Category: Uncategorized
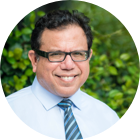
MMS • RSS
Posted on mongodb google news. Visit mongodb google news
MongoDB, Inc. (NASDAQ:MDB – Get Free Report) has been assigned a consensus recommendation of “Moderate Buy” from the thirty-three research firms that are currently covering the stock, MarketBeat.com reports. Eight analysts have rated the stock with a hold recommendation, twenty-four have issued a buy recommendation and one has issued a strong buy recommendation on the company. The average 1 year price target among analysts that have covered the stock in the last year is $282.47.
A number of research firms recently commented on MDB. Oppenheimer decreased their price objective on shares of MongoDB from $400.00 to $330.00 and set an “outperform” rating for the company in a research note on Thursday, March 6th. Cantor Fitzgerald raised their target price on MongoDB from $252.00 to $271.00 and gave the stock an “overweight” rating in a research report on Thursday, June 5th. Loop Capital lowered MongoDB from a “buy” rating to a “hold” rating and decreased their price objective for the stock from $350.00 to $190.00 in a research report on Tuesday, May 20th. Barclays raised their price objective on MongoDB from $252.00 to $270.00 and gave the stock an “overweight” rating in a research report on Thursday, June 5th. Finally, Royal Bank Of Canada restated an “outperform” rating and set a $320.00 price objective on shares of MongoDB in a research report on Thursday, June 5th.
View Our Latest Research Report on MDB
Insider Activity at MongoDB
In other news, CAO Thomas Bull sold 301 shares of the firm’s stock in a transaction dated Wednesday, April 2nd. The shares were sold at an average price of $173.25, for a total transaction of $52,148.25. Following the completion of the transaction, the chief accounting officer now directly owns 14,598 shares of the company’s stock, valued at approximately $2,529,103.50. This trade represents a 2.02% decrease in their position. The transaction was disclosed in a legal filing with the Securities & Exchange Commission, which is accessible through the SEC website. Also, Director Dwight A. Merriman sold 2,000 shares of the firm’s stock in a transaction dated Thursday, June 5th. The stock was sold at an average price of $234.00, for a total transaction of $468,000.00. Following the transaction, the director now directly owns 1,107,006 shares of the company’s stock, valued at $259,039,404. The trade was a 0.18% decrease in their ownership of the stock. The disclosure for this sale can be found here. Over the last 90 days, insiders sold 49,208 shares of company stock worth $10,167,739. Company insiders own 3.10% of the company’s stock.
Hedge Funds Weigh In On MongoDB
Hedge funds and other institutional investors have recently modified their holdings of the stock. Vanguard Group Inc. lifted its holdings in MongoDB by 6.6% during the 1st quarter. Vanguard Group Inc. now owns 7,809,768 shares of the company’s stock valued at $1,369,833,000 after purchasing an additional 481,023 shares during the last quarter. Franklin Resources Inc. raised its stake in shares of MongoDB by 9.7% in the fourth quarter. Franklin Resources Inc. now owns 2,054,888 shares of the company’s stock valued at $478,398,000 after acquiring an additional 181,962 shares during the last quarter. UBS AM A Distinct Business Unit of UBS Asset Management Americas LLC raised its stake in shares of MongoDB by 11.3% in the first quarter. UBS AM A Distinct Business Unit of UBS Asset Management Americas LLC now owns 1,271,444 shares of the company’s stock valued at $223,011,000 after acquiring an additional 129,451 shares during the last quarter. Geode Capital Management LLC raised its stake in shares of MongoDB by 1.8% in the fourth quarter. Geode Capital Management LLC now owns 1,252,142 shares of the company’s stock valued at $290,987,000 after acquiring an additional 22,106 shares during the last quarter. Finally, Amundi raised its position in MongoDB by 53.0% in the 1st quarter. Amundi now owns 1,061,457 shares of the company’s stock valued at $173,378,000 after purchasing an additional 367,717 shares during the last quarter. Institutional investors own 89.29% of the company’s stock.
MongoDB Trading Down 0.7%
NASDAQ:MDB opened at $204.15 on Thursday. MongoDB has a twelve month low of $140.78 and a twelve month high of $370.00. The company has a 50-day moving average of $184.32 and a 200-day moving average of $223.66. The firm has a market cap of $16.68 billion, a price-to-earnings ratio of -179.08 and a beta of 1.39.
MongoDB (NASDAQ:MDB – Get Free Report) last issued its earnings results on Wednesday, June 4th. The company reported $1.00 earnings per share for the quarter, topping analysts’ consensus estimates of $0.65 by $0.35. The firm had revenue of $549.01 million during the quarter, compared to analysts’ expectations of $527.49 million. MongoDB had a negative net margin of 4.09% and a negative return on equity of 3.16%. The firm’s revenue for the quarter was up 21.8% compared to the same quarter last year. During the same period last year, the firm posted $0.51 earnings per share. On average, research analysts forecast that MongoDB will post -1.78 earnings per share for the current year.
MongoDB Company Profile
MongoDB, Inc, together with its subsidiaries, provides general purpose database platform worldwide. The company provides MongoDB Atlas, a hosted multi-cloud database-as-a-service solution; MongoDB Enterprise Advanced, a commercial database server for enterprise customers to run in the cloud, on-premises, or in a hybrid environment; and Community Server, a free-to-download version of its database, which includes the functionality that developers need to get started with MongoDB.
Featured Articles
This instant news alert was generated by narrative science technology and financial data from MarketBeat in order to provide readers with the fastest and most accurate reporting. This story was reviewed by MarketBeat’s editorial team prior to publication. Please send any questions or comments about this story to contact@marketbeat.com.
Before you consider MongoDB, you’ll want to hear this.
MarketBeat keeps track of Wall Street’s top-rated and best performing research analysts and the stocks they recommend to their clients on a daily basis. MarketBeat has identified the five stocks that top analysts are quietly whispering to their clients to buy now before the broader market catches on… and MongoDB wasn’t on the list.
While MongoDB currently has a Moderate Buy rating among analysts, top-rated analysts believe these five stocks are better buys.
