Category: Uncategorized
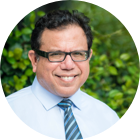
MMS • RSS
Posted on mongodb google news. Visit mongodb google news
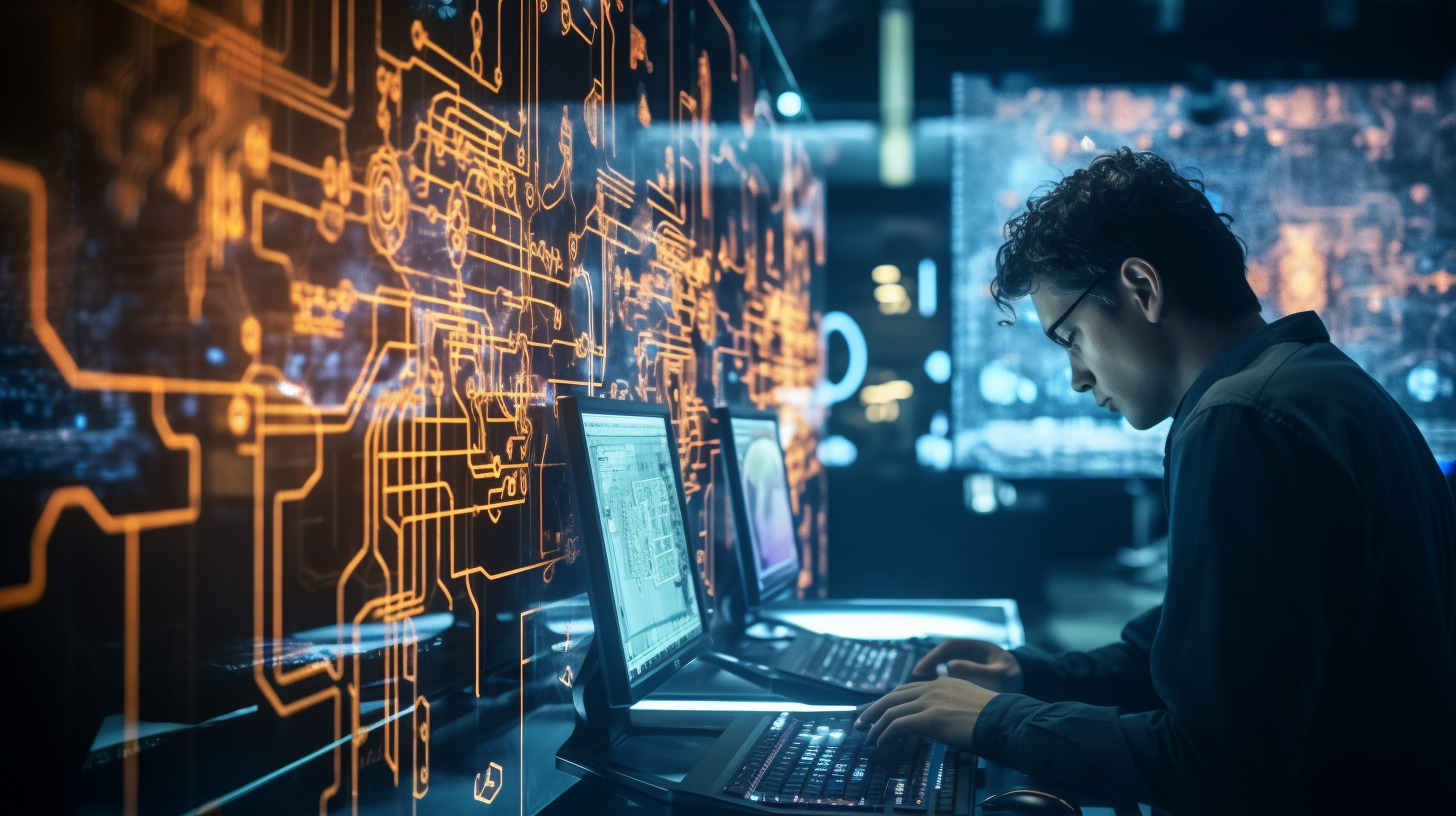
Artificial intelligence is the greatest investment opportunity of our lifetime. The time to invest in groundbreaking AI is now, and this stock is a steal!
The whispers are turning into roars.
Artificial intelligence isn’t science fiction anymore.
It’s the revolution reshaping every industry on the planet.
