Month: April 2025
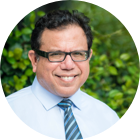
MMS • RSS
Posted on mongodb google news. Visit mongodb google news
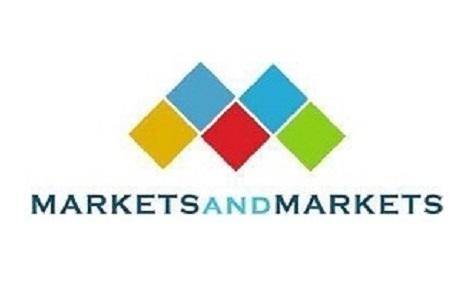
Microsoft (US), Elastic (US), Alibaba Cloud (China), MongoDB (US), Redis (US), SingleStore (US), Zilliz (US), Pinecone (US), Google (US), AWS (US), Milvus (US), Weaviate (Netherlands), and Qdrant (Berlin) Datastax (US), KX (US), GSI Technology (US), Clari
Vector Database Market by Offering (Solutions and Services), Technology (NLP, Computer Vision, and Recommendation Systems), Vertical (Media & Entertainment, IT & ITeS, Healthcare & Life Sciences) and Region – Global Forecast to 2028.
The global vector database market [https://www.marketsandmarkets.com/Market-Reports/vector-database-market-112683895.html?utm_campaign=vectordatabasemarket&utm_source=abnewswire.com&utm_medium=paidpr] is projected to expand from USD 1.5 billion in 2023 to USD 4.3 billion by 2028, reflecting a compound annual growth rate (CAGR) of 23.3%. This growth is being fueled by the rapid advancement of artificial intelligence (AI) and machine learning (ML), a rising demand for real-time data processing, and the increasing adoption of cloud computing technologies.
Download PDF Brochure@ https://www.marketsandmarkets.com/pdfdownloadNew.asp?id=112683895 [https://www.marketsandmarkets.com/pdfdownloadNew.asp?id=112683895&utm_campaign=vectordatabasemarket&utm_source=abnewswire.com&utm_medium=paidpr]
The vector database market is expanding, and vendors are adopting a strategic focus to attract customers. Vector databases are a powerful new technology well-suited for many applications. As the demand for machine learning and AI applications grows, vector databases will likely become even more popular. Vector databases are essential for many machine learning and AI applications, such as natural language processing, image recognition, and fraud detection; this is because vector databases can efficiently store and query large amounts of high-dimensional data, which is the type of data used in machine learning and AI. These services are increasing the demand for the vector database market.
The NLP segment holds the largest market size during the forecast period.
In the Natural Language Processing (NLP) context, the vector database market is a rapidly evolving sector driven by various factors. Vector database is instrumental in NLP applications for efficient storage, retrieval, and querying of high-dimensional vector representations of textual data. In NLP, a vector database is used for tasks like document retrieval, semantic search, sentiment analysis, and chatbots. They help store and search through large text corpora efficiently. Companies like Elasticsearch, Milvus, and Microsoft have been actively serving NLP applications. Many organizations also develop custom solutions using vector databases. The proliferation of text data on the internet and within organizations drives the need for an efficient vector database for text indexing and retrieval. Storing and searching for text embeddings enables content tagging, which is vital for content classification and organization in NLP applications.
The growth of the vector database market in NLP is due to the increasing importance of efficient text data management and retrieval. As NLP plays a significant role in various industries, including healthcare, finance, e-commerce, and content generation, the demand for advanced vector database solutions will persist and evolve. This trend will likely drive further innovations in vector databases, making them increasingly efficient and tailored to NLP-specific needs. NLP-driven applications aim to understand the context and meaning behind text data. Traditional databases may struggle to capture complex semantic relationships between words, phrases, and documents. Vector databases excel in storing and retrieving high-dimensional vector representations of text, which capture semantic relationships; this enables semantic search capabilities, allowing users to find information based on the meaning and context rather than relying solely on keywords.
Semantic search involves finding documents or pieces of text that are semantically similar to a given query. It goes beyond keyword matching and considers the meaning of words and phrases. NLP techniques enable understanding the semantic meaning of words, phrases, and documents. It goes beyond traditional keyword-based search and considers the context and relationships between terms.
Healthcare and Life Sciences vertical to record the highest CAGR during the forecast period.
The healthcare industry vertical is seeing a rise in using vector databases as a valuable tool. It offers medical professionals assistance in various areas, such as diagnosing diseases and creating new drugs. Vector database algorithms learn from vast sets of medical images and patient records, allowing them to detect patterns and anomalies that may go unnoticed by humans; this leads to more accurate and faster diagnoses and personalized treatments for patients. Vector database is used in healthcare, particularly in medical imaging. Generating high-resolution images of organs or tissues aids doctors in detecting early-stage diseases. Additionally, vector databases can assist in identifying new drug candidates for drug discovery by generating virtual molecules and predicting their properties. Furthermore, it can analyze patients’ medical history and predict the efficacy of different treatments, enabling the development of personalized treatment plans.
Our analysis shows North America holds the largest market size during the forecast period.
As per our estimations, North America will hold the most significant market size in the global vector database market in 2023, and this trend will continue. There are several reasons for this, including numerous businesses with advanced IT infrastructure and abundant technical skills. Due to these factors, North America has the highest adoption rate of the vector database. The presence of a growing tech-savvy population, increased internet penetration, and advances in AI have resulted in an enormous usage of vector database solutions. Most of the customers in North America have been leveraging vector databases for application-based activities that include, but are not limited to, text generation, code generation, image generation, and audio/video generation. The rising popularity and higher reach of vector databases are further empowering SMEs and startups in the region to harness vector database technology as a cost-effective and technologically advanced tool for building and promoting business, growing consumer base, and reaching out to a broader audience without a substantial investment into sales and marketing channels. Several global companies providing vector databases are in the US, including Microsoft, Google, Elastic, and Redis. Additionally, enterprises’ increased acceptance of vector database technologies to market their products modernly has been the key factor driving the growth of the vector database market in North America.
Request Sample Pages@ https://www.marketsandmarkets.com/requestsampleNew.asp?id=112683895 [https://www.marketsandmarkets.com/requestsampleNew.asp?id=112683895&utm_campaign=vectordatabasemarket&utm_source=abnewswire.com&utm_medium=paidpr]
Unique Features in the Vector Database Market
Vector databases are specifically designed to handle high-dimensional data, such as feature vectors generated by AI and machine learning models. Unlike traditional databases that manage structured rows and columns, vector databases enable fast similarity search and efficient handling of complex, unstructured data formats like images, audio, text embeddings, and video.
One of the standout features of vector databases is their ability to perform real-time similarity searches using Approximate Nearest Neighbor (ANN) algorithms. This allows applications such as recommendation engines, semantic search, fraud detection, and image recognition to deliver instant and highly accurate results.
Modern vector databases are built for scalability, supporting billions of vectors across distributed environments. With support for parallel computing and hardware acceleration (such as GPU-based processing), these databases maintain low latency and high throughput even as data volume grows.
Vector databases are often designed to work directly within AI/ML ecosystems. They support native integration with model inference engines, data preprocessing tools, and popular ML frameworks like TensorFlow, PyTorch, and Hugging Face, allowing for streamlined development and deployment workflows.
Major Highlights of the Vector Database Market
As artificial intelligence and machine learning continue to proliferate across industries, the need to store, manage, and search high-dimensional vector data has become essential. Vector databases serve as a foundational layer in AI/ML infrastructures, powering functions like recommendation systems, natural language processing, and image recognition.
Use cases requiring real-time, context-aware search capabilities-such as chatbots, intelligent virtual assistants, and fraud detection systems-are on the rise. Vector databases uniquely enable these applications by supporting similarity-based searches that go beyond keyword matching, offering deeper and more intuitive results.
While initially centered around tech giants and research labs, vector databases are now gaining traction in a wide range of industries including healthcare, e-commerce, finance, and media. Organizations are leveraging vector data to enhance personalization, automate decision-making, and extract insights from unstructured content.
The market is witnessing a rise in cloud-native vector databases and open-source solutions, making them more accessible and scalable. Vendors are offering managed services and seamless integration with popular cloud platforms, enabling faster deployment and lower operational overhead.
Inquire Before Buying@ https://www.marketsandmarkets.com/Enquiry_Before_BuyingNew.asp?id=112683895 [https://www.marketsandmarkets.com/Enquiry_Before_BuyingNew.asp?id=112683895&utm_campaign=vectordatabasemarket&utm_source=abnewswire.com&utm_medium=paidpr]
Top Companies in the Vector Database Market
The prominent players across all service types profiled in the vector database market’s study include Microsoft (US), Elastic (US), Alibaba Cloud (China), MongoDB (US), Redis (US), SingleStore (US), Zilliz (US), Pinecone (US), Google (US), AWS (US), Milvus (US), Weaviate (Netherlands), and Qdrant (Berlin) Datastax (US), KX (US), GSI Technology (US), Clarifai (US), Kinetica (US), Rockset (US), Activeloop (US), OpenSearch (US), Vespa (Norway), Marqo AI (Australia), and Clickhouse (US).
Microsoft is a prominent global information technology leader, providing software and diverse licensing suites. The company develops and maintains software, services, devices, and solutions. Its product offerings include Operating Systems (OS), cross-device productivity applications, server applications, business solution applications, desktop and server management tools, software development tools, and video games. The company also designs, manufactures, and sells devices like PCs, tablets, gaming and entertainment consoles, other intelligent devices, and related accessories. It offers a range of services, which include solution support, consulting services, and cloud-based solutions. The company also offers online advertising. Microsoft is a global leader in building analytics platforms and provides production services for the AI-infused intelligent cloud. It generates revenue by licensing and supporting a range of software products. Microsoft caters to various verticals, including finance and insurance, manufacturing and retail, media and entertainment, public sector, healthcare, and IT and telecommunications. It has a geographical presence in more than 190 countries across North America, Asia Pacific, Latin America, the Middle East, and Europe. In November 2020, the company pledged a USD 50 million investment in the ‘AI for Earth’ project to accelerate innovation. As large-scale models become potent platforms, the company continues to bring rich AI capabilities directly into the data stack. In the past year, OpenAI achieved advanced training models such as GPT-3-the world’s largest and most advanced language model-on Azure AI supercomputer. Microsoft exclusively licensed GPT-3, allowing it to leverage its technical innovations to deliver cutting-edge AI solutions for its customers and create new solutions that harness the power of advanced natural language generation.
