynchronously. Kinesis has no performance impact on a table that it’s streaming from. You can take advantage of longer data retention time—and with enhanced fan-out capability, you can simultaneously reach two or more downstream applications. Other benefits include additional audit and security transparency.
The Kinesis data stream records might appear in a different order than when the item changes occurred. The same item notifications might also appear more than once in the stream. You can check the ApproximateCreationDateTime attribute to identify the order that the item modifications occurred in, and to identify duplicate records.
3. Using an open-source Kafka connector from the GitHub repository deployed to Amazon MSK Connect to replicate the events from Kinesis data stream to Amazon MSK. With Amazon MSK Connect, a feature of Amazon MSK, you can run fully managed Apache Kafka Connect workloads on AWS. This feature makes it easy to deploy, monitor, and automatically scale connectors that move data between Apache Kafka clusters and external systems.
4. Amazon MSK Connect needs IAM execution role with appropriate permissions to manage connectivity with Amazon Kinesis Data Streams.
5. Amazon MSK makes it easy to ingest and process streaming data in real time with fully managed Apache Kafka. Once the events are on Amazon MSK, you get the flexibility to retain or process the messages based on your business need. It gives you the flexibility to bring in various downstream Kafka consumers to process the events. SingleStore has a managed Pipelines feature, which can continuously load data using parallel ingestion as it arrives in Amazon MSK without you having to manage code.
6. SingleStore pipeline supports connection LINK feature, which provides credential management for AWS Security credentials.
This pattern gives you the flexibility to use the data change events in MSK to incorporate other workloads or have the events for longer than 24 hours.
Customer Story
ConveYour, a leading Recruitment Experience Platform, faced a challenge when Rockset, their analytical tool, was acquired by OpenAI and set for deprecation. Demonstrating remarkable agility, ConveYour swiftly transitioned to SingleStore for their analytical needs.
ConveYour CEO, Stephen Rhyne said “Faced with the impending deprecation of Rockset’s service by the end of 2024, ConveYour recognized the urgent need to transition our complex analytical workloads to a new platform. Our decision to migrate to SingleStore proved to be transformative. The performance improvements were remarkable, particularly for our most intricate queries involving extensive data sets. SingleStore’s plan cache feature significantly enhanced the speed of subsequent query executions. Furthermore, the exceptional support provided by SingleStore’s solutions team and leadership was instrumental in facilitating a swift and efficient migration process. This seamless transition not only addressed our immediate needs but also positioned us for enhanced analytical capabilities moving forward.”
Conclusion and Call to Action
In this blog, we have walked through two patterns to set up a CDC stream from DynamoDB to SingleStore. By leveraging either of these patterns, you can utilize SingleStore to serve up sub-second analytics on your DynamoDB data.
Start playing around with SingleStore today with their free trial, then chat with a SingleStore Field Engineer to get technical advice on SingleStore and/or code examples to implement the CDC pipeline from DynamoDB.
To get started with Amazon DynamoDB, please refer to the following documentation – https://docs.aws.amazon.com/amazondynamodb/latest/developerguide/GettingStartedDynamoDB.html.
.
SingleStore – AWS Partner Spotlight
SingleStore is an AWS Advanced Technology Partner and AWS Competency Partner that provides fully managed, cloud-native database that powers real-time workloads needing transactional and analytical capabilities.
Contact SingleStore | Partner Overview | AWS Marketplace
About the Authors
Ben Paul is a Solutions Engineer at SingleStore with over 6 years of experience in the data & AI field.
Aman Tiwari is a General Solutions Architect working with Worldwide Commercial Sales at AWS. He works with customers in the Digital Native Business segment and helps them design innovative, resilient, and cost-effective solutions using AWS services. He holds a master’s degree in Telecommunications Networks from Northeastern University. Outside of work, he enjoys playing lawn tennis and reading books.
Saurabh Shanbhag has over 17 years of experience in solution integration for highly complex enterprise-wide systems. With his deep expertise in AWS services, he has helped AWS Partners seamlessly integrate and optimize their product offerings, enhancing performance on AWS. His industry experience spans telecommunications, finance, and insurance, delivering innovative solutions that drive business value and operational efficiency.
Srikar Kasireddy is a Database Specialist Solutions Architect at Amazon Web Services. He works with our customers to provide architecture guidance and database solutions, helping them innovate using AWS services to improve business value.
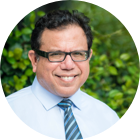
MMS • RSS
Posted on mongodb google news. Visit mongodb google news
MongoDB’s MDB short percent of float has fallen 17.84% since its last report. The company recently reported that it has 3.85 million shares sold short, which is 5.39% of all regular shares that are available for trading. Based on its trading volume, it would take traders 2.25 days to cover their short positions on average.
Why Short Interest Matters
Short interest is the number of shares that have been sold short but have not yet been covered or closed out. Short selling is when a trader sells shares of a company they do not own, with the hope that the price will fall. Traders make money from short selling if the price of the stock falls and they lose if it rises.
Short interest is important to track because it can act as an indicator of market sentiment towards a particular stock. An increase in short interest can signal that investors have become more bearish, while a decrease in short interest can signal they have become more bullish.
See Also: List of the most shorted stocks
MongoDB Short Interest Graph (3 Months)
As you can see from the chart above the percentage of shares that are sold short for MongoDB has declined since its last report. This does not mean that the stock is going to rise in the near-term but traders should be aware that less shares are being shorted.
Comparing MongoDB’s Short Interest Against Its Peers
Peer comparison is a popular technique amongst analysts and investors for gauging how well a company is performing. A company’s peer is another company that has similar characteristics to it, such as industry, size, age, and financial structure. You can find a company’s peer group by reading its 10-K, proxy filing, or by doing your own similarity analysis.
According to Benzinga Pro, MongoDB’s peer group average for short interest as a percentage of float is 6.15%, which means the company has less short interest than most of its peers.
Did you know that increasing short interest can actually be bullish for a stock? This post by Benzinga Money explains how you can profit from it.
This article was generated by Benzinga’s automated content engine and was reviewed by an editor.
Market News and Data brought to you by Benzinga APIs
© 2024 Benzinga.com. Benzinga does not provide investment advice. All rights reserved.
Article originally posted on mongodb google news. Visit mongodb google news
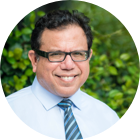
MMS • RSS
Posted on nosqlgooglealerts. Visit nosqlgooglealerts
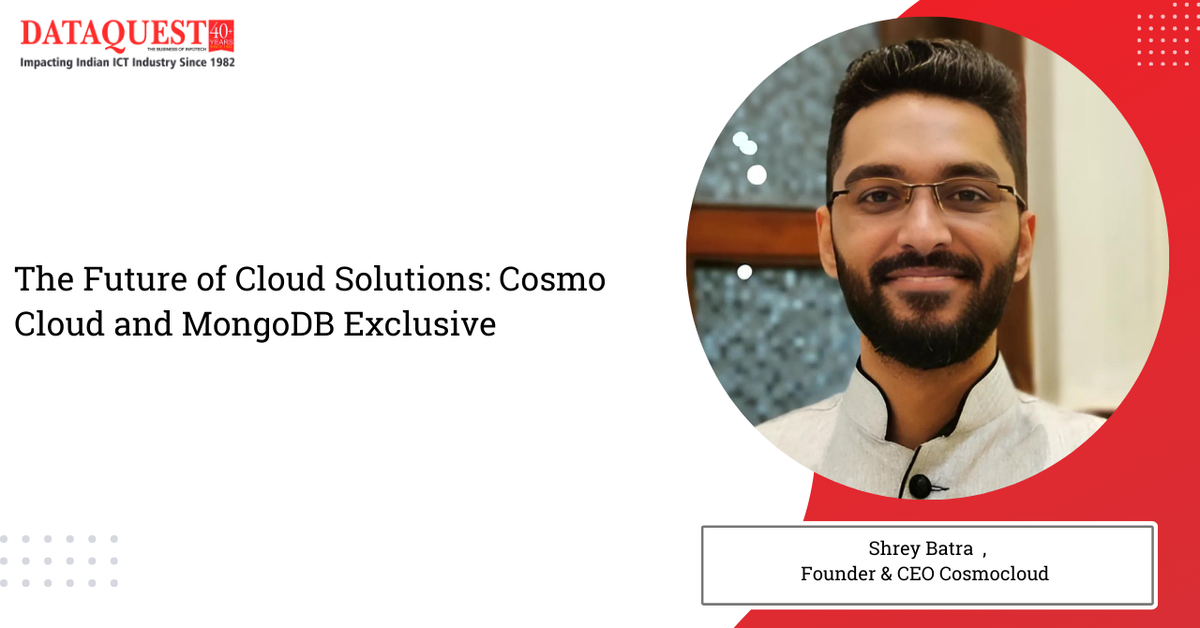
One of the top developer data platforms, MongoDB offers a scalable and adaptable data management and analytics approach. Because it is a NoSQL database, it does not follow the structure of a conventional relational database. Alternatively, MongoDB employs a document-oriented framework that gives developers a more flexible and user-friendly means of storing and retrieving data. The MongoDB for Academia in India program offers free credits to utilise MongoDB technology, certificates to assist people launch careers in the technology sector, and training for students and curriculum tools for educators.
Businesses increasingly use cloud-based solutions to improve operations in today’s thriving, upskilled digital economy. Cosmo Cloud and MongoDB have partnered strategically to provide modern businesses with a scalable cloud solution. By combining the advantages of both systems, this partnership provides companies with a reliable and adaptable data management solution. Shrey Batra is the CEO and founder of Cosmo Cloud. Dataquest got an opportunity to interact with him exclusively.
What makes Cosmo Cloud unique, and how is it different from other cloud providers? What sets you apart?
