Category: Uncategorized
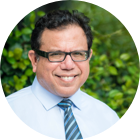
MMS • RSS
Posted on mongodb google news. Visit mongodb google news
MongoDB (NASDAQ:MDB – Get Free Report) was downgraded by research analysts at Loop Capital from a “buy” rating to a “hold” rating in a research report issued to clients and investors on Tuesday, MarketBeat reports. They presently have a $190.00 price target on the stock, down from their prior price target of $350.00. Loop Capital’s target price would suggest a potential upside of 0.52% from the stock’s previous close.
A number of other brokerages have also commented on MDB. Macquarie decreased their price target on shares of MongoDB from $300.00 to $215.00 and set a “neutral” rating on the stock in a report on Friday, March 7th. China Renaissance assumed coverage on MongoDB in a research report on Tuesday, January 21st. They set a “buy” rating and a $351.00 price target on the stock. Truist Financial cut their price objective on MongoDB from $300.00 to $275.00 and set a “buy” rating for the company in a report on Monday, March 31st. Stifel Nicolaus decreased their price objective on MongoDB from $340.00 to $275.00 and set a “buy” rating on the stock in a report on Friday, April 11th. Finally, Wells Fargo & Company lowered MongoDB from an “overweight” rating to an “equal weight” rating and cut their target price for the stock from $365.00 to $225.00 in a research note on Thursday, March 6th. Nine equities research analysts have rated the stock with a hold rating, twenty-three have given a buy rating and one has issued a strong buy rating to the company’s stock. Based on data from MarketBeat, the company currently has an average rating of “Moderate Buy” and a consensus target price of $288.91.
Check Out Our Latest Research Report on MDB
MongoDB Trading Down 1.2%
MongoDB stock opened at $189.01 on Tuesday. MongoDB has a 1-year low of $140.78 and a 1-year high of $379.06. The firm has a market capitalization of $15.35 billion, a PE ratio of -68.98 and a beta of 1.49. The firm’s 50 day simple moving average is $174.77 and its 200 day simple moving average is $238.15.
MongoDB (NASDAQ:MDB – Get Free Report) last posted its quarterly earnings data on Wednesday, March 5th. The company reported $0.19 earnings per share (EPS) for the quarter, missing the consensus estimate of $0.64 by ($0.45). MongoDB had a negative net margin of 10.46% and a negative return on equity of 12.22%. The business had revenue of $548.40 million for the quarter, compared to the consensus estimate of $519.65 million. During the same period in the prior year, the business posted $0.86 EPS. Research analysts forecast that MongoDB will post -1.78 earnings per share for the current year.
Insider Buying and Selling
In related news, CFO Srdjan Tanjga sold 525 shares of the stock in a transaction dated Wednesday, April 2nd. The shares were sold at an average price of $173.26, for a total value of $90,961.50. Following the completion of the transaction, the chief financial officer now directly owns 6,406 shares in the company, valued at $1,109,903.56. This trade represents a 7.57% decrease in their position. The sale was disclosed in a legal filing with the Securities & Exchange Commission, which is available through this hyperlink. Also, insider Cedric Pech sold 1,690 shares of the company’s stock in a transaction dated Wednesday, April 2nd. The stock was sold at an average price of $173.26, for a total transaction of $292,809.40. Following the completion of the sale, the insider now owns 57,634 shares in the company, valued at approximately $9,985,666.84. This trade represents a 2.85% decrease in their position. The disclosure for this sale can be found here. Insiders sold a total of 33,538 shares of company stock valued at $6,889,905 over the last three months. Corporate insiders own 3.60% of the company’s stock.
Institutional Inflows and Outflows
A number of large investors have recently added to or reduced their stakes in MDB. Strategic Investment Solutions Inc. IL purchased a new position in shares of MongoDB in the 4th quarter worth about $29,000. Cloud Capital Management LLC purchased a new position in MongoDB in the first quarter worth about $25,000. NCP Inc. bought a new position in shares of MongoDB in the fourth quarter worth approximately $35,000. Hollencrest Capital Management purchased a new stake in shares of MongoDB during the first quarter valued at approximately $26,000. Finally, Cullen Frost Bankers Inc. lifted its position in MongoDB by 315.8% in the 1st quarter. Cullen Frost Bankers Inc. now owns 158 shares of the company’s stock valued at $28,000 after acquiring an additional 120 shares in the last quarter. Hedge funds and other institutional investors own 89.29% of the company’s stock.
MongoDB Company Profile
MongoDB, Inc, together with its subsidiaries, provides general purpose database platform worldwide. The company provides MongoDB Atlas, a hosted multi-cloud database-as-a-service solution; MongoDB Enterprise Advanced, a commercial database server for enterprise customers to run in the cloud, on-premises, or in a hybrid environment; and Community Server, a free-to-download version of its database, which includes the functionality that developers need to get started with MongoDB.
See Also
This instant news alert was generated by narrative science technology and financial data from MarketBeat in order to provide readers with the fastest and most accurate reporting. This story was reviewed by MarketBeat’s editorial team prior to publication. Please send any questions or comments about this story to contact@marketbeat.com.
Before you consider MongoDB, you’ll want to hear this.
MarketBeat keeps track of Wall Street’s top-rated and best performing research analysts and the stocks they recommend to their clients on a daily basis. MarketBeat has identified the five stocks that top analysts are quietly whispering to their clients to buy now before the broader market catches on… and MongoDB wasn’t on the list.
While MongoDB currently has a Moderate Buy rating among analysts, top-rated analysts believe these five stocks are better buys.
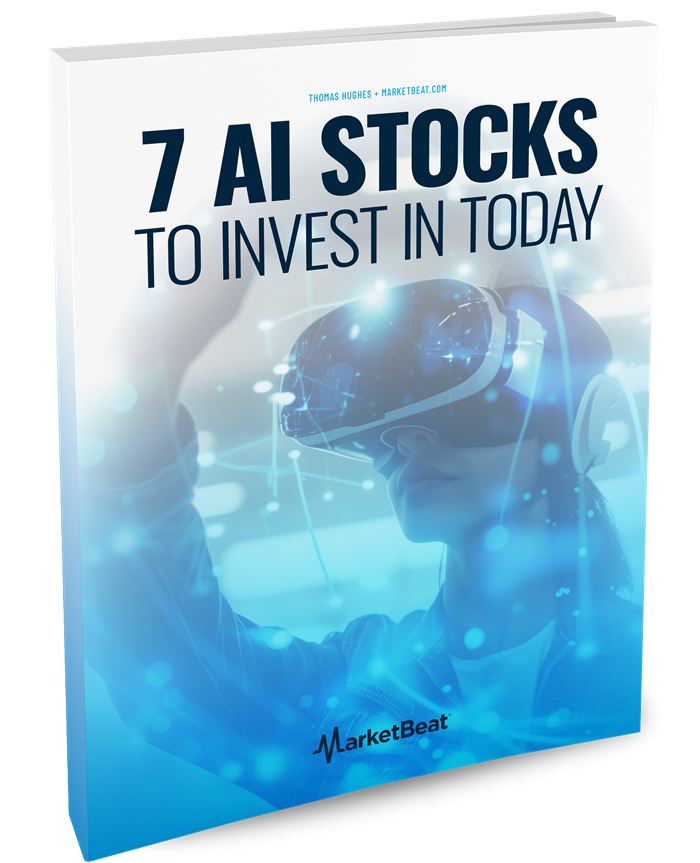
Discover the top 7 AI stocks to invest in right now. This exclusive report highlights the companies leading the AI revolution and shaping the future of technology in 2025.
