- Apple Inc.: KeyBanc Capital Markets upgrades to sector weight from underweight.
- Freeport-Mcmoran Inc.: HSBC upgrades to buy from hold with a target price reduced from USD 41 to USD 40.
- Icon Public Limited Company: TD Cowen downgrades to hold from buy with a target price reduced from USD 254 to USD 157.
- Medpace Holdings, Inc.: TD Cowen downgrades to hold from buy with a target price reduced from USD 370 to USD 328.
- Tpg Inc.: Morgan Stanley downgrades to equalwt from overwt with a target price reduced from USD 80 to USD 47.
- Wells Fargo & Company: Phillip Securities upgrades to buy from accumulate with a target price reduced from USD 85 to USD 75.
- Blackstone Inc.: Evercore ISI maintains its outperform recommendation and reduces the target price from USD 191 to USD 152.
- Blue Owl Capital Inc.: Evercore ISI maintains its outperform recommendation and reduces the target price from USD 26 to USD 20.
- Caterpillar Inc.: JP Morgan maintains its overweight recommendation and reduces the target price from 490 to USD 380.
- Dell Technologies Inc.: Citigroup maintains its buy recommendation and reduces the target price from USD 145 to USD 105.
- Devon Energy Corporation: Jefferies maintains its hold recommendation with a price target reduced from USD 43 to USD 32.
- Elf Beauty: Baird maintains its outperform recommendation and reduces the target price from USD 110 to USD 85.
- Estee Lauder: Citigroup remains neutral recommendation with a price target reduced from USD 77 to USD 55.
- Hp Inc.: Citigroup remains neutral recommendation with a price target reduced from USD 36.50 to USD 25.
- Hubspot, Inc.: Mizuho Securities maintains its outperform recommendation and reduces the target price from USD 900 to USD 700.
- Kkr & Co. Inc.: Morgan Stanley maintains its market weight recommendation and reduces the target price from 156 to USD 114.
- Micron Technology, Inc.: CTBC Securities Investment Service Co LTD maintains its buy recommendation and reduces the target price from USD 114 to USD 81.
- Mongodb, Inc.: Mizuho Securities maintains a neutral recommendation with a price target reduced from USD 250 to USD 190.
- Murphy Oil Corporation: Jefferies maintains its hold recommendation with a price target reduced from USD 30 to USD 23.
- Southwest Airlines Co.: Citigroup maintains its sell recommendation with a price target reduced from USD 30 to USD 23.
- Stellantis N.v.: Equita SIM maintains its hold recommendation with a price target reduced from 13.90 to EUR 11.
- The Carlyle Group Inc.: Morgan Stanley maintains its market weight recommendation and reduces the target price from 57 to USD 44.
- Twilio Inc.: Mizuho Securities maintains its outperform recommendation and reduces the target price from USD 165 to USD 125.
- United Rentals, Inc.: JP Morgan maintains its overweight recommendation and reduces the target price from 1000 to USD 750.
- Vertiv Holdings Co: Citigroup maintains its buy recommendation with a price target reduced from USD 153 to USD 98.
Category: Uncategorized
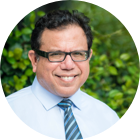
MMS • RSS
Posted on nosqlgooglealerts. Visit nosqlgooglealerts
InfluxData has released InfluxDB 3 Core and Enterprise editions in a bid to speed and simplify time series data processing.
InfluxDB 3 Core is an open source, high-speed, recent-data engine for real-time applications. According to the pitch, InfluxDB 3 Enterprise adds high availability with auto failover, multi-region durability, read replicas, enhanced security and scalability for production environments. Both products run in a single-node setup, and have a built-in Python processing engine “elevating InfluxDB from passive storage to an active intelligence engine for real-time data.” The engine brings data transformation, enrichment, and alerting directly into the database.
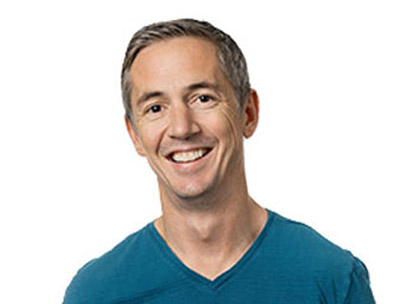
Founder and CTO Paul Dix claimed: “Time series data never stops, and managing it at scale has always come with trade-offs – performance, complexity, or cost. We rebuilt InfluxDB 3 from the ground up to remove those trade-offs. Core is open source, fast, and deploys in seconds, while Enterprise easily scales for production. Whether you’re running at the edge, in the cloud, or somewhere in between, InfluxDB 3 makes working with time series data faster, easier, and far more efficient than ever.”
A time-series database stores data, such as metrics, IoT and other sensor readings, logs, or financial ticks, indexed by time. It typically features high and continuous ingest rates, compression to reduce the space needed, old data expiration to save space as well, and fast, time-based queries looking at averages and sums over time periods. Examples include InfluxDB, Prometheus, and TimescaleDB.
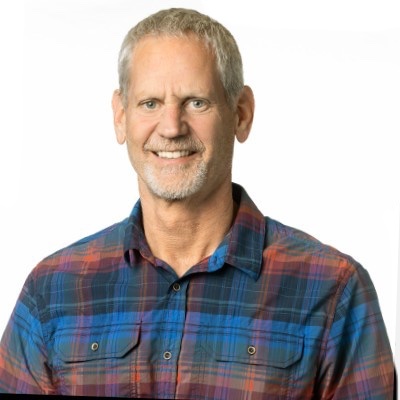
InfluxData was founded in 2012 to build an open source, distributed time-series data platform. This is InfluxDB, which is used to collect, store, and analyze all time-series data at any scale and in real-time. CEO Evan Kaplan joined in 2016. The company raised around $800,000 in a 2013 seed round followed by a 2014 $8.1 million A-round, a 2016 $16 million B-round, a 2018 $35 million C-round, a 2019 $60 million D-round, and then a 2023 $51 million E-round accompanied by $30 million in debt financing.
Kaplan has maintained a regular cadence of product and partner developments:
- January 2024 – InfluxDB achieved AWS Data and Analytics Competency status in the Data Analytics Platforms and NoSQL/New SQL categories.
- January 2024 – MAN Energy Solutions integrated InfluxDB Cloud as the core of its MAN CEON cloud platform to help achieve fuel reductions in marine and power engines through the use of real-time data.
- March 2024 – AWS announced Amazon Timestream for InfluxDB, a managed offering for AWS customers to run InfluxDB within the AWS console but without the overhead that comes with self-managing InfluxDB.
- September 2024 – New InfluxDB 3.0 product suite features to simplify time series data management at scale, with performance improvements for query concurrency, scaling, and latency. The self-managed InfluxDB Clustered, deployed on Kubernetes, went GA, and featured decoupled, independently scalable ingest and query tiers.
- February 2025 – InfluxData announced Amazon Timestream for InfluxDB Read Replicas to boost query performance, scalability, and reliability for enterprise-scale time series workloads.
The new InfluxDB 3 engine is written in Rust and built with Apache Arrow, DataFusion, Parquet, and Flight. We’re told it delivers “significant performance gains and architectural flexibility compared to previous open source versions of InfluxDB.” The engine can ingest millions of writes per second and query data in real-time with sub-10 ms lookups.
The Python engine “allows developers to transform, enrich, monitor, and alert on data as it streams in, turning the database into an active intelligence layer that processes data in motion – not just at rest – and in real-time.” This reduces if not eliminates the need for external ETL pipelines.
Both new products fit well with the existing InfluxDB 3 lineup, which is designed for large-scale, distributed workloads in dedicated cloud and Kubernetes environments and has a fully managed, multi-tenant, pay-as-you-go option.
InfluxDB 3 Core is now generally available as a free and open source download. InfluxDB 3 Enterprise is available for production deployments with flexible licensing options. Read more here.
Google’s Cybersecurity Model Sec-Gemini Enables SecOps Workflows for Root Cause and Threat Analysis
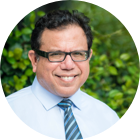
MMS • Srini Penchikala
Article originally posted on InfoQ. Visit InfoQ
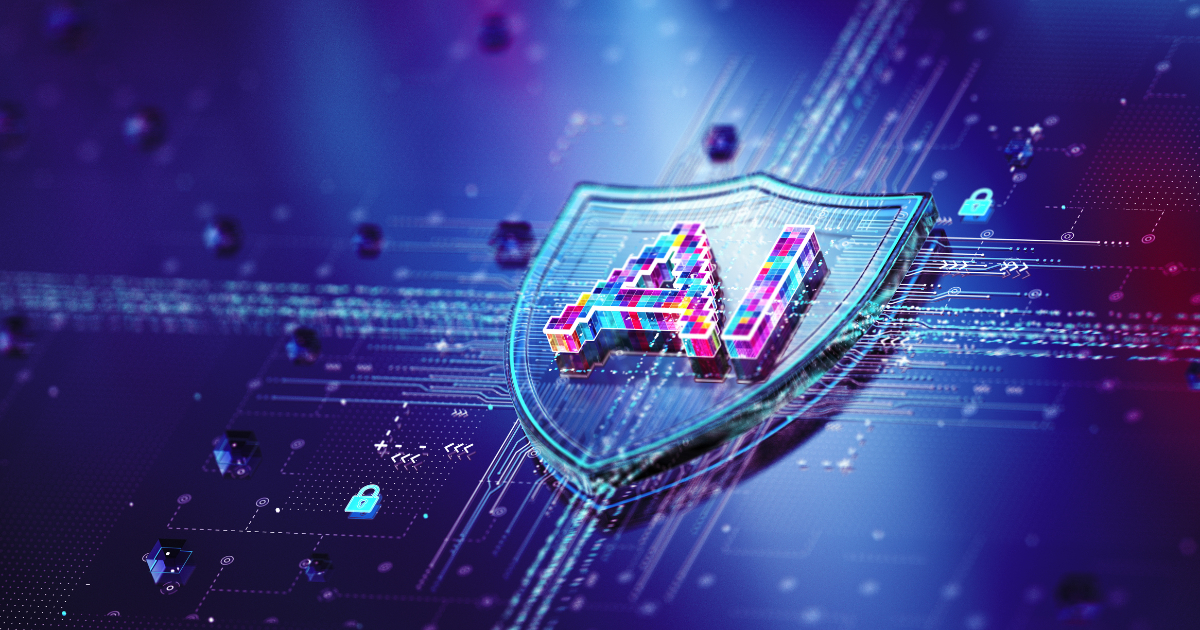
Google’s new cybersecurity model Sec-Gemini focuses on cybersecurity AI to enable SecOps workflows for root cause analysis (RCA) and threat analysis, and vulnerability impact understanding. Google Cybersecurity x AI Research Lead Elie Bursztein announced last week the release of Sec-Gemini v1.
