Category: Uncategorized
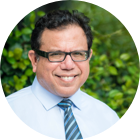
MMS • RSS
Posted on mongodb google news. Visit mongodb google news
On May 20, 2025, RBC Capital analyst Rishi Jaluria reiterated his positive outlook on MongoDB (MDB, Financial). The analyst maintained an “Outperform” rating for the company, reflecting continued confidence in its market position and growth potential.
In addition to reaffirming the rating, RBC Capital kept the price target steady at $320.00 USD. This price target remains unchanged from the previous analysis, indicating consistent expectations for MongoDB’s stock performance in the near future.
MongoDB (MDB, Financial) continues to garner attention from analysts as a key player in the database management sector. The reaffirmed rating and price stability suggest a sustained belief in the company’s strategic initiatives and market opportunities.
Wall Street Analysts Forecast
Based on the one-year price targets offered by 34 analysts, the average target price for MongoDB Inc (MDB, Financial) is $273.14 with a high estimate of $520.00 and a low estimate of $160.00. The average target implies an
upside of 46.33%
from the current price of $186.66. More detailed estimate data can be found on the MongoDB Inc (MDB) Forecast page.
Based on the consensus recommendation from 37 brokerage firms, MongoDB Inc’s (MDB, Financial) average brokerage recommendation is currently 2.0, indicating “Outperform” status. The rating scale ranges from 1 to 5, where 1 signifies Strong Buy, and 5 denotes Sell.
Based on GuruFocus estimates, the estimated GF Value for MongoDB Inc (MDB, Financial) in one year is $438.57, suggesting a
upside
of 134.96% from the current price of $186.66. GF Value is GuruFocus’ estimate of the fair value that the stock should be traded at. It is calculated based on the historical multiples the stock has traded at previously, as well as past business growth and the future estimates of the business’ performance. More detailed data can be found on the MongoDB Inc (MDB) Summary page.
Article originally posted on mongodb google news. Visit mongodb google news
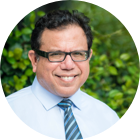
MMS • RSS
Posted on mongodb google news. Visit mongodb google news
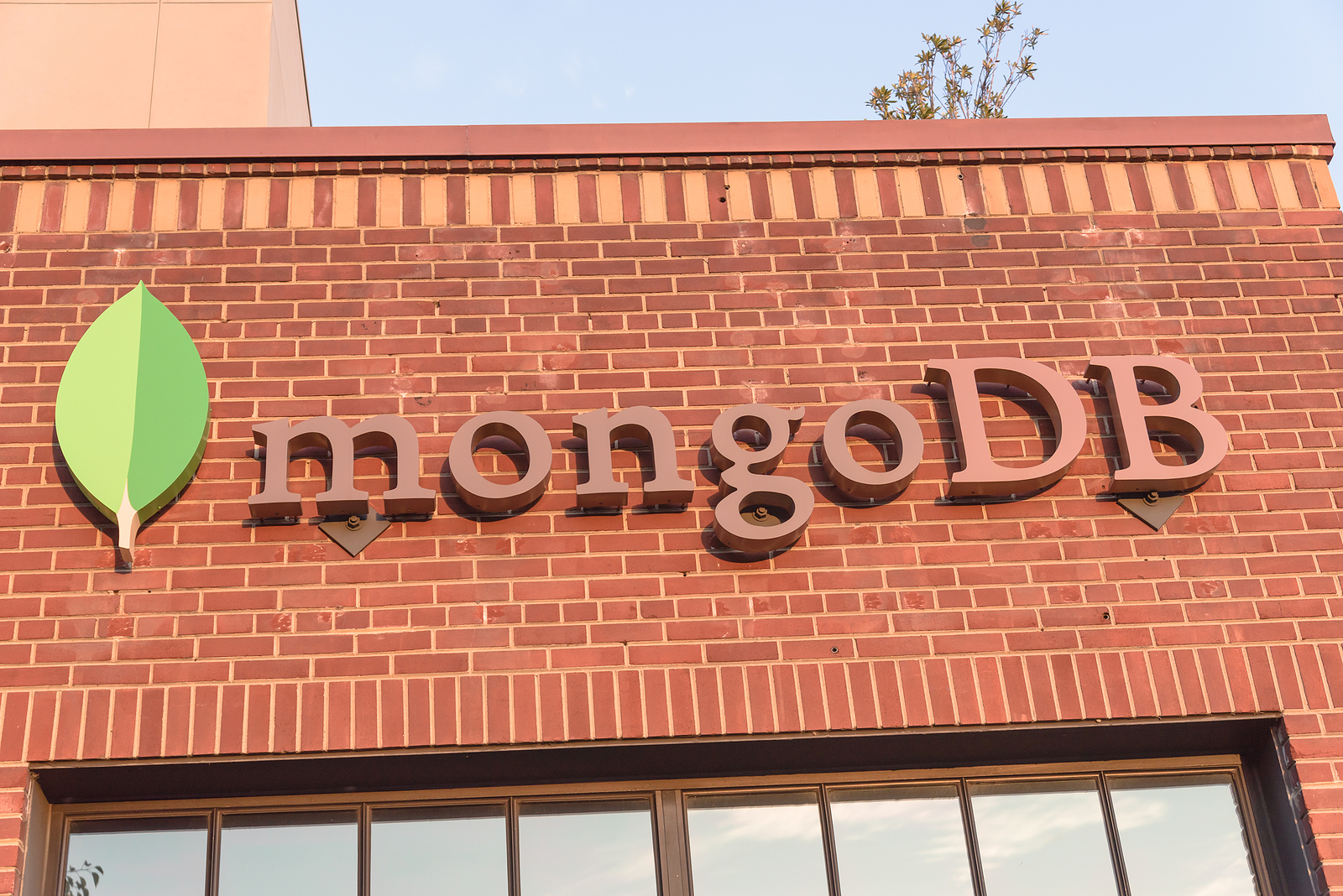
Loop Capital downgraded MDB to “hold” from “buy”
Software stock MongoDB Inc (NASDAQ:MDB) is down 1.5% at $186.09 at last glance, after a downgrade from Loop Capital to “hold” from “buy,” with a steep price-target cut to $190 from $350. The firm sees slowing adoption of the company’s artificial intelligence (AI) platform Atlas, with limited potential for progress in the near future.
On the charts, MongoDB stock has been slowly climbing since its April 7 two-year low of $140.78, though the $200 level, which rejected the shares in March and earlier this month, still lingers above. Since the start of the year, MDB is down roughly 20%.
The majority of analysts are still bullish on the stock. Twenty-seven of the 37 in coverage carry a “buy” or better rating, while the 12-month consensus price target of $273.14 sits at a 45% premium to current levels. Should MDB continue to struggle, analysts may be forced to adjust their tune, which could in turn weigh on the equity even more.
Options traders have been bullish over the last 10 weeks as well. MDB’s 50-day call/put volume ratio of 2.19 at the International Securities Exchange (ISE), Cboe Options Exchange (CBOE), and NASDAQ OMX PHLX (PHLX) ranks higher than 97% of readings from the past year.
Options are an intriguing route, regardless of direction. MDB’s Schaeffer’s Volatility Scorecard (SVS) of 90 out of 100 indicates it has exceeded options traders’ volatility expectations over the past year.
Article originally posted on mongodb google news. Visit mongodb google news
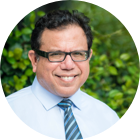
MMS • RSS
Posted on mongodb google news. Visit mongodb google news
The most talked about and market moving research calls around Wall Street are now in one place. Here are today’s research calls that investors need to know, as compiled by The Fly.
Top 5 Upgrades:
-
UBS upgraded Mettler-Toledo (MTD) to Buy from Neutral with a price target of $1,350, down from $1,530. The firm cites the company’s “incremental opportunities” for service sales, “industry leading” pricing power, “beneficial” portfolio exposure, and medium-term tailwind from reshoring for the upgrade.
-
Melius Research upgraded Kroger (KR) to Hold from Sell with a two-year price target of $70, up from $58. The firm cites share gains from widespread pharmacy closures, limited exposure to tariffs within the retail landscape, and consistent free cash flow generation to support on-going investment and share repurchases for the upgrade.
-
Evercore ISI upgraded HP Enterprise (HPE) to Outperform from In Line with a price target of $22, up from $17. The firm thinks the current risk/reward is “fairly attractive,” especially for investors that have some duration, the firm tells investors.
-
Wolfe Research upgraded LivaNova (LIVN) to Outperform from Peer Perform with a $60 price target. The firm says that since its downgrade, LivaNova’s valuation has compressed, its Italian legal overhang has lifted at a total liability a little less than “worst case,” and it made a commitment to expand oxygenator capacity into next year, which could produce “step change” in its ability to supply 2026.
-
Citi upgraded Air Lease (AL) to Buy from Neutral with a price target of $68, up from $45. The firm says that after pivoting away from a long-held strategy of growing organically via direct purchases from aerospace manufacturers, Air Lease “now seems to be pursuing an AerCap-style capital allocation strategy.”
Top 5 Downgrades:
-
Loop Capital downgraded MongoDB (MDB) to Hold from Buy with a price target of $190, down from $350. The firm’s most recent industry checks indicate that MongoDB’s Atlas platform “continues to show lackluster market adoption.”
-
Deutsche Bank downgraded Chubb (CB) to Hold from Buy with a $303 price target. The company’s year-to-date outperformance versus the S&P 500 Index is unlikely to continue, as a “calmer” equity market shifts its focus back to underlying fundamentals, the firm tells investors in a research note.
-
Morgan Stanley downgraded Asana (ASAN) to Underweight from Equal Weight with an unchanged price target of $14. The firm says that over this period of time, its channel checks and the broader macro backdrop do not support the prospect of improving fundamentals.
-
Raymond James downgraded Nutanix (NTNX) to Market Perform from Outperform without a price target. The shares are appropriately valued following the recent rally, the firm says.
-
Jefferies downgraded Onto Innovation (ONTO) to Hold from Buy with a price target of $110, down from $135. The firm believes the drivers of the artificial intelligence packaging correction are likely to extend through 2026, leaving Onto’s 2027 as a “show-me story based upon regaining share.”
Top 5 Initiations:
-
William Blair initiated coverage of OneStream (OS) with an Outperform rating. The firm says OneStream has seen strong growth in recent years and was one of the few public software vendors to grow revenue over 30% in 2024. It believes the company has a “fast-growing” software platform.
-
TD Cowen initiated coverage of Zymeworks (ZYME) with a Buy rating and no price target. The firm believes Ziihera has blockbuster potential and impending data will be the next key catalyst for the stock in the second half of 2025.
