Category: Uncategorized
Presentation: Making Change Stick: Lessons Learned From Helping Teams Improve at the Co-Op
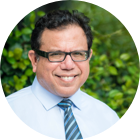
MMS • Neil Vass
Article originally posted on InfoQ. Visit InfoQ
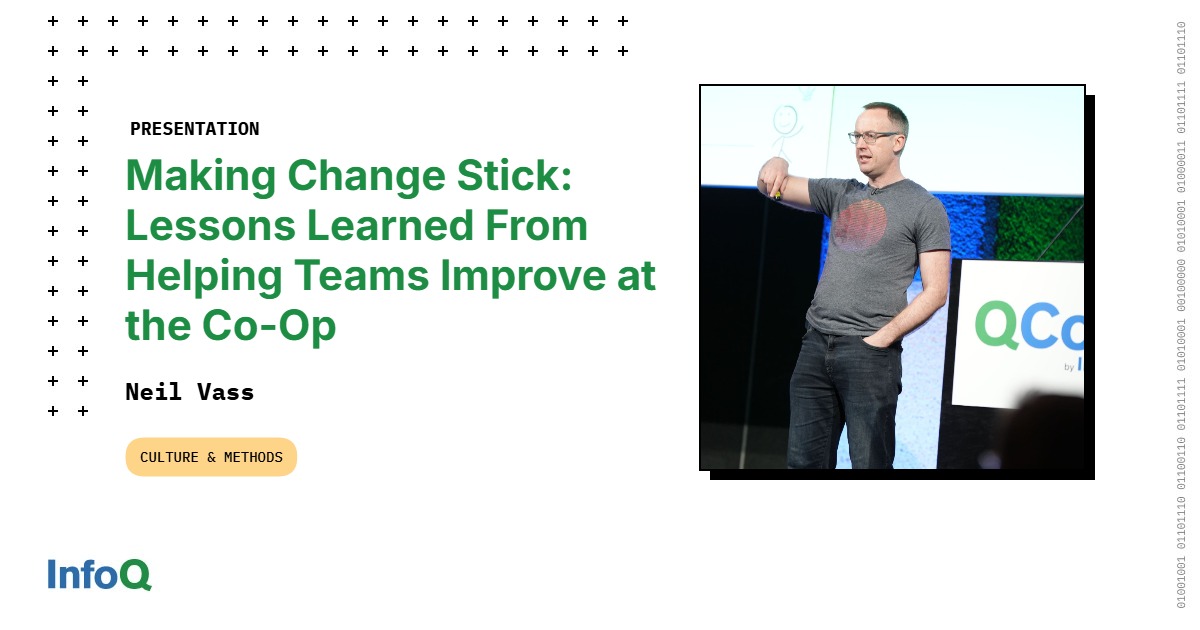
Transcript
Vass: This is about helping software teams improve. We’re going to start with a little bit of history. The Co-op that I work at today has its roots in 1844 when the Rochdale Pioneers got together to try a different way of doing business. There was lots of different co-operatives followed from them and merged into one bigger one. At the time it’s the kind of stereotype you can imagine of the rich landowning classes oppressing the working people. There was lots of low wages, hard to get high quality food, lots of like flour cut with things and price gouging going on. These people got together, you would put a pound in for membership and try and have a different way of doing business. Those rich landowning types hated it. Over time they tried to improve. As well as being a successful business, the co-operative ideals are about improving what’s going on in society. Some of the reasons we’ve got not just food stores today comes from this history.
Big challenges in those days were funeral poverty where you hadn’t planned for that, and you’ve got that expense, or getting into legal troubles. Starting businesses to help with those in the olden days is the reason you’ve got Co-ops confusing a collection of businesses nowadays, as it does funeral care, it does insurance, it does legal services, and a whole collection of other things. Some of the stories I really like from those days about how they were at odds with the traditional model of doing business there. Lots of industry said, you’re not allowed to give a discount, we have price fixed this. If you want to sell medicines, if you’re doing aspirin or anything, you can’t make it any cheaper. They wanted to do member pricing, which is still a thing today, so they started making their own.
Similarly, they couldn’t offer electronics at a discount, so they opened factories started making their own radios under the brand name Defiant. When I joined the Co-op, the building we worked in, was one of the traditional Co-op warehouses from the 19th century. Our meeting room, one of them was called Defiant. The history is all around you. I like that. Where it came from was about rebelliousness, about not going along with the status quo, about changing things. I think some of that’s still there today.
There’s a quote from Parkinson, you know about Parkinson’s Law that work expands to fill the time available. He’s also done work on the growth of bureaucracy. He’s got a formula about how it accretes about 5% to 7% per year for no other reason. The Co-op is coming up for 180 years old, and it’s got 65,000 employees. There’s been a lot of time. Some of that’s necessary, just keeping track of what’s going on and understanding what’s happening. There’s a lot of bureaucratic processes.
If you’ve worked in any big organization, you absolutely know the frustrations of, how do we keep on top of this? Who’s changed that now? What’s the priority? What’s this team doing? In addition to that new and different and rebellious side, and try to do good for society that I love, there’s all these traditional big company balances. I do think when I find myself getting frustrated with, they don’t know what they’re doing, there’s lots of software and agile teams to do this. If only they did something different, you’d be better. I think, what are the chances that I could have a business almost two centuries old and keep it still successful? Nothing. What experience do I have of running somewhere that’s got multiple billion pounds turnover every year? Absolutely none. It puts me in my place. They’re obviously doing something right. I don’t know everything as I walk about trying to tell people how to change their jobs.
We’re almost coming back to the present day. I will talk about software. Let’s just use a timeline, zoom us with a tech focus up to modern times. Here, 1844, that’s those Rochdale Pioneers, and the first Co-op starting. It wasn’t a software company then. If you think about, traditionally, where do you think about when you think of tech companies? It’s Silicon Valley, is the famous one. A few years after the Co-op started, this is the state of California getting founded. We predate Silicon Valley by a fair bit.
Zooming on to 1911, I think, you can guess our next tech milestone. I’ve just popped on, this is when IBM got founded. That’s International Business Machines, because computer wouldn’t be a word people really used for a long time after that. That’s IBM’s punch card business. Zooming on again to 1991, this is CERN, the physics lab where the world’s first ever website got made in 1991. Thanks to the Wayback Machine and some ICANN digging, I think just 1996, just a couple of years after the website was a thing at all, there’s the Co-op’s first website. Our last stop, very important landmark, is 2018, where I joined Co-op Digital. Looking at the length of the history and trying to understand what’s going on was difficult, but I think I’ve actually been at the Co-op for 3% of its existence, which is more than I thought. It’s not nothing.
Scope
This talk is going to give you a tour of modern-day Co-op. What are the software teams? I’ll show around what are the ways of working you see there, and how have I tried to do my best to give advice and help and support where I can. You may get ideas on how a big company with such varied types of business gets things done. You might be able to steal some ideas about how you organize or how you use your own things.
How It Started
How it started. It was 2018 when I joined the Co-op. This is not how the Co-op started. When I joined, Co-op Digital was a fairly new thing. Co-op has such varied landscapes. Just in food, there was different parts of tech and different parts of the big food business. They were almost not talking to or aware of what some of the other businesses did. There’s agile. There’s cloud development. There are other things that have been going on for a while. By and large, lots of parts of the Co-op, their experience with tech was do a big RFP, get some vendor like IBM or one of those other big companies to come and make something. We don’t really get involved, and you specify for years in advance what it’s going to be and it gets ready later. In about 2015, Co-op felt that it was interesting trying something different. A lot of the team who set up gov.uk and the government digital service came to the Co-op to try and install some of the ways of working there and show us what went on. That’s when we moved. Co-op Digital was born, a little bubble with some teams to try out something different that hadn’t really been seen at the Co-op much before.
If you’re familiar with GDS, you will have recognized some of these things before. Some of the things they introduced was like service design, working in the open, doing discovery work, to like, what is the problem we’re trying to solve, and let’s slow ourselves down before we jump to giving out a contract and build something two years from now. They had teams working closely with the business. Something I really enjoyed was some of the teams that got someone when we were redoing how admin and funeral care works. I think it was the first change for the funeral industry in 400 years, to get rid of lots of paperwork and lots of overhead. They had funeral directors who come and sit with the digital team to explain at length like, we can’t actually do that. Do you know what I’m doing when this comes up? To go back out to the business and say they’re solving real problems. Here’s a new version. It was good. It was fun.
The other thing that got introduced from GDS was the different phases. The discovery, alpha, beta, live process, as, do not jump straight, to, I think we should have an app. Can I get millions of pounds of budget and go and launch one? You should start way over here, as, are you sure there’s a problem, and how can we define it? Who should we get involved in doing that? At one point in Co-op stores, if you worked in a Co-op shop, the only way to find out what shift you’re doing is to look for a bit of paper in the back room of the shop. That wasn’t too many years ago. This was a huge step forward as we brought out shifts and understanding what people wanted, what would be useful for them for the business and the colleagues, was really good. In your discovery phase, you work out what are the challenges, what do people do.
The business and colleagues would love to be able to do things like mark yourself, “I’m happy to work in any stores in a particular area. Just shout if something comes up”. That’s a huge unlock for stores flexibility, and the colleagues love it, which just wasn’t something that was possible before. You put them on a backlog, and from your alpha, you don’t jump straight to, so we should make this then. Your alpha, you’ve got lots of options. You try things out ideally in paper or throw away prototypes until you’re sure you’ve got it right. That’s the process that got introduced quite a lot in different bits of work we did.
One of the first teams I worked on was Co-op’s One Web team, which is still a team today. Thanks to the Wayback Machine, I’ve dug in and I found out that first one website we made in 1996 optimized for Netscape 3.0. There had been a lot going on since then. For quite a while, I think anyone in any part of the Co-op who had the budget could go and pay any agency to go and make them a website, and we didn’t quite know how many websites there were. It was a lot. It was far too many. I don’t know what it looked like when you were as a user Googling Co-op and just finding all these different top-level domains. I’d just moved from the BBC when I joined here and they had iPlayer and Sport and News, and they had one website.
I think at most Co-op probably needs one, was what we went for. We started. We started moving over the recipes site. Co-op’s food website looked like one but it was actually I think four or five different top-level domains that would change as you did it, but skinned up to look the same. It was different contracts with different agencies. Moving the recipes website over was good because we got someone from food marketing to come and sit with us and understand the problem, the mess of updating it, and things going on. It looked quite nice. For lots of the things I just skim over here, if you do want to hear about them you can go and have a look on Co-op Digital blog because loads of these stories are still there worked in the open.
One reason that I was so pleased with the one Co-op look of it was the design system which has now grown into the experience library. This was one place where the designers and other roles had got together and said, things look different everywhere you go. Things are worded differently wherever you go. Can we agree, as a group, what’s a sensible way to do that? The approach they took that I really like as with those different businesses, people were understandably quite worried. They’ve had a lot of flexibility before in the business, “I want this to look amazing. I want this to look different”. Is it all going to be the same? Is somebody looking for Easter food offers going to see a similar thing to like legal services forums? Familiar not consistent is what they talked about doing, like recognizing the variety of what’s going on here. Make the simple things repeatable and do them again. There’s web components and stuff you can build there.
The other thing I really liked from this was the content guidelines. There was a quote, it’s probably still on there from the head of content design, about everything you write on your site makes it harder to find everything else. I think that’s really stuck with me. I think there’s an instinct sometimes when you’ve got a website, look how much amazing stuff we’ve got? Getting people from the team to sit with us as we do content audits or I printed stuff out and put it on the wall, they just look at, that’s too much, isn’t it? I think as well as the technical side of things, we’re doing things in containers, so that you had full control over your own website without affecting other people’s, but you could benefit from any new features like scheduled publishing and stuff like that. That was a good model and it was quite an interesting technical challenge to work on, as much as it was a culture shift internally.
This idea that you show me three designs and I’ll pick one, just shouldn’t go because we’re looking for a big picture. How does a Co-op make sense? I like more words, can I get more words, is a difficult thing to talk about what you’re doing.
It went all right. We started taking down and moving things over. Over time, it started to get complicated. There were some cases where the team just needed the ability to change pages. The wine team at the Co-op, at one point their contract was, you have to keep going back and paying to make changes. They didn’t need much technical support, apart from, we’ll give you a wine site and you can log into the CMS here and you can add a new page anytime you like. They were delighted. Other places have more complex ongoing needs. The web team grew a bit and it evolved into, there’s some sites we look after and change because there’s things that need changing. There’s things that don’t have a digital team, but they do need things updated. Maybe we can get some skills in there. Then for other digital teams, they were taking on what we’d done. I was starting to get confused because I was thinking it feels like we’re more like a platform for other people to use.
At the time, I’d never really worked in that team before. I was wondering about setting objectives, about, how do we measure ourselves and what direction do we go in? It felt like an anti-pattern. You know in the old days where there’s a database team and there’s some other component team, and there’s somebody who does the frontend. Shouldn’t we care about the whole end-to-end stack? Don’t we need to be closer to the users? I wasn’t sure.
Then I saw on Twitter this advert for something called team topologies. These slides I was looking at was, these are the slides I want. Look, it’s got metrics. It’s got things we need to think about, adoption costs. That was my first encounter with team topologies. I got to go on a two-day course with this where I could ask specifically, it was Manuel Pais, when you say things like don’t make it mandatory, do you actually mean not mandatory? I can absolutely see something like that. Give people a choice, like, if you do it like a product then in other teams, it forces you to make it good. I can see that in theory. Who really thinks about the big picture in a complicated organization? It’s often easier for some other team to say, I don’t know Python, so I’d rather write my own thing from scratch in React over here. Don’t we need to? It was really good to speak to them about crossing the chasm about they might have a point about that, and 100 other things that were super useful.