Security defender teams typically face the task of securing against all cyber threats, while attackers need to successfully find and exploit only a single vulnerability. This asymmetry has made securing systems difficult, time consuming and error prone. AI-powered cybersecurity workflows can help shift the balance back to the defenders by force multiplying cybersecurity professionals. They also help security analysts understand the risk and threat profile associated with specific vulnerabilities faster.
Enabling SecOps workflows requires state-of-the-art reasoning capabilities and current cybersecurity knowledge. Sec-Gemini v1 achieves this by combining Google Gemini’s Large Language Model (LLM) capabilities with near real-time cybersecurity knowledge and tooling. This allows it to achieve a better performance when using it on cybersecurity workflows like incident root cause analysis, threat analysis, and vulnerability impact understanding.
Sec-Gemini v1 leverages various data sources including Google Threat Intelligence (GTI), Open-Source Vulnerabilities database operated by Google (OSV) and Mandiant Threat intelligence data. An example shown on the website highlights Sec-Gemini v1’s answers in response to key cybersecurity questions where the framework, using up-to-date accurate threat actor information, is able to determine that Salt Typhoon is a threat actor and provides a description of that threat actor. The output includes not only vulnerability details (based on OSV data), but also the contextualization of vulnerabilities with respect to threat actors (using Mandiant data). The framework performs well on key cybersecurity benchmarks like Cyber Threat Intelligence Multiple Choice Questions (CTI-MCQ), a leading threat intelligence benchmark and the Cybersecurity Threat Intelligence-Root Cause Mapping (CTI-RCM) benchmark.
Since last year Google has been integrating Gemini product into AI enabled security and compliance strategy programs, including AI-driven security with Google Cloud and Google Security Operations. Last year’s The State of AI and Security Survey Report published by Google Cloud and Cloud Security Alliance (CSA) highlights the AI’s potential to enhance security measures and improve threat detection and response capabilities. Several other organizations including NVIDIA and RedHat are increasingly leveraging AI technologies in cybersecurity use cases to quickly detect anomalies, threats, and vulnerabilities by processing large amounts of data in a short time. Some of these use cases include anomaly detection and AI-assisted code scanning.
It’s important to note that Sec-Gemini v1 is still an experimental cybersecurity model. The team is making it freely available to select organizations, institutions, professionals, and NGOs for research purposes. Google team provided an early access request for their Trusted Tester recruitment program but the forum is currently closed due to the team receiving a lot of requests from the community.
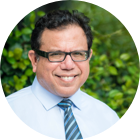
MMS • Anthony Alford
Article originally posted on InfoQ. Visit InfoQ
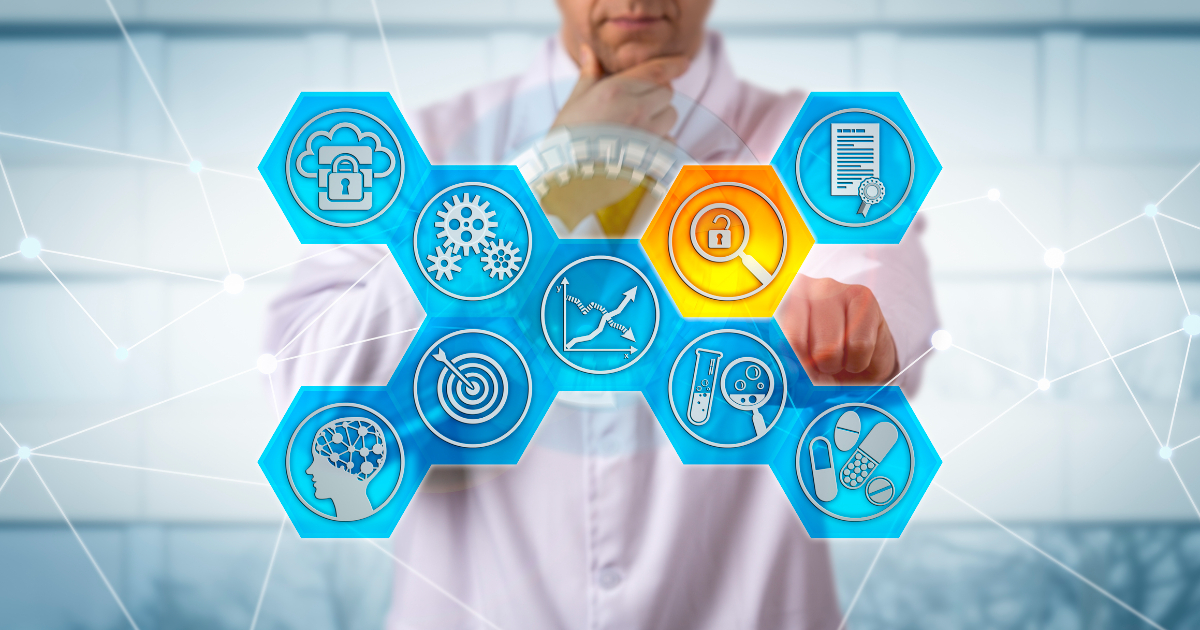
Google released the Agent2Agent (A2A) Protocol, an open-source specification for building AI agents that can connect with other agents that support the protocol. Google has enlisted over 50 technology partners to contribute to A2A’s development.
Google announced the release at the recent Google Cloud Next conference. A2A is billed as a “complement” to Anthropic’s Model Context Protocol (MCP) and defines a client-server relationship between AI agents. Google developed the protocol with help from partners like Salesforce, Atlassian, and LangChain, with the goal of creating an interoperability standard for any agent, regardless of vendor or framework. According to Google,
A2A has the potential to unlock a new era of agent interoperability, fostering innovation and creating more powerful and versatile agentic systems. We believe that this protocol will pave the way for a future where agents can seamlessly collaborate to solve complex problems and enhance our lives. We’re committed to building the protocol in collaboration with our partners and the community in the open. We’re releasing the protocol as open source and setting up clear pathways for contribution.
InfoQ covered Anthropic’s MCP release last year. Intended to solve the “MxN” problem—the combinatorial difficulty of integrating M different LLMs with N different tools—MCP defines a client-server architecture and a standard protocol that LLM vendors and tool builders can follow.
Google’s documentation points out that A2A solves a different problem than MCP does: it “allows agents to communicate as agents (or as users) instead of as tools.” The difference between a tool and an agent is that tools have structured I/O and behavior, while agents are autonomous and can solve new tasks using reasoning. In Google’s vision, an agentic application requires both tools and agents. However, A2A docs do recommend that “applications model A2A agents as MCP resources.”
A2A defines three types of actor: remote agents, which are “blackbox” agents on an A2A server; clients that request action from remote servers; and users (human users or services) that want to accomplish tasks using an agentic system. Like MCP, A2A uses JSON-RPC over HTTP for communication between clients and remote agents. The core abstraction used in the communication spec between agents is the task, which is created by a client and fulfilled by a remote agent.
In a Hacker News discussion, several users compared A2A to MCP; some were not sure what value A2A proved over MCP, while others saw it as a “superset” of MCP and praised its “clear documentation and explanation” compared to MCP. User TS_Posts claimed to be working on A2A and wrote:
[T]he current specification and samples are early. We are working on many more advanced examples and official SDKs and client/servers. We’re working with partners, other Google teams, and framework providers to turn this into a stable standard. We’re doing it in the open – so there are things that are missing because (a) it’s early and (b) we want partners and the community to bring features to the table. tldr – this is NOT done. We want your feedback and sincerely appreciate it!
The A2A source code is available on GitHub. Google also released a demo video showing collaboration between agents from different frameworks.
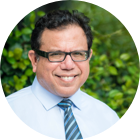
MMS • RSS
Posted on mongodb google news. Visit mongodb google news
Mizuho analyst Siti Panigrahi has revised the price target for MongoDB (MDB, Financial), reducing it from $250 to $190 while maintaining a Neutral rating on the stock. This adjustment is part of a broader assessment ahead of the first-quarter results in the software sector, where price targets have been cut due to a recent compression in software multiples.
Despite the reduction, Mizuho views the recent downturn in the software market as offering an “attractive buying opportunity” for investors. While Mizuho anticipates strong performance reports for the first quarter, it also notes that company management might exhibit caution regarding expectations for the fiscal year.
The firm asserts that ongoing tariff issues are not expected to disrupt the core fundamentals of the software-as-a-service industry, indicating a resilient outlook for the sector despite external challenges.
Wall Street Analysts Forecast
Based on the one-year price targets offered by 34 analysts, the average target price for MongoDB Inc (MDB, Financial) is $294.39 with a high estimate of $520.00 and a low estimate of $180.00. The average target implies an
upside of 86.27%
from the current price of $158.04. More detailed estimate data can be found on the MongoDB Inc (MDB) Forecast page.
Based on the consensus recommendation from 38 brokerage firms, MongoDB Inc’s (MDB, Financial) average brokerage recommendation is currently 2.0, indicating “Outperform” status. The rating scale ranges from 1 to 5, where 1 signifies Strong Buy, and 5 denotes Sell.
Based on GuruFocus estimates, the estimated GF Value for MongoDB Inc (MDB, Financial) in one year is $433.07, suggesting a
upside
of 174.03% from the current price of $158.04. GF Value is GuruFocus’ estimate of the fair value that the stock should be traded at. It is calculated based on the historical multiples the stock has traded at previously, as well as past business growth and the future estimates of the business’ performance. More detailed data can be found on the MongoDB Inc (MDB) Summary page.
Article originally posted on mongodb google news. Visit mongodb google news
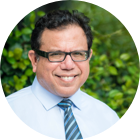
MMS • Craig Risi
Article originally posted on InfoQ. Visit InfoQ
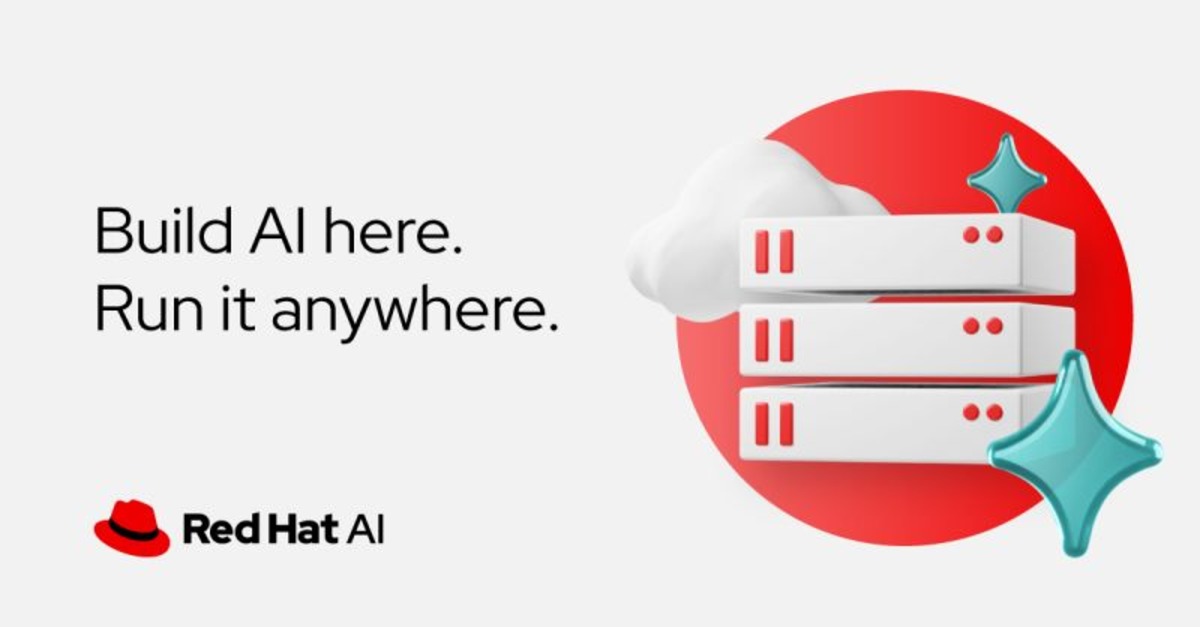
Red Hat has recently announced enhancements to its Red Hat AI portfolio, aiming to accelerate the development and deployment of artificial intelligence (AI) solutions across hybrid cloud environments. This initiative focuses on integrating AI into enterprise operations, offering tools that support both predictive and generative AI models.