Shrey says, “So Cosmo Cloud is more than just a cloud provider. It’s a backend service platform. Now, you would have heard that there are many no-code and developer platforms. So we built we are building a no-code developer platform where the same developers can spend most of their time in solutionizing and having creative thinking and not just writing code of whatever you are trying to do, be it Java, Python or any other programming language out there, and just 20% time on creative thinking.
So Cosmo Cloud is a platform where you can build your applications back in layers, back in layers like APIs, using a drag-drop approach, create complex applications in a no-code fashion, click off a button, and get it deployed in any cloud you choose. You can select the cloud you want, such as AWS, Azure, or Google Cloud; we also have DigitalOcean.”
This disparity emphasises how industry and academics must work together more to upskill Indian educators and students to satisfy the expectations of the nation’s sizable and expanding economy. Dataquest asked Shrey Batra, CEO and founder of Cosmo Cloud, about Data safety.
How do you keep your data safe?
Shrey exclaimed, “We build applications for other enterprises and large customers as a platform. We follow the same practices as the industry leaders out there, right? So, as with Google Cloud, Azure, AWS, and other SaaS platforms, platforms, and services, providers are there. We also have our own cybersecurity practices at various levels, such as the cloud, security, networking, and application development levels. Users can add and tweak their security protocols, authentication layers, and so on.”
“We must follow everything we think about in a secure world. It has been part of our platform since day zero because, without it, the customers won’t be able to do anything. And I think that’s the best part. MongoDB’s document-oriented format enhances Cosmo Cloud’s cloud-based design, providing enterprises with a dependable data management and analysis approach.” Shrey told Dataquest.
What’s next for Cosmo Cloud? What are you planning next?
Shrey says, “We are making developers productive, right? An average developer spends about three to five days building whatever code they want to develop, especially on the backend side, right? And they do that in three to five days, then push it to their cloud production or live application, right? What we do is we make the same development cycle in 10 minutes to an hour. So that is a 10x increase in developer productivity, right? Now, while using Cosmo Cloud, you can build any kind of application that is there. So, it can be like a website, like the back end of the website, the back end of an app, Android, iOS, and so on. And what’s next for Cosmo Cloud is now we, while the platform is ready, it’s being used by enterprises; we always look for more things that can be there in the platform so that we complete the backend as a service tagline, right? Now, the backend as a service means that anything can be possible. While 90 to 95% of the things are possible on Cosmo Cloud, it’s an ever-growing journey.”
The tech is constantly evolving, the features are always coming, and no new technologies are getting integrated. He says, “This is where we come into the picture and say, okay, these are the latest tech stacks. So you are basically all prepared for the integrations and all the new things that are coming out that you forget. We release a lot of stuff repeatedly, an ever-growing cycle.”
Cosmo Cloud’s seamless integration with other cloud services enables companies to create extensive, data-driven apps. Dataquest asked about how they manage so much data. Here is what Shrey has to say.
Companies have a lot of data. So, how do you make sure that it works fast and efficiently?
“While talking to you about the no-code backend layer, I want you to understand the primary layers of a software application. The first is the front end, which is what you see: the website, the app, the design, whatever. The back end is where all your brain works. What happens when you click on the book or tab? What happens when you add some things to your cart or process a payment? All those logical things, all that automation, the brain that is there, happen on the back end of Cosmo Cloud. The third layer is the database layer, where all your data is stored. It is queried, retrieved, updated, and so on.”
He thoroughly explained Cosmo Cloud. “All that data layer is the database layer, which is a third one. The fourth is a cloud layer. Cosmo Cloud is the back end plus the back end deployable cloud. That is what Cosmo Cloud is.”
What is the role of MongoDB?
He is optimistic about the collaboration with MongoDB and says, “The best part is that we partnered with MongoDB, our data provider. Everything that the customer stores or, you know, integrates the databases you can get directly from MongoDB, especially MongoDB Atlas. So, the headache of managing the database and adding secure protocols goes out of the Cosmo Cloud scope and into the MongoDB Atlas scope. As we all know, MongoDB Atlas is already very safe. It is a very scalable platform that gives you so many things out of the box. So, all those performance things on the data and database sides are MongoDB Atlas because they are an official partner. Any performance and impact that happens on the code part or the backend part basically, that is where Cosmo Cloud comes into the picture, and that’s a drop-in replacement for your, you know, the older technologies like Java, Python, Node.js and all of those things. So, you are addressing the rapidly growing data with MongoDB. “
So basically, when we say backend as a service platform, many features are based on the data layer—for example, the database itself. For example, we need specialized search databases for those when we want to build a search engine and capabilities. We need AI to boom right now.
Shrey exclusively told Dataquest what goes behind adapting AI to your application.
How do you get AI in your application?
“We say everyone’s focusing on AI, AI, AI, but no one is focusing on how to get that AI into your or your real-time application, right? How to build that feature. A lot of the components or features come directly from MongoDB. For example, MongoDB Atlas Search or MongoDB Atlas Vector Search. These are two capabilities that come directly from MongoDB and Cosmo Cloud. Adding many other features and goodness on top of it brings a whole solution out of the box. You have a database capable of AIs and vector search. Cosmo Cloud, capable of having APIs for the search engine, builds the whole solution for you. Use it on your website or an app to make the entire search engine experience, be it a movie search, e-commerce search, or something like that, just out of the box in a couple of weeks.”
He made it sound easy, but a lot of hard work and dedication goes into creating a powerful and flexible data management solution that meets ever-evolving needs.
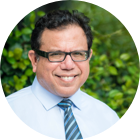
MMS • RSS
Posted on nosqlgooglealerts. Visit nosqlgooglealerts
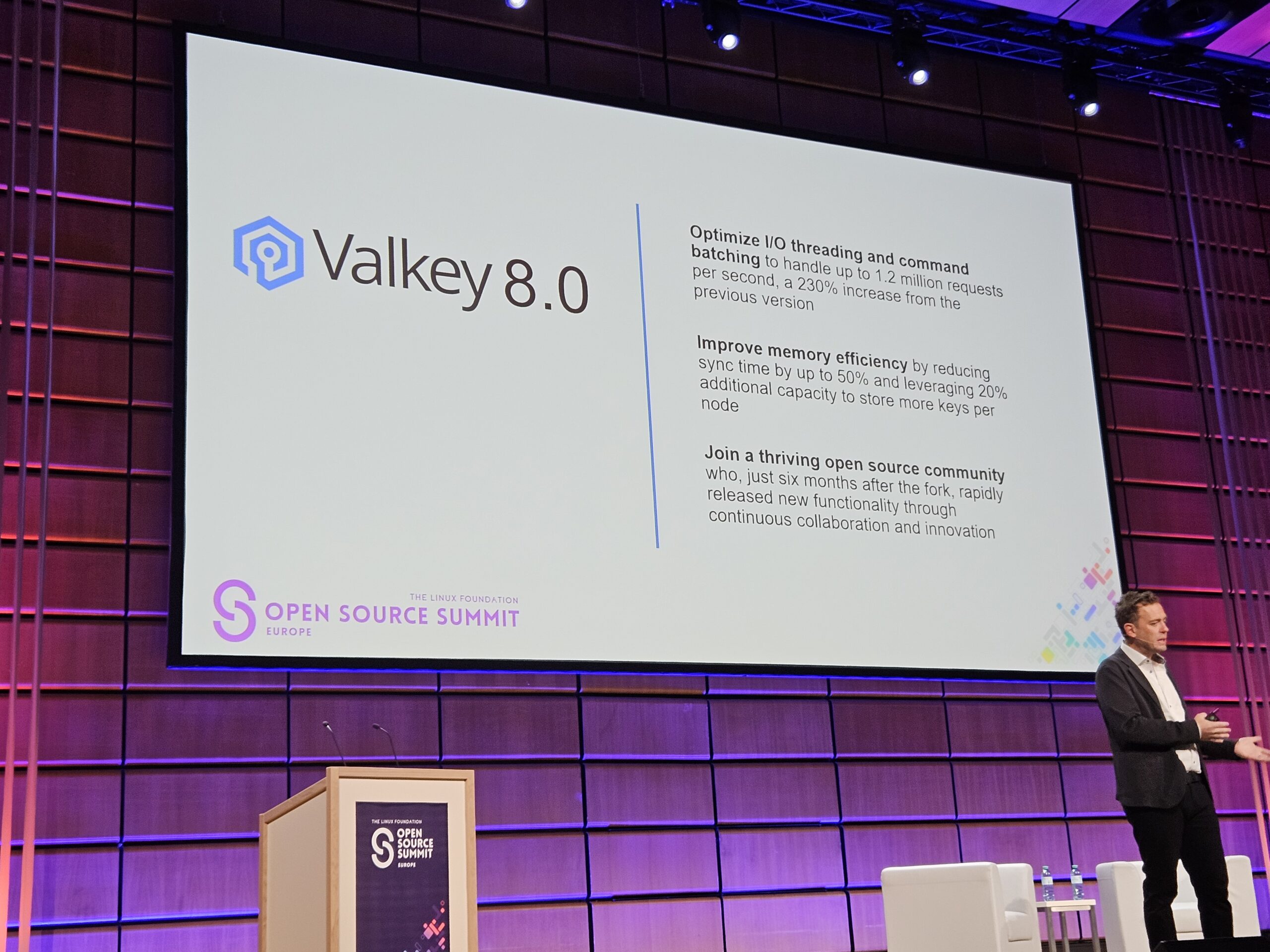
Valkey 8.0 promises across-the-board improvements in performance, reliability, and observability over previous versions and, thus, Redis as well. At the Open Source Summit in Vienna, the Linux Foundation announced the latest version of this alternative to key-value store Redis, which was forked barely six months ago.
