Category: Uncategorized
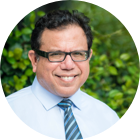
MMS • Igor Canadi
Article originally posted on InfoQ. Visit InfoQ
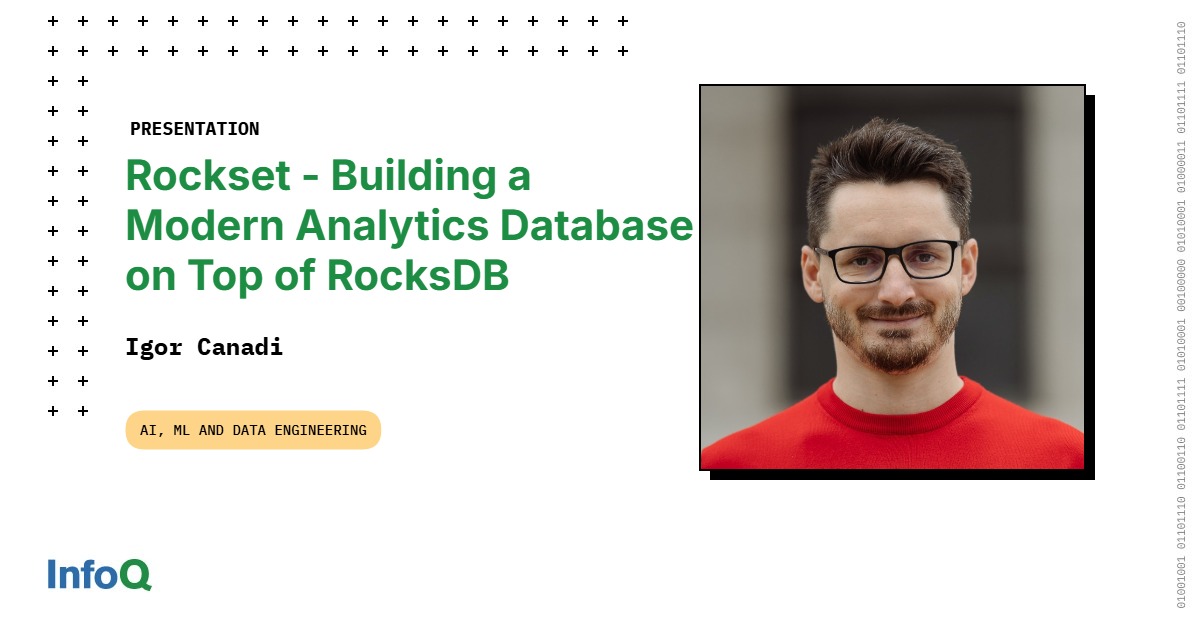
Transcript
Canadi: My name is Igor. I’m a founding engineer at Rockset. I’m very excited to talk to you about how we built a modern search analytics database on top of RocksDB. Let’s say this is the system we want to build, the box with a question mark here, where the system should be receiving some updates from a stream, where the updates could be either document insertions or document mutations. The system should store that data and then respond to different kinds of queries with the SQL language. These queries could be search queries, which means we would probably want to build some inverted index on top of the data. They could be vector search queries, meaning we would probably want to build a similarity search index. Then, finally, we also want to support very fast and efficient real-time analytics queries.
On the right-hand side, you can see that the consumer of our database should be an application, which puts some constraints on how we want to build this. If there’s a human, there’s a UI waiting on the other side. We want to make sure that our query latencies are very low. We also support high-concurrency queries. Then, finally, we also want to make sure that the latency between the document coming into the stream and the document being reflected in the query, something we call ingest latency, is low. In our case, our budget is 1 second, so we want to make our ingest latency sub-second. This is a generic system that we’ll talk about here. Rockset, obviously, it’s not a surprise it does all of that. Different architecture pressures would apply to any system that looks like this, any stateful system in the cloud. A lot of the lessons would apply to other system builders, I hope.
Rockset is a search analytics SQL database. It’s real-time, meaning that our ingest latency is sub-second. It’s cloud-native. It only runs in the cloud. We try to take full advantage of the fact that we run in the cloud. It’s optimized for applications. We need to make our queries very low latency and high concurrency. There might be a lot of users of our applications of our database. Rockset is built on RocksDB. How many of you have heard of RocksDB before? There will be some parts that are specific to RocksDB, but we’ll get through them. It’s a key-value store based on log-structured merge tree data structures. It was open-sourced by Facebook in 2013. We just recently celebrated the 10th anniversary. In the last decade, it has seen a wide adoption across the industry, but not typically for analytical use cases. Typically, it’s been used for some sort of search or as a key-value store. It hasn’t been used for analytical engines. Here, Rockset is a bit of an example, which makes this talk hopefully interesting.
Outline
The talk will be four parts. The first, I’ll talk about cloud-native design and what that means for us. I’ll talk about RocksDB replication, which is something we built. It’s not yet part of the upstream. It is open source, but not part of the upstream RocksDB yet. I’ll talk about shared hot storage: why we use it, and how we designed it. Then, finally, I’ll talk about how we built analytics on top of RocksDB and made it efficient.
Cloud-Native Design
First, cloud-native design. We will go back to our slide before. The question mark is no longer a question mark. This is Rockset. The first choice we have to make how we will build this system is we want it to be scalable. If we want to make a system scalable, that means we want some sharding. One box is not going to be enough to deal with the data footprint that we want to support. We’ll have to have more than one box. We’ll have to have more than one compute node. Then when you have more than one compute node, you have to somehow decide, how do you store data on different machines, on different boxes? We decide we want to do sharding. The question is, which sharding? There’s a very big body of research on sharding and how to pick one. It comes down to a couple of choices. We’ll go through these choices and how we made our choice.
The first question is, can our documents change? There are systems out there where they say our documents are immutable. That makes a lot of things easy. We do believe that it makes it easy for the developer of the database, but not for the user of the database. We decided we want our documents to be fully mutable, they can change in whatever way you want them to change. The next question is, for a particular document, how do we pick a shard that it lives on? Are we using a value within that document itself? We can call this value a clustering key, for example. If you do that, we call it value-dependent mapping or clustering. That gives us an opportunity to issue bigger I/Os because we get some locality.
If you have a query with a predicate on clustering key, all of that data that it cares about lives on a particular shard, and all the data is close together. It means our I/Os are bigger. If you think about it, if you actually make that choice and your data is mutable, that means your clustering key can change as well. If your clustering key changes, that means a document from one shard needs to be donated to another shard as part of the ingest process. If you also combine that with our requirement of low ingest latencies, you get this coordination overhead that grows incredibly large to the point where it’s not actually feasible.
The way systems solve this, and a lot of systems build this clustering or value-dependent sharding, is by batching, saying our ingest latencies are 30 minutes, and within that 30 minutes, we can amortize the cost of moving data across shards. For us, if our write latency is sub-second, we just don’t have that compute power, and we don’t want to pay that cost. We are not going to do that. What we will do is we will use this technique called doc sharding. Doc sharding is a technique out of the search engine community, where the way you map documents onto shard is based on document identity rather than value within the document itself. You can think where you’re just randomly spreading documents around shards.
The downside of that is your I/Os are now smaller, because your query needs to talk to every single shard, and every single shard will have some small portion of that query. What you get is you get very efficient streaming ingest. Because everything that you care about for that particular document lives on that shard, and it will always live on that shard. All of the ingest process happens within the shard, and there’s no communication between shards. You also get consistent indexes, because we decided to put indexes and data together on the same shard. We made them consistent by using RocksDB or any other key-value store that supports atomic writes across keys. Most of them actually do.
To illustrate this I/O question, in the clustering with your data locality, you might decide, I’m going to read only one shard, and all of my data will be contiguous. I’m not actually reading less data, but I’m just reading data with smaller number of I/Os. In doc sharding, you can see that data can be anywhere, and then small parts of the data on each shard. The number of I/Os that I have to issue to storage is going to grow. Now we have our choices. We will do doc sharding, which gives us scalability, because we can spread our data across multiple boxes. It also gives us streaming ingest. Getting the sub-second latency when you have doc sharding is quite easy.
The next problem we have in this architecture is that our boxes, our system, is doing two things at the same time. It is ingesting data from the stream, trying to keep that very low write latency, and also answering queries at the same time. If you have some spike in our ingest throughput, that is going to affect our latency for the queries. Because queries are part of applications, that’s going to regress our application, and our customers will get mad. The way to solve that in the architecture diagram is quite easy. You just have two boxes. You have one box, which we call ingest worker, that is doing the work of ingest, and then one box that is a query worker, it is doing the compute that is used on queries. This is illustrated here with colors. You have the color of orange, which signifies ingest, and then the green color is queries. We want to make sure that our query worker keeps our write latency low, so below 1 second, while also not spending any or very little cost on ingest. That’s quite hard to achieve.
The key part here will be that ingest worker will need to subscribe to our logical stream. Then, what ingest worker gives to the query worker will have to be very cheap to apply. We’ll talk about that in our RocksDB replication part of the talk. Now that we have this isolation between ingest and query compute, there’s no reason to also keep just one query worker. We can have many. For different applications, we can have different query workers. If you have a temporary application, let’s say we’re running a big reporting job, we can scale up another query worker. It can run the big query, and we can shut it down. You can have as many as you want. If application A suffers increasing load, we can scale that application query worker up without touching anything else.
Now we have something that’s good. It’s much better than what we started with. The question here becomes, if you have a lot of applications, then our storage footprint grows. You can see the storage is part of our compute node here. If you have 10 query workers, you’ll copy the storage 10 times. Not only that, it also actually doesn’t work well with our elasticity. If application A gets a spike in load, it gets very popular. Yes, that’s awesome. To serve that, we will want to scale up the query worker. To scale up, we need to shuffle this compute around. The new worker will have to ask AWS for a new machine. Then before the machine starts actually being useful, you’ll have to load up with its compute. That’s quite slow.