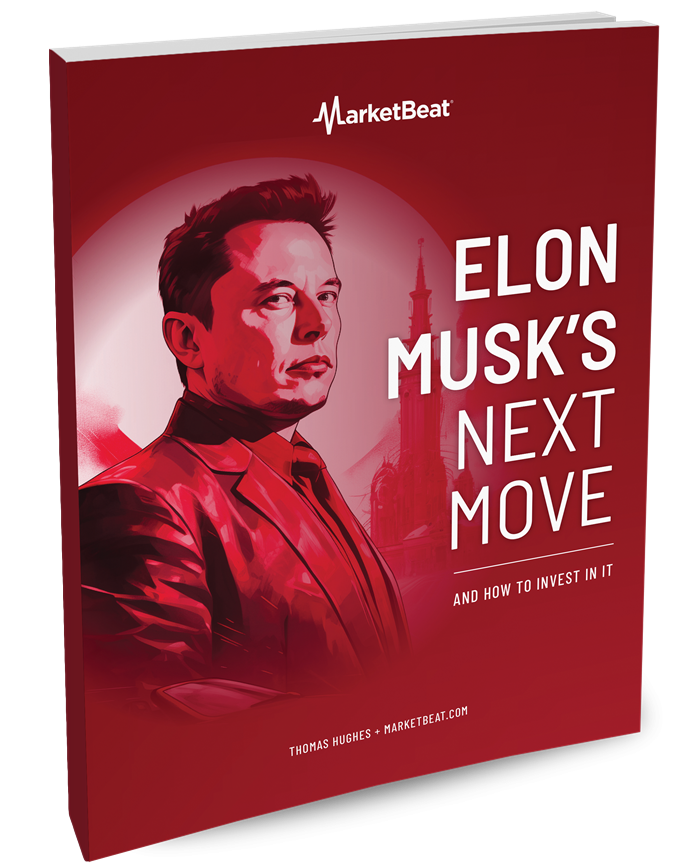
Explore Elon Musk’s boldest ventures yet—from AI and autonomy to space colonization—and find out how investors can ride the next wave of innovation.
Article originally posted on mongodb google news. Visit mongodb google news
Cohesity & MongoDB expand integration for enterprise data resilience – IT Brief Australia
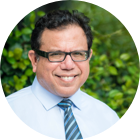
MMS • RSS
Posted on mongodb google news. Visit mongodb google news
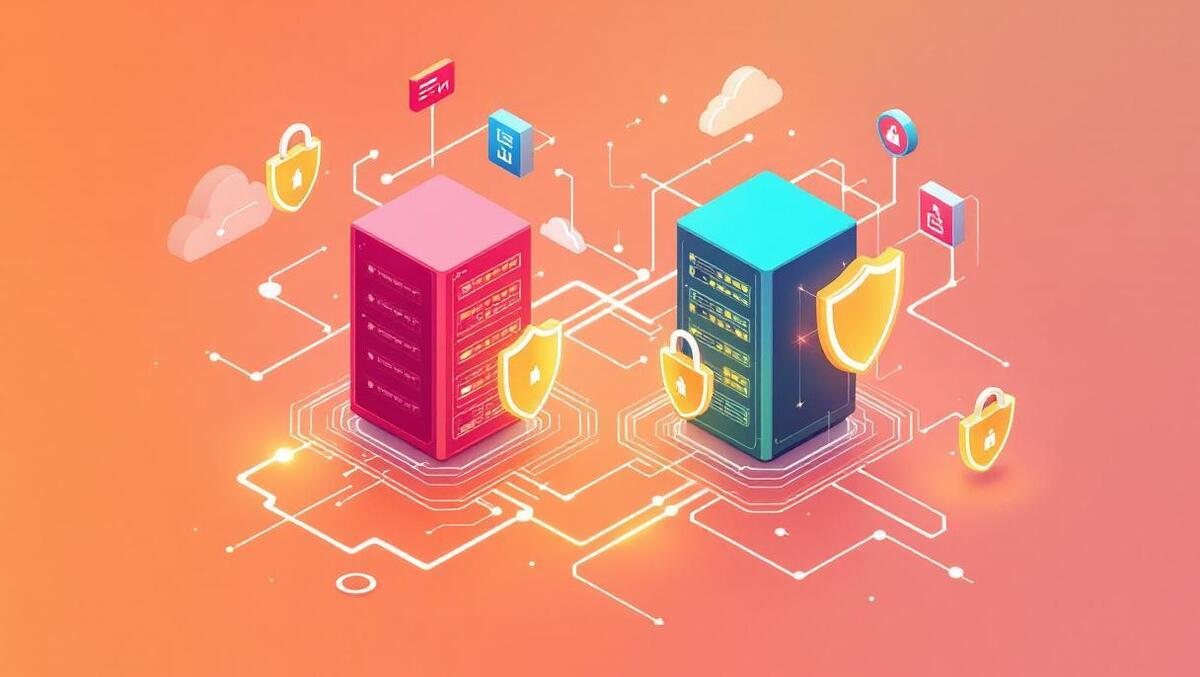
Cohesity has announced an expanded integration with MongoDB to deliver enhanced data protection and recovery performance for large enterprise workloads.
The deepened integration allows Cohesity customers to utilise the MongoDB Third Party Backup Ops Manager API, offering new controls and capabilities tailored to scenarios involving extensive datasets and stringent resilience requirements. This is particularly targeted at sectors such as financial services and the global banking industry, alongside Fortune 500 organisations, that demand minimal downtime and heightened security.
According to Cohesity, this functionality is now available for users of its DataProtect solution. By leveraging the new API-based integration, the company states that enterprises can better address data recovery speed and backup process efficiencies.
Cyber resilience focus
Vasu Murthy, Senior Vice President and Chief Product Officer at Cohesity, commented on the growing challenges faced by large organisations due to the increasing threat of ransomware attacks and the related business risks.
“With ransomware attacks now commonplace, cyber resilience is a strategic priority for all organisations. This is particularly true of large enterprises, which have a very low tolerance for risk,” said Vasu Murthy. “Downtime for any reason can mean millions of dollars and massive reputational damage. As trusted providers for many of the world’s largest companies, Cohesity and MongoDB are working together to strengthen our customers’ ability to bounce back fast.”
This expanded partnership between Cohesity and MongoDB aims to address the growing need for robust, enterprise-grade data protection. Paul Henaghan, Managing Director, Cohesity, Australia and New Zealand, highlighted the benefits for institutions across the region in the context of the evolving cyber threat environment.
“In today’s cyber threat landscape, organisations across Australia and New Zealand require data resilience that goes beyond basic backup, especially among large enterprises with complex technology environments and systems. Our expanded integration with MongoDB gives customers the enterprise-grade speed, scale and security needed to confidently protect and recover their most vital workloads. This is a powerful step forward in helping ANZ businesses reduce downtime risk and maintain trust in the face of escalating cyber threats,” said Paul Henaghan.
Industry research and capabilities
Cohesity’s own research indicates a gap between organisations’ perceived and actual cyber resilience. Only a small fraction—2%—of participants in a global survey reported being able to recover data and restore business processes within 24 hours following a cyberattack. The company suggests that the new MongoDB integration helps close this gap for customers seeking to meet rigorous recovery point and time objectives.
Some of the advanced features introduced through this integration include improved speed, as parallel data streams allow for the simultaneous processing of billions of objects. Cohesity states that intelligent backups can bring MongoDB databases online four times faster than traditional approaches. The system also boasts a scale-out architecture capable of accommodating petabyte-sized workloads on a single platform, introducing efficiencies such as global, variable-length deduplication and compression to reduce data footprint.
Additional security measures include support for immutable write once, read many (WORM) storage, end-to-end data encryption, continuous data protection, and secure SSL authentication—all underpinned by Zero Trust principles and a multi-layered defence model.