Alibaba Group operates as an online and mobile commerce company. Alibaba Cloud is a cloud computing arm and a BU of the Alibaba Group. Alibaba Cloud, founded in 2009, has headquarters in Hangzhou, China. It is a publicly held company and operates as a subsidiary of Alibaba Group. It offers cloud computing services, such as database, elastic computing, storage and Content Delivery Network (CDN), large-scale computing, security, and management and application services. Alibaba Cloud provides a comprehensive suite of cloud computing services to power international customers’ online businesses and Alibaba Group’s eCommerce ecosystem. Alibaba Cloud’s global operations are registered and headquartered in Singapore. The company has international teams stationed in Dubai, Frankfurt, Hong Kong, London, New York, Paris, San Mateo, Seoul, Singapore, Sydney, and Tokyo. As of 2019, Alibaba Cloud has 55 availability zones across 19 regions worldwide. AnalyticDB for PostgreSQL provides vector analysis to help implement approximate search and study of unstructured data. AnalyticDB for PostgreSQL vector databases is a DBMS that integrates the in-house FastANN vector engine. AnalyticDB for PostgreSQL vector databases also provides end-to-end database capabilities such as ease of use, transaction processing, high availability, and high scalability.
Elastic, based in the US, is renowned for its Elastic Stack, which includes Elasticsearch, a highly scalable search and analytics engine designed for storing, searching, and analyzing structured and unstructured data in real-time. While Elasticsearch is not a traditional vector database per se, its capabilities in handling large volumes of data with near-instantaneous search and analysis make it relevant in contexts requiring fast retrieval and analysis of vectors or similar data structures. Elastic’s solutions are widely used across industries for logging, security information and event management (SIEM), application performance monitoring (APM), and more, emphasizing flexibility and scalability in data management and analytics.
Weaviate, based in the Netherlands, specializes in providing a scalable and flexible vector database designed specifically for handling large-scale, complex data sets. It leverages a schema-first approach to organize data into structured vector representations, enabling efficient querying and retrieval of complex relationships and patterns within the data. Weaviate’s database is optimized for handling high-dimensional vectors and supports advanced search capabilities, making it suitable for applications requiring real-time analysis, natural language processing (NLP), recommendation systems, and other AI-driven use cases. Their platform emphasizes the integration of machine learning models and IoT devices, facilitating the creation of intelligent, data-driven applications across various domains.
MongoDB, headquartered in the US, is a prominent player in the vector database market. MongoDB offers a robust document-oriented database that supports JSON-like documents with dynamic schemas, making it highly flexible for handling complex data structures and unstructured data. In the vector database market, MongoDB provides features that cater to real-time analytics, high-speed transactions, and scalability across distributed systems. Its capabilities in managing large volumes of data efficiently and its ability to integrate with various programming languages and frameworks position MongoDB as a versatile choice for organizations seeking scalable and performant database solutions in the vector database market.
Media Contact
Company Name: MarketsandMarkets Trademark Research Private Ltd.
Contact Person: Mr. Rohan Salgarkar
Email:Send Email [https://www.abnewswire.com/email_contact_us.php?pr=vector-database-market-worth-43-bn-by-2028-key-companies-include-microsoft-elastic-mongodb-redis-singlestore]
Phone: 18886006441
Address:1615 South Congress Ave. Suite 103, Delray Beach, FL 33445
City: Florida
State: Florida
Country: United States
Website: https://www.marketsandmarkets.com/Market-Reports/vector-database-market-112683895.html
Legal Disclaimer: Information contained on this page is provided by an independent third-party content provider. ABNewswire makes no warranties or responsibility or liability for the accuracy, content, images, videos, licenses, completeness, legality, or reliability of the information contained in this article. If you are affiliated with this article or have any complaints or copyright issues related to this article and would like it to be removed, please contact retract@swscontact.com
This release was published on openPR.
Article originally posted on mongodb google news. Visit mongodb google news
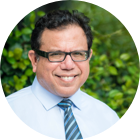
MMS • RSS
Posted on nosqlgooglealerts. Visit nosqlgooglealerts
About two years ago, popular cache database Redis was among a wave of vendors that added vector search capabilities to their platforms, driven by the surge of interest in generative AI.
Vector embeddings are produced by foundational models such as OpenAI’s ChatGPT and are used to represent chunks of language – like words or phrases. The idea is to add vector search to a database, which allows enterprise systems to store, index, and search millions of vectors extending beyond the foundational models to enterprise data.
Since 2023, so many database systems have announced vector search as a core feature that it hardly distinguishes them from the competition. For example, MongoDB, Cassandra, PostgreSQL, Snowflake, and SingleStore all announced the same feature in the same year.
Vendors are now trying to differentiate themselves with more in-depth features in the race to become indispensable in the modern AI stack.
For example, Redis announced LangCache. The fully managed REST service is designed to reduce expensive and latency-prone calls to LLMs by caching previous responses to semantically similar queries.
“It sits between your application and whatever LLMs you’re using, and you pass in the query, and we see if there’s a response that matches, and then we just hand it back to you instead of hitting the LLM inferencing engine,” Redis CEO Rowan Trollope told The Register earlier this month.
YugabyteDB, the PostgreSQL-compatible database with a distributed back end, is also on a mission to more deeply adapt its software to support AI. With Paramount, GM, and Kroger among its customers, the vendor said that integrating vector indexing libraries including Usearch, HNSWLib, and Faiss would boost the performance of the PostgreSQL vector search extension, pgvector.
Founder and co-CEO Karthik Ranganathan told The Register that Yugabyte had replicated and auto-sharded these indexes on its distributed back end to accelerate the performance of pgvector. He argued that while pgvector had the massive community of PostgreSQL behind it, it was reimplementing vector search in the longstanding relational system. It needs performance enhancements to keep up with specialist vector databases.
“Everything in the AI world is going really fast, so we started directly interfacing with some of these open source libraries,” he said.
Gartner said last year that “moonshot” AI projects in business were seeing high failure rates. Expectations of what GenAI could achieve in business would come down, and 2025 was set to be the “year of the slide.”
In other research, Gartner warned that a lack of preparedness in data infrastructure was hampering progress in bringing GenAI to business problems. It predicted that in 2025 and 2026, organizations would abandon 60 percent of AI projects because their data wasn’t ready.
Roxane Edjlali, senior director analyst at Gartner, said that including vector data stores was one of the ways organizations could help extend and improve data management to support new use cases such as GenAI.
“Remember that AI-ready data is not ‘one and done.’ Think of it as a practice where the data management infrastructure needs constant improvement based on existing and upcoming AI use cases,” she said.
She recommended that organizations invest in AI, develop an AI-ready data practice, and ensure continued investment and ongoing maturity in metadata management, data observability, analytics, and AI governance.
Redis and Yugabyte are transactional systems looking to add AI support. The dominant transactional database, in terms of deployments, is Oracle, which analysts describe as an early leader in bringing natural language vector search capabilities to the relational data in business systems.
Yet analytics system vendors are also striving to update their technology to meet the requirements of GenAI.
Teradata has a 40-year history in business intelligence and data warehousing and counts HSBC, Unilever, and American Airlines among its customers. Last year, it announced it was integrating Nvidia NeMo and NIM microservices into the Vantage cloud platform to accelerate AI workloads and support the development of foundation and customized large language models (LLMs) and retrieval-augmented generation (RAG) applications.
Further back, Teradata began investing in machine learning about the time it bought specialist analytics vendor Aster for $263 million.
Martin Willcox, VP of analytics and architecture at Teradata, told The Register that demand for analytics and BI services is rising as businesses explore AI agents and LLM-powered customer interactions. He said Teradata’s Massively Parallel Processing (MPP) shared-nothing architecture is capable of running inference for AI models in the 150 to 250 million parameter range. Terdata is, we’re told, also improving its API integration with hyperscalers to support their LLMs.
At the same time, clients are creating large stores of unstructured data – images, audio, PDFs, or email, for example – and employing vector search on top to make sense of their own information in natural language, in combination with LLMs.
“Current vector database technologies essentially come in two flavors,” Willcox said. “There are special-purpose technologies which perform well for small datasets, but don’t always scale and quite often lack enterprise fit and finish. Secondly, there are Model-View-Presenter (MVP) frameworks like Apache Spark, which can scale linearly for these tasks, but often perform pretty poorly for them. And so we think there’s a gap in the middle for a vector store that both scales and performs and has all the traditional fit and finish that you would expect of an enterprise-class database management system.
“When you see what our customers do [with AI agents] that also means more BI, more predictive machine learning. Agents by themselves are very sophisticated models, but entirely ignorant of organizational context. In order to make any kind of worthwhile decisions at all, they have to ask lots of questions of back-end database systems.”
SingleStore – formerly known as MemSQL – has built a database that supports both OLTP and OLAP in a single system and counts Uber, Kellogg’s, and engineering giant GE among its customers. It too has anticipated the demand on databases to support GenAI and has supported vector search since 2017 using an exact-nearest-neighbor approach. Last year, it previewed indexed approximate-nearest-neighbor (ANN) search, a move it claimed offers orders-of-magnitude faster vector search, and makes it easier for developers to build applications.
Technical evangelist Akmal Chaudhri said SingleStore has 400 customers globally, and around 45 use the company’s GenAI capabilities to some degree, including vector search. “They cover a wide range of verticals, many of them in highly regulated industries as well. They build chatbots specifically for that environment,” he said.
“A lot of them are using the vector capabilities simply because it gives you more than just doing sort of metadata filtering – using SQL, for example, or just searching on individual words. It’s really trying to get more context out of what we have. Lots of organizations today have huge amounts of data and don’t know what to do with it, and so this gives them the opportunity to kind of interrogate the data.”
According to a survey by Gartner, 63 percent of organizations say they either do not have or are unsure if they have the right data management practices for AI. It has also pointed out that despite the current obsession with GenAI, there are other kinds of machine learning that can solve business problems. Simulation, for example, is better suited to planning and forecasting than GenAI, Gartner pointed out (see its slide below).
At the same time, it reckons the overall DBMS market – an already mature software category – is expected to grow by 16 percent in 2025, to hit a value of around $137 billion. The vendors are likely to continue to chase customer spending by attempting to show how they can help AI solve business problems. ®
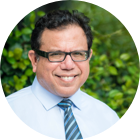
MMS • RSS
Posted on mongodb google news. Visit mongodb google news
Shares of MongoDB Inc (MDB, Financial) surged 6.67% in mid-day trading on Apr 24. The stock reached an intraday high of $174.83, before settling at $173.51, up from its previous close of $162.66. This places MDB 55.19% below its 52-week high of $387.19 and 23.25% above its 52-week low of $140.78. Trading volume was 1,706,970 shares, 61.2% of the average daily volume of 2,787,846.
Wall Street Analysts Forecast
Based on the one-year price targets offered by 34 analysts, the average target price for MongoDB Inc (MDB, Financial) is $283.92 with a high estimate of $520.00 and a low estimate of $170.00. The average target implies an upside of 63.63% from the current price of $173.51. More detailed estimate data can be found on the MongoDB Inc (MDB) Forecast page.
Based on the consensus recommendation from 38 brokerage firms, MongoDB Inc’s (MDB, Financial) average brokerage recommendation is currently 2.0, indicating “Outperform” status. The rating scale ranges from 1 to 5, where 1 signifies Strong Buy, and 5 denotes Sell.