In our case, we’ve seen it take tens of minutes. If you have a spike in load, you don’t want to wait tens of minutes for a database to scale up. To solve that, we have this concept of disaggregated storage, where storage is not attached to the compute node. It is somewhere on the side. If you can do that, and if you can make sure that every single ingest worker, query worker, have the same view of the data, so the files it accesses are byte identical, then we can also dedupe them. The query hot storage can keep only one copy of our dataset. That also means that our elasticity is much improved, or it’s much more responsive. If application A now gets a spike in load, we can get more machines from AWS, and they can start running those queries very quickly. We’ve seen in our examples that process takes 30 seconds. Imagine if your application A now gets very popular, 30 seconds later, your database has more horsepower to run your queries.
The next question that happens, and that ties back into our doc sharding problem, is, what technology do we want to use for disaggregated storage? The storage technologies that AWS and any other cloud provider uses come in two flavors. One flavor is an object storage. In AWS’s case, it’s S3. That is cold side of the spectrum of our data, where it’s actually quite cheap in terms of number of dollars per gigabyte. It’s highly durable. It’s probably the most durable technology out there for the small amount of dollars it costs. The benefit of S3 is that you also get just SDK. You download the library. You can start using on day one, which is very easy. However, the big downside is the latency of IOP from S3 is quite high. It’s hundreds of milliseconds. I told you that Rockset is optimized for application, meaning that for a lot of our tail latency budgets are hundreds of milliseconds. We want the entire query to return hundreds of milliseconds. If you use S3, then just single IOP will do it. The major downside of S3 is that cost per IOP is extremely high. If you do a lot of small IOPS, which you do with doc sharding, then this cost actually becomes the majority of your total cost.
Then the right side of the spectrum, we have something like hot storage, which is flash. It’s very cheap in terms of dollars per IOP. EBS is more expensive. NVMe is what we use. You get a lot of IOPS. It’s super low latency. The downside is you have to build your own service, like you have to build your own hot storage service. We like to build, but it’s a long time to build this properly. Then the upside is you can build it the way you want it, and you can build it very efficiently. You can hopefully pass those cost savings on to your customers. The major downside of using flash, obviously, is that it’s extremely expensive in terms of dollars per gigabyte. Then you want to stay somewhere in the spectrum, you want to be a blend of the two. For Rockset, we mostly stay on the right side of the spectrum, with some plans of making S3 a bigger part of some of the queries. Today, it’s not.
To finalize this section, we talked about, how do you build cloud-native search analytics? We picked people to use doc sharding with indexes. We call this technology converged indexing, which gives us scalability and streaming ingest with sub-second latencies. We talked about post-ingest replication, and we’ll talk a little bit more about that later. We call this compute-compute separation, where we separate out query and ingest compute. That gives us isolation between those types of compute. Also gives us elasticity, where we can scale each worker based on its needs. Then, finally, we talked about this aggregated hot storage, we call this compute-storage separation. It gives us compute elasticity. It also gives us high disk utilization, because we can scale our storage tier based on the storage needs, not based on the compute needs. It also gives us storage elasticity, where we can scale it up and down based on our needs.
RocksDB Replication
The next thing I want to talk about is RocksDB replication. This is going to be more interesting to people who actually have heard of RocksDB before, but I’ll try to make it accessible for everybody. RocksDB is a library. It’s a log-structured merge tree. The way log-structured merge trees work is that all of your writes are buffered in some in-memory write buffer. We call this memtable. Then when the memtable is full, we flush it to storage. Then the cool part of when we flush it to storage, we also have this process called compaction, where we take some files from storage and produce new files again. The cool part here is the files never change.
Once the file is written, the only thing that’s going to happen to it is going to get deleted. It’s never going to get updated. This is underpinning of a lot of our decisions and a lot of our simplicity in our design is the fact that our storage files are immutable. They never change. This comes from RocksDB itself. The second key point is when the document comes in to Rockset, and Rockset supports any sort of JSON document on ingest, we actually store it in different layouts in different places in RocksDB. It’s still a single RocksDB instance, but the way we store it, it’s a bit complicated. We store it in a search-optimized format, which is an inverted index, and that’s what we use when we have queries with a very selective WHERE clause. We store it in a scan-optimized format, which resembles a column scan.
Then, finally, we also store it in a document store, where it’s just a map between a primary key and a document value. The major point here is that the mapping between a document that comes from our ingest stream and the keys and values in RocksDB is quite complex.
You can think of ingest, that you have this logical update coming into RocksDB, and then ingest is the process that turns the logical update into a set of physical deltas. By physical deltas, I mean a set of RocksDB keys and values that need to be updated. Then those deltas are inserted into the memtable, and then later on, RocksDB takes care of it by merging it through its LSM tree. It turns out this ingest process, this process that turns logical updates, JSON documents, into physical deltas is quite expensive. It is one order of magnitude more expensive than applying those physical deltas into RocksDB memtable. The way we built RocksDB replication is by having the ingest worker, you can see it on the left-hand side, and also the query worker. The ingest worker is the one that takes the logical updates and produces the RocksDB key-value pairs we need to update.
Then we apply those updates to the memtable on our ingest worker. Then we also send it through the replication stream onto the query worker. The query worker will still do the work where it applies those keys and values into the RocksDB memtable, but that is a cheap part. In our case, we’ve seen it take 6 to 10 times less CPU than the work of actual ingest. That is what happens in our replication stream.
The second part of our replication stream, going from our ingest to query workers, is flush and compaction. Flush is a process where RocksDB takes the memtable. Memtable is full. It goes above a certain size. Then it flushes into disk. That means it produces a file. That process only happens on the ingest worker. When the file is ready, then it sends the notification about, this file is ready for you, through the replication stream into the query worker. The query worker, the only thing it has to do, it applies that notification into its metadata. The metadata says, now there’s a file for me to read. That update is extremely cheap.
Then the 30% of CPU on the ingest worker is actually spent running compactions. Compaction is a process where RocksDB takes some files in an input, produces files in the output, and deduplicates all the values, merges them together, and so on. That also only happens on the ingest worker. The query worker only gets a metadata update saying, there are some files for you. Some files you need to remove, they’re obsolete. Then some files that you need to add to your metadata. All of those files are communicated through our shared hot storage. That’s the way we also make sure that the query workers and ingest worker storage is deduplicated. They are actually accessing the same files. That’s our story of RocksDB replication in brief. This is a custom thing that we built. It’s part of the open-source RocksDB cloud library. It’s not yet part of the upstream RocksDB.
Shared Hot Storage
Next, I want to talk a little bit about our shared hot storage tier. We’ll go back to the RocksDB. RocksDB is a log-structured merge tree. Now we’re going to look at it from the prism of, what are the actual I/O patterns that we need to support? The I/O patterns, this is the slide for the writes side. We talked about the data comes into our memtable. Memtable, when it’s full, it’s flushed to disk. When it’s flushed to disk, we use 64 megabytes as the default value of the file. 64 megabytes of values of the file is written in one go. Then on the compaction side, we again take some files in the input, read them, and then rewrite them again in one go. Our writes are huge. The other thing that is important to note is we actually don’t care about the latency too much. The compaction is so expensive that the file writes at the end are small, tiny parts of its latencies. We have big writes, and we also have async writes. We don’t care about their latency too much.
On the read side, the story is a bit more complicated. We have different indexes, indexes that are good for search queries, indexes that are good for analytical queries. We have our cost-based optimizer that, based on the query fragment, not the query itself, but part of the query that reads from a collection, decides which of those two indexes we want to use. We have more than two indexes, but that’s just the simplification. You can see that for some column scan, our I/O pattern will be just a big scan, like big I/O. Here, scanning from hot storage, we’ll have large read, and we’ll mostly be bandwidth limited. That’s actually quite easy to support. Big reads are easy.
However, the challenge comes from search I/O patterns. On search, you first access the inverted index. Accessing the inverted index is a lot of random reads. The worst part actually happens, the inverted index gives you a list of doc IDs, not the actual documents. Now you have to take those doc IDs and go to the document store to actually get the other values of the document that you care about. That is, again, a lot of random reads. That’s the one we’ll actually care about here. Here, we are bottlenecked on small reads. We’re bottlenecked on latency. We actually care about those latencies a lot. Then we’re also IOPS limited. The simplifying insight here is that our big writes need to go to S3. There is no reason not to. We don’t care about the latency. The additional latency of S3 put is not big in terms of our compute cost.
Then our writes are big enough where the cost per IOP doesn’t matter. For small reads, especially because we are limited by IOPS with our doc sharding scheme, we need to read from SSD. There’s no way we can do anything with S3. Then the final architecture looks something like this. The ingest worker is the worker that produces files. Those files go to S3. Before those files are marked as committed, we also ask our hot storage tier to download those files. That’s additional latency on our write. Again, we don’t care too much about write latency. We wait for the hot storage tier to have the file.
Only when the file is already there, then we can mark it as committed. We say this transaction is now committed. We send it to our query worker. As soon as query worker touches our hot storage tier, that file is there. We have big writes go to S3. S3 is also our durability layer, which is awesome. Our reads go to SSD, which gives us low latency, high IOPS, all the good stuff. Small reads are not a problem. We also get high space utilization because we size our hot storage tier based on the storage needs, not based on the compute needs. We get some compute from AWS because that’s how you buy it. It’s a minor part of the puzzle here.
If you think about it, our hot storage tier is essentially a cache. It just caches what is in S3. The biggest challenge in this cache is that your miss, if you have a miss, then you have to go to S3. Our hot storage, one IOP latency is about hundreds of microseconds. Your cache miss to S3 could be hundreds of milliseconds. That’s three orders of magnitude slower. If you have a cache where your cache miss is 100,000 times slower, you get a very unstable system. What we did is we went through and enumerated all the reasons why you can have a cache miss in this kind of system, and we fixed all of them. We’ll go through them one by one.
First problem is you can have a cached cold miss. Cold miss is that you have cache access for a file that the cache doesn’t know about. It’s the first time it’s heard about that file. To fix that, we mentioned this before, where after we write a file to S3, we don’t consider that file as committed before we prefetch the file in hot storage. Only after the hot storage has the file, then we can say, now we can commit the file. Then we also have a secondary mechanism, catch-all, where we periodically list S3. If you find some file in S3 that we don’t have, we download it, just to be on the safe side. The second challenge is a capacity miss. That means we just don’t have enough capacity for the data we need to store in our cache. To fix that, we have autoscaling controller that we built that gives us some buffer.