Valkey is an open-source, in-memory NoSQL data store and aims to build on the strengths of previous versions. That makes it sound like Valkey already has a long and rich prior history. That’s true, although ‘long’ is pushing it a bit, as the previous versions have all gone live in recent months. The new version 8.0 is the first major release since being forked from Redis. The latter has had a more limited license since version 7.4.
Setting an example
Not surprisingly, Valkey 8.0’s going live was an important announcement during this week’s Open Source Summit in Vienna. By adding or improving functionality in scalability, efficient resource utilization, and system monitoring, among other things, the Linux Foundation wants to use Valkey as an example of how quickly the open-source community can shift gears.
The open-source community grumbled about the eponymous company’s change in Redis’s license. This change meant that managed service providers were being sidelined.
Tip: Flight to Valkey appears to be response to removal of Redis’ open source license
One of the most notable improvements the community has implemented is the intelligent use of multi-cores and asynchronous I/O threading. According to the Linux Foundation, this increases throughput to an impressive 1.2 million requests per second on AWS r7g instances running on AWS’ Graviton3 processors.
The example using AWS is telling, as this company has contributed quite a bit to Valkey. Indeed, one AWS employee is on the technical committee, having previously done the same for Redis. It is not only AWS that has thrown its weight behind Valkey; Google is currently courting the community for the right to install its Vector Search, just like AWS, which wants to do the same. Both companies are eager to add this functionality in light of its use for AI applications. According to an AWS spokesperson, this shows the ‘pent up’ desire of companies to add their contributions.
Mitigating data loss
In addition, Valkey 8.0 improves cluster scaling with automatic failover. This means the database automatically creates new shards (subsets of data) and distributes them to additional nodes without manual intervention. If a node fails, the system automatically activates failover, so the data on the failed node is replicated and remains available on other nodes. In addition, by replicating ‘migration states,’ Valkey mitigates data loss during data migration between nodes or during rearrangements thereof.
Dual-channel RDB (Redis Database) and replica backlog streaming have also improved the data replication process. This speeds up the process and provides faster system response when there is a high real-time demand for data. Across the board, these improvements should result in data that remains consistent and replicated correctly, with minimal data loss, even when hitting peak data demand.
Improved observability
In the area of observability, Valkey 8.0 provides comprehensive statistics per slot and per client. These include statistics for pub-sub-clients, rehash memory, event loop latency, and command-level heavy traffic logging. This helps users get a detailed look into system performance and resource utilization. Memory overhead has also been optimized in Valkey 8.0, saving up to 10 percent key storage.
Valkey 8.0 is now available for download from the official website valkey.io. Existing users can upgrade relatively easily directly through the source code or a pre-built container image.
Also read: Google Cloud adds graph and vector search features to Spanner database
Java News Roundup: Payara Platform, Piranha Cloud, Spring Milestones, JBang, Micrometer, Groovy
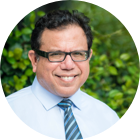
MMS • Michael Redlich
Article originally posted on InfoQ. Visit InfoQ
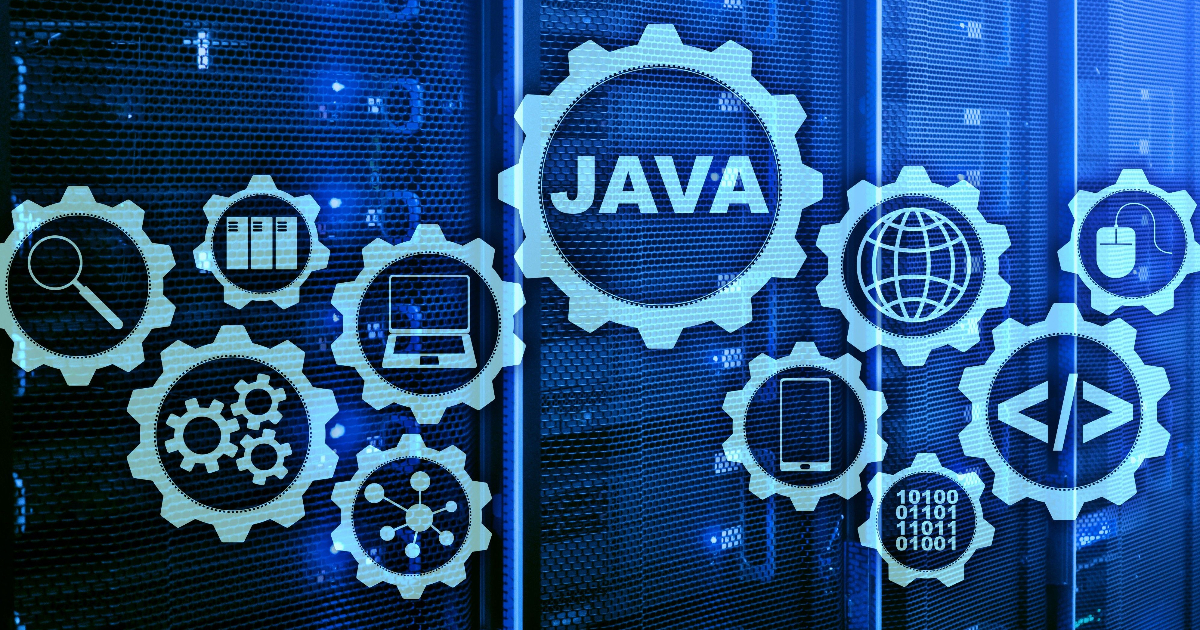
This week’s Java roundup for September 9th, 2024 features news highlighting: the September 2024 Payara Platform, Piranha Cloud and Micrometer releases, Spring Framework 6.2.0-RC1, Spring Data 2024.1.0-M1, JBang 0.118.0 and Groovy 5.0.0-alpha-10.
JDK 23
Build 37 remains the current build in the JDK 23 early-access builds. Further details on this release may be found in the release notes and details on the new JDK 23 features may be found in this InfoQ news story.
JDK 24
Build 15 of the JDK 24 early-access builds was made available this past week featuring updates from Build 14 that include fixes for various issues. More details on this release may be found in the release notes.
For JDK 23 and JDK 24, developers are encouraged to report bugs via the Java Bug Database.
GraalVM
Oracle Labs has released version 0.10.3 of Native Build Tools, a GraalVM project consisting of plugins for interoperability with GraalVM Native Image. This latest release provides notable changes such as: a refactor of the MergeAgentFilesMojo
class (and related classes) to remove the macro from the merger
init
command and throw a more informative message from the MojoExecutionException
if the command doesn’t exist; a resolution to incorrect results while parsing command-line arguments due to the presence of whitespaces in the Windows file system; and a resolution to the nativeTest
command unable to be executed when using JUnit 5.11.0-M2. More details on this release may be found in the changelog.
Spring Framework
The first release candidate of Spring Framework 6.2.0 delivers bug fixes, improvements in documentation, dependency upgrades and new features such as: an instance of the ResponseBodyEmitter
now allows the registration of multiple state listeners; a rename of some class names for improved consistency in the org.springframework.http.client
package due to the recent introduction of the ReactorNettyClientRequestFactory
class; and a refactor of the ETag
record class for improved comparison logic and exposing it on methods defined in the HttpHeaders
class. More details on this release may be found in the release notes and what’s new page.
Similarly, Spring Framework 6.1.13 has also been released providing bug fixes, improvements in documentation, dependency upgrades and new features such as: errors thrown from the stop()
method, defined in the SmartLifeycle
interface, results in an unnecessary wait for the shutdown timeout; and an end to logging the value of result
after changes made to the WebAsyncManager
class as it was decided to allow applications to do so via other classes. More details on this release may be found in the release notes.
The Spring Framework team has disclosed CVE-2024-38816, Path Traversal Vulnerability in Functional Web Frameworks, a vulnerability in which an attacker can create a malicious HTTP request to obtain any file on the file system that is also accessible to the process on the running Spring application. The resolution was implemented in version 6.1.3 and backported to versions 6.0.4 and 5.3.40.
Versions 2024.1.0-M1, 2024.0.4 and 2023.1.10 of Spring Data have been released feature bug fixes and respective dependency upgrades to sub-projects such as: Spring Data Commons 3.4.0-M1, 3.3.4 and 3.2.10; Spring Data MongoDB 4.4.0-M1, 4.3.4 and 4.2.10; Spring Data Elasticsearch 5.4.0-M1, 5.3.4 and 5.2.10; and Spring Data Neo4j 7.4.0-M1, 7.3.4 and 7.2.10. These versions may be consumed by the upcoming releases of Spring Boot 3.4.0-M3, 3.3.4 and 3.2.10, respectively.
Version 4.25.0 of Spring Tools has been released with notable changes such as: improvements to Microsoft Visual Studio Code with the addition of code lenses to explain SPEL expressions and AOP annotations with Copilot, and syntax highlighting and validation for CRON expressions inside the Spring Framework @Scheduled
annotation. More details on this release may be found in the release notes.
Open Liberty
IBM has released version 24.0.0.9 of Open Liberty featuring: support for the MicroProfile Telemetry 2.0 specification that now includes observability with metrics; the continued use of third-party cookies in Google Chrome with Cookies Having Independent Partitioned State (CHIPS); and a resolution to CVE-2023-50314, a vulnerability in IBM WebSphere Application Server Liberty versions 17.0.0.3 through 24.0.0.8 that would allow an attacker, with access to the network, to conduct spoofing attacks resulting in obtaining a certificate issued by a trusted authority to obtain sensitive information.