Collaborative efforts
Representatives from MongoDB and its partners echoed the significance of the collaboration. Benjamin Cefalo, Senior Vice President of Product Management at MongoDB, highlighted the broader mission of supporting performance and agility without sacrificing enterprise resilience.
“As the leading document database for modern applications, MongoDB empowers organisations to build, scale, and innovate faster,” said Benjamin Cefalo. “Our collaboration with Cohesity reinforces that mission by helping customers protect their data with robust, enterprise-grade resilience—without compromising the agility and performance developers expect from our platform.”
Juan Orlandini, Chief Technology Officer for North America at Insight, commented on the immediate applicability of these improvements to large organisations operating with critical datasets.
“Cohesity continues to broaden and enhance its support for enterprise workloads, including those with critical customer datasets at petabyte scale. The deepened integration with MongoDB addresses the most pressing data security concerns for large enterprises and financial organisations by delivering the speed, scalability, and security they require.”
Market demand
The integration between Cohesity and MongoDB is geared towards organisations with large-scale, mission-critical database workloads that cannot afford significant interruptions. Cohesity cites ongoing demand among its global enterprise customer base, which includes over 85 of the Fortune 100 and nearly 70% of the Global 500, for enhanced backup and recovery processes.
Article originally posted on mongodb google news. Visit mongodb google news
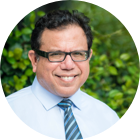
MMS • Bruno Couriol
Article originally posted on InfoQ. Visit InfoQ
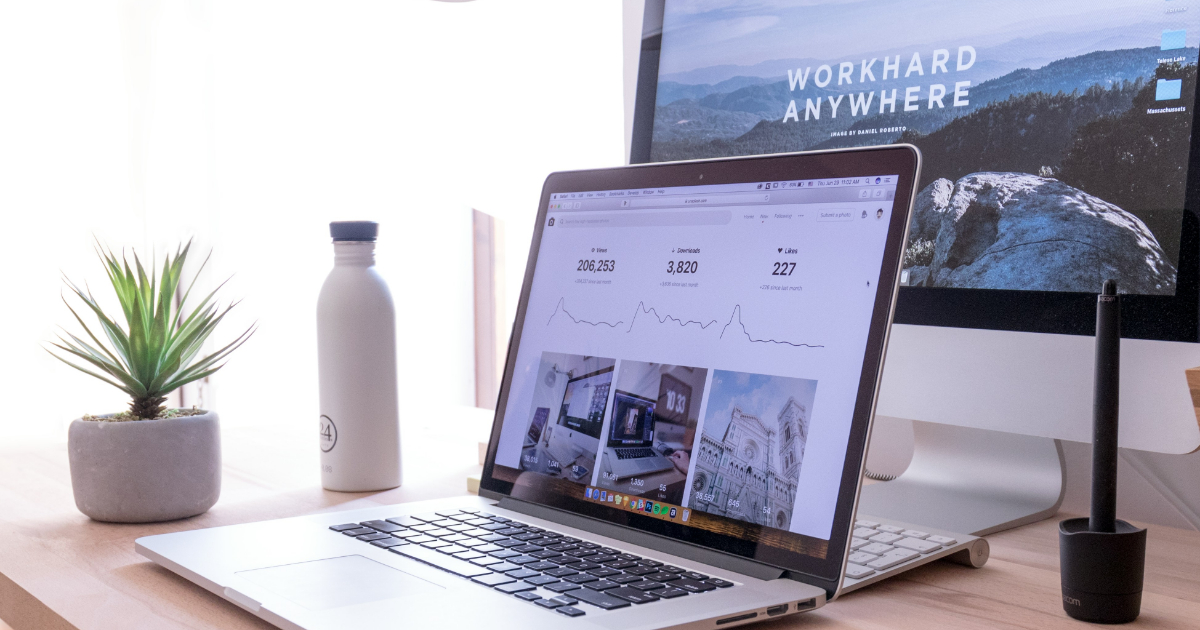
Deno Land recently released Deno 2.3, an update of the Deno runtime that adds support for local NPM packages. Deno 2.3 also brings improvements to deno compile
.
Deno 2.3 makes testing and developing an npm package locally possible. Deno thus provides mechanisms to override dependencies, enabling developers to use custom or local versions of libraries. The mechanism is similar to npm link
in Node.js, and is configured through the patch
field in deno.json
:
{
"patch": [
"../path/to/local_npm_package"
]
}
Developers can review the provided example.
deno compile
compiles a project into a single standalone binary, thus facilitating the executable distribution. This allows distributing Deno applications to systems that do not have Deno installed. Under the hood, it bundles a slimmed-down version of the Deno runtime along with the JavaScript or TypeScript code.
Deno 2.3 extends deno compile
to support programs that use Foreign Function Interface (FFI) and Node native add-ons. FFI provides a bridge between Deno’s JavaScript runtime and native code, allowing developers to use existing native libraries within Deno applications, implement performance-critical code in languages like Rust or C, or access operating system APIs and hardware features not directly available in JavaScript.
Developers can additionally now reduce the size of executables by excluding specific files from being embedded during the compilation process (e.g., exclude development or test files from production builds).
To upgrade to Deno 2.3, developers need simply run the following command in the terminal:
deno upgrade
Deno 2.3 includes additional features, including improvements to deno fmt
(allowing developers to format embedded CSS, HTML, and SQL in tagged templates), expanded OpenTelemetry support (basic event recording, span context propagators, and more), faster dependency installation, and more. For the full list of features, developers are invited to review the release note.
Deno is open-source software that is available under the MIT license. Contributions are encouraged via the Deno Project and should follow the Deno contribution guidelines.
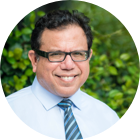
MMS • RSS
Posted on mongodb google news. Visit mongodb google news
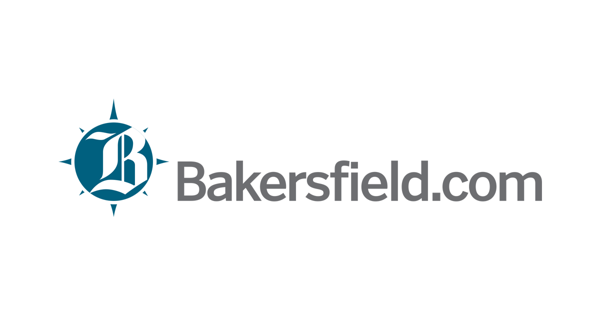
SANTA CLARA, Calif.–(BUSINESS WIRE)–Jun 18, 2025–
Cohesity, the leader in AI-powered data security, today announced a deeper integration with MongoDB, the leading database for modern applications. The new integration provides advanced performance and control capabilities for backup and recovery of MongoDB databases. Cohesity is among the first data protection software providers to deliver MongoDB workload protection through the MongoDB Third Party Backup Ops Manager API. Designed with large, critical datasets in mind, the integration supports the strict resilience requirements of global banking, financial services, and Fortune 500 enterprises. The integration is currently available for Cohesity DataProtect.