-
Raymond James initiated coverage of Lionsgate Studios (LION) with an Outperform rating and $10 price target. The firm says that unlike many other media stocks, Lionsgate has no direct exposure to the declining linear TV ecosystem.
-
Raymond James initiated coverage of Starz Entertainment (STRZ) with an Outper0form rating and $19 price target. Starz is a “misunderstood asset” given its small size and the fact it has been hidden in the legacy Lionsgate structure under the “sexier” studio business since 2016, the firm tells investors in a research note.
-
Roth Capital initiated coverage of Capricor Therapeutics (CAPR) with a Buy rating and $31 price target. The firm’s optimism on the shares is driven by “first-in-indication” deramiocel’s ability to improve cardiac and skeletal muscle function in Duchenne muscular dystrophy patients with cardiomyopathy.
Article originally posted on mongodb google news. Visit mongodb google news
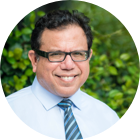
MMS • RSS
Posted on mongodb google news. Visit mongodb google news
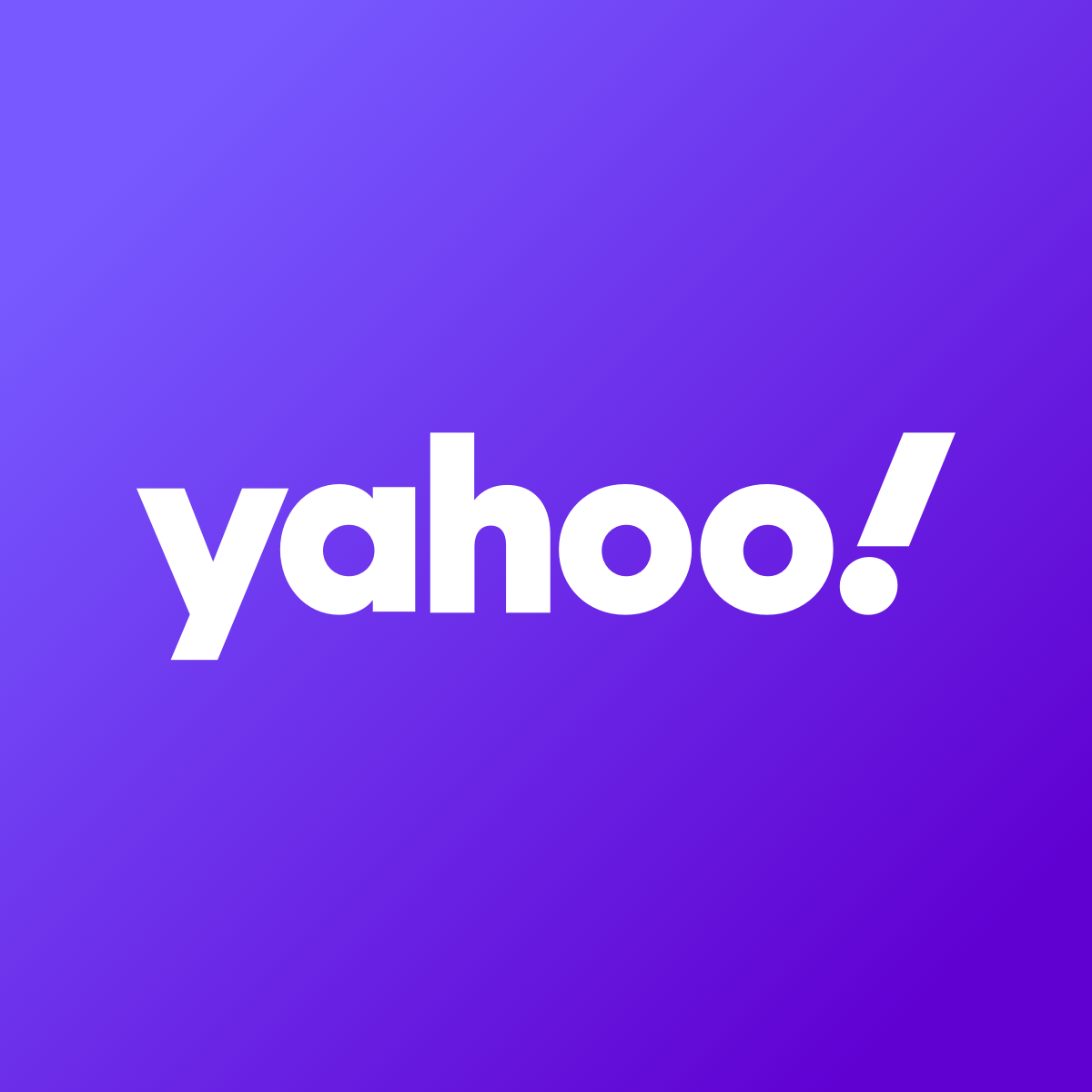
Investing.com — Loop Capital downgraded MongoDB (NASDAQ:MDB) to Hold from Buy and slashed its price target to $190 from $350 in a note Tuesday.
The firm highlighted concerns over slowing adoption of the company’s Atlas platform and delayed benefits from artificial intelligence (AI) workloads.
“Our most recent industry checks indicate that its highly strategic Atlas platform continues to show lackluster market adoption,” Loop Capital analysts wrote. “This decelerating trend could continue, which we believe could result in slower ramp of AI-related workloads on the Atlas platform.”
Advertisement: High Yield Savings Offers
Loop warned that while AI hype continues to grow, MongoDB may not see a proportional benefit in the near term.
The firm noted that MongoDB’s target market for cloud database platforms remains “highly fragmented,” with organizations unlikely to standardize on a single vendor like MDB for all AI deployments.
“This scenario will likely result in a slower ramp of AI-related workloads running on the MDB platform vs. the pace of overall AI deployments,” the analysts said.
Additionally, industry contacts are said to have told Loop that large enterprises may be less inclined to consolidate their database platforms due to GenAI reducing development complexity.
“This could lead to organizations opting for low-cost alternatives, including open source platforms such as PostgreSQL,” explained the firm.
Despite heavy investment in targeting large enterprises, Loop sees limited progress.
“The need to consolidate and standardize on one platform within a large organization to generate more efficiency and lower maintenance cost is becoming less relevant,” the note said.
Loop did acknowledge MongoDB’s long-term strengths, including “a large, loyal community consisting of 7M+ developers,” but flagged short-term risks, including transition-related uncertainty as new CFO Mike Berry joins the company later this month.
While first-quarter estimates remain unchanged, Loop lowered its Atlas growth trajectory going forward due to persistent weakness in new workloads and a slower AI ramp.
Related articles
MongoDB downgraded as AI tailwinds are likely slower than expected
Paramount Group raised to buy at Evercore ISI
Elon Musk says Tesla is already back
Article originally posted on mongodb google news. Visit mongodb google news
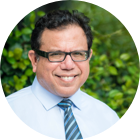
MMS • Courtney Nash
Article originally posted on InfoQ. Visit InfoQ
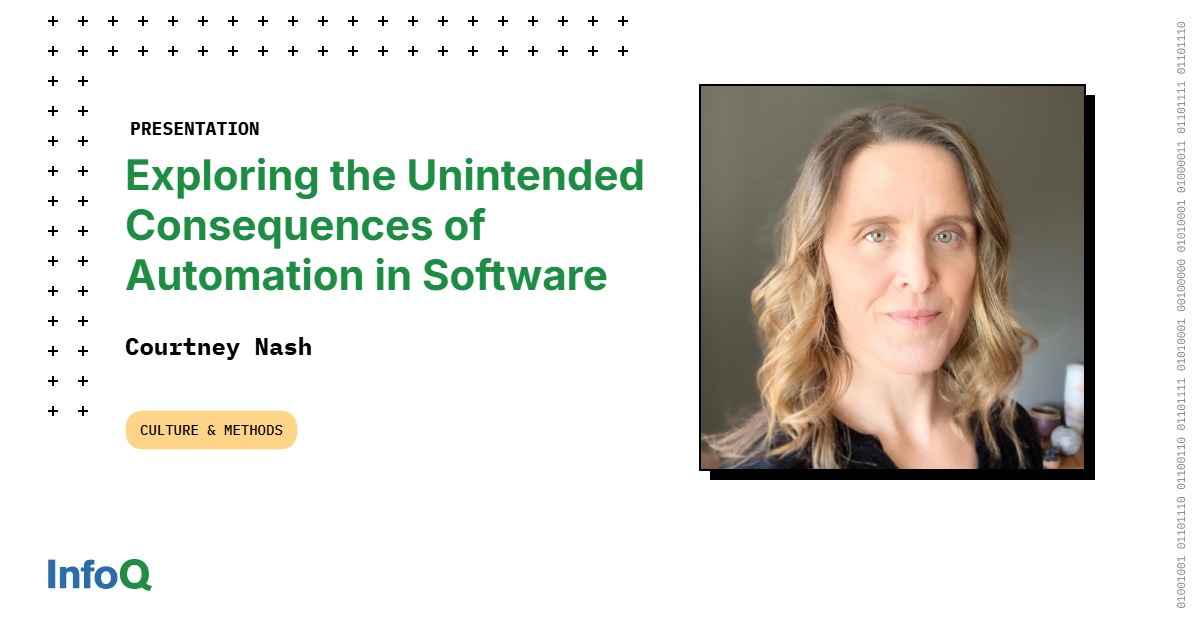
Transcript
Nash: We’re going to kick off this talk with vultures. We’re going to talk about predators a lot. In the early 1990s, or the late 1900s, a painkiller called diclofenac became available in a new and affordable generic form in India. Farmers quickly realized that this generic form of diclofenac was incredibly useful in treating pain and inflammation. It’s a widely used human drug, but they were using it in their livestock. This is a country where there’s lots of livestock, and in general, they’re not to be eaten. They are there to help out, to exist. It was adopted almost universally across India, which had at the time, approximately 500 million said livestock animals. What no one knew at the time was that even small residue amounts of diclofenac, when consumed by vultures, would cause kidney failure, killing the vulture in a matter of weeks. The crisis that followed was historic in its speed and its scope.
The three most common species of vultures disappeared from India. They all died in less than a decade. The region’s primary scavenger became carrion. It quickly became obvious that India was actually dependent on vultures to clean up livestock animals as they passed away. Cows, sheep, lots of other livestock. Without the infrastructure, which had previously been the vultures, to process these carcasses, the shock of the vulture collapse led to carcasses piling up all over India. A public health emergency followed, the depth of which researchers are really now just starting to fully disentangle.