Based on GuruFocus estimates, the estimated GF Value for MongoDB Inc (MDB, Financial) in one year is $432.89, suggesting a upside of 149.49% from the current price of $173.51. GF Value is GuruFocus’ estimate of the fair value that the stock should be traded at. It is calculated based on the historical multiples the stock has traded at previously, as well as past business growth and the future estimates of the business’ performance. More detailed data can be found on the MongoDB Inc (MDB) Summary page.
This article, generated by GuruFocus, is designed to provide general insights and is not tailored financial advice. Our commentary is rooted in historical data and analyst projections, utilizing an impartial methodology, and is not intended to serve as specific investment guidance. It does not formulate a recommendation to purchase or divest any stock and does not consider individual investment objectives or financial circumstances. Our objective is to deliver long-term, fundamental data-driven analysis. Be aware that our analysis might not incorporate the most recent, price-sensitive company announcements or qualitative information. GuruFocus holds no position in the stocks mentioned herein.
Article originally posted on mongodb google news. Visit mongodb google news
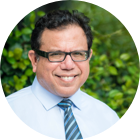
MMS • RSS
Posted on mongodb google news. Visit mongodb google news
Financial giants have made a conspicuous bullish move on MongoDB. Our analysis of options history for MongoDB MDB revealed 18 unusual trades.
Delving into the details, we found 61% of traders were bullish, while 33% showed bearish tendencies. Out of all the trades we spotted, 3 were puts, with a value of $190,928, and 15 were calls, valued at $1,031,973.
What’s The Price Target?
Analyzing the Volume and Open Interest in these contracts, it seems that the big players have been eyeing a price window from $140.0 to $290.0 for MongoDB during the past quarter.
Volume & Open Interest Development
Assessing the volume and open interest is a strategic step in options trading. These metrics shed light on the liquidity and investor interest in MongoDB’s options at specified strike prices. The forthcoming data visualizes the fluctuation in volume and open interest for both calls and puts, linked to MongoDB’s substantial trades, within a strike price spectrum from $140.0 to $290.0 over the preceding 30 days.
MongoDB 30-Day Option Volume & Interest Snapshot
Noteworthy Options Activity:
Symbol | PUT/CALL | Trade Type | Sentiment | Exp. Date | Ask | Bid | Price | Strike Price | Total Trade Price | Open Interest | Volume |
---|---|---|---|---|---|---|---|---|---|---|---|
MDB | CALL | TRADE | BEARISH | 12/17/27 | $42.6 | $38.35 | $38.35 | $290.00 | $141.8K | 73 | 90 |
MDB | CALL | TRADE | BULLISH | 09/19/25 | $26.95 | $25.0 | $26.5 | $180.00 | $132.5K | 245 | 153 |
MDB | CALL | TRADE | BULLISH | 09/19/25 | $26.6 | $24.55 | $26.2 | $180.00 | $117.9K | 245 | 102 |
MDB | CALL | SWEEP | BEARISH | 05/16/25 | $5.85 | $5.5 | $5.5 | $180.00 | $110.9K | 986 | 210 |
MDB | CALL | SWEEP | BULLISH | 06/20/25 | $3.2 | $2.65 | $3.15 | $230.00 | $102.4K | 376 | 341 |
About MongoDB
Founded in 2007, MongoDB is a document-oriented database. MongoDB provides both licenses as well as subscriptions as a service for its NoSQL database. MongoDB’s database is compatible with all major programming languages and is capable of being deployed for a variety of use cases.
Following our analysis of the options activities associated with MongoDB, we pivot to a closer look at the company’s own performance.
MongoDB’s Current Market Status
- With a volume of 1,074,806, the price of MDB is up 5.74% at $172.0.
- RSI indicators hint that the underlying stock is currently neutral between overbought and oversold.
- Next earnings are expected to be released in 35 days.
Professional Analyst Ratings for MongoDB
A total of 5 professional analysts have given their take on this stock in the last 30 days, setting an average price target of $246.0.
Turn $1000 into $1270 in just 20 days?
20-year pro options trader reveals his one-line chart technique that shows when to buy and sell. Copy his trades, which have had averaged a 27% profit every 20 days. Click here for access.
* Consistent in their evaluation, an analyst from Truist Securities keeps a Buy rating on MongoDB with a target price of $275.
* Maintaining their stance, an analyst from Piper Sandler continues to hold a Overweight rating for MongoDB, targeting a price of $200.
* An analyst from Citigroup has decided to maintain their Buy rating on MongoDB, which currently sits at a price target of $330.
* Consistent in their evaluation, an analyst from Mizuho keeps a Neutral rating on MongoDB with a target price of $190.
* An analyst from Morgan Stanley persists with their Overweight rating on MongoDB, maintaining a target price of $235.
Options trading presents higher risks and potential rewards. Astute traders manage these risks by continually educating themselves, adapting their strategies, monitoring multiple indicators, and keeping a close eye on market movements. Stay informed about the latest MongoDB options trades with real-time alerts from Benzinga Pro.
Market News and Data brought to you by Benzinga APIs
© 2025 Benzinga.com. Benzinga does not provide investment advice. All rights reserved.
Article originally posted on mongodb google news. Visit mongodb google news
Presentation: Lessons & Best Practices from Leading the Serverless First Journey at CapitalOne
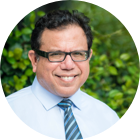
MMS • George Mao
Article originally posted on InfoQ. Visit InfoQ
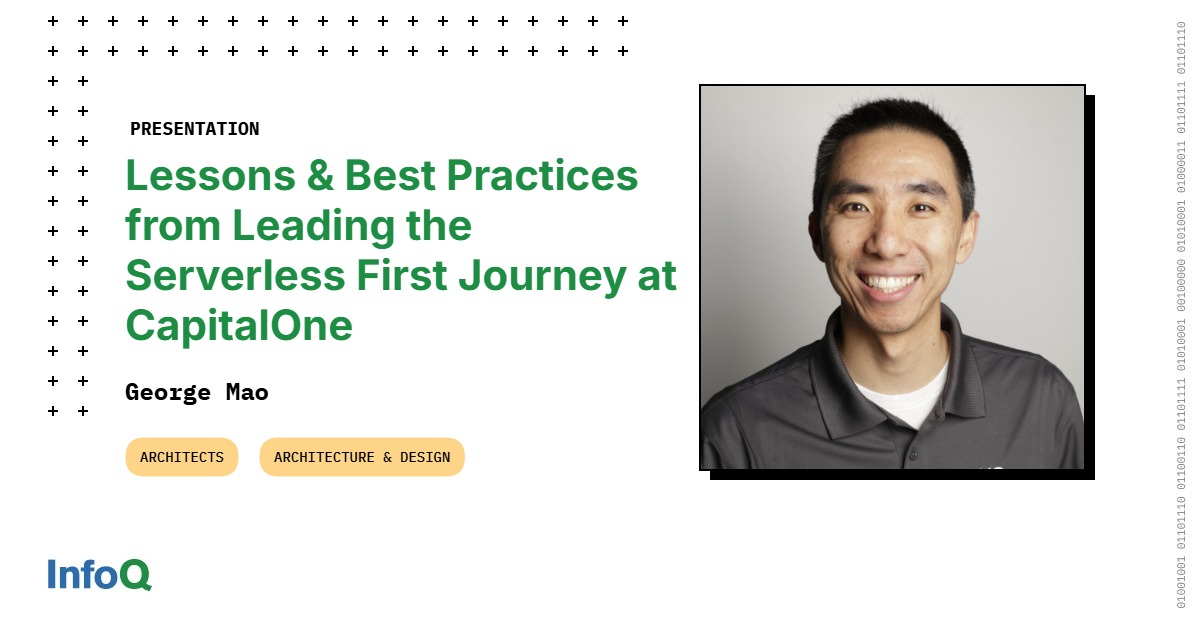
Transcript
Mao: My name is George. I am currently a Senior Distinguished Engineer at Capital One. I lead a lot of our AWS serverless technology implementations. I’m responsible for helping our teams implement best practices and everything we do on AWS. Before I joined Capital One, I was the tech leader at AWS for serverless computing, so I spent a lot of time, basically since the beginning of 2015, where serverless was first created at Amazon.
Capital One is one of the largest banks in the United States. We’re generally somewhere at 10 or 11 in terms of ranking. We’re not that big internationally. We do have a pretty good presence in the UK, but that’s about it. What’s unique about us is we’re mostly structured like a tech organization, so we have about 9,000 software engineers. In 2020, we completed our all-in migration into AWS. As far as I know, I think we’re one of the only major banks in the world that has ever done an all-in like this. Now what we’re trying to do is modernize our entire tech stack running in the cloud. What that means is becoming more cloud-native, taking advantage of all of the AWS managed services, and then becoming more efficient in the cloud.
Outline
This is what we’re going to talk about. I’ll cover why we decided to make this journey. In chapter 2, we’ll talk about some of the lessons that we’ve learned, and I’ll share with you so that you might not run into some of the trouble that we ran into. Then we’ll go through a bunch of best practices that you can take home and implement in your organizations.
Chapter 1: Why Did Capital One Adopt a Serverless-First Approach?
Why did Capital One adopt a serverless-first approach? Many of you are in the financial industry, in banking, or in related industries. Capital One has a ton of regulations and a ton of things that we have to follow to meet our auditing and compliance needs. A lot of that stuff stems from vulnerability assessments, to addressing problems and all kinds of issues that we find that has to be addressed immediately. An example is like, every 60 to 90 days, we have to rehydrate an EC2 instance, regardless of what we’re doing with that instance. By our measurements, on an average team of 5 engineers, that team spends 20% of our time simply working on EC2, delivering things that don’t really add value, but we have to do because of the industry that we’re in. This is basically the gold standard of a traditional architecture that Amazon tells us to implement.
For highly available, you would deploy EC2 instances across multiple availability zones, two at least, at Capital One we do at least three. Then you would just create autoscaling groups so that they can spin up and down as they need. The goal here is to allow Amazon to handle the scaling of your instances based on metrics or failure. Then you have load balancers and NAT gateways ahead of them so that they can front your traffic and then spread load across your clusters. When you have an environment like this, think about the things that you have to maintain. This is just a small list. We have to maintain the EC2 infrastructure, the networking behind it, all the IP addresses, the VPC subnets, the AMIs that go on to the instances, updates, patches, scaling policies, everything that is in that picture, some engineer has to touch. What you’ll notice is none of this stuff adds any value to your customers. All of this is basic needs that you have to deliver to make your applications work in a traditional architecture.
Pre-serverless, our responsibility looked like this. We would deploy stuff to the cloud, and then we’d deploy infrastructure to AWS, and what that really means is EC2 compute. We’d choose operating systems that go on the EC2 instances. Then, generally, we containerize our applications. I think that’s becoming the standard these days. Then we run app servers on these containers. This is a tried-and-true method that most enterprises run today. Then we deploy our business apps that run on top of them. When you go to capitalone.com, all of the stuff that the customers see go top-down through this stack. Everything below business apps is what we call run-the-engine tasks, so things that are necessary behind the scenes to even begin deploying applications on top. If you talk to AWS, they’ll use a term called undifferentiated heavy lifting.