Another idea that we got from this as a team was about, you shouldn’t start off as a platform team thinking about, what’s the fastest we can go? How do you get those DORA metrics up? How can we deploy more stuff? Because you can just get yourself very quickly into a mess. The hard problem is about interaction modes. This is something that really stuck with me. Lots of us haven’t had to practice that at all. As you work with other teams, collaborate. Just get everyone together and work it out. It’s fabulous in lots of cases just because it’s not done enough. If you do that for everything, it’s super wasteful.
Before you know it, you are spending your life, especially as we were supporting more teams around the place, collaborating with them all the time. Thinking about interaction was really important. What do we want to offer as a standard service? You ask this, you get that. Who are we supporting for a limited amount of time? The idea of putting a deadline on it, how many teams are we supporting now with what, and when do we want that to end? You can move that deadline if you want. Bearing in mind that we expect this to stop at some point, prompted some really good conversations. Some other digital teams felt like for that platform, for the AWS side of things, that’s always going to be you doing that for us? We shout when something needs changed. They’re like, no, the intention is, we’re training you to do it, and we’re going to stop soon. That prompts a different conversation that I just hadn’t realized needed to be had. We got all kinds of value out of that. Working on that team day to day as it grew and changed over time, I’ve worked at a few places, I’ve seen a lot at the Co-op, I feel like I’m helping here.
Improving Things
I moved on. I moved to a role where I was managing people on teams rather than non-teams directly. I’ve got ambition to help. I thought, I can improve things. I talked earlier about not getting too big for your boots, thinking you’ve got the right answers everywhere. What I saw was lots of teams doing quite well, but then falling into the same problems and pitfalls that I thought we’d solved some time ago. I talked earlier about having an on-site customer, like who from the part of the business you’re working with is with your team day to day. That’s a practice that just fell away. There was a few different things about prioritizing work. How do you choose what you’re doing? There was teams I talked to who were struggling with, we’re not sure about our agile process, like how we put work on the board and stuff. I said, do you talk about it in your retros? Says, we don’t have retros. I assumed that’s the trouble. We’d never written it down. There was wide freedom.
That idea of just get qualified people together and let them work it out, sounds great, but then it’s doing people a disservice. I think how we want to work here is a question that doesn’t need answered. At different companies, you do something different. There are probably hundreds of different ways you could do agile well. Helping people with that. Also, for lots of people, just learning how to program or learning how to design can be a pretty much full-time job in your other part of your career. You look up, there’s a Kanban board and sometimes you have a retro. You’ve never really talked about why are they there? How do we do that well? That was something that was getting missed. I thought, and we talked to other senior people around teams in Co-op Digital, like we could improve things. We’re doing well, but we could get a lot better.
It’s important to hesitate before you steam in and tell people how to work. I think you can tell people anything and lay down standards, but you can’t make them find it useful. We’re looking for what’s a challenge that you’d like some help with, and do I have anything that helps you? I think it’s difficult sometimes when you’re working out what practices would help people. Sometimes I think you have to adapt it to your context. I’m not really going to be on your team for a while. I sometimes feel like the advice I give people is, read these books, watch these talks, and then try it out for a year or two and you’ll work it out. That’s not as helpful as I’d like.
The other thing is giving people bad advice. There’s a good quote from Ron Jeffries, talks about what it’s like when you’re a bit experienced and you’re coaching other teams, and you try to give them advice. You’ve got to be super aware of that thinking. Lots of coaches think, we did X, so you should do X. That doesn’t imply that X helped you at all. That may have just been something going on in your team. Actually, it does not imply X is the best choice. There’s loads of things people could do. They could happen across something much smarter than what you would have picked. It really does not imply that X is something you should do. I try to keep this in mind. On this, how do we help people? I think there’s whole talks involved in all of those things. Does anybody want your help? Do you have good advice for them? The topic for today’s talk is specifically about making change stick. What I found again, was people did want some help. We did coach them or talk them through ideas, or put them in touch with someone else. That did solve the problem they had.
Then you look back just a few months later, and either some folk have moved off the team, or the type of work had changed, or they just took their eye away from it. They’re saying, we are struggling with that same thing that you helped us with before. I said, and what about that thing that helped with it? We stopped doing that. What happened? Sometimes you go back, it’s just six months or so, a couple of team changes, some people have joined and left, different ideas. They don’t even know what you’re talking about. I thought, as I cycle round and other people are trying to help, how do we make that last? One thing that will either give you cheer or give you the idea that nobody can do this, so we shouldn’t bother, is it’s not just a me problem, and it’s not just a Co-op problem.
There’s a fabulous book from Henrik Kniberg about, “Lean from the Trenches”. This is a book-length case study about some people who’ve really solved it. They had a big piece of work to do. As an organization, with our stakeholders and bosses and the teams involved, they got Kanban working for them. They go into loads of detail about how they broke things down, about how they avoided the big traps of big-bang releases and not knowing what you’re doing. It’s really detailed, and it really felt like everyone involved came to an understanding of, we won’t do those silly things anymore, we’ll fix it. In his very next presentation that I saw him give, once he was at Spotify, he put up a slide that stopped me in my tracks. This is the same team with all the same stakeholders in management who had just learned all the projects. The very next thing they went on to, everything they’d learned had gone out the window and it’s all gone wrong. I don’t know how you can actually make change stick, but we can try.
On the topic of improving things, we got talking to lots of different people around Co-op Digital as it was at the time, about what challenges are you seeing. I think some things people hadn’t really taken into account. Some of the things we’d solved about, how do you start a team off? How do you get your stakeholders engaged? How do you set up your processes and set a goal and stuff? Was for those earlier stages. Alpha and beta are time-bound things. Once you’re live, there’s a whole lifetime after it. It had just been just about 18 months before we were talking about this challenge, I think every single thing that Co-op Digital made across its 12 or so teams was no further along than beta stage.
Now, over time, we’d suddenly evolved. You just turn around and things have shipped, everything’s live. Everything’s been going for a while. We’re a long way from the initial team kickoff and initially getting show and tells going and stuff like that. We’re just in a different phase now. We tried to draw up who are all the teams. What do you mean by a team is something that confuses people quite a lot, so drawing it out. I think another good bit of advice from the team topologies course was a team, their definition is five to nine people with a shared purpose. Larger groups are called groups. That’s an important distinction. I think that gets missed. The design team, the digital team, and things like that, confuses people sometimes.
Who are the teams we’re talking about helping? What challenges do we think we had? We did some user research. We talked to different people. We started seeing themes, the same things from on teams and the senior leadership team around it and other things like that. We pulled it together. Some things about accountability, that issue about, is it the business that decides what direction we’re going in or what we should change next, or is it the teams that do it? Some things we’re interested in was consistency. I do like the quote from Ralph Waldo Emerson, that foolish consistency is a hobgoblin of little minds. It looks neat if everyone’s sprint starts on a Thursday or they all do the same number of story points or something like that.
Teams have such different ways of working and different challenges. I don’t think doing exactly the same thing everywhere is the right answer. Make it familiar enough, but not totally consistent. That idea of easy things becoming easy and what works for the Co-op was good, because there’s lots of debate. There’s hundreds of different ways you could set up teams. There’s all kinds of what’s the perfect way to do a software team. It doesn’t actually matter. We should pick something fairly sensible and get onto the interesting hard problems, like, how do we understand our users and how do we make some business value happen?
From this and from talking to loads of people, it was as fat as a phone book: lots of big ideas about what we should change. I’d love to start with the leadership around team, taking some responsibility and understanding what’s going on. What tools you can use and what’s going on. If you want to know how do software teams work effectively, I think you’ve got this leadership thing. You’ve got those ways of working. You’ve got technical approaches are important. I think user research. It was so much. People agreed this was good, but when you try and look at it and use it, it was just too big a first step. We stepped back. Just seven things. We’ll keep it small. Each one was just a sentence. I think this was useful because it’s the size that fits in your head and it’s something we can say, are you doing this or not? We got one thing there. I’ll zoom you through some others, a few more here. You should have regular retros.
Sometimes teams say we don’t need them because we just talk about problems as they come up. That can be true sometimes, but not as often as people are just saying that. Each of these was something we were seeing just teams not finding a way to do. No one’s going to our show and tells. Stakeholders don’t really talk to us. Then they come up annoyed later when we’ve done something. I think a team charter is important. I kept banging on about team topologies to see who would pick it up. Things about understanding team health, like we’re not saying that for fun and we’re not doing it to be nice, we think these are the ways you deliver more effectively. The reason I’m not spending too much time on what the seven things are is that I don’t think which seven things you pick in particular is that important. There’s advice on how to do things well, but I don’t think that it’s these seven are magic. I repeated this again. I think it’s pick something sensible and see how you can make it stick.
We did lots of visits to teams and walking around, warning people about traps. It is the kind of thing you put in place and then you’ve forgotten it. You turn around, did we have a talk one time about things that were important? Other traps is mistaking the measure. It might be that everyone has a planning session, everyone has a show and tell, everyone has a retro. It might be no good improvements ever come out of them, no one actually comes to the show and tell. We’re not doing anything right. If a team was working well, you’d expect to see these things happening. Let’s talk about, are you getting value out of them? If you’re not, can we improve it? The last one, I mentioned before about trying to pick the perfect answers, going back and forth about, should we do this and should we do that? It doesn’t really matter. We should try something. If it doesn’t work, we can try something else. If we get this right, we could try anything.
How It’s Going
How is that going? Initially, this was a bit tricky. Like I said, that first, here’s a phone book of things to do, I got away from that. The seven things were interesting because we went around different teams, and if you talk it through like this, people see, this is useful, this is helpful. If there’s one of them your team doesn’t have the skills or time or capability to do, you’ll get help with it. It was good. It’s to help you reflect and surface issues. You can go around teams and get someone external to try and do the Fist of Five rating, which gives you really eye-opening things. If somebody says, five, we have retros all the time. Someone else says, two, nobody actually goes, and it’s always the same person who runs them, and we never get any improvements out of it. That was good. There was also the bad. Just a few steps away from me as we tried to scale this out, I found somebody calling it the seven things audit. Someone would come out with quite a formal forum and audit you. I was like, that’s not what we talked about at all.
I think that was eye-opening for me, how hard it is to have influence wider. I could understand if a different company picks something up and they misunderstand what you’re talking about. This is just over there. It was quite humbling to think, if I’m not with people all the time talking about what we mean, is that how quickly it goes apart? Over time, banging on about this has been super useful. As Co-op Digital ways of working and long-running teams have spread wider to a department that’s now well over 700 people, it’s been a super-useful, short, clear introduction to what we think is important. There’s lots of people who still use this today to run training based around it and to check in with teams like, are these things going ok? You’d be astonished how often it’s one of those, it’s something to pick and work on. It’s also reassuring for people. If it feels like you’ve got 100 problems, let’s rank these and pick one. The rest aren’t forgotten, we’ll come back to it. It has been a super-useful tool.
The Cynefin Framework, and Wardley Mapping
My next example of trying to help things was when I spent some time with the project managers. Outside of software, the Co-op does like, preparing for new rules around Brexit, opening new warehouses, doing things about distribution, all kinds of things. They’ve got projects and program managers and a PMO. They’ve got a waterfall process with just what you’d imagine if you’ve seen PRINCE2 or other things like that, so stage gates and lots of formalism around it. There is some confusion about sometimes when work comes in, if it goes to that part, we do some agile thing. If it goes over there, we do something else. With software or with other things, it’s just your luck about which part of the business it comes into. They were interested in helping the Co-op understand, what are the options and how would you pick? When would you choose different methods of delivery? Because sometimes it just feels like, I like agile, we should do agile. Someone else would say something different.
The best tool I found for helping talk people through was Cynefin, which if you’ve not come across it, definitely look it up. If you have come across it, I recommend this book hugely. There’s so much depth and understanding in it. Tianyi mentioned bounded applicability. That is definitely something that Cynefin talks about a lot. The idea, I think Dave Snowden invented it, to get away from new management fads. The idea that this is what you should do now. It turns out we’re all stupid for doing that thing before. It’s this. It’s this new thing. The fairer answer, the thing that people are happier hearing, and actually what’s true, is probably everything we do has value, but only within a set context. That project management stuff is useful for some kind of work, and this agile stuff is useful for some other kind of work. The difficulty we have is identifying what situation we’re in. That bounded applicability is definitely something that’s talked about a lot.
This is a diagram from the book. There’s different versions of it. It looks quite simple, Cynefin, as it talks about one of these four square things. What kind of thing are you in? When things are clear, you can write down the rules. You can write a recipe to follow. This is the only area where best practice is a sensible idea to talk about. The problem we have is when you’re doing something complex, where you can only experiment, you’re not sure what’s going to change or what the right answer is in this kind of work. We try and apply the same thing everywhere, is where you get in trouble. I’ve seen it go the other way as well. I absolutely love trying things out, seeing what’s wrong. Let’s not assume anything. Maybe we shouldn’t even sell sandwiches, which is fabulous and needs to be done more often in some cases.
Another time, that’s just me wasting time when we know we need to replace network switches in stores. Let’s just get on with it, make a plan and do it. This is good, and it was useful to talk people through. Something that you don’t see in this version is this gap right here. Some of these you can move between. You can understand more about your work and try and move it around there. Or if you want to put more process into something, if you’re sure the way you’re approaching something doesn’t change, you can try and haul something around, put more formalism onto it, try and bound things up. This is the way you do it. Always do it like this. What you don’t see in this one, but you do in a fabulous diagram from somebody else, it’s Martin Berg and Rob England, is that gap, that move between clear and chaotic is a cliff.
The fact that you can’t see it too well in the 2D version is on purpose, because you actually can’t. This is a good example that really made project managers sit up and take notice. If you’ve ever felt like the wheels are coming off the bus, something’s changed in my project, and I don’t actually have tools to put this back together, and I don’t know what to do, that’s what’s happened here. You have fallen into chaos, and the only way back is through experimenting the long way around and hauling back up to there if it makes sense. With that and a few other things, it really got their attention. Rather than asking them to read books and do lots of lessons on it, I think that made people feel, I see what you’re talking about here, I get this, and I’ve been in situations like it. What do you do?