This was the consequence that came out of that. This was not 500,000 people that died in India total, this was an extra 500,000 people that died, above the standard population death over the course of those 5 years, that was attributed by researchers to the disappearance of the vulture population. These other deaths came from other sources. Increased rates of disease, like rabies, and decreased water quality due to contamination from carcasses piling up in the local water supplies. This is a very fancy graphic of that.
In addition to the loss of life and death, the vulture crisis was costing India billions of dollars. The cleanup that the vultures had simply done in the past had to be accomplished with human crews, vehicles. Eventually, they had to develop expensive new facilities like rendering plants, which if you want to sleep at night, don’t Google animal rendering plants like I did. There were two researchers who primarily dug into this, Saudamini Das and her co-author N.M. Ishwar, and they estimated that each vulture, singular vulture in India, was providing between $3,000 and $4,000 of economic value. Probably more than that, but that’s what they could really tie to the factors that they were able to study.
This is one example of intertwined cultural, biological, and agricultural systems. Another one was the Great Hunger or the Potato Famine in Ireland, and also the Four Pests Campaign that led to the Great Chinese Famine in the middle of the 20th century. What’s really key to this is that vultures were an unexamined, unknown source of resilience within the agricultural landscape in India. We’re going to kick this off with that little story, and we’ll talk about some of the kinds of systems where you’re looking for resilience as well, day in and day out.
Background
I have a background in cognitive neuroscience. Then, I ran off and joined the internet and worked at all these places. In 2020, I started something called The VOID. The VOID is a large database of public incident reports. The goal of this when I first started it, and still to this day, was to make these incident reports available to anyone to raise awareness of how software failures impact our lives, and to increase understanding, and try to help us learn from those failures, and make the internet a more resilient and safe place. This is Honeycomb. Fred Hebert writes a lot of their incident reports.
My favorite part of this is where it says things are bad. You may be familiar with that phenomenon. There are over 10,000 public incident reports in the database at this point, from I think about 2008 up to present day, in a variety of formats. Big, long, comprehensive postmortems, but there’s also things like tweets, and media articles, and conference talks, and all those things. There’s a lot of metadata in The VOID. In the past, I’ve done a lot of analysis on that.
Why the Interest in Automation and AI?
I started to get very interested in what I could learn about automation. That’s what we’re really here to talk about. Much of my work prior in The VOID has been looking at quantitative data around things like duration and severity, trying to dispel some common beliefs and myths around things like MTTR. There’s a bunch of my previous work that had done that. There’s a lot of reasons why I started wanting to dig into automation and AI. Let’s start off with an example you might be familiar with. Anybody know what this is? Knight Capital? On August 1st, 2012, Knight Capital Group, a financial services company, released an update that ended up losing the company $460 million in 20 minutes, severely impacting the valuation in numerous other industries and companies.
By the next day, Knight Capital’s value had plummeted by 75%. Within a week, the company basically ceased to exist. It was an extinction event for that company. This is a quote from the Security Exchange Commission’s report on that Knight Capital event. I’m not going to go into a great detail of this, but I wanted to talk about this quote where they talk about being able to keep pace. John Allspaw has done a really great deep dive into this write-up. He wrote about our sharp contrast between our ability to create complex and valuable automation and our ability to reason about, influence, control, and understand it in even normal operating conditions. Forget about the time pressured emergency situations we often find ourselves in. I have some links and references at the end if you really want to dig into this. The SEC went into all the ways that they wanted to think that Knight Capital screwed up.
The biggest one being how an automated process could lead to something like this. Two caveats. I hate that write-up. Don’t go to that thinking that’s like some source of truth or anything. It was fascinating to watch a body like that have to dive into technical details and start to wrap their heads around something as complex as automation in financial services. That’s the first thing. Go read John’s blog post. The second thing is, I’m not anti-automation or necessarily AI. I’m just a smidge more pro people. Automation is not going away. It’s a necessary and generally beneficial thing for businesses, for all of us. What I’m here to challenge is our current mental models about automation and AI, which are founded in some not just outdated but originally misguided ideas.
What Is Automation?
Before we go down that road, we want to talk about what do we mean by this. Let’s try to have at least a shared definition, because it’s arguable that all software embodies some form of automation. It’s a computer doing something for you. That’s fine. I wanted to have a shared definition that I could use for the research that I did, based on what people assume automation does. Here’s the definition. Automation refers to the use of machines to perform tasks, often repetitive, in place of humans, with the aim of accelerating progress, enhancing accuracy, or reducing errors compared to manual human work. How many of you feel like that’s not bad? My goal is by the end, I’m going to convince you we’re all wrong. We’re all wrong, because I’ve wandered into this with that belief and assumption as well.
Functional Allocation and the Substitution Myth
Let’s get into the nerdy cognitive science and research stuff. The underlying and often unexamined assumptions about automation is the notion that computers and machines are better at some things and humans are better at others. Historically, this has been characterized as HABA-MABA, humans are better at. It used to be MABA-MABA, men are better at. We got that one figured out, supposedly. Also known as the Fitts List, based on the work of Paul Fitts. He was a psychologist and a researcher at The Ohio State University in the mid-1900s.
More recently, researchers who are starting to dig into this have described this as functional allocation by substitution, or the substitution myth. These were researchers such as Erik Hollnagel, Sidney Dekker, David Woods, trying to dig into the idea that we can just substitute machines for people. Research from other domains has recently really started to challenge this substitution myth and functional allocation. One of the primary ones comes from aviation and automated cockpits. That research, I’ll get into it here in a bit more, but really often found that automation, contrary to what we think and assume, contributes to incidents and accidents in unforeseen ways. It tends to impose unexpected and unforeseen burdens on the humans responsible for operating in those systems with automation.
We go back to the Fitts List right here. There’s fixed strengths and weaknesses that computers and humans have, and all we have to do is just give separate tasks. We’ll just budget this stuff out over here and this over here, and we’ll all just go off and do more stuff, and everything will be happy and great. Right? Not right? Not how it usually works? It’s certainly not always the experience of a lot of people who work in complex software systems. I mentioned a couple of researchers, Sidney Dekker and David Woods. This is from a paper that they wrote in 2002. I’m going to just step through a few of the things that they talked about having the consequences of this assumption built into your automated systems. As you’re thinking about this in your world, you could think about things like CI/CD deployment pipelines, or load balancing, or anything that automatically does those things for you.
The first one is this, that as designers, either us designing our own little bits of automation, or people who are putting automation into tools, tend to have the desired outcomes in mind, and only those outcomes in mind. They don’t really know necessarily or understand or think about the other consequences that could come out of the automation that they’re designing. The second one is, it doesn’t really have access to all of the real-world parameters that we have. This is something that you have probably already heard quite a lot about, about autonomous cars and other kinds of systems like that, that their model is their model that we give them, but it’s not entirely the real-world model. Of course, people are trying to work on developing much more real-world models, but we’re certainly not there yet. What happens is, without that larger broader context, it actually makes it harder for the humans who have to solve problems when they arise in those environments.
If you’ve ever not known what dashboard to look at when the shit’s hitting the fan, that’s a very simple way of thinking about this particular problem with our assumptions around how we build automation into systems. The third one is that automation just doesn’t take stuff away, automation adds in ways that we may not have thought of as designers of automation. It transforms people’s work and tasks, often forcing them to adapt in unexpected and novel ways. Digging through logs, I’d mentioned that before, but you have to start looking in other places, you don’t actually know what’s happening, and you don’t have any access to what that automated system is necessarily doing.
Then the last one is, it doesn’t really necessarily replace human weaknesses. What it can do actually is it creates new human weaknesses, or new pockets of problems. You’ll see this in the development of knowledge silos or pockets or silos of expertise. Some people have developed a certain degree of expertise with that system, but no one else has because they haven’t had experience with it. Amy the Kafka wizard is on vacation when things go sideways and nobody but Amy knows how to fix it, that’s because she’s the only one who’s actually developed that set of expertise with that automated system, and for everyone else it’s effectively a new weakness that they don’t have in the face of that system. That’s one piece of work done.
The Ironies of Automation
This next one is the ironies of automation. This was work done by a woman named Lisanne Bainbridge in the mid-80s, probably before Alanis Morissette wrote this song. Lisanne Bainbridge was a cognitive psychologist and a human factors researcher. A lot of her work was in the ’60s to ’80s. She obtained a doctorate for work on process controllers, but then went to work on things like mental load, cognitive complexity, and related topics. Her work was based primarily on direct observation of and interviews with experts, in this case pilots. She spoke to them. She watched them doing the work that they needed to do. This was as we were beginning to increasingly automate cockpits in aviation that pilots were then having to work with on a daily basis. She found this set of ironies of automation. In the paper that I referenced right there, it’s not like there’s a solid one to seven list or something. I’ve glommed them into four just to try to make things a little bit easier for us to get through here.
The first irony is that humans are the designers of automation, and yet their designs often have unanticipated negative consequences. I’ve already mentioned this, but she really saw how that worked for pilots working with automation in cockpits. Second, it’s monitoring turtles all the way down. I don’t know if any of you know Shitty Robots. She just has this whole project. It’s amazing. Human operators still have to make sure that the automation is doing what it’s supposed to be doing. Even if they don’t necessarily have access to exactly what it’s doing or why it’s doing that. The automation has supposedly been put in place because it can do the job better, but then you have to be certain that it’s doing that job better than you. The paradox of not knowing what to do or knowing what to look for, these systems are generally impenetrable to that real-time inspection of what it is they’re doing.
Then, the worst part of this is that when that automation does fail and the human who was supposed to be monitoring it does have to take over, they often lack the knowledge or the context to do so. This is the Amy the Kafka expert example, writ large. It’s due to the fact that proper knowledge of how a system works and then therefore how the automation within it works, requires frequent usage of and experience with that system. Now you’ve been put over here and the automation is over here, so how do you have experience with that system? When you have to come in and deal with that, you actually are at a disadvantage, given that.
This is the irony three that’s leading into the irony three. When it does fail, the scale and the expertise and the knowledge required for the tasks to deal with that is often much larger and much more complex than humans who have no experience with that stuff. They don’t really necessarily know what to do. This is one of my favorite all time. It was like the food cart for the airport. It was just going crazy. This one guy finally just rolled up and bulldozed his whatever cart thing into it. It creates new types of work and it often leaves humans left to cope with a situation that’s actually almost worse or more complex than the one that was supposed to have the automation in there to do that work for them in the first place. The last one, this is Homer. This is the power plant one. He’s like, it’s as big as a phone book, and he’s supposed to be reading the instructions.