If anybody has spoken to AWS people, they like to say that a lot. It’s basically things that your developers hate doing. I don’t know how to do any of this stuff. I know how to write app code. I’m not a EC2 engineer. When you move into serverless, your architectures generally are event-based, and they really become one of three types. Synchronous, an example would be, you create a REST API. Requests come through API Gateway, and then API Gateway drives requests to your Lambda functions. An example would be, maybe you have an order submitted on your website, and that’s an event, but that’s a synchronous event because it needs to return an order ID to your customer who is waiting for that confirmation. If you can do asynchronous workloads, that’s even better, because then you can decouple the work that’s happening at the frontend with what’s happening at the backend. Has anybody purchased something from amazon.com before? I have a package arriving every other day or something at my garage.
All the orders are asynchronous. You click order, your credit card isn’t charged immediately, they have an order processing system. They can take hundreds and millions of orders without even having a system up on the backend that’s processing them. It’s decoupled and asynchronous. That’s actually the best way to write serverless applications. The last piece is poll-based. One of the best and unknown features of AWS is they have something called a poller system. A poller system is their fleet of workers that will poll certain event sources on your behalf and deliver records from those event sources to your Lambda functions. You don’t have to do any of that work. Examples are DynamoDB, Kinesis, SQS, anything that’s in those data sources, AWS will poll and deliver to you. That removes all of the scaling and the need that you have to do in order to process those events.
If you look at serverless architectures, generally, all of that stuff at the bottom is just handled by AWS. We don’t have to do any of that stuff. We just decide, do we want to run Lambda, which is Functions as a Service, or Fargate, which is Containers as a Service. Then, we just write our business logic right on top of that. Our engineers are basically only working with that top box. The first thing they do is write application code. They don’t have to worry about patching and operating systems and all that stuff. Engineers love this. Our developers really like this type of development. That means there’s no more burden on our developers. All of that time spent doing all those EC2 activities are just entirely gone. We all know that human costs are generally the most expensive piece of any application team. That’s why we moved into serverless. Today, we are trying to be serverless first, everywhere, where possible. That’s our goal. We’re still pushing forward into that space.
Chapter 2: Lessons Learned, and the Launch of Our Serverless Center of Excellence
We’ve learned a lot of lessons, and I’ll share some with you, so that if you’re doing this exercise, you won’t run into some of the challenges that we learned. There is going to be a learning curve. A beginner serverless developer generally will write Lambda functions in the console. Who’s done this before? You can write app code directly in the console. It’s really cool because you can save it and execute that function immediately. The bad news is there’s no CI/CD, and this goes right out to production if it’s there, and you can change it at any time without any version control. You also can’t debug or trace a Lambda function in the console.
For those who have worked on Lambda, there is no way to debug or trace. What do you do? Basically, you write print statements everywhere. Don’t copy this code and put it into production, but all it’s doing is writing print statements so that I can see the value of these variables that I have. Back when Lambda was first released, this was the only way to test functions. Everybody did this because there was no other way to test functions. Today, there’s a tool called SAM. It’s the Serverless Application Model. It comes in two pieces. One is the CLI, which you install locally on your machine. What that will do is you’ll basically install an image of the Lambda container on your machine as a Docker image. This will allow you to run your Lambda functions locally exactly as it would be in the AWS environment. That means you’ll see log generation. You’ll see exactly the same thing you would see if you ran it live in AWS.
Second, you can use SAM to perform your CI/CD deployment. It’ll do deploys. It’ll do code synchronization. It’ll do everything that you can do to push it through your development stack. If anybody has used CloudFormation, it’s pretty verbose. You can have a 50-page template for your application. That’s not great. What Amazon has done is they’ve created a shorthand syntax for serverless components that make it a lot more concise. Here’s an example. I’m writing two Lambda functions. First one is called FooFunction. Second one is called BarFunction. They’re both Node.js based 16, both memory size 128. Entry points are defined by the handler property. Just with five lines of code for each function, this will deploy into AWS without a massive CloudFormation template. The backend AWS is translating this to a real CFT, CloudFormation. You don’t have to worry about any of that translation. We use this everywhere. I encourage all of our engineers to move to this method because you can test applications really easily.
The next thing that was new for us is that the unit of scale for Lambda is concurrency. That’s a brand-new concept to almost everybody touching serverless. The traditional unit of scale is TPS, RPS, transactions per second, requests per second. That drives how wide you need to scale your EC2 cluster. With Lambda, it’s a little bit different. Concurrency is the number of in-flight requests that your Lambda functions are processing at any given second. Lambda only bills us when we run them. If you’re not running anything, there’s no cost. That’s really cool. What that means is when you’re not running anything, there are no environments available to run your functions. The very first time you have to run your function, it goes through something called a cold start.
A cold start is all of the work Amazon has to do to bring your code into memory, initialize the runtime, and then execute your code. That pink box right there is all of the overhead that’s going to happen before your function can begin executing. Once your function’s warm, the second time it’s invoked, it doesn’t have to go through that method. It’s going to be warm, and that’s what Amazon people will talk to you about as warm starts. The second invoke is going to be really fast. This will drive your concurrency across your entire fleet of Lambda functions. You could have 1,000 concurrent functions that you need to scale to 2,000. All of those new containers are going to go through this cold start. Keep that in mind. That’s usually the first thing Lambda engineers run into. I talk about this formula all the time with our engineers. This is the formula that Amazon uses to measure concurrency, and it’s average requests per second, TPS, driven to Lambda, multiplied by the average duration in seconds.
If you look at these three examples here, we’re all driving 100 TPS, RPS. These Lambda functions run at about half a second, so 500 milliseconds. That means your concurrency needs are going to be 50. It actually drives down your concurrency needs because you’re running for under a second. If you double your duration to 1 full second, your concurrency now is going to be 100. If you double that again, same TPS, but now you’re running for 2 seconds, your concurrency needs are 200. You’re going to need 200 warm containers serving all of this traffic, and you have to be able to scale into that. This is a concept that you’ll likely have to work through as you walk into your serverless journey.
The next thing here is, before we ran into serverless, or started working on serverless, our infrastructure costs were generally managed by our infrastructure team, and our developers were not really concerned with cost. With Lambda, everybody is responsible for cost. At re:Invent 2023, one of the 10 top tenets that Amazon gave us was, everybody is responsible for cost, and that’s 100% true when you move into serverless. Lambda has two pricing components.
First is number of invocations per month, and it’s tiny, it’s 20 cents per million. We don’t even look at this. This first component of the formula, we just ignore, because it’s basically dollars. The second is compute. If compute is measured in gigabyte seconds, and that sounds complicated, but gigabyte seconds is the combination of memory allocated to your function multiplied by the duration that function runs for. Memory allocated in megabytes times the milliseconds that that function runs for. The bottom line there is just focus on the compute cost. The number of invocations is relevant. You can run 1 million invokes for free on every account forever. If you’re under that, you could run Lambda for very cheap. Going along the same thing that we learned is every Lambda function operates and generates a report structure in CloudWatch logs every single time it’s invoked. There’s always going to be a start, always going to be an end, and always going to be a report line. The report line is the most important line that you should be aware of.
What you’re going to see there is, at the bottom in the report line, they’ll give you all of the metrics that you need to understand how your function executed. One of the most important ones is duration. This function ran for, it’s a little bit small, but 7.3 milliseconds. It was billed for 8 milliseconds. Anybody know why? Lambda rounds us up to the nearest 1 millisecond. It’s the most granular service that AWS, or I think any cloud provider offers. Everybody else is either at 1 second or 100 milliseconds. This really represents pay-for-use. It’s the best service that we can find that’s pay-for-use. I configured this function at 256 megs, max memory used is 91 megs. Remember, Amazon bills us at memory configured, not used. This is a piece of confusion that my engineers run into a lot. It doesn’t matter if you use 1 out of a gig, Amazon’s going to bill you for a gig of memory. We’ll get into that. Sometimes there’s a great reason for why you might overprovision memory.
Capital One, we operate thousands of accounts. We have over 1000 accounts. We have tens of thousands of Lambda functions spread out across those accounts, which means we have to be able to handle compliance. We have to be able to control these functions. We have to have standards so we can do these things. Metrics and logs, we have to understand how long to save them for and be able to maintain these functions.
In order to do that, we learned that we needed to create a center of excellence because what we were doing before was, we were making isolated decisions across single lines of businesses that would affect other lines of businesses. That creates tech debt and it creates decisions that have to be unrolled. We created a center of excellence and now we use it to basically talk to all of our representatives in each line of business that we can make a correct decision. I’ll talk through some examples that we’ve worked on.
Some of the sample things that our center of excellence leads is everything from Lambda defaults. What should a Lambda default be? What are the programming languages that we even allow? What are their naming conventions or the default memory settings that we’re going to choose? AWS regularly deprecates runtimes because Java 8 is deprecated. They don’t want to support Java 8. We also talk about how we want to deprecate our runtimes because if we wait too long and Amazon’s deprecated theirs, we’re not going to be able to deploy on these deprecated runtimes anymore. The center of excellence also handles something really important, which is training and enablement. We host a serverless tech summit twice a year. We have internal certifications on serverless. We have continuous enablement, and to educate our engineers on a regular basis.
Here’s an example of a development standard. You can create an alias that points to a Lambda function, and that alias is just like a pointer. You can use that to invoke your function. We mandate that every development team uses a standard alias called LIVE_TRAFFIC. That is the only entry point for my function. What this does is it allows me to jump across any development team and understand where this function is executed from and what all the permissions are. I work across every dev team that exists at Capital One, and this helps me a lot. Many other people could be transitioning from one team to another and they can easily onboard really quickly. Another thing that we standardize is we require versioned rollouts for all Lambda functions so that we can roll back if there’s a problem. We require encryption on our environment variables. We don’t want to have sensitive data exposed in environment variables.
Other thing is, if you’re working at AWS, you can tag nearly every resource out there. It’s just a key-value pair to give you some metadata. Basically, we have a set of standardized tags that will help us understand who owns this application, who to contact if there’s a problem, and who gets paged, essentially. Some other things here, IAM, we have some standardized rules on IAM and what you can and can’t do. Mostly is with no wildcards anywhere in your IAM policies.
Then, we have open-sourced an auditing tool called Cloud Custodian. It’s just cloudcustodian.io, but we actually use this to audit all of these rules that we’re putting in place. If anybody deploys anything that doesn’t meet these standards, it immediately gets caught. Also, I highly encourage you to use multi-account strategies. What we do is we deploy an account per application group. Then, we give that application group multiple accounts representing each development tier, so dev all the way through pod. What that allows you to do is separate blast radius, but also give you separate limits, AWS limits on every account.