Article originally posted on mongodb google news. Visit mongodb google news
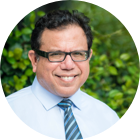
MMS • RSS
Posted on nosqlgooglealerts. Visit nosqlgooglealerts
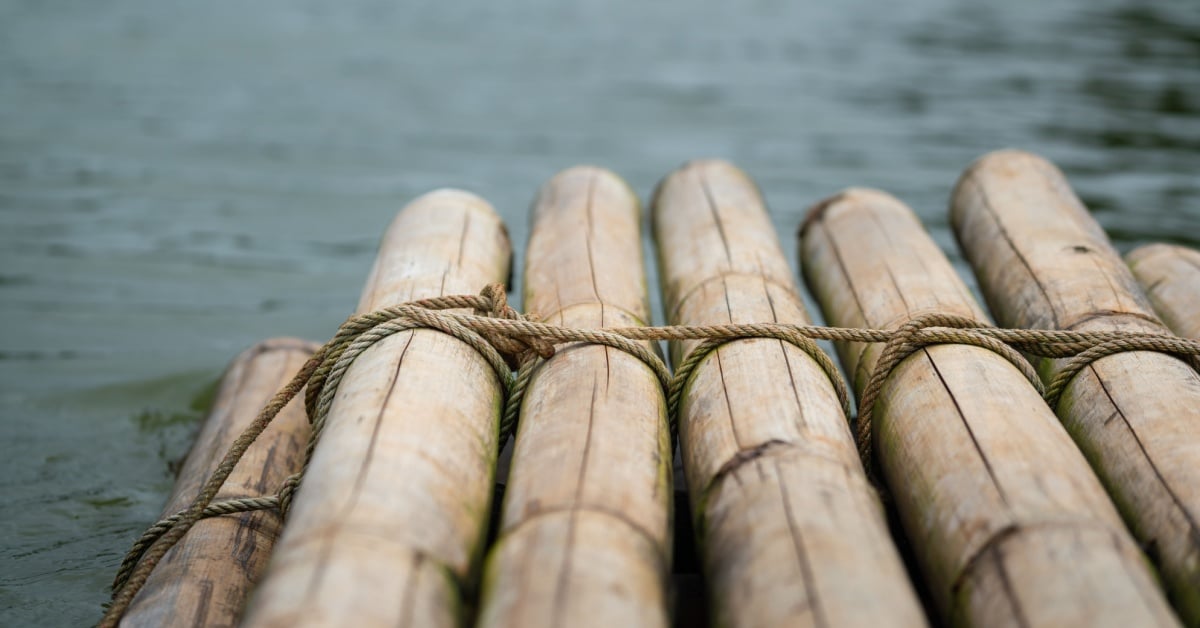
ScyllaDB, the wide-column database used by Comcast, Samsung, and banking giant Santander, has released a new database service it claims improves scalability and lowers cost.
The company also recently reviewed its licensing terms to move away from the GNU Affero General Public License (AGPL) – ScyllaDB 6.2 is the final version under that license – to a source-available license to address the “constant challenge for vendors whose core product is open source,” according to the company’s CEO.
ScyllaDB launched its first DBaaS in 2019 based on vnode data replication as used by Cassandra, another wide-column database similarly used for low-latency, globally distributed applications. The new service, dubbed ScyllaDB X, uses Raft – a consensus algorithm for distributed systems – to introduce a new tablets architecture.
“Tablets are designed to support flexible and dynamic data distribution across the cluster,” CTO Avi Kivity said in a blog post. “Based on Raft, this new approach provides new levels of elasticity with near-instant bootstrap and the ability to add new nodes in parallel – even doubling an entire cluster at once.”
“Since new nodes begin serving requests as soon as they join the cluster, users can spin up new nodes that start serving requests almost instantly. This means teams can quickly scale out in response to traffic spikes – satisfying latency SLAs without needing to overprovision ‘just in case.'”
ScyllaDB claims the new system will scale from 100,000 OPS to 2 million in minutes with steady single-digit millisecond latency while also reducing costs against comparable systems.
Announcing licensing changes in January, the company said its first source-available product would be ScyllaDB Enterprise 2025.1, released in April.
Dor Laor, co-founder and CEO, said at the time that while the team had contributed to dozens of open source projects including drivers, a Kubernetes operator, test harnesses, and various tools, it was difficult to maintain two code bases for its main product.
“For almost a decade, we have been maintaining two separate release streams: one for the open source database and one for the enterprise product. Balancing the free vs paid offerings is a never-ending challenge that involves engineering, product, marketing, and constant sales discussions,” he said.
ScyllaDB claims that the unpredictable and sluggish performance of its rival, Apache Cassandra, is prompting users to migrate to its system. Whether that holds true, it will have to overcome the intransigence of Cassandra’s market penetration if it is to progress.
Like ScyllaDB, Cassandra is designed to support highly distributed systems where writes exceed reads, and so-called ACID compliance is not important. Netflix, for example, has been using Cassandra since 2013, replacing Oracle databases and using the NoSQL system to support global accounts and customer data worldwide. Apple is another flagship Cassandra user.
In February, IBM bought DataStax, the AI and data biz that supports and contributes to Cassandra, which remains an Apache project.
At the time, Big Blue said it intended DataStax to continue to work with the open source Apache Cassandra, Langflow, Apache Pulsar, and OpenSearch communities in which DataStax participates.
IBM planned for the DataStax Cassandra database service, AstraDB, to improve the existing vector capabilities of IBM watsonx.data, IBM’s data lake for AI and analytics. It also sees Langflow, the open source visual framework for building AI applications, adding middleware capabilities to IBM watsonx.ai, as its AI development studio. ®
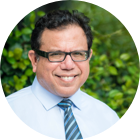
MMS • RSS
Posted on mongodb google news. Visit mongodb google news
Chicago Capital LLC acquired a new position in shares of MongoDB, Inc. (NASDAQ:MDB – Free Report) during the first quarter, according to the company in its most recent Form 13F filing with the Securities and Exchange Commission. The institutional investor acquired 1,801 shares of the company’s stock, valued at approximately $316,000.
Other large investors also recently made changes to their positions in the company. OneDigital Investment Advisors LLC boosted its holdings in MongoDB by 3.9% in the fourth quarter. OneDigital Investment Advisors LLC now owns 1,044 shares of the company’s stock worth $243,000 after acquiring an additional 39 shares in the last quarter. Aigen Investment Management LP raised its position in shares of MongoDB by 1.4% during the fourth quarter. Aigen Investment Management LP now owns 3,921 shares of the company’s stock worth $913,000 after purchasing an additional 55 shares during the period. Handelsbanken Fonder AB raised its position in shares of MongoDB by 0.4% during the first quarter. Handelsbanken Fonder AB now owns 14,816 shares of the company’s stock worth $2,599,000 after purchasing an additional 65 shares during the period. O Shaughnessy Asset Management LLC lifted its holdings in shares of MongoDB by 4.8% in the 4th quarter. O Shaughnessy Asset Management LLC now owns 1,647 shares of the company’s stock worth $383,000 after purchasing an additional 75 shares in the last quarter. Finally, Fifth Third Bancorp grew its position in MongoDB by 15.9% in the 1st quarter. Fifth Third Bancorp now owns 569 shares of the company’s stock valued at $100,000 after purchasing an additional 78 shares during the period. 89.29% of the stock is currently owned by institutional investors and hedge funds.