If this space goes under that buffer, we get new machines. The cool part about our system, we actually know how much capacity we need well ahead of time. You can have a customer that comes in. We have this process called bulk load, where they give us hundreds of terabytes of data. It takes some time for us to process that, index that, build that. While that’s happening, we already can notify our hot storage tier, I have 100 terabytes here. Can you please ask AWS for some machines? Because we’ll need them in tens of minutes, maybe half an hour is when we’ll need that process. It takes half an hour. It has maybe like one hour to load hundreds of terabytes of data into Rockset. This is not a problem.
The next challenge is, at some point, you have to deploy. We actually deploy quite frequently. When you deploy, the default way of how Kubernetes deploys, it kills your pod, brings a new pod, downloads a new image, and then it brings a new process up. That’s not great for us, because when the pod is down, then who’s going to serve those files? You’ll get cache misses during the deploy. To fix this, we built this thing called zero-downtime deploy, where we have two processes temporarily. They have the same state, because our state is actually in POSIX file system, so they can interact and actually have the same consistent state. If one process downloads a file, this other one sees it. Then, obviously, we slowly drain the old process, get the new process up and running. If everything’s ok, we kill the old process, and our deploy is done.
Next challenge is cluster resizing. Let’s say we get a new machine in our cluster, and now our hashing policy, which hashes file to node, keeps it, decides, there’s a new node in our system in our cluster, therefore, it probably has our data. If it’s new, it still hasn’t bootstrapped. It hasn’t downloaded all the data yet. What we do is we have this process called rendezvous hashing, which gives us a very nice property, where if you add a server to your cluster, it will tell you this is now a new primary. It will also tell you whoever was the primary before. It keeps this list sorted based on some hash value. In this case, let’s say we add server-12, that server-12 will now be primary server for our file. In the new config, we will also know that server-3 was the primary before. We’ll first try, ok, we’ll try to read server-12. From server-12, there is a miss.
Then you’ll have a second chance to go to server-3 and hopefully get that file there. Failure recovery is also a problem, where if a machine dies, we use AWS i3en for our hot storage tier. If you just divide your disk capacity with disk bandwidth, you get 48 minutes is how long it takes to warm it all up. That’s obviously too slow. Rendezvous hashing helps. If you have 100 machines in your tier and one machine fails, everybody else gets 1% of the recovery work. That brings the recovery work down to 28 seconds. Not only that, we also keep an LRU list of hot files. Then we prioritize downloading those hot files early, just so that we reduce the chance of cache misses.
As a result of all this, once we did all of that, our cache hit rate is actually quite high. We got to six nines of cache hit rate. This was actually true at the time when we wrote those slides. It has been six days since the last cache miss in production. I think it’s probably higher now, where every time the cache miss happens, we have an alert. It doesn’t wake anybody up, but it does send you an email. Then we go in and investigate. We try to make it super high, because as soon as you have a cache miss, that might mean that that query will time out for your customer if it’s an application query.
Analytics On Top of RocksDB
Then, finally, I want to talk about analytics on top of RocksDB. This is the hard part. This is something that people are not usually doing, and for good reason, if you ask me. Here, we go back to our slide on converged indexing, where we store our document in search index, in column store, and in document index. In this case, we’ll talk about column store specifically. That’s the harder part to get right here. If you talk to any analytics vendor, they are mostly storing analytics data in column stores. The two superpowers of a column store are, because it stores values together for a particular column, it can encode and pack them very tightly.
Then it can also operate on those packed values itself. You can use this thing called vectorized processing, where you have a bunch of values, and you can just easily iterate through them, and even use SIMD, if you fancy, to make that process faster. Let’s see how naive implementation of RocksDB fares on those two properties. This is how it would look like if you just started building column store on top of RocksDB. On the encoding side, just to store one value, you’re paying so much cost. You still have a key size, because RocksDB’s keys are variable length, so you have to know what the size is. Then you have the key. Then you have the type, which could be update, merge, or delete. Then you have a sequence number that’s 8 bytes, because RocksDB offers MVCC through the sequence numbers. Then the value size, in case it’s 1 byte, it just says 1. Then you have 1 byte of the value. This is too much fluff to store one value. Then in the vectorized processing side, let’s say we want to find all the values that are greater than 5, and this is an integer column, you’re paying so much cost. The RocksDB’s iterators are per row. They don’t have any batch access.
To do that, the overhead of just getting the next element in the iterator is way too high. This is not vectorized processing at all. All of these are also virtual method calls, and the implications are huge. Here, the RocksDB overhead will dominate anything you try to do. Compare this with a typical columnar store, which would store those 1-byte values as a vector of bytes or a vector of Bools or whatever. Then your loop will be super simple. You just iterate through, and you find all the values greater than 5, and you’re done. This is no contest. RocksDB here is many orders of magnitude slower. The key thing here, what we can do, is instead of storing one value per key, we can store a batch of values per key. Now, this bold thing is what changed. Instead of storing one value, we store a batch.
Then on the vectorized processing side, you can see that we still pay RocksDB costs, but we pay RocksDB costs once per batch instead of once per row. Then our hot loop, where the compute is spending most of the time, is actually the same as in the columnar store. We pay some amount of RocksDB cost. If you increase the batch sizes, then it gets negligible. On your actual kernel, on what you care about, you’re going to be as fast as a typical columnar store.
We have this thing called batched column store, where we store more values in a single RocksDB key. We map our document’s primary key into doc ID, which you can imagine is just a monotonically increasing integer. Then we have a map between a column and doc ID range into a batch of values. We made the RocksDB overhead once per batch instead of once per value. We have no RocksDB operations at all in our tight loop. If you look at our CPU profile of Rockset today, there’s very little RocksDB there on the query workers. This is somewhat of a side note. This is what we also offer, which is a clustered column store, where instead of column and doc ID range, we have column and cluster key range, which maps to batch of values.
The benefit of clustered column store is we can do cluster pruning, where we can decide ahead of time, we don’t care about this cluster, because of our predicates that we run. The challenges here is we have a challenge of dynamic mapping, where cluster key can change. We have fully mutable documents, and if the cluster key changes, you have to move the document from one cluster to another. This is still within a single shard, so it’s a much smaller problem. It’s still some coordination overhead within a particular process. Then, if the cluster grows too big, because now we have this dynamic mapping, we have to split it. Those are the challenges we have fixed. It does add some amount of overhead, but the cluster pinning makes it worth it.
The challenge then becomes, if I go to store a batch of values in RocksDB, is how do I change it? Let’s say I have a modification, and what we care about here is write amplification. Write amplification is the ratio between how much data you want to change and how much data you actually end up changing. In this case, we want to change just one value. We have this full column, and we want to change the document 1 to the new value of 2. It was 3 before. To do that, we need to rewrite the entire batch. To change 1 byte of value, in this case, if your batch size is 4,000, we need to write 4,000 values into RocksDB. This is super high overhead on our write side. What we can do instead, is we can have a merge update, where RocksDB offers a merge update, where instead of rewriting the whole value, you just have a delta. In this case, our delta could be very small. We can say, change my document 1, the new value is 2. We use this thing called RocksDB merge operators, where we write deltas to the memtable.
Then those deltas are merged together in the process called compaction. When the compaction happens, when two keys come together, then merge operator is invoked, and the new operation is written out. The challenge here is that we also have to compute this result during the read time. If you have the base, and then you have deltas, that merging happens during the read time. This is a side note for people who used RocksDB before, the API of RocksDB is not good in this regard, where it asks the merge operator, it’s a callback that takes these operands and produces a value. It produces the value in a string format. If you think about what merge operator has to do, it has to take all of these operands, decode them, merge them.
Then because RocksDB has a string interface, it has to serialize it, give it to RocksDB. RocksDB gives this to our application, and now we have to deserialize this again. We have this serialize, deserialize that’s completely unnecessary. To work around this API limitation, we have this thing called lazy merge operator. I don’t know why it’s called lazy. I think because it doesn’t do anything. It doesn’t actually merge anything. It just gives us the operands through this thread_local side channel, where we say, this is a read. We don’t care about the actual result. Tell RocksDB you did, it worked. Just give it an empty string, and give us the operands, and then we do the merge in the application layer.
The next challenge is that, you can imagine now there’s a lot of column scans happening. We have high QPS, so a lot of them will repeat the same work again and again. Obviously, if you have a change, then that compute will not repeat. If you have two column scans on the same snapshot of the data, then they will do this merge operation. Both of them will do them. Maybe you can have more than two. The challenge here is that, obviously, if this merge is expensive, what we want to do is we don’t want to repeat that work. How we avoid repeating the work in our system is we want to cache the results post-merge. RocksDB doesn’t offer anything like that out of the box. The only cache that RocksDB offers is a block cache, which caches parts of the file.
In files, it’s not post-merge operation, it’s pre-merge operands that you get in the files. Not only is it pre-merge, it’s also serialized. What we built is we built this application-level cache. You can think of it as a RocksDB key is what we key the cache on. The value is specific to its in-memory format of our application. It’s not serialized format. It’s deserialized in our in-memory format, the one we care about. Also, it’s post-merge. This is something we built. It’s actually quite tricky to get right because you also need to support MVCC semantics. If there’s a mutation to the RocksDB and you get your snapshot post-mutation, you’re not allowed to touch the cached values before that mutation. You have to make sure that whatever you get from the cache is exactly the same as you would get from RocksDB based on that snapshot. This is MVCC cache. We made this generic, where different keys can have different types of values.
In the case of the column scan key, the value will be this post-merge in-memory data format that we can just send into our query execution engine. We also store things in the row store and in our document store, where the value is, again, something that is not serialized. It’s deserialized in our format, and makes a lot of the access easier. That helps for people who have very big Rockset deployments because they also get a lot of memory. A big part of their dataset is actually cached. This makes their query much faster than if the data is not cached. The interesting problem here is, how do you size this? RocksDB gives you a cache, and we have a cache. How do you decide dynamically what is the optimal size of these two caches? Currently, it’s static, but we’re trying to make it dynamic and try to make this smarter. We don’t know how to do it yet.