Chapter 3: Best Practices for All – Development Best Practices
We’re going to talk about best practices that I’ve learned throughout 10 years of working with serverless. We’ll start with development best practices. Here’s a piece of sample code. Basically, the concept here is, don’t load code until you need it, so lazy load when you can. If you look at the top here, the very first line, that is a static load of the AWS SDK, just the DynamoDB client, and it’s just some SDKs allowing me to list tables in my account. It’s going to do that on every single invocation of this function, but if you look at the handler method, down below, there are two code paths. The first code path actually will use this SDK. It’s going to do this interaction with Dynamo. The second code path isn’t going to do anything with Dynamo.
However, on every invoke of this function, any cold start is going to load in this SDK. 50% of my invocations, in this case, are going to go through a longer cold start because I’m pulling in bigger libraries and more things than I need. What you can do, a really good strategy is lazy load. In the same example, if you define the same variables up ahead in the global scope, but you don’t initialize them, down in the handler method, you can initialize those SDKs only when you need them, so on the first code path, right there, that first if statement. What you need to do is you need to check if those variables are initialized already.
If they’re already initialized, don’t do it again. This is going to avoid extra initialization, and 50% of the time, it’s going to go to the second code path. You need to look at the profile and anatomy of your function and see what code path your applications are following. If you have anything that has separate paths like this, I highly encourage you to lazy load what you can, not just the SDK, but anything else that you might be using as dependencies.
Next concept is, use the right AWS SDK. If you look at Java, the version 1 of the Java SDK was created before Lambda was even in existence. What that meant was the SDK team had no idea that they needed to optimize for Lambda. That SDK is 30-plus megs. If you were to use the version 1 Java SDK, you’re going to have 30 megs of dependencies. Use all of the latest SDKs for Java. You want to use version 2. It allows you to modularize and only pull in the pieces that you need. Same thing with Node. For those who are using Python, you’re lucky. They do upgrade in place on Boto3, so you don’t have to do anything. We continue to use Boto3. Next thing here is, try to upgrade to the latest runtimes for Lambda. Because what Amazon does is they will upgrade the images they use behind the scenes. What you’ll notice is the latest runtimes for Lambda, so Node 20, Java 21, and Python 3.12 and beyond, use what Amazon calls Amazon Linux 2023. That image is only 40 megs. Everything else before uses AL2, Amazon Linux 2, which is 120 megs.
Behind the scenes, it’s just a lot more efficient. You’re going to cold start better, perform a lot better. Then, I know you guys have Java 8 running around everywhere. We did. We still do. If you can get out of it, simply moving from Java 8 to Java 17 gives you a 15% to 20% performance boost. That’s a free upgrade if you can get there. Next is just import what you need. Don’t import extra things like documentation and sample code and extra libraries, because in AWS, when you’re running these, they’re not going to be useful. You’re not going to be able to read them.
An example here, this is a Node package.json. I accidentally imported mocha, which is my test suite, and esbuild. None of those things are going to be useful when I’m running my Lambda function. All they’re going to do is add to the package size. Lambda actually has a package size limit. You can only deploy a 50-meg zip or 250 megs uncompressed. If you have too many libraries, you’re going to run into this limit and you’re not going to be able to deploy.
One of Gregor Hohpe’s main concepts is always to use AWS configuration and integration instead of writing your own code where possible. Think about this piece of architecture where if your Lambda function needs to write a record to Dynamo, and then there’s some other resource waiting to process that record, we could do it like this, where the Lambda function first writes to Dynamo, it waits for the committed response, and then it publishes a notification to SNS or SQS telling the downstream service that, ok, we’re done and we’re ready to process.
Then that downstream service may live on Lambda or EC2, wherever, and then it goes and queries the Dynamo table and processes the work. This is a fully functional app, it’ll work, but we can do better. What I would do is take advantage of out-of-the-box AWS features. You can write to Dynamo, and then within Dynamo, there’s a feature called DynamoDB Streams, and it’s basically a stream of changes that have happened on that table. You can set up Lambda to listen to that stream, so you don’t even have to poll the stream. All you’re really doing in this example is two Lambda functions: one is writing, one is receiving events. You’re not even polling. These will be cheaper, faster, easy to scale. In general, think about your application architectures and try to move towards this type of architecture. Use Lambda to transform data, not to move data. That’s the key principle that we have.
Then, the last development tip I have here is establish and reuse. Objects that are going to be used more than once should only be loaded once, globally. Every Lambda function has an entry point, and it’s called the handler method, right there in the middle. Everything outside of that is global scope. During a cold start, everything above that will be executed. During a warm start, entry point begins right at the handler method. All of the global scope stuff is held in memory and ready to go. A lot of times, we have to pull secrets in order to hit some downstream system. Secrets don’t change that often. What you can do is load it once during global scope and reuse it every time you get warm invokes down below. Just make sure you’re checking to see if that warm secret is available, not expired, and ok to use. You can use the same concept for pretty much anything that can be reused across Lambda invocations.
Build and Deploy Tricks
Next part is some tips on how to build and deploy your Lambda functions. We talked a little bit about this. Make sure you’re deploying small packages, as small as possible. Minify, optimize, and remove everything that you don’t need. Here’s a Node-based Lambda function. It’s written in SAM, which we talked about earlier. The name is called first function. It’s pretty basic. It’s just a Node.js function, memory size 256, and it’s using something called arm64 as the application, the CPU architecture. We’ll talk a little bit about that. This is a strategy for how I can build a really optimized function. I’m using esbuild.
For those who are doing Node stuff, esbuild is a very common build process. Once I use esbuild, it will create and generate a single minified file for deployment, and it will combine all dependencies and all source code into a single file. It’s not going to be human-readable, which doesn’t really matter, because you can’t debug in production anyways. I’m formatting as an es module, and then I’m just outputting into the esbuild. When I do an esbuild, this function is 3.3 megs in size. It’s got the AWS SDK in it, and it’s tiny. If I don’t do esbuild, it’s a 24-meg package, a standard zip. This is zipped and compressed with the AWS SDK. I have almost no source code in this, 24 megs. The largest I can get to is 50, so I’m already almost halfway there just because I included the AWS SDK. If we look at performance, this is a screenshot of a service called AWS X-Ray. X-Ray gives me a trace of the entire lifecycle of my function’s invocation. You can see it topped it down.
The first line is initialization, and that’s the cold start time my function took to really become ready to run. This is my esbuild function, and it took 735 milliseconds to cold start. The actual runtime was 1.2 seconds, so 1.2 minus 735 milliseconds is the actual invocation of my function. If we look at my standard zip file build for that function, it was at over 1,000 milliseconds, so 300 milliseconds slower. That’s basically 40% faster because I used esbuild, simply by changing the method of build for my application. This type of optimization exists for pretty much every language out there, but Node is my default programming language, so this is the example that I have. Next thing is, remove stuff that you don’t need or turn off things that you don’t want.
In Java, Java has a two-tier compilation process. By default, it’s going to go through both tiers. Tier one is standard compilation, tier two is optimization. Lambda functions are not generally living that long for tier two to have any good effect. You can just turn it off. There’s an environment variable called JAVA_TOOL_OPTIONS. You can set this and it’ll turn it off. I think 90% of the time, you’ll see cold start performance improvements when you do this.
Optimize Everything
Optimize, so memory allocation controls CPU allocation. What that means is, there’s a direct proportional relationship between memory and CPU. If you notice, you can’t specify CPU on your lambda function, only memory. If you have a 256-meg function, if you were to drop that to 128, that cuts your CPU allocation in half. Same thing, 512. If you were to double that to a gig, you get double the CPU power. Think about this. If you double your memory for your functions, can you run twice as fast in all scenarios? Is that fact or fiction? The answer is, it depends. Depends on your code and depends on if you’ve multithreaded your code to take advantage of the number of vCPUs Amazon gives you. It’s all dependent on the use case. The principle here is, you must test your application.
The best way to do that, that I found, is using Lambda Power Tuner. It’s an open-source tool. It’s going to generate a graph by creating multiple versions of your Lambda function at many different memory settings, and it’ll show you exactly which one is the best. Red line here represents performance. Basically, invocation time, lower the better. Blue line represents cost. Also, lower the better, but we’ll walk through this.
At 256 megs, we can see the cost is ok, pretty low, but performance is really bad, upwards of 10,000 milliseconds. If we move this function to 512, you can see cost actually drops a little bit, but performance increases drastically, time drops by a factor of two. If we continue increasing to 1 gig, we see more performance improvements, almost at no cost. Go to 1.5 gigs, we start seeing some increase in the invocation cost, and then past that, we’re basically wasting money. Every single Lambda function is going to perform differently based on your use case, based on your memory, based on your runtime. Make sure you’re running this code against your functions as you go through your QA and performance tests.
Billing basics. Lambda pricing, remember this, the formula is always memory configured times the duration it runs for. If you look at this chart here, it’s very interesting. We have three lambda functions, all running 1 million invokes per month, 128 megs for the first one, if it runs for 1,000 milliseconds, it’s going to cost you $2.28. That same function bumped up to 256 megs, if it were to run twice as fast, it’s going to cost you the exact same amount. However, if you bump it to 512, so you 4x the memory, you don’t improve performance, so back in that chart that we saw, it’s going to get a 4x increase in cost. Anytime you’re thinking about performance and cost tradeoff, it’s a direct proportional relationship on both sides of this formula. We talked a little bit about ARM. ARM is that little chip that’s in all of our mobile phones. It’s faster. It’s more cost efficient. It’s more power efficient. It’s going to be cheaper, 20% generally from AWS. Try to move to ARM if you can. It’s free to move, it doesn’t cost us anything.
Then, logs cost money. Logs are pretty expensive. They’re 50 cents to ingest, 3 cents to store. You get charged every month, forever. I’ve seen applications where logging costs more than the Lambda compute itself. When somebody from finance finds that, that’s generally not a fun conversation. Reduce logging when you can. Think about swapping to infrequent access, which reduces cost by 50%. There’s a tradeoff, you won’t be able to do live subscription features on those logs. You can set a retention policy as well. You can age out these logs as you need to based on your data retention policy. I like to use this as a guide here between levels of environments. That way, you don’t have logs around too long.
Observe Everything
The last area we’re going to talk about is observability. If you’re in AWS, there are tons of metrics out there and it really gets confusing. One of the most important ones at the account level is a metric called ClaimedAccountConcurrency. This is really just the sum of all possible Lambda configurations that are actively using concurrency in your account. By default, AWS will only give you 1,000 concurrent Lambda functions as a cap. It’s a soft cap. You can ask for more. Your goal here is to create an alarm off of this metric so that your SRE team can be warned when you’re approaching that hard cap, or the soft cap, which you can lift if you start approaching that.