Analyst Upgrades and Downgrades
Several research analysts have weighed in on the stock. Stifel Nicolaus lowered their price objective on shares of MongoDB from $340.00 to $275.00 and set a “buy” rating on the stock in a report on Friday, April 11th. Monness Crespi & Hardt upgraded shares of MongoDB from a “neutral” rating to a “buy” rating and set a $295.00 target price on the stock in a report on Thursday, June 5th. Wells Fargo & Company cut shares of MongoDB from an “overweight” rating to an “equal weight” rating and dropped their price target for the company from $365.00 to $225.00 in a research report on Thursday, March 6th. Wedbush reissued an “outperform” rating and set a $300.00 price objective on shares of MongoDB in a report on Thursday, June 5th. Finally, Daiwa America upgraded MongoDB to a “strong-buy” rating in a report on Tuesday, April 1st. Eight analysts have rated the stock with a hold rating, twenty-four have given a buy rating and one has issued a strong buy rating to the company’s stock. According to MarketBeat, the stock has an average rating of “Moderate Buy” and an average target price of $282.47.
Read Our Latest Stock Analysis on MDB
Insider Activity at MongoDB
In related news, Director Hope F. Cochran sold 1,175 shares of the company’s stock in a transaction that occurred on Tuesday, April 1st. The shares were sold at an average price of $174.69, for a total transaction of $205,260.75. Following the sale, the director now owns 19,333 shares of the company’s stock, valued at approximately $3,377,281.77. This represents a 5.73% decrease in their position. The transaction was disclosed in a legal filing with the SEC, which is accessible through this hyperlink. Also, insider Cedric Pech sold 1,690 shares of the stock in a transaction that occurred on Wednesday, April 2nd. The stock was sold at an average price of $173.26, for a total transaction of $292,809.40. Following the completion of the sale, the insider now owns 57,634 shares in the company, valued at $9,985,666.84. This represents a 2.85% decrease in their ownership of the stock. The disclosure for this sale can be found here. In the last ninety days, insiders sold 49,208 shares of company stock worth $10,167,739. Corporate insiders own 3.10% of the company’s stock.
MongoDB Price Performance
Shares of MDB opened at $205.60 on Wednesday. MongoDB, Inc. has a 1 year low of $140.78 and a 1 year high of $370.00. The stock’s fifty day moving average price is $183.66 and its two-hundred day moving average price is $223.80. The firm has a market cap of $16.80 billion, a PE ratio of -180.35 and a beta of 1.39.
MongoDB (NASDAQ:MDB – Get Free Report) last released its quarterly earnings data on Wednesday, June 4th. The company reported $1.00 EPS for the quarter, beating the consensus estimate of $0.65 by $0.35. The business had revenue of $549.01 million for the quarter, compared to the consensus estimate of $527.49 million. MongoDB had a negative net margin of 4.09% and a negative return on equity of 3.16%. The company’s revenue was up 21.8% compared to the same quarter last year. During the same period in the prior year, the firm earned $0.51 earnings per share. Sell-side analysts anticipate that MongoDB, Inc. will post -1.78 earnings per share for the current fiscal year.
MongoDB Company Profile
MongoDB, Inc, together with its subsidiaries, provides general purpose database platform worldwide. The company provides MongoDB Atlas, a hosted multi-cloud database-as-a-service solution; MongoDB Enterprise Advanced, a commercial database server for enterprise customers to run in the cloud, on-premises, or in a hybrid environment; and Community Server, a free-to-download version of its database, which includes the functionality that developers need to get started with MongoDB.
Further Reading
This instant news alert was generated by narrative science technology and financial data from MarketBeat in order to provide readers with the fastest and most accurate reporting. This story was reviewed by MarketBeat’s editorial team prior to publication. Please send any questions or comments about this story to contact@marketbeat.com.
Before you consider MongoDB, you’ll want to hear this.
MarketBeat keeps track of Wall Street’s top-rated and best performing research analysts and the stocks they recommend to their clients on a daily basis. MarketBeat has identified the five stocks that top analysts are quietly whispering to their clients to buy now before the broader market catches on… and MongoDB wasn’t on the list.
While MongoDB currently has a Moderate Buy rating among analysts, top-rated analysts believe these five stocks are better buys.
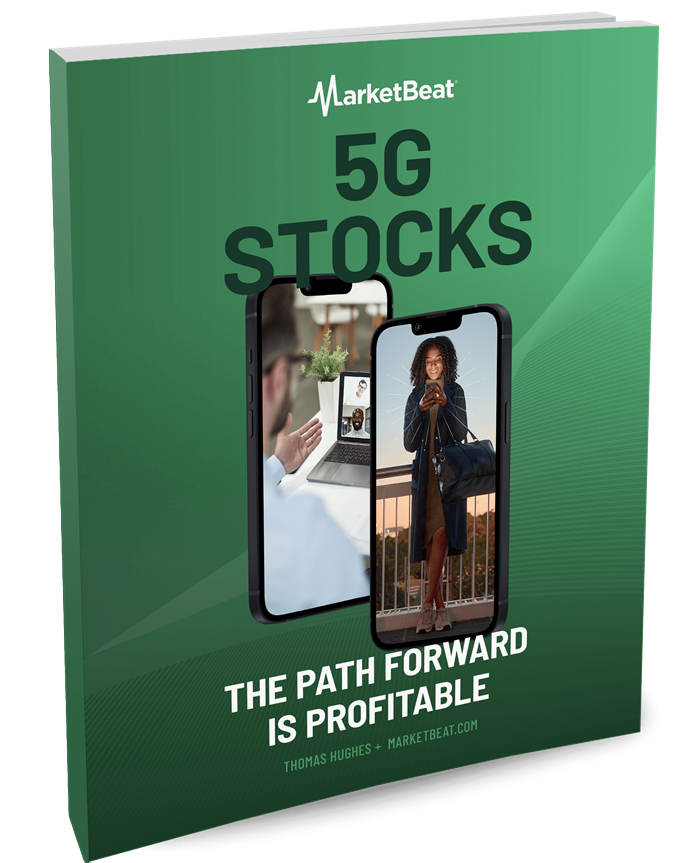
Enter your email address and we’ll send you MarketBeat’s guide to investing in 5G and which 5G stocks show the most promise.
Article originally posted on mongodb google news. Visit mongodb google news
Artificial Intelligence (AI) Software Sales Could Soar 580%: 2 AI Stocks to Buy Now (Hint
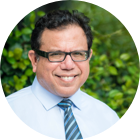
MMS • RSS
Posted on mongodb google news. Visit mongodb google news
Artificial intelligence (AI) is quickly weaving its way into daily life. According to Goldman Sachs, 9.2% of U.S. companies now use AI to produce goods and services, which is twice the percentage that used the technology at the same time last year. But the market is still in its early stages.