Another really good way to learn more about Cynefin is Liz Keogh, has written loads of accessible blog posts that simplify it. This is something we actually used to rate different types of work, if you think about it. On this scale, people can really relate to that and can understand then, if no one has done this before, then we absolutely cannot do a year-long Gantt chart and tell you exactly what’s going to happen over time. We’re going to have to try and work it out. That idea about, for us, how complex and unknown is this, was important to do.
Another thing I like, and similarly to Cynefin, I think some of the writing or things about it can be quite daunting and off-putting. Even stronger than that is Wardley Mapping. That is another tool that is fabulous to use, but it feels like you have to do a PhD before you properly grasp what’s going on. Then, when you try and walk out to somebody else and be like, can I tell you about this? They’re like, what’s going on? I do not have time to read through all this. Similar to Cynefin, there’s some ideas that can really catch people. Simon Wardley talks about where things are new and uncertain and just coming into being, and where things are just commodities, they’re utilities and plug-in. At one point, it was a sensible decision for businesses to think, should I make my own power for my factories? Now it’s, no, just plug it in. Why would you do that? That shift’s happening all the time with all different kinds of work.
Rather than getting people to draw maps, although there are some folks in the Co-op who have had great success with doing that with stakeholders and getting views on things, we talked about this. The other thing that helped, there’s a list of different qualities that would help you identify what kind of work or what kind of thing you’re going to be doing. If the work you’re doing is to gain a competitive advantage, that’s more to that side. That’s more to the parts of Cynefin where best practice doesn’t really apply. Or maybe it’s more cost of doing business and we want to be more efficient. People are like, I get you. I could identify that.
The interesting thing was, in the same project or program of work, some things might be the key competitive advantage that are uncertain and you want to use something different there. Whereas others, you don’t have to do everything the same way. Others you can plan out and there is no need to have workshops and think about it. There’s a list of different ones of these. For different people, different ones catch them out. It’s, yes, volume. That’s what we’re doing with some of our work. Whereas other people, other types of work. It’s like, yes, we do need to experiment. What does that mean for which tools we should use? That really helped us get away from the idea that if project management isn’t working for you, you just need to plan harder, is what some people think. Or on the agile side, we can’t assume anything. We should always start with discovery and we should work it out from first principles. I think there’s right answers for both.
I put together a decision guide and it was interesting doing this as I got to talk to people in lots of parts of the Co-op. We’ve got such depth of experience and understanding. There is no way I would have been able to write this up and put some of the ideas we’ve talked about together myself. Apart from writing a guide and helping people navigate through how to do different things, so many people got in touch with people and had such useful conversations. I’d recommend doing that. Some interesting things that came out of it was things like, shouldn’t we have discovery, alpha, beta, live everywhere? Project management side are very familiar with, you’ve got defined processes and everyone goes through them. I would say, no, that’s designed to slow you down. That’s designed to make you stop and think. Sometimes that’s useful. Give an example of the operational innovation store. It does lots of work about store processes, like how can we change them and make them more efficient? They’ve got a version of that discovery, alpha, beta, live.
When they look at something like leakage, where’s our stock going? Is that getting stolen, or spilled, or misrecorded? They have no idea what the problem is. There’s no way you could get funding and start off saying, this is how we’re going to solve it. There’s an example here of a smart gap. At one point you had paper, you had to go around and see what the gaps in the shelves were and do that. That’s part of the inventory process, knowing what you have to reorder. It took ages and it wasted so many trees. Through an exploratory process of what would work and actually going into a store and spending lots of time with the people there, they came up with this technical solution that saves 15 minutes per worker, per store, per day across thousands of stores, and something like 5 million sheets of paper a year as we don’t have to keep shoeboxes full of paper in the back anymore. That started out very exploratory. Then their alpha phase would be trying things out in that one store.
Then you would move on to, let’s go to a couple of stores but let’s put a lot of attention in and be prepared to tweak it and change it as we realize it’s not working. Then later that just flips to quite unknown. It’s still tricky to implement, but how do we buy thousands of these? What order do we visit the stores in? How do we set up training? I can absolutely see that flipping from exploratory agile through to trying to optimize it, through to something plan driven, and waterfall seems like a sensible answer at the end. Should everyone do that? No. If you’ve got something like our online e-commerce site, when we want to try something, you can just go and try it.
If it works, you leave it on, and if it doesn’t work, you turn it off. I think right now if we all went to shop.coop.co.uk, I think 1% of us would see the different version of this site that they’re trying out now and some new technology in a different setup. If that goes well, that will ramp up to 10%, 50%, as everybody. When we’re making those tweaks and incremental improvements, there’s no need to put that formal process around it.
How is it going? What I would have loved, that idea of Cynefin and what kind of work is this, I would have loved people to formally go through each new bit of work, how can we split it down, answer the questions, and build up like a case library. It’s not just saying it has these qualities, it’s like, do you remember this? Is this one of those? That would be so helpful. Record, when we’ve suggested working in this way, did it actually help? Come back to the projects later and look at them. That’s absolutely not happening. It’s a big read. People are busy, and they’re not going for it.
One thing I like from Simon Wardley’s work, he says, quite often it’s the act of making the map that is more valuable than what you get out at the end. Again, I might be kidding myself to make myself feel better but those conversations and that understanding, the light bulb moments I had as I talked to people, felt super valuable. The fact that the process as described in the 20-page document I’ve got about the decision process isn’t getting used, maybe it doesn’t matter and maybe it will get used one day.
Building Community
I did mention briefly earlier that the Co-op Digital was separate to lots of parts of the Co-op. In different areas, they had some agile teams but they also had some software written in quite a traditional project way, so you might never meet the testers, you might be 20% on this and 20% on something else. Possibly due to time with the project managers, possibly due to all those other conversations and really qualified people around the Co-op, that’s changed. Digital technology and data isn’t scattered anymore, it’s now something over 700 people. By and large, every single team, if you’ve got a software system, that’s not just for Christmas. Everything’s owned, and it should be in a sensible size of team. That team has responsibility for keeping it maintained, for keeping the tech debt down, for helping navigate their own roadmaps and stuff. For loads of people this was brand new. To help, I tried building a community. It was called the Ways of Working community. I suggested, let’s just get together.
A lot of people don’t actually know what agile looks like, what do those teams do? I quite like that since COVID times Co-ops stayed pretty much, mostly remote. You can go in the office if you want. In the old days, if I thought, I don’t know who will come to the community, might be 5, might be 200, I don’t know where you’d find a room for that. I really do like the idea that you can just put a calendar invite in and put a few messages online and see who turns up. Loads of people did. I think when something’s new and exciting people come along to it, but it can die away if you’re not clear about what it’s for.
In building community, if you’re interested in doing that at all, I thoroughly encourage you to check out Emily Webber’s work. We started talking about purpose. A lot of people hadn’t seen a community of practice yet before. There’s these posters on Emily Webber’s website about like, what’s it for? This was useful. That’s the “Communities of Practice” book that I also recommend getting. Getting people together and some of them using Miro for the first time, we all encouraged each other to talk in small groups and apart. I think there was something like 90 people in the first session.
We managed to collaboratively put together, what is this community for? Because I wanted to come back and we put things up, and if you agree with it, you can add some emojis, if you disagree, you can add different post-its. What if we came back together in three months, and how many people would you want to get together? What statements would they agree with? How would we measure that? I think this was good because it was something we kept checking back in on. Lots of things start off with loads of excitement and then fizzle. What keeps it going? This is something we came back to every couple of months. The other innovation I super liked was these little profiles. Write down why you’re here, what do you want to learn? Help us choose topics. Also, what could you share? Because I’ll come and nudge you, and try and get you to do things. We made a souvenir badge for each topic you went on. You can see the kickoff was there.
Some of the topics, the things people were willing to share was really impressive to me. We had modern agile practices and how close I’ve seen Co-op teams get to them, which was interesting. It was a tour. People like, that’s agile stuff, could it ever work at the Co-op? It does. It has. That’s got practical examples from all kinds of places. Technical excellence and why it’s important. Some of the ones I really liked were from teams brand new to working as a long-running agile team. Cloud journey and the identity team were talking about, we’re trying this. In many ways that’s more useful to people. If somebody has been doing something for absolutely years, you’re like, I don’t know when I’ll learn. How do you know how to work these ways? If it’s somebody who’s just tried it this week and they’re really happy to share what they’re trying out and whether it’s working or not, often, that’s what people want to hear. It was really good. Potentially more successful than some of the talks and write-ups we shared was the badges.
If you’re interested in making your own, here’s a detailed guide to setting them up in Miro. When people agree to speak, they really like the chance to design their own badge and see what’s going to be on people’s profiles afterwards.
One other thing that grew out of that was another experiment I tried out, Lean Agile University. I’d come across this at one point, so Jez Humble co-wrote “Accelerate,” and “Lean Enterprise,” and loads of other stuff. He teaches at Berkeley University in the States, and he’s put his short lectures and the activities you can do online. He teaches a course in product management to people learning software engineering. Why does any of this stuff matter? I could not describe how excited I was to find this. I said at the start of running the training course, if you’re doing like a fantasy football style, who’s your best team for teaching you this stuff? I think Jez Humble might be my first one. I cannot believe he’s put this all here. We tried. We made Miro boards about all the topics, and worked through it. I think it’s all Creative Commons, so you can remix this, you can use that, you can do things like that.
We all watched the short videos separately, but you make notes and comments on it together on the Miro board, and then you come back together to do some of the different activities, try out user story mapping. It was a really good two-day course. There were loads of people who had never met each other in the Co-op. There were some fabulous eye-opening moments, so somebody said, so a team that has metrics it wants to move, and you choose your work depending on what happens every week. That could never happen here. The way teams get funded, you get set up with a project, you have to do a thing that the stakeholder has decided. Someone else in the course said, no, we do that. We’re doing that right now. I think the legal services team actually shared some screenshots and we kept it for future versions of the course, of here’s an A/B test we’re running right now, here’s the metrics we’re trying to move, and if that doesn’t work, we’re going to do something different.
Just that eye-opening, this isn’t just some Silicon Valley dream story, this is happening in a team right next to me, was fabulous. Other things that came out of that that were really useful was, I was learning absolutely loads as well. Jez Humble talks about personas and how important that is. I got people to share, did we do that at the Co-op? There are some examples there of what’s going on. At one point a senior designer said, actually, X, Y, and Z are reasons why personas don’t really work for us as much, we’re moving more towards these missions that people are going on. That matters more than the individual. There’s a Co-op Digital blog post about that. I was like, I had no idea. That was something that we shifted. I think that designer actually came and co-hosted a two-day training course to help share the load.
It was just interesting to get different perspectives. We moved towards the rare and coveted university facilitator badge as people in different roles. There was people quite new to their engineering career talking about their perspective of it. There was designers talking about what they see, as well as senior engineering leaders. Everyone put their own different focus on what they thought was important in the course. That was called Operation Not Just New, and it handed over quite well.
How’s it going? Lean Agile University is still going. You can get bespoke versions for your own team. Because I think that that go wide and meet people you’ve never met before is fascinating. Doing it for a specific team is really useful. There’s also a self-paced version. It’s only internal at the moment. It’s been useful. It’s been eye-opening. It’s still something that people refer to now about, do you know like we did on the course? It warms my heart when I hear about somebody using a technique, years later, that they first saw on that course.
What’s Next?
What’s next? There’s other things going on. There’s too much to talk about. It’s always hard. It’s never perfect. There’s always more things to try. I think if I was to sum up what I’ve learned from putting this talk together and reflecting on it, is, be boring. Keep banging the drum. I’ve written advice for people about, with the seven things or other things that are important, your job is to be a broken record. Because you’d be astonished how fast things get forgotten about. The kind of help people need is I think they can do anything. They can copy the outer forms of whatever you tell them is important. That’s not what I want. Be super clear on that. There’s something here that is meant to solve a problem. It will feel like this problem is getting solved if you’re doing this thing, and you can’t see it solving a problem. You should either stop or you should ask somebody about it. If you’ve ever seen a user story starting with, as a database, I want to be updated. You know what I mean by people can copy the outer forms of things. That’s not what I want you to do.
The last one is, make it fun. Make it welcoming. The Ways of Working community and the lean agile unit in particular is some of the most fun I’ve had at work. Those rare and coveted facilitator badges are still up for grabs, I think, as more people hopefully will co-facilitate that in future.
Resources
If you want to know more about the Co-op, I’ve mentioned the digital blog a few times, digitalblog.coop.co.uk. There are years of what teams have done, how the Co-op works, and things like that.
See more presentations with transcripts
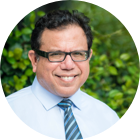
MMS • RSS
Posted on mongodb google news. Visit mongodb google news
MongoDB (NASDAQ:MDB – Free Report) had its target price trimmed by Truist Financial from $300.00 to $275.00 in a research report sent to investors on Monday morning,Benzinga reports. The firm currently has a buy rating on the stock.
A number of other analysts have also weighed in on the stock. Guggenheim upgraded shares of MongoDB from a “neutral” rating to a “buy” rating and set a $300.00 price objective for the company in a research note on Monday, January 6th. Barclays reduced their price target on shares of MongoDB from $330.00 to $280.00 and set an “overweight” rating for the company in a research report on Thursday, March 6th. Wells Fargo & Company cut MongoDB from an “overweight” rating to an “equal weight” rating and lowered their price objective for the company from $365.00 to $225.00 in a research report on Thursday, March 6th. KeyCorp downgraded MongoDB from a “strong-buy” rating to a “hold” rating in a research note on Wednesday, March 5th. Finally, Scotiabank restated a “sector perform” rating and issued a $240.00 price target (down previously from $275.00) on shares of MongoDB in a research note on Wednesday, March 5th. Seven equities research analysts have rated the stock with a hold rating and twenty-three have given a buy rating to the company. Based on data from MarketBeat, MongoDB has a consensus rating of “Moderate Buy” and a consensus target price of $319.87.