Next thing here is, at a function level, we talked about Lambda operating a poller and then delivering those records to your functions on your behalf. There’s no metric that AWS gives us for that. I don’t know why there isn’t, but they don’t give us a metric. If SQS is delivering 5, 10, 20, 100 messages per second to your function, there’s no way for you to tell how many you’re getting. Make sure you create a metric on your own. What I would do is use Lambda Powertools for that. It’s a free SDK, open source. Here’s an example in Node on how to do that. It’s really easy. You can use something called the EMF format, which is the embedded metric format. It looks just like that. That’s the EMF format. It writes a JSON log into CloudWatch logs, which gets auto-ingested by AWS, and creates that metric for you.
That’s basically the cheapest way to create metrics. It’s much cheaper than doing PutMetricData calls. Those are really expensive calls. Try to avoid that API call at all costs. It’s really cool because it’s all asynchronous. There’s no impact on your Lambda performance.
Then these are the top things that we’ve put together that have caused us a lot of pain. Just be careful about setting maximum configurations for your Lambda functions. Usually, that results in high bills. You want to set lower configs. You want your functions to error and timeout, rather than allowing them to expand to the largest possible setting. Number two, don’t PutMetricData. That’s really expensive. Number three is, there’s a mode called provisioned concurrency. You can actually tell AWS to warm your functions up when you need them and keep them warm. The downside is, if you do that, it’s going to cost you money if you don’t use that concurrency. Be careful about setting that too high and be careful about setting the provisioned concurrency equal to anything that’s close to your account concurrency because that will cause other functions to brown out. Then, just think through the rest here.
The very last one I’ll talk a little bit about, which is, don’t use the wrong CPU architecture. Back when we talked about moving to ARM, not every workload performs better on ARM. If you think about your mobile phones, we can watch videos, we can send messages, and those cost no power. If you go on your computer, desktop at home, and you watch some YouTube video, it consumes a gigantic amount of power because it’s running on an x86 architecture. Your use case will have a heavy impact on the right CPU architecture. Use the right libraries compiled for the right CPU architecture. A lot of us are doing, like compression is a good example, or image manipulation. All of those libraries have compilation libraries for ARM and x86, and make sure you’re using the right one for the right place.
Questions and Answers
Participant 1: What’s the incentive for Amazon to provide decent performance? If the metric is time times memory, then why wouldn’t they just give all the serverless all the cheap rubbish CPUs that don’t perform very well?
Mao: If you think about how Lambda functions work, they’re not magic. Behind the scenes, when you want to invoke a Lambda function, that function has to be placed on an EC2 instance somewhere. What Amazon wants to do is optimize the placement of that container in their EC2 fleet so that they can optimize the usage of a single EC2 instance. If you think about an EC2 instance, it may have 3 gigs of memory. If I have a 1 gig function that I run for a long amount of time, and you’re not doing anything else, I might get placed on that 3-gig instance, and the rest of that instance is empty. That’s extremely wasteful for AWS. They don’t want to do that. What they actually want to do is they want to pack that instance as much as possible so that they can have high utilization and then pass on the EC2 savings to the rest of AWS. They’re incentivized for us to improve performance.
The worst-case scenario for them is I create a Lambda function and I run it once and never again, because they have to allocate that environment, and based on your memory setting, they have to decide what to do. There’s a gigantic data science team behind the scenes at Amazon that’s handling all of this. I don’t know the details anymore, but that’s what they’re paid to do.
Participant 2: Can you talk more about how Capital One does automated testing with so many Lambdas? You mentioned you use, I think it was called SAM. Do you use that in your CI pipelines as well for testing?
Mao: Every release that goes out there, basically every merge or commit into main ends up running our entire test suite and we use SAM to do most of that stuff. SAM is integrated right into our pipeline, so it executes all of our unit tests and acceptance tests right in the pipeline. We customize all of it to work for SAM, but at the beginning, none of this existed, because EC2 doesn’t have any of this. We had to upgrade our entire pipeline suite to handle all of that.
Participant 3: Lambda functions now can support containers and it has way higher resources, you can have bigger container images. My question is about performance, especially cold starts. Have you tested using containers for Lambda functions and did it have any implication on the performance and especially cold starts?
Mao: Remember I said Lambda functions are packaged as zip files, 50-meg zip, 250 uncompressed. There’s a secondary packaging mechanism called just containers. You can package your function as a Docker image, that allows you to get to 10 gig functions if you need to have a lot of dependencies. I don’t recommend defaulting to that because there are a lot of caveats once you go there. You lose a lot of features with Lambda.
For example, you can’t do Lambda layers. Behind the scenes, it’s a packaging format. It’s not an execution format. What AWS is doing is they’re taking that container and they’re extracting the contents of it, loading it into the Lambda environment and running that, just like your zip is run. You’re not really getting any of the benefits of a container and you’re going to end up with container vulnerabilities. I recommend just using it if you have a large use case where you can’t fit under 50 or 250 megabytes. Generally, I see that when you’re doing like large AI, ML models that can’t fit in the 50-meg package or you just have a lot of libraries that all get put together, so like if you’re talking to a relational database, Oracle, you might be talking to Snowflake, and just a ton of libraries you need that can’t fit. I recommend just stay with zip if you can. If you can’t, then look at containers.
Participant 4: Following up on the testing question from earlier. Lambda function tends to be almost like an analogy of a Unix tool, a small unit of work. It might talk to a Dynamo SNS, SQS. One of the challenges I’ve at least encountered is that it’s hard to mock all of that out. As far as I know, SAM doesn’t mock the whole AWS ecosystem. There are tools that can try to do that, like LocalStack. How do you do local development at Capital One given so many integrations with other services?
Mao: I get this question from our engineers all the time. SAM only mocks three services, I think. It’s Lambda itself. It’s the API Gateway, which is the REST endpoint. It can integrate with Step Functions local and DynamoDB local. Everything else, if you’re doing SQS or SNS, it cannot simulate locally. AWS is not interested in investing more effort in adding more simulation. LocalStack is an option. If you use LocalStack, you can stand up, basically, mocks of all of these services. What you’re going to have to do on the SAM side is configure the endpoints so they’re talking to local endpoints, all of it. What I usually recommend our team do is, SAM actually has an ability to generate payload events for almost every AWS service. You can do sam local generate, and then there’s a SQS and then the event type.
Then you can invoke your function using that payload that it generates. Then you can simulate what it would look like if you were to get a real event from one of those sources. That’s usually the best place to start. LocalStack is good as well. We actually just test integrating into development, so like your local SAM might talk to a development SQS resource. That’s really the best way to test.
Ellis: You’ve done a lot already. What’s on your to-do list? What’s the most interesting thing that you think you’re going to get to in the next year?
Mao: Right now, we’ve been focused on compute, like moving our compute away from EC2. I think the next is data. Our data platforms, we do a lot of ETL. I think everybody does a lot of ETL. We use a lot of EMR. We’d like to move away from that. EMR is one of the most expensive services that you can put into production at AWS. You pay for EC2, you pay for the EMR service, and then you pay for your own staff to manage that whole thing. We want to move to more managed services in general, so like Glue, and other things that don’t require management of EC2. I think data transformation or data modernization is definitely big.
See more presentations with transcripts
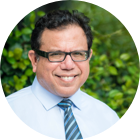
MMS • Craig Risi
Article originally posted on InfoQ. Visit InfoQ
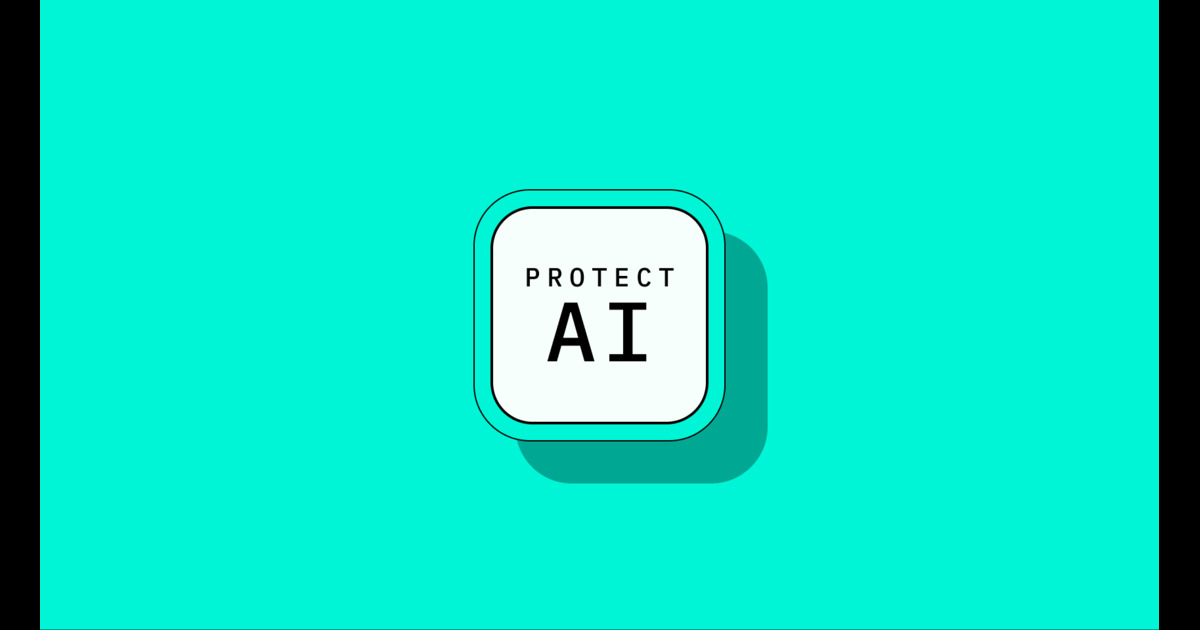
Edera has announced the general availability of Edera Protect 1.0, a Kubernetes security solution designed to enhance container isolation and address longstanding security challenges in cloud-native environments. Unlike traditional container security tools that focus on post-deployment detection, Edera Protect introduces a “zone”-based architecture, providing strong isolation between containers by default. This approach aims to eliminate entire classes of threats, such as container escapes and credential theft, by re-architecting the standard container runtime.
Edera Protect integrates with existing Kubernetes infrastructure, allowing organizations to enhance their security posture without disrupting developer workflows. In the general availability release of Edera Protect 1.0, several technical enhancements have been introduced to support secure, scalable container isolation in Kubernetes environments. One of the most significant changes is improved scalability: the system now supports over 250 secure zones per node on hardware with 64 GB of RAM. This advancement enables denser multi-tenant workloads, a common requirement in enterprise Kubernetes clusters.
A key improvement in resource management comes with the introduction of memory ballooning. This feature allows zones to dynamically adjust their memory allocation based on real-time demand, helping reduce resource overprovisioning while maintaining strong isolation boundaries. To address performance concerns around container startup times, warm zones were introduced. This capability should reduce the time it takes to spin up containers, bringing performance levels closer to what teams expect from native Docker environments.
The release also broadens platform compatibility. Amazon Linux 2023 is now supported, and integration with the Cilium Container Network Interface (CNI) allows users to combine Edera’s security architecture with existing advanced networking and observability tools. These integrations aim to support a wider range of infrastructure setups without requiring major changes to existing environments.