Morgan Stanley estimates AI software revenues will increase 580% in the next three years, topping $400 billion in 2028. While Palantir is well positioned to benefit, the stock carries a steep valuation. Investors should consider more reasonably priced stocks such as MongoDB(NASDAQ: MDB) and Okta(NASDAQ: OKTA).
Where to invest $1,000 right now? Our analyst team just revealed what they believe are the 10 best stocks to buy right now. Learn More »
Read on to learn more.
Image source: Getty Images.
1. MongoDB
Databases are used to store, manage, and retrieve application data. MongoDB’s document database handles structured and unstructured data, which differentiates it from traditional relational databases designed solely for structured data. To elaborate, structured data fits neatly into rows and columns, but unstructured data (e.g., images, videos, and text) does not.
The flexibility of the document model means MongoDB is especially well-suited for content management systems, e-commerce platforms, and artificial intelligence (AI) applications. MongoDB earlier this year leaned into the AI opportunity by acquiring Voyage AI, a company that develops embedding and reranking models that improve AI application accuracy.
CEO Dev Ittycheria told analysts, “As AI redefines how applications are built and how businesses operate, MongoDB is exceptionally well positioned. Real-world applications require high-quality, context-rich, and often unstructured data to deliver trustworthy outputs.”
MongoDB’s document model is also well-suited to real-time analytics applications, which are often used to personalize customer experiences across the internet. Consultancy Gartner recently ranked MongoDB as a leader in database management systems, mentioning its support for artificial intelligence and real-time analytics.
MongoDB shares currently trade at 49 times adjusted earnings. While that is not cheap, the valuation is reasonable for a company whose adjusted earnings increased 96% in the most recent quarter. Additionally, shares trade at 7.4 times sales, a material discount to the one-year average of 9.5 times sales and the three-year average of 13.4 times sales.
2. Okta
Okta is a cybersecurity company that develops identity and access management (IAM) software. Its platform lets administrators enforce contextual access policies that define which users and devices can connect to which applications and resources. It leans on AI to score risk with each login and authenticate accounts (including AI agents) based on criteria like identity, location, and behavior.
Importantly, Okta recently introduced a new product called Identity Threat Protection, an AI-powered tool that measures session risk. To elaborate, whereas login risk is calculated only one time during the authentication phase, session risk is determined continuously by analyzing every request post-authentication.
Okta has multiple tailwinds at its back. First, cybersecurity is a nondiscretionary budget expense because businesses cannot afford to suffer a data breach. Second, identity-based attacks account for 30% of cybersecurity incidents, and the identity market is projected to grow at 12.6% annually through 2030 as AI creates new threats. Third, industry analysts recently ranked Okta as a leader in workforce identity (for employees) and customer identity.
Okta shares currently trade at 33 times adjusted earnings. That is quite reasonable for a company whose adjusted earnings increased 32% in the most recent quarter. Additionally, shares currently trades at 6.6 times sales, roughly in line with the three-year average of 6.5 times sales. The stock fell after the recent earnings report because management provided cautious guidance. Patient investors should lean into that opportunity.
Should you invest $1,000 in MongoDB right now?
Before you buy stock in MongoDB, consider this:
The Motley Fool Stock Advisor analyst team just identified what they believe are the 10 best stocks for investors to buy now… and MongoDB wasn’t one of them. The 10 stocks that made the cut could produce monster returns in the coming years.
Consider whenNetflixmade this list on December 17, 2004… if you invested $1,000 at the time of our recommendation,you’d have $660,821!* Or when Nvidiamade this list on April 15, 2005… if you invested $1,000 at the time of our recommendation,you’d have $886,880!*
Now, it’s worth notingStock Advisor’s total average return is791% — a market-crushing outperformance compared to174%for the S&P 500. Don’t miss out on the latest top 10 list, available when you joinStock Advisor.
*Stock Advisor returns as of June 9, 2025
Trevor Jennewine has positions in Palantir Technologies. The Motley Fool has positions in and recommends Goldman Sachs Group, MongoDB, Okta, and Palantir Technologies. The Motley Fool recommends Gartner. The Motley Fool has a disclosure policy.
Article originally posted on mongodb google news. Visit mongodb google news
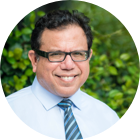
MMS • RSS
Posted on mongodb google news. Visit mongodb google news
Mn Services Vermogensbeheer B.V. trimmed its stake in shares of MongoDB, Inc. (NASDAQ:MDB – Free Report) by 7.1% during the first quarter, according to its most recent filing with the Securities and Exchange Commission. The institutional investor owned 26,100 shares of the company’s stock after selling 2,000 shares during the quarter. Mn Services Vermogensbeheer B.V.’s holdings in MongoDB were worth $4,578,000 as of its most recent filing with the Securities and Exchange Commission.
Other hedge funds and other institutional investors also recently made changes to their positions in the company. Vanguard Group Inc. raised its holdings in shares of MongoDB by 0.3% in the 4th quarter. Vanguard Group Inc. now owns 7,328,745 shares of the company’s stock worth $1,706,205,000 after purchasing an additional 23,942 shares in the last quarter. Franklin Resources Inc. raised its holdings in shares of MongoDB by 9.7% in the 4th quarter. Franklin Resources Inc. now owns 2,054,888 shares of the company’s stock worth $478,398,000 after purchasing an additional 181,962 shares in the last quarter. Geode Capital Management LLC raised its holdings in shares of MongoDB by 1.8% in the 4th quarter. Geode Capital Management LLC now owns 1,252,142 shares of the company’s stock worth $290,987,000 after purchasing an additional 22,106 shares in the last quarter. First Trust Advisors LP raised its holdings in shares of MongoDB by 12.6% in the 4th quarter. First Trust Advisors LP now owns 854,906 shares of the company’s stock worth $199,031,000 after purchasing an additional 95,893 shares in the last quarter. Finally, Norges Bank bought a new stake in shares of MongoDB in the 4th quarter worth approximately $189,584,000. 89.29% of the stock is currently owned by institutional investors.
Analysts Set New Price Targets
A number of analysts recently commented on MDB shares. Royal Bank Of Canada reiterated an “outperform” rating and issued a $320.00 target price on shares of MongoDB in a report on Thursday, June 5th. Wells Fargo & Company downgraded shares of MongoDB from an “overweight” rating to an “equal weight” rating and dropped their target price for the stock from $365.00 to $225.00 in a research report on Thursday, March 6th. Wedbush reissued an “outperform” rating and issued a $300.00 target price on shares of MongoDB in a research report on Thursday, June 5th. Redburn Atlantic raised shares of MongoDB from a “sell” rating to a “neutral” rating and set a $170.00 target price on the stock in a research report on Thursday, April 17th. Finally, The Goldman Sachs Group dropped their target price on shares of MongoDB from $390.00 to $335.00 and set a “buy” rating on the stock in a research report on Thursday, March 6th. Eight analysts have rated the stock with a hold rating, twenty-four have assigned a buy rating and one has assigned a strong buy rating to the company. According to MarketBeat, the company presently has an average rating of “Moderate Buy” and an average price target of $282.47.