Article originally posted on mongodb google news. Visit mongodb google news
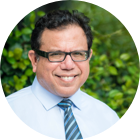
MMS • Ben Linders
Article originally posted on InfoQ. Visit InfoQ
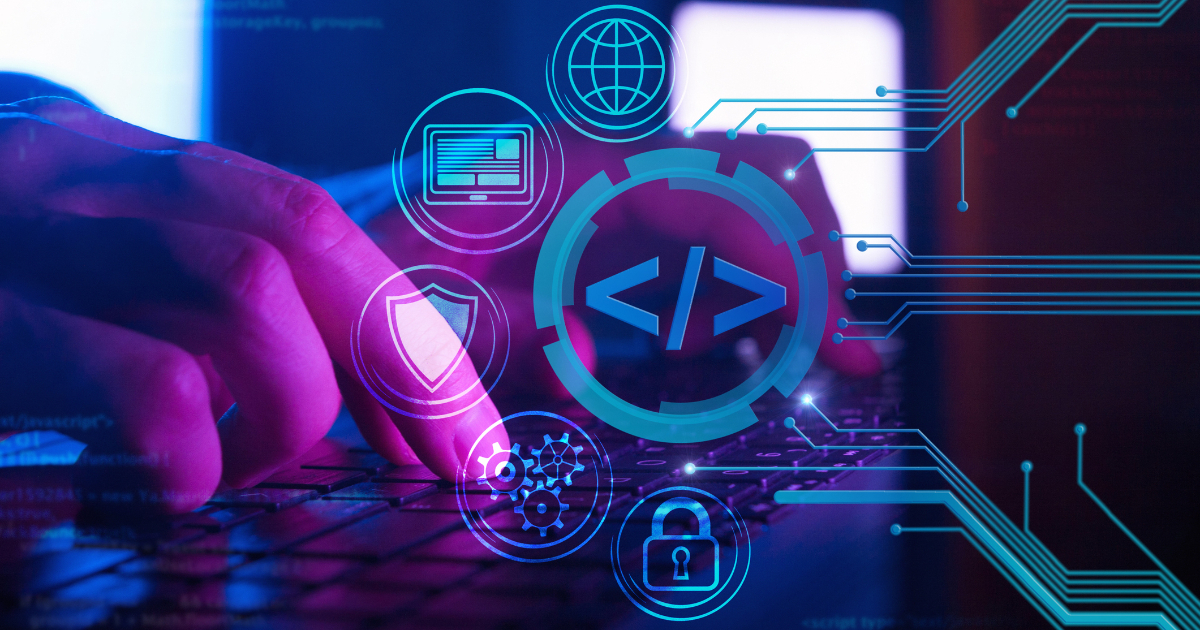
Web accessibility ensures content is usable by people with disabilities. According to Joanna Falkowska it can give a competitive edge, improve SEO, and support basic human rights. She emphasizes using WCAG standard and making accessibility a shared team responsibility from the start of development, to prevent costly fixes later in the process.
Joanna Falkowska spoke about creating accessible websites at DEV: Challenge Accepted.
Web accessibility is about making web content available to users with disabilities. Falkowska suggested using the Web Content Accessibility Guidelines to improve accessibility and create an inclusive website.
Web accessibility should be considered a basic human right, said Falkowska. We should care about website accessibility because most of us either are affected by disability directly or have family members, friends or colleagues who have it, she added. Product accessibility can give you a competitive edge over other businesses.
Some companies consider accessibility as a natural consequence of their DEI policy and a basic human right. There are also those who care about accessibility primarily due to SEO results, as search engines prioritise accessible sites in their search results, Falkowska said.
Accessibility may be important for companies due to legislation. Many countries and institutions implement dedicated digital accessibility laws that concern specific institutions and/or businesses, Falkowska mentioned:
One of the most recent ones is the European Accessibility Act. It is an EU directive that will come into force in July 2025, addressing a wide range of services, among others: e-commerce, banking and transport.
The Web Content Accessibility Guidelines is a standard that is recognized worldwide. Any legal act that requires conformance with accessibility rules quotes WCAG as its reference point, Falkowska said. It is available on-line and completely free. There is also a thorough list of international accessibility policies available. Falkowska invited people to read it and adapt their web content according to its success criteria.
Falkowska suggested that team members should be fluent in accessibility standard, at least in relation to the success criteria that refer to their role:
For example, it is the role of the designer to address all of the colour contrast issues there may be but also, if the authoring team has some flexibility, they should be aware of the colour contrast rules they need to follow in order for the final content to be accessible.
Accessibility issues, just as any other type of bugs, tend to be more expensive to fix in the later stages of development, Falkowska said. Therefore, development teams who want to achieve and maintain an accessibility standard, need to make accessibility part of the earliest stages of development.
Falkowska suggested making it part of the process to discuss and add precise accessibility acceptance criteria to the ticket description while grooming new stories:
Many teams do not do it, and as a result, accessibility gets added either after the ticket is rejected in the testing phase or even later – when the accessibility audit is completed.
Accessibility is undeniably a team sport. If we want to integrate it with the development process, we need to address it at all of its steps, Falkowska concluded.
InfoQ interviewed Joanna Falkowska about developing accessible websites.
InfoQ: What’s your advice to developers who want to start with accessibility?
Joanna Falkowska: If you are new to the subject of accessibility, I would recommend taking the time to read and understand all of the success criteria WCAG provides. It may look overwhelming at first but you can learn them in chunks, according to subsequent sections (guidelines) or based on conformance levels (from A up to AAA).
The second thing would be learning how to use assistive technology, especially screen readers, but also simple things like navigating with a keyboard instead of a mouse.
Finally, once you learn all of that, the first thing to do is… make friends with and, if needed, educate the design team. Many accessibility issues would not pop up at the development stage if the designs were following WCAG standards right from the start.
InfoQ: What can be done to make accessibility an integral part of your development process?
Falkowska: Make sure that accessibility is not simply “outsourced” to an accessibility team from a different department or an external auditing company.
The accessibility team should be there to support you only in the issues that go beyond the basic scope, e.g. you are struggling to decide what order to implement for keyboard navigation and need someone to share the most convenient solution.