Finally, to build fast analytics on top of RocksDB, we have this challenge where iterator overhead is too high. Then we work with batches to make sure that we pay the cost of iterator once per batch instead of once per document. Our batch updates are too expensive if you work with batches. To fix that, we use the merge operator. Then, finally, because when we use merge operator, our merging layer becomes too expensive, especially at high concurrency, and the result here is cached result. This all gives us a very nice analytical implementation on top of RocksDB.
Then, finally, RocksDB gives us superpowers. It gives us file immutability. Files never change, which is the underpinning of our cloud-native design, and makes building our shared hot storage much simpler. It gives us real-time writes. We talked about our write latency needs to be sub-second. RocksDB supports this out of the box. Something we didn’t talk about is our converged indexing is actually quite expensive. The document that comes in to Rockset is shredded into many different places, and that’s quite expensive. RocksDB is actually a write-efficient storage engine compared to other data structures out there. This comes out to the LSM. Its write efficiency is actually the underpinning of our converged indexing design.
In any alternative, the converged indexing would just not be possible. You would not be able to process that amount of writes, and that amount of write amplification with this converged indexing approach. The downside of RocksDB is it’s actually not built for analytics. We want it to be built for analytics because our customers are running analytical queries on top of Rockset. It actually could be made to work. I hope some of these ideas come into the upstream RocksDB. For us, currently, it works well. This is the blueprint of how we made it work.
Questions and Answers
Participant 1: The move from the object store to the flash, how do you actually do that? How do you make it fast enough? Because if you’re trying to do everything in sub-second and it’s hundreds of millis to interact with the object store, and you’re doing two hops, then you’re already running out of time.
Do you have any interesting data consistency problems with the analytics? If you parallelize your querying so you’re running sub-sections of it simultaneously, but the store is changing underneath your feet, how do you make sure that the results correspond to a consistent view of the data?
Canadi: How do we actually achieve sub-second latency with the overhead of writing the file to S3 and then uploading that file into hot storage? That is actually not on the critical path of ingest. The critical path of ingest is inserting data into our memtable, into the in-memory write buffer. As soon as the data is in the memtable, that data is present in any further query that happens. Then in the background, async mode, you get that memtable once it becomes full. Then you flush that memtable into S3 file. You do that all fairly slow. The only downside is that memtable needs to be alive for a little bit longer.
The fact that we also need to account for this S3 flush and then also uploads to hot storage means that my memtable will be maybe couple hundreds of milliseconds longer, is how long it will be alive before we switch from memtable to the file. It’s not on the critical path of ingest. As soon as we put the document into the memtable, that document is present in the query, both on the ingest side and on the query side.
Do we have any interesting consistency challenges? RocksDB gives you MVCC semantics, which means you can create a snapshot. Then every time you read from RocksDB, you give it that snapshot, and it will ensure that the data you’re seeing is consistent. That’s within a shard. We talked about our shard has indexes and data that are consistent with each other. That is built on top of RocksDB via MVCC. Even if you have a query that’s running for a long time and the data is changing, you’re going to get the snapshot view of the data, which is provided by RocksDB’s MVCC semantics.
Participant 2: I noticed that you showed the use case of inserting data, that you’re writing data from Rockset into S3, and then telling the NVMe backend to fetch it from S3 and download it. Is there a reason why you don’t send it to S3 and simultaneously to your storage backend? Why do you go the way of telling the backend to download it from S3?
Canadi: Why do we, when we have this ingest worker create the file, that file goes to S3, and then our hot storage tier downloads from S3 instead of being sent to the file directly from our ingest worker that has it? The reason why we do that is because we don’t want to add more compute needs and compute demands on our ingest worker. Our ingest worker is something that is precious. We don’t want to have it do two things, where you upload the file to S3, and then we have to spend, obviously, hot storage compute to download that file. We don’t want to touch the ingest worker and make it spend even more compute to now send the file to two destinations. Also, networking side as well. We might want to do that, but if you do that, then the only thing you do is you’re reducing the time to commit the file during the write time. We actually don’t care about that latency at all.
The additional latency of downloading the file in the hot storage tier is about hundreds of milliseconds. That’s small compared to the whole flush and compaction process that RocksDB runs. It’s similar to a chain replication idea. You don’t want to have the ingest worker do too much. You want to do as little as possible. S3 is a nice multi-tenant system that can handle that download, no problem.
Participant 2: You said you consider the file committed once in S3. What about the time between the file being committed and the file being available in your hot storage? What happens if you try to read it while it’s committed, but not in the hot storage, or not available in hot storage? Would that be a miss in that case?
Canadi: What happens if the file that we write is not yet present in the hot storage before the query worker goes and reads it? That would be fairly bad, because then the query worker would go to hot storage, would have a cache miss, and it would then have to go talk to S3. It’s possible. It could happen. We talked about six nines of reliability. There’s still one there at the end of the six nines. It does happen sometimes, every six days, apparently. We try to avoid that. The way we avoid that is by making sure that we just don’t commit it. The commit protocol for a file, you can imagine a file, you first write it to local storage.
Then you upload it to S3. Then you synchronously wait for the hot storage to download it. Then only when the hot storage says, I promise I have this file, hopefully it’s telling the truth. Then it says, now I can actually commit that file. The fact that the file exists goes into the replication stream after the file is part of the hot storage. It’s best effort. It’s true. Because sometimes, you might have a cluster recess at the same time, and the new one doesn’t know about it yet. Then we have other technologies to go back to the previous primary to see if their file is still there. We work around that fact.
Participant 3: What do you do in compaction? What’s the criteria? What’s the logic behind that?
Canadi: What do we do about compaction? We use off-the-shelf RocksDB compaction. We use LevelDB with dynamic level sizes. There’s not much that we configure there. It works pretty well for us, mostly off-the-shelf. There are some numbers we configure, but not too much. The big difference for our compaction is the fact that every time you write the file, you also upload it to S3. That’s the only thing that changes on the compaction size. Then also the commit protocol, when the compaction is done, we need to make sure it makes its way to the replication stream.
See more presentations with transcripts
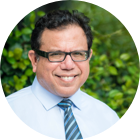
MMS • RSS
Posted on mongodb google news. Visit mongodb google news
It’s been a challenging year for the U.S. stock market. The S&P 500 (SNPINDEX: ^GSPC) has declined 8% from its high, and the technology-focused Nasdaq Composite (NASDAQINDEX: ^IXIC) has fallen 14%. However, both indexes have recovered from every past decline, so the recent losses create a no-brainer buying opportunity, especially for Nasdaq-listed technology stocks.
With that in mind, investors can purchase one share each of Shopify (NASDAQ: SHOP) and MongoDB (NASDAQ: MDB) for less than $300. Here’s why both Nasdaq stocks are worth owning.
Shopify develops commerce software that helps merchants run their businesses across physical and digital channels. It also offers supplemental services for marketing, logistics, and cross-border commerce, as well as financial services for payments, billing, and taxes. The International Data Corp. recently ranked Shopify as a leader in digital commerce platforms for mid-market business ($100 million to $500 million in revenue).
The company’s merchants collectively account for more than 12% of retail e-commerce sales in the U.S. and 6% of retail e-commerce sales in Western Europe. That makes Shopify the second largest e-commerce company behind Amazon in those geographies, and puts the company in an enviable position because online retail sales are forecast to increase at 11% annually through 2030.
Shopify was recently ranked as a leader in business-to-business (B2B) commerce solutions by Forrester Research. “Shopify has strength in innovation, as evidenced by the rapid pace of delivering features for its core B2B audience: consumer goods brands selling wholesale to small retail partners.” That matters because the B2B e-commerce market is three times bigger and growing nearly twice as fast as retail e-commerce.
Shopify reported solid financial results in the fourth quarter. Revenue increased 31% to $2.8 billion, the second-straight acceleration, and non-GAAP earnings increased 29% to $0.44 per diluted share. The company also reported a 10 basis-point increase in take rate, signaling that merchants are relying more heavily on Shopify by adopting more adjacent services.
There are 55 Wall Street analysts following Shopify. The median stock price target is $135 per share, which implies 42% upside from the current share price of $95.
Earnings are expected to increase 24% in 2025, which makes the current valuation of 79 times earnings look expensive. But Shopify beat the consensus estimate by an average of 16% over the last four quarters, and I think it will continue to top expectations. With the stock 26% off its high, patient investors should feel comfortable buying today.
Business data usually flows from transactional to operational to analytical systems. For example, e-commerce transactions could inform operational data stored in a customer relationship management system, which itself could be queried by an analytical system. Databases that support all three workloads are called translytical platforms, and Forrester Research recently ranked MongoDB as a leader in the space.
MongoDB was recently ranked as a leader in cloud database management systems by consultancy Gartner. The report highlighted strength in transaction processing, analytical capabilities, and flexibility in supporting complex applications. Use cases range from content management and commerce to mobile games and artificial intelligence. MongoDB also ranked as the fifth-most-popular database (out of 35) in a recent survey of over 50,000 developers.
MongoDB reported solid financial results for the fourth quarter of fiscal 2025, which ended in January. Customers increased 14% to 54,500, and the number of customers that spend at least $100,000 annually climbed about 17%. In turn, revenue rose 20% to $548 million, a slight deceleration from 22% in the previous quarter, and non-GAAP net income increased 49% to $1.28 per share.
There are 37 Wall Street analysts following MongoDB. The median target price is $300 per share, implying 73% upside from the current share price of $173.
The company gave disappointing guidance that calls for earnings to decline 30% in fiscal 2026, which ends in January. That caused the stock to plunge. But its current valuation of 65 times forward earnings is the cheapest in company history. Investors should feel comfortable buying a share (or a few more) today.
Ever feel like you missed the boat in buying the most successful stocks? Then you’ll want to hear this.