A central component of this portfolio is Red Hat OpenShift AI, which provides a platform for managing AI lifecycles across hybrid clouds. It encompasses machine learning operations (MLOps) and large language model operations (LLMOps) capabilities, facilitating the building, tuning, and deployment of AI models. The platform includes tools for data science pipelines, model monitoring, and governance, streamlining the process of bringing AI models from development to production.
Complementing OpenShift AI is Red Hat Enterprise Linux AI (RHEL AI), a foundation model platform designed for developing, testing, and running generative AI models. RHEL AI integrates open-source Granite models and InstructLab tooling, enabling organizations to align AI models with specific business requirements. This integration allows domain experts to contribute to model development without extensive data science expertise, broadening the accessibility of AI technologies.
Red Hat’s commitment to AI innovation is further demonstrated by its acquisition of Neural Magic, a company specializing in software and algorithms that accelerate generative AI inference workloads. This acquisition enhances Red Hat’s capabilities in optimizing AI performance across hybrid cloud environments. Additionally, collaborations with major cloud providers, such as Microsoft Azure, expand the deployment options for RHEL AI, offering organizations flexibility in their AI strategies.
Presentation: Opening the Box: Diagnosing Operating-System Task-Scheduler Behavior on Highly Multicore Machines
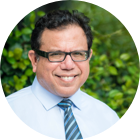
MMS • Julia Lawall
Article originally posted on InfoQ. Visit InfoQ
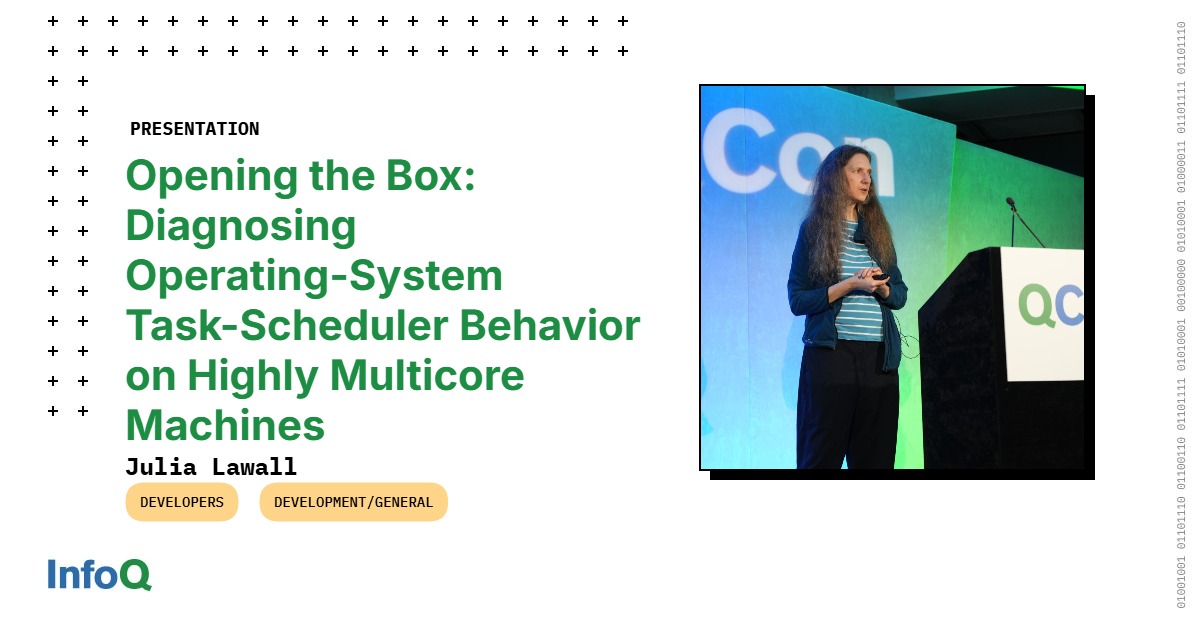
Transcript
Lawall: I’m going to talk about, Opening the Box: Diagnosing Operating System Task-Scheduler Behavior on Highly Multicore Machines. Basically, what our situation is, is we have our machines. They can be simplified as applications on the top, hardware on the bottom. Then, basically, the application does what the user wants. The hardware provides all the resources to make it possible. We need something in the middle to mediate between them to ensure all the interactions are safe, correct, not introducing security violations, and so on. That’s the operating system. That’s going to be the subject of our talk.
The operating system as a piece of software that’s mediating the access to the hardware, can have an important and unexpected influence on the performance of our applications. Sometimes, we need to actually understand that. Most of the time, hopefully, we can just ignore the operating system and hope everything works for the best. Sometimes, for example, from changing from one operating system version to the next one, things might be unexpected. It might be different. Then one has to actually understand what’s going on. In this talk, we’re focusing on Linux, just because it’s open source, available, and familiar to me. We’re focusing on the task scheduler. Task scheduler is the thing that figures out what tasks should be running on what core at what time. What we’re interested in is diagnosing performance regressions. Our target is large multicore machines, so server-class machines.
The Task Scheduler of the Linux kernel
The task scheduler of Linux kernel, what is its role? Basically, it has two things to do. One of them is to decide where each different task should run. This should run on core 27. This should run on core 148. Then, once those tasks have been placed on those cores, then maybe there’s three different tasks on a particular core. It should decide which of those gets to run at a particular point in time. If you’re familiar with Linux kernel source code, we’re talking about the files core.c and fair.c. We’ll think about how this task scheduler can impact the performance of applications.
Basically, the problem is that to do these things that a task scheduler needs to do, it needs to make decisions. The other problem is that actually a general-purpose operating system, such as Linux kernel, or Windows, or Mac, iOS, any of these operating systems that people are just using for doing random things, actually the operating system has absolutely no information on which it can base this decision. No real information. It can get some hints from previous behavior, but it doesn’t know what’s going to happen in the future.
Then the problem is that poor decisions can slow tasks down. This can have not just the short-term effect, but it can go on for some time and actually have a visible performance impact on how the application runs. This is a slide that actually we’re going to come back to in the end. This is where we’re going. This is an example of performance impact. This red line here is the vanilla Linux kernel. What we have on the x-axis is a bunch of runs. I’ve just sorted the runs by running time. The fastest runs are on the left. The slowest runs are on the right. It’s not chronological. The y-axis is the running time. You can see that the running time, you can focus on the red line. That’s the vanilla Linux kernel. The running time slowly increases. Some runs are a little bit faster than the others.
At the end, actually what we’re concerned about is this thing over here. Things are going up sharply. Same inputs, same computation, but very different running time. These numbers here are random. How badly things go just depends on some unknown thing that happens during the execution. This is where we’re going. You’ll see this slide again later.
The Basic Issues for Scheduling (Work Conservation and Locality)
First, I’m going to start out, what are the basic issues for scheduling? One very important issue, which will come up over and over during the talk, is called work conservation. The basic, simple idea of work conservation, we have a task that’s waking up, and where should we put it? Actually, there are four cores available. None of them are doing anything. We can put it anywhere. It doesn’t really matter. There we have our task 1. We’ve put it on core 0. Now we have another task, task 2. It’s waking up, we ask ourselves the same question, where should it go? It could go on core 1, core 2, or core 3. Those are all available. Putting it on core 0 would not be a very good choice. If we put task 2 on core 0, either it will have to wait for task 1 to finish, or maybe it will preempt task 1, and now task 1 will have to wait for task 2 to finish. That’s all very unfortunate, because there’s plenty of empty space over here for those other tasks to run.
The idea of work conservation is that no core should be overloaded if any core is idle. This is just like a concept that you can think about. It’s not the overriding concept. There could be another good decision in certain situations. This is a concept that is important in many cases. Another concept, which is perhaps in contradiction with work conservation, is the concept of locality. Locality here, we already have task 1 running on core 0. We have the same questions again. We have a task 2, where should we put it? Now we have this little two bar thing here. It means it’s a two-socket machine. We have basically two NUMA nodes. Now we might be concerned about memory accesses. We again have our situation with our three empty cores. If task 2 is sharing a lot of memory with task 1 or communicating a lot with task 1, then it could be a good idea to put task 2 on core 1.
On the other hand, if task 2 has no interaction with task 1, it might be a good idea to put it over here so that it doesn’t destroy the cache of task 1. There are some different tradeoffs. Again, we have to know what’s going to happen in the future. We have absolutely no idea about that information. That’s what makes everything complicated.
A Challenge
Basically, we have seen these principles. If you do things right, things go well. If you do things wrong, things go badly. Overall, the task scheduler controls the application’s access to the CPU, and so it controls the performance of the application. Work conservation, locality issues also, it’s not like the cost of scheduling. It’s going to impact all of the execution of the application after you have placed it in a particular place, and so it can have a real impact on the application performance.
Someone named Brendan Gregg, who works on a tool called perf, says we need to be able to understand the performance quickly and completely. This talk is pushing you in the direction of completely, so you’re not just saying, this is faster, this is slower, I don’t really know what’s going on, but this is better because it’s faster, something like that. Here we want to really understand what the operating system is doing and why, and how it’s impacting the performance of the application. I’ve been working on this problem for the last year, actually, so I’m not sure if I can really say that we’re doing things quickly, but at least we try to understand things completely. The problem that we have is the operating system, and the scheduler itself.
First, we don’t want to look at the operating system in the first place, and then the scheduler is buried deep within that operating system, so how can we actually understand what it’s doing, what decisions it’s making at what point, and so on. That’s what we’re getting at in this talk.