A lot of times we’re told, don’t worry about that, but when things go wrong, just use the runbook. Runbooks, all of those things can’t cover all the possibilities of the things that the designers couldn’t imagine when they were designing the automation and writing the runbooks about it. As we all know, they’re often not updated regularly. Again, the human is supposed to monitor the monitoring or the automation and then fill in the gaps. We’ve taken them out of that situation and told, go do something else. To debug a system with automation in it, you need to know the system overall, but what the automation is doing and how it’s doing it. You can see how this whole thing starts to collapse on itself and become fundamentally more complex than anybody intended. This is the quote, my favorite Bainbridge quote, “The more advanced a control system is, the more crucial may be the contribution of the human operator”.
Research from The VOID (Thematic Analysis)
I thought to myself, I probably have some data on this stuff. I’ve got over 10,000 incident reports with lots of words in them. As I had mentioned in the past, I had done a lot of qualitative research from incident reports in The VOID. I’ve looked at reports on duration and severity and the relationships between all of those things. This time I decided to take a different tack. The work that I did on the data I could find in The VOID falls under a category of research called thematic analysis. This is something that’s very prevalent and common in especially social sciences and also in areas that just have a huge amount of unstructured data, also known as text. 10,000 something incident reports with people talking about what went wrong and what happened. This is the fancy pants diagram, but the idea is you read all of the data that you can, and then you’re going to code those data.
Then you’re going to look at the codes, go back, revisit that again. Does this fit? Does this not fit? You’re creating your own model right here. Then eventually you look at those again, and those start to cohere, hopefully coalesce into themes. You can think of codes almost as like tags for similar items of text, not necessarily just individual words, but conceptually similar things. Then, as you roll those up to themes, those capture hopefully a prominent aspect of the data in some way. This is something, like I said, that’s done a lot in sociology work, anthropology, but also not just social sciences, but large bodies of unstructured text. I did not use a large language model to help me do this. Irony, I know.
Here’s what I did have to deal with. Over 10,000 incident reports and me. I did this work. I did not have, I wish, a round of lots of folks to help me with this. I’ll explain why I didn’t use a large language model. I had to get through, how do I get 10,000 down to a number that I can manage to sift through myself? Also, not every report in there is going to talk about automation. I had to find the ones where when people write up a report, they actually talk about that. Some of that was easy to knock away. All of the ones that were like media articles, like, no, the Facebook went down.
Those were not necessarily included out of the gate. In the end, what I did was I did a search query through all of the data based on keywords that I solicited from experts in their field. Folks who have deep, dark experience with their systems going down involving automation. I was like, what keywords should I include to find things that might have had automation involved when things went sideways? This is how we ended up with things like automation, automated, obviously. Like load balancing, self-healing, retry storms, these kinds of things. There’s probably more. The idea was to get a sample of the population.
If any of you ever took your psychology or social sciences class, hopefully that will make some sense. We’re not going to look at everything, but we’re going to look at a subset of it, assuming that it’s a pretty good representation of the larger whole. We took this 10,000-plus set of incident reports, and that query set reduced it down to just shy of about 500. Then, the next thing I had to do was actually read all of those. The good news was I had actually read a lot of them, but then I had to go back and read them very carefully and start looking for this.
Here’s the biggest reason why I didn’t use a large language model or other automated forms of doing this, as it requires expertise with the subject matter to a degree that you could read something and be like, that was an automated process. This gave me a set to look at, but I still even whittled some of them out. There were things where tooling that automates stuff broke, but that wasn’t actually the cause of the incident. There were incident reports for like Chef’s automated something, something broke for Chef, and they’re like, this happened. If that makes sense, I had to whittle that down. You couldn’t just turn a large language model at this and expect it to have that level of expertise. Maybe they will get there.
Right now, I can tell you that it requires a human to look at and read all of these to start doing this coding. I did an initial pass, and then, I didn’t really know what I was looking for. I was just looking for incidents that looked like they had automation in them. This is the way this process works. You do this, you keep reading, you start to notice patterns, you start to notice things that share similar effects, or context, or what have you. The codes start to develop from there. As I started to get what looked like a set of codes of automation, which I’ll show you, I went back and re-read everything again. Was this right? Did I see that again? There was a lot of reading, re-reading, refining.
The only thing that I did not do in this process that I would love to be able to do at some point or go back and do again is from a purely academic perspective, if you were to try to go publish this in an academic journal, I would have had a set of other coders, other reviewers come through and look at and decide if those codes actually were what I said they were and they were showing up in the way that I said. I would have a score of interviewer reliability. For full transparency and for any academic nerds, I didn’t do that. I do hope to.
What did we find? These are the set of codes that came out of this work. Then, here’s a fun pro tip. If you were working in an environment where people demand quantitative numbers, you can take qualitative work like this and you can put a number on it. If you have to give somebody a number, this is the way you do that. This is the literal methodology for doing that. You go read a bunch of stuff, you set the codes to it and you say, 77% of those codes had to do with automation being a contributing factor in the incident. We’ll walk through these just a little bit more here. These don’t add up to 100. The reason they don’t add up to 100 is because multiple codes could be present in a given incident. This isn’t supposed to be a pie chart where one thing is only one piece of it. That’s actually going to become important shortly. The vast majority of the time, automation was a part of the problem and it took humans to solve it. Those are the first two pieces of that.
Automation in some way, shape, or form was one of the reasons why that incident happened, potentially, definitely usually more than one. There is no root cause. Then, humans had to come in most equally as often, three-fourths of the time, to try to resolve that situation. Here’s the other codes that came out of this. Automation, work on automation, development of automation, fixing automation, adding more automation, that’s an action item code. When automation came up as an action item in the incident report, that’s where that came from. Detection. I have problems with my own self with this one because I think automation being involved in detecting incidents is a lot higher.
The only way I could do that, if somebody said, our automated monitoring system picked up an issue, and if you don’t tell me that, then I don’t know. I honestly suspect that we detect a lot of incidents because of automation. I think that number is maybe not the most reflective, but I think it’s also just the nature of the way people write incident reports. They’re three or four degrees removed, a public incident report, from what actually happened. A lot is assumed and a lot is not said. The other two places, the other two codes for automation in software incidents in The VOID was that it was actually part of the solution or maybe even it was the solution. It’s very small where automation detected the problem, automation had caused the problem, and automation solved it. It’s like very small. I think there were two. They were really happy about that, the folks that found those.
Then, the last one is when automation would hinder the remediation. How many of you have had this experience? Yes, this one’s fun. I call this one the gremlin. They all have their own little names and personas. This is the set of the ways in which automation might be involved. These were the codes and the quantitative summation of those codes. This is the one. It’s Dave and HAL. It’s hidden behind the text. If you remember one thing, it’s this, 75% of the time, you all still have to come in and fix something when automation makes it a lot harder.
Automation Themes
These are the themes then that came out of all of that. The first one, and this was what I was trying to get at in that slide where the numbers don’t add up to 100, is that automation can and often does play multiple roles in software incidents. It’s not nearly as clear cut as we want to imagine it as when we design it and the things that it will do.
Then when it doesn’t work as designed or intended, it often is part of the problem, requires humans, and it frequently becomes a contributing factor without the ability to resolve those independently. That’s the first big theme. The second one is that automation can unexpectedly make things worse. Not only does it contribute to, but it behaves in ways that make incidents worse. This can take the form of things like retry storms, interference with access to automated logs or data, unexpected interactions with other components that then expand the surface area of the incident. It could mean you can’t get into your database building. Facebook, that was a fun one. It can really show up in incredibly unexpected ways and ways that then require a lot more work and effort to try to figure out while you’re in the midst of trying to resolve an incident.
Then, this was the biggest one. Humans are still essential in automated systems. We still have to be there, not just to do the work that we do day-to-day to make those systems work, but to help solve problems and figure out what to do when the thing that was supposed to do our work stops being able to do that work for us.
Better Automation Through Joint Cognitive Systems
That’s really not very optimistic and fun, you say, and I know. I like to believe that there is hope. Despite all of these challenges and the ways that I’ve brought up that automation can be a total pain in our butts, it’s not going away. There are ways that we have found it to be beneficial. As I said, I’m not anti-automation. I’m really just more pro people. What can you all do? What can developers, DevOps folks, site reliability engineers, but especially people who are building and designing automation and AI tooling, what can you do? I’m advocating for a paradigm shift, an adjustment to our mental models, as I said at the beginning. Instead of designing and perceiving automation and AI as replacements for things that humans aren’t as good at, we should view it as a means to augment and support and improve human work. The goal is to transform automation from an unreliable adversary, a bad coworker into a collaborative team player. The fancy pants academic research term for that is joint cognitive systems. Let’s talk a little bit more about that.
I’m going to talk through this just really briefly. As I’ve mentioned, automation often ends up being a bad team player, because we’ve fallen prey to these Fitts style conceptualizations of automation. Failing to realize that it adds to system complexity and coupling and all these other things, while transforming the work we have to do and forcing us to adapt our skills and routines. David Woods and some of his colleagues have proposed an alternate approach or an alternate set of capabilities and ways of thinking about working with machines, this is their un-Fitts List. It emphasizes how the competencies of humans and machines can be enhanced through intentional forms of mutual interaction. Instead of, you’re better at this and I’m better at that, and we’re going to just go do these things separate from each other, we’re going to enhance the things that we do intentionally and thinking about that as mutual interaction, joint cognitive systems.
I think that the biggest one that I just really want to point out about this, and then I talked a little bit about this at the beginning, is the bottom one really. Machines aren’t aware of the fact that the model of the world is itself their model. It’s all this fancy stuff around ontology but in the way that machines model the world that we give them and the way that we model the world that we exist in with them. Starting to rethink what that looks like versus you go do this and I’ll go do that, and other things. That’s the machine side on the left and the people side on the right. We have all these other abilities and skills that we’re not limited in in the way that machines are constrained. We have high context, a lot of knowledge and attention driven tasks that we do. We’re incredibly adaptable to change, and typically because we have so much context expertise, we can recognize an anomaly in those systems really easily. We have this different ontological view of the system than machines do.
Text stuff number two. The paper is called, 10 Challenges for Making Automation a Team Player in Joint Human-Agent Activity. This stuff was written a little while ago but the words agents still work in this context if you think of them as machines, computers, automation, what have you. They make an argument, a case for what characteristics you need to have a joint cognitive system that supports this work that we do with machines. They provide 10 challenges. I always get asked, what are the most important of these that I think we should focus on? Here they are. To be an effective team player, intelligent agents must be able to adequately model the other participants’ intentions and actions vis-a-vis the joint activity state, vis-a-vis what you’re trying to do, aka establishing common ground.
If you think about the way you work with team members, let’s say in an incident or in trying to figure out some complex design of a system or something like that, you have to make sure that you understand each other’s intentions, that you have common ground of what it is you’re trying to do and how you think you should get there. This is a really important part about how joint cognitive systems, whether it’s your brain and my brain or my brain and a computer brain actually successfully work together, not how they necessarily currently work together.