From driverless cars to medical breakthroughs, AI is on the cusp of a global explosion, and savvy investors stand to reap the rewards.
Here’s why this is the prime moment to jump on the AI bandwagon:
Exponential Growth on the Horizon: Forget linear growth – AI is poised for a hockey stick trajectory.
Imagine every sector, from healthcare to finance, infused with superhuman intelligence.
We’re talking disease prediction, hyper-personalized marketing, and automated logistics that streamline everything.
This isn’t a maybe – it’s an inevitability.
Early investors will be the ones positioned to ride the wave of this technological tsunami.
Ground Floor Opportunity: Remember the early days of the internet?
Those who saw the potential of tech giants back then are sitting pretty today.
AI is at a similar inflection point.
We’re not talking about established players – we’re talking about nimble startups with groundbreaking ideas and the potential to become the next Google or Amazon.
This is your chance to get in before the rockets take off!
Disruption is the New Name of the Game: Let’s face it, complacency breeds stagnation.
AI is the ultimate disruptor, and it’s shaking the foundations of traditional industries.
The companies that embrace AI will thrive, while the dinosaurs clinging to outdated methods will be left in the dust.
As an investor, you want to be on the side of the winners, and AI is the winning ticket.
The Talent Pool is Overflowing: The world’s brightest minds are flocking to AI.
From computer scientists to mathematicians, the next generation of innovators is pouring its energy into this field.
This influx of talent guarantees a constant stream of groundbreaking ideas and rapid advancements.
By investing in AI, you’re essentially backing the future.
The future is powered by artificial intelligence, and the time to invest is NOW.
Don’t be a spectator in this technological revolution.
Dive into the AI gold rush and watch your portfolio soar alongside the brightest minds of our generation.
This isn’t just about making money – it’s about being part of the future.
So, buckle up and get ready for the ride of your investment life!
Act Now and Unlock a Potential 10,000% Return: This AI Stock is a Diamond in the Rough (But Our Help is Key!)
The AI revolution is upon us, and savvy investors stand to make a fortune.
But with so many choices, how do you find the hidden gem – the company poised for explosive growth?
That’s where our expertise comes in.
We’ve got the answer, but there’s a twist…
Imagine an AI company so groundbreaking, so far ahead of the curve, that even if its stock price quadrupled today, it would still be considered ridiculously cheap.
That’s the potential you’re looking at. This isn’t just about a decent return – we’re talking about a 10,000% gain over the next decade!
Our research team has identified a hidden gem – an AI company with cutting-edge technology, massive potential, and a current stock price that screams opportunity.
This company boasts the most advanced technology in the AI sector, putting them leagues ahead of competitors.
It’s like having a race car on a go-kart track.
They have a strong possibility of cornering entire markets, becoming the undisputed leader in their field.
Here’s the catch (it’s a good one): To uncover this sleeping giant, you’ll need our exclusive intel.
We want to make sure none of our valued readers miss out on this groundbreaking opportunity!
That’s why we’re slashing the price of our Premium Readership Newsletter by a whopping 70%.
For a ridiculously low price of just $29.99, you can unlock a year’s worth of in-depth investment research and exclusive insights – that’s less than a single restaurant meal!
Here’s why this is a deal you can’t afford to pass up:
• Access to our Detailed Report on this Game-Changing AI Stock: Our in-depth report dives deep into our #1 AI stock’s groundbreaking technology and massive growth potential.
• 11 New Issues of Our Premium Readership Newsletter: You will also receive 11 new issues and at least one new stock pick per month from our monthly newsletter’s portfolio over the next 12 months. These stocks are handpicked by our research director, Dr. Inan Dogan.
• One free upcoming issue of our 70+ page Quarterly Newsletter: A value of $149
• Bonus Reports: Premium access to members-only fund manager video interviews
• Ad-Free Browsing: Enjoy a year of investment research free from distracting banner and pop-up ads, allowing you to focus on uncovering the next big opportunity.
• 30-Day Money-Back Guarantee: If you’re not absolutely satisfied with our service, we’ll provide a full refund within 30 days, no questions asked.
Space is Limited! Only 1000 spots are available for this exclusive offer. Don’t let this chance slip away – subscribe to our Premium Readership Newsletter today and unlock the potential for a life-changing investment.
Here’s what to do next:
1. Head over to our website and subscribe to our Premium Readership Newsletter for just $29.99.
2. Enjoy a year of ad-free browsing, exclusive access to our in-depth report on the revolutionary AI company, and the upcoming issues of our Premium Readership Newsletter over the next 12 months.