On rare occasions, our expert team of analysts issues a “Double Down” stock recommendation for companies that they think are about to pop. If you’re worried you’ve already missed your chance to invest, now is the best time to buy before it’s too late. And the numbers speak for themselves:
-
Nvidia: if you invested $1,000 when we doubled down in 2009, you’d have $285,647!*
-
Apple: if you invested $1,000 when we doubled down in 2008, you’d have $42,315!*
-
Netflix: if you invested $1,000 when we doubled down in 2004, you’d have $500,667!*
Right now, we’re issuing “Double Down” alerts for three incredible companies, and there may not be another chance like this anytime soon.
*Stock Advisor returns as of April 1, 2025
John Mackey, former CEO of Whole Foods Market, an Amazon subsidiary, is a member of The Motley Fool’s board of directors. Trevor Jennewine has positions in Amazon and Shopify. The Motley Fool has positions in and recommends Amazon, MongoDB, and Shopify. The Motley Fool recommends Gartner. The Motley Fool has a disclosure policy.
2 No-Brainer Nasdaq Stocks to Buy With $300 in April Before They Soar was originally published by The Motley Fool
Article originally posted on mongodb google news. Visit mongodb google news
2 No-Brainer Nasdaq Stocks to Buy With $300 in April Before They Soar | The Motley Fool
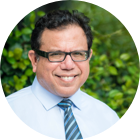
MMS • RSS
Posted on mongodb google news. Visit mongodb google news
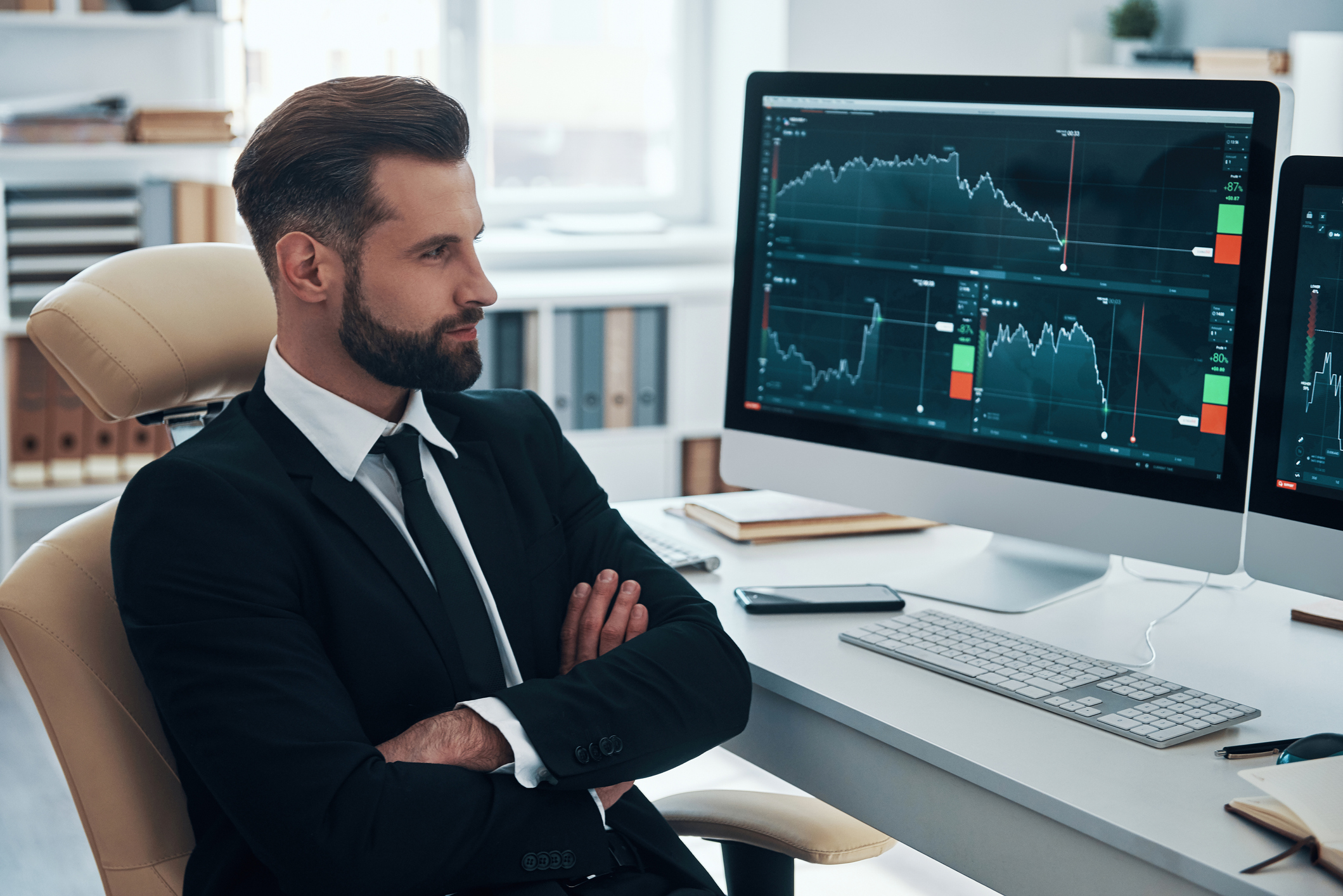
It’s been a challenging year for the U.S. stock market. The S&P 500 (^GSPC 0.67%) has declined 8% from its high, and the technology-focused Nasdaq Composite (^IXIC 0.87%) has fallen 14%. However, both indexes have recovered from every past decline, so the recent losses create a no-brainer buying opportunity, especially for Nasdaq-listed technology stocks.
With that in mind, investors can purchase one share each of Shopify (SHOP 3.00%) and MongoDB (MDB 2.13%) for less than $300. Here’s why both Nasdaq stocks are worth owning.
Shopify: 42% upside implied by Wall Street’s median target price
Shopify develops commerce software that helps merchants run their businesses across physical and digital channels. It also offers supplemental services for marketing, logistics, and cross-border commerce, as well as financial services for payments, billing, and taxes. The International Data Corp. recently ranked Shopify as a leader in digital commerce platforms for mid-market business ($100 million to $500 million in revenue).
The company’s merchants collectively account for more than 12% of retail e-commerce sales in the U.S. and 6% of retail e-commerce sales in Western Europe. That makes Shopify the second largest e-commerce company behind Amazon in those geographies, and puts the company in an enviable position because online retail sales are forecast to increase at 11% annually through 2030.
Shopify was recently ranked as a leader in business-to-business (B2B) commerce solutions by Forrester Research. “Shopify has strength in innovation, as evidenced by the rapid pace of delivering features for its core B2B audience: consumer goods brands selling wholesale to small retail partners.” That matters because the B2B e-commerce market is three times bigger and growing nearly twice as fast as retail e-commerce.
Shopify reported solid financial results in the fourth quarter. Revenue increased 31% to $2.8 billion, the second-straight acceleration, and non-GAAP earnings increased 29% to $0.44 per diluted share. The company also reported a 10 basis-point increase in take rate, signaling that merchants are relying more heavily on Shopify by adopting more adjacent services.
There are 55 Wall Street analysts following Shopify. The median stock price target is $135 per share, which implies 42% upside from the current share price of $95.
Earnings are expected to increase 24% in 2025, which makes the current valuation of 79 times earnings look expensive. But Shopify beat the consensus estimate by an average of 16% over the last four quarters, and I think it will continue to top expectations. With the stock 26% off its high, patient investors should feel comfortable buying today.
MongoDB: 73% upside implied by Wall Street’s median target price
Business data usually flows from transactional to operational to analytical systems. For example, e-commerce transactions could inform operational data stored in a customer relationship management system, which itself could be queried by an analytical system. Databases that support all three workloads are called translytical platforms, and Forrester Research recently ranked MongoDB as a leader in the space.
MongoDB was recently ranked as a leader in cloud database management systems by consultancy Gartner. The report highlighted strength in transaction processing, analytical capabilities, and flexibility in supporting complex applications. Use cases range from content management and commerce to mobile games and artificial intelligence. MongoDB also ranked as the fifth-most-popular database (out of 35) in a recent survey of over 50,000 developers.
MongoDB reported solid financial results for the fourth quarter of fiscal 2025, which ended in January. Customers increased 14% to 54,500, and the number of customers that spend at least $100,000 annually climbed about 17%. In turn, revenue rose 20% to $548 million, a slight deceleration from 22% in the previous quarter, and non-GAAP net income increased 49% to $1.28 per share.
There are 37 Wall Street analysts following MongoDB. The median target price is $300 per share, implying 73% upside from the current share price of $173.
The company gave disappointing guidance that calls for earnings to decline 30% in fiscal 2026, which ends in January. That caused the stock to plunge. But its current valuation of 65 times forward earnings is the cheapest in company history. Investors should feel comfortable buying a share (or a few more) today.
John Mackey, former CEO of Whole Foods Market, an Amazon subsidiary, is a member of The Motley Fool’s board of directors. Trevor Jennewine has positions in Amazon and Shopify. The Motley Fool has positions in and recommends Amazon, MongoDB, and Shopify. The Motley Fool recommends Gartner. The Motley Fool has a disclosure policy.
Article originally posted on mongodb google news. Visit mongodb google news
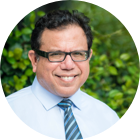
MMS • RSS
Posted on mongodb google news. Visit mongodb google news
MongoDB, Inc. (NASDAQ:MDB – Get Free Report) saw some unusual options trading on Wednesday. Traders purchased 23,831 put options on the stock. This is an increase of approximately 2,157% compared to the average volume of 1,056 put options.