Tooling
Fortunately, some help is available. There’s a tool which is called trace-cmd. You run your application here, and trace-cmd record will record all the scheduling events, and then you can see precisely what’s going on at every nanosecond, basically. Then you can use trace-cmd report on your trace, and then you get this output. This is obviously extremely abbreviated. You can have gigabytes of data coming out, because many things happen on your machine all the time, and this trace is going to include all of them. This trace gives you all the information. You know what command’s running, what its PID is, what it’s doing, what time, and so on. The trace is sorted chronologically, which is in some sense helpful, but it’s hard to really trace through what’s going on. Here things are happening on core 26, core 62, and so on. They don’t really have anything to do with each other, so it’s hard to look at this textual output and actually understand what’s going on with the application.
Fortunately, there’s also another tool that comes with it. This is called KernelShark. KernelShark will show you the different CPUs that are on your machine and what the CPU is doing at each unit of time. Then we have the same output we saw on the slide before when you want more detail. This is a very nice tool. You can zoom in, and then you get more information about a particular point. It’s a bit frustrating to use if you have a very big machine, because here we see two cores. It’s already taking up most of my slide just to see those two cores. It’s a bit spacious, and it’s a bit too friendly in certain directions that are not useful for all tasks. It’s definitely useful for certain tasks, but maybe not for everything. Actually, in my own work, I found it useful. I wanted to have an overview of all of my cores at once to see what’s going on everywhere, and so this tool was not really completely successful in that direction.
Basically, we want to be able to visualize these traces in some way, or we’re going to see a bunch of different ways we can visualize the traces. We want to see all the activity and all the cores at once. We want it to scale to multiple gigabytes of data. I don’t know about your applications. I don’t know if that’s enough to understand your applications, but that’s at least enough for what I was looking into. I found it useful also to have something that would produce files that I could save, that I could compare, that I could share with my colleagues, and so I wanted to produce PDFs. Then, something that my colleagues complain about a lot is that I’ve totally given up on interactivity. With KernelShark, you can zoom. It’s very nice. You see something immediately. With all of my tools, you have to actually run it again and say what range you want. I haven’t actually found any drawing tool that really gave the interactivity and scalability up to the size of the data I was interested in.
Basically, I’m proposing four tools. This is the current state of the work. Dat2graph, it’s a horizontal bar graph. It’s like KernelShark. It shows you all the cores and what they’re doing at any point in time. Running_waiting is a summary. How many tasks are running? How many tasks are waiting to be able to run? That gives you an idea of, what are the overloads on your machine? In some regions of your execution, are there some overloads? Stepper, is like zooming in even more. It shows you all the cores, all the tasks on the cores, the command name, the PID. It’s good when you know there’s this problem at this particular point and you want to see exactly what’s going on in the machine at that point. Finally, enter events, which interleaves. Eventually, you’ll need to make your own kernel events in your own print case, basically printfs that print out the information that you want. This manages that and interacts with the scheduling. We’ll see how all of these tools can be useful in debugging a particular performance problem. All these tools are publicly available. The URL will be at the end of the talk.
The example that we’re going to look at is based on an application from the NAS benchmark suite. These are somehow high-performance computing applications. The application we’re considering is BT, Block Tri-diagonal solver. The only thing that we need to know about this is it has N tasks on N cores. If we have 64 cores, we have 64 tasks. 64 tasks on 64 cores sounds like an extremely trivial scheduling problem. You just put one task on each core and let them do what they want, and then everything should be fine. Everything would be fine if the Linux kernel scheduler would actually do that. Unfortunately, we’ll see that it does not. Here’s a bunch of runs of the application, this is 20 runs. Again, I’ve sorted them by runtime. We have here the fastest run. There’s a bunch of runs that are close to that. Maybe they have some other locality issues, for example.
Then you see, like I mentioned before, we have this upward trend at the far end. Most of our runs run perfectly well as we expect them to, but every now and then there’s one that is much slower, and we want to know why. This dotted line compares the fastest run and the slowest run. You can see it’s about a 20% overhead for the slowest runs. To start out with, we can just get to know our benchmark. This is a scientific computing application. The standard thing to do with scientific computing applications is just to pin all the threads onto the cores and let them do what they want. Our interest is to find out how the Linux kernel scheduler is going to manage these threads. We don’t want to pin them, but it’s interesting to compare the difference.
If we pin them, we get even more performance improvement, and we don’t get this huge jump at the end. We just get a small increase. Here I have not actually pinned specific threads to specific cores. I have said the first half of the thread goes on one socket. This is a two-socket machine. Put half of them on one socket and put half of them on the other socket and let them do whatever they want beyond that. The fact that we see a difference between the execution times suggest that memory locality is actually an issue for this benchmark. That’s something just like having some idea of what the benchmark does and what its issues are.
Now what we can do is we can make some runs and try to see what’s going on in those runs. This is the tool dat2graph. We run the application with trace-cmd. We obtain this dat file. It’s fairly big, maybe. Then we can pass the tool to dat2graph, and then we get output like this. In the x-axis, we have the execution time. In the y-axis, we have the set of cores. These lines here represent the different tasks that are running on the different cores. Basically, there is a different color for each PID, so for each different task, but there’s only a finite number of colors. For example, here we have two green tasks. They’re obviously not the same PID. It’s a bit approximate, but it’s never really been found that to be a problem in practice. Maybe we could use random numbers, but when I tried random numbers, the colors sometimes came out too close to each other anyway, and so it wasn’t really beneficial.
Basically, what we see here is that these tasks run along. Sometimes here we have a task that was on this core. These two tasks have switched with each other, but there’s not much other excitement going on here. Here’s a somewhat slower run, not very much slower, just a little bit slower. Here is a very slow run. Before we were around 8, 8-and-a-half seconds, and now we have jumped up to over 10 seconds.
Gaps, and Their Impact
Now what we’re going to do is we’re going to look into, like how is this run different from the first run, and what’s going on? Basically, the issue that we find is that once we start looking at these slower runs, we start to see these gaps. There’s a gap here. There’s a gap here. What does a gap mean? A gap means that the task was running along, and now one core is not being used at all. Using fewer cores, less CPU time available, so it seems natural that we should get slower. If we look here, this one actually has a huge gap. This is the slowest run, and it has a huge gap. These gaps seem to be important with respect to the performance. What’s the impact of a gap? Maybe there’s just nothing to do at those points, and in that case, the gap is just nothing to do, so we don’t do anything, so it doesn’t matter. You can think back to what I said previously. This is N tasks on N cores. There probably is something to do, so this is probably not our situation.
The other situation is that if some core is idle, that means that some other core is probably overloaded, and those overloaded cores are going to be running at half speed. Basically, you have two people standing in the same place, and they can’t both walk forward at the same time. One of them will walk forward for a little while, and the other one will walk forward, and then another one will walk forward, and so they’ll be going half as fast as all of the other tasks that can run freely by themselves. This is actually also an application that synchronizes very often. We have these two tasks that are walking forward slowly, and then we’ll have all those other fast tasks, and they will be waiting for the slow ones. That will slow everybody down, and not just those two slow tasks. That can have a big performance impact.
Basically, we want to see which situation we’re in. I’ve hinted that we’re definitely in the second one, but we can see that in practice. The tool, running_waiting, is going to show us where there are overloads. Here again we have x-axis is the time. Now the y-axis is the number of threads that are either running, which are the green ones. We have a 64-core machine, and so it’s not surprising that we have 64 running threads. When we are up at this line, that means that everything is as we hope it to be.
Then the red line is the total number of all of the threads, which means that the difference between the red line and the green line is the number of waiting threads. These are tasks that are waiting in the run queue of some core. They would like to run, they are able to run, but there’s no space on that core for them to run. That’s slowing things down. You see lots of red here. This is a graph, it’s made with some graphing tool which has some resolution, and so on. What we’re interested in is really the width of these lines, and since they’re lines, they have a minimum width, and so this is perhaps a bit misleading. What we can do instead is we can focus on what we’re really interested in, which is the application. The application threads would be the one that have been forked since the start of the application. If we just focused on the forked ones, then we have quite a different picture. This is the fast run. In the fast run, most of the time, the application is running at full speed on all of the cores, and things are as they should be.
On the other hand, this is the slow run. In the slow run, now you can clearly see that there is a problem. We saw the gap in this region here, and what we see from this particular graph is that, up to this point, we have 64 threads running. Now we have only 63 threads running at any point in time, but we have 64 threads that want to run. One of our application threads wants to run, but it’s not able to run. This gap is indicating a work conservation problem. Basically, somewhere on the machine, there is an idle core. Somewhere else on the machine, there is a task that wants to use that idle core, but it’s not able to get there for some reason. This is a long overload period. It’s lasting from 7 seconds, that’s like 3 seconds. It’s basically one-third of the execution time. Things are not going as we hope they should go. This is really undesirable for the behavior of the application.
Now we can try to understand what happened, what caused this bad situation to arise? We can go back to dat2graph. Dat2graph has an option, which is color-by-command. Color-by-command colors all the tasks, not by their PID, by what command they’re trying to execute. These blue lines are BT, that’s the application we’re interested in. At the beginning of our gap, we have a green line, and the green line is called migration. Then you have to ask yourself, so what does migration do? Migration is a task that comes from the kernel. It’s a kernel thread, and its purpose is basically to take some happily running task on some CPU, yank it off that CPU, and put it some other place.
Basically, migration wakes up, it preempts the running task. Then the running task can be moved off to some other CPU, and then migration goes to sleep once its evil work has been done. Migration is used for load balancing. Now our question is, why do we need load balancing? We have N threads on N cores, the load should be balanced. The kernel should just deal with it. Clearly, the kernel was dealing with it before. We can see what’s going on.
Is Load Balancing Needed?
Now we go to the stepper tool, where we see exactly what’s happening at a particular point in the code. Here we have all of our cores, and here we have the running task. Here, this bar means, it separates the running task from the waiting tasks. Here we have a task that is waiting. It has just woken up, and it has not been able to run yet. It will get to run very shortly. This is the situation just before our migration tasks run. We can see down here, what is the event at the kernel level that’s happening? The event, it says, sched_move_numa. This is a special kind of load balancing, which is actually not moves with respect to the load, it’s with respect to the memory usage. NUMA balancing is, you study all of your tasks, and what memory are they accessing, and if you find out that a task that’s running on one NUMA node is actually accessing a lot of data on another NUMA node, then you move it over there in the assumption that it will be happier.
Basically, what we want to do here is we are moving this task. This is one NUMA node, this is the other NUMA node. It’s moving it from this NUMA node into this space. This looks like a really excellent idea, because now it will be over there with its own memory, and this space here looks like it’s empty, so that will be a good place for it. We know we have N tasks on N cores, we have 64 of these BT threads, that space is not going to be empty very long.
There is some other BT task that was actually already planning to run on this socket and run in this place. 214 is the one that we moved, and even before this 214 task ever gets to run on core 51, the task that had wanted to be there before has woken up. Actually, here’s another overloaded core, but there’s another space for it, so hopefully that won’t get moved over soon. This is the migration task here that will go away, then we’ll have two slots, and this one will get moved in there. Basically, we are now in a situation where we have two sockets, each one has 32 cores, and this one over here has 31 threads running on it, and this one has 32 threads running on it. That’s a bad thing.