The auditing company should come only in the last stage of the development. They are there to certify your accessibility level rather than teaching you what should have been done at the beginning of the development. We all know that changing the designs while the app is running costs much more than designing the wireframes with accessibility in mind.
Suppose we want to integrate accessibility into the development process. In that case, the product owner should raise the topic of accessibility repeatedly: right at the design stage, before the development starts, and up until the testing phase.
If your team members do not know what to consider while developing an accessible solution, you may want to request an accessibility specialist to join your team and help you draft requirements with WCAG in mind, teaching everyone what to consider during grooming sessions.
Developing accessible solutions might bring more clients to your website. Adding accessibility skills to your personal portfolio will make you a more competitive employee on the IT market. Companies that are legally obliged to deliver accessible solutions will quickly learn that it is more cost-effective to employ team members that know what role they play in delivering accessible apps.
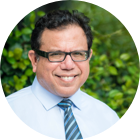
MMS • RSS
Posted on mongodb google news. Visit mongodb google news
MongoDB (NASDAQ:MDB – Get Free Report)‘s stock had its “outperform” rating restated by equities research analysts at Royal Bank of Canada in a report released on Tuesday,Benzinga reports. They presently have a $320.00 target price on the stock. Royal Bank of Canada’s target price indicates a potential upside of 72.96% from the company’s current price.
Several other equities research analysts have also recently commented on MDB. Barclays dropped their target price on shares of MongoDB from $280.00 to $252.00 and set an “overweight” rating for the company in a research report on Friday, May 16th. Needham & Company LLC lowered their price target on MongoDB from $415.00 to $270.00 and set a “buy” rating for the company in a research report on Thursday, March 6th. Stifel Nicolaus decreased their price target on MongoDB from $340.00 to $275.00 and set a “buy” rating for the company in a research note on Friday, April 11th. Monness Crespi & Hardt upgraded MongoDB from a “sell” rating to a “neutral” rating in a research note on Monday, March 3rd. Finally, Cantor Fitzgerald assumed coverage on MongoDB in a report on Wednesday, March 5th. They issued an “overweight” rating and a $344.00 target price on the stock. Nine analysts have rated the stock with a hold rating, twenty-three have issued a buy rating and one has given a strong buy rating to the stock. According to data from MarketBeat.com, MongoDB presently has an average rating of “Moderate Buy” and a consensus price target of $288.91.
Check Out Our Latest Analysis on MDB
MongoDB Trading Down 2.1%
MDB opened at $185.01 on Tuesday. MongoDB has a 12 month low of $140.78 and a 12 month high of $379.06. The business’s 50-day moving average price is $174.84 and its 200-day moving average price is $237.45. The company has a market cap of $15.02 billion, a P/E ratio of -67.52 and a beta of 1.49.
MongoDB (NASDAQ:MDB – Get Free Report) last posted its quarterly earnings results on Wednesday, March 5th. The company reported $0.19 earnings per share (EPS) for the quarter, missing the consensus estimate of $0.64 by ($0.45). MongoDB had a negative return on equity of 12.22% and a negative net margin of 10.46%. The company had revenue of $548.40 million for the quarter, compared to the consensus estimate of $519.65 million. During the same quarter in the previous year, the business earned $0.86 earnings per share. Equities research analysts forecast that MongoDB will post -1.78 earnings per share for the current fiscal year.
Insider Activity at MongoDB
In other news, CEO Dev Ittycheria sold 8,335 shares of the company’s stock in a transaction that occurred on Wednesday, February 26th. The shares were sold at an average price of $267.48, for a total value of $2,229,445.80. Following the completion of the sale, the chief executive officer now owns 217,294 shares of the company’s stock, valued at approximately $58,121,799.12. This represents a 3.69% decrease in their position. The sale was disclosed in a document filed with the Securities & Exchange Commission, which is accessible through this link. Also, CAO Thomas Bull sold 301 shares of the business’s stock in a transaction dated Wednesday, April 2nd. The stock was sold at an average price of $173.25, for a total transaction of $52,148.25. Following the completion of the transaction, the chief accounting officer now owns 14,598 shares of the company’s stock, valued at $2,529,103.50. This represents a 2.02% decrease in their ownership of the stock. The disclosure for this sale can be found here. In the last ninety days, insiders have sold 33,538 shares of company stock worth $6,889,905. Insiders own 3.60% of the company’s stock.
Institutional Investors Weigh In On MongoDB
A number of large investors have recently made changes to their positions in MDB. Cloud Capital Management LLC acquired a new stake in MongoDB during the 1st quarter valued at approximately $25,000. Hollencrest Capital Management acquired a new stake in shares of MongoDB in the first quarter valued at about $26,000. Cullen Frost Bankers Inc. increased its stake in MongoDB by 315.8% during the 1st quarter. Cullen Frost Bankers Inc. now owns 158 shares of the company’s stock worth $28,000 after buying an additional 120 shares in the last quarter. Strategic Investment Solutions Inc. IL purchased a new stake in shares of MongoDB during the fourth quarter worth $29,000. Finally, NCP Inc. acquired a new stake in shares of MongoDB in the fourth quarter valued at approximately $35,000. 89.29% of the stock is owned by hedge funds and other institutional investors.
MongoDB Company Profile
MongoDB, Inc, together with its subsidiaries, provides general purpose database platform worldwide. The company provides MongoDB Atlas, a hosted multi-cloud database-as-a-service solution; MongoDB Enterprise Advanced, a commercial database server for enterprise customers to run in the cloud, on-premises, or in a hybrid environment; and Community Server, a free-to-download version of its database, which includes the functionality that developers need to get started with MongoDB.
Further Reading
Receive News & Ratings for MongoDB Daily – Enter your email address below to receive a concise daily summary of the latest news and analysts’ ratings for MongoDB and related companies with MarketBeat.com’s FREE daily email newsletter.
Article originally posted on mongodb google news. Visit mongodb google news
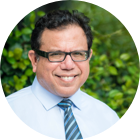
MMS • RSS
Posted on mongodb google news. Visit mongodb google news
MongoDB (NASDAQ:MDB – Get Free Report) is expected to be issuing its Q1 2026 quarterly earnings data before the market opens on Thursday, May 29th. Analysts expect the company to announce earnings of $0.65 per share and revenue of $527.49 million for the quarter. MongoDB has set its Q1 2026 guidance at 0.630-0.670 EPS and its FY 2026 guidance at 2.440-2.620 EPS.
MongoDB (NASDAQ:MDB – Get Free Report) last released its earnings results on Wednesday, March 5th. The company reported $0.19 earnings per share for the quarter, missing the consensus estimate of $0.64 by ($0.45). The company had revenue of $548.40 million for the quarter, compared to analysts’ expectations of $519.65 million. MongoDB had a negative net margin of 10.46% and a negative return on equity of 12.22%. During the same quarter last year, the firm posted $0.86 earnings per share. On average, analysts expect MongoDB to post $-2 EPS for the current fiscal year and $-2 EPS for the next fiscal year.