Analyst Ratings Changes
MDB has been the topic of a number of analyst reports. JMP Securities reissued a “market outperform” rating and set a $380.00 price objective on shares of MongoDB in a report on Wednesday, December 11th. Wells Fargo & Company cut MongoDB from an “overweight” rating to an “equal weight” rating and cut their price objective for the stock from $365.00 to $225.00 in a research report on Thursday, March 6th. Mizuho raised their target price on MongoDB from $275.00 to $320.00 and gave the company a “neutral” rating in a report on Tuesday, December 10th. Daiwa Capital Markets assumed coverage on MongoDB in a report on Tuesday. They set an “outperform” rating and a $202.00 price target for the company. Finally, DA Davidson upped their target price on shares of MongoDB from $340.00 to $405.00 and gave the company a “buy” rating in a research note on Tuesday, December 10th. Seven analysts have rated the stock with a hold rating and twenty-four have issued a buy rating to the company. According to data from MarketBeat, the company currently has an average rating of “Moderate Buy” and a consensus price target of $312.84.
Insider Buying and Selling at MongoDB
In other news, Director Dwight A. Merriman sold 3,000 shares of the stock in a transaction on Monday, March 3rd. The stock was sold at an average price of $270.63, for a total value of $811,890.00. Following the completion of the sale, the director now owns 1,109,006 shares in the company, valued at $300,130,293.78. This represents a 0.27 % decrease in their ownership of the stock. The transaction was disclosed in a document filed with the Securities & Exchange Commission, which is available at this link. Also, CEO Dev Ittycheria sold 8,335 shares of the company’s stock in a transaction on Tuesday, January 28th. The shares were sold at an average price of $279.99, for a total value of $2,333,716.65. Following the transaction, the chief executive officer now directly owns 217,294 shares in the company, valued at $60,840,147.06. The trade was a 3.69 % decrease in their ownership of the stock. The disclosure for this sale can be found here. Over the last 90 days, insiders have sold 35,857 shares of company stock valued at $9,613,306. Corporate insiders own 3.60% of the company’s stock.
Institutional Investors Weigh In On MongoDB
Several hedge funds and other institutional investors have recently added to or reduced their stakes in the business. Norges Bank acquired a new position in shares of MongoDB during the 4th quarter worth about $189,584,000. Marshall Wace LLP bought a new stake in MongoDB during the fourth quarter worth about $110,356,000. Raymond James Financial Inc. acquired a new position in MongoDB during the fourth quarter valued at approximately $90,478,000. D1 Capital Partners L.P. bought a new position in MongoDB in the fourth quarter valued at approximately $76,129,000. Finally, Amundi increased its position in shares of MongoDB by 86.2% during the 4th quarter. Amundi now owns 693,740 shares of the company’s stock worth $172,519,000 after purchasing an additional 321,186 shares during the last quarter. 89.29% of the stock is currently owned by institutional investors and hedge funds.
MongoDB Price Performance
MDB stock opened at $180.19 on Thursday. The stock has a market capitalization of $14.63 billion, a P/E ratio of -65.76 and a beta of 1.30. MongoDB has a fifty-two week low of $170.66 and a fifty-two week high of $387.19. The business has a 50-day moving average of $240.77 and a 200-day moving average of $263.72.
MongoDB (NASDAQ:MDB – Get Free Report) last released its quarterly earnings results on Wednesday, March 5th. The company reported $0.19 earnings per share for the quarter, missing analysts’ consensus estimates of $0.64 by ($0.45). MongoDB had a negative return on equity of 12.22% and a negative net margin of 10.46%. The firm had revenue of $548.40 million for the quarter, compared to analyst estimates of $519.65 million. During the same period last year, the company earned $0.86 EPS. Equities analysts expect that MongoDB will post -1.78 EPS for the current year.
About MongoDB
MongoDB, Inc, together with its subsidiaries, provides general purpose database platform worldwide. The company provides MongoDB Atlas, a hosted multi-cloud database-as-a-service solution; MongoDB Enterprise Advanced, a commercial database server for enterprise customers to run in the cloud, on-premises, or in a hybrid environment; and Community Server, a free-to-download version of its database, which includes the functionality that developers need to get started with MongoDB.
Recommended Stories
Receive News & Ratings for MongoDB Daily – Enter your email address below to receive a concise daily summary of the latest news and analysts’ ratings for MongoDB and related companies with MarketBeat.com’s FREE daily email newsletter.
Article originally posted on mongodb google news. Visit mongodb google news
Powering India’s Data Future: A Candid Conversation with Pranoti Deshmukh of IndiaDataHub
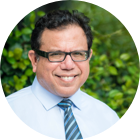
MMS • RSS
Posted on mongodb google news. Visit mongodb google news
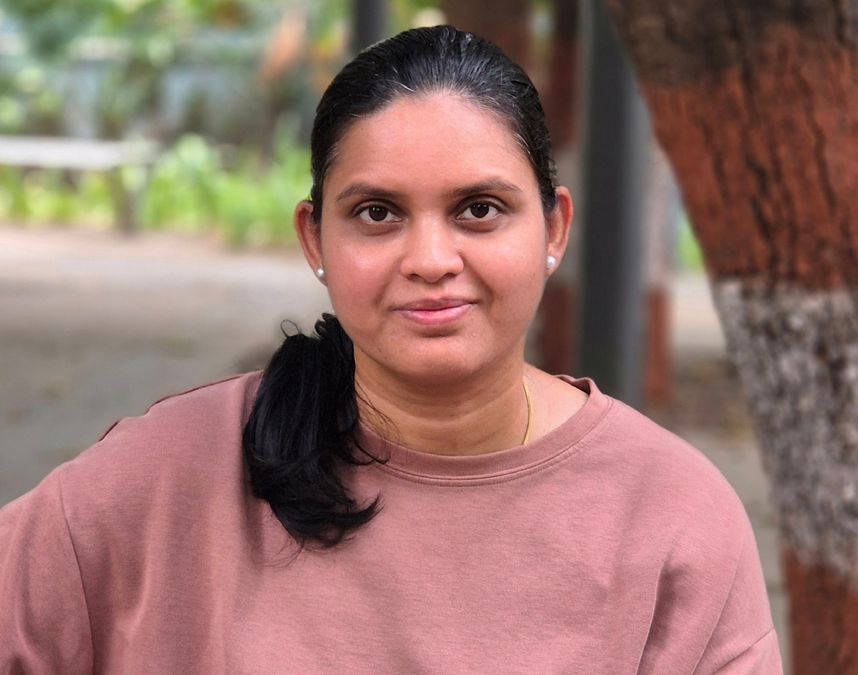
In the ever-evolving world of data and analytics, IndiaDataHub is making powerful strides to redefine how data is accessed, analyzed, and utilized across industries. Known for its robust platform housing tens of thousands of time-series datasets, the company is on a mission to make data-driven decision-making more intuitive and effective.
In this exclusive conversation, Faiz Askari, Founder of SMEStreet, sits down with Pranoti Deshmukh, Co-Founder of IndiaDataHub, to discuss the company’s evolving role in India’s data ecosystem, its strategic collaboration with MongoDB, and the game-changing impact of AI in shaping data accessibility and innovation.
Exclusive Interview:
Faiz Askari (SMEStreet): Pranoti, could you start by telling us more about the core offerings of IndiaDataHub?
Pranoti Deshmukh: Absolutely. IndiaDataHub is a comprehensive data platform designed to empower businesses, investors, and policymakers. We provide access to an extensive collection of time-series datasets covering a wide range of sectors like banking, infrastructure, energy, agriculture, climate, and more. Our data is granular—available at national, state, and even district levels. This allows for tailored analysis supporting everything from financial forecasting to strategic decision-making.
Faiz Askari: Your recent partnership with MongoDB has generated a lot of buzz. What are the key objectives behind this collaboration?
Pranoti Deshmukh: Our main focus with MongoDB has been improving the data discovery and search experience on our platform. As our catalog grew, finding the right data became more complex. So, we implemented MongoDB’s Vector Search—a cutting-edge AI-driven technology—to help users find semantically relevant datasets quickly. We’ve also applied Retrieval-Augmented Generation (RAG) to refine this process further, making it possible to surface highly relevant indicators, like the top five among 1,000 inflation metrics, with up to 95% accuracy.
Analytics and Visualization
Faiz: That’s impressive. How does this technical backbone support your analytics and visualization goals?
Pranoti: We’ve streamlined our backend operations using MongoDB Atlas, ensuring our data pipelines are both scalable and cost-effective. It’s especially helpful when processing unstructured data like PDFs of mutual fund portfolios. We’ve also significantly enhanced dashboard performance—reducing latency from 2 seconds to just under 200 milliseconds. All of this contributes to a much smoother and faster user experience.
Faiz: Which industries do you think will benefit the most from these advancements?
Pranoti: Definitely the investment and financial services sectors. Asset managers, banks, mutual funds—they all need real-time, actionable insights. Beyond that, corporates across industries can leverage our data for strategic planning, market expansion, and benchmarking. For instance, a marketing team could analyze consumer behavior trends to launch more targeted campaigns.
AI-Powered Data Management
Faiz Askari: What unique challenges did you face in the context of AI-powered data management?
Pranoti Deshmukh: Two major ones: handling multi-dimensional, often unstructured data, and improving discoverability of that data. Traditional databases struggle with the former. MongoDB’s flexible schema design and AI search tools helped us overcome both. We’re also working with them to bring AI into our data quality workflows—automating issue detection and streamlining data sourcing.
Faiz Askari: Could you share any early success stories or promising use cases from this partnership?
Pranoti Deshmukh: One great example is our mutual fund dataset project. We’re building analytics at both aggregate and instrument levels—something investors find invaluable. Curating this from unstructured PDFs is tough, but MongoDB helped us automate much of the process. With our new vector store, users can pinpoint relevant data without digging manually. In the future, we aim to introduce sentiment indices and a full-fledged research copilot, powered by MongoDB’s AI suite.
Faiz Askari: Exciting times ahead! Thanks for sharing these insights, Pranoti. Any closing thoughts for the SMEStreet readership?
Pranoti Deshmukh: I’d just like to say that data is no longer a back-office function—it’s central to decision-making. Whether you’re an SME, a large corporation, or a policymaker, leveraging structured and unstructured data effectively can be your biggest competitive edge. We’re excited to be part of that journey.