The 1.0 release includes Prometheus metrics and health endpoints, making it easier for teams to monitor zone health, resource usage, and system behavior. Additionally, a Terraform module has been introduced for Amazon EKS, simplifying the process of deploying Edera Protect into AWS-based Kubernetes clusters.
The release of Edera Protect 1.0 represents a step towards addressing the inherent tension between platform velocity and security in Kubernetes environments. By providing strong isolation at the architectural level, Edera aims to reduce the reliance on complex, layered security tools and enable organizations to run secure, multi-tenant workloads more efficiently.
Looking ahead, Edera has said they plan to expand the capabilities of Protect by introducing support for defining security boundaries at the Kubernetes namespace layer and deeper integration with cloud provider security features. This continued development underscores Edera’s commitment to enhancing container security and supporting the evolving needs of cloud-native organizations.
Inflection Points in Engineering Productivity for Improving Productivity and Operational Excellence
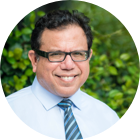
MMS • Ben Linders
Article originally posted on InfoQ. Visit InfoQ
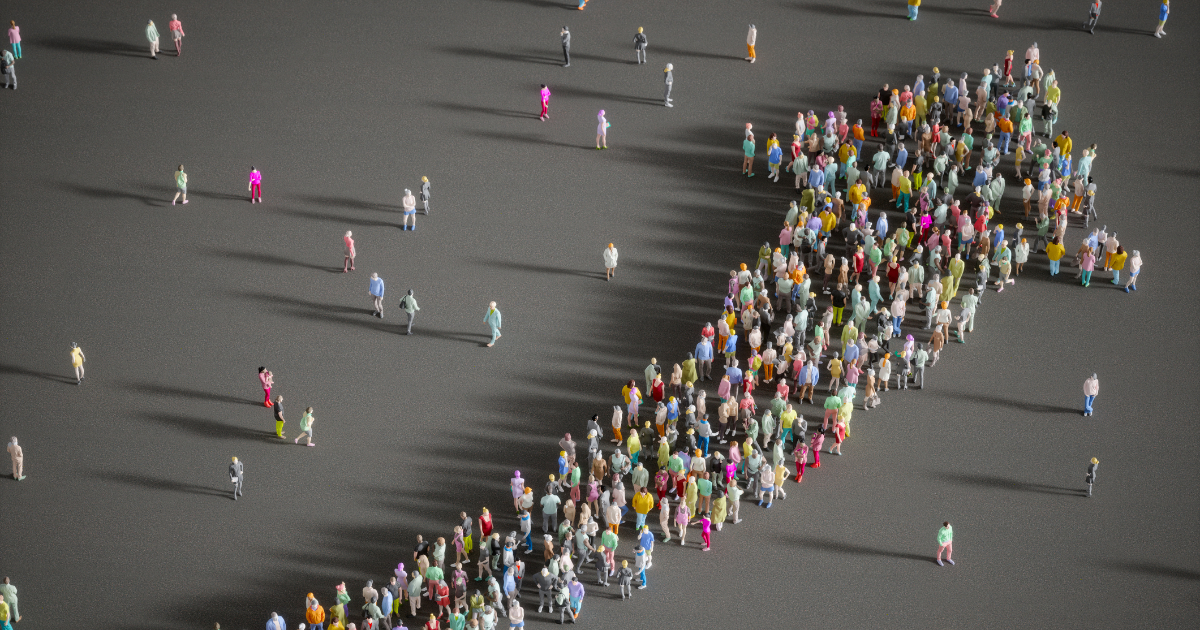
As a company grows, investing in custom developer tools may become necessary. Initially, standard tools suffice, but as the company scales in engineers, maturity, and complexity, industry tools may no longer meet needs, Carlos Arguelles said at QCon San Francisco. Inflection points, such as a crisis, hyper-growth, or reaching a new market, often trigger these investments, providing opportunities for improving productivity and operational excellence.
Carlos Arguelles gave a talk about Amazon’s inflection points in engineering productivity. When a company first starts, it doesn’t make sense for it to create its own developer tools, as there are plenty of excellent ones available in the industry, he said. But as a company grows (in number of engineers, in maturity, in customer adoption, in domains), investing in its own developer tools starts making sense:
An inflection point is when that investment in engineering productivity that didn’t make sense before now suddenly does. This could be because the industry tools do not scale, or because the internal tools can be optimized to integrate better with the rest of the ecosystem, as Arguelles explained.
A little papercut where each developer is wasting a couple of minutes per day in toil can add to hundreds of millions of dollars of lost productivity in a company like Amazon or Google.
The more obvious inflection point that made investments in engineering productivity become feasible is the number of engineers. Maybe it didn’t make sense for your company to have its own CI/CD proprietary tooling when there were 3000 engineers, but it does when there are 10,000 engineers, because the savings in developer productivity with a toolchain optimized for the ecosystem have a significant return on investment, Arguelles said.
He mentioned that the opposite is true as well. When your company is in hypergrowth it may make sense to have duplicate tools (each organization creating its bespoke tool so that it can independently move fast), but when the company stops growing (which is what happened in 2023 with all the big tech layoffs), it makes sense to consolidate tooling and defragment the world.
Arguelles gave some more examples of inflection points, like reaching a certain level of maturity where you need to raise the bar in terms of engineering or operational excellence can provide an inflection point or entering an entirely different and new market. Sometimes the inflection point is a crisis or even a single operational issue that could have been prevented with the right tooling:
For example, Amazon invested significantly in a number of load, stress, and chaos testing tools after the Prime Day incident of 2018 (where the Amazon Store was unavailable for hours during the busiest shopping day of the year). We had been talking about doing that for years, but that incident helped us sharpen our focus and build a solid case for funding those investments.
Inflections can also happen when an organization experiences hyper-growth:
I saw Amazon double in size every year, from 3000 engineers when I started in 2009, to 60k-70k in 2022. What this meant in practice is that we needed to be thinking about skating to where the puck was going to be, not where it currently was.
Scaling needs and security needs often meant sooner or later we needed to create our own developer tools, Arguelles said. Over time, they developed tools to scale source code repositories and build their own tools for code reviews and CI/CD (including testing and deployment):
Because of that hyperscale, we often found ourselves needing to re-think our architecture much sooner than we had envisioned. But it also provided ample opportunities to innovate and think differently!
Inflection points are inevitable and occur naturally in many situations: a company drastically increasing or shrinking in terms of number of engineers, a crisis, reaching a certain level of maturity where you need to raise the bar in terms of engineering or operational excellence, or entering an entirely different and new market, Arguelles said. He concluded that it is important to have your eyes open, recognize when these inflection points are around the corner, proactively shape your engineering productivity tooling for the future, and seize the opportunities.
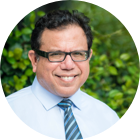
MMS • Steef-Jan Wiggers
Article originally posted on InfoQ. Visit InfoQ
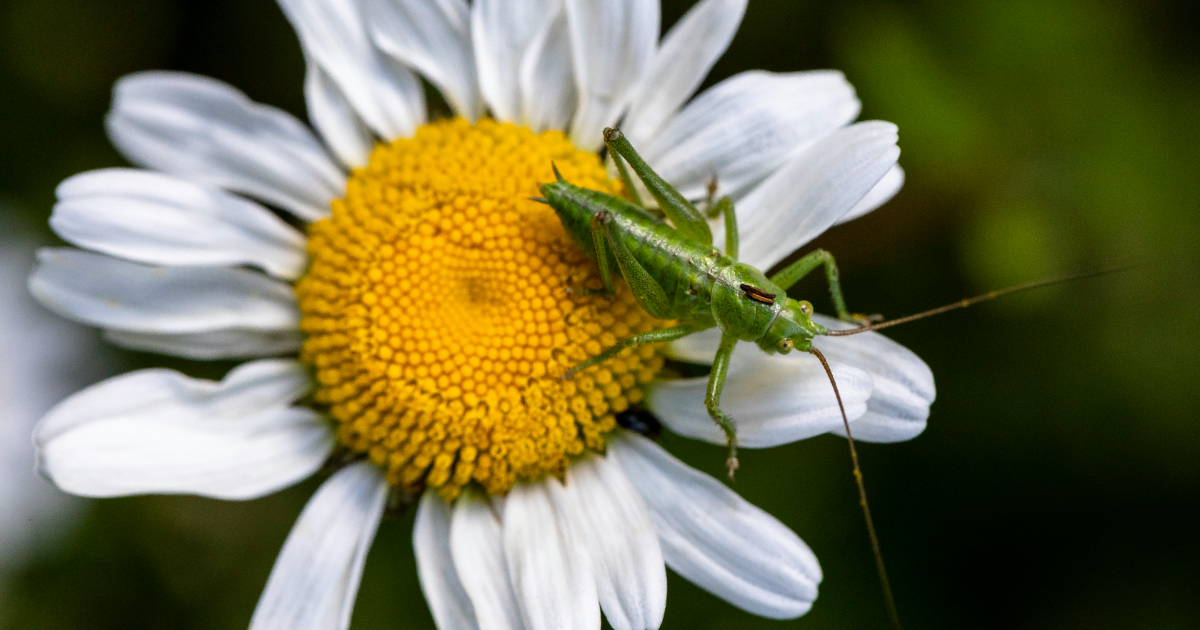
Google has announced that its Gemini models will be available on Google Distributed Cloud (GDC), bringing its advanced AI capabilities to on-premises environments. The public preview is slated for Q3 2025.
With this move, the company aims to allow organizations to leverage Gemini’s AI while adhering to strict regulatory, sovereignty, and data residency requirements. The company is collaborating with NVIDIA to make this possible by utilizing NVIDIA Blackwell systems, allowing customers to purchase the necessary hardware through Google or other channels.
Sachin Gupta, vice president and general manager of infrastructure and solutions at Google Cloud, said in a NVIDIA blog post:
By bringing our Gemini models on premises with NVIDIA Blackwell’s breakthrough performance and confidential computing capabilities, we’re enabling enterprises to unlock the full potential of agentic AI.
GDC is a fully-managed on-premises (available since 2021) and edge cloud solution offered in connected and air-gapped configurations. It can scale from a single server to hundreds of racks and provides Infrastructure-as-a-Service (IaaS), security, data, and AI services. GDC is designed to simplify infrastructure management, enabling developers to focus on building AI-powered applications, assistants, and agents.
According to Google, bringing Gemini to GDC will allow organizations to use advanced AI technology without compromising their need to keep data on-premises. The GDC air-gapped product already holds authorization for US Government Secret and Top Secret missions, providing high levels of security and compliance.
Keith Townsend stated in a LinkedIn post:
For security-conscious industries like manufacturing, this is a game-changer. Let’s say you’re running a complex OT environment. Machines generate massive volumes of telemetry—temperatures, vibration patterns, run times. With Distributed Gemini Flash, you can deploy lightweight agents on-prem, behind your firewall, to analyze that data in real time.