MongoDB Stock Performance
NASDAQ MDB opened at $185.01 on Thursday. The business’s fifty day moving average is $174.84 and its 200 day moving average is $237.45. The company has a market capitalization of $15.02 billion, a PE ratio of -67.52 and a beta of 1.49. MongoDB has a fifty-two week low of $140.78 and a fifty-two week high of $379.06.
Analyst Upgrades and Downgrades
Several brokerages have recently issued reports on MDB. Scotiabank reaffirmed a “sector perform” rating and issued a $160.00 target price (down from $240.00) on shares of MongoDB in a report on Friday, April 25th. Morgan Stanley reduced their price objective on MongoDB from $315.00 to $235.00 and set an “overweight” rating on the stock in a research note on Wednesday, April 16th. Wedbush reduced their price objective on MongoDB from $360.00 to $300.00 and set an “outperform” rating on the stock in a research note on Thursday, March 6th. Canaccord Genuity Group cut their target price on MongoDB from $385.00 to $320.00 and set a “buy” rating on the stock in a research note on Thursday, March 6th. Finally, Daiwa Capital Markets started coverage on MongoDB in a research note on Tuesday, April 1st. They set an “outperform” rating and a $202.00 target price on the stock. Nine research analysts have rated the stock with a hold rating, twenty-three have assigned a buy rating and one has issued a strong buy rating to the company. Based on data from MarketBeat, the stock has an average rating of “Moderate Buy” and an average target price of $288.91.
Get Our Latest Report on MongoDB
Insiders Place Their Bets
In other news, Director Dwight A. Merriman sold 3,000 shares of the company’s stock in a transaction that occurred on Monday, March 3rd. The shares were sold at an average price of $270.63, for a total value of $811,890.00. Following the completion of the sale, the director now directly owns 1,109,006 shares of the company’s stock, valued at approximately $300,130,293.78. This represents a 0.27% decrease in their position. The sale was disclosed in a legal filing with the SEC, which is accessible through this link. Also, CAO Thomas Bull sold 301 shares of the stock in a transaction that occurred on Wednesday, April 2nd. The shares were sold at an average price of $173.25, for a total transaction of $52,148.25. Following the sale, the chief accounting officer now directly owns 14,598 shares of the company’s stock, valued at approximately $2,529,103.50. This represents a 2.02% decrease in their ownership of the stock. The disclosure for this sale can be found here. In the last 90 days, insiders sold 33,538 shares of company stock worth $6,889,905. Insiders own 3.60% of the company’s stock.
Institutional Inflows and Outflows
A hedge fund recently raised its stake in MongoDB stock. Integrated Wealth Concepts LLC increased its position in shares of MongoDB, Inc. (NASDAQ:MDB – Free Report) by 31.6% in the 1st quarter, according to the company in its most recent filing with the Securities and Exchange Commission. The firm owned 1,363 shares of the company’s stock after buying an additional 327 shares during the period. Integrated Wealth Concepts LLC’s holdings in MongoDB were worth $239,000 at the end of the most recent reporting period. Institutional investors own 89.29% of the company’s stock.
MongoDB Company Profile
MongoDB, Inc, together with its subsidiaries, provides general purpose database platform worldwide. The company provides MongoDB Atlas, a hosted multi-cloud database-as-a-service solution; MongoDB Enterprise Advanced, a commercial database server for enterprise customers to run in the cloud, on-premises, or in a hybrid environment; and Community Server, a free-to-download version of its database, which includes the functionality that developers need to get started with MongoDB.
Further Reading
Receive News & Ratings for MongoDB Daily – Enter your email address below to receive a concise daily summary of the latest news and analysts’ ratings for MongoDB and related companies with MarketBeat.com’s FREE daily email newsletter.
Article originally posted on mongodb google news. Visit mongodb google news
Azure Logic Apps Introduces ‘Agent Loop’ for Building AI Agents in Enterprise Workflows
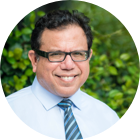
MMS • Steef-Jan Wiggers
Article originally posted on InfoQ. Visit InfoQ
At the annual Build conference, Microsoft announced agent loop, a new capability within Azure Logic Apps that allows developers to build AI agents directly into their enterprise workflows.
Agent loop is a central component for AI Agent development within Logic Apps. It is a new action type integrating a chosen AI model (like Azure OpenAI), domain-specific tools (via Logic Apps connectors), and enterprise knowledge sources. With the component, developers can create various types of AI agents, including autonomous agents for tasks like loan approvals, conversational agents for customer support, and multi-agent systems for coordinated activities such as sales report generation.
(Source: Microsoft Tech community blog post)
Built upon the kernel object in the Semantic Kernel, the agent loop leverages an LLM to determine the necessary steps. At the same time, the Azure Logic Apps runtime handles the execution of these plans. This approach offers significant flexibility, allowing for the creation of both conversational and fully autonomous agents that can respond to real-time events via Logic Apps’ extensive library of connectors.
Divya Swarnkar, a program manager at Microsoft, told InfoQ :
With over 1,400 connectors, Logic Apps is uniquely positioned to power AI Agents with rich context and seamless access to enterprise systems and APIs, enabling them to reason and act reliably.
Agent loop operates through an iterative “Think, Act, and Learn cycle.” The AI agent reasons about its goal and context, takes action by invoking connectors, and then reflects on the results to adjust its plan if needed. Azure Logic Apps manages this cycle automatically.
Microsoft highlights several potential use cases for AI Agents built with agent loop, including:
- Product Return Agent: Verifying order details, return eligibility, and processing refunds or requesting further information.
- Loan Approval Agent: Evaluating credit scores, income, and risk profiles to auto-approve or route applications.
- Recruiting Agent: Screening resumes, summarizing qualifications, and drafting personalized outreach.
- Sales Report Generation Workflow: Utilizing multiple agents for drafting, reviewing, and publishing reports.
- IT Operations Agent: Triaging alerts, checking changes, and resolving common issues or escalating when necessary.
- Multi-Agent Retail Supply Chain Solution: Coordinating inventory and logistics agents for timely restocks and optimized fulfillment.
Furthermore, the key benefits of building AI Agents in Logic Apps with agent loop include declarative orchestration, code extensibility, access to a vast library of integrated tools, observability with full traceability of agent decisions, enterprise-grade governance inheriting the security and compliance of Azure Logic Apps, straightforward human-in-the-loop and multi-agent coordination, and faster time to value by abstracting away the boilerplate of agent architecture.
Kent Weare, a Principal Program Manager for Logic Apps at Microsoft, states:
Building agents or workflows isn’t a binary choice. The most effective solutions often combine both — and that’s where Logic Apps excels. With Agent Loop, customers have full control to dial up the level of agentic automation that fits their needs. Logic Apps is where traditional workflows and AI agents come together, combining forces to solve complex business problems — all within a trusted, enterprise-grade platform. I don’t think any other platform offers this!