Assessment
Now we understand exactly what’s going on. We have our NUMA balancing, the goal is to address locality issues. Moving a task to another NUMA node, if it has a locality issue, that should improve its performance in theory. Remember, it might be important for BT, because BT, when we had everything perfectly placed according to the NUMA nodes, the performance was better. There are two types of NUMA balancing, one of them is when you switch cores, you take a thread here, a thread here, and you exchange them. That seems fine. We’re going to stay with the same number of threads on each core, no work conservation issues.
The other one is the one that we actually had in our situation, which is move, and in that one you just take some thread over here and put it on the socket over here, and hope for the best. The problem is, in this case, we risk introducing an overload, and that’s actually what happens in our case. We have an overload, but the scheduler is supposed to manage these kinds of issues for us. The really important question is, we have this overload, why does it not get corrected? Why does the scheduler not just realize that this is a very bad situation? It never resolves it, the application just ends. Why does it take so much time for it to figure out what to do, or why does it spend so much time not doing anything at all?
To understand that, we need to actually delve into the code, and figure out what the load balancer actually does, because that’s what we hope will do the work for us. We have overload now, it’s not a NUMA issue. We have overload, so load balancing should help us deal with overloads. Load balancing has several parts. First, it has a bunch of filters. The first filter is, should_we_balance? Basically, what happens with load balancing is, on every clock tick, each core gets to reflect on whether it wants to steal load from some other part of the machine. The problem is that we don’t want all of those cores to be stealing tasks from other parts of the machine at the same time, because they will have the same vision of what’s going on, and they will all naturally try to steal the same task. That task will have to bounce around the machine with no practical benefit. Should_we_balance addresses that issue.
Then, if we are the core that should be allowed to balance at the current moment, then we have to find who are we going to steal from. We find the busiest group, and in our situation, that’s going to be the busiest socket. It should be obvious to you which is the busiest socket, it’s the one with the 33 threads on it. Then we want to find the busiest queue. The core that is going to steal, it’s just going to identify one other core that it should steal from, and then it will take some threads from that core. If it needs to, it can actually then go around, there’s a loop, and it can steal from some other cores later. In our situation, we just have one extra thread on one socket that we will need to steal, so there’s not that complexity.
Basically, we see if the current CPU is the one that should steal, and then we see if that CPU can find another CPU that it can steal from. If any of these conditions are violated, then we just abort, and then we try again in the future. If we manage to find a core that we can steal from, then we look through its different tasks and see if there’s one that we can steal, or more that we can steal. If we are able to steal something, that’s great, that’s success. There’s a bunch of heuristics here that help you try to decide which is the task that you should steal. If you’re not able to steal anything, there’s another kind of stealing, which is called active balance. Active balance is the last resort. Active balance is able to steal a task that’s running. Otherwise, you only steal tasks that are just waiting, because they don’t have any context, they’re easier to steal somehow.
Custom Tracing
Basically, we have this flowchart here, and we want to understand, why do we not end up at the success places in the flowchart? Since we never manage to steal anything, nothing ever runs on this core, it seems that we always end up in the abort context. We want to figure out why does this unfortunate thing happen? All of the graphs that we have made before are based on events that exist already in the Linux kernel. In this case, we want to put in some specialized things, because we want to understand, actually, how we get through this function, and that’s not something that other people have wanted to do before. Basically, we want to add custom events into the kernel. You just think about how you debug things in the same situation in user space, and you might put in prints, or you might use a debugger. It’s not super easy to use a debugger at the kernel level, but you can put in prints. It’s a nice command called trace_printk. It works just like ordinary printf at the user level with the same percents and formats and everything. It’s very simple to use.
Then we have another tool, which is called events, which shows when these events happen and how that relates to the other scheduling activities. I’ve put trace_printks at the different places in this flowchart, like when we fail here, when we fail here, when we fail here, and when we fail here. The events tool has lots of options, and one of them is this cpu 40. Basically, I have compiled my kernel. I have installed my kernel. I have run my application under this new kernel. I have got a new trace. Then I want to print out the events, and I can use dat2graph to study the trace to figure out where the gap is and what core it’s on. Now I use the events thing, because there’s lots of events. I use the events thing to focus on the core that has all the idles. That’s why we only see events on this core. There are lots of events that happen on the other ones, but they’re not interesting. What we’re interested in is, what does this core do and how does that prevent it from actually being able to steal anything.
You can look over here. Here we have the names of the little strings that I put in my trace_printks, so the names of my events. I also know how many of those events are and what percentage of the total number of events they are. If you do a little math, then you can see that here we start load balancing 864 times, and every single one of those times we fail for one of these different reasons. Most of the time we fail because we are not able to find a busiest group. This might seem surprising, because we clearly have a busiest group. One of them has 33 threads on it, one of them has 31 threads on it.
The Linux kernel has some different characteristics, like categories and different heuristics for deciding that one socket is busier than the other one. Often it fails just at this level. This is 693 out of 864. The next one is we succeed to find the busiest group, but now we don’t find a busiest queue. These are little red diamonds. You can see that there are many green Xs, those find_busiest_group is failing. There are also many red diamonds, and those red diamonds are scattered around a lot. There’s 149 of them.
Again, this is failing because we have a bunch of different heuristics. Even though we see that there is an imbalance between the different cores, the Linux kernel developers have decided that the correct heuristic in this situation is that that imbalance is not enough to motivate doing anything. It’s surprising because we have an imbalance situation that persists for multiple seconds, which is a long time from the point of view of an operating system.
Again, it’s like heuristics, they don’t know the future, they don’t really know that much about the past either. According to its strategy making process, it makes this particular decision. There are 21 cases where we managed to find a busiest group, we managed to find a busiest queue, but we still don’t manage to steal anything. This is for the active balance. Active balancing is a violent operation because we’re stealing a task that’s actually happily running on some other core. There’s a threshold, and you have to get over the threshold of failures before you can actually do this active balancing. There’s these very few cases where we even reach active balancing, but we always fail to get over the threshold. Our counter value is only 0 or 1, and the threshold we have to get to is being greater than 4. All a bit arbitrary, we don’t make it.
Observations
Basically, as I’ve summarized so far, the find_busiest_group fails a lot, but from time to time we get through it, we get to find_busiest_queue. Find_busiest_queue almost always decides that the load is balanced. This is due to something called a migration type, which is chosen to be migration_util. It’s not very important what that means. The thing that will allow us to get into the active balancing is called migrate_task. We want to have it be migrate_task instead of migrate_util. What we’re going to do now is we identify the problems, we’re just going to violently fix those problems, and then see if it makes things work. I’m not necessarily going to propose a correction for the Linux kernel, I’m going to propose, do something that makes my application give the right performance.
Then once we validate that our hypotheses are correct, then we can at some future time think about what is the better way to get the Linux kernel. The problem with the Linux kernel is it has to work for everything, it doesn’t have to just work for this specific application. That’s the direction in which we’re going. We’re going to try to switch to migrate_task, get rid of this migrate_util thing, that will get us through the find_busiest_queue, then we will try to do actually normal stealing of waiting threads. That’s going to fail as well, because actually all of our threads that are on that other socket, the NUMA balancing have decided they would prefer to be on that socket. Actually, we have a tradeoff between memory locality and work conservation. Then we fall down to the active balancing, that’s the last hope.
As I said, the threshold doesn’t get high enough. We can see how that happens here. If I changed from migrate_util to migrate_task, we still have most of our failures being find_busiest_group. Find_busiest_queue happens only one time. We end up with a lot of these need_active_balances with our counter, since we reach that code more often, the counter gets to 0 to 1 to 2 to 3 to 4, but it never gets to the 5 that we need to get over the threshold to actually do the active balancing. That suggests that the counter is the bottleneck, it’s maybe too high, or maybe it shouldn’t be there at all. To investigate the problem, we are just going to get rid of it.
Resulting Performance
We want to avoid the find_busiest_queue failures, we did that already. We want to avoid this threshold, this counter failure, and so we’re going to do that now. Basically, I just modified the Linux kernel to make the situation be as I want it to be. Then we end up with this graph that you saw previously in the beginning. The red line is the vanilla Linux 6.7. The green line is when we use migrate_task. These lines, they’re ordered in a certain way, but these numbers are actually fairly random, so using migrate_task can give you worse performance or better performance than the original Linux kernel. It doesn’t really have any impact at all. It’s like we fail early, or we fail later, but we still fail, so it doesn’t make any difference.
The nice thing is that when we put the two changes together, we switch to migrate_task and we remove the threshold that we need to do the active balancing, then we have this blue line. This blue line shows a very slow increase in the running time, but the difference between the fastest time and the slowest time is not that great, actually I don’t know what percentage it is. We can also look at this from a different point of view, which is the work conservation, that’s what you see at the bottom. This is showing you what percentage of the time does the application spend with a work conservation problem. At the fastest times, with all of the different variants, it has basically no work conservation problem, maybe very small time.
The middle times, there’s some work conservation problems, sometimes the issue maybe is work conservation, sometimes the issue maybe it’s memory locality. It’s kind of a tradeoff. When things go badly, we’re spending up to a third of our time with a work conservation problem, that is with idle cores and overloaded cores. The nice thing that we can see is if we make all of our changes in terms of work conservation, basically the work conservation problem has gone away. There are executions that are slower than other executions, but the problem is not work conservation. Then we could go on and try to understand what the rest of the problem is, but I don’t know what it is, I haven’t done that yet. I just want to emphasize, these changes that I made, I don’t consider them to be a solution that we should use for all applications, it’s just a way of probing the system and understanding what’s going on.
Conclusion
To understand the scheduler behavior, you have to have a precise understanding of what’s going on at every point in time. What’s going on at every point in time is like a really huge amount of information. You need some tools to help you organize the data, so you can zoom in on the part that’s actually interesting for you. We have these four tools that we have found interesting in a general situation. Maybe we have too many tools, maybe we should just have one tool. It’s awkward to have to remember the different lists of arguments that different tools have, and so maybe just one tool would be good. Maybe you could also have a domain-specific language that would let you program the thing that you are interested in seeing. You wouldn’t have to program an entire visualization tool.
It’s a lot of work to make the colors come out in a nice way and make the thing be visible, allow you to zoom and all these kinds of things. You know what things you are interested in seeing, and so it’d be nice to be able to somehow easily specify what things you should want to see and what should be their relationships to each other, and what should be the format in which they are presented, and so on. This is the URL where you can find the tools, https://gitlab.inria.fr/schedgraph/schedgraph.git. It’s a little bit of documentation. There’s a link to a paper that gives a description of how it all works.
Questions and Answers
Participant 1: You mentioned the possibility of a DSL to specify visualization of large quantities of events. That seems to be something that would be pretty generically useful for the analysis of distributed systems as well, because of the problem of collecting lots of event data and then needing to somehow visualize it. It seems to be something that comes up a lot in all sorts of performance analysis, not only of a single system, but of larger systems as well.