Gemini models are designed to deliver breakthrough AI performance. They can analyze million-token contexts, process diverse data formats (text, image, audio, and video), and operate across over 100 languages. The Gemini API is intended to simplify AI inferencing by abstracting away infrastructure, OS management, and model lifecycle management. Key features include:
- Retrieval Augmented Generation (RAG) to personalize and augment AI model output.
- Tools to automate information processing and knowledge extraction.
- Capabilities to create interactive conversational experiences.
- Tools to tailor agents for specific industry use cases.
In addition to Gemini, Google highlights that Vertex AI is already available on GDC. Vertex AI is a platform that accelerates AI application development, deployment, and management. It provides pre-trained APIs, generative AI building tools, RAG, and a built-in embeddings API with the AlloyDB vector database.
Lastly, the company also announced that Google Agentspace search will be available on GDC (public preview in Q3 2025). Google Agentspace search aims to provide enterprise knowledge workers with out-of-the-box capabilities to unify access to data in a secure, permissions-aware manner.
Capital World Investors Has $53.69 Million Stock Position in MongoDB, Inc. (NASDAQ:MDB)
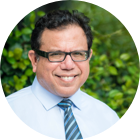
MMS • RSS
Posted on mongodb google news. Visit mongodb google news
Capital World Investors cut its position in shares of MongoDB, Inc. (NASDAQ:MDB – Free Report) by 34.4% during the fourth quarter, according to the company in its most recent disclosure with the Securities and Exchange Commission. The firm owned 230,606 shares of the company’s stock after selling 120,885 shares during the period. Capital World Investors owned about 0.31% of MongoDB worth $53,687,000 at the end of the most recent reporting period.
Other large investors have also modified their holdings of the company. B.O.S.S. Retirement Advisors LLC acquired a new position in shares of MongoDB in the 4th quarter worth approximately $606,000. Union Bancaire Privee UBP SA acquired a new position in MongoDB during the fourth quarter worth $3,515,000. HighTower Advisors LLC lifted its holdings in shares of MongoDB by 2.0% during the fourth quarter. HighTower Advisors LLC now owns 18,773 shares of the company’s stock valued at $4,371,000 after acquiring an additional 372 shares during the period. Nisa Investment Advisors LLC boosted its stake in shares of MongoDB by 428.0% in the 4th quarter. Nisa Investment Advisors LLC now owns 5,755 shares of the company’s stock valued at $1,340,000 after purchasing an additional 4,665 shares in the last quarter. Finally, Covea Finance bought a new stake in shares of MongoDB in the 4th quarter worth about $3,841,000. 89.29% of the stock is currently owned by institutional investors and hedge funds.
Analyst Upgrades and Downgrades
MDB has been the topic of a number of recent analyst reports. Redburn Atlantic upgraded shares of MongoDB from a “sell” rating to a “neutral” rating and set a $170.00 price target on the stock in a research report on Thursday, April 17th. Cantor Fitzgerald started coverage on MongoDB in a research report on Wednesday, March 5th. They set an “overweight” rating and a $344.00 price target on the stock. Barclays cut their price objective on MongoDB from $330.00 to $280.00 and set an “overweight” rating for the company in a research report on Thursday, March 6th. Scotiabank restated a “sector perform” rating and set a $240.00 target price (down previously from $275.00) on shares of MongoDB in a report on Wednesday, March 5th. Finally, Mizuho lowered their target price on MongoDB from $250.00 to $190.00 and set a “neutral” rating for the company in a research note on Tuesday, April 15th. Eight research analysts have rated the stock with a hold rating, twenty-four have given a buy rating and one has assigned a strong buy rating to the company’s stock. According to MarketBeat, the stock currently has an average rating of “Moderate Buy” and an average target price of $299.78.
Check Out Our Latest Stock Report on MDB
Insider Activity
In related news, CFO Srdjan Tanjga sold 525 shares of the company’s stock in a transaction dated Wednesday, April 2nd. The stock was sold at an average price of $173.26, for a total transaction of $90,961.50. Following the sale, the chief financial officer now owns 6,406 shares in the company, valued at approximately $1,109,903.56. This represents a 7.57 % decrease in their ownership of the stock. The transaction was disclosed in a legal filing with the SEC, which is accessible through this hyperlink. Also, insider Cedric Pech sold 1,690 shares of the firm’s stock in a transaction that occurred on Wednesday, April 2nd. The shares were sold at an average price of $173.26, for a total value of $292,809.40. Following the completion of the transaction, the insider now owns 57,634 shares of the company’s stock, valued at approximately $9,985,666.84. This represents a 2.85 % decrease in their ownership of the stock. The disclosure for this sale can be found here. Insiders have sold a total of 47,680 shares of company stock worth $10,819,027 over the last three months. Corporate insiders own 3.60% of the company’s stock.
MongoDB Price Performance
NASDAQ:MDB opened at $151.67 on Tuesday. The business’s 50-day simple moving average is $205.34 and its 200-day simple moving average is $251.62. MongoDB, Inc. has a fifty-two week low of $140.78 and a fifty-two week high of $387.19. The stock has a market capitalization of $12.31 billion, a PE ratio of -55.35 and a beta of 1.49.
MongoDB (NASDAQ:MDB – Get Free Report) last released its quarterly earnings results on Wednesday, March 5th. The company reported $0.19 earnings per share for the quarter, missing the consensus estimate of $0.64 by ($0.45). The company had revenue of $548.40 million during the quarter, compared to analyst estimates of $519.65 million. MongoDB had a negative net margin of 10.46% and a negative return on equity of 12.22%. During the same quarter last year, the company earned $0.86 EPS. On average, research analysts expect that MongoDB, Inc. will post -1.78 earnings per share for the current year.
About MongoDB
MongoDB, Inc, together with its subsidiaries, provides general purpose database platform worldwide. The company provides MongoDB Atlas, a hosted multi-cloud database-as-a-service solution; MongoDB Enterprise Advanced, a commercial database server for enterprise customers to run in the cloud, on-premises, or in a hybrid environment; and Community Server, a free-to-download version of its database, which includes the functionality that developers need to get started with MongoDB.
Further Reading
This instant news alert was generated by narrative science technology and financial data from MarketBeat in order to provide readers with the fastest and most accurate reporting. This story was reviewed by MarketBeat’s editorial team prior to publication. Please send any questions or comments about this story to contact@marketbeat.com.
Before you consider MongoDB, you’ll want to hear this.
MarketBeat keeps track of Wall Street’s top-rated and best performing research analysts and the stocks they recommend to their clients on a daily basis. MarketBeat has identified the five stocks that top analysts are quietly whispering to their clients to buy now before the broader market catches on… and MongoDB wasn’t on the list.
While MongoDB currently has a Moderate Buy rating among analysts, top-rated analysts believe these five stocks are better buys.
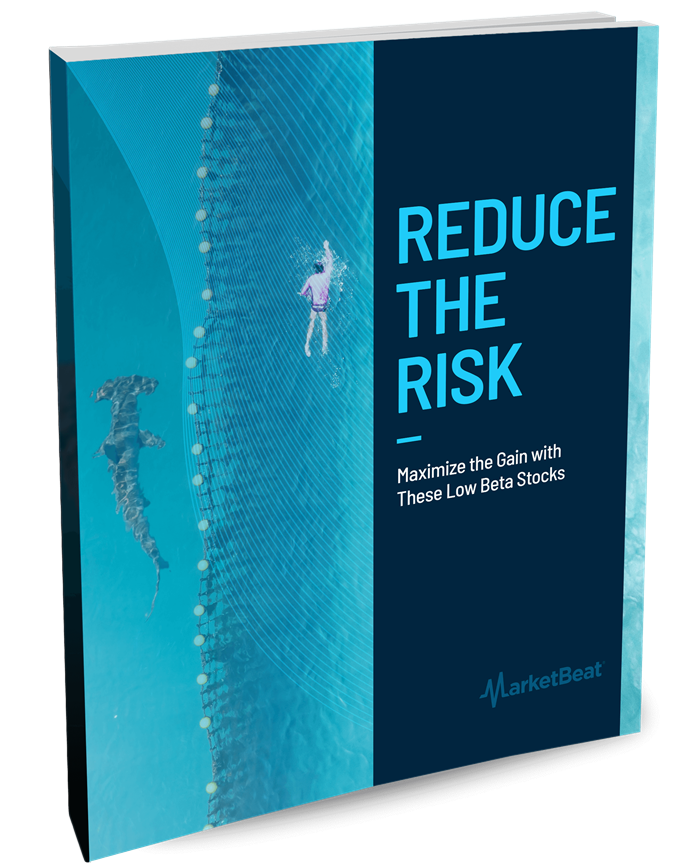
Market downturns give many investors pause, and for good reason. Wondering how to offset this risk? Enter your email address to learn more about using beta to protect your portfolio.
Article originally posted on mongodb google news. Visit mongodb google news
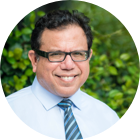
MMS • RSS
Posted on mongodb google news. Visit mongodb google news
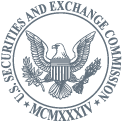
MONGODB INC
1633 BROADWAY 38TH FLOOR NEW YORK NY 10019
BlackRock, Inc.
In accordance with SEC Release No. 34-39538 (January 12, 1998), this Schedule 13G reflects the securities beneficially owned, or deemed to be beneficially owned, by certain business units (collectively, the “Reporting Business Units”) of BlackRock, Inc. and its subsidiaries and affiliates. It does not include securities, if any, beneficially owned by other business units whose beneficial ownership of securities are disaggregated from that of the Reporting Business Units in accordance with such release.
BlackRock, Inc., 50 Hudson Yards New York, NY 10001
See Item 4 of Cover Page
Common Stock
60937P106
4692038
5.8 %
4292036
0
4692038
0
If any other person is known to have the right to receive or the power to direct the receipt of dividends from, or the proceeds from the sale of, such securities, a statement to that effect should be included in response to this item and, if such interest relates to more than 5 percent of the class, such person should be identified. A listing of the shareholders of an investment company registered under the Investment Company Act of 1940 or the beneficiaries of employee benefit plan, pension fund or endowment fund is not required.
Various persons have the right to receive or the power to direct the receipt of dividends from, or the proceeds from the sale of the common stock of MONGODB INC. No one person’s interest in the common stock of MONGODB INC is more than five percent of the total outstanding common shares.
If a parent holding company has filed this schedule, pursuant to Rule 13d-1(b)(ii)(G), so indicate under Item 3(g) and attach an exhibit stating the identity and the Item 3 classification of the relevant subsidiary. If a parent holding company has filed this schedule pursuant to Rule 13d-1(c) or Rule 13d-1(d), attach an exhibit stating the identification of the relevant subsidiary.
See Exhibit 99
Not Applicable
Not Applicable
Article originally posted on mongodb google news. Visit mongodb google news