3. Sit back, relax, and know that you’re backed by our ironclad 30-day money-back guarantee.
Don’t miss out on this incredible opportunity! Subscribe now and take control of your AI investment future!
No worries about auto-renewals! Our 30-Day Money-Back Guarantee applies whether you’re joining us for the first time or renewing your subscription a year later!
Article originally posted on mongodb google news. Visit mongodb google news
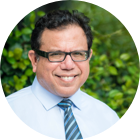
MMS • RSS
Posted on nosqlgooglealerts. Visit nosqlgooglealerts
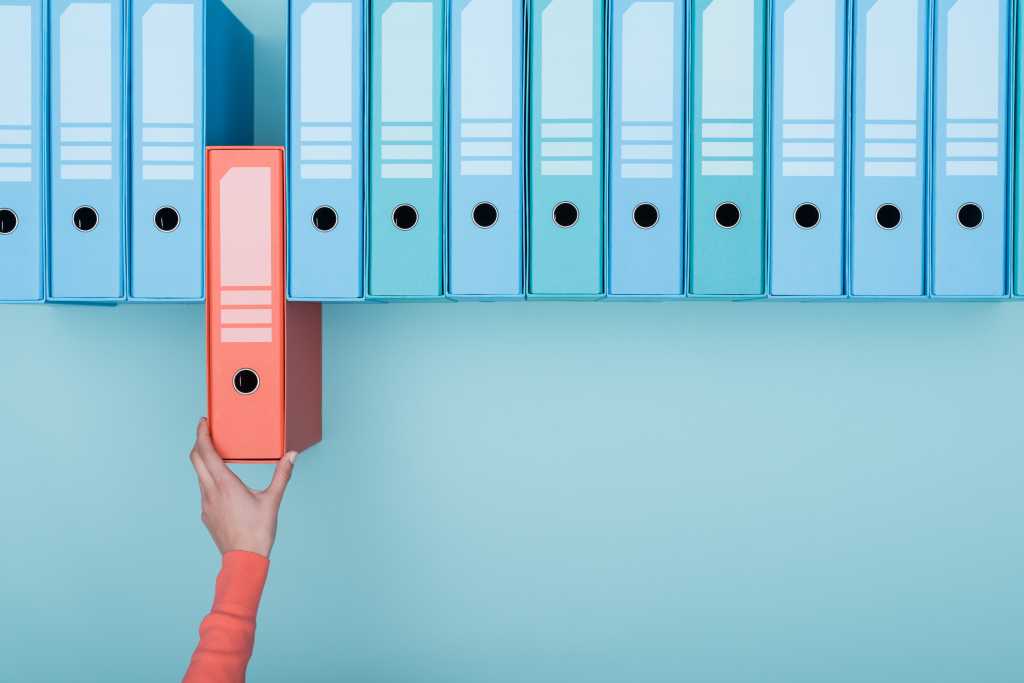
From the shell, you can embed API calls in select statements. This allows you to experiment with operations before adding them to calls from your code. The shell lets you build collections, add items, and experiment with CRUD operations. Other operations apply filters and support queries, as well as building indexes across one or more fields in a collection. You can find a lengthy list of documented API functions in the project wiki, grouped into common sets of operations.
For now, the GitHub wiki is the main source of documentation for DocumentDB. It’s a little on the thin side and could do with more examples. However, DocumentDB is currently intended for developers who want an alternative to MongoDB, one that’s available with an open source license rather than a source-available license. For now, as there’s no SDK, you’ll need to build your own calls to the API. These are based on MongoDB, so porting applications shouldn’t be too complex.
Why this? Why now?
The reasoning behind the DocumentDB project seems to be the big ambition to deliver a standard NoSQL API and engine, much like that developed for SQL. Microsoft has a lot of experience working in standards bodies, especially building and delivering the essential tests needed to make sure that any implementation of the resulting standard meets the necessary requirements.