Payara
Payara has released their September 2024 edition of the Payara Platform that includes Community Edition 6.2024.9 and Enterprise Edition 6.18.0 and Enterprise Edition 5.67.0. Along with bug fixes and dependency upgrades, all three releases primarily address security issues, namely: an attacker having the ability to inject a malicious URL via a Host header allowing an HTML page generated by the REST interface to target the /management/domain
endpoint; and an exposure in which a new password being logged via the admin GUI when the logging is set to the FINEST
level. Further details on these releases may be found in the release notes for Community Edition 6.2024.9 and Enterprise Edition 6.18.0 and Enterprise Edition 5.67.0.
Micronaut
The Micronaut Foundation has released version 4.6.2 of the Micronaut Framework featuring Micronaut Core 4.6.5, bug fixes, improvements in documentation and updates to modules: Micronaut Data Micronaut OpenAPI/Swagger Support, Micronaut SQL Libraries, Micronaut JAX-RS, Micronaut Cache, Micronaut Views and Micronaut Security. Further details on this release may be found in the release notes.
Quarkus
Quarkus 3.14.3, the second maintenance release (the first one was skipped) delivers bug fixes, dependency upgrades and a new feature that provides initial support for a Software Bill of Materials (SBOM) using the CycloneDX standard. More details on this release may be found in the changelog.
Micrometer
The third milestone release of Micrometer Metrics 1.14.0 provides bug fixes, improvements in documentation, dependency upgrades and new features such as: no registration of metrics from the CaffeineCacheMetrics
class (and related classes) when statistics are not enabled; and a resolution to metrics not being collected when an instance of the Java ExecutorService
interface, wrapped in the monitor()
method, defined in the ExecutorServiceMetrics
class, shuts down. More details on this release may be found in the release notes.
Similarly, versions 1.13.4 and 1.12.10 of Micrometer Metrics feature notable bug fixes: a situation where Spring Boot configuration specifying metric percentiles in a standard application.yaml
file are being overwritten; and a non-resolvable dependency, io.micrometer:concurrency-tests
, incorrectly added to the Bill of Materials (BOM). Further details on these releases may be found in the release notes for version 1.13.4 and version 1.12.10.
Versions 1.4.0-M3, 1.3.4 and 1.2.10 of Micrometer Tracing 1.4.0 provide dependency upgrades and a resolution to a dependency convergence error when trying to use the io.micrometer:micrometer-tracing-bridge-otel
dependency after upgrading to Micrometer Tracing 1.3.1. Further details on these releases may be found in the release notes for version 1.4.0-M3, version 1.3.4 and version 1.2.10.
Apache Software Foundation
Versions 11.0.0-M25, 10.1.29 and 9.0.94 of Apache Tomcat deliver bug fixes, dependency upgrades and notable changes such as: ensure that any instance of the Jakarta Servlet ReadListener
interface is notified via a call to the onError()
method if an HTTP/2 client resets a stream before the servlet request body is fully written; and an improvement in exception handling with methods annotated with the Jakarta WebSocket @OnMessage
annotation that avoids the connection to automatically close. More details on these releases may be found in the release notes for version 11.0.0-M25, version 10.1.29 and version 9.0.94.
A regression affecting these versions, shortly after they were released, was reported and confirmed with configurations using HTTP/2. The Apache Tomcat team recommends a temporary fix by setting the property, discardRequestsAndResponses
, to true
on instances of the UpgradeProtocol
element for HTTP/2. The Tomcat team plans to release a fix for this regression during the week of September 16, 2024.
The tenth alpha release of Apache Groovy 5.0.0 delivers bug fixes, dependency upgrades and improvements that support: method references and method pointers in annotations; and the use of multiple @Requires
, @Ensures
and @Invariant
annotations, located in the groovy-contracts
package, that enable class-invariants, pre- and post-conditions. More details on this release may be found in the release notes.
Similarly, the release of Apache Groovy 4.0.23 features bug fixes and dependency upgrades. More details on this release may be found in the release notes.
Project Reactor
The sixth milestone release of Project Reactor 2024.0.0 provides dependency upgrades to reactor-core 3.7.0-M6
. There was also a realignment to version 2024.0.0-M6 with the reactor-netty 1.2.0-M5
, reactor-pool 1.1.0-M5
, reactor-addons 3.6.0-M2
, reactor-kotlin-extensions 1.3.0-M2
and reactor-kafka 1.4.0-M1
artifacts that remain unchanged. Further details on this release may be found in the changelog.
Next, Project Reactor 2023.0.10, the tenth maintenance release, provides dependency upgrades to reactor-core 3.6.10
. There was also a realignment to version 2023.0.10 with the reactor-netty 1.1.22
, reactor-pool 1.0.8
, reactor-addons 3.5.2
, reactor-kotlin-extensions 1.2.3
and reactor-kafka 1.3.23
artifacts that remain unchanged. More details on this release may be found in the changelog.
Finally, Project Reactor 2022.0.22, the twenty-second maintenance release, provides dependency upgrades to reactor-core 3.5.20
and reactor-netty 1.1.22
and reactor-pool 1.0.8
, reactor-addons 3.5.2
and reactor-kotlin-extensions 1.2.3
. There was also a realignment to version 2022.0.22 with the reactor-kafka 1.3.23
artifacts that remain unchanged. Further details on this release may be found in the changelog. This version is also the last in the 2022.0 release train as per the OSS support schedule.
Piranha Cloud
The release of Piranha 24.9.0 delivers notable changes such as: TCK updates in the Piranha Core Profile to support a number of Jakarta EE specifications (Jakarta Annotations 2.1.1, Jakarta Dependency Injection 2.0.2, Jakarta JSON Binding 3.0.0, etc.); and updates in their Arquillian adapter for improved deployment and un-deployment, and expose the ability to set the HTTP port and JVM arguments. Further details on this release may be found in their documentation and issue tracker.
JHipster
The release of JHipster Lite 1.18.0 delivers bug fixes, improvements in documentation, dependency upgrades and new features/enhancements such as: a new class, OpenApiContractApplicationService
, part of a new API that checks for backwards incompatible changes to OpenAPI contracts; and a refactor of the vue-core
module for improved testing. There was also removal of deprecated code that may cause a breaking change. More details on this release may be found in the release notes.
JBang
Version 0.118.0 of JBang provides bug fixes and a new linuxdistro
provider that searches a developer’s /usr/lib/jvm
folder to detect JDKs that have already been installed. More details on this release may be found in the release notes.
JetBrains Ktor
The first release candidate of Ktor 3.0.0 delivers bug fixes and new features such as: support for Kotlin 2.0.0; an improved staticZip
utility that watches for changes and reloading of ZIP files; and support for handling HTTP errors. More details on this release may be found in the release notes.
Gradle
Gradle 8.10.1, the first maintenance release, provides resolutions to issues: a performance degradation with version 8.10.0 due to dependency resolutions with detached configurations; an instance of the LifecycleAwareProject
class is equal, via the equals()
method, to an instance of it corresponding DefaultProject
class, but not the other way around; and Gradle validating isolated projects when the configuration cache is disabled. More details on this release may be found in the release notes.
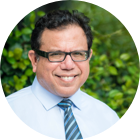
MMS • RSS
Posted on mongodb google news. Visit mongodb google news
Forsta AP Fonden reduced its holdings in shares of MongoDB, Inc. (NASDAQ:MDB – Free Report) by 8.3% in the 2nd quarter, according to the company in its most recent 13F filing with the Securities and Exchange Commission. The fund owned 14,300 shares of the company’s stock after selling 1,300 shares during the quarter. Forsta AP Fonden’s holdings in MongoDB were worth $3,574,000 as of its most recent SEC filing.
A number of other hedge funds have also made changes to their positions in the stock. Pier Capital LLC grew its holdings in MongoDB by 112.5% during the 2nd quarter. Pier Capital LLC now owns 3,064 shares of the company’s stock worth $766,000 after acquiring an additional 1,622 shares in the last quarter. Headlands Technologies LLC acquired a new position in MongoDB in the second quarter worth about $622,000. Daiwa Securities Group Inc. increased its holdings in MongoDB by 6.5% in the second quarter. Daiwa Securities Group Inc. now owns 9,191 shares of the company’s stock valued at $2,297,000 after buying an additional 563 shares during the last quarter. Levin Capital Strategies L.P. acquired a new stake in MongoDB during the second quarter valued at approximately $250,000. Finally, Exane Asset Management bought a new position in MongoDB during the 2nd quarter worth approximately $1,066,000. Institutional investors and hedge funds own 89.29% of the company’s stock.
Insider Transactions at MongoDB
In other MongoDB news, Director John Dennis Mcmahon sold 10,000 shares of the business’s stock in a transaction dated Monday, June 24th. The stock was sold at an average price of $228.00, for a total value of $2,280,000.00. Following the completion of the sale, the director now owns 20,020 shares of the company’s stock, valued at $4,564,560. The sale was disclosed in a legal filing with the Securities & Exchange Commission, which is available through this link. In related news, Director John Dennis Mcmahon sold 10,000 shares of the stock in a transaction that occurred on Monday, June 24th. The stock was sold at an average price of $228.00, for a total transaction of $2,280,000.00. Following the completion of the sale, the director now owns 20,020 shares in the company, valued at approximately $4,564,560. The sale was disclosed in a filing with the SEC, which is available through the SEC website. Also, Director Dwight A. Merriman sold 1,000 shares of the stock in a transaction that occurred on Thursday, June 27th. The shares were sold at an average price of $245.00, for a total value of $245,000.00. Following the completion of the sale, the director now directly owns 1,146,003 shares of the company’s stock, valued at $280,770,735. The disclosure for this sale can be found here. Insiders have sold a total of 32,005 shares of company stock worth $8,082,746 in the last 90 days. 3.60% of the stock is owned by insiders.