Lawall: I don’t even have an answer for my particular case. I did make a language and then I proposed it to some colleagues and I said, what does this specification do? They had no idea. I don’t think my language was a success. So far, I don’t have an answer. It is a good point that maybe such a language, even maybe these visualization tools, if you can also generate your data in the format that it expects, maybe it could be also interesting for other large-scale systems.
Participant 2: To what degree does the scheduler take into account the L0, L1, L2 caching and the different number of processes that they might encompass, or is it just not important?
Lawall: The first question is about the different levels of cache. I didn’t emphasize it because we were only interested in the inter-socket thing, but there’s actually a hierarchy of load balancing. The bottom of the hierarchy is the hyper-threads, and then there are the things on the same socket, and then across the machine. It favors moving things around locally. I think that addresses that issue.
Participant 2: Is there any way we can give hints to the operating system about how it should schedule?
Lawall: There are some knobs that you can turn. There’s some runtime, I think it’s more for favoring performance versus energy consumption. I am not sure if there’s any hints that would address this specific very low-level issue.
Participant 3: Is there any specific like gaps you saw in the visualization tools you were using from the massive scale problems? When you’re actually evaluating the movement of tasks around, is there any kind of quantization of how much it costs to really move a task, because context switching is always expensive? What’s the threshold of when moving actually makes sense?
Lawall: First question is visualization tools. These graphs are all made with a quite old tool, which is called JGraph. Basically, if your data is very large, then you may want to have some coffee while it’s dealing with the issue. Actually, I had an intern who used Plotly, I believe. The nice feature was that it allowed interactivity. The not-so-nice feature was as soon as the data got a bit larger, actually nothing happened at all.
Participant 3: Task switching and context switching is always expensive.
Lawall: Part of it is the question that was already asked about the L0. Part of it is the fact that the active balancing is the least favorite option. It’s very hard actually to get into that. That’s the most costly thing because it preempts active tasks. There’s actually several context switches that are involved in that. The whole scheduler is just a large collection of heuristics. Sometimes they’re very intertwined. A certain heuristic here is actually for some impact here as things like that, and it’s very complex.
Participant 1: You ended up adding printks and recompiling your kernel. Do you think it would be feasible to get the same information by using a kprobe and eBPF, or would that be too intrusive? A lot of people find, for better or worse, recompiling the kernel to be intimidating, but the eBPF tooling has gotten better.
Lawall: I’m not an expert on eBPF. I just would point out that what we’re doing is like there’s a specific if or something like that. It’s not on function boundaries. I don’t know if eBPF is limited to that or not, but there’s specific ifs that we need to do. To make the specific changes, that was involving an if test, it’s like if x and-and-and y, and I want to get rid of y. I’m not sure that eBPF can actually make that kind of change at the kernel level.
See more presentations with transcripts
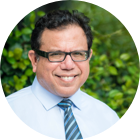
MMS • RSS
Posted on mongodb google news. Visit mongodb google news
Bank of Nova Scotia lessened its position in shares of MongoDB, Inc. (NASDAQ:MDB – Free Report) by 100.0% during the fourth quarter, according to the company in its most recent 13F filing with the Securities & Exchange Commission. The fund owned 11,640 shares of the company’s stock after selling 26,995,360 shares during the period. Bank of Nova Scotia’s holdings in MongoDB were worth $2,708,000 as of its most recent SEC filing.
Other hedge funds also recently made changes to their positions in the company. Hilltop National Bank lifted its holdings in MongoDB by 47.2% in the fourth quarter. Hilltop National Bank now owns 131 shares of the company’s stock worth $30,000 after acquiring an additional 42 shares during the last quarter. NCP Inc. acquired a new stake in shares of MongoDB during the 4th quarter worth approximately $35,000. Wilmington Savings Fund Society FSB bought a new stake in shares of MongoDB in the 3rd quarter worth approximately $44,000. Versant Capital Management Inc boosted its position in MongoDB by 1,100.0% during the 4th quarter. Versant Capital Management Inc now owns 180 shares of the company’s stock valued at $42,000 after purchasing an additional 165 shares during the period. Finally, Morse Asset Management Inc bought a new position in MongoDB during the 3rd quarter worth $81,000. Institutional investors and hedge funds own 89.29% of the company’s stock.
Analyst Ratings Changes
MDB has been the subject of several analyst reports. Cantor Fitzgerald assumed coverage on shares of MongoDB in a report on Wednesday, March 5th. They set an “overweight” rating and a $344.00 price target for the company. The Goldman Sachs Group reduced their target price on shares of MongoDB from $390.00 to $335.00 and set a “buy” rating for the company in a research note on Thursday, March 6th. Stifel Nicolaus cut their price target on MongoDB from $340.00 to $275.00 and set a “buy” rating on the stock in a report on Friday. Macquarie decreased their price objective on MongoDB from $300.00 to $215.00 and set a “neutral” rating for the company in a report on Friday, March 7th. Finally, KeyCorp downgraded MongoDB from a “strong-buy” rating to a “hold” rating in a research note on Wednesday, March 5th. Seven investment analysts have rated the stock with a hold rating, twenty-four have issued a buy rating and one has assigned a strong buy rating to the stock. Based on data from MarketBeat.com, the stock has a consensus rating of “Moderate Buy” and an average price target of $310.74.
Read Our Latest Report on MongoDB
Insider Activity
In related news, Director Dwight A. Merriman sold 1,045 shares of the firm’s stock in a transaction that occurred on Monday, January 13th. The shares were sold at an average price of $242.67, for a total transaction of $253,590.15. Following the completion of the sale, the director now directly owns 85,652 shares in the company, valued at approximately $20,785,170.84. This trade represents a 1.21 % decrease in their ownership of the stock. The transaction was disclosed in a document filed with the SEC, which can be accessed through the SEC website. Also, CEO Dev Ittycheria sold 18,512 shares of the company’s stock in a transaction that occurred on Wednesday, April 2nd. The shares were sold at an average price of $173.26, for a total transaction of $3,207,389.12. Following the completion of the transaction, the chief executive officer now owns 268,948 shares in the company, valued at approximately $46,597,930.48. This represents a 6.44 % decrease in their position. The disclosure for this sale can be found here. Over the last quarter, insiders have sold 58,060 shares of company stock worth $13,461,875. 3.60% of the stock is owned by corporate insiders.
MongoDB Trading Up 0.7 %
NASDAQ MDB opened at $162.24 on Friday. MongoDB, Inc. has a 1 year low of $140.78 and a 1 year high of $387.19. The stock’s 50-day moving average price is $220.46 and its two-hundred day moving average price is $256.10. The company has a market cap of $13.17 billion, a PE ratio of -59.21 and a beta of 1.49.
MongoDB (NASDAQ:MDB – Get Free Report) last issued its quarterly earnings results on Wednesday, March 5th. The company reported $0.19 EPS for the quarter, missing the consensus estimate of $0.64 by ($0.45). MongoDB had a negative net margin of 10.46% and a negative return on equity of 12.22%. The firm had revenue of $548.40 million during the quarter, compared to analysts’ expectations of $519.65 million. During the same quarter last year, the company posted $0.86 EPS. Research analysts forecast that MongoDB, Inc. will post -1.78 EPS for the current fiscal year.
MongoDB Profile
MongoDB, Inc, together with its subsidiaries, provides general purpose database platform worldwide. The company provides MongoDB Atlas, a hosted multi-cloud database-as-a-service solution; MongoDB Enterprise Advanced, a commercial database server for enterprise customers to run in the cloud, on-premises, or in a hybrid environment; and Community Server, a free-to-download version of its database, which includes the functionality that developers need to get started with MongoDB.
Read More
This instant news alert was generated by narrative science technology and financial data from MarketBeat in order to provide readers with the fastest and most accurate reporting. This story was reviewed by MarketBeat’s editorial team prior to publication. Please send any questions or comments about this story to contact@marketbeat.com.
Before you consider MongoDB, you’ll want to hear this.
MarketBeat keeps track of Wall Street’s top-rated and best performing research analysts and the stocks they recommend to their clients on a daily basis. MarketBeat has identified the five stocks that top analysts are quietly whispering to their clients to buy now before the broader market catches on… and MongoDB wasn’t on the list.
While MongoDB currently has a Moderate Buy rating among analysts, top-rated analysts believe these five stocks are better buys.
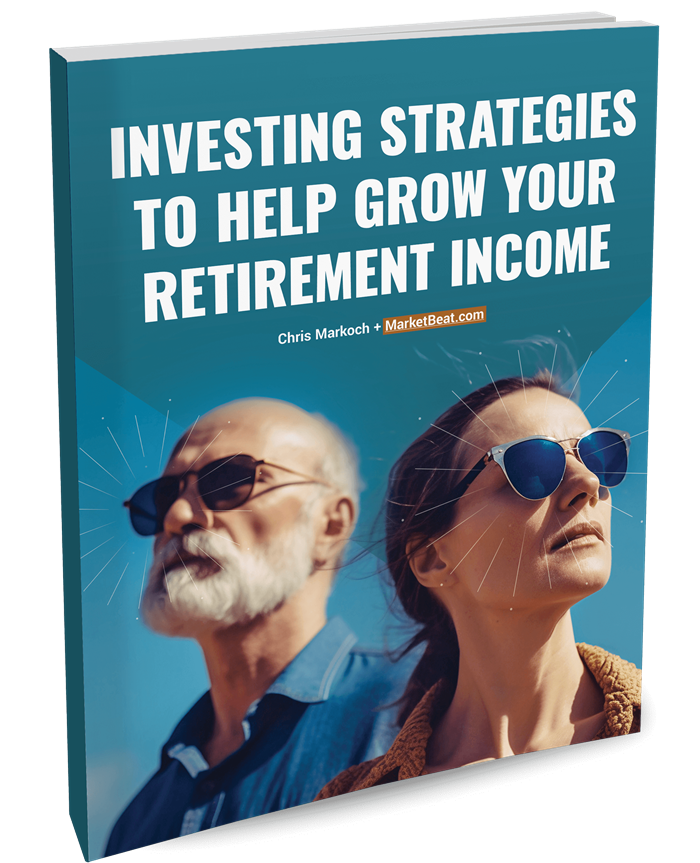
Need to stretch out your 401K or Roth IRA plan? Use these time-tested investing strategies to grow the monthly retirement income that your stock portfolio generates.