Check Out Our Latest Stock Analysis on MDB
MongoDB Stock Down 1.5 %
NASDAQ MDB opened at $175.40 on Monday. The stock’s 50 day moving average is $244.01 and its 200 day moving average is $265.11. The firm has a market capitalization of $14.24 billion, a PE ratio of -64.01 and a beta of 1.30. MongoDB has a twelve month low of $170.85 and a twelve month high of $387.19.
MongoDB (NASDAQ:MDB – Get Free Report) last released its earnings results on Wednesday, March 5th. The company reported $0.19 earnings per share for the quarter, missing analysts’ consensus estimates of $0.64 by ($0.45). MongoDB had a negative return on equity of 12.22% and a negative net margin of 10.46%. The business had revenue of $548.40 million during the quarter, compared to analysts’ expectations of $519.65 million. During the same period in the prior year, the company earned $0.86 earnings per share. On average, research analysts anticipate that MongoDB will post -1.78 earnings per share for the current year.
Insider Buying and Selling
In other MongoDB news, CEO Dev Ittycheria sold 8,335 shares of the stock in a transaction on Friday, January 17th. The shares were sold at an average price of $254.86, for a total transaction of $2,124,258.10. Following the completion of the transaction, the chief executive officer now owns 217,294 shares in the company, valued at $55,379,548.84. This trade represents a 3.69 % decrease in their ownership of the stock. The transaction was disclosed in a document filed with the Securities & Exchange Commission, which is available through this link. Also, CFO Michael Lawrence Gordon sold 1,245 shares of the business’s stock in a transaction dated Thursday, January 2nd. The stock was sold at an average price of $234.09, for a total transaction of $291,442.05. Following the completion of the sale, the chief financial officer now owns 79,062 shares in the company, valued at approximately $18,507,623.58. This trade represents a 1.55 % decrease in their ownership of the stock. The disclosure for this sale can be found here. Over the last quarter, insiders sold 43,139 shares of company stock worth $11,328,869. 3.60% of the stock is owned by insiders.
Institutional Trading of MongoDB
Institutional investors have recently added to or reduced their stakes in the company. B.O.S.S. Retirement Advisors LLC purchased a new stake in shares of MongoDB in the fourth quarter worth approximately $606,000. Geode Capital Management LLC increased its stake in MongoDB by 2.9% in the third quarter. Geode Capital Management LLC now owns 1,230,036 shares of the company’s stock worth $331,776,000 after purchasing an additional 34,814 shares during the period. Union Bancaire Privee UBP SA bought a new stake in shares of MongoDB during the fourth quarter worth $3,515,000. Nisa Investment Advisors LLC boosted its stake in shares of MongoDB by 428.0% during the fourth quarter. Nisa Investment Advisors LLC now owns 5,755 shares of the company’s stock valued at $1,340,000 after purchasing an additional 4,665 shares during the period. Finally, HighTower Advisors LLC grew its holdings in shares of MongoDB by 2.0% in the fourth quarter. HighTower Advisors LLC now owns 18,773 shares of the company’s stock worth $4,371,000 after purchasing an additional 372 shares during the last quarter. Institutional investors own 89.29% of the company’s stock.
About MongoDB
MongoDB, Inc, together with its subsidiaries, provides general purpose database platform worldwide. The company provides MongoDB Atlas, a hosted multi-cloud database-as-a-service solution; MongoDB Enterprise Advanced, a commercial database server for enterprise customers to run in the cloud, on-premises, or in a hybrid environment; and Community Server, a free-to-download version of its database, which includes the functionality that developers need to get started with MongoDB.
Featured Stories
Receive News & Ratings for MongoDB Daily – Enter your email address below to receive a concise daily summary of the latest news and analysts’ ratings for MongoDB and related companies with MarketBeat.com’s FREE daily email newsletter.
Article originally posted on mongodb google news. Visit mongodb google news
Azure Container Apps Serverless GPUs Reach General Availability with NVIDIA NIM Support
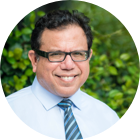
MMS • Steef-Jan Wiggers
Article originally posted on InfoQ. Visit InfoQ
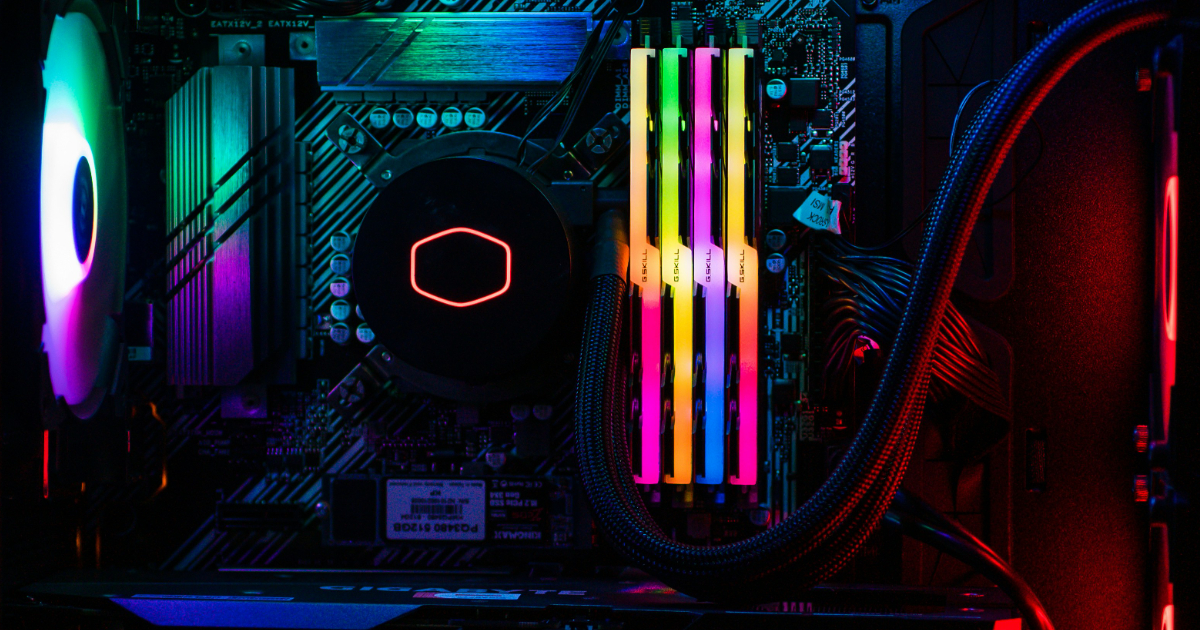
Azure has expanded its GPU computing offerings with the general availability of Serverless GPUs within Azure Container Apps, providing an on-demand execution environment for AI workloads. This new capability, powered by NVIDIA A100 and T4 GPUs and now supporting NVIDIA NIM microservices, simplifies the deployment and management of GPU-accelerated tasks such as real-time custom model inference, automatically scaling resources and billing only for active compute time.
Positioned to offer a more flexible option between fully-managed AI services and self-managed GPU VMs, Serverless GPUs enable developers to focus on their containerized AI applications. At the same time, Azure handles the underlying infrastructure, offering a cost-effective and scalable solution for various AI inference and compute-intensive scenarios.
The GA release introduces support for NVIDIA NIM microservices, part of NVIDIA AI Enterprise. NVIDIA NIM offers a suite of user-friendly microservices tailored for the secure and reliable deployment of high-performance AI model inference at scale. Supporting a wide array of AI models, including open-source and NVIDIA AI Foundation models, NVIDIA NIM integrates with industry-standard APIs for scalable AI inferencing.
In an Azure Compute blog post, the company states:
With the support of NVIDIA NIM, development teams can easily build and deploy generative AI applications alongside existing applications within the same networking, security, and isolation boundary.
To utilize NVIDIA NIM with serverless GPUs, users can explore the NVIDIA API catalog and select a ‘Run Anywhere’ NIM. An NGC_API_KEY must be set as an environment variable during Azure Container Apps deployment. Detailed instructions for adding a NIM to a container app are available. It’s important to note that each NIM model has specific hardware requirements, and Azure Container Apps serverless GPUs support NVIDIA A100 and T4 GPUs.
Steve Nouri, a co-founder of GenAI Works, Tweeted on X what NVIDIA NIM provides:
Supports a wide range of AI models, including large language models (LLMs), vision language models (VLMs), and models for speech, images, video, 3D, drug discovery, and medical imaging. Ideal for building chatbots, AI assistants, and advanced analytics systems.
While the key benefits of serverless GPUs include:
- Scale-to-zero GPUs: Automatic serverless scaling of NVIDIA A100 and T4 GPUs.
- Per-second billing: Payment only for the GPU compute consumed.
- Built-in data governance: Data remains within the container boundary.
- Flexible compute options: Choice between NVIDIA A100 and T4 GPUs.
- Middle-layer for AI development: Ability to bring custom models to a managed, serverless compute platform.
When you create a container app through the Azure portal, users can configure their container to utilize GPU resources:
- NVIDIA T4: Real-time and batch inferencing using custom open-source models for dynamic applications.
- NVIDIA A100: For compute-intensive machine learning scenarios, such as fine-tuning custom generative AI models, deep learning, and neural networks, as well as high-performance computing (HPC) and data analytics.
Raul E Garcia, an applied mathematician and software engineer, concluded in a LinkedIn blog post the benefits of T4 and A100 GPUs:
Together, the T4 and A100 GPUs make AI more accessible, affordable, and efficient, from research and training to large-scale deployment. By optimizing for both training and inference, they provide a balanced foundation for expanding AI capabilities across industries and application types.
With the GA release, default GPU quotas are being introduced for both enterprise and pay-as-you-go customers, covering quotas for A100 and T4 GPUs. The feature is currently supported in the West US 3, Australia East, and Sweden Central regions.
In the announcement blog post, comments were made by BC2112 about the availability:
Honestly, when is this going to be supported in more regions? Frustrating.
Users can enable GPUs for Consumption apps during Container App or Container App Job creation in the Azure portal’s container tab. Existing Container App environments can also have a new consumption GPU workload profile added via the portal’s workload profiles UX or CLI commands. For optimal performance, utilizing an Azure Container Registry (ACR) with artifact streaming enabled is recommended [link to enable artifact streaming].
For more details on getting started with serverless GPUs, the company refers to the QuickStart.
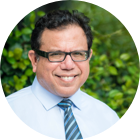
MMS • RSS
Posted on mongodb google news. Visit mongodb google news
Korea Investment CORP grew its stake in shares of MongoDB, Inc. (NASDAQ:MDB – Free Report) by 20.3% in the fourth quarter, according to the company in its most recent filing with the Securities and Exchange Commission (SEC). The fund owned 58,871 shares of the company’s stock after acquiring an additional 9,936 shares during the quarter. Korea Investment CORP owned about 0.08% of MongoDB worth $13,706,000 at the end of the most recent quarter.
Other large investors have also modified their holdings of the company. Hilltop National Bank raised its stake in MongoDB by 47.2% during the fourth quarter. Hilltop National Bank now owns 131 shares of the company’s stock worth $30,000 after purchasing an additional 42 shares during the period. NCP Inc. acquired a new stake in shares of MongoDB during the 4th quarter worth $35,000. Continuum Advisory LLC raised its position in shares of MongoDB by 621.1% in the 3rd quarter. Continuum Advisory LLC now owns 137 shares of the company’s stock worth $40,000 after buying an additional 118 shares during the period. Versant Capital Management Inc boosted its holdings in MongoDB by 1,100.0% in the fourth quarter. Versant Capital Management Inc now owns 180 shares of the company’s stock valued at $42,000 after acquiring an additional 165 shares during the last quarter. Finally, Wilmington Savings Fund Society FSB purchased a new stake in MongoDB during the third quarter valued at about $44,000. 89.29% of the stock is currently owned by hedge funds and other institutional investors.
MongoDB Price Performance
Shares of MDB opened at $178.03 on Monday. The stock has a market capitalization of $14.45 billion, a PE ratio of -64.97 and a beta of 1.30. The business’s fifty day moving average price is $245.56 and its 200 day moving average price is $265.95. MongoDB, Inc. has a 12-month low of $173.13 and a 12-month high of $387.19.
MongoDB (NASDAQ:MDB – Get Free Report) last posted its quarterly earnings results on Wednesday, March 5th. The company reported $0.19 earnings per share (EPS) for the quarter, missing analysts’ consensus estimates of $0.64 by ($0.45). MongoDB had a negative return on equity of 12.22% and a negative net margin of 10.46%. The business had revenue of $548.40 million during the quarter, compared to the consensus estimate of $519.65 million. During the same quarter in the previous year, the business posted $0.86 earnings per share. As a group, research analysts anticipate that MongoDB, Inc. will post -1.78 earnings per share for the current fiscal year.
Insider Activity at MongoDB
In other MongoDB news, insider Cedric Pech sold 287 shares of the business’s stock in a transaction dated Thursday, January 2nd. The shares were sold at an average price of $234.09, for a total transaction of $67,183.83. Following the completion of the transaction, the insider now directly owns 24,390 shares of the company’s stock, valued at $5,709,455.10. The trade was a 1.16 % decrease in their position. The transaction was disclosed in a document filed with the SEC, which is available at this hyperlink. Also, Director Dwight A. Merriman sold 3,000 shares of the firm’s stock in a transaction that occurred on Monday, March 3rd. The shares were sold at an average price of $270.63, for a total transaction of $811,890.00. Following the completion of the sale, the director now owns 1,109,006 shares in the company, valued at $300,130,293.78. The trade was a 0.27 % decrease in their position. The disclosure for this sale can be found here. Insiders sold 43,139 shares of company stock worth $11,328,869 in the last 90 days. 3.60% of the stock is currently owned by company insiders.