In addition, further emphasizing the potential, Cameron McKay, an Azure Application Architect, concluded in a LinkedIn blog post about the agent loop feature in Logic Apps:
This functionality has a lot of potential, and the number of use cases is up to the business and implementer; a few use cases include responding to error events and performing processes based on conversations with humans. I’m excited to see how the use cases for this functionality evolve; without a doubt, this is a highly transformative and useful piece of functionality being added to the Azure Logic Apps toolkit.
Agent loop is available in Azure Logic Apps Standard, and the company has provided documentation and demos to help developers get started. It has also outlined future plans for multi-agent hand-off support, A2A (Agent-to-Agent) protocol support, and OBO Auth for Logic Apps Agents.
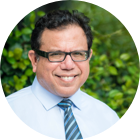
MMS • Matt Foster
Article originally posted on InfoQ. Visit InfoQ
HashiCorp has released the Terraform MCP Server, an open-source implementation of the Model Context Protocol designed to improve how large language models interact with infrastructure as code. By exposing real-time Terraform Registry data—such as module metadata, provider schemas, and resource definitions—in a structured format, the server enables AI systems to ground their suggestions in current, validated configuration patterns. This allows tools like Claude, Co-Pilot and ChatGPT to generate more accurate, context-aware Terraform code by prioritizing canonical sources over outdated or hallucinated examples from training data.
The Model Context Protocol (MCP) is a standard designed to help large language models retrieve structured, machine-readable data from external systems in real time. Rather than relying solely on static training data, AI tools can use MCP to query live sources via JSON-over-gRPC, enabling grounded, context-aware responses. In the Terraform MCP Server implementation, the protocol serves as a bridge between AI systems and the Terraform Registry, exposing data about modules, providers, resources, and their schemas.
This setup allows an AI model to retrieve up-to-date configuration details—such as input arguments for a provider, usage patterns for a popular module, or the latest available version—by issuing standardized queries to an MCP endpoint.
Source Hashicorp
Surfacing this data in a structured format, the server allows AI-assisted tools to align more closely with the latest Terraform standards and configurations. While HashiCorp does not claim specific accuracy improvements yet, it is fair to infer that this approach may help mitigate issues that arise when models rely on outdated or hardcoded infrastructure knowledge (hallucinations).
Although the Terraform MCP Server itself is still in early development, HashiCorp has already demonstrated its integration with GitHub Copilot at Microsoft Build 2025, allowing developers to retrieve context-aware Terraform recommendations grounded in live registry data directly from their IDEs.
Independent projects are also experimenting with the MCP protocol for Terraform: terraform-docs-mcp implements a Node.js-based MCP server to surface module metadata for AI assistants, offering a lightweight alternative for exposing registry data outside the Terraform ecosystem. Meanwhile, tfmcp explores a CLI-driven approach to managing Terraform workflows via LLMs like Claude, enabling tasks such as reading configuration files and analyzing plans through structured prompts. While these community efforts don’t rely on HashiCorp’s implementation, they signal growing interest in the MCP ecosystem as a machine-readable interface to infrastructure knowledge.
Together with other emerging efforts, Terraform MCP Server is another example of a broader pattern in AI-assisted tooling to unify developer workflows. While HashiCorp has not explicitly stated strategic intentions behind MCP, the adoption of such protocols suggests a shift from product-specific AI integrations toward interoperable interfaces designed to support a diverse ecosystem of assistants, clients, and automation workflows.
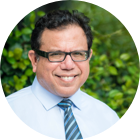
MMS • RSS
Posted on mongodb google news. Visit mongodb google news
Quantinno Capital Management LP raised its stake in MongoDB, Inc. (NASDAQ:MDB – Free Report) by 100.2% during the fourth quarter, according to the company in its most recent Form 13F filing with the Securities and Exchange Commission. The fund owned 14,262 shares of the company’s stock after acquiring an additional 7,137 shares during the period. Quantinno Capital Management LP’s holdings in MongoDB were worth $3,321,000 as of its most recent SEC filing.
A number of other institutional investors have also recently modified their holdings of MDB. Strategic Investment Solutions Inc. IL purchased a new stake in shares of MongoDB in the fourth quarter worth $29,000. NCP Inc. purchased a new stake in shares of MongoDB in the fourth quarter worth $35,000. Coppell Advisory Solutions LLC grew its stake in shares of MongoDB by 364.0% in the fourth quarter. Coppell Advisory Solutions LLC now owns 232 shares of the company’s stock worth $54,000 after acquiring an additional 182 shares during the last quarter. Smartleaf Asset Management LLC boosted its position in shares of MongoDB by 56.8% during the fourth quarter. Smartleaf Asset Management LLC now owns 370 shares of the company’s stock valued at $87,000 after buying an additional 134 shares during the last quarter. Finally, Manchester Capital Management LLC boosted its position in shares of MongoDB by 57.4% during the fourth quarter. Manchester Capital Management LLC now owns 384 shares of the company’s stock valued at $89,000 after buying an additional 140 shares during the last quarter. 89.29% of the stock is owned by hedge funds and other institutional investors.
Wall Street Analyst Weigh In
MDB has been the topic of several recent analyst reports. Monness Crespi & Hardt raised MongoDB from a “sell” rating to a “neutral” rating in a research report on Monday, March 3rd. Citigroup dropped their price objective on MongoDB from $430.00 to $330.00 and set a “buy” rating on the stock in a research report on Tuesday, April 1st. Rosenblatt Securities reaffirmed a “buy” rating and set a $350.00 price objective on shares of MongoDB in a research report on Tuesday, March 4th. Scotiabank reaffirmed a “sector perform” rating and set a $160.00 price objective (down from $240.00) on shares of MongoDB in a research report on Friday, April 25th. Finally, Oppenheimer dropped their price objective on MongoDB from $400.00 to $330.00 and set an “outperform” rating on the stock in a research report on Thursday, March 6th. Eight analysts have rated the stock with a hold rating, twenty-four have issued a buy rating and one has given a strong buy rating to the stock. Based on data from MarketBeat.com, the company presently has an average rating of “Moderate Buy” and an average price target of $293.91.
Read Our Latest Stock Report on MDB
MongoDB Trading Down 1.2%
NASDAQ:MDB opened at $189.01 on Tuesday. MongoDB, Inc. has a 12-month low of $140.78 and a 12-month high of $379.06. The stock has a 50-day moving average price of $174.77 and a 200 day moving average price of $238.15. The stock has a market capitalization of $15.35 billion, a P/E ratio of -68.98 and a beta of 1.49.