MongoDB Trading Down 1.7 %
Shares of MDB stock opened at $290.09 on Monday. The stock has a 50-day simple moving average of $255.85 and a 200 day simple moving average of $302.21. The stock has a market capitalization of $21.28 billion, a P/E ratio of -103.23 and a beta of 1.15. MongoDB, Inc. has a fifty-two week low of $212.74 and a fifty-two week high of $509.62. The company has a quick ratio of 5.03, a current ratio of 5.03 and a debt-to-equity ratio of 0.84.
MongoDB (NASDAQ:MDB – Get Free Report) last announced its quarterly earnings data on Thursday, August 29th. The company reported $0.70 earnings per share (EPS) for the quarter, topping the consensus estimate of $0.49 by $0.21. MongoDB had a negative net margin of 12.08% and a negative return on equity of 15.06%. The company had revenue of $478.11 million for the quarter, compared to analysts’ expectations of $465.03 million. During the same quarter in the previous year, the company posted ($0.63) EPS. The business’s revenue was up 12.8% compared to the same quarter last year. On average, sell-side analysts expect that MongoDB, Inc. will post -2.46 earnings per share for the current fiscal year.
Analyst Upgrades and Downgrades
MDB has been the subject of a number of research analyst reports. Morgan Stanley boosted their price target on MongoDB from $320.00 to $340.00 and gave the company an “overweight” rating in a report on Friday, August 30th. Truist Financial raised their price target on shares of MongoDB from $300.00 to $320.00 and gave the stock a “buy” rating in a research report on Friday, August 30th. Bank of America boosted their price objective on shares of MongoDB from $300.00 to $350.00 and gave the company a “buy” rating in a research report on Friday, August 30th. Oppenheimer raised their target price on shares of MongoDB from $300.00 to $350.00 and gave the stock an “outperform” rating in a report on Friday, August 30th. Finally, Scotiabank upped their target price on MongoDB from $250.00 to $295.00 and gave the company a “sector perform” rating in a report on Friday, August 30th. One investment analyst has rated the stock with a sell rating, five have assigned a hold rating and twenty have given a buy rating to the stock. According to data from MarketBeat.com, the company presently has a consensus rating of “Moderate Buy” and an average price target of $337.56.
Get Our Latest Analysis on MongoDB
About MongoDB
MongoDB, Inc, together with its subsidiaries, provides general purpose database platform worldwide. The company provides MongoDB Atlas, a hosted multi-cloud database-as-a-service solution; MongoDB Enterprise Advanced, a commercial database server for enterprise customers to run in the cloud, on-premises, or in a hybrid environment; and Community Server, a free-to-download version of its database, which includes the functionality that developers need to get started with MongoDB.
Read More
Want to see what other hedge funds are holding MDB? Visit HoldingsChannel.com to get the latest 13F filings and insider trades for MongoDB, Inc. (NASDAQ:MDB – Free Report).
Receive News & Ratings for MongoDB Daily – Enter your email address below to receive a concise daily summary of the latest news and analysts’ ratings for MongoDB and related companies with MarketBeat.com’s FREE daily email newsletter.
Article originally posted on mongodb google news. Visit mongodb google news
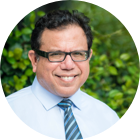
MMS • Anthony Alford
Article originally posted on InfoQ. Visit InfoQ
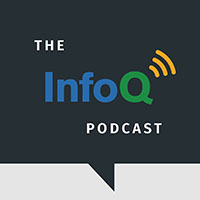
Subscribe on:
Transcript
Introduction [00:42]
Thomas Betts: Hi, everyone. Here at InfoQ, we try to provide our audience with information about the latest software innovations and trends. And I personally recognize that sometimes there’s a lot of new information out there and we tend to focus on the subjects that are most relevant to what we’re currently working on and what we’re interested in. Then sometimes you realize that what used to be one of those subjects off to the side is now right in front of you and you can’t ignore it anymore. And I’ll admit that that was my approach for a lot of the news over the past decade or so about big data, machine learning, artificial intelligence. I found it interesting, but because it wasn’t what I was working with, I had this very thin, high-level understanding of most of those topics. And that’s fine. That’s how software architects usually approach a problem.
We tend to be T-shaped in our knowledge. We have a broad range of subjects we need to know about, and we only go deep in our understanding of a few of them until we have to go deeper in our understanding for something else. That’s where I think we’ve gotten with ML and AI. It’s no longer something off to the side. Architects have to deal with these every day. They’re front and center because product owners, CTOs, CEOs, maybe even our customers are asking, “Can you put some AI in that?” to just about everything, it seems.
That gets me to today’s episode. I’ve invited Anthony Alford on to help explain some of these ML and AI concepts that are now, I think, required knowledge to be an effective software architect. Anthony’s voice will probably sound familiar because he’s another InfoQ editor. He co-hosts the Generally AI podcast with Roland Meertens, and I believe that just started its second season. Anthony, thanks for joining me on my episode of the InfoQ Podcast.
Anthony Alford: Thank you for having me.
Thomas Betts: I think a useful way to go through this today in our discussion is to do this big AI glossary. There’s a lot of terms that get thrown around, and that’s where, I think, architects need to understand what is that term and then figure out how much do I need to know about it so they can have intelligent conversations with their coworkers. I want to provide today just enough information so that those architects can go and have those conversations and realize when something comes up and they have to start implementing that for a project or thinking about a design, they have a little bit more context and that will help them be more successful as they do more research. Sound like a plan?
Anthony Alford: Sounds great.
AI usually means deep learning or neural networks [03:00]
Thomas Betts: All right. First give me your definition. What is AI?
Anthony Alford: AI is artificial intelligence.
Thomas Betts: And we’re done.
Anthony Alford: Yay. And, in fact, when I talk to people about this, I say, “AI really tells you more about the difficulty of the problem you’re trying to solve”. It’s not an actual solution. The good news is when most people are talking about AI, they’re actually talking about some type of machine learning. And machine learning is definitely a technology. It’s a well-studied, well-defined branch of science. And, in fact, the part of machine learning that most people mean now is something called deep learning, which is also known as neural networks. This has been around since the 1950s, so it’s pretty widely studied.
ML models are just functions that take input and provide output [03:48]
Thomas Betts: Yes, I think that’s the idea that AI is not a product you can go buy. You can go buy a machine learning model. You can build a machine learning model. You can add it to your system, but you can’t just say, “I want an AI”. But that’s the way people are talking about it. Let’s start talking about the things that exist, the tangible elements. Give me some examples of what people are thinking when they say, “I want AI in my system”. What are the machine learning elements they’re talking about?
Anthony Alford: Of course, most people are talking about something like a large language model or a generative AI. What I like to tell people as software developers, the way you can think about these things is it’s a function. We write code that calls functions in external libraries all the time. At one level you can think about it. It is just a function that you can call. The inputs and outputs are quite complex, right? The input might be an entire image or a podcast audio, and the output might also be something big like the transcript of the podcast or a summary.
Thomas Betts: And that’s where we get into the… Most people are thinking of generated AI, gen AI. Give me some text, give me an image, give me some sound. That’s the input. Machine learning model, it all comes down to ones and zeros, right? It’s breaking that up into some sort of data it can understand and doing math on it, right?
Anthony Alford: Yes, that’s right. Again, when I talk to software developers, I say, “When you think about the input and output of these functions, the input and output is just an array of floats”. Actually, it’s possibly a multidimensional array. The abstract term for that is a tensor. And if you look at some of the common machine learning libraries, they’re going to use the word tensor. It just means a multidimensional array, but you have to be able to express all your inputs and outputs as these tensors.
Building an ML model is like writing a lot of unit tests and refining the function [05:42]
Thomas Betts: Yes, these are the things I learned in math back in university years ago, but because I’m not a data scientist, I don’t use those words every day and forget, “Oh yes, multidimensional array, I understand what that is”. But exactly. That’s like several extra syllables I don’t need to say. I’ve got these tensors I’m putting in. What do I do with it? How do I build one of these models?
Anthony Alford: Okay, if you want to build your own model, which you actually might want to consider not doing that, we can talk about that later. But in general, the way these models are built is a process called training and supervised learning. What you really need, again, from our perspective as software developers, we need a suite of unit tests. A really big suite of unit tests, which means just what we expect, some inputs to the function and expected outputs from the function. The training process essentially is randomly writing a function. It starts with a random function and then just keeps fixing bugs in that function until the unit test pass somewhat. They don’t actually have to exactly pass. You also tell it, “Here’s a way to compute how bad the tests are failing and just make that number smaller every time”.
Thomas Betts: That’s where you get to the probability that this all comes down to math. Again, I’m used to writing unit tests and I say, “My inputs are A and B, and I expect C to come out”. You’re saying, “Here’s A and B and I expect C”. But here’s how you can tell how close you are to C?
Anthony Alford: Exactly, yes. It depends on the data type. I mentioned they all turn into tensors, but the easiest one is, let’s say, you’re building a model that outputs an actual number. Maybe you’re building a model that the inputs are things like the square feet of a house and the number of rooms, et cetera. And the output is the expected house price. If you give it unit tests, you can get a measure of how off the unit test is just by subtracting the number that you get out from the number that you expect. You can do sum of squared errors. Then the machine learning will just keep changing the function to make that sum of squared errors lower. With something like text or an image, it may be a little trickier to come up with a measurement of how off the unit tests are.
Language models are trained using sentences to predict the probability of the next word in the sentence [07:59]
Thomas Betts: We’re getting into all the ideas of gen AI. Let’s just take the text example for now and we’ll leave off audio and images and everything else, because it’s the same principles. Most people are familiar with interacting with ChatGPT. I type in something and it gives me a bunch of text. How did those come about and how did we create these LLMs that people said, “When I type in this sentence, I expect this sentence in response”.
Anthony Alford: Okay, so how long of the story do you want Here? We can go back to 2017 or even earlier.