The second one is that us and our agent friends, our computers, our automated systems must be mutually predictable. How many of you feel like your automated systems are mutually predictable? The last one is that agents must be directable. When we have goal negotiation, which is further down here, number seven, if we’re going to have goal negotiation, then one of the other of us has to be able to say, “I’m going to do that, you do that”. That’s not usually the case with our automated systems. Not currently. It’s the capacity to deliberately assess and modify your actions in a joint activity.
Conclusion
This is the set of references. I just want to conclude by begging people who are designing automated systems, who are working towards AI in especially developer tooling environments where the complexity is incredibly high, to really dive into this stuff. Take this work seriously. This is research that has changed the way that healthcare systems work, that nuclear power plants work, that airplane cockpits that we fly in every day work. That is my challenge to you and my hope that we can rethink the way that we work with automated and artificial intelligence systems that is mutually beneficial and helps make the work we do better.
Questions and Answers
Participant 1: Have you seen any examples in all of the incidents that you’ve looked at of companies adapting in the way that you’ve recommended they adapt?
Nash: I would argue almost exclusively no. Not that they’re not adapting, because we know that adaptive capacity in these systems is what we do and how we manage these things, but because they don’t write about it. It’s a really important distinction. I started this talk off with an example from an incident report from Honeycomb, because I consider that, and the work that Fred Hebert does, analyzing and writing up their incidents to be almost exclusively the gold standard high bar of doing that. There are organizations that do adapt and learn, and they don’t talk about it in their incident reports. They talk about the action items and the things that they do. Honeycomb, a few others, talk about the adaptations. They talk about what they learn, both about their technical systems and their sociotechnical systems. The short answer is no. I wish people would write about that more, because if I could study that, I would be a really happy camper.
Participant 2: I work in building automated tools, and I have colleagues who work in building automated tools. The thing that really makes me just slam my forehead down on my desk every so often is the one where we have an outage or an incident because an automated tool presented a change nominally to the humans who were going to review the change, and the human went, the tool knows what it’s doing, and they rubber stamp it. It looks ok, but they’ve lost the expertise to actually understand the impact of that particular config change or whatever it was. There doesn’t seem to be a common pattern of saying, other people wrote this tool. They are just software developers like you, and this thing might have bugs. This is before you put AI in it.
Nash: Yes, not even bugs, you may not know the extent of what it does, and you don’t know all the conditions it’s exposed to.
Participant 2: Correct. Given that, in terms of this compact idea, like giant flashing lights saying, don’t trust me, I’m trying to help you, but I could be wrong, would be great. How does that actually happen from what you’ve seen?
Nash: The other question I commonly get is, what examples have you seen of this? The answer is none, because no one’s tried to really do this yet, that I know of. I don’t know what that is, because it’s generally very context-specific. The goal would be for the tool, the automation, to tell you, this is about to happen, and here’s the set of things that I expect to happen when that happens, or here’s what we’re expecting to see. That’s part of the being mutually predictable and then being directable. Yes, do that, don’t do that, some of that. I think, giant warning lights, I might be wrong, but I’m trying to help you. That’s also what our colleagues are like. It’s being able to introspect what it is that the tool is trying to do. No, I don’t just mean documentation. I mean the way that we interact with that thing.
The other thing I do want to bring up is something you mentioned, which is another term in the industry that comes up a lot in autonomous driving situations is automation complacency. I didn’t talk about that in here, it wasn’t in this set of research that I brought to this talk. It is also this notion that as we say, “Automation will just do this stuff for you”, then you’re like, “I’m going to not worry about that anymore”. It’s not just the lack of expertise with the system, it’s like, no, I trust that to do that, you become complacent. This is how accidents happen in a self-driving car situation, but it’s also a phenomenon within these systems as well, and like, “Yes. No, don’t worry about that red light, that one comes on all the time”. It’s in that same sphere of influence.
The biggest thing I would ask for people designing these is, when some form of automation is going to do something that it could give you as much possible information about what it’s going to do and what the consequences of that might be, and then you get to have some thought about and input into what that would look like.
Participant 3: I am working in the AI agent space, so I am dealing with this stuff every day. I think among the pillars of the joint collaboration that you listed, don’t you think that the mutual predictability is somehow the most problematic one because actually it has to do with the tooling test in some sense? You are assuming basically that you are predictable for the machine and the machine is predictable for you. It’s a loop. You know that there are some advanced LLMs which started to fake it in some extent in order to escape from your expectations. That’s something which is about a mutual trust. I see it personally as a problematic point.
Nash: It is. Some of these aren’t as separable. You can’t just be like, I’ll do this one thing. If you’re mutually predictable, if something’s not predictable enough, you have to be able to engage in goal negotiation. Then other things here, like observe and interpret signals of status and intentions. When the predictability isn’t there, what other avenues do you have to try to understand what’s going to happen? The goal is to be mutually predictable, but even we’re not all mutually predictable with each other.
Participant 3: Also, maybe engaging in a goal negotiation is not enough. Maybe you should be able to assess the results of the goal negotiation to say, ok, we understood the same thing. That’s really challenging.
Participant 4: A lot of this puts in mind something many of you have possibly seen before. It’s an IBM presentation slide from about half a century ago, which reads, a computer must never make a management decision because a computer can never be held accountable. Or as a friend of mine framed it to me, a computer must never fuck around because a computer can never find out.
Nash: Accurate.
Participant 4: It seems to me, someone I’ll admit as being a little bit of an AI pessimist or whatever, that there are a lot of cases where that lack of accountability is really more of a feature than it is a bug. Even less of a pessimistic framing, there’s a lot of instances of like AI boosterism where a complete lack of human involvement being necessary is touted as a goal rather than something concerning about it. Do you have any insights or input on how we can apply this framework or paradigm shift you’re talking about in cases where bringing up those sorts of concerns are very much not wanted?
Nash: Get venture capitalism out of tech? All I have are spicy takes on this one. Like late-stage capitalism is a hell of a drug. As long as our priorities are profit over people, we will always optimize towards what you’re discussing. The goal is to fight back in that locally as much as we can and where we can, which is why especially I make the appeal not so much to people who are trying to make broad-based cultural AI things, but people who are building tooling for developers in this space who then build things that impact our world so heavily to care about this stuff. Capitalism’s hard, but hopefully locally folks could care about this a bit more and improve the experience for us.
See more presentations with transcripts
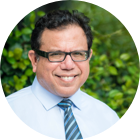
MMS • RSS
Posted on mongodb google news. Visit mongodb google news
Tairen Capital Ltd purchased a new position in shares of MongoDB, Inc. (NASDAQ:MDB – Free Report) during the fourth quarter, according to the company in its most recent disclosure with the Securities & Exchange Commission. The firm purchased 49,900 shares of the company’s stock, valued at approximately $11,617,000. MongoDB accounts for about 1.7% of Tairen Capital Ltd’s investment portfolio, making the stock its 18th largest position. Tairen Capital Ltd owned approximately 0.07% of MongoDB at the end of the most recent reporting period.
Other large investors also recently bought and sold shares of the company. Strategic Investment Solutions Inc. IL purchased a new position in MongoDB during the fourth quarter worth approximately $29,000. NCP Inc. purchased a new position in shares of MongoDB in the 4th quarter valued at $35,000. Coppell Advisory Solutions LLC raised its stake in shares of MongoDB by 364.0% in the 4th quarter. Coppell Advisory Solutions LLC now owns 232 shares of the company’s stock valued at $54,000 after acquiring an additional 182 shares during the period. Smartleaf Asset Management LLC raised its stake in shares of MongoDB by 56.8% in the 4th quarter. Smartleaf Asset Management LLC now owns 370 shares of the company’s stock valued at $87,000 after acquiring an additional 134 shares during the period. Finally, Manchester Capital Management LLC raised its stake in shares of MongoDB by 57.4% in the 4th quarter. Manchester Capital Management LLC now owns 384 shares of the company’s stock valued at $89,000 after acquiring an additional 140 shares during the period. Hedge funds and other institutional investors own 89.29% of the company’s stock.
Insider Activity at MongoDB
In related news, CEO Dev Ittycheria sold 8,335 shares of MongoDB stock in a transaction dated Wednesday, February 26th. The shares were sold at an average price of $267.48, for a total value of $2,229,445.80. Following the sale, the chief executive officer now directly owns 217,294 shares in the company, valued at $58,121,799.12. This trade represents a 3.69% decrease in their position. The sale was disclosed in a document filed with the SEC, which is available through this hyperlink. Also, CAO Thomas Bull sold 301 shares of MongoDB stock in a transaction dated Wednesday, April 2nd. The shares were sold at an average price of $173.25, for a total transaction of $52,148.25. Following the completion of the sale, the chief accounting officer now owns 14,598 shares in the company, valued at approximately $2,529,103.50. This represents a 2.02% decrease in their ownership of the stock. The disclosure for this sale can be found here. Insiders have sold a total of 34,423 shares of company stock worth $7,148,369 in the last ninety days. 3.60% of the stock is owned by insiders.
Wall Street Analysts Forecast Growth
A number of equities analysts have recently commented on MDB shares. Redburn Atlantic raised shares of MongoDB from a “sell” rating to a “neutral” rating and set a $170.00 target price on the stock in a research note on Thursday, April 17th. Scotiabank reissued a “sector perform” rating and issued a $160.00 target price (down previously from $240.00) on shares of MongoDB in a research note on Friday, April 25th. The Goldman Sachs Group cut their target price on shares of MongoDB from $390.00 to $335.00 and set a “buy” rating on the stock in a research note on Thursday, March 6th. Rosenblatt Securities reaffirmed a “buy” rating and set a $350.00 price target on shares of MongoDB in a research note on Tuesday, March 4th. Finally, KeyCorp downgraded shares of MongoDB from a “strong-buy” rating to a “hold” rating in a research note on Wednesday, March 5th. Eight investment analysts have rated the stock with a hold rating, twenty-four have assigned a buy rating and one has assigned a strong buy rating to the stock. According to MarketBeat.com, the stock has a consensus rating of “Moderate Buy” and a consensus price target of $293.91.
Get Our Latest Research Report on MDB
MongoDB Stock Performance
NASDAQ MDB opened at $191.29 on Friday. MongoDB, Inc. has a one year low of $140.78 and a one year high of $379.06. The company has a 50-day simple moving average of $174.91 and a 200-day simple moving average of $238.95. The firm has a market capitalization of $15.53 billion, a PE ratio of -69.81 and a beta of 1.49.