MongoDB (NASDAQ:MDB – Get Free Report) last released its earnings results on Wednesday, March 5th. The company reported $0.19 earnings per share (EPS) for the quarter, missing the consensus estimate of $0.64 by ($0.45). MongoDB had a negative return on equity of 12.22% and a negative net margin of 10.46%. The business had revenue of $548.40 million for the quarter, compared to analysts’ expectations of $519.65 million. During the same quarter in the prior year, the business posted $0.86 EPS. On average, research analysts predict that MongoDB, Inc. will post -1.78 earnings per share for the current fiscal year.
Insider Buying and Selling
In other MongoDB news, insider Cedric Pech sold 1,690 shares of the firm’s stock in a transaction that occurred on Wednesday, April 2nd. The stock was sold at an average price of $173.26, for a total transaction of $292,809.40. Following the completion of the transaction, the insider now owns 57,634 shares in the company, valued at $9,985,666.84. This trade represents a 2.85% decrease in their position. The transaction was disclosed in a filing with the SEC, which is available through this link. Also, CFO Srdjan Tanjga sold 525 shares of the firm’s stock in a transaction that occurred on Wednesday, April 2nd. The shares were sold at an average price of $173.26, for a total value of $90,961.50. Following the transaction, the chief financial officer now owns 6,406 shares of the company’s stock, valued at $1,109,903.56. This represents a 7.57% decrease in their position. The disclosure for this sale can be found here. Over the last 90 days, insiders sold 33,538 shares of company stock worth $6,889,905. 3.60% of the stock is owned by corporate insiders.
About MongoDB
MongoDB, Inc, together with its subsidiaries, provides general purpose database platform worldwide. The company provides MongoDB Atlas, a hosted multi-cloud database-as-a-service solution; MongoDB Enterprise Advanced, a commercial database server for enterprise customers to run in the cloud, on-premises, or in a hybrid environment; and Community Server, a free-to-download version of its database, which includes the functionality that developers need to get started with MongoDB.
Featured Articles
This instant news alert was generated by narrative science technology and financial data from MarketBeat in order to provide readers with the fastest and most accurate reporting. This story was reviewed by MarketBeat’s editorial team prior to publication. Please send any questions or comments about this story to contact@marketbeat.com.
Before you consider MongoDB, you’ll want to hear this.
MarketBeat keeps track of Wall Street’s top-rated and best performing research analysts and the stocks they recommend to their clients on a daily basis. MarketBeat has identified the five stocks that top analysts are quietly whispering to their clients to buy now before the broader market catches on… and MongoDB wasn’t on the list.
While MongoDB currently has a Moderate Buy rating among analysts, top-rated analysts believe these five stocks are better buys.
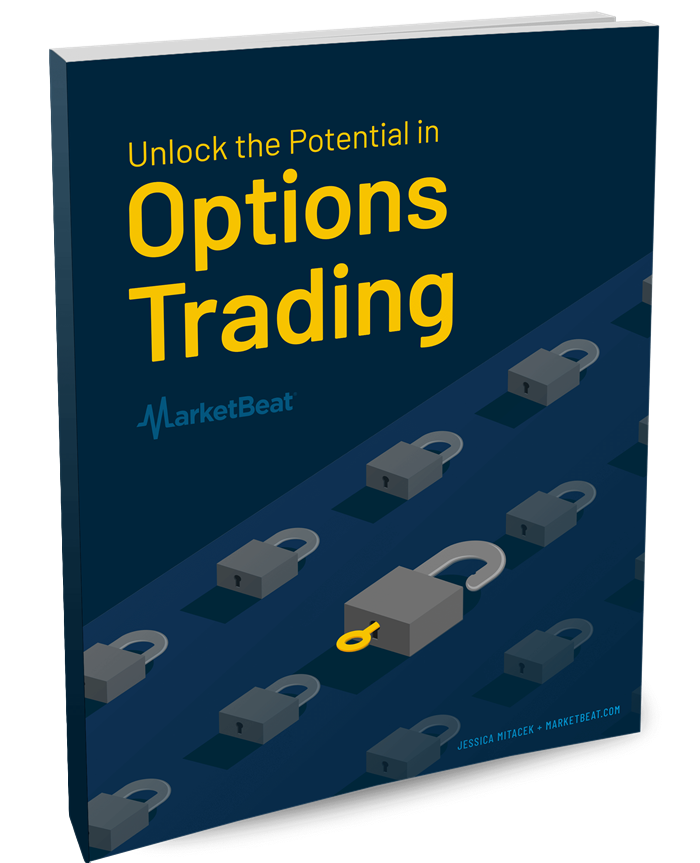
Learn the basics of options trading and how to use them to boost returns and manage risk with this free report from MarketBeat. Click the link below to get your free copy.
Article originally posted on mongodb google news. Visit mongodb google news
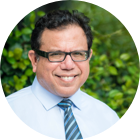
MMS • RSS
Posted on mongodb google news. Visit mongodb google news
MongoDB (NASDAQ:MDB – Get Free Report) was downgraded by research analysts at Loop Capital from a “buy” rating to a “hold” rating in a research report issued on Tuesday, Marketbeat Ratings reports. They presently have a $190.00 target price on the stock, down from their previous target price of $350.00. Loop Capital’s price target indicates a potential upside of 2.70% from the stock’s current price.
A number of other analysts also recently issued reports on MDB. UBS Group set a $350.00 price objective on shares of MongoDB in a report on Tuesday, March 4th. Royal Bank of Canada reduced their price target on MongoDB from $400.00 to $320.00 and set an “outperform” rating on the stock in a research report on Thursday, March 6th. Scotiabank reaffirmed a “sector perform” rating and issued a $160.00 price objective (down from $240.00) on shares of MongoDB in a report on Friday, April 25th. Monness Crespi & Hardt upgraded MongoDB from a “sell” rating to a “neutral” rating in a research report on Monday, March 3rd. Finally, Mizuho decreased their price target on MongoDB from $250.00 to $190.00 and set a “neutral” rating on the stock in a research report on Tuesday, April 15th. Nine equities research analysts have rated the stock with a hold rating, twenty-three have assigned a buy rating and one has issued a strong buy rating to the stock. According to data from MarketBeat.com, the stock presently has a consensus rating of “Moderate Buy” and an average target price of $288.91.
Check Out Our Latest Analysis on MongoDB
MongoDB Stock Performance
Shares of MDB stock opened at $185.01 on Tuesday. MongoDB has a 52 week low of $140.78 and a 52 week high of $379.06. The company has a market capitalization of $15.02 billion, a price-to-earnings ratio of -67.52 and a beta of 1.49. The company has a 50-day simple moving average of $174.84 and a 200 day simple moving average of $237.45.
MongoDB (NASDAQ:MDB – Get Free Report) last released its quarterly earnings results on Wednesday, March 5th. The company reported $0.19 earnings per share for the quarter, missing the consensus estimate of $0.64 by ($0.45). The firm had revenue of $548.40 million for the quarter, compared to analyst estimates of $519.65 million. MongoDB had a negative return on equity of 12.22% and a negative net margin of 10.46%. During the same period in the previous year, the business posted $0.86 earnings per share. Sell-side analysts forecast that MongoDB will post -1.78 EPS for the current year.