Analysts Set New Price Targets
Several research firms have recently weighed in on MDB. Morgan Stanley reduced their price objective on MongoDB from $350.00 to $315.00 and set an “overweight” rating on the stock in a research note on Thursday, March 6th. JMP Securities reiterated a “market outperform” rating and set a $380.00 price objective on shares of MongoDB in a research note on Wednesday, December 11th. Wells Fargo & Company downgraded shares of MongoDB from an “overweight” rating to an “equal weight” rating and cut their target price for the company from $365.00 to $225.00 in a research note on Thursday, March 6th. China Renaissance began coverage on shares of MongoDB in a research report on Tuesday, January 21st. They set a “buy” rating and a $351.00 price target for the company. Finally, Needham & Company LLC lowered their price target on MongoDB from $415.00 to $270.00 and set a “buy” rating for the company in a report on Thursday, March 6th. Seven research analysts have rated the stock with a hold rating and twenty-three have issued a buy rating to the company’s stock. Based on data from MarketBeat.com, the company currently has an average rating of “Moderate Buy” and a consensus price target of $320.70.
Check Out Our Latest Analysis on MongoDB
MongoDB Company Profile
MongoDB, Inc, together with its subsidiaries, provides general purpose database platform worldwide. The company provides MongoDB Atlas, a hosted multi-cloud database-as-a-service solution; MongoDB Enterprise Advanced, a commercial database server for enterprise customers to run in the cloud, on-premises, or in a hybrid environment; and Community Server, a free-to-download version of its database, which includes the functionality that developers need to get started with MongoDB.
Featured Stories
This instant news alert was generated by narrative science technology and financial data from MarketBeat in order to provide readers with the fastest and most accurate reporting. This story was reviewed by MarketBeat’s editorial team prior to publication. Please send any questions or comments about this story to contact@marketbeat.com.
Before you consider MongoDB, you’ll want to hear this.
MarketBeat keeps track of Wall Street’s top-rated and best performing research analysts and the stocks they recommend to their clients on a daily basis. MarketBeat has identified the five stocks that top analysts are quietly whispering to their clients to buy now before the broader market catches on… and MongoDB wasn’t on the list.
While MongoDB currently has a Moderate Buy rating among analysts, top-rated analysts believe these five stocks are better buys.
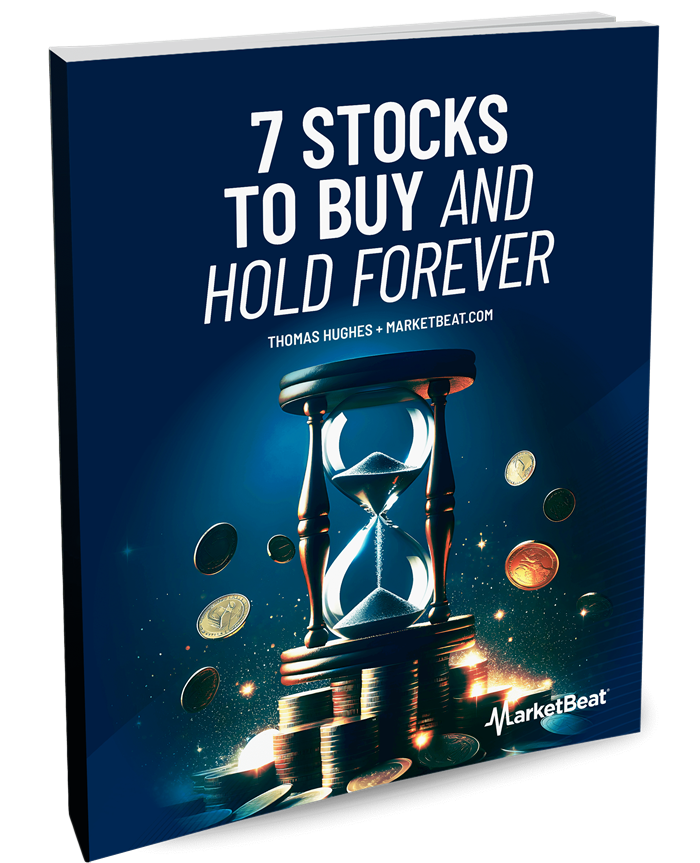
Enter your email address and we’ll send you MarketBeat’s list of seven stocks and why their long-term outlooks are very promising.
Article originally posted on mongodb google news. Visit mongodb google news
Interop 2025: Anchor Positioning, View Transitions, Storage Access Soon Stable Across Browsers
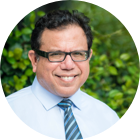
MMS • Bruno Couriol
Article originally posted on InfoQ. Visit InfoQ
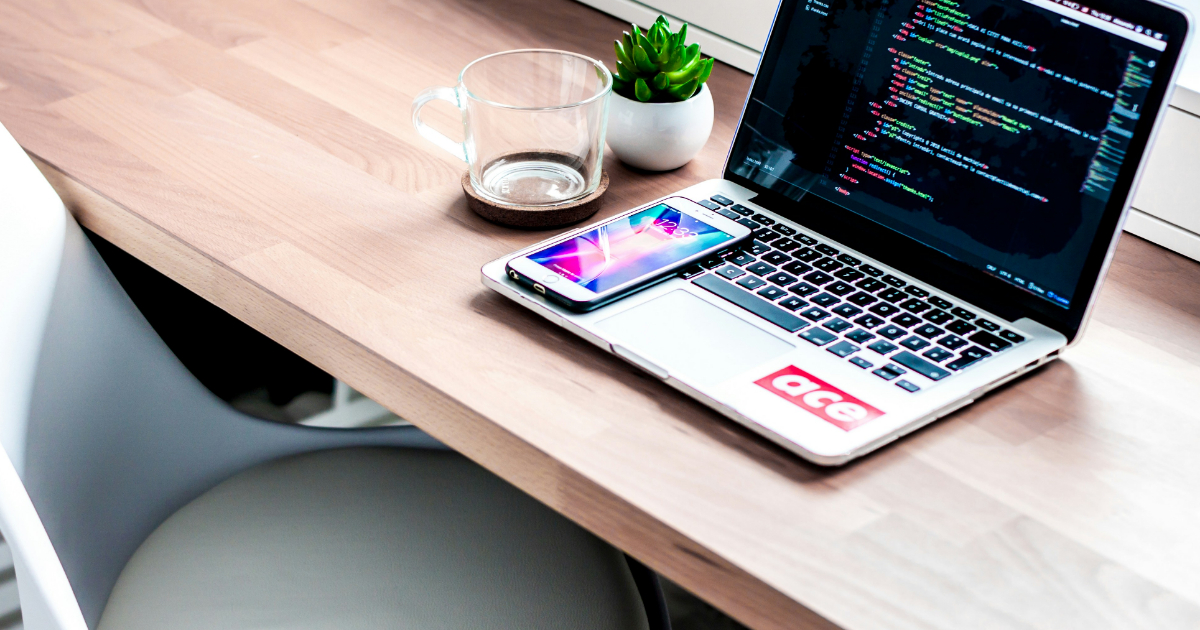
The features of Interop 2025 are now known. The list, whose items are to be made implemented and stable across browsers by the end of the year, includes anchor positioning, the View Transitions API, the Storage Access API, and more for a total of 19 focus areas.
With popovers already at over 90% browser support, CSS anchor positioning allows positioning an element in relation to another element. Positioning tooltips, popovers, and dropdowns thus becomes easier and declarative, eliminating the need for JavaScript and third-party libraries. Anchor positioning is currently not supported in Firefox or Safari.
The View Transition API provides a mechanism for easily creating animated transitions between different website views. The API has two major use cases: same-document view transitions (e.g., in a SPA), and transitioning between documents in a multi-page application (MPA). Same-document view transitions should be stable in every browser by the end of 2025.
Transitions have numerous benefits for users. Well-animated transitions between pages are likely to reduce the perception of latency and help users stay in context. Transitions are also a common feature of mobile applications. Web developers can use view transitions to reduce the user experience gap between native mobile applications and web applications.
As with CSS anchor positioning, support across all major browsers would eliminate the need for custom JavaScript or third-party libraries. Performance and smoothness are key requirements for animations. View transitions previously required significant effort and the use of large amounts of CSS and JavaScript, which introduced jank in animations, thus defeating the original purpose.
The Storage Access API helps manage cookies across different origins while respecting privacy and security standards. The API provides methods that allow embedded resources that have a legitimate need for third-party cookies or unpartitioned state access to check whether they currently have access and, if not, to request access from the user agent.
The MDN website explains:
Depending on the browser, the user will be asked whether to grant access to the requesting embed in slightly different ways.
– Safari shows prompts for all embedded content that has not previously received storage access.
– Firefox only prompts users after an origin has requested storage access on more than a threshold number of sites.
– Chrome shows prompts for all embedded content that has not previously received storage access. It will however automatically grant access and skip prompts if the embedded content and embedding site are part of the same related website set.
In addition to the three features detailed previously, Interop totals 19 active focus areas for 2025 and 5 active investigations (e.g., Accessibility testing, Gamepad API testing, Mobile testing, Privacy testing, and WebVTT). Developers can review progress on the listed items at any time through the online Interop 2025 Dashboard.
Developers on Reddit emphasized the importance of the new features.
[sharlos]:
Excited for the Navigation API finally getting support. Will finally eliminate the need for complex libraries for SPA navigation.[nickbreaton]:
Anchor positioning is going to be huge!
Browser makers Apple, Google, Microsoft, and Mozilla, alongside consultancies Bocoup and Igalia are sponsoring Interop, a project to promote web browser interoperability. Interop 2024 ended with a median of 98% of tests passing across browsers in the stable release channel and 99% in the canary/nightly release channel.
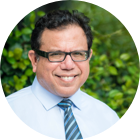
MMS • RSS
Posted on mongodb google news. Visit mongodb google news
MongoDB, Inc. (NASDAQ:MDB – Get Free Report)’s stock price reached a new 52-week low on Monday after Truist Financial lowered their price target on the stock from $300.00 to $275.00. Truist Financial currently has a buy rating on the stock. MongoDB traded as low as $171.21 and last traded at $174.84, with a volume of 155238 shares traded. The stock had previously closed at $178.03.
Several other equities analysts also recently weighed in on MDB. The Goldman Sachs Group dropped their price objective on MongoDB from $390.00 to $335.00 and set a “buy” rating for the company in a research note on Thursday, March 6th. Piper Sandler dropped their price target on MongoDB from $425.00 to $280.00 and set an “overweight” rating for the company in a research report on Thursday, March 6th. China Renaissance initiated coverage on shares of MongoDB in a research report on Tuesday, January 21st. They issued a “buy” rating and a $351.00 price objective on the stock. Macquarie lowered their price objective on shares of MongoDB from $300.00 to $215.00 and set a “neutral” rating on the stock in a research note on Friday, March 7th. Finally, Stifel Nicolaus cut their target price on shares of MongoDB from $425.00 to $340.00 and set a “buy” rating for the company in a research report on Thursday, March 6th. Seven investment analysts have rated the stock with a hold rating and twenty-three have issued a buy rating to the company’s stock. Based on data from MarketBeat.com, MongoDB has a consensus rating of “Moderate Buy” and an average price target of $319.87.
View Our Latest Stock Report on MongoDB
Insider Buying and Selling at MongoDB
In related news, CAO Thomas Bull sold 169 shares of the stock in a transaction on Thursday, January 2nd. The stock was sold at an average price of $234.09, for a total value of $39,561.21. Following the completion of the sale, the chief accounting officer now directly owns 14,899 shares in the company, valued at approximately $3,487,706.91. This represents a 1.12 % decrease in their position. The sale was disclosed in a document filed with the Securities & Exchange Commission, which is accessible through the SEC website. Also, Director Dwight A. Merriman sold 3,000 shares of the business’s stock in a transaction dated Monday, March 3rd. The stock was sold at an average price of $270.63, for a total value of $811,890.00. Following the transaction, the director now owns 1,109,006 shares in the company, valued at approximately $300,130,293.78. This represents a 0.27 % decrease in their position. The disclosure for this sale can be found here. Over the last 90 days, insiders sold 43,139 shares of company stock valued at $11,328,869. Company insiders own 3.60% of the company’s stock.
Institutional Trading of MongoDB
Several large investors have recently bought and sold shares of the company. Cerity Partners LLC grew its holdings in MongoDB by 8.3% during the 3rd quarter. Cerity Partners LLC now owns 9,094 shares of the company’s stock valued at $2,459,000 after purchasing an additional 695 shares in the last quarter. The Manufacturers Life Insurance Company boosted its position in MongoDB by 3.8% in the 3rd quarter. The Manufacturers Life Insurance Company now owns 28,101 shares of the company’s stock valued at $7,596,000 after buying an additional 1,022 shares during the last quarter. MetLife Investment Management LLC boosted its position in MongoDB by 1.6% in the 3rd quarter. MetLife Investment Management LLC now owns 4,450 shares of the company’s stock valued at $1,203,000 after buying an additional 72 shares during the last quarter. Zurcher Kantonalbank Zurich Cantonalbank grew its stake in shares of MongoDB by 23.3% during the third quarter. Zurcher Kantonalbank Zurich Cantonalbank now owns 15,143 shares of the company’s stock valued at $4,094,000 after acquiring an additional 2,858 shares in the last quarter. Finally, Icon Wealth Advisors LLC purchased a new stake in shares of MongoDB during the third quarter worth about $109,000. Institutional investors and hedge funds own 89.29% of the company’s stock.
MongoDB Stock Down 1.5 %
The firm has a market cap of $14.24 billion, a price-to-earnings ratio of -64.01 and a beta of 1.30. The company has a 50 day moving average of $244.01 and a 200 day moving average of $265.11.
MongoDB (NASDAQ:MDB – Get Free Report) last announced its earnings results on Wednesday, March 5th. The company reported $0.19 earnings per share (EPS) for the quarter, missing analysts’ consensus estimates of $0.64 by ($0.45). MongoDB had a negative net margin of 10.46% and a negative return on equity of 12.22%. The business had revenue of $548.40 million during the quarter, compared to analyst estimates of $519.65 million. During the same period in the previous year, the company earned $0.86 earnings per share. As a group, equities research analysts expect that MongoDB, Inc. will post -1.78 earnings per share for the current fiscal year.