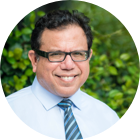
MMS • Aditya Kulkarni
Article originally posted on InfoQ. Visit InfoQ
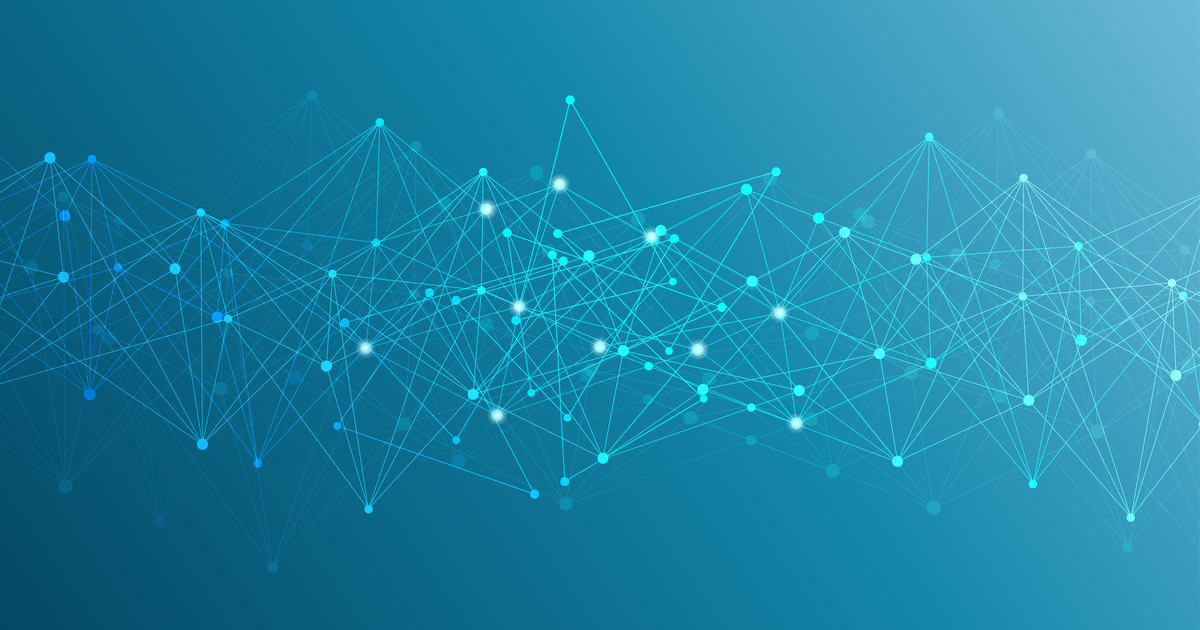
Git 2.48 now supports the Meson build system, a modern alternative to the existing Makefile, Autoconf, and CMake-based systems. This change addresses limitations in IDE support and maintenance issues with older systems.
According to Christian Couder, GitLab’s Git Expert, Meson offers several key advantages over previous build systems in Git 2.48. These include a user-friendly syntax, broad compatibility, support for modern features, and easy access to build options. The Meson build process involves setting up a build directory, compiling, testing, and installing using straightforward commands.
Git 2.48 has achieved a major milestone: it is now free of memory leaks, as exercised by its test suite. Previously, 223 test files had memory leaks, which were reduced to 60 by Git 2.47 and are now resolved. This accomplishment is aligned with the goal of “libifying” Git’s internal components (turning them into libraries) and optimizing memory usage. Unless exceptional justification is provided, new tests must now be leak-free by default.
Similar to regular fetches, fetches using bundle URIs can now undergo comprehensive validation using the fsck mechanism
. This enhancement provides granular control over what issues are accepted or rejected based on severity levels. Previously unavailable for bundle fetches, this feature improves repository safety and usability.
In Git 2.48, work on reference consistency checks has progressed further. The git-fsck
command can now detect issues such as invalid reference content or symbolic references pointing to non-existent targets. These checks were integrated into git-fsck
after being developed as part of the Google Summer of Code (GSoC) 2024.
The tech communities on Lobster and Reddit engaged in discussions and shared their opinions on the announcement. This conversation thread on Lobsters noted that Git and GitHub still rely on SHA-1, despite known vulnerabilities. While Git includes safety measures, concerns exist about interoperability issues and the potential for accidental triggering of collision detection mechanisms.
Furthermore, Reftables, introduced in Git 2.45 as a backend for storing references (e.g., branches and tags), have seen performance improvements through iterator reuse. Previously, reading references required creating new iterators for each operation, which was inefficient for repeated reads. Now, a single iterator can be reused across multiple reads, resulting in a 7% speedup in creating references during transactions with random reads and the potential for further optimizations by reusing more states within iterators.