Insider Buying and Selling
In related news, CAO Thomas Bull sold 301 shares of MongoDB stock in a transaction that occurred on Wednesday, April 2nd. The shares were sold at an average price of $173.25, for a total value of $52,148.25. Following the completion of the transaction, the chief accounting officer now directly owns 14,598 shares in the company, valued at approximately $2,529,103.50. The trade was a 2.02% decrease in their position. The transaction was disclosed in a filing with the Securities & Exchange Commission, which is accessible through this hyperlink. Also, CFO Srdjan Tanjga sold 525 shares of the stock in a transaction on Wednesday, April 2nd. The stock was sold at an average price of $173.26, for a total value of $90,961.50. Following the sale, the chief financial officer now owns 6,406 shares in the company, valued at approximately $1,109,903.56. This represents a 7.57% decrease in their position. The disclosure for this sale can be found here. Over the last ninety days, insiders have sold 33,538 shares of company stock worth $6,889,905. 3.60% of the stock is owned by insiders.
Institutional Inflows and Outflows
Large investors have recently bought and sold shares of the company. Acadian Asset Management LLC raised its position in MongoDB by 181.8% during the first quarter. Acadian Asset Management LLC now owns 562,190 shares of the company’s stock valued at $98,586,000 after purchasing an additional 362,705 shares in the last quarter. IFM Investors Pty Ltd boosted its stake in MongoDB by 4.3% in the first quarter. IFM Investors Pty Ltd now owns 13,796 shares of the company’s stock worth $2,420,000 after buying an additional 569 shares in the last quarter. UBS AM A Distinct Business Unit of UBS Asset Management Americas LLC boosted its stake in MongoDB by 11.3% in the first quarter. UBS AM A Distinct Business Unit of UBS Asset Management Americas LLC now owns 1,271,444 shares of the company’s stock worth $223,011,000 after buying an additional 129,451 shares in the last quarter. Woodline Partners LP grew its position in MongoDB by 30,297.0% during the first quarter. Woodline Partners LP now owns 322,208 shares of the company’s stock valued at $56,515,000 after acquiring an additional 321,148 shares during the last quarter. Finally, Meiji Yasuda Life Insurance Co raised its stake in shares of MongoDB by 36.1% during the first quarter. Meiji Yasuda Life Insurance Co now owns 3,997 shares of the company’s stock worth $701,000 after acquiring an additional 1,060 shares in the last quarter. 89.29% of the stock is owned by institutional investors.
About MongoDB
MongoDB, Inc, together with its subsidiaries, provides general purpose database platform worldwide. The company provides MongoDB Atlas, a hosted multi-cloud database-as-a-service solution; MongoDB Enterprise Advanced, a commercial database server for enterprise customers to run in the cloud, on-premises, or in a hybrid environment; and Community Server, a free-to-download version of its database, which includes the functionality that developers need to get started with MongoDB.
Further Reading
Receive News & Ratings for MongoDB Daily – Enter your email address below to receive a concise daily summary of the latest news and analysts’ ratings for MongoDB and related companies with MarketBeat.com’s FREE daily email newsletter.
Article originally posted on mongodb google news. Visit mongodb google news
Prime Intellect Releases INTELLECT-2: A 32B Parameter Model Trained via Decentralized Reinforcement
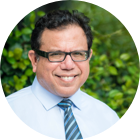
MMS • Robert Krzaczynski
Article originally posted on InfoQ. Visit InfoQ
Prime Intellect has released INTELLECT-2, a 32 billion parameter language model trained using fully asynchronous reinforcement learning across a decentralized network of compute contributors. Unlike traditional centralized model training, INTELLECT-2 is developed on a permissionless infrastructure where rollout generation, policy updates, and training are distributed and loosely coupled.
The system is built around PRIME-RL, a new training framework designed for asynchronous RL in untrusted environments. It separates the tasks of generating rollouts, updating models, and broadcasting weights. Policy updates are handled by SHARDCAST, a component that distributes model weights using a tree-based HTTP network. Inference rollouts submitted by workers are verified through TOPLOC, a locality-sensitive hashing mechanism that detects tampering or numerical discrepancies before allowing the results to influence training.
Source: https://arxiv.org/html/2505.07291v1
INTELLECT-2 was trained on 285,000 math and coding tasks sourced from datasets such as NuminaMath-1.5 or SYNTHETIC-1. The reward signal combines binary task success with token-length penalties or bonuses, allowing fine-grained control over inference-time compute budgets. Training stability was supported by techniques such as two-sided GRPO clipping, gradient norm management, and both offline and online filtering of high-value tasks.
The asynchronous training process overlaps inference, communication, and model updates, avoiding typical bottlenecks found in centralized RL systems. A Rust-based orchestrator running on a testnet coordinates the global pool of contributors, handling hardware checks, heartbeats, task assignments, and contribution tracking—operating similarly to peer-to-peer or blockchain-based systems.
Performance evaluations showed improvements on targeted math and programming tasks, particularly over QwQ-32B, a previous RL-trained model. Broader benchmark improvements were more modest, suggesting gains were mostly confined to training data domains. Prime Intellect noted that improvements might be more significant using stronger base models, such as Qwen3, or by integrating more complex environments and reasoning tools.
One Reddit user remarked on the broader implications:
Distributed training and distributed inference seem like the way to go. Maybe something similar to P2P or blockchain with some kind of rewards for computational contributions/transactions. Not necessarily yet another cryptocurrency, but maybe credits that can be used for free computing on the network.
Future work includes increasing the inference-to-training compute ratio, enabling multi-turn reasoning with integrated tools like web search or Python, crowdsourcing RL tasks, and experimenting with decentralized model merging methods such as DiLoCo.
The model, code, training framework, and documentation are publicly available on the Prime Intellect website. Additional tools and interfaces, including a Hugging Face release and a chat demo, are also publicly accessible.
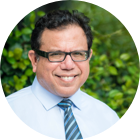
MMS • RSS
Posted on mongodb google news. Visit mongodb google news
Spyglass Capital Management LLC increased its position in shares of MongoDB, Inc. (NASDAQ:MDB – Free Report) by 5.8% during the fourth quarter, according to its most recent filing with the Securities and Exchange Commission. The firm owned 249,683 shares of the company’s stock after acquiring an additional 13,712 shares during the period. MongoDB makes up about 3.3% of Spyglass Capital Management LLC’s holdings, making the stock its 19th biggest position. Spyglass Capital Management LLC owned 0.34% of MongoDB worth $58,129,000 at the end of the most recent reporting period.