Read Our Latest Analysis on MongoDB
Insider Transactions at MongoDB
In related news, CAO Thomas Bull sold 301 shares of the company’s stock in a transaction on Wednesday, April 2nd. The shares were sold at an average price of $173.25, for a total transaction of $52,148.25. Following the completion of the transaction, the chief accounting officer now directly owns 14,598 shares in the company, valued at $2,529,103.50. This represents a 2.02% decrease in their position. The transaction was disclosed in a document filed with the Securities & Exchange Commission, which is available through this link. Also, CEO Dev Ittycheria sold 25,005 shares of the company’s stock in a transaction on Thursday, June 5th. The shares were sold at an average price of $234.00, for a total transaction of $5,851,170.00. Following the transaction, the chief executive officer now owns 256,974 shares of the company’s stock, valued at approximately $60,131,916. The trade was a 8.87% decrease in their ownership of the stock. The disclosure for this sale can be found here. Over the last three months, insiders sold 49,208 shares of company stock worth $10,167,739. 3.10% of the stock is owned by insiders.
MongoDB Stock Up 1.5%
MDB stock opened at $205.60 on Wednesday. The firm has a 50 day moving average of $183.66 and a two-hundred day moving average of $223.80. MongoDB, Inc. has a 12 month low of $140.78 and a 12 month high of $370.00. The company has a market capitalization of $16.80 billion, a price-to-earnings ratio of -180.35 and a beta of 1.39.
MongoDB (NASDAQ:MDB – Get Free Report) last issued its quarterly earnings data on Wednesday, June 4th. The company reported $1.00 earnings per share for the quarter, topping analysts’ consensus estimates of $0.65 by $0.35. MongoDB had a negative net margin of 4.09% and a negative return on equity of 3.16%. The business had revenue of $549.01 million during the quarter, compared to analysts’ expectations of $527.49 million. During the same period in the previous year, the company earned $0.51 earnings per share. MongoDB’s revenue for the quarter was up 21.8% on a year-over-year basis. On average, research analysts expect that MongoDB, Inc. will post -1.78 EPS for the current fiscal year.
MongoDB Profile
MongoDB, Inc, together with its subsidiaries, provides general purpose database platform worldwide. The company provides MongoDB Atlas, a hosted multi-cloud database-as-a-service solution; MongoDB Enterprise Advanced, a commercial database server for enterprise customers to run in the cloud, on-premises, or in a hybrid environment; and Community Server, a free-to-download version of its database, which includes the functionality that developers need to get started with MongoDB.
See Also
This instant news alert was generated by narrative science technology and financial data from MarketBeat in order to provide readers with the fastest and most accurate reporting. This story was reviewed by MarketBeat’s editorial team prior to publication. Please send any questions or comments about this story to contact@marketbeat.com.
Before you consider MongoDB, you’ll want to hear this.
MarketBeat keeps track of Wall Street’s top-rated and best performing research analysts and the stocks they recommend to their clients on a daily basis. MarketBeat has identified the five stocks that top analysts are quietly whispering to their clients to buy now before the broader market catches on… and MongoDB wasn’t on the list.
While MongoDB currently has a Moderate Buy rating among analysts, top-rated analysts believe these five stocks are better buys.
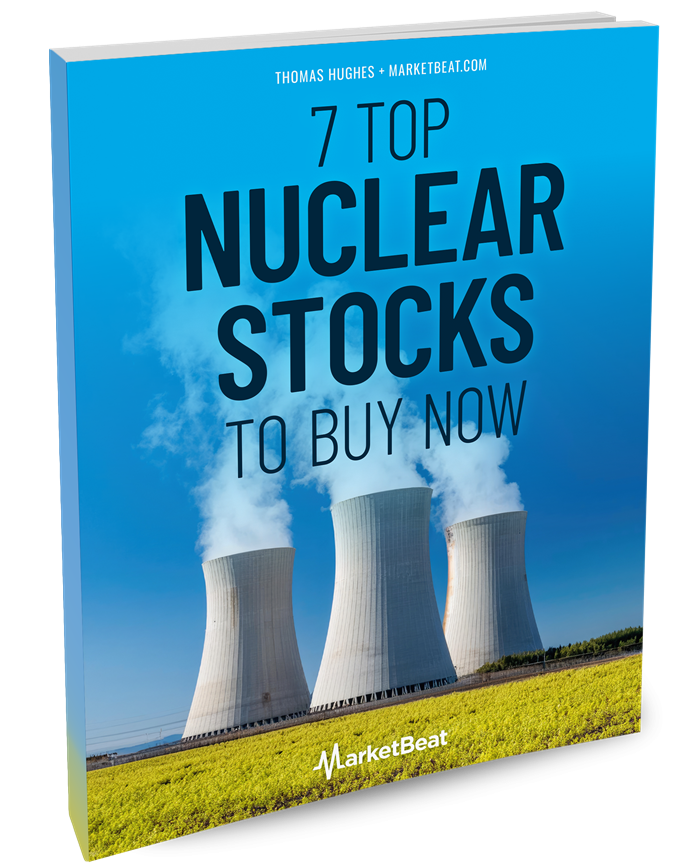
Nuclear energy stocks are roaring. It’s the hottest energy sector of the year. Cameco Corp, Paladin Energy, and BWX Technologies were all up more than 40% in 2024. The biggest market moves could still be ahead of us, and there are seven nuclear energy stocks that could rise much higher in the next several months. To unlock these tickers, enter your email address below.