MongoDB (NASDAQ:MDB – Get Free Report) last posted its quarterly earnings results on Wednesday, March 5th. The company reported $0.19 EPS for the quarter, missing the consensus estimate of $0.64 by ($0.45). MongoDB had a negative net margin of 10.46% and a negative return on equity of 12.22%. The business had revenue of $548.40 million during the quarter, compared to analyst estimates of $519.65 million. During the same period last year, the firm posted $0.86 earnings per share. Equities analysts forecast that MongoDB, Inc. will post -1.78 earnings per share for the current year.
MongoDB Profile
MongoDB, Inc, together with its subsidiaries, provides general purpose database platform worldwide. The company provides MongoDB Atlas, a hosted multi-cloud database-as-a-service solution; MongoDB Enterprise Advanced, a commercial database server for enterprise customers to run in the cloud, on-premises, or in a hybrid environment; and Community Server, a free-to-download version of its database, which includes the functionality that developers need to get started with MongoDB.
Read More
Want to see what other hedge funds are holding MDB? Visit HoldingsChannel.com to get the latest 13F filings and insider trades for MongoDB, Inc. (NASDAQ:MDB – Free Report).
This instant news alert was generated by narrative science technology and financial data from MarketBeat in order to provide readers with the fastest and most accurate reporting. This story was reviewed by MarketBeat’s editorial team prior to publication. Please send any questions or comments about this story to contact@marketbeat.com.
Before you consider MongoDB, you’ll want to hear this.
MarketBeat keeps track of Wall Street’s top-rated and best performing research analysts and the stocks they recommend to their clients on a daily basis. MarketBeat has identified the five stocks that top analysts are quietly whispering to their clients to buy now before the broader market catches on… and MongoDB wasn’t on the list.
While MongoDB currently has a Moderate Buy rating among analysts, top-rated analysts believe these five stocks are better buys.
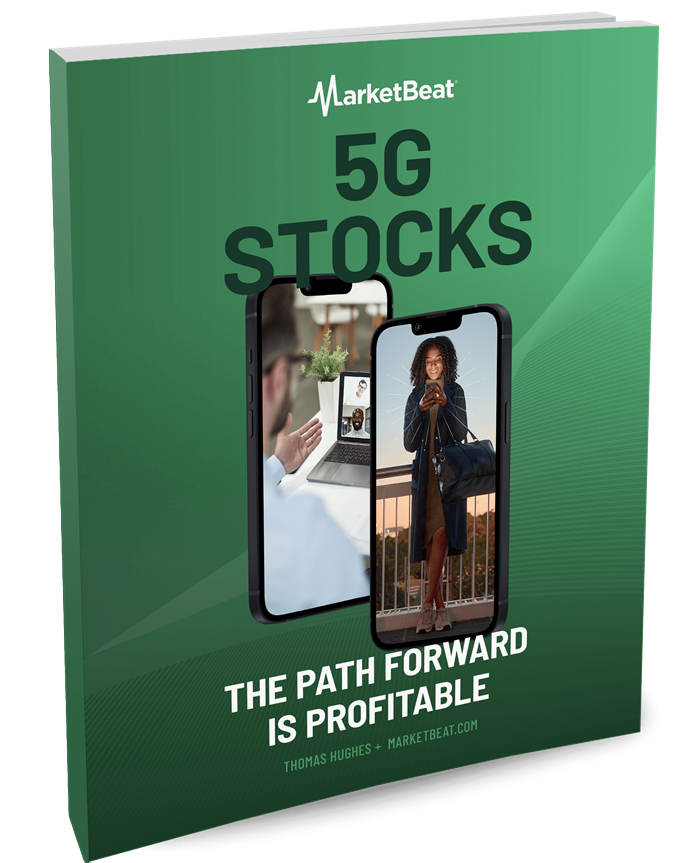
Enter your email address and we’ll send you MarketBeat’s guide to investing in 5G and which 5G stocks show the most promise.
Article originally posted on mongodb google news. Visit mongodb google news
Spring AI 1.0 Released, Streamlines AI Application Development with Broad Model Support
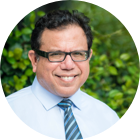
MMS • A N M Bazlur Rahman
Article originally posted on InfoQ. Visit InfoQ
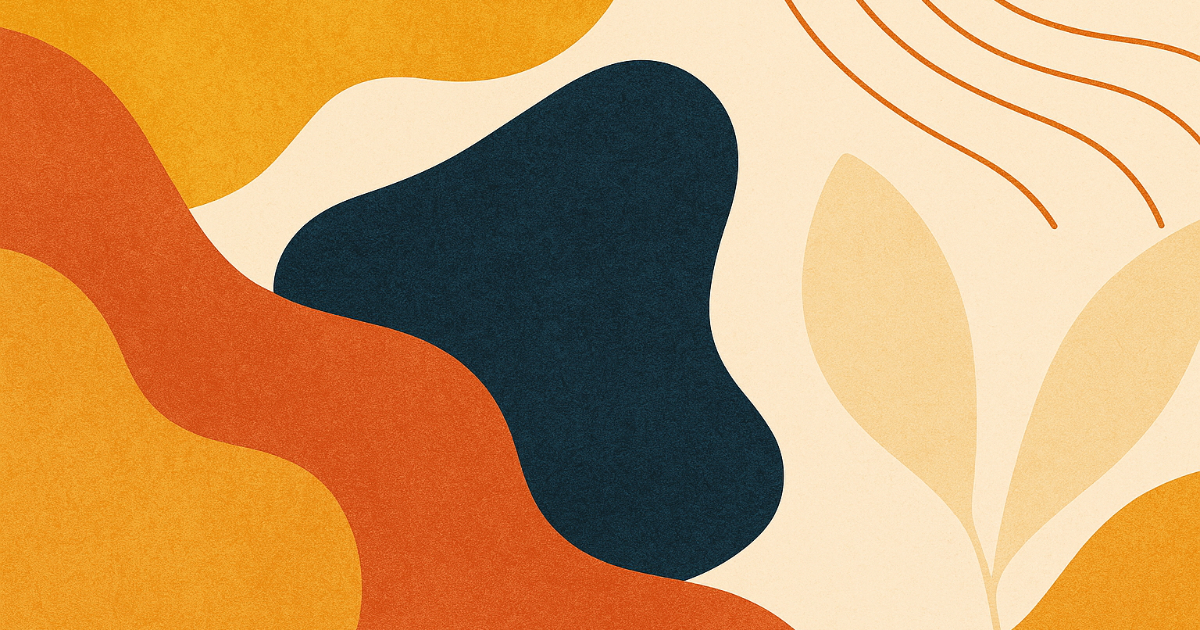
The Spring team has announced the general availability of Spring AI 1.0, a framework designed to simplify the development of AI-driven applications within the Java and Spring ecosystem. This release, the result of over two years of development and eight milestone iterations, delivers a stable API. It integrates with a wide range of AI models for chat, image generation, and transcription. Key features include portable service abstractions, support for Retrieval Augmented Generation (RAG) via vector databases, and tools for function calling. Spring AI 1.0 enables developers to build scalable, production-ready AI applications by aligning with established Spring patterns and the broader Spring ecosystem.
Spring AI provides out-of-the-box support for numerous AI models and providers. The framework integrates with major generative AI providers, including OpenAI, Anthropic, Microsoft Azure OpenAI, Amazon Bedrock, and Google Vertex AI, through a unified API layer. It supports various model types across modalities, including chat completion, embedding, image generation, audio transcription, text-to-speech synthesis, and content moderation. This enables developers to integrate capabilities such as GPT-based chatbots, image creation, or speech recognition into Spring applications.
The framework offers portable service abstractions, decoupling application code from specific AI providers. Its API facilitates switching between model providers (e.g., from OpenAI to Anthropic) with minimal code changes, while retaining access to model-specific features. Spring AI supports structured outputs by mapping AI model responses to Plain Old Java Objects (POJOs) for type-safe processing. For Retrieval Augmented Generation (RAG), Spring AI integrates with various vector databases, including Cassandra, PostgreSQL/PGVector, MongoDB Atlas, Milvus, Pinecone, and Redis, through a consistent Vector Store API, enabling applications to ground LLM responses in enterprise data. The framework also includes support for tools and function calling APIs, allowing AI models to invoke functions or external tools in a standardized manner to address use cases like “Q&A over your documentation” or “chat with your data.”
Spring AI 1.0 includes support for the Model Context Protocol (MCP), an emerging open standard for structured, language-agnostic interaction between AI models (particularly LLMs) and external tools or resources. The Spring team has contributed its MCP implementation to ModelContextProtocol.io
, where it serves as an official Java SDK for MCP services. This reflects Spring AI’s focus on open standards and interoperability.
To facilitate MCP integration, Spring AI provides dedicated client and server Spring Boot starters, enabling models to interact with tools like this example weather service:
import org.springframework.ai.tool.annotation.Tool;
import org.springframework.stereotype.Component;
@Component
public class WeatherTool {
@Tool(name = "getWeather", description = "Returns weather for a given city")
public String getWeather(String city) {
return "The weather in " + city + " is 21°C and sunny.";
}
}
These starters are categorized as follows:
- Client Starters:
spring-ai-starter-mcp-client
(providing core STDIO and HTTP-based SSE support) andspring-ai-starter-mcp-client-webflux
(offering WebFlux-based SSE transport for reactive applications). - Server Starters:
spring-ai-starter-mcp-server
(for core STDIO transport support),spring-ai-starter-mcp-server-webmvc
(for Spring MVC-based SSE transport in servlet applications), andspring-ai-starter-mcp-server-webflux
(for WebFlux-based SSE transport in reactive applications).
Developers can begin new Spring AI 1.0 projects using Spring Initializr, which preconfigures necessary dependencies. Including the desired Spring AI starter on the classpath allows Spring Boot to auto-configure the required clients or services.
An example of a simple chat controller is as follows:
import org.springframework.ai.chat.client.ChatClient;
import org.springframework.web.bind.annotation.GetMapping;
import org.springframework.web.bind.annotation.RequestParam;
import org.springframework.web.bind.annotation.RestController;
@RestController
public class ChatController {
private final ChatClient chatClient;
public ChatController(ChatClient.Builder chatClientBuilder) {
this.chatClient = chatClientBuilder.build();
}
@GetMapping("/ask")
public String ask(@RequestParam String question) {
return chatClient.prompt()
.user(question)
.call()
.content();
}
}
At a minimum, the following key-value in application.properties
is necessary to run the above example.
spring.ai.openai.api-key=YOUR_API_KEY
spring.ai.openai.chat.model=gpt-4
Spring AI introduces higher-level APIs for common AI application patterns. A fluent ChatClient
API offers a type-safe builder for chat model interactions. Additionally, an Advisors
API encapsulates recurring generative AI patterns such as retrieval augmentation, conversational memory, and question-answering workflows. For instance, a RAG flow can be implemented by combining ChatClient
with QuestionAnswerAdvisor
:
ChatResponse response = ChatClient.builder(chatModel)
.build()
.prompt()
.advisors(new QuestionAnswerAdvisor(vectorStore))
.user(userText)
.call()
.chatResponse();
In this example, QuestionAnswerAdvisor
performs a similarity search in the VectorStore
, appends relevant context to the user prompt, and forwards the enriched input to the model. An optional SearchRequest
with an SQL-like filter can constrain document searches.