Git 2.48 has also added support for migrating reflogs between backends using the git refs migrate
command. Previously, reflogs could not be converted between file-based and reftables backends during migration efforts introduced in Git 2.46. While this tool still cannot handle repositories with multiple worktrees, it removes a critical limitation for users adopting reftables in their repositories.
The ref-filter
subsystem, used by commands like git branch
, git tag
, and git for-each-ref
, has been optimized to improve performance when processing large numbers of references in repositories.
The original announcement blog highlights a number of contributors for their efforts and a complete list of contributors can be found in the project release notes.
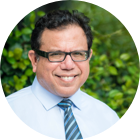
MMS • Edin Kapic
Article originally posted on InfoQ. Visit InfoQ
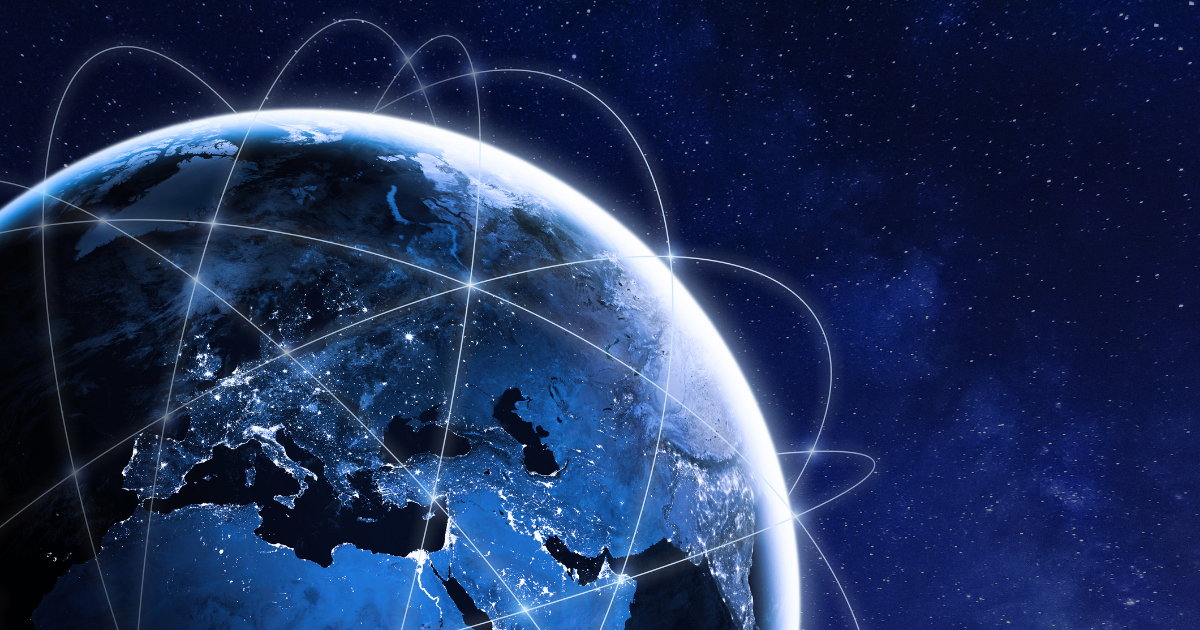
Fluent Assertions, a well-known .NET library that allows expressive asserts in unit tests, has launched version 8 with a proprietary license in partnership with Xceed, replacing the existing Apache 2.0 licence. The new license allows free non-commercial use, but any commercial use will require a paid license. The move has been negatively received by the developer community.
On January 13th 2025, Xceed Software published a notice of partnership with Fluent Assertions, where “Fluent Assertions will be integrated into (Xceed’s) suite of development tools”. The notice mentioned that the library will be licensed under a paid Xceed commercial license, but will continue to honour free licenses for open-source and non-commercial projects.
Xceed Software is a Canadian .NET component provider, with UI controls and other helper libraries such as .ZIP format tooling. Fluent Assertions is now shown as a library offered with a commercial license and support on the company website. The license for a single developer seat is stated to be 129.95 USD per year.
At the same time, the previous major version of the library, v7, will be kept “indefinitely” under its existing Apache license, allowing for free use in any kind of project, commercial or not.