Thomas Betts: Let’s give the high level details. If it’s an important milestone, I think it’s useful to sometimes have the origin story.
Anthony Alford: You’re right. The short answer is these things like ChatGPT or what are called language models, the input of the function is a sequence of words or more abstractly tokens. The output is all possible tokens along with their probability of being the next one. Let me give you an example. If I give you the input sequence once upon a… What’s the next word?
Thomas Betts: I’m going to guess time.
Anthony Alford: Right. What the LLM will give you is will give you every possible word with its probability and time will have a very high probability of being the next one. Then something like pancake would have a lower probability. That’s a probability distribution. We actually know the answer. In training, we know that in the unit test, the word time has the probability of 100%. Every other word has a probability of zero. That’s one probability distribution. The probability distribution it gives us is another one. And there’s a measure of how different those are. That’s called cross-entropy loss.
That’s how you can train it to improve that. It’ll shift its output distribution to have time be closer to 100% and everything else zero. That’s a language model, and the method that I described is really how they’re trained. You take a whole bunch of text and you take sequences of that text and you chop out a word or multiple words and you have it fill in those words. The way it fills it in is it gives you a probability distribution for every possible word. Ideally, the one you chopped out has the highest probability.
Thomas Betts: Got you. It’s like the image recognitions that we’ve seen for years.
Anthony Alford: Exactly.
Thomas Betts: We’ve had image recognition models. It’s like, “How do I identify this is a dog? This is a cat?” and we trained it. It’s like, “This is a cat. This is a dog”. And it started putting that into its model somehow. It’s like when I see this array of pixels, the answer is such a probability that it is a dog in that picture.
Anthony Alford: Yes. And in general, if we want to talk about data types, this is an enumeration. With enumeration data types, this thing is what you might call a classifier. You were talking about a dog or a cat. It’ll give you an answer for every possible output class. Every possible enumeration value has a probability associated with it. You want the real one to be close to 100%, and you want the rest of them to be close to zero. It’s the same for text. The entire vocabulary is given in a probability distribution.
Neural networks are doing matrix multiplication, with extremely large matrices [11:14]
Thomas Betts: That’s when you hear about how big these models are, it’s how much they’ve been trained on. The assumption is that ChatGPT and GPT-4 was basically trained on everything that you could possibly get off the internet. I don’t know how true that is, but that’s the way people talk about.
Anthony Alford: It’s close enough to be true. That’s the data set. There’s also the number of parameters that make up the model. When we’re talking about these deep learning models, those are neural networks. And neural networks are, at heart, matrix multiplication. I mentioned those input tensors. You could think of them as like matrices. You can multiply that times the model’s matrix. We talk about those matrix entries are sometimes called weights because ultimately what you’re doing is a weighted sum of the input values. When we talk about how big is the model, we’re talking about how many matrix parameters are in that thing. For GPT-4, we don’t know. We were not told. If you go all the way back to GPT-2, there was like one and a half billion parameters in the matrices inside it.
Thomas Betts: Yes, I think we’re now seeing…
Anthony Alford: Hundreds of billions.
Thomas Betts: Hundreds of billions, yes.
Where does large language model come in? Is it in the billion?
Anthony Alford: Yes. Well, it’s not a hard number. But what we’re seeing now is if something is tens or hundreds of billions, that’s probably large. We have smaller ones now where you’ll see Llama or something like… What is it, Gemma from Google? And Phi from Microsoft. Those are still billions, but they’re only… From 1 to 10 billion is considered a small model now. That’s small enough to run on your laptop actually.
Thomas Betts: Okay, you just threw out several other names and these are the things that I’m talking about that architects were like, “Oh, I think I’ve heard of Llama. Gemma sounds familiar”. And was it Psi?
Anthony Alford: Phi, P-H-I, right. The Greek letter. Here in America, Phi, but other places it’s Phee.
Hugging Face is like GitHub for language models [13:28]
Thomas Betts: I know you can go out and find details of some of these. There’s a site called Hugging Face that I don’t understand, but you can go and find the models and you can test the models. What is that?
Anthony Alford: Hugging Face, you can think of as the GitHub for language models. In fact, I mentioned a library. They have an SDK. They have a library, Python library you can install on your laptop that will behind the scenes download and run these smaller language models that you can actually run on your machine. What they do is they have files that contain those matrix entries that I mentioned.
Thomas Betts: That’s the composed model, if you will, right? I always think the training is I’m going to run my program and the output is the model. The training process might take hours or days, but once it’s done, it’s done and it’s baked. Now I have the model, and now that model, for large language models or small language models, you’re saying it’s something that I can put on my laptop. Some of those, if they were smaller machine learning models, we’ve been able to move those around for a while, right?
Two phases of the machine learning life cycle [14:35]
Anthony Alford: Oh, yes. We can think of two phases in the life cycle of machine learning model, the training that you mentioned. We could think of that as developing a function, and then once it’s developed, once we’ve written the function, we might build it and deploy it as a jar, for example, or some kind of library that you can use. The trained model is like that, and when you load it up and you put an input into it and get an output out, that’s called inference. The model infers some output from your input. Those are the two big chunks of the model lifecycle.
Auto-regressive models take the output and feed it back in as the next input, adding to the context [15:12]
Thomas Betts: Back to the large language models where you’re talking about predict the next word and then predict the next word. This is where it’s feeding it back in. The way I’ve understood is it’s just auto-complete on steroids, one letter, one word. It’s like, “I’ll just do all of it”. It keeps feeding that sentence that it’s building back into the context, and so that’s the next thing.
Anthony Alford: That’s right. And you’ll hear these models referred to as autoregressive, and that’s exactly what they’re doing. You start with initial input, which sometimes we call that the prompt. We also call the input to the model, the context. The prompt is the initial context and then it outputs one more token that’s stuck on the end and then it feeds back as the new context and the process just repeats. These things also are able to output a token that basically says, “Stop”. And that’s how they know to stop. Whereas I’ve tried that auto-complete with my phone where I just keep auto-completing over and over. It eventually produces gibberish, but it is the exact same idea.
Tokens are the words or parts of words that the model can respond with [16:18]
Thomas Betts: You’ve now said token a few times, and I keep saying word. And I know the layman is usually interchanging those, and it’s not exactly the same thing. That a token is not a word all the time. What is a token in terms of these language models?
Anthony Alford: When people first started, it was words. We’re probably familiar with the idea with search engines of doing things like stemming or things like that where the word itself doesn’t actually become the token. The reason you want to do something that’s not exactly the word is I mentioned you can only get an output that is one of the tokens that it knows about. You’ve seen things like, “Well, let’s just use the bytes as tokens”. I think now it’s byte pairs. Basically, it’s no longer at the word level. A token is smaller than a word. You might see a token be a couple of letters or characters or bytes.
Thomas Betts: And what’s the advantage of shrinking those down? Instead of predicting the next word is once upon a time, it would predict T and then I and then M then E.
Anthony Alford: Or something like that, or TI. The reason is so that you can output words that are not real words, that wouldn’t be in the regular vocabulary.
Thomas Betts: Now is it smart enough to say that time is one possible token and TI might be a different one? Does it break it down both ways?
Anthony Alford: The tokenization is, that’s almost become a commodity in itself. Most people are not really looking at what the specific token data set is. I think typically you want something a little bigger than one character, but you want something smaller than a word. This is something that researchers have experimented with.
Thomas Betts: And my interaction with knowing the number of tokens counts is… When I’ve played around these things, used a ChatGPT or OpenAI, API, it’s measuring how many tokens are being used. And you’re being sometimes billed by the number of tokens.
Anthony Alford: Yes, that’s right. Because essentially the output is a token, and the input we mentioned, that’s called the context, the models have a maximum size of the context or input in the number of tokens. It’s in the order of thousands or maybe even hundreds of thousands now with a lot of these models. But eventually, it will have to stop because effectively you can’t take a larger input.
Thomas Betts: Yes, and I remember people found those limits when ChatGPT came out is you’d have this conversation that would go on and on and on, and pretty soon you watched the first part of your conversation just fall off the stack, if you will.
Anthony Alford: Yes, the maximum context length is built into the model. And there’s a problem with the algorithmic complexity is the square of that context size. As you get bigger, the model gets bigger as the square of that, and that’s how the runtime increases as the square of that, et cetera.
Efficiency and power consumption [19:15]
Thomas Betts: That’s where you’re getting into the efficiency of these models. There’s been some discussion of how much power is being consumed in data centers all around the world to build these models, run these models, and that’s one of those things that you can get your head around. If you have this thing it takes…
Anthony Alford: It’s an awful lot.
Thomas Betts: It’s a lot. It’s an awful lot. Say it takes 30,000, 32,000 tokens and you’re saying the square of that, that suddenly gets very, very large.
Anthony Alford: Oh, yes. Not only does it grow as a square of that, but it’s like there’s a big multiplier as well. Training these models consumes so much power, only the people who do it know how much. But really they’re just looking at their cloud bill. Nobody knows what the cloud bill was for training GPT-3 or 4, but it’s a lot.
Thomas Betts: Yes, that’s why people are looking not to build your own model. Most people are not in the business of needing to create their own LLM. These things are done, but people are using them to replace Google searches. One of the problems is you don’t have the context because the model wasn’t trained on current events. It’s not searching Google and giving you results. It’s just predicting words.
Anthony Alford: Exactly. Now they are trying to build that in. If you use Bing, Bing is actually using GPT-4, and it will include search results in its answer, which when we get to the… I don’t want to spoiler, when we get to RAG, we can talk about that.
Transformers – GPT means Generative, Pretrained Transformer [20:43]
Thomas Betts: Well, let’s leave RAG off to the side a little bit. Let’s dig a little bit into transformer without rewriting the entire history. I think you and Roland have talked about that a little bit on your podcast.