Article originally posted on mongodb google news. Visit mongodb google news
Firms partner to accelerate West Africa’s digital transformation – The Guardian Nigeria News
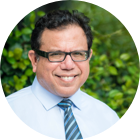
MMS • RSS
Posted on mongodb google news. Visit mongodb google news
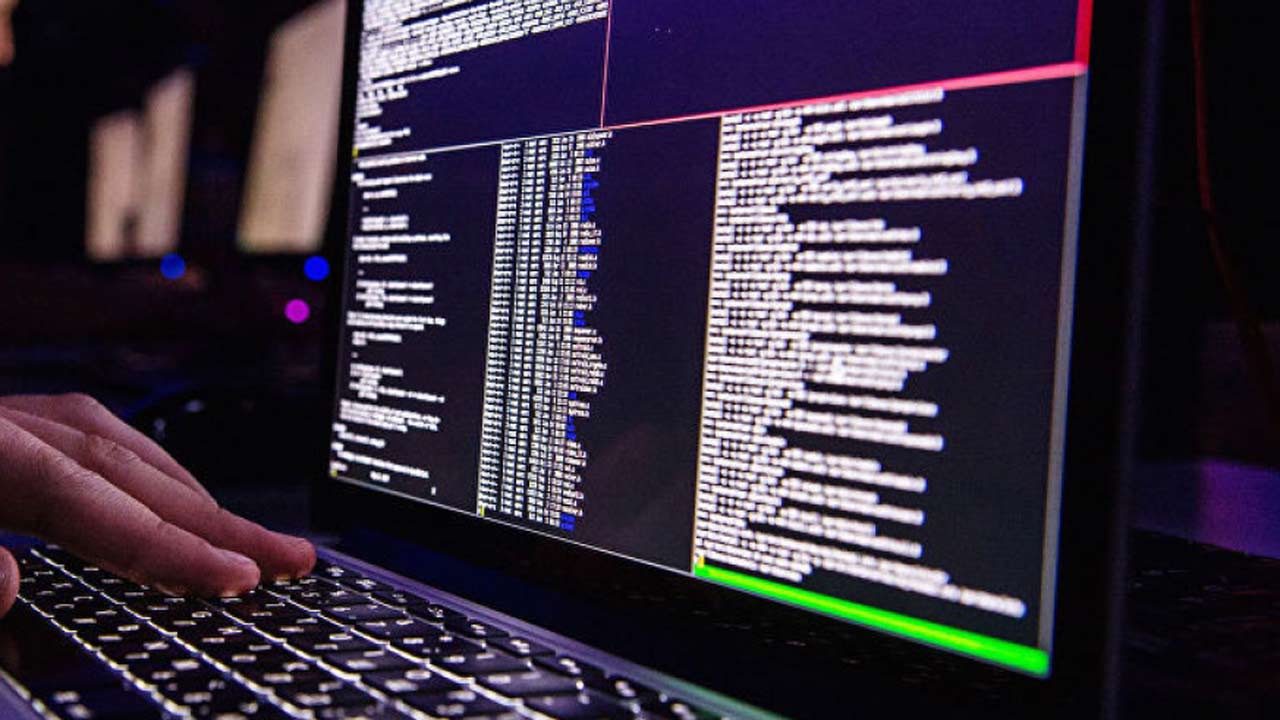
Tier 5 Technologies, a West African enterprise IT and cloud services provider, has announced a partnership with MongoDB, a database application platform, to aid its expansion in the West African market.
This partnership, which focuses on helping organisations modernise their infrastructure to capitalise on the opportunities of AI, was revealed at a recent “Legacy Modernisation Day” event in Lagos, co-hosted by the two companies.
The Tier 5 Technologies-MongoDB partnership aimed to harness Africa’s enormous potential, with the continent’s digital economy projected to reach $100 billion. MongoDB’s mission is to empower innovators to create, transform, and disrupt industries with software and data, and MongoDB is the most widely available, globally distributed database on the market.
Millions of developers and more than 50,000 customers across almost every industry—including 70 per cent of the Fortune 100—rely on MongoDB for their most important applications.
Regional Director for Middle East and Africa at MongoDB, Anders Irlander Fabry, said: “Partnering with Tier 5 Technologies is a pivotal step in deepening our presence in Nigeria—the most populous country in Africa and a key economic engine for the continent.
“Tier 5 Technologies brings a proven track record, a strong local network, and a clear commitment to MongoDB as their preferred data platform. They’ve already demonstrated their dedication by hiring MongoDB-focused sales specialists, connecting us with top C-level executives, and closing our first enterprise deals in Nigeria in record time. We’re excited about the impact we can make together in this strategic market.”
MongoDB’s product suite includes self-managed MongoDB Enterprise Advanced and MongoDB Atlas, a fully managed integrated suite of cloud database and data services available across AWS, Google Cloud, and Microsoft Azure. MongoDB Atlas is particularly relevant for the African market, where cloud adoption is on the rise and traditional IT infrastructure can present challenges. By delivering scalable, low-latency databases via the cloud, MongoDB aims to overcome infrastructure limitations and empower developers across the continent to build world-class applications.
Director of Sales at Tier 5 Technologies, Afolabi Bolaji, noted the strategic nature of the Tier 5 Technologies-MongoDB partnership.
“This isn’t just a reseller deal. We’ve made significant investments in MongoDB because we believe it will underpin the next generation of African innovation. Many of our customers—from nimble fintechs to established banks—already rely on it. Now, they’ll have access to enterprise-grade features, local support, and global expertise,” he stated.
Article originally posted on mongodb google news. Visit mongodb google news
ScyllaDB X Cloud’s autoscaling capabilities meet the needs of unpredictable workloads in real time
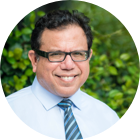
MMS • RSS
Posted on nosqlgooglealerts. Visit nosqlgooglealerts
The team behind the open-source distributed NoSQL database ScyllaDB has announced a new iteration of its managed offering, this time focusing on adapting workloads based on demand.
ScyllaDB X Cloud can scale up or down within a matter of minutes to meet actual usage, eliminating the need to overprovision for worst-case scenarios or deal with latency while waiting for autoscaling to occur. For example, the company says it only takes a few minutes to scale from 100K to 2M OPS.
According to the company, applications like retail or food delivery services often have peaks aligned with customer work hours and then a low baseline in the off-houses. “In this case, the peak loads are 3x the base and require 2-3x the resources. With ScyllaDB X Cloud, they can provision for the baseline and quickly scale in/out as needed to serve the peaks. They get the steady low latency they need without having to overprovision – paying for peak capacity 24/7 when it’s really only needed for 4 hours a day,” Tzach Livyatan, VP of product for ScyllaDB, wrote in a blog post.
ScyllaDB X Cloud also reaps the benefits of tablets, which were introduced last year in ScyllaDB Enterprise. Tablets distribute data by splitting tables into smaller logical pieces, or tablets, that are dynamically balanced across the cluster.
“ScyllaDB X Cloud lets you take full advantage of tablets’ elasticity. Scaling can be triggered automatically based on storage capacity (more on this below) or based on your knowledge of expected usage patterns. Moreover, as capacity expands and contracts, we’ll automatically optimize both node count and utilization,” Livyatan said.
Tablets also increase the maximum storage utilization that ScyllaDB can safely run at from 70% to 90%. This is because tablets can move data to new nodes faster, allowing the database to defer scaling until the last minute.
Support for mixed instance sizes supports the 90% storage utilization as well. For example, a company can start with tiny instances and then replace them with larger instances later if needed, rather than needing to add the same instance size again.
“Previously, we recommended adding nodes at 70% capacity. This was because node additions were unpredictable and slow — sometimes taking hours or days — and you risked running out of space. We’d send a soft alert at 50% and automatically add nodes at 70%. However, those big nodes often sat underutilized. With ScyllaDB X Cloud’s tablets architecture, we can safely target 90% utilization. That’s particularly helpful for teams with storage-bound workloads,” Livyatan said.
Other new features in ScyllaDB X Cloud include file-based streaming, dictionary-based compression, and a new “Flex Credit” pricing option, which combines the cost benefits of an annual commitment with the flexibility of on-demand pricing, ScyllaDB says.