Fluent Assertions was created by Denis Doomen, a Dutch developer, with the first version dating back to 2010. Since 2018, Jonas Nyrup, a Danish developer, has joined Denis in maintaining and evolving the library. Fluent Assertion is one of the most popular libraries in the .NET space, with over 450 million downloads on NuGet. It allows for more natural specification of asserts in unit tests, reading almost like a sentence in English.
string actual = "ABCDEFGHI";
actual.Should().StartWith("AB").And.EndWith("HI").And.Contain("EF").And.HaveLength(9);
The license change in v8 has led to a strongly-worded exchange on the project GitHub pull request comments. Developers argue that the price tag for the library is excessive, given its nature as a syntactic sugar for unit tests. Other developers think that maintaining all prereleases of the v8 under the existing Apache license while changing it to a proprietary license in the final pull request was a bad-faith move by the library maintainers. While the previous Apache 2.0 license allows derivative works with a different license, there are opinions among the developers that the change is against the license’s terms.
Some developers have mentioned that this move is reminiscent of other .NET ecosystem libraries that moved to commercial licenses, like the .NET drawing library ImageSharp or the Excel formatting library EPPlus.
Developers wanting to stay on the v7 branch should block the major version number in their .csproj file using a bracketed version tag to ensure that a package update doesn’t inadvertently put them in breach of the new license model.
One Fluent Assertions user has created a fork with the pre-release code of the library, still under the original license, with a new name of AwesomeAssertions. It is to be used as a direct replacement for current features only, without any evolution.
Alternatively, developers can remove the dependency on Fluent Assertions and use a similar library called Shouldly. There is an onboarding guide to Shouldly for Fluent Assertions users.
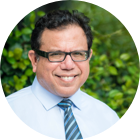
MMS • Ben Linders
Article originally posted on InfoQ. Visit InfoQ
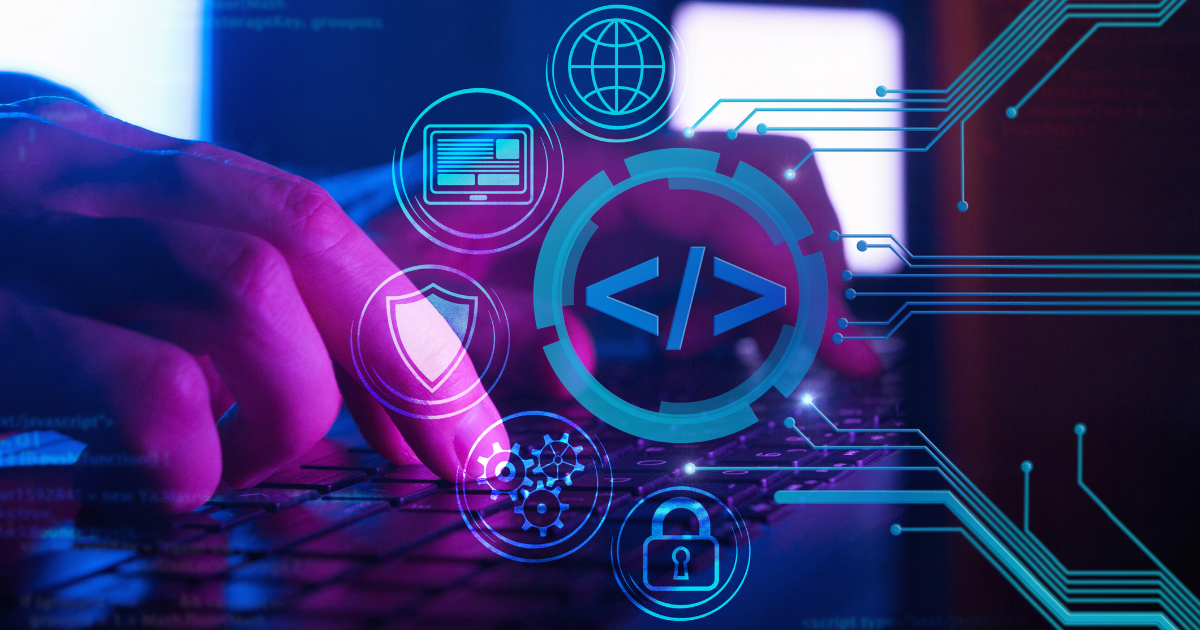
Web accessibility is about making web content available to users with disabilities. Development teams can use the success criteria of the Web Content Accessibility Guidelines to improve accessibility and create an inclusive website.
Joanna Falkowska gave a talk about creating accessible websites at DEV: Challenge Accepted.
There are different kinds of disabilities, Falkowska said. Think of users with sensory limitations (e.g. visual, hearing), physical ones (e.g. missing limbs), neurological diseases (e.g. Parkinson’s disease), or cognitive disability (e.g. Down syndrome).