Anthony Alford: Right, we’ve mentioned LLMs in general and GPT family in particular. Well, the T in GPT stands for transformer, and this was something that a Google research team came up with in 2017. They wrote a paper called Attention is All You Need. They were working on translation and before that the translation models were using recursion, which is different from what we were talking about with autoregression. Anyway, they came up with this model that really just uses a feature called attention or a mechanism called attention. They called it the transformer.
Now really all the language models are based on this. That’s what the T in GPT stands for. GPT stands for generative pre-trained transformer, and they all use this attention mechanism. You could think of attention as a way for the model to pick out what’s important in that input sequence. The word is, I think, sometimes used in… It’s similar to information retrieval, so it uses a lot of concepts like queries and keys and values. But at a high level, it’s a way for the model to… Given that input sequence, identify the important parts of it and use that to generate the next token.
Attention is weighting the input [22:13]
Thomas Betts: It might throw out some of your input or recategorize and say, “These are the important words in that context”.
Anthony Alford: The mathematics is, it finds keys that match the query and then returns the values that are associated with those. A lot of times it does focus on certain parts of the input versus other pieces.
Thomas Betts: That’s where weighting comes into play, right?
Anthony Alford: Exactly. That’s how it is.
Thomas Betts: You mentioned that these matrices have weights on them. It’s going to figure out which words or parts of that input, and that one word doesn’t always have the same weight. It’s in the context of that input, it might have more weight.
Anthony Alford: Yes, you did a better job explaining that than I did.
Thomas Betts: It’s not my first time trying to explain this. I get a little bit better every time. Again, one of the points of why I wanted to do this episode.
Adding an LLM to your product [23:03]
Thomas Betts: We’ve got transformers, it’s just a term, and the attention, that’s how we’re figuring out what goes in. That outputs, in the case of GPT outputs, GPT, but that’s a branded term. LLM is the generic term, right?
Anthony Alford: Right.
Thomas Betts: It’s like Kleenex versus tissue. Let’s say I want to use one of these LLMs in my application. This is the thing that my product owner, my CEO is like, “Put some AI on it”. I want to look like we’re being innovative. We’ve got to have something that is this predictive thing like, “Look at how it looked at our model and comes up with something”. How do we go about doing that?
Anthony Alford: Can I plug an InfoQ piece already? Just earlier this year I edited the eMag, the Practical Applications of Generative AI e-magazine. And we had several experts on LLMs in particular to talk about this. Definitely recommend everybody read that, but what they recommended is… You have publicly available commercial LLMs like GPT for ChatGPT. There’s also Claude. There’s also Google’s Gemini. AWS has some as well. Anyway, if you find one of these that seems to work, try it out. So you can quickly adopt LLM functionality by using one of these commercial ones. It’s just an API. It’s a web-based API. You call it using an SDK, so it looks like any kind of web service.
That’s number one. Number two, for long-term cost maybe, right? Because it’s a web service and API, like we said, we’re paying per token. It’s actually probably pretty cheap. But longer term there’s cost concerns, and there may be privacy concerns because these commercial LLMs have gotten better at their promises about, “We’re not gonna keep your data. We’re going to keep your data safe”. But there’s also the data that it gives you back in the case of, say, like code generation.
I think there was a lawsuit just recently. I think people whose code was used to train this, they’re saying that this thing is outputting my code, right? There’s concerns about copyright violation. Anyway, longer term, if you want to bring that LLM capability in house, you can use one of these open source models. You can run it in your own cloud, or you can run it in a public cloud but on your own machine. Then you have more control over that.
Thomas Betts: Yes, it’s kind of the build versus buy model. Right?
Anthony Alford: Exactly.
Thomas Betts: And I like the idea of, “Let’s see if this is going to work”. Do the experiments. Run those tests on the public one and maybe put some very tight guardrails. Make sure you aren’t sending private data. I think it was to plug another InfoQ thing. Recently the AI, ML trends report came out. I listened to that podcast. That was one where it mentioned that because they were setting up so many screens to filter and clean out the data before sending it to OpenAI or whichever API they were using, that scrubbed out some of the important context and the results coming back weren’t as good. Once you brought the model in house and you could say, “Oh, we own the data. It never leaves our network. We’ll send it everything”. All of a sudden your quality goes up too.
Anthony Alford: It’s definitely very easy to experiment with. And if you find that the experiment works, it may make sense to bring it in house. There’s the short answer.
Hosting an open-source LLM yourself [26:36]
Thomas Betts: Like you said, “If you want to pay per use and it’s easy to get started”. That’s one way to go. When you’re talking about bringing in house, you mentioned you can have it on your own cloud. Like we’re on Azure, AWS. Is that basically I spin up an EC2 instance and I just install my own.
Anthony Alford: That’s one way. Of course, the service providers like AWS are going to give you a value add version where they spin it up for you and it’s very much like the regular model where you pay per use. But yes, you could do that. You could do it right on EC2.
Thomas Betts: Yes. Are you doing the product as a service, the platform as a service, the infrastructure as a service, then you can do whatever you want on it. Your results may vary, but that might be another way to do that next phase of your experiment as you’re trying to figure out what this is. How easy is it for me to spin up something, put out a model there and say, “Okay, here’s our results using this public API, and here’s if we bring it in house with our private API”. Maybe you look at the cost. Maybe look at the quality of the results.
Anthony Alford: Yep, for sure.
Comparing LLMs [27:37]
Thomas Betts: How are people comparing those things? What is the apples to apples comparison of, “I’m going to use OpenAI versus one of the things I pull off of Hugging Face?”
Anthony Alford: This is actually a problem. As these things get better, it’s tricky to judge. In the olden days where we had things like linear regression and we had that supervised learning where we know the answer, we can get a metric that’s based on something like accuracy. What is the total sum of squared error? But nowadays, how good is the output of ChatGPT? Well, if you’re having it do your homework, if you get an A, then it was pretty good. And, in fact, believe it or not, this is very much a common thing that they’re doing now with these models is they’re saying, “We train this model, it can take the AP Chemistry exam and make a passing grade”.
Another thing I see a lot in the literature is if they’re comparing their model to a baseline model, they’ll have both models produce the output from the same input and have human judges compare them. It’s like Coke versus Pepsi, which four out of five people chose Pepsi. And even more fun is do that, but with ChatGPT as the judge. And believe it or not, a lot of people are doing that as well. I guess the answer is it’s not easy.
Thomas Betts: Yes, that’s where I tend to say these things are non-deterministic. You talked about the probability, you don’t know that the answer is going to come out. Your test is not… I asked this question, I got this answer. Because you don’t necessarily know what types of questions are going to be going in, so you don’t know what outputs are going to come out.
Anthony Alford: Yes, exactly. That’s actually one of the most scary things is you don’t know what’s going to come out. Something very unpleasant or embarrassing might come out and that’s really got people concerned about using these things in production environments.
Thomas Betts: Yes.
Before you adopt LLMs in your application, define your success criteria [29:38]
Anthony Alford: But I will say one thing… Again, talking back the e-magazine, one of my experts said, “Before you adopt LLMs in your application, you should have good success criteria lined out for that”. That may be the harder part to do. How will I know if it’s successful? It’s going to depend on your application, but it’s something you should think hard about.
Thomas Betts: Well, I like that because it puts back the question on the product owners. The CTOs are saying, “I need some AI in it”. What do you want to have happen? Because there’s a lot of places where you shouldn’t put AI. I work on an accounting system. You should not have it just guess your books.
Retrieval-Augmented Generation (RAG) should be an early step for improving your LLM adoption [30:19]
Thomas Betts: When we’re talking about using these for ourselves, whether we’re hosting them or bringing them in house, how do we get those better quality results? Do we just use them out of the box? I had a podcast a while ago and learned about retrieval augmented generation. I hear RAG talked about a lot. Give me the high level overview of what that is and why that should be a first step to make your LLM adoption better.
Anthony Alford: Again, on my expert panel, they said, “The first thing is to try better prompts”. We’ve probably heard of prompt engineering. We know that the way you phrase something to ChatGPT makes a big difference in how it responds. Definitely try doing stuff with prompts. The next step, retrieval augmented generation or RAG. I think we mentioned, the LLMs, they’re trained and they don’t know anything that happened after that training. If we ask who won the football game last night? It doesn’t know, or it might not say it doesn’t know, it might actually make up something completely not true. This is also a problem for a business where you want it to know about your internal knowledge base, right? If you want it to know things that are on your Wiki or in your documentation, things like that. What RAG is is you take your documents, you break them up into chunks, but essentially you take a big chunk of text and you run it through an LLM that generates a single vector for that chunk of text.
This is called an embedding. And that vector in some way encodes the meaning of that text. You do this with all your documents and then you have a database where each document has a vector associated with it that tells you something about its meaning. Then when you go and ask the LLM a question, you do the same thing. You take your question and you turn that into a vector, and the vector database lets you quickly and efficiently find vectors that are close to that and therefore are close to your question in meaning. It takes the content from that and shoves that into the LLM context along with your question. And now it knows all that stuff along with your question. We know that these LLMs are very good at… If you give it a chunk of text and say, “Explain this”. Or, “Here’s a question about this chunk of text”. It is quite good. That’s what the intention mechanism does, is it lets it find parts of that chunk of text that answer the question or solve the problem that you’re asking.
Thomas Betts: The way I’ve heard that explained is, let’s say I do my search and instead of me writing a really elaborate prompt, because I’m willing to sit there and type for 30 seconds. That’s all the words I’m going to come up with. Instead, I would say, “Answer the question based on these documents”. And I can give all those documents in the context and now it knows, “Okay, that’s what I’m going to use”. I’m not going to use just my base level LLM predict the next word. I’m going to predict the next word based on this context.