The release incorporates Micrometer for observability, allowing developers to monitor AI-driven applications. These integrations facilitate embedding AI capabilities into Spring-based projects for various applications, including real-time chat, image processing, and transcription services.
For more information, developers can explore the Spring AI project page or begin building with Spring AI at start.spring.io
. This release provides Java developers with a solution for integrating AI capabilities, offering features for scalability and alignment with idiomatic Spring development.
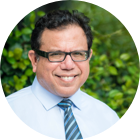
MMS • RSS
Posted on mongodb google news. Visit mongodb google news
Loop Capital has revised its rating on MongoDB (MDB, Financial), moving it from a Buy to a Hold, and adjusted the price target downwards to $190 from $350. Recent evaluations suggest that the Atlas platform by MongoDB is seeing underwhelming market adoption. This trend of deceleration in platform use is likely to persist, potentially hindering the growth of artificial intelligence workloads on Atlas, as per the firm’s analysis.
Loop Capital foresees continued slowing in the consumption growth of Atlas until MongoDB demonstrates advancements in reaching its current market goals, particularly in boosting its presence among large enterprise customers. The firm also notes that the cloud database platform market, which MongoDB targets, remains fragmented and has not consolidated around leading vendors.
Wall Street Analysts Forecast
Based on the one-year price targets offered by 34 analysts, the average target price for MongoDB Inc (MDB, Financial) is $273.14 with a high estimate of $520.00 and a low estimate of $160.00. The average target implies an
upside of 44.51%
from the current price of $189.01. More detailed estimate data can be found on the MongoDB Inc (MDB) Forecast page.
Based on the consensus recommendation from 37 brokerage firms, MongoDB Inc’s (MDB, Financial) average brokerage recommendation is currently 2.0, indicating “Outperform” status. The rating scale ranges from 1 to 5, where 1 signifies Strong Buy, and 5 denotes Sell.
Based on GuruFocus estimates, the estimated GF Value for MongoDB Inc (MDB, Financial) in one year is $438.57, suggesting a
upside
of 132.04% from the current price of $189.01. GF Value is GuruFocus’ estimate of the fair value that the stock should be traded at. It is calculated based on the historical multiples the stock has traded at previously, as well as past business growth and the future estimates of the business’ performance. More detailed data can be found on the MongoDB Inc (MDB) Summary page.
MDB Key Business Developments
Release Date: March 05, 2025
- Total Revenue: $548.4 million, a 20% year-over-year increase.
- Atlas Revenue: Grew 24% year-over-year, representing 71% of total revenue.
- Non-GAAP Operating Income: $112.5 million, with a 21% operating margin.
- Net Income: $108.4 million or $1.28 per share.
- Customer Count: Over 54,500 customers, with over 7,500 direct sales customers.
- Gross Margin: 75%, down from 77% in the previous year.
- Free Cash Flow: $22.9 million for the quarter.
- Cash and Cash Equivalents: $2.3 billion, with a debt-free balance sheet.
- Fiscal Year 2026 Revenue Guidance: $2.24 billion to $2.28 billion.
- Fiscal Year 2026 Non-GAAP Operating Income Guidance: $210 million to $230 million.
- Fiscal Year 2026 Non-GAAP Net Income Per Share Guidance: $2.44 to $2.62.
For the complete transcript of the earnings call, please refer to the full earnings call transcript.
Positive Points
- MongoDB Inc (MDB, Financial) reported a 20% year-over-year revenue increase, surpassing the high end of their guidance.
- Atlas revenue grew 24% year over year, now representing 71% of total revenue.
- The company achieved a non-GAAP operating income of $112.5 million, resulting in a 21% non-GAAP operating margin.
- MongoDB Inc (MDB) ended the quarter with over 54,500 customers, indicating strong customer growth.
- The company is optimistic about the long-term opportunity in AI, particularly with the acquisition of Voyage AI to enhance AI application trustworthiness.
Negative Points
- Non-Atlas business is expected to be a headwind in fiscal ’26 due to fewer multi-year deals and a shift of workloads to Atlas.
- Operating margin guidance for fiscal ’26 is lower at 10%, down from 15% in fiscal ’25, due to reduced multi-year license revenue and increased R&D investments.
- The company anticipates a high-single-digit decline in non-Atlas subscription revenue for the year.
- MongoDB Inc (MDB) expects only modest incremental revenue growth from AI in fiscal ’26 as enterprises are still developing AI skills.
- The company faces challenges in modernizing legacy applications, which is a complex and resource-intensive process.
Article originally posted on mongodb google news. Visit mongodb google news
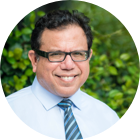
MMS • RSS
Posted on mongodb google news. Visit mongodb google news
Loop Capital has revised its rating for MongoDB (MDB, Financial), moving it from a Buy to a Hold. Alongside this change, Loop Capital has established a price target of $190 for the stock. This adjustment reflects the analysts’ current assessment of the company’s valuation and market conditions.
Wall Street Analysts Forecast
Based on the one-year price targets offered by 34 analysts, the average target price for MongoDB Inc (MDB, Financial) is $273.14 with a high estimate of $520.00 and a low estimate of $160.00. The average target implies an
upside of 44.51%
from the current price of $189.01. More detailed estimate data can be found on the MongoDB Inc (MDB) Forecast page.
Based on the consensus recommendation from 37 brokerage firms, MongoDB Inc’s (MDB, Financial) average brokerage recommendation is currently 2.0, indicating “Outperform” status. The rating scale ranges from 1 to 5, where 1 signifies Strong Buy, and 5 denotes Sell.
Based on GuruFocus estimates, the estimated GF Value for MongoDB Inc (MDB, Financial) in one year is $438.57, suggesting a
upside
of 132.04% from the current price of $189.01. GF Value is GuruFocus’ estimate of the fair value that the stock should be traded at. It is calculated based on the historical multiples the stock has traded at previously, as well as past business growth and the future estimates of the business’ performance. More detailed data can be found on the MongoDB Inc (MDB) Summary page.
MDB Key Business Developments
Release Date: March 05, 2025
- Total Revenue: $548.4 million, a 20% year-over-year increase.
- Atlas Revenue: Grew 24% year-over-year, representing 71% of total revenue.
- Non-GAAP Operating Income: $112.5 million, with a 21% operating margin.
- Net Income: $108.4 million or $1.28 per share.
- Customer Count: Over 54,500 customers, with over 7,500 direct sales customers.
- Gross Margin: 75%, down from 77% in the previous year.
- Free Cash Flow: $22.9 million for the quarter.
- Cash and Cash Equivalents: $2.3 billion, with a debt-free balance sheet.
- Fiscal Year 2026 Revenue Guidance: $2.24 billion to $2.28 billion.
- Fiscal Year 2026 Non-GAAP Operating Income Guidance: $210 million to $230 million.
- Fiscal Year 2026 Non-GAAP Net Income Per Share Guidance: $2.44 to $2.62.
For the complete transcript of the earnings call, please refer to the full earnings call transcript.
Positive Points
- MongoDB Inc (MDB, Financial) reported a 20% year-over-year revenue increase, surpassing the high end of their guidance.
- Atlas revenue grew 24% year over year, now representing 71% of total revenue.
- The company achieved a non-GAAP operating income of $112.5 million, resulting in a 21% non-GAAP operating margin.
- MongoDB Inc (MDB) ended the quarter with over 54,500 customers, indicating strong customer growth.
- The company is optimistic about the long-term opportunity in AI, particularly with the acquisition of Voyage AI to enhance AI application trustworthiness.
Negative Points
- Non-Atlas business is expected to be a headwind in fiscal ’26 due to fewer multi-year deals and a shift of workloads to Atlas.
- Operating margin guidance for fiscal ’26 is lower at 10%, down from 15% in fiscal ’25, due to reduced multi-year license revenue and increased R&D investments.
- The company anticipates a high-single-digit decline in non-Atlas subscription revenue for the year.
- MongoDB Inc (MDB) expects only modest incremental revenue growth from AI in fiscal ’26 as enterprises are still developing AI skills.
- The company faces challenges in modernizing legacy applications, which is a complex and resource-intensive process.
Article originally posted on mongodb google news. Visit mongodb google news
Article: RxJS Best Practices in Angular 16: Avoiding Subscription Pitfalls and Optimizing Streams
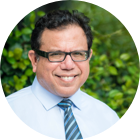
MMS • Shrinivass Arunachalam Balasubramanian
Article originally posted on InfoQ. Visit InfoQ
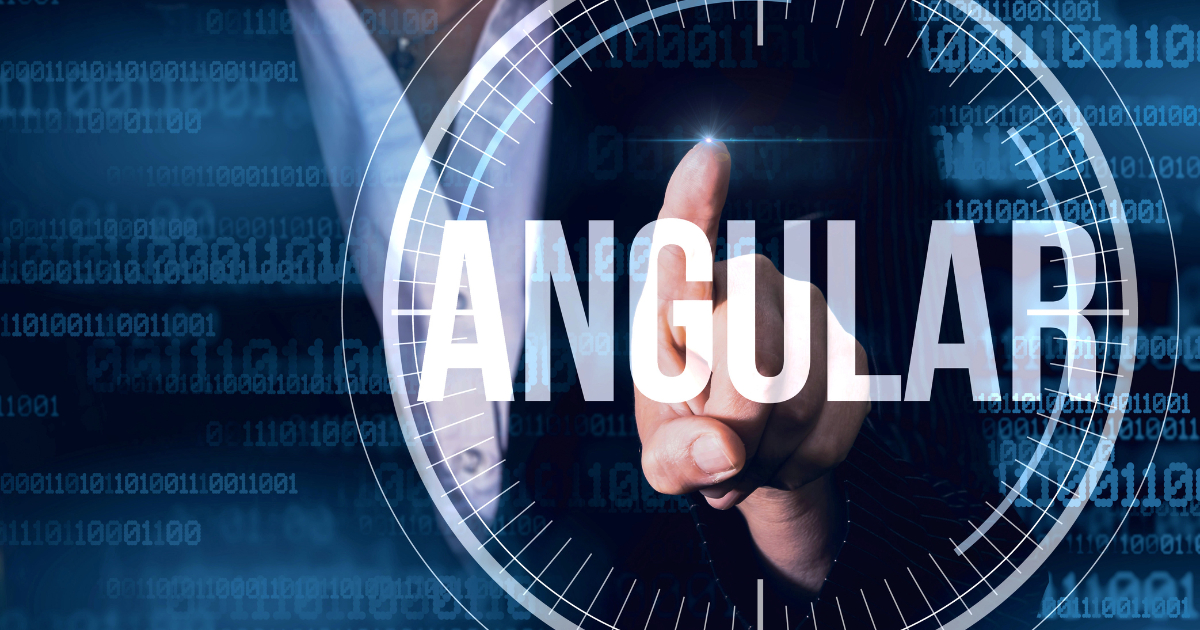
Key Takeaways
- Use AsyncPipe to handle observable subscriptions in templates. It manages unsubscriptions without the need for manual cleanup, thus preventing memory leaks.