About MongoDB
MongoDB, Inc, together with its subsidiaries, provides general purpose database platform worldwide. The company provides MongoDB Atlas, a hosted multi-cloud database-as-a-service solution; MongoDB Enterprise Advanced, a commercial database server for enterprise customers to run in the cloud, on-premises, or in a hybrid environment; and Community Server, a free-to-download version of its database, which includes the functionality that developers need to get started with MongoDB.
Read More
Receive News & Ratings for MongoDB Daily – Enter your email address below to receive a concise daily summary of the latest news and analysts’ ratings for MongoDB and related companies with MarketBeat.com’s FREE daily email newsletter.
Article originally posted on mongodb google news. Visit mongodb google news
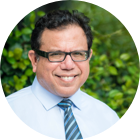
MMS • RSS
Posted on mongodb google news. Visit mongodb google news
Temporal Technologies, a Seattle, WA-based inventor of open-source durable execution platforms Temporal.io and Temporal Cloud, raised $146M in funding, at $1.72 Billion valuation.
The round was led by Tiger Global with participation from StepStone Group, Amplify Partners, Index Ventures, MongoDB Ventures, Sequoia Capital, Conversion Capital, Hanwha Next Generation Opportunity Fund, and 137 Ventures.
The company intends to use the funds to accelerate product development for its enterprise-grade managed service Temporal Cloud and expand the reach of its durable execution platform beyond mission-critical workloads in payments, e-commerce, air travel, and disruptive digitally native companies, and into AI use cases of all types.
Temporal provides an open-source durable execution platform that guarantees the execution of complex workflows even in the face of system failures and allows developers to focus entirely on business logic. Its polyglot capabilities allow orchestration across multiple programming languages, making it ideal for both traditional enterprise applications and next-generation AI workloads. Temporal Cloud, the company’s managed service backed by the originators of the project, has been adopted by over 2,500 customers globally,
Temporal recently added B2B software veteran Jim Cyb as President to lead go-to-market efforts and announced the appointment of Sahir Azam, Chief Product Officer of MongoDB, as its first independent board member.
FinSMEs
31/03/2025
Article originally posted on mongodb google news. Visit mongodb google news
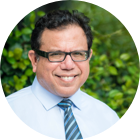
MMS • Amitai Stern
Article originally posted on InfoQ. Visit InfoQ
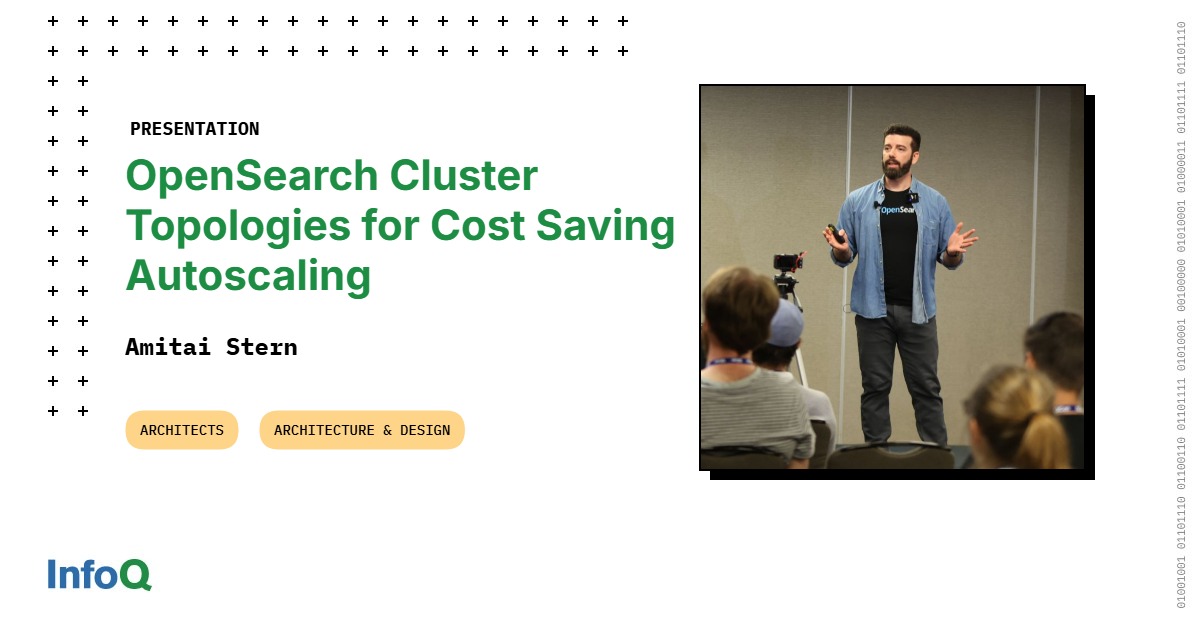
Transcript
Stern: We’re going to talk about topologies for cost-saving autoscaling. Just to get you prepared, it’s not going to be like I’m showing you, this is how you’re going to autoscale your environment, but rather ways to think about autoscaling, and what are the pitfalls and the architecture of OpenSearch that limit autoscaling in reality. I’m going to start talking about storing objects, actual objects, ice-core samples. Ice-core samples are these cylinders drilled from ice sheets or glaciers, and they provide us a record of Earth’s climate and environment.
The interesting thing, I believe, and relevant to us in these ice-core samples is that when they arrive at the storage facility, they are parsed. If you think about it, this is probably the most columnar data of any columnar data that we have. It’s a literal column. It’s sorted by timestamp. You have the new ice at the top and the old ice at the bottom. The way the scientific community has decided to parse this data is in the middle of the slide. It’s very clear to them that this is how they want it parsed. This person managing the storage facility is going to parse the data that way, all of it. Because the scientific community has a very narrow span of inquiry regarding this type of data, it is easy to store it. It is easy to make it very compact. You can see the storage facility is boring. It’s shelves. Everything is condensed. A visitor arriving at the facility has an easy time looking for things. It’s very well-sorted and structured.
If we take a hypothetical example of lots of visitors coming, and the person here who is managing the storage facility wants to scale out, he wants to be able to accommodate many more visitors at a time. What he’ll do is he’ll take all those ice-core samples, and cut them in half. That’s just time divided by two. That’s easy. Add a room. Put all these halves in another room. You can spread out the load. The read load will be spread out. It really makes things easy. It’s practically easy to think about how you’d scale out such a facility. Let’s talk about a different object storage facility, like a museum, where we don’t really know what kind of samples are coming in. If you have a new sample coming in, it could be a statue, it could be an archaeological artifact, it could be a postmodern sculpture of the Kraken or dinosaur bones. How do we index these things in a way that they’re easy to search? It’s very hard.
One of the things that’s interesting is that a visitor at a museum has such a wide span of inquiry. Like, what are they going to ask you? Or a person managing the museum, how does he index things so that they’re easily queryable? What if someone wants the top-k objects in the museum? He’ll need these too, but they’re from completely different fields. When your objects are unstructured, it’s very hard to store them in a way that is scalable. If we wanted to scale our museum for this hypothetical situation where many visitors are coming, it’s hard to do. Would we have to take half of this dinosaur skeleton and put it in another room? Would we take samples from each exhibit and make a smaller museum on the side? How do you do this? In some real-world cases, there’s a lot of visitors who want to see a specific art piece and it’s hard. How do you scale the Mona Lisa? You cannot. It’s just there and everybody is going to wait in line and complain about it later.
Similarly, to OpenSearch, you can scale it. That’s adding nodes. It’s a mechanical thing. You’re just going to add some machines. Spreading the load when your data is unstructured is difficult. It’s not a straightforward answer. This is why in this particular type of system and in Elasticsearch as well, you don’t have autoscaling native to the software.
Background
I’m Amitai Stern. I’m a member of the Technical Steering Committee of the OpenSearch Software Foundation, leading the OpenSearch software. I’m a tech lead at Logz.io. I manage the telemetry storage team, where we manage petabytes of logs and traces and many terabytes of monitoring and metric data for customers. Our metrics are on Thanos clusters, and everything else that I mentioned, logs and traces, are all going to be stored on OpenSearch clusters.
What Is OpenSearch?
What is OpenSearch? OpenSearch is a fork of Elasticsearch. It’s very similar. It’s been a fork for the last three years. The divergence is not too great. If you’re familiar with Elasticsearch, this is very much relevant as well. OpenSearch is used to bring order to unstructured data at scale. It’s the last line over here. It is a fork of Elasticsearch. Elasticsearch used to be open source. It provided an open-source version, and then later they stopped doing that. OpenSearch is a fork that was primarily driven by AWS, and today it’s completely donated to the Linux Foundation. It’s totally out of their hands at this point.
OpenSearch Cluster Architecture
OpenSearch clusters are monolithic applications. You could have it on one node. From here on in the talk, this rounded rectangle will represent a node. A node in OpenSearch can have many roles. You can have just one and it’ll act as its own little cluster, but you could also have many and they’ll interact together. That’s what monolithic applications are. Usually in the wild, we’ll see clusters divided into three different groups of these roles. The first one would be a cluster manager. The cluster manager nodes are managing the state where indexes are, creating and deleting indexes. There’s coordinating nodes, and they’re in charge of the HTTP requests. They’re like the load balancer for the cluster. Then there’s the data nodes. This is the part that we’re going to want to scale. Normally this is where the data is. The data is stored within a construct called an index. This index contains both the data and the inverted index that makes search fast and efficient.
These indices are split up, divided between the data nodes in a construct called a shard. Shards go from 0 to N. A shard is in fact a Lucene index, just to make things a little bit confusing. You already used the term index, so you don’t need to remember that. They’re like little Lucene databases. On the data nodes are three types of pressure if you’re managing one. You’re managing your cluster. You have the read pressure, all the requests coming in to pull data out as efficiently as possible and quickly as possible, and this write pressure of all these documents coming in. There’s the third pressure when you’re managing a cluster, which is the financial one. Since if your read and writes are fairly low, you’ll get a question from your management or from the CFO like, what’s going on? These things cost a lot of money: all this disk space, all the memory, and CPU cores. It’s three types of pressure.
Why Autoscale?
Let’s move on to why would you even autoscale? Financially, cluster costs a lot of money. We want to reduce the amount of nodes that we have. What if we just had enough to handle the scale? This blue line will be the load. The red line is the load that we can accommodate for with the current configuration. Leave it vague that way. Over-provisioned is blue, and under-provisioned is the red over there. If we said the max load is going to be x, and we’re just going to say, we just provision for there. We’ll have that many nodes. The problem would be that we’re wasting money. This is good in some cases if you have the money to spend. Normally, we’re going to try and reduce that. You opt for manual scaling. Manual scaling is the worst of both worlds. You wait too long to scale up because something’s happening to the system. It’s bad performance. You scale up.
Then you’re afraid to scale down at this point because a second ago, people were complaining, so you’re going to wait too long to scale down. It’s really the worst. Autoscaling is following that curve automatically. That’s what we want. This is the holy grail, some line that follows the load. When we’re scaling OpenSearch, we’re scaling hardware. We have to think about these three elements that we’re scaling. We’re going to scale disk. We’re going to scale memory. We’re going to scale CPU core. These are the three things we want to scale. The load splits off into these three. Read load doesn’t really affect the disk. You can have a lot of read load or less read load. It doesn’t mean you’re going to add disk. We’re going to focus more on the write load and its effects on the cluster, because it affects all three of these components. If there’s a lot of writes, we might need to add more disk, or we might need more CPU cores because the type of writing is a little more complex, or we need more memory.
Vertical and Horizontal Scaling
I have exactly one slide devoted to vertical scaling because when I was going over the talk with other folks, they said, what about vertical scaling? Amazon, behind the scenes when they’re running your serverless platform, they’re going to vertically scale it first. If you have your own data center, it could be easy to do that relatively. Amazon do this because they have the capability to vertically scale easily. If you’re using a cloud, it’s harder. When you scale up, usually you go from one set of machines to the next scale of machines. It means you have to stop that machine and move data. That’s not something that’s easily done normally. Vertically scaling, for most intent and purposes in most companies, is really just the disk. That is easy. You can increase the number of EBS instances. You can increase the disk over there. Horizontal scaling is the thing you need to know how to do.
If you’re managing and maintaining clusters, you have to know how to do this. OpenSearch, you just have to add a node, and it gets discovered by the cluster, and it’s there. Practically, it’s easy. There’s a need to do this because of the load, the changing load. There’s an expectation, however, that when you add a node, the load will just distribute. This is the case in a lot of different projects. Here, similar to the example with the museum, it’s not the case. You have to learn how the load is spread out. You have to actually change that as well. How you’re maintaining the data, you have to change that as you are adding nodes.
If the load is disproportionately hitting one of the nodes, we call that a hotspot. Any of you familiar with what hotspots are? You get a lot of load on one of those nodes, and then writes start to lag. Hotspots are a thing we want to avoid. Which moves us into another place of, how do we actually distribute this data so it’s going to all these nodes in the same fashion and we’re not getting these hotspots? When we index data into OpenSearch, each document gets its own ID. That ID is going to be hashed, and then we’re going to do a Mod of N. N being the number of shards. In this example, the Mod is something that ends with 45, and Mod 4, because we have 4 shards. That would be equal to 1, so it’s going to go to shard number 1. If you have thousands of documents coming in, each with their own unique ID, then they’re going to go to these different shards, and it more or less balances out. It works in reality.
If we wanted to have the capability to just add a shard, make the index just slightly bigger, why can’t we do that? The reason is this hash Mod N. If we were to potentially add another shard, our document is now stored in shard number 1, and we want it to scale up, so we extended the index just a bit.