Article originally posted on mongodb google news. Visit mongodb google news
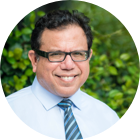
MMS • RSS
Posted on mongodb google news. Visit mongodb google news
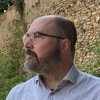
Benjamin Lorenz has been a pivotal member of MongoDB since 2016, contributing significantly to the growth of the Central European Sales region. With a depth of experience in strategic customer projects, Benjamin engages with decision-makers to thoroughly understand their needs and craft solutions anchored on the solid and powerful developer data platform MongoDB. As the Industry Solutions Principal for Telco & Media, Benjamin plays a crucial role in supporting global customers in these sectors through their digital transformation journeys. He is dedicated to helping organizations establish innovative, data-driven revenue streams.
Article originally posted on mongodb google news. Visit mongodb google news
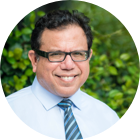
MMS • RSS
Posted on mongodb google news. Visit mongodb google news
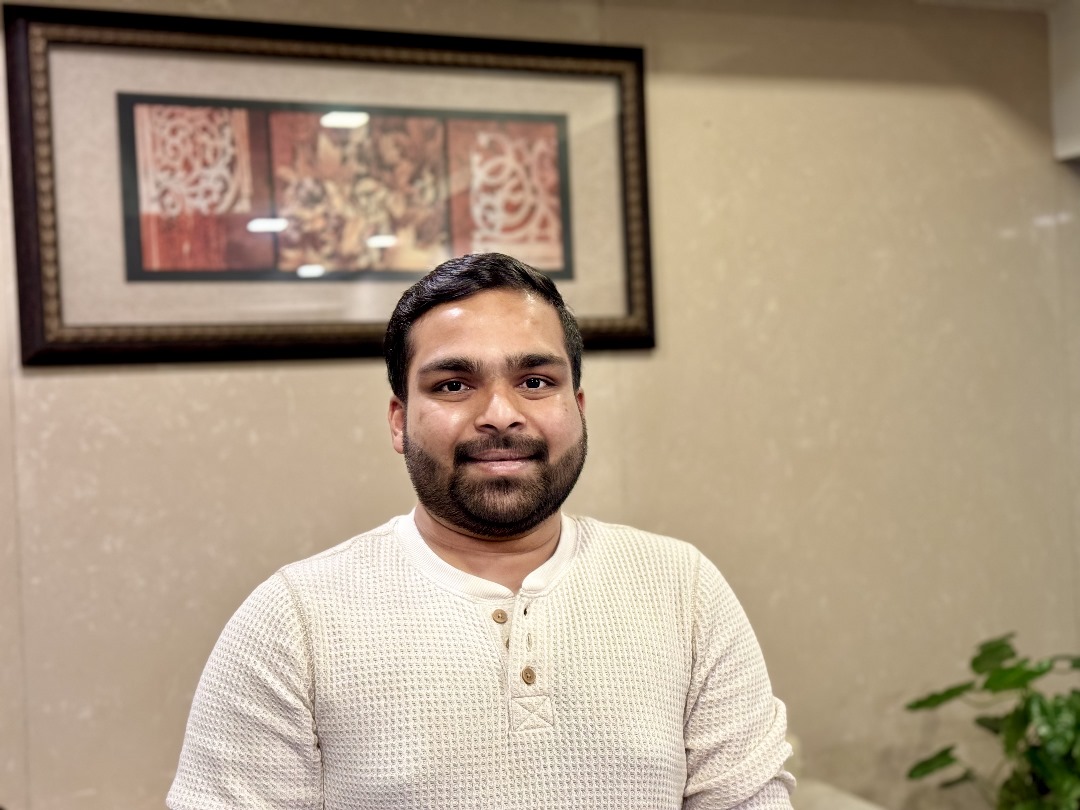
By Tunde Ibrahim
Rohith Varma Vegesna has been officially immortalized as the 222nd Certified Global Tech Hero, a prestigious recognition celebrating his exceptional contributions to software development, cloud architecture, and enterprise solutions. With over seven years of experience, Rohith has consistently delivered cutting-edge solutions, driving digital transformation across critical infrastructure sectors, particularly in fuel retail technology and automation.
As a Software Engineer II at 7-Eleven, Rohith has spearheaded groundbreaking innovations in transactional systems, cloud integrations, and IoT-driven automation. His architectural redesign of the fuel transaction system, leveraging Java Spring, AWS microservices, and MongoDB optimizations, has significantly improved transaction reliability and system efficiency across thousands of store locations. His methodology for optimizing MongoDB queries resulted in a 40% reduction in read loads during peak transactions, enhancing both performance and cost-effectiveness.
One of Rohith’s most impactful achievements is his pioneering work in EMV-based fuel dispenser communication. His development of secure, byte-level messaging protocols between dispensers and fuel controllers using Java and AWS Lambda has set a new industry standard in transaction security and efficiency. Additionally, his expertise in software-driven automation led to the creation of a Python-based simulator for fuel dispensers, a tool now widely adopted for testing and quality assurance.
Beyond implementation, Rohith is a thought leader in software engineering, contributing to industry research through several white papers, including Designing Software for Real-Time Pump Dispenser Data Streaming Using AWS Kinesis, Developing Encrypted Communication Protocols for Fuel Controllers, Software-Driven Automated Leak Detection in Fuel Storage Tanks, and Proactive Maintenance Alerts Based on Usage Patterns with AWS IoT. These publications have provided strategic insights into scalable, AI-enabled software architectures that enhance security, efficiency, and predictive analytics in fuel retail technology.
Rohith’s technical acumen extends beyond development; he has led CI/CD pipeline deployments using Jenkins and GitLab, integrated cloud-based observability with Log4j and Splunk, and built developer-friendly interfaces such as a React-based fuel controller configuration portal. His ability to merge technical depth with scalable system design has had a profound impact on both business outcomes and customer experiences.
This recognition as a Certified Global Tech Hero underscores Rohith’s unwavering commitment to innovation, problem-solving, and industry leadership. His influence on software-driven automation, cloud-native applications, and secure transaction systems solidifies his status as a visionary technologist shaping the future of enterprise solutions.
As Qazeem Oladejo, the founder of The Connected Awards, once remarked about another tech luminary, Rohith Varma Vegesna saying “True innovation is measured by the depth of impact, not just the breadth of adoption. The most outstanding professionals are those who solve real-world problems while pioneering new technological frontiers.”
This sentiment perfectly encapsulates Rohith Varma Vegesna’s journey and the legacy he continues to build in the global tech ecosystem.
Article originally posted on mongodb google news. Visit mongodb google news
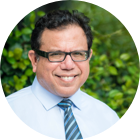
MMS • Robert Krzaczynski
Article originally posted on InfoQ. Visit InfoQ

Amazon has announced an expansion of its generative AI capabilities with the introduction of nova.amazon.com, a platform designed to give developers easier access to its foundation models. This includes the newly unveiled Amazon Nova Act, an AI model specifically trained to execute actions within web browsers.
Nova Act is available as an early research preview through the Amazon Nova Act SDK. It allows developers to build AI agents capable of performing complex tasks by breaking them into smaller, more manageable steps. The SDK supports additional customization through Python code, enabling developers to interleave tests, breakpoints, assertions, and thread pooling for parallelization.
In the words of Shubham Katiyar, a director of Generative Artificial Intelligence at Amazon:
This represents a fundamental shift in how AI agents operate in digital environments, enabling reliable execution of complex web-based tasks from form submissions to calendar management with unprecedented accuracy.
Amazon first introduced its Nova foundation models at re:Invent 2024, integrating them into AWS services and Amazon Bedrock. The Nova family includes three text generation models—Nova Micro, Lite, and Pro—along with Nova Canvas for image generation and Nova Reel for video creation. Now, with nova.amazon.com, developers can explore these models and experiment with their capabilities.
The launch of Nova Act comes with certain disclosures. Amazon emphasizes that the tool remains experimental, and users are responsible for monitoring its actions. Nova Act may make mistakes, and interactions—including prompts and screenshots—are collected for improvement purposes. Developers are advised not to share API keys or input sensitive information, as it could be captured in screenshots when the agent is active.
Reactions to the new models have been positive. Wesley Kurosawa, a business data analyst, shared his excitement about the platform, stating:
Absolutely incredible news from Amazon! With nova.amazon.com, we can now access cutting-edge AI models directly and experiment with frontier intelligence capabilities that were previously out of reach. This is an excellent tool for developers like us to quickly test ideas and then scale them through Amazon Bedrock. The ability to build web agents with the Nova Act SDK opens up entirely new possibilities for automation and assistance. Amazon has truly democratized access to advanced AI—can’t wait to start building with it!
However, some users have raised concerns about how Nova Act’s browsing capabilities might be perceived. One Reddit user reflected:
Very interesting, all these make me think that some websites might see it as web scraping techniques, as it might be too quick to be considered normal human activities. I’m sure these will be very interesting times. Where the border between web scraping and normal use will kind of overlap.
Looking ahead, Amazon plans to further refine its AI models, enhancing their accuracy and expanding their capabilities. The company is also exploring options for developers to create custom voices while maintaining a strong commitment to ethical and safety standards. In addition to advancements in audio and text, Amazon is investing in multimodal AI, including video, to enable more sophisticated and interactive AI-driven experiences.
U.S.-based users with an Amazon account can start exploring nova.amazon.com, where they can experiment with Nova models, generate images using Nova Canvas, and access the research preview of the Nova Act SDK.
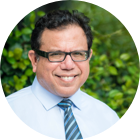
MMS • RSS
Posted on mongodb google news. Visit mongodb google news
By Seth Payne, Lead Product Manager at MongoDB
By Daniel Ernst, Search Engineering Lead at MongoDB
By Arjun Gurumurthy, Staff Engineer at MongoDB
By Archana Srinivasan, Sr. Technical Account Manager at AWS
By Rashmikant Vyas (RK), Sr. Technical Account Manager at AWS
By Sergio Ariel de la Campa, Sr. Technical Account Manager at AWS
![]() |
![]() |
MongoDB customers were seeking a solution to handle Atlas Search index rebuild in case of a problem with underlying hardware or scaling activities. This blog post discusses how MongoDB has enhanced the indexing capabilities of its Atlas Search service by leveraging Amazon S3. The approach allows MongoDB to reduce the time required to rebuild search indexes, achieving a 14x improvement compared to the previous method.