The WHO estimates that about 16% of the world’s population is affected by significant disability:
If we add all of the mild ones, as well as groups that usually do not consider themselves disabled but might be facing similar problems, such as the elderly, the number becomes even higher.
Falkowska mentioned that development teams who do not know where to start their accessibility journey may feel overwhelmed by the number of disabilities they should take into consideration. They may have no idea about the limitations different users with disabilities face when opening their web app:
What they need is a benchmark that gives them clear criteria as to what makes an app accessible.
The Web Content Accessibility Guidelines (WCAG) is a document that serves people who create web apps. It has been drafted to make the web accessible and it gets updated every now and then to keep up with the development of the technology people use, Falkowska said. Just as the offline world can be more or less friendly for people with disabilities, the same way the websites that we browse can be more or less accessible, she added.
The WCAG gives you a set of success criteria that have assigned conformance levels, as Falkowska explained:
The lowest and the most basic one is A. The Web Accessibility Initiative (WAI), who is responsible for drafting and updating WCAG, states that any website we browse should address at least this level of conformance.
AA is a bit more complex, but at the same time it is the level that the authors (and many legal acts) recommend to follow, Falkowska said. The third and generally the most difficult to achieve is AAA. Usually the entities that address triple A will either be governmental/federal offices or companies/associations that serve specific groups of people with disabilities, Falkowska mentioned.
The levels are a bit like the wooden nesting doll “matryoshka”, Falkowska said; if you want to address AAA with your website, you also need to cover AA as well as A’s success criteria.
Falkowska mentioned that one of the most commonly missing success criteria tells you to add alternative text to any content that makes sense only if you can perceive it with your eyes. For example, if your website contains an image, a person who cannot see and uses a screen reader will only hear the name of the file, which may just be a meaningless string of numbers and characters. In order to make sure they can perceive this content in a meaningful way, developers add an “alt” attribute in html, Falkowska said. The content of this attribute will not be visible on the website, but the screen reader will read it to a person using assistive technology.
InfoQ interviewed Joanna Falkowska about the success criteria for an accessible website.
InfoQ: Can you give some examples of success criteria?
Joanna Falkowska: An example would be keyboard focus. It should be possible for the user to navigate all of the interactive elements with a keyboard, which is achieved with the “tabindex” attribute in html. It is beneficial not only for users with visual disabilities, but also to those who cannot use a mouse due to hand tremors, e.g. because of Parkinson’s disease. It is also a very good example of how a success criterion may support users without disability. I cannot count the number of situations when my mouse was discharged and I needed to navigate the web with a keyboard only while waiting for the mouse battery to fill up…
Another example is that of screen orientation (which is mainly important for tablets and smartphones). It should not be limited to landscape or portrait-only mode. Some users may use a mobile device in one orientation only. Think of the users with quadriplegia who have their phone attached to a special handle or a tripod. They cannot move their phone around. We should not lock their display to one specific orientation with the CSS rotate and/or transform property.
One of the newest success criteria discusses authentication issues the users may have. Authentication should not strain short-term memory with puzzles, so we need to make sure that our login feature allows for copy-paste and/or the use of a password manager. Make sure your input fields provide proper “label”, “type” and “autocomplete” html attributes. Apart from that, we should no longer require the users to solve the CAPTCHa in which the content needs to be deciphered and then typed into an input field. Object recognition is still allowed at AA level, but if you wish to succeed with AAA, even this type of CAPTCHa should be removed.