The next time we want to search for that ID, we’re going to do hash Mod to see where it is. N just changed, it’s 5 and not 4. We’re looking for the document in a different shard, and it is now gone. That’s why we have a fixed number of shards in our indices. We actually can’t change that. When you’re scaling OpenSearch, you have to know this. You can’t just add shards to the index. You have to do something we call a rollover. You take the index that you’re writing to, and you add a new index with the same aliases. You’re going to start writing to the new index atomically. This new index would have more shards. That’s the only way to really increase throughput.
Another thing that’s frustrating when you’re trying to horizontally scale a cluster is that there’s shared resources. Each of our data nodes is getting hit with all these requests to pull data out and at the same time to put data in. If you have a really heavy query with a leading wildcard, RegEx, something like that, hitting one or two of your nodes, the write throughput is going to be impacted. You’re going to start lagging in your writes. Why is this important to note? Because autoscaling, often we look at the CPU and we say, CPU high at nodes. That could be because of one of these two pressures. It could be the write pressure or the read. If it’s the read, it could be momentary, and you just wasted money by adding a lot of nodes.
On the one hand, we shouldn’t look at the CPU, and we might want to look at the write load and the read load. On the other hand, write load and read load could be fine, but you have so many shards packed in each one of your nodes because you’ve been doing all these rollover tasks that you get out of memory. I’m just trying to give you the feeling of why it’s actually very hard to do this thing where you’re saying, this metric says scale up.
Horizontally Scaling OpenSearch
The good news is, it’s sometimes really simple. It does come at a cost, similarly to eating cake. It is still simple. If the load is imbalanced on one of those three different types, disk, memory, or CPU, we could add extra nodes, and it will balance out, especially if it’s disk. Similarly, if the load is low on all three, it can’t be just one, on all three of those, so low memory, low CPU, low disk, we can remove nodes. That’s when it is simple, when you can clearly see the picture is over-provisioned or under-provisioned. I want to devote the rest of the talk to when it’s actually complicated because the easy is really easy. Let’s assume that we’re looking at one of those spikes, the load goes up and down. Let’s say we want to say that when we see a lot of writes coming in, we want to roll over. When they go down, we want to roll over again because we don’t want to waste money. The red is going to say that the writes are too high. We’re going to roll over.
Then we add this extra node, and so everything is ok. Then the writes start to go down, we’re wasting money at this point. There’s 20% load on each of these nodes. If we remove a node, we get a hotspot because now we just created a situation where 40% is hitting one node, a disproportionate amount of pressure on one. That’s bad. What do we do? Do another rollover task, and now it’s 25% to each node. We could do this again and again on each of these. If it’s like a day-night load, you’d have way too many shards already in your cluster, and you’d start hitting out of memory. Getting rid of those extra shards is practically hard. You have to find a way to either do it slowly by changing to size-based retention, or you can do merging of indexes, which you can do, but it’s very slow. It takes a lot of CPU.
Cluster Topologies for Scaling
There is a rather simple way to overcome this problem, and that is to overshard. Rather than have shards spread out one per node, I could have three shards per node. Then, when I want to scale, I’ll add nodes and let those shards spread out. The shards are going to take up as much compute power as it can from those new nodes, so like hulking out. That’s the concept. However, finding the sweet spot between oversharding and undersharding becomes hard. It’s difficult to calculate. In many cases, you’d want to roll over again into an even bigger index. I’m going to suggest a few topologies for scaling in a way that allows us to really maintain this sweet spot between way too many, way too few shards. The first kind is what I’d call a burst index.
As I mentioned earlier, each index has a write alias. That’s where you’re going to atomically be writing. You can change this alias, and it’ll switch over to whatever index you point to. It’s an important concept to be familiar with if you’re managing a cluster. What we’re suggesting is to have these burst indices prepared in case you want to scale out. They can be maintained for weeks, and they will be that place where you direct traffic when you need to have a lot of it directed there. That’s what we would do. We just change the write alias to the write data stream. That would look something like this. There’s an arbitrary tag, a label we can give nodes called box_type. You could tell an index to allocate its shards on a specific box_type or a few different box_types. The concept is you have burst type, the box_type: burst, and you have box_type: low.
As long as you have low throughput in your writes, and again, that is probably the best indicator of I need more nodes, is the write throughput. If we have a low throughput on the writes, we don’t need our extra nodes. The low write throughput index is allocated to indexes that have the low box_type. If throughout the day the throughput is not so low and we anticipate that we’re going to have a spike, and this, again, it’s so tailored to your use case that I can’t tell you exactly what that is. If you see, in many cases, it is that the write throughput is growing on a trend, then what you do is you add these extra nodes. You don’t need to add nodes that are as expensive as the other ones. Why? Because you don’t intend to have that amount of disk space used on them. They’re temporary. You could have a real small and efficient disk there on these new box_types. You create the new ones. The allocation of our burst index says it can be on either low or burst or both. All you have to do is tell that index that you’re allowed to have total shards per node, 1.
Then it automatically will spread out to all of these nodes. At this point, you’re prepared for the higher throughput, and you switch the write alias to be the high throughput index. This is the burst index type. As it goes down, you can move the shards back by doing something called exclude of the nodes. You just exclude these nodes, and shards just fly off of it to other nodes. Then you remove them. This is the first form of autoscaling. It works when you don’t have many tenants on your cluster. If you have one big index, and it may grow or shrink, this makes sense.
However, in some cases, we have many tenants, and they’re doing many different things all at the same time. Some throughputs spike, when others will go down. You don’t want to be in a situation where you’re having your cluster tailored just for the highest throughput tenant. Because then, again, you are wasting resources.
Which brings me to the second and last topology that I want to discuss here, which is called the burst cluster. It is very similar to the previous one, but the difference is big. We’re not just changing the index that we’re going to within the cluster, we’re changing the direction to a completely different cluster. We wouldn’t be using the write alias, we would be diverting traffic. It would look something like this. If each of these circles is a cluster, and each of them have that many nodes, why would we have a 10, and a 5, and a 60? The reason is we’d want to avoid hotspots. You should fine-tune your clusters initially for your average load. The average load for a low throughput might be 5 nodes, so you want only 5 shards. For a higher throughput, you want a 10-node cluster, so you have 10 shards each. If you’re suffering from hotspots, all you have to do to fix that is spread the shards perfectly on the cluster. That means zero hotspots.
In this situation, we’ve tailored our system so that on these green clusters, the smaller circles, they’re fine-tuned for the exact amount of writes that we’re getting. Then one of our tenants spikes while the others don’t. We move only that tenant to send all their data, we divert it to the 60-node cluster, capable of handling very high throughputs, but not capable of handling a lot of disk space. It’s not as expensive as six times these 10-node clusters. It is still more expensive. Data is being diverted to a totally different environment. We use something called cross-cluster search in order to search on both. From the perspective of the person running the search, nothing has changed at any point. It’s completely transparent for them.
In terms of the throughput, nothing has changed. They’re sending much more data, but they don’t get any lag, whoever is sending it. All the other tenants don’t feel it. There are many more tenants on this 10-node cluster, and they’re just living their best life over there. You could also have a few tenants sending to this 60-node cluster. You just have to manage how much disk you’re expecting to fill at that time of the burst. A way to make this a little more economical is to have one of your highest throughput tenants always on the 60-node cluster. You still maintain a reason to have them up when there’s no high throughput tenants on these other clusters. This is a way to think of autoscaling in a way that is a bit outside of the box and not just adding nodes to a single cluster. It is very useful, if you are running a feature that is not very used in OpenSearch, but is up and coming, called searchable snapshots.
If you’re running searchable snapshots, all your data is going to be on S3, and you’re only going to have metadata on your cluster. The more nodes you have that are searching S3, the better. They can be small nodes with very small disk, and they could be searching many terabytes on S3. If you have one node with a lot of disk trying to do that, the throughput is going to be too low and your search is going to be too slow. If you want to utilize these kinds of features where your data is remote, you have to have many nodes. That’s another reason to have such a cluster just up and running all the time. You could use it to search audit data that spans back many years. Of course, we don’t want to keep it there forever.
A way to do that is just snapshot it to S3. Snapshots in OpenSearch are a really powerful tool. They’re not the same as they are in other databases. It takes the index as it is. It doesn’t do any additional compression, but it stores it in a very special way, so it’s easy to extract it and restore a cluster in case of a disaster. We would move the data to S3 and then restore it back into these original clusters that we had previously been running our tenants on. Then we could do a merge task. Down the line, when the load is low, we could merge that data into smaller indexes if we like. Another thing that happens usually in these kinds of situations is that you have retention. Once the retention is gone, just delete the data, which is great. Especially if you’re in Europe, you have to delete it right on time. This is the burst cluster topology.
Summary
There are three different resources that we want to be scaling. You have to be mindful when you’re maintaining your cluster which one is the one that causes the pressure. If you have very long retention, then disk space. You have to start considering things like searchable snapshots or maintaining maybe a cross-cluster search where you have just data sitting on a separate cluster that’s just accumulating in very large disks, whereas your write load is on a smaller cluster. That’s one possibility. If it’s memory or CPU, then you would definitely have to add stronger machines. We have to think about these things ahead of time. Some of them are a one-way door.
If you’re using AWS and you add to your disk space, in some cases, you may find it difficult to reduce the disk space again. This is a common problem. When I say that, the main reason it is is because when you want to reduce a node, you have to shift the data to the other nodes. In certain cases, especially after you’ve added a lot of disk, that can take a lot of time. Some of them are a one-way door. Many of them require a restart of a node, which is potential downtime. We talked about these two topologies, I’ll remind you, the burst index and the burst cluster, which are very important to think about as completely different options. I like to highlight that that first option that I gave, the hulking out, like the oversharding proposition, is also viable for many use cases.
If you have a really easy trend that you can follow, your data is just going up and down, and it’s the same at noon. People are sending 2x. Midnight, it goes down to half of that. It keeps going up and down. By all means, have a cluster that has 10 nodes with 20 shards on it. When you hit that afternoon peak, just scale out and let it spread out. Then when it gets to the evening, then scale down again. If that’s your use case, you shouldn’t be implementing things that are this complex. You should definitely use the concept of oversharding, which is well-known.
Upcoming Key Features
I’d like to talk about some upcoming key features, which is different than when I started writing this presentation. These things changed. The OpenSearch Software Foundation, which supports OpenSearch, one of the things that’s really neat is that from it being very AWS-centric, it has become much more widespread. There’s a lot of people from Uber and Slack, Intel, Airbnb, developers starting to take an interest and developing things within the ecosystem. They’re changing it in ways that will benefit their business.
If that business is as big as Uber, then the changes are profound. One of the changes that really affects autoscaling is read/write separation. That’s going to come in the next few versions. I think it’s nearly beta, but a lot of the code is already there. I think this was in August when I took this screenshot, and it was a 5 out of 11 tasks. They’re pretty much there by now. This will allow you to have nodes that are tailored for write and nodes that are tailored for read. Then you’re scaling the write, and you’re scaling the read separately, which makes life so much more simple.
The other one, which is really cool, is streaming ingestion. One of the things that really makes it difficult to ingest a lot of data all at once is that today, in both Elasticsearch and OpenSearch, we’re pushing it in. The index is trying to do that, trying to push the data and ingest it. The node might be overloaded, in which case the shard will just say, I’m sorry, CPU up to here, and you get what is called a write queue. Once that write queue starts to build, someone’s going to be woken up, normally. If you’re running observability data, that’s a wake-up call. In pull-based, what you get is the shard is hardcoded to listen and retrieve documents from a particular partition in for example, Kafka. It would be pluggable, so it’s not only Kafka.
Since it’s very common, let’s use Kafka as an example. Each shard will read from a particular partition. A topic would represent a tenant. You could have a shard reading from different partitions from different topics, but per topic, it would be one, so shard 0 from partition 0. What this gives us is the capability for the shard to read as fast as it can, which means that you don’t get the situation of a write queue, because it’s reading just as fast as it possibly can, based on the machine, wherever you put it. If you want to scale, in this case, it’s easy. You look at the lag in Kafka. You don’t look at these metrics in terms of the cluster. The metrics here are much easier. Is there a lag in Kafka? Yes. I need to scale. Much easier. Let’s look at CPU. Let’s look at memory. Let’s see if the shards are balanced. It’s much harder to do. In this case, it will make life much easier.
Questions and Answers
Participant 1: I had a question about streaming ingestion. Beyond just looking at a metric, at the lag in Kafka, does that expose a way to know precisely up to which point in the stream this got in the document? We use OpenSearch in a bunch of places where we need to know exactly what’s available in the index so that we can propagate things to other systems.
Stern: It is an RFC, a request for comments.
Participant 1: There’s not a feature yet.
Stern: Right now, it’s in the phase of what we call a feature branch, where it’s being implemented in a way that it’s totally breakable. We’re not going to put that in production. If you have any comments like that, please do comment in the GitHub. That would be welcome. It’s in the exact phase where we need those comments.
Participant 2: This is time-series data. Do you also roll your indexes by time, like quarterly or monthly, or how do you combine this approach with burst indexes with a situation where you have indexes along the time axis.
Stern: If it’s retention-based? One of the things you can do is you have the burst index. You don’t want it to be there for too long. The burst index, you want it to live longer than the retention?
Participant 2: It’s not just the burst indexes, your normal indexes are separated by time.
Stern: In some cases, if your indexes are time-based and you’re rolling over every day, then you’re going to have a problem of too many shards if you don’t fill them up enough. You’ll have, basically, shards that have 2 megabytes inside them. It just inflates too much. If you have 365 days or two years of data, that becomes too many shards. I do recommend moving to size-based, like a hybrid solution of size-based, as long as it’s less than x amount of days, so that you’re not exactly on the date but better. Having said that, the idea is that you have your write alias pointed at the head. Then after a certain amount of time, you do a rollover task. The burst index, you don’t roll over, necessarily. That one, what you do instead of rolling over, you merge, or you do a re-index of that data into the other one. You can do that. It just takes a lot of time to do. You can do that in the background. There’s nitty-gritty here, but we didn’t go into that.