Background
MongoDB Atlas a comprehensive cloud database platform, was launched on Amazon Web Services (AWS) in 2016. Today’s service update includes global expansion, now available in 34 AWS regions worldwide. With AWS, MongoDB Atlas offers a user-friendly, scalable, and secure cloud platform for deploying, managing, and monitoring MongoDB databases.
One of the key features of MongoDB Atlas is its integrated search and vector search capabilities. This eliminates the need to run a separate search system alongside your database. Initially, MongoDB ran search and database on same hardware, causing contention and scaling limitations. In 2023, MongoDB introduced dedicated search nodes on Amazon EC2 Graviton to address this. The current challenge with MongoDB Atlas search deployments is that they require complete index rebuilding for each search process, causing operational overhead and longer deployment times.
Challenges with legacy approach
MongoDB Atlas (Full-Text) Search launched 5 years ago with Atlas Vector Search being added within the last couple of years. They have become popular among developers because of the native experience within MongoDB and the evolving query capabilities and enhancements. This launch helps MongoDB Atlas users to seamlessly leverage full-text and vector search capabilities within their transactional database systems. MongoDB’s Search capabilities eliminate the need for complex ETL to keep search and transaction data in sync. With this MongoDB now supports complex search workloads with high demands for queries per second (QPS), data ingestion, and low latency. Atlas Search and Vector Search are based on Apache Lucene. The initial architecture collocated the database process (mongod) and search process (mongot) on the same hardware, which had the potential to create workload contention and scaling challenges. While this collocated approach can still be used, MongoDB released dedicated search nodes in 2023. The new architecture allows users to isolate and independently scale their search (or vector search) processes from their database processes. With the dedicated search node approach, the search process (mongot) runs on its own hardware on Amazon EC2 Graviton. The search process communicates the search results to the database process (mongod) via the network. This method eliminates resource conflicts and allows efficient scaling for large-scale search applications handling high query loads, data ingestion, and storage. Leveraging dedicated search nodes is the optimal approach for any team running search and/or vector search processes in production.
When deploying or scaling search infrastructure, MongoDB Atlas clusters rebuild indices for each search process from source MongoDB collection. Creating search indexes this way is predictable and reliable, but it means reading the entire MongoDB source collection, which may take a long time. Therefore, implementing new search infrastructure is slow and burdens the MongoDB Atlas cluster’s read operations.
Solution overview
This blog post details new Atlas Search indexing features that leverage Amazon Simple Storage Service (Amazon S3). Atlas Search in this context refers to both the full-text and vector search capabilities. MongoDB has improved the indexing experience for users with large datasets, using Amazon S3 to enhance index rebuilding during deployments, scaling, and recovery. The system periodically backs up its Amazon S3 search indexes instead of constantly rebuilding them from the MongoDB source. Rebuilding an index on a fresh instance uses the recent Atlas Search file snapshot, rebuilding only since the last capture. This speeds up deployment and scaling while improving search availability, especially for large datasets. These benefits come with no changes to current configuration for the end users.
MongoDB Atlas Search, launched in 2019, and its recent Atlas Vector Search feature provide developers with native search capabilities within MongoDB. This eliminates the need for complex ETL processes to synchronize search and transactional data. The search solutions initially ran on the same hardware as the database process, causing resource contention and scaling limitations. MongoDB addressed these challenges in 2023 by introducing dedicated search nodes on Amazon EC2 Graviton hardware. However, a significant challenge remains: when deploying or scaling search infrastructure, MongoDB Atlas must rebuild indices for each search process by performing a complete read of the source collection. This creates operational burden and extends deployment times.
Architecture overview
The MongoDB Atlas Control Plane comprises services whose functionality includes handling search index management command, node configuration updates, retrieving index definitions, and provisioning mongot and mongod nodes. Atlas Search indexes are created by specifying a mongod collection as the data source, along with an index configuration. Whenever a customer creates or updates a search index, mongots retrieves its definition from MongoDB Atlas. It then builds the index and dynamically updates it to reflect changes in the collection’s documents.
These search indexes are built on Lucene, and comprise a set of immutable files on a disk. MongoDB Atlas Search takes an index snapshot which is essentially a set of the files corresponding to a valid queryable Lucene index. Figure 1 architecture allows for periodic and efficient uploading of search indexes to Amazon S3, and retrieval of this data when an index rebuild is required. If index files remain unchanged between snapshots, they don’t need to be re-uploaded, optimizing storage and transfer processes.
Figure 1: Architecture of Atlas Search using Amazon S3
The design ensures that index uploads and downloads are atomic and resilient to mongot restarts and transient errors. If a download fails, the system gracefully falls back to building the search index from scratch.
The upload index process involves retrieving Amazon S3 credentials, capturing the latest index snapshot, uploading the index in parallel, and storing index metadata in Amazon S3. For downloads, mongots retrieve credentials, query Amazon S3 for index metadata, and download files in parallel if a snapshot is available.
MongoDB uses GetFederationTokens to vend credentials scoped per customer, ensuring that a customer’s data remains inaccessible to others. The testing strategy is rigorous, involving unit, integration, and end-to-end tests. MongoDB even employed LocalStack to create fault-injectable Amazon S3 clients, simulating various exceptions and errors to verify mongot behavior under different conditions.
Amazon S3 plays a crucial role in enhancing the speed and efficiency of Atlas Search’s index rebuilding process. Amazon S3 provides a reliable and scalable storage solution for periodic snapshots of search index states. This enables quick retrieval of the latest index data when rebuilding is necessary. By using this approach, we get the latest index snapshot from Amazon S3, which speeds up rebuilding compared to using the MongoDB source.
Efficient storage management involves the asynchronous deletion of older snapshots once new ones are uploaded. MongoDB leverages Amazon S3 Lifecycle policies to automatically delete orphaned files, ensuring optimal use of storage resources and maintaining a clean, up-to-date snapshot repository.
The design also incorporates other critical safeguards: limiting resource utilization during S3 access preserves core search functionality, and distributed uploads across multiple search hosts prevent system overload.
Benefits
Traditional MongoDB index recreation during scaling or node rebuilds takes hours, posing scalability challenges due to its time-consuming nature. With its new Amazon S3 powered feature, MongoDB Atlas Search now offers customers faster search index rebuild times. MongoDB’s internal tests have shown this time is reduced by 14X with Amazon S3. This new feature is transparent to MongoDB users, requiring no action from customers.
Amazon S3 based decoupling of the indexing layer allows for a scalable and evolving design. Previously, preventing concurrent index uploads required implementing concurrency control mechanisms. However, with the recently announced conditional writes support in S3, this process is simplified – the native S3 feature can automatically prevent concurrent uploads.
Conclusion
MongoDB Atlas has improved its Search capabilities by implementing an innovative Amazon S3-based approach to index rebuilding. The new method delivers performance and efficiency gains. This new method lets us periodically save copies of search indexes to Amazon S3, which speeds up index rebuilding when deploying, scaling, or recovering. MongoDB’s new method of rebuilding indexes, using Amazon S3’s latest Atlas Search file-level snapshot and starting from the last capture point, is 14 times faster than the old method.
MongoDB customers using Atlas Search dedicated nodes experience faster scaling and node rebuilding, decreasing index reconstruction time from hours to minutes. The Amazon S3 based approach serves as a foundation for future features.
MongoDB – AWS Partner Spotlight
MongoDB is an AWS Competency Partner. Their modern, general purpose database platform, is designed to unleash the power of software and data for developers and the applications they build.
To learn more, refer to these getting started guides for Atlas Search or Atlas Vector Search for step-by-step instructions.
Contact MongoDB | Partner Overview | AWS Marketplace
Article originally posted on mongodb google news. Visit mongodb google news
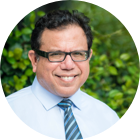
MMS • RSS
Posted on mongodb google news. Visit mongodb google news
Shares of MongoDB Inc (MDB, Financial) surged 2.87% in mid-day trading on Apr 2. The stock reached an intraday high of $182.00, before settling at $181.68, up from its previous close of $176.61. This places MDB 53.08% below its 52-week high of $387.19 and 6.45% above its 52-week low of $170.66. Trading volume was 1,267,571 shares, 55.0% of the average daily volume of 2,302,646.
Wall Street Analysts Forecast
Based on the one-year price targets offered by 34 analysts, the average target price for MongoDB Inc (MDB, Financial) is $304.56 with a high estimate of $520.00 and a low estimate of $180.00. The average target implies an upside of 67.64% from the current price of $181.68. More detailed estimate data can be found on the MongoDB Inc (MDB) Forecast page.
Based on the consensus recommendation from 38 brokerage firms, MongoDB Inc’s (MDB, Financial) average brokerage recommendation is currently 2.0, indicating “Outperform” status. The rating scale ranges from 1 to 5, where 1 signifies Strong Buy, and 5 denotes Sell.
Based on GuruFocus estimates, the estimated GF Value for MongoDB Inc (MDB, Financial) in one year is $432.97, suggesting a upside of 138.32% from the current price of $181.675. GF Value is GuruFocus’ estimate of the fair value that the stock should be traded at. It is calculated based on the historical multiples the stock has traded at previously, as well as past business growth and the future estimates of the business’ performance. More detailed data can be found on the MongoDB Inc (MDB) Summary page.
This article, generated by GuruFocus, is designed to provide general insights and is not tailored financial advice. Our commentary is rooted in historical data and analyst projections, utilizing an impartial methodology, and is not intended to serve as specific investment guidance. It does not formulate a recommendation to purchase or divest any stock and does not consider individual investment objectives or financial circumstances. Our objective is to deliver long-term, fundamental data-driven analysis. Be aware that our analysis might not incorporate the most recent, price-sensitive company announcements or qualitative information. GuruFocus holds no position in the stocks mentioned herein.
Article originally posted on mongodb google news. Visit mongodb google news