Other hedge funds and other institutional investors have also bought and sold shares of the company. B.O.S.S. Retirement Advisors LLC bought a new stake in MongoDB during the 4th quarter worth approximately $606,000. Union Bancaire Privee UBP SA bought a new stake in MongoDB during the 4th quarter worth approximately $3,515,000. HighTower Advisors LLC increased its holdings in MongoDB by 2.0% during the 4th quarter. HighTower Advisors LLC now owns 18,773 shares of the company’s stock worth $4,371,000 after acquiring an additional 372 shares during the period. Nisa Investment Advisors LLC increased its holdings in MongoDB by 428.0% during the 4th quarter. Nisa Investment Advisors LLC now owns 5,755 shares of the company’s stock worth $1,340,000 after acquiring an additional 4,665 shares during the period. Finally, Jones Financial Companies Lllp increased its holdings in MongoDB by 68.0% during the 4th quarter. Jones Financial Companies Lllp now owns 1,020 shares of the company’s stock worth $237,000 after acquiring an additional 413 shares during the period. Institutional investors own 89.29% of the company’s stock.
Insider Activity
In related news, Director Dwight A. Merriman sold 3,000 shares of the business’s stock in a transaction dated Monday, March 3rd. The shares were sold at an average price of $270.63, for a total transaction of $811,890.00. Following the sale, the director now owns 1,109,006 shares of the company’s stock, valued at $300,130,293.78. The trade was a 0.27% decrease in their ownership of the stock. The transaction was disclosed in a legal filing with the SEC, which is available at this link. Also, CEO Dev Ittycheria sold 8,335 shares of the business’s stock in a transaction dated Wednesday, February 26th. The stock was sold at an average price of $267.48, for a total transaction of $2,229,445.80. Following the sale, the chief executive officer now directly owns 217,294 shares in the company, valued at $58,121,799.12. The trade was a 3.69% decrease in their position. The disclosure for this sale can be found here. In the last 90 days, insiders have sold 33,538 shares of company stock valued at $6,889,905. Corporate insiders own 3.60% of the company’s stock.
MongoDB Price Performance
MongoDB stock traded down $3.93 during trading hours on Wednesday, reaching $185.01. The stock had a trading volume of 2,005,037 shares, compared to its average volume of 1,926,229. The stock has a market cap of $15.02 billion, a PE ratio of -67.52 and a beta of 1.49. MongoDB, Inc. has a 52 week low of $140.78 and a 52 week high of $379.06. The company has a fifty day simple moving average of $174.84 and a 200-day simple moving average of $237.45.
MongoDB (NASDAQ:MDB – Get Free Report) last announced its quarterly earnings results on Wednesday, March 5th. The company reported $0.19 earnings per share (EPS) for the quarter, missing analysts’ consensus estimates of $0.64 by ($0.45). The firm had revenue of $548.40 million during the quarter, compared to the consensus estimate of $519.65 million. MongoDB had a negative return on equity of 12.22% and a negative net margin of 10.46%. During the same period last year, the business earned $0.86 EPS. Research analysts predict that MongoDB, Inc. will post -1.78 earnings per share for the current fiscal year.
Analyst Upgrades and Downgrades
A number of analysts recently weighed in on the stock. Wells Fargo & Company lowered shares of MongoDB from an “overweight” rating to an “equal weight” rating and decreased their target price for the stock from $365.00 to $225.00 in a report on Thursday, March 6th. China Renaissance initiated coverage on shares of MongoDB in a research note on Tuesday, January 21st. They issued a “buy” rating and a $351.00 price target for the company. Stifel Nicolaus cut their price target on shares of MongoDB from $340.00 to $275.00 and set a “buy” rating for the company in a research note on Friday, April 11th. UBS Group set a $350.00 price target on shares of MongoDB in a research note on Tuesday, March 4th. Finally, Wedbush cut their price target on shares of MongoDB from $360.00 to $300.00 and set an “outperform” rating for the company in a research note on Thursday, March 6th. Nine analysts have rated the stock with a hold rating, twenty-three have issued a buy rating and one has assigned a strong buy rating to the company. According to MarketBeat.com, the stock has an average rating of “Moderate Buy” and a consensus price target of $288.91.
View Our Latest Analysis on MongoDB
About MongoDB
MongoDB, Inc, together with its subsidiaries, provides general purpose database platform worldwide. The company provides MongoDB Atlas, a hosted multi-cloud database-as-a-service solution; MongoDB Enterprise Advanced, a commercial database server for enterprise customers to run in the cloud, on-premises, or in a hybrid environment; and Community Server, a free-to-download version of its database, which includes the functionality that developers need to get started with MongoDB.
Featured Articles
Before you consider MongoDB, you’ll want to hear this.
MarketBeat keeps track of Wall Street’s top-rated and best performing research analysts and the stocks they recommend to their clients on a daily basis. MarketBeat has identified the five stocks that top analysts are quietly whispering to their clients to buy now before the broader market catches on… and MongoDB wasn’t on the list.
While MongoDB currently has a Moderate Buy rating among analysts, top-rated analysts believe these five stocks are better buys.
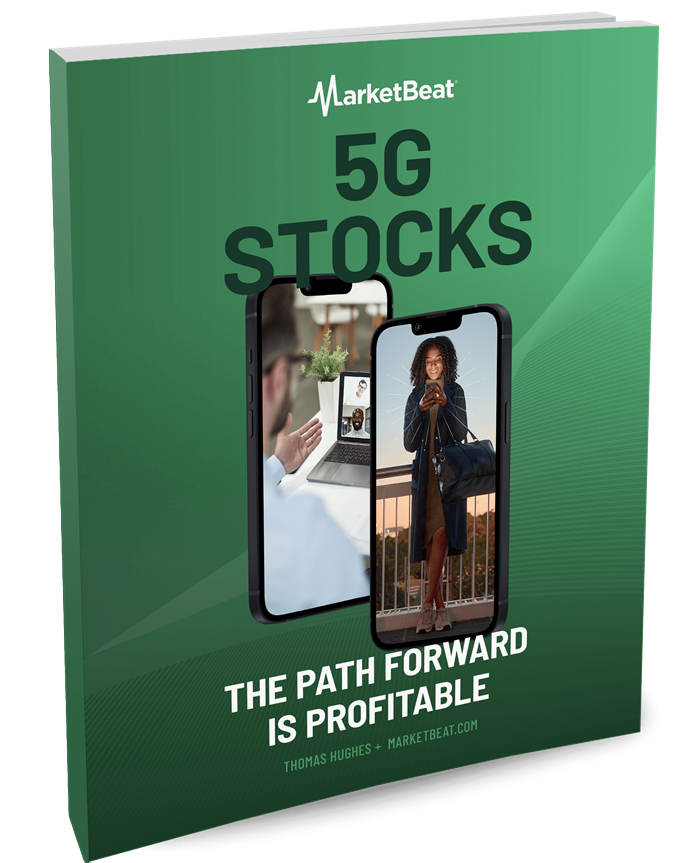
Enter your email address and we’ll send you MarketBeat’s guide to investing in 5G and which 5G stocks show the most promise.
Article originally posted on mongodb google news. Visit mongodb google news