Participant 3: I think you mentioned separation of reading and writing. It’s already supported in OpenSearch Serverless in AWS. Am I missing something? The one that you are talking about, is it going to come for the regular OpenSearch, and it’s not yet implemented?
Stern: I don’t work at AWS. I’m representing open source. Both of these are going to be completely in open source.
Participant 3: That’s what I’m saying. It seems like it’s already there in version, maybe 2.13, 2.14, something like that. You mentioned it is a version that is coming, but I have practically observed that it’s already there, in Amazon serverless.
Stern: Amazon serverless is a fork of OpenSearch. It took a nice amount of engineers more than a year to separate these two things, these concepts of OpenSearch is a multi-application and having to read/write. A lot of these improvements, they’re working upstream. They like to add these special capabilities, like read/write separation. Then they contribute a lot of the stuff back into the open source. In some cases, you’ll have features already available in the Amazon OpenSearch offering, then later, it’ll get introduced into the OpenSearch open source.
Participant 3: The strategies that you explained just now, and they are coming, especially the second one, one with the Kafka thing, is there a plan?
Stern: Again, this is very early stage, the pull-based indexing. That one is at a stage where we presented the API that we imagine would be useful for this. We developed the concept of it’ll be pluggable, like which streaming service you’d use. It’s at a request for comments stage. I presented it because I am happy to present these things and ask for comments. If you have anything that’s relevant, just go on GitHub and say, we’re using it for this, and one-to-one doesn’t make sense to us. If that’s the case, then yes.
Participant 3: It can take about six months to a year?
Stern: That particular one, we’re trying to get in under a year. I don’t think it’s possible in six months. That’s a stretch.
Participant 4: I think this question pertains to both the burst index and the burst cluster solution. I think I understand how this helps for writing new documents. If you have an update or a delete operation, where you’re searching across your old index, or your normal index, and then either the burst index or the burst cluster, and that update or that delete is reflected in the burst cluster, how does that get rectified between those two?
Stern: One of the things you have to do if you’re maintaining these types of indexes, like a burst index, is you would want to have a prefix that signifies that tenant, so that any action you do, like a deletion, you’d say, delete based on these aliases. You have the capability of specifying either the prefix with a star in the end, like a wildcard. You could also give indexes, and it’s very common to do this, especially if it’s time-series data, to give a read alias per day. You have an index, and it contains different dates with the tenant ID connected to them. When you perform a search, that tenant ID plus November 18th, then that index is then made available for search. You can do the same thing when you’re doing operations like get for a delete. You can say, these aliases, I want to delete them, or I want to delete documents from them. It can go either to the burst cluster, or it could go to the indexes that have completely different names, as long as the alias points to the correct one.
The cluster means you have to really manage it. You have to have some place where you’re saying, this tenant has data here and there, and the date that I changed the tenant to be over there, and the date that I changed them back. It’s very important to keep track of those things. I wouldn’t do it within OpenSearch. A common mistake when you’re managing OpenSearch, is to say, I have OpenSearch, so I’m going to just store lots of information in it, not just the data that I built the cluster for. It should be a cluster for a thing and not for other things. Audit data should be separated from your observability data. You don’t want to put them in the same place.
Participant 5: A question regarding the burst clusters, as well as the burst nodes that you have. With clusters, how do you redirect the read load directly? Is the assumption that we do cross-cluster search? With OpenSearch dashboards in particular, when you have all your alerts and all that, and with observability data, you’re acquiring a particular set of indexes, so when you move the data around clusters, how do you manage the search?
Stern: For alerting, it is very difficult to do this if you’re managing alerting using just the index. If you use a prefix, it could work. If you’re doing cross-cluster search, the way that that feature works is that, in the cluster settings, you provide the clusters that it can also search on. Then when you run a search, if you’re doing it through Amazon service, it should be seamless. If you’re running it on your own, you do have to specify, instead of just search this index, it doesn’t know that it has to go to the other cluster. You have to say, within this cluster, and that cluster, and the other cluster, search for this index. You have to add these extra indexes to your search.
Participant 5: There is a colon mechanism where you put in. Basically, what you’re expecting here is, in addition to write, with read, we have to keep that in mind before spinning up a burst cluster.
Stern: You have to keep track where your data is when you’re moving it.
Participant 5: The second part of the question with burst nodes is, I’m assuming you’re amortizing the cost of rebalancing. Because whenever the node goes up and down, so your cluster capacity, or the CPU, because shards are moving around, and that requires CPU, network storage, these transport actions are happening. You’re assuming, as part of your capacity planning, you have to amortize that cost as well.
Stern: Yes. Moving a shard while it’s being written to, and it has already 100 gigs on it, moving that shard is a task that is just going to take time. You need high throughput now. It’s amortized, but it’s very common to do a rollover task with more shards when your throughput is big. It’s the same. You’d anyway be doing this. You’d anyway be rolling over to an index that has more shards and more capability of writing on more nodes. It’s sort of amortized.
Participant 5: With the rollover, you’re not moving the data, though. It’s new shards getting created.
Stern: Yes. We don’t want to move data when we’re doing the spread-out. That really slows things down.
See more presentations with transcripts
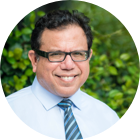
MMS • Steef-Jan Wiggers
Article originally posted on InfoQ. Visit InfoQ
In collaboration with NVIDIA, Microsoft has announced the integration of NVIDIA NIM microservices and the NVIDIA AgentIQ toolkit into Azure AI Foundry. This strategic move aims to significantly accelerate the development, deployment, and optimization of enterprise-grade AI agent applications, promising streamlined workflows, enhanced performance, and reduced infrastructure costs for developers.
The integration directly addresses the often lengthy enterprise AI project lifecycles, extending from nine to twelve months. By providing a more efficient and integrated development pipeline within Azure AI Foundry, leveraging NVIDIA’s accelerated computing and AI software, the goal is to enable faster time-to-market without compromising the sophistication or performance of AI solutions.
NVIDIA NIM (NVIDIA Inference Microservices), a key component of the NVIDIA AI Enterprise software suite, offers a collection of containerized microservices engineered for high-performance AI inferencing. Built upon robust technologies such as NVIDIA Triton Inference Server, TensorRT, TensorRT-LLM, and PyTorch, NIM microservices provide developers with zero-configuration deployment, seamless integration with the Azure ecosystem (including Azure AI Agent Service and Semantic Kernel), enterprise-grade reliability backed by NVIDIA AI Enterprise support, and the ability to tap into Azure’s NVIDIA-accelerated infrastructure for demanding workloads. Developers can readily deploy optimized models, including Llama-3-70B-NIM, directly from the Azure AI Foundry model catalog with just a few clicks, simplifying the initial setup and deployment phase.
Once NVIDIA NIM microservices are deployed, NVIDIA AgentIQ, an open-source toolkit, takes center stage in optimizing AI agent performance. AgentIQ is designed to seamlessly connect, profile, and fine-tune teams of AI agents, enabling systems to operate at peak efficiency.
Daron Yondem tweeted on X:
NVIDIA’s AgentIQ treats agents, tools, and workflows as simple function calls, aiming for true composability: build once, reuse everywhere.
The toolkit leverages real-time telemetry to analyze AI agent placement, dynamically adjusting resources to reduce latency and compute overhead. Furthermore, AgentIQ continuously collects and analyzes metadata—such as predicted output tokens per call, estimated time to following inference, and expected token lengths—to dynamically enhance agent performance and responsiveness. The direct integration with Azure AI Foundry Agent Service and Semantic Kernel further empowers developers to build agents with enhanced semantic reasoning and task execution capabilities, leading to more accurate and efficient agentic workflows.
(Source: Dev Blog post)
Drew McCombs, vice president of cloud and analytics at Epic, highlighted the practical benefits of this integration in an AI and Machine Learning blog post, stating:
The launch of NVIDIA NIM microservices in Azure AI Foundry offers Epic a secure and efficient way to deploy open-source generative AI models that improve patient care.
In addition, Guy Fighel, a VP and GM at New Relic, posted on LinkedIn:
NVIDIA #AgentIQ will likely become a leading strategy for enterprises adopting agentic development. Its ease of use, open-source nature, and optimization for NVIDIA hardware provide a competitive advantage by reducing development complexity, optimizing performance on NVIDIA GPUs, and integrating with cloud platforms like Microsoft Azure AI Foundry for scalability.
Microsoft has also announced the upcoming integration of NVIDIA Llama Nemotron Reason, a powerful AI model family designed for advanced reasoning in coding, complex math, and scientific problem-solving. Nemotron’s ability to understand user intent and seamlessly call tools promises to enhance further the capabilities of AI agents built on the Azure AI Foundry platform.
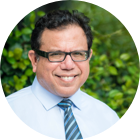
MMS • RSS
Posted on mongodb google news. Visit mongodb google news
MongoDB, Inc. (NASDAQ:MDB – Get Free Report) saw a significant increase in short interest in March. As of March 15th, there was short interest totalling 3,040,000 shares, an increase of 84.2% from the February 28th total of 1,650,000 shares. Currently, 4.2% of the company’s stock are sold short. Based on an average daily volume of 2,160,000 shares, the days-to-cover ratio is presently 1.4 days.
Analyst Upgrades and Downgrades
A number of equities research analysts recently commented on the company. Wedbush decreased their target price on MongoDB from $360.00 to $300.00 and set an “outperform” rating for the company in a report on Thursday, March 6th. UBS Group set a $350.00 price objective on shares of MongoDB in a report on Tuesday, March 4th. Robert W. Baird cut their price objective on shares of MongoDB from $390.00 to $300.00 and set an “outperform” rating on the stock in a research note on Thursday, March 6th. DA Davidson boosted their target price on shares of MongoDB from $340.00 to $405.00 and gave the company a “buy” rating in a research report on Tuesday, December 10th. Finally, Macquarie cut their price target on MongoDB from $300.00 to $215.00 and set a “neutral” rating on the stock in a research report on Friday, March 7th. Seven analysts have rated the stock with a hold rating and twenty-three have assigned a buy rating to the company. According to MarketBeat.com, the company has a consensus rating of “Moderate Buy” and an average target price of $320.70.
View Our Latest Analysis on MDB
MongoDB Trading Down 5.6 %
MDB stock opened at $178.03 on Monday. The company has a market capitalization of $14.45 billion, a P/E ratio of -64.97 and a beta of 1.30. The business has a 50 day simple moving average of $245.56 and a 200-day simple moving average of $265.95. MongoDB has a twelve month low of $173.13 and a twelve month high of $387.19.
MongoDB (NASDAQ:MDB – Get Free Report) last issued its quarterly earnings data on Wednesday, March 5th. The company reported $0.19 earnings per share (EPS) for the quarter, missing the consensus estimate of $0.64 by ($0.45). The business had revenue of $548.40 million for the quarter, compared to analysts’ expectations of $519.65 million. MongoDB had a negative return on equity of 12.22% and a negative net margin of 10.46%. During the same period in the previous year, the business posted $0.86 earnings per share. Research analysts predict that MongoDB will post -1.78 EPS for the current fiscal year.
Insider Activity at MongoDB
In other news, insider Cedric Pech sold 287 shares of MongoDB stock in a transaction on Thursday, January 2nd. The shares were sold at an average price of $234.09, for a total transaction of $67,183.83. Following the completion of the sale, the insider now directly owns 24,390 shares in the company, valued at approximately $5,709,455.10. The trade was a 1.16 % decrease in their ownership of the stock. The transaction was disclosed in a filing with the SEC, which is accessible through the SEC website. Also, CAO Thomas Bull sold 169 shares of the company’s stock in a transaction on Thursday, January 2nd. The shares were sold at an average price of $234.09, for a total value of $39,561.21. Following the completion of the transaction, the chief accounting officer now directly owns 14,899 shares in the company, valued at approximately $3,487,706.91. The trade was a 1.12 % decrease in their ownership of the stock. The disclosure for this sale can be found here. Insiders have sold a total of 43,139 shares of company stock valued at $11,328,869 over the last quarter. 3.60% of the stock is owned by corporate insiders.
Hedge Funds Weigh In On MongoDB
Hedge funds and other institutional investors have recently bought and sold shares of the company. B.O.S.S. Retirement Advisors LLC purchased a new stake in shares of MongoDB in the 4th quarter worth about $606,000. Geode Capital Management LLC lifted its holdings in shares of MongoDB by 2.9% in the third quarter. Geode Capital Management LLC now owns 1,230,036 shares of the company’s stock valued at $331,776,000 after purchasing an additional 34,814 shares in the last quarter. Union Bancaire Privee UBP SA acquired a new stake in shares of MongoDB in the fourth quarter valued at approximately $3,515,000. Nisa Investment Advisors LLC increased its stake in shares of MongoDB by 428.0% during the 4th quarter. Nisa Investment Advisors LLC now owns 5,755 shares of the company’s stock worth $1,340,000 after purchasing an additional 4,665 shares in the last quarter. Finally, HighTower Advisors LLC raised its position in shares of MongoDB by 2.0% during the 4th quarter. HighTower Advisors LLC now owns 18,773 shares of the company’s stock worth $4,371,000 after purchasing an additional 372 shares during the last quarter. 89.29% of the stock is owned by institutional investors and hedge funds.
MongoDB Company Profile
MongoDB, Inc, together with its subsidiaries, provides general purpose database platform worldwide. The company provides MongoDB Atlas, a hosted multi-cloud database-as-a-service solution; MongoDB Enterprise Advanced, a commercial database server for enterprise customers to run in the cloud, on-premises, or in a hybrid environment; and Community Server, a free-to-download version of its database, which includes the functionality that developers need to get started with MongoDB.
Featured Stories
Receive News & Ratings for MongoDB Daily – Enter your email address below to receive a concise daily summary of the latest news and analysts’ ratings for MongoDB and related companies with MarketBeat.com’s FREE daily email newsletter.
Article originally posted on mongodb google news. Visit mongodb google news