- Favor flattening and combining streams over nesting streams. RxJS operators like switchMap, mergeMap, exhaustMap, or even debounceTime declaratively describe the desired dataflow and automatically manage subscription/unsubscription of their dependencies.
- Combine takeUntil with DestroyRef for clear subscription cleanup.
- Use catchError and retry to gracefully manage failure and recovery from failure
- Use Angular signals for updates triggered by the UI. For event streams, stick with RxJS observables. This combination helps you leverage both tools to their full potential.
Introduction
Angular 16 marks the introduction of the modern reactive Angular version, It introduces foundational tools like DestroyRef and signals. These new introductions have redefined how developers handle reactivity, lifecycle management and state updates, setting the stage for Angular 17/18 and beyond.
This article explores RxJS best practices focusing on the modern ecosystem and extending seamlessly to Angular 17/18, ensuring your code remains efficient and future proof.
The Evolution of RxJS Management in Angular
Before Angular 16, developers mostly relied on manual lifecycle management such as ngOnDestroy
and lacked native tools for lightweight reactivity. Angular 16’s DestroyRef and signals address this need for tools by abstracting cleanup logic and enabling granular state reactivity. Version 16 laid the groundwork for a modern reactivity paradigm, which has been further refined by Angular 17/18 without altering core principles.
DestroyRef is a game-changing tool that streamlines observable cleanup by abstracting lifecycle management. The introduction of this class marks the beginning of a modern reactive ecosystem, where developers can focus more on logic and less on boilerplate. Angular 17/18 further refines these patterns, such as improving signal-observable interoperability and enhancing performance optimizations. The best practices outlined here are developed for Angular 16, but they apply equally to Angular 17/18.
Similarly, while RxJS operators such as switchMap
and mergeMap
have long helped flatten nested streams, their proper use was often obscured by over-reliance on multiple, ad-hoc subscriptions. The goal now is to combine these techniques with Angular’s new capabilities, such as signals, to create reactive code that is both concise and maintainable.
Angular 16’s signals marks a turning point in state management, enabling lightweight reactivity without subscriptions. When combined with RxJS, they form a holistic reactive toolkit for modern angular application.
Best Practices
AsyncPipe
In the modern Angular ecosystem (starting with Angular 16), the AsyncPipe is the cornerstone of reactive UI binding. It automatically unsubscribes when components are destroyed, a feature critical for avoiding memory leaks. This pattern remains a best practice in Angular 17/18, ensuring your templates stay clean and reactive. Subscriptions and unsubscriptions can now be handled by the AsyncPipe without your intervention. This results in a much cleaner template and less boilerplate code.
For example, consider a component that displays a list of items:
- {{ item.name }}
When you use AsyncPipe to bind the observable to the template, Angular checks for updates. The component also cleans up when it destroys itself. This approach is beautiful due to its simplicity; you write less code and avoid memory leaks.
Flatten Observable Streams with RxJS Operators
For Angular developers, handling nested subscriptions is a common source of frustration. You may have encountered a situation in which a series of observables have to occur sequentially. RxJS operators like switchMap
, mergeMap
, and concatMap
offer a sophisticated alternative to nesting subscriptions within subscriptions, the latter of which leads to a complex issue in your code very quickly.
Imagine a search bar that retrieves potential plans as the user inputs. If you don’t have the right operators, you can wind up recording every keystroke. Instead, debounce input using a combination of operators, and when the user modifies their query, switch to a new search stream.
// plan-search.component.ts
import { Component, OnInit } from '@angular/core';
import { Subject, Observable } from 'RxJS';
import { debounceTime, distinctUntilChanged, switchMap } from 'RxJS/operators';
import { PlanService } from './plan.service';
@Component({
selector: 'app-plan-search',
template: `
- {{ plan }}
`
})
export class PlanSearchComponent implements OnInit {
private searchTerms = new Subject();
plans$!: Observable;
constructor(private planService: PlanService) {}
search(term: string): void {
this.searchTerms.next(term);
}
ngOnInit() {
this.plans$ = this.searchTerms.pipe(
debounceTime(300),
distinctUntilChanged(),
switchMap(term => this.planService.searchPlans(term))
);
}
}
Using operators this way flattens multiple streams into a single, manageable pipeline and avoids the need to manually subscribe and unsubscribe for every action. This pattern makes your code not only cleaner but also more responsive to user interactions.
Unsubscription and Error Handling
Letting observables run endlessly, which results in memory leaks, is one of the traditional anti-patterns in Angular. Having a good unsubscribe plan is essential. Although unsubscription is frequently handled by the AsyncPipe in templates, there are still situations in which TypeScript code requires explicit unsubscription. In certain situations, it might be quite beneficial to use operators like takeUntil
or Angular’s onDestroy
lifecycle hook.
For example, when subscribing to a data stream in a component:
import { Component, OnDestroy } from '@angular/core';
import { Subject } from 'rxjs';
import { takeUntil } from 'rxjs/operators';
import { DataService } from './data.service';
@Component({
selector: 'app-data-viewer',
template: ``
})
export class DataViewerComponent implements OnDestroy {
private destroy$ = new Subject();
constructor(private dataService: DataService) {
this.dataService.getData().pipe(
takeUntil(this.destroy$)
).subscribe(data => {
// handle data
});
}
ngOnDestroy() {
this.destroy$.next();
this.destroy$.complete();
}
}
Using operators like catchError
and retry
in conjunction with unsubscription strategies helps make sure that your application handles unforeseen errors with grace. By combining problem discovery with quick fixes, this integrated method produces code that is strong and maintainable.
Combining Streams
Often, you’ll need to merge the outputs of several observables. You can display data from various sources using operators such as combineLatest
, forkJoin
, or zip
. They help you merge streams with simplicity. This method keeps a reactive and declarative style. It also updates without manual intervention when one or more source streams change.
Imagine combining a user’s profile with settings data:
import { combineLatest } from 'rxjs';
combineLatest([this.userService.getProfile(), this.settingsService.getSettings()]).subscribe(
([profile, settings]) => {
// process combined profile and settings
}
);
This strategy not only minimizes complexity by avoiding nested subscriptions, but also shifts your mindset toward a more reactive, declarative style of programming.
Integrate Angular 16 Signals for Efficient State Management
While RxJS continues to play a pivotal role in handling asynchronous operations, Angular 16’s new signals offer another layer of reactivity that simplifies state management. Signals are particularly useful when the global state needs to trigger automatic updates in the UI without the overhead of observable subscriptions. For example, a service can expose a signal for the currently selected plan:
// analysis.service.ts
import { Injectable, signal } from '@angular/core';
@Injectable({ providedIn: 'root' })
export class AnalysisService {
currentPlan = signal('Plan A');
updateCurrentPlan(newPlan: string) {
this.currentPlan.set(newPlan);
}
}
By combining signals with RxJS streams in your components, you can enjoy the best of both worlds: a clean, declarative state management model alongside powerful operators to handle complex asynchronous events.
Signals vs.s Observables
Angular 16 contains RxJS Observables and Signals, which allow for reactive programming, but they serve different needs. Signals simplify UI state management, while Observables handle asynchronous operations. Signals are a core part of Angular 16’s modern reactivity model, designed for scenarios where UI state needs immediate updates (such as toggling a modal or theme)
Signals are lightweight variables that automatically update the UI when their value changes. For example, tracking a modal’s open/close functionality (isModalOpen = signals.set(false)
) or a user’s theme preference like dark and white mode. No subscriptions are needed; the changes trigger updates instantly.
Observables excel at managing sync operations like API calls. They use operators like debounceTime and switchMap to process data over time. For example, consider this example for a search result with retries:
this.service.search(query).pipe(retry(3), catchError(error => of([])))
Use Signals for local state (simple, reactive state) and Observables for async logic. Here is an example for a search bar where a signal track input, converted to an observable for debouncing an API calls:
query = signal('');
results$ = toObservable(this.query).pipe(
debounceTime(300),
switchMap(q => this.service.search(q))
);
Adopt a Holistic Approach to Reactive Programming
The key to writing maintainable and efficient Angular applications is to integrate these best practices into a cohesive, holistic workflow. Rather than viewing these techniques as isolated tips, consider how they work together to solve real-world problems. For instance, using the AsyncPipe minimizes manual subscription management, which, when combined with RxJS operators to flatten streams, results in code that is not only efficient but also easier to understand and test.
In real-world scenarios, such as a live search feature or a dashboard that displays multiple data sources, these practices collectively reduce code complexity and improve performance. Integrating Angular 16 signals further simplifies state management, ensuring that the user interface remains responsive even as application complexity grows.
Conclusion
As Angular evolves, so do the best practices we use to manage state, handle user input, and compose complex reactive streams. Leveraging the AsyncPipe simplifies template binding; flattening nested subscriptions with operators like switchMap
makes your code more readable; and smart unsubscription strategies prevent memory leaks – all while error handling and strong typing add additional layers of resilience.
By adopting these strategies, you ensure your application thrives in Angular’s modern ecosystem (16+), leveraging RxJS for asynchronous logic and Angular’s native tools for state and lifecycle management. These practices we went over are forward-compatible with Angular 17/18, ensuring your code remains efficient and maintainable as the framework evolves.
For more advanced asynchronous processing, RxJS remains indispensable. But when it comes to local or global state management, Angular signals offer a fresh, concise approach that reduces boilerplate and automatically updates the UI. Merging these practices ensures that your Angular 16 applications remain efficient, maintainable, and, importantly, easy to comprehend, even as they grow in complexity.