Article originally posted on mongodb google news. Visit mongodb google news
Java News Roundup: WildFly 36, Spring Milestones, Betas for Open Liberty, Hibernate, LangChain4j
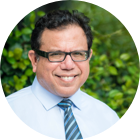
MMS • Michael Redlich
Article originally posted on InfoQ. Visit InfoQ
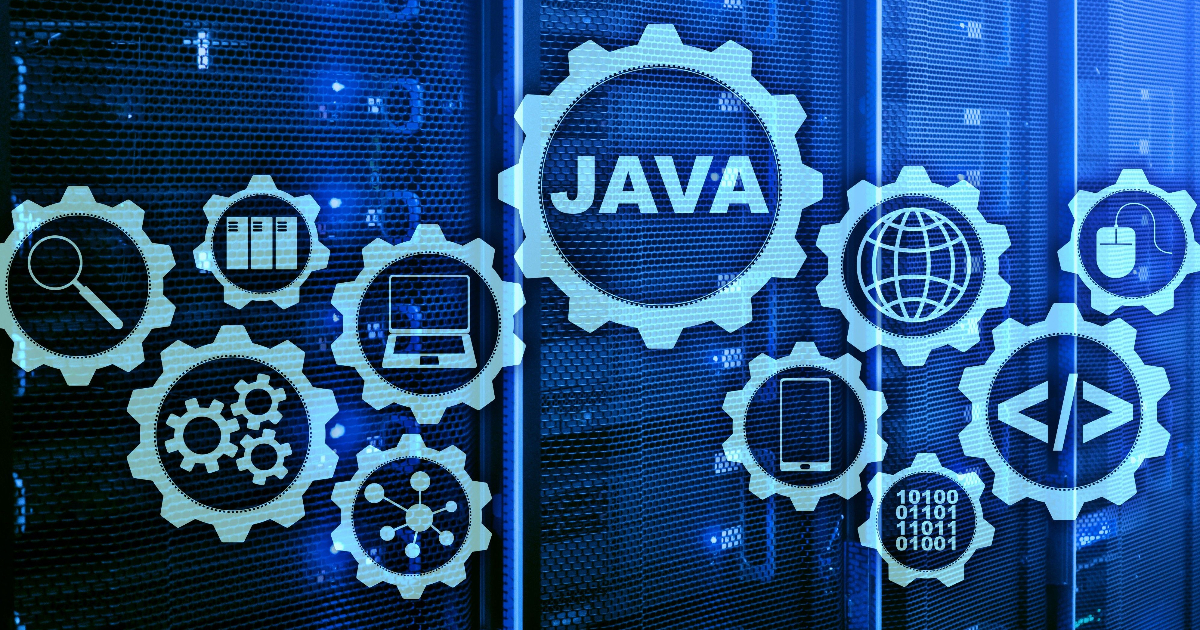
This week’s Java roundup for April 7th, 2025 features news highlighting: milestone releases of Spring AI 1.0 and Spring Cloud 2025; beta releases of Open Liberty 2025.0.0, Hibernate Reactive 3.0 and LangChain4j 1.0; and the sixth release candidate of Vert.x 5.0.
JDK 25
Build 18 of the JDK 25 early-access builds was made available this past week featuring updates from Build 17 that include fixes for various issues. More details on this release may be found in the release notes.
For JDK 25, developers are encouraged to report bugs via the Java Bug Database.
Jakarta EE
In his weekly Hashtag Jakarta EE blog, Ivar Grimstad, Jakarta EE Developer Advocate at the Eclipse Foundation, provided an update on Jakarta EE 11 and Jakarta EE 12, writing:
We are getting closer and closer to finalizing the refactoring of the Jakarta EE Platform TCK. The goal right now is to have the Jakarta EE 11 Platform ready for release review by the end of May 2025. Stay tuned for updates.
Plan reviews for Jakarta EE 12 are going on as we speak. Check out the progress in the Jakarta EE 12 Plan Review status board. My guess is that we will see quite a few more coming forward as the April 15 deadline is fast approaching.
The road to Jakarta EE 11 included four milestone releases, the release of the Core Profile in December 2024, the release of Web Profile in April 2025, and a fifth milestone and first release candidate of the Platform before its anticipated release in 2Q 2025.
Spring Framework
The seventh milestone release of Spring AI 1.0.0 features several breaking changes that align with the structural improvements to “create a more modular and maintainable codebase while reducing unnecessary dependencies in your applications.” Core architecture improvements include: a comprehensive modular architecture; modular autoconfiguration; package reorganization; and framework enhancements. This will be the last milestone release as the team plans a release candidate and the GA release in time for the Spring I/O conference in May 2025.
The third milestone release of Spring Cloud 2025.0.0, codenamed Northfields, features bug fixes and notable updates to sub-projects: Spring Cloud Kubernetes 3.3.0-M3; Spring Cloud Function 4.3.0-M2; Spring Cloud Stream 4.3.0-M3; and Spring Cloud Circuit Breaker 3.3.0-M3. This release is based upon Spring Boot 3.5.0-M3. More details on this release may be found in the release notes.
Versions 4.3.0-M3, 4.2.1, 4.1.6, 4.0.10 and 3.1.10 of Spring Cloud Config have been released to address CVE-2025-22232, a vulnerability where, under certain conditions, the Spring Cloud Config server may not use the Spring Cloud Vault token sent by clients using a X-CONFIG-TOKEN
header.
WildFly
The release of WildFly 36 delivers notable features such as: support for MicroProfile 7.0; experimental support to launch WildFly from JBang; improvements to the WildFly AI Feature Pack; support for the Prometheus Registry in the micrometer
extension; and enhancements to the WildFly documentation page. More details on this release may be found in the release notes.
Open Liberty
IBM has released version 25.0.0.4-beta of Open Liberty featuring support for JDK 24; a file-based health check mechanism with MicroProfile Health as an alternative to the traditional /health
endpoints; and InstantOn support for the J2EE Management 1.1, Application Client Support for Server 1.0, Jakarta Application Client Support for Server 2.0 and Web Security Service 1.1 features.
Hibernate
The third beta release of Hibernate Reactive 3.0.0 ships with: the additional of an upsertAll()
and upsertMultiple()
methods to the implementation of the Hibernate ORM StatelessSession
interface; and a resolution to an UnexpectedAccessToTheDatabase
exception upon merging a detached entity with annotated with @OneToMany
or @ManyToMany
. This release is compatible with Hibernate ORM 7.0.0-M5. More details on this release may be found in the release notes.
Eclipse Vert.x
The sixth release candidate of Eclipse Vert.x 5.0.0 provides new features such as: support for the Java Platform Module System (JPMS); and a new VerticleBase
class that replaces the deprecated AbstractVerticle
class due to the removal of the callback asynchronous model in favor of the future model. The team anticipates the GA release to be available in May 2025. More details on this release may be found in the release notes and list of deprecations and breaking changes.
Apache Tomcat
Versions 11.0.6 and 9.0.104 of Apache Tomcat (announced here and here, respectively), deliver new features: the Java Runtime Environment (JRE) in no longer required to provide an implementation of the Message-Digest Algorithm (MD5) specification; improvements to the JsonErrorReportValve
class that eliminates a NullPointerException
; and improved handling of the %nn
URL encoding in the RewriteValve
class. More details on these releases may be found in the release notes for version 11.0.6 and version 9.0.104.
LangChain4j
The third beta release of LangChain4j 1.0.0 provide notable changes such as: support for additional return types in the JSON schema of Structured Outputs; and support for streaming and observability in Amazon Bedrocks. Breaking changes include the removal of several deprecated interfaces that include ChatModelRequest
and ChatModelResponse
. More details on this release may be found in the release notes.
Analyst recommendations: Apple, Blackstone, Estee Lauder, Mongodb, Stellantis… | MarketScreener
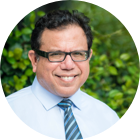
MMS • RSS
Posted on mongodb google news. Visit mongodb google news
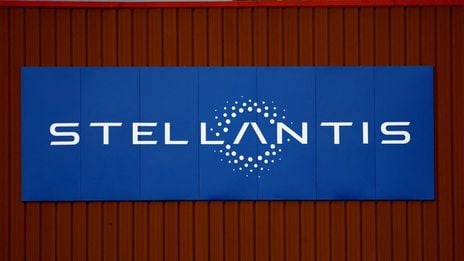
Every morning, find a selection of analysts’ recommendations covering North America and the UK. The list only includes companies that have been the subject of a change of opinion or price target, or of a new coverage. It may be updated during the session. This information can be used to shed light on movements in certain stocks or on market sentiment towards a company.

Article originally posted on mongodb google news. Visit mongodb google news
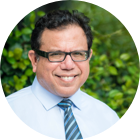
MMS • Thomas Much
Article originally posted on InfoQ. Visit InfoQ
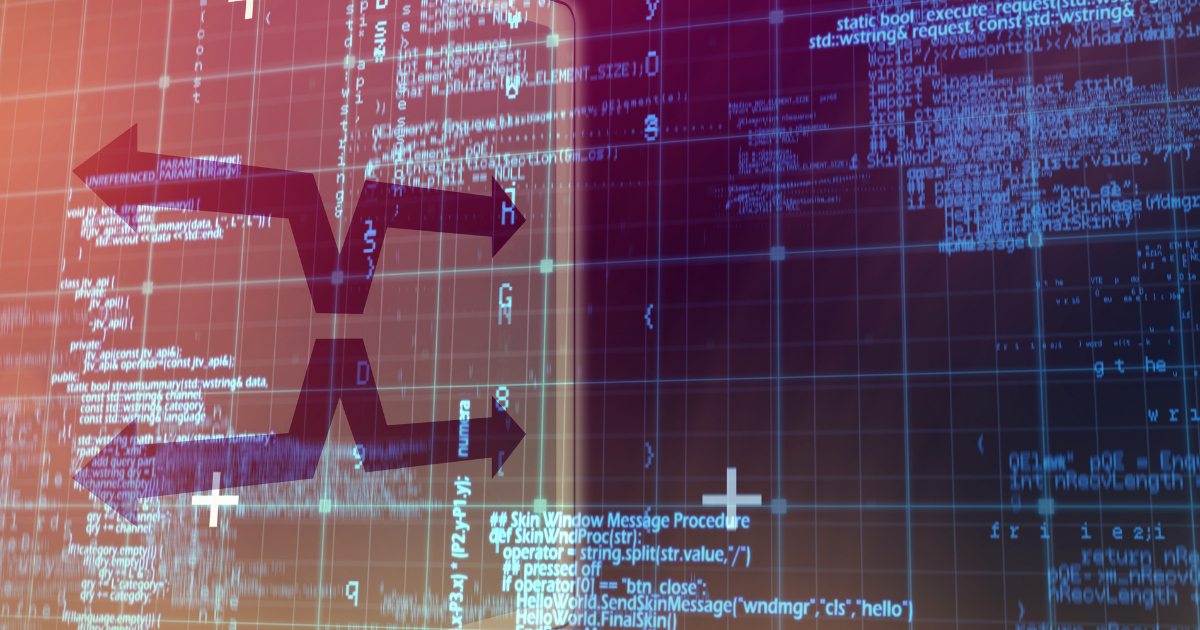
Key Takeaways
- Software architecture must evolve to keep up with changing requirements.
- We need protection against erratic and unwanted changes, guardrails that ensure architecture evolution stays within the desired direction.