Anthony Alford: Right. And the retrieval part is finding those documents automatically and including them in the context for you. That’s the key component… If you actually know the documents, and let’s say somebody gave you, “Here’s our user manual, answer questions about it”. Which is a pretty cool use case for someone who’s, say, in customer service. If the user manual is small enough to fit into the context, which it probably is at hundreds of thousands of tokens, then that’s great. But maybe you don’t have that. Maybe you have a bunch of knowledge base articles. This will go and find the right knowledge base article and then answer the question based on that.
Thomas Betts: Right, because our knowledge base has tens of thousands of articles opposed to a couple of hundred pages.
Anthony Alford: Exactly.
Thomas Betts: And you’re still using the LLM, which has all of its knowledge of, “Here’s how I complete a sentence”.
Anthony Alford: Yep.
Fine-tuning is one option to make an LLM better suited for your needs [34:07]
Thomas Betts: You are not building a new model based off of your knowledge base or your training documents.
Anthony Alford: Exactly. But let’s say you did want to do that and that might be a better solution in some cases, this process is called fine-tuning. I mentioned the T in GPT was transformer. The P is pre-trained. This is a whole subfield of machine learning called transfer learning where you train a model, you pre-train it and it’s general purpose. Then you can fine tune it for a specific case. In the case of GPT-2, 3 and higher, they found out you don’t need to. It’s pretty good on its own. But what the fine-tuning does is its additional training on that model. Instead of using the model as is, you restart the training process. You’ve got your own set of unit tests. You got your own fine-tuning data where the know the inputs, you know the outputs. The advantage is for fine-tuning, it can be much smaller than what is needed to train the full GPT.
Thomas Betts: And that’s because you’re starting from what already exists.
Anthony Alford: Exactly. Right.
Thomas Betts: You’re not starting from baseline or nothing. It’s just saying tweak your model. That’s going back to things that I understood, again, at a superficial level with machine learning training is like, “You can overtrain the data”. If you give too many answers in one area, it’s like, “Look, we got to a 99.9%”. But then something comes in and it doesn’t know about and it has no answer. It’s way off base. In this case, if I’m trying to get the model to be very specific to my company’s applications, my data, that might be the desired outcome. I don’t want someone to be using my customer service chatbot to ask about when’s the next Taylor Swift show?
Anthony Alford: Yes, exactly. In fact, the original ChatGPT and newer models, they do fine tune them to give more helpful answers and follow instructions. This is something with GPT-3.5, again, that model is pre-trained on basically the whole internet, and it could give you answers that were pretty good, but they found that sometimes it would just give you answers that were… It’s that whole joke about this is technically true but not at all useful. So they fine-tuned it to give you answers that are more helpful to follow instructions. They call it alignment, and the way they do that is they have a small data set of, “This was the input. Here’s the output you gave, but this output here is better”. They fine-tune it to work towards the more appropriate output.
Vector databases provide nearest-neighbor searches [36:45]
Thomas Betts: I need to back up just a little bit. When you mentioned we’re going to create these vectors, I’m going to have a vector database, I’m going to do a vector search. Another one of those terms that gets thrown around and people are like, “Well, do I have a vector database?” I think Azure just announced that they’re going to… I think it’s in beta right now. Basically turn your Cosmos database into a vector database, like flip a checkbox in the portal and all of a sudden you have vectors. What does that do for me? Why is that an advantage?
Anthony Alford: Okay, I have an upcoming podcast on this very problem. We mentioned that for a chunk of text you can create from that a vector that encodes the meaning. The vector is very high dimensional. It’s hundreds, maybe thousands of dimensions. You’re trying to solve the problem of given one vector, how do you find those vectors in the database that are close to that input vector? You could just run through all of them. You do a table scan basically, and sort the output. That probably is actually fine. The complexity is high enough that at scale it’s not going to perform great. What you need is something more like a B-tree lookup, which is log-N. The vector database is actually… The vectors are probably not the important part, it’s the nearest neighbor search. This is the problem we’re solving, is given a vector input what are the nearest neighbors to that vector in your database? That’s the problem that you want to solve in an efficient, scalable way.
Thomas Betts: Got you. It’s going through and looking at my data and saying, “Here are the vectors for all the parameters”. And based on that, these are related words…?
Anthony Alford: Well, no, literally just it doesn’t look it. It’s just given two vectors. How close are..
Thomas Betts: How close are the vectors? It doesn’t know what it came from?
Anthony Alford: Exactly, right. Now, once it finds the ones that are closed, then those are in the same database row or there’s a pointer to the content that it came from, which is what you actually care about.
Thomas Betts: Got you.
Anthony Alford: But the database, its purpose is to do the nearest neighbor search where you give it a vector and it finds the top K in its database that are closest to it.
Thomas Betts: Yes. This is where, I think, we’re going back to the beginning that AI as a product isn’t something that exists. We’ve had fuzzy search techniques for a while. This has been something people have wanted and everyone’s gotten used to Google. I can type in whatever I want, and it figures out. Like you said, you take the stems of the words… This is another one of those, I didn’t give you exactly what the answer asked for. So it’s not a find this row in the database, but find records that are close to what I intended and that’s what they’re doing.
Anthony Alford: Yes, I think you might find this referred to as semantic search or maybe neural search. The neural meaning that’s how the vectors are generated from a neural network.
Thomas Betts: But it’s all about that. I don’t have a specific thing in mind to find the intent.
An LLM is a tool to solve natural language processing (NLP) problems [39:45]
Thomas Betts: I guess LLMs really fall under in my head the category of natural language processing, right?
Anthony Alford: Yes, exactly.
Thomas Betts: Because that used to be a thing. I had data scientists on my team who were working in the field of natural language processing. Is that still a thing? Is that just a subset or has it just gotten overwhelmed in the news by LLMs?
Anthony Alford: I think you could think of an LLM as a tool to solve natural language processing problems. For example, we used to look at things like named-entity recognition, parts of speech recognition, that kind of thing. That’s still something you have to do, but an LLM can do it.
Thomas Betts: Right.
Anthony Alford: And it can do it pretty well and works out of the box. If you look at what, I think… Again, we were talking about Google and Attention is All You Need. They came up with a version of that called BERT, and it would do this stuff like named entities and parts of speech, tagging and things like that.
LLMs are useful because they are very general, but that does not make them general AI [40:44]
Thomas Betts: Got you. And that’s one of those LLMs are these generalists. Find ways to make them more specific. If you have a specific use case in mind, you can go down a fine-tuning route. You can find a different model that’s just closer, and that’s going to have those benefits of… It’s going to cost less to run. It’s probably going to be better quality answers. It’s probably going to return faster, I’m assuming if it’s less computational.
Anthony Alford: Yes, this is one of the reasons people are excited about LLMs is that they are very general. That’s one of the things where people started saying, “Is this general AI?” That’s been the holy grail of AI research forever. Yes, we can make a program that plays chess very well, but it can’t drive a car. The holy grail is to build one model that can solve just about any problem. Just if we flatter ourselves as human beings, we can do lots of different tasks. We can do podcasts. We can build model race cars or read books. The holy grail of AI is one model to rule them all, and LLMs could do so much without additional training. That’s what one of the early GPT papers was like, “Look, we built this thing out of the box. It can do summarization, question answering, translation, code generation, all these tasks”. And that’s one of the things that got people really excited about it. It looks like it could do everything.
Thomas Betts: Yes, I think it’s the now how do we use it? Because that seems so powerful. But going back to your point, you need to have a specific output in mind. What is your goal? Why would you add this? Because it sounds exciting. Everyone wants to use it, but you have an intent of how does that fit into your product? How does that fit into your solution?
Anthony Alford: Yes. It’s always about what business problem am I trying to solve? And how do I know if I succeeded?
AI copilots versus AI agents [42:38]
Thomas Betts: We’re over on time. I’m going to have one last bonus round question and we’ll wrap it up.
Anthony Alford: Yes.
Thomas Betts: A lot of people talk about having AI Copilots. I can’t remember how many Microsoft Copilots and GitHub Copilots. Everything’s a copilot. Distinguish that from an AI agent because that’s another term that’s being thrown around. They both sound like the embodiment of this thing as a person. There’s a whole different discussion about that. But these are two different things. What’s a co-pilot versus an agent?
Anthony Alford: I think we did talk about this on the trends podcast. An agent has some degree of autonomy. The co-pilot is you’ve got to push the button to make it go eventually. Again, I don’t want to turn this into AI fear. The fear that people have of AI is autonomous AI, in my opinion. If we can mitigate that fear by keeping things as co-pilots, then maybe that’s the way to go. But I think the key is autonomy. You have to agree to the co-pilots answer and make it go.
Thomas Betts: The agents can do stuff on their own, but maybe we have supervisor agents. And like you said, “I don’t know how to tell the output, so I’m going to ask ChatGPT, ‘Did I train my model correctly?'” And you feed it back into yet another AI. The AI agent story is you have supervisor agents who watch the other ones, and then it’s who’s watching the watchers?
Anthony Alford: Watches the watchers? Yes, indeed.
Thomas Betts: Well, I really appreciate all your time. I learned a lot. I hope this was useful for the audience.
Anthony Alford: Me too.
Thomas Betts: It’s always good to go through and do this little refresher of here’s what I think I understand, but bounced off someone who really knows. I’ll be sure to provide links to the things we mentioned. The eMag is great.
Anthony Alford: Yes.
Thomas Betts: Then the trends report and podcast and some other stuff. Anthony, thanks again for joining me on the InfoQ Podcast.
Anthony Alford: It was a pleasure. Thanks for having me.
Thomas Betts: And we hope you’ll join us again next time.
.
From this page you also have access to our recorded show notes. They all have clickable links that will take you directly to that part of the audio.