- Fitness functions provide such guardrails with objective measures; they can be thought of as automated (unit) tests for your architecture.
- With libraries like ArchUnit it becomes feasible to write fitness functions for the structural aspects of architectural fitness.
- Using fitness functions fosters discussions and collaboration between architects and developers.
Software, its size, its requirements, and its infrastructure environment evolve over time. Software architecture should evolve accordingly. Otherwise, we risk an architecture that no longer meets current and future operational and developmental requirements. We even risk the ability to implement feature changes and additions. Fitness functions are guardrails that enable continuous evolution of your system’s architecture, within a range and a direction, that you desire and define.
What are fitness functions?
Fitness functions come in various flavors and are applicable in different domains. Let’s focus on fitness functions for software here, more precisely software architecture. I like to use the definition from the book, Building Evolutionary Architectures, by Neal Ford, Rebecca Parsons, Patrick Kua, and Pramod Sadalage: “An architectural fitness function is any mechanism that provides an objective integrity assessment of some architectural characteristic(s)”.
In case that reads too vaguely: fitness functions offer us self-defined guardrails for certain aspects of our architecture. If we stay within certain (self-chosen) ranges, we’re safe (our architecture is “good”). If we’re outside those ranges we get fast feedback in our build pipeline, for example, or even faster if we run the fitness functions locally on our development machine. Developers and architects can react quickly, fix the violations, and make the code “fit” again.
An objective measure of each fitness function is important! Measurements and measures should not be based on personal opinion. For instance, “readability” is probably not a good fitness function: “Your code does not look readable to me. I’d write it differently”.
Objective numbers are a better choice: “We have 17 violations which is within our tolerable range of up to 20 violations. So we’re good for now. Let’s talk about changing the tolerable range to allow only 10 violations for the next iteration”. Or “We found two unwanted dependencies between the modules. We must remove these dependencies”. These measurements are objective, based on numbers. Possible actions are clear.
The purpose of fitness functions in evolutionary architectures
Fitness functions are the essential foundation for evolutionary architectures: They enable evolution in an advantageous direction. We can define what “advantageous” means in our context.
There will always be deviations in systems, and changes to the code, the architecture, and the product. Good changes (the ones that we need for the future, for the “survival” of our software) as well as bad changes that might endanger the viability of our system.
Fitness functions help us decide which deviations or changes are acceptable, which can be tolerated for the time being, and which deviations or changes we should revert. In that respect, architectural fitness functions are similar to unit tests. While the latter are used to assert certain characteristics of our domain code, the former are tests for our architecture. Of interest, there are tools that allow us to write architectural fitness functions in a unit test style.
Some examples of using fitness functions
Many projects already use some kinds of fitness functions, although they might not use the term. For example, metrics from static code checkers, linters, and verification tools (such as PMD, FindBugs/SpotBugs, ESLint, SonarQube, and many more). Collecting the metrics alone doesn’t make it a fitness function, though. You’ll need fast feedback for your developers, and you need to define clear measures: limits or ranges for tolerated violations and actions to take if a metric indicates a violation.
In software architecture, we have certain architectural styles and patterns to structure our code in order to improve understandability, maintainability, replaceability, and so on. Maybe the most well-known pattern is a layered architecture with, quite often, a front-end layer above a back-end layer. To take advantage of such layering, we’ll allow and disallow certain dependencies between the layers. Usually, dependencies are allowed from top to down, i.e. from the front end to the back end, but not the other way around. A fitness function for a layered architecture will analyze the code to find all dependencies between the front end and the back end. This function will issue a warning or maybe even “break” (stop) the build if it finds a dependency from the back end to the front end.
For example, a simple fitness function to assert correct layering might look like this:
noClasses()
.that().resideInAPackage("..backend.".)
.should().dependOnClassesThat().resideInAPackage("..frontend.".);
I’ve used ArchUnit for Java for most of the examples shown here. ArchUnit is a small library that you include as usual as a dependency on your project. The ArchUnit fitness functions, or “rules”, can be executed by any unit testing framework; there is a convenient integration into JUnit.
If you have more layers, this simple approach will become cumbersome. For such cases, ArchUnit offers predefined rule sets like layeredArchitecture()
, where defining your structures becomes more declarative, less tedious, and less error-prone. JMolecules, a library that builds upon ArchUnit (and other tools), goes even further, allowing you to put tags (markers) onto your structures (package Annotations in Java). Executing the fitness function becomes an expression as simple as
JMoleculesArchitectureRules.ensureLayering();
JMolecules is the Java implementation of the xMolecules project, which is available for .NET and PHP as well.
There are other styles and patterns, like vertical “slices”, which better support domain-based architectures. There are also inside-outside patterns like “hexagonal” and “clean” as well as “onion” architectural styles, where the idea is to separate the (domain) core of your application, which is under your control, from the outside world (external systems) that often you cannot control as much. We can easily write fitness functions for the allowed and disallowed dependencies that enforce the respective patterns.
For example, to assess that our vertically sliced domains are free of cyclic dependencies, we could write an ArchUnit fitness function as follows:
slices()
.matching("com.myapp.(*).".)
.should().beFreeOfCycles();
We can even combine these patterns and implement multiple fitness functions. Quite often that makes sense, depending on your context.
Not only can we use fitness functions for the dependencies between structures, we can also use them to improve the code design within one structure. If, for example, the architects intend to make the API of existing modules independent from implementation details, we could write an ArchUnit fitness function like
noClasses()
.that().resideInAPackage("..api.".)
.should().dependOnClassesThat().resideInAPackage("..impl.".);
You’ll find more examples on the projects’ web pages. I’ve collected some examples in my GitHub repo as well.
How fitness functions and agility relate to each other
Agility is the ability to quickly adapt to change. This ability requires a certain fitness, in both nature (imagine a gazelle) and sports, as well as in software architecture. If your software products lack the necessary fitness, the need to move quickly (i.e. the need to implement changing requirements) leads to pain, stumbling, and deterioration.
Fitness functions help us gain and maintain fitness in certain aspects that we consider critical for the evolvability of our systems. This way, they enable agility on an architectural level. And that’s interesting, because changing (evolving) the architecture of a currently successful software system often clashes with how governance is implemented in many larger companies. Governance is seen as an obstacle to change, an impediment to agility.
But governance is probably not the problem, long and slow feedback loops are. If your developers get feedback on violations in their code, in their implementation of the desired architecture, weeks or even months after they’ve worked on a feature, you will earn their frustration.
Fitness functions “shift left” governance to the development teams to give them fast feedback, while they’re working on the code. That shift shortens the feedback loop dramatically. Governance might even be seen as an “enabler” for agility, because suddenly it becomes a safety net that springs into action.
We don’t need late inspections; instead, teams can ensure compliance with our architectural guidelines right from the start, in an agile way. Playing with a famous quote: You can’t inspect (architectural) fitness into a frail system, it must be built into it.
Bridging the gap between architects and software developers
Large enterprises tend to prescribe architectural rules from somewhere “above”. That approach often does not work well and yields unnecessarily complex systems, because developers will not understand the intent of the rules and the big picture of the enterprise architecture.They will unintentionally write code that undermines the objective of the rules.
Small startups or “agile shops” tend to ignore architectural rules or leave architecture decentralized to hopefully capable developers in their respective teams. This lack of awareness can easily lead to architectural chaos. Again, a big picture of an overall architecture is easily missed.
The best architects I’ve met offer guidance, not prescription. They offer a direction in which to think and to design the code. With fitness functions, architects can write their intentions into executable code. Even better, architects and developers can discuss their architectural rules together and the developers can write the fitness functions themselves. This collaboration fosters communication about architecture and the intent (and meaningfulness) of certain rules.
By “shifting left” the assessment of architectural characteristics into the development teams, architecture becomes a concern of all the people involved in building the software, rather than a few in certain positions or job descriptions. By implementing this approach, architects can focus on explaining and teaching and can keep an eye on the overall architecture. Architecture becomes a role that many people can take: developers as well as architects. This approach closes the gap between architects and software developers step by step, fitness function by fitness function.
Architecture as a team sport – not limited to architecture teams
Fitness functions take architecture out of its silo, out of the heads of fixed job positions. Now, all the people involved in software development are concerned with a viable architecture and its further evolution.
Fitness functions are simultaneously safety net, documentation, and architectural evolution enabler. Because many brilliant people now regularly think about architecture and its implementation in code, the architecture improves, not only within the products, but overall.
Another thing I noticed when architects and developers collaborated on fitness functions: those teams and departments usually worked in an atmosphere of creativity and innovation. I’m not sure if fitness functions are the cause or the consequence, but there probably is a correlation.
Fitness functions – a silver bullet?
It almost sounds too good to be true. Shouldn’t every project and every team use fitness functions then? Well, more and more are, at least in my observation. However, their use is often confined to the more obvious structural fitness functions that we saw above.
It’s rather hard to identify and define good fitness functions apart from the structural ones, especially with regards to if a function is violated for:
- Runtime behaviors such as network traffic between services
- Properties of data products like adherence to data contracts
- Output of GenAI solutions to stay within certain ranges
It’s definitely possible to define good fitness functions, but it requires some experience, some experimentation, and sometimes some unconventional ideas. So, starting with the easier structural fitness functions is a good choice. There might be areas where having fitness functions in place is an obstacle rather than a useful tool. Consider the three stages of product innovation and development:
- Explore the domain
- Build the right thing
- Build the thing right
If you’re in the first stage and you don’t know the direction yet, fitness functions might impose too much unwanted direction. But in the second stage, using fitness functions might make sense already, although you might want to use rather broad guardrails. These guardrails may be narrowed in the third stage, where the desired direction should be clearer.
Alternatives to using fitness functions
Of course, we managed to build software systems for many years without the use of fitness functions. So we can always fall back to the previous way to assert architectural compliance: conducting architecture reviews and assessments. This might still be a valid choice, for example, if someone requires such explicit events. You can always do this in addition to using fitness functions, which might yield even better architecture implementations and understanding.
As these manual reviews and assessments are usually not (completely) automated, they come at the cost of speed and scalability. Companies that prefer architecture reviews and assessments usually have a centralized architecture, defined (and controlled) by an architecture team with a limited number of people.
If you see your architecture as static or you deliberately need to be slow in changing the architecture, manual reviews might be your first choice. Using fitness functions will still protect you from unwanted deviations from your target architecture.
Bottom line
If you haven’t heard of fitness functions yet or haven’t used them so far, it might be worth looking into them. They might not be suitable for each and every project, but most projects will benefit from using fitness functions in one or the other way, especially if maintenance is required over many years.
Fitness functions keep our software and its architecture “soft”, and continuously evolvable. This matters because change is not the exception, but the rule. Having some kind of guardrails gives us safety to implement changes in existing, successful software systems, which makes evolutionary architecture possible.
Even if you cannot use the libraries that I presented in this article, the concept of fitness functions is independent of programming languages and technologies.