Presentation: Unleashing the Potential of VR: Building Immersive Experiences with Familiar Tools
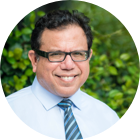
MMS • Ian Thomas
Article originally posted on InfoQ. Visit InfoQ
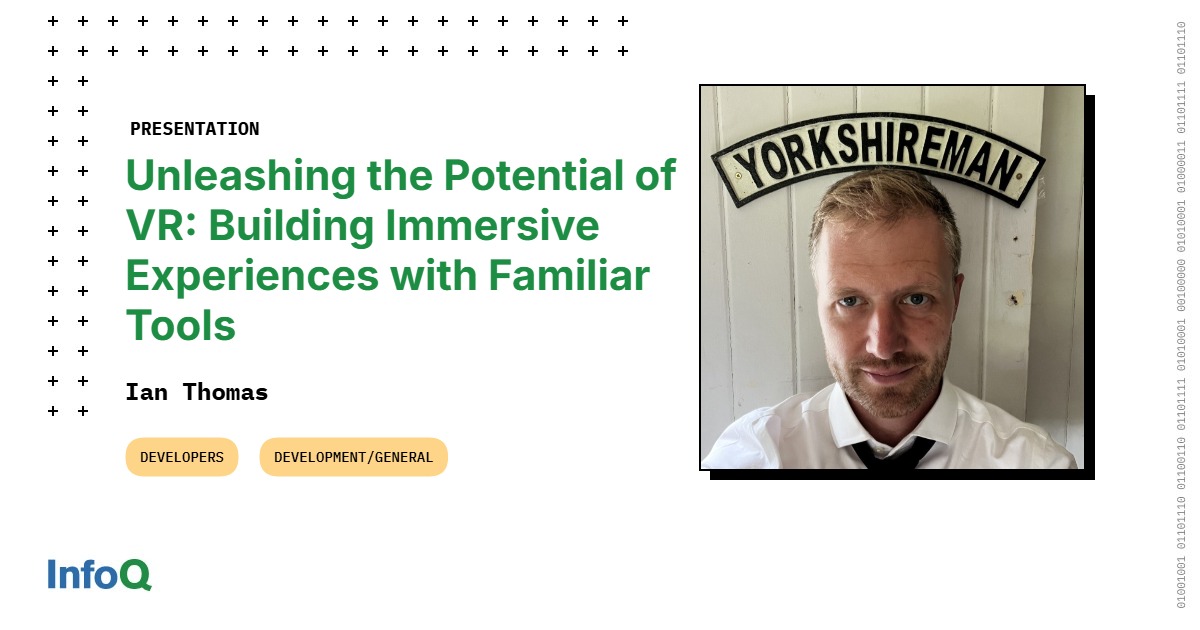
Transcript
Thomas: I’m going to talk about the potential of VR in the context of what we’re building at Meta. First, though, given this is the emerging trends in frontend, let’s go back 15 years to 2009. Some remarkable things happened that year, some less remarkable things. I’m wondering, does anybody remember this at all? This was the coolest thing I’d ever seen at the time. If I play this video, hopefully it will give you a demonstration of how that worked. This was something that North Kingdom produced. It was a Flash experience, if anyone can remember Flash. You held up the sheet of paper, stuff popped out, animations, you could blow on it. It was really cool. This was the most amazing thing I’d ever seen.
At the time, I was working as a designer in an agency. I thought, if an internationally renowned agency can do this, why can’t I? I had a play around with this. This is probably the first introduction I ever had to working in 3D. It was the last until about six months ago. It was really cool, though, and it opened my eyes to what’s possible for different things that you can do with 3D and how you can make immersive, interactive experiences that span between the real world and not.
Obviously, Steve Jobs came along not too long after this, and killed Flash quite dramatically. My nascent interest in that and generative art in Flash stopped, but thankfully, JS was there, and I started to pick up more of this kind of work. This quote from Brendan Eich in 2011 still holds true today, and I think it’s part of the theme of this talk, is that there are technologies that you can pick up as part of developing for more commonplace platforms like the web, that you can then transition and use throughout your development experiences in other platforms too. From that point, web technologies, they’ve come a long way.
Most people, I’m sure, will have experiences building for the web, potentially for mobile as well, maybe even using crossover technologies like Flutter or React Native to build for both using web technologies. The thing to think about is, if you’ve learned these technologies, what do you do if your primary delivery platform isn’t a desktop computer, a laptop computer, or a mobile device? What if it looks a bit more like this, where you’ve got multiple different sensors, you have real-world tracking. It’s head mounted. It’s immersive. You have very non-typical interaction patterns.
I’m Ian. I’m a software engineer at Meta. I work in Reality Labs as part of the Meta for Work area. I work on a product called Workrooms, which I’m going to be talking through, to give you a bit of a flavor about how we’re building some of our apps for VR and AR.
What Is Workrooms?
First, what is Workrooms? This is our immersive, collaborative office. It’s a way for you to be present with your colleagues without actually having to be physically present. I think, as much as I dislike advertising blurb and videos, they’re probably the best way to show you how this product actually works. This will give you a bit of a flavor about what Workrooms is.
We actually provide a few different experiences for Workrooms. There’s the main one I’m going to talk about in VR. There are also some that use the web and video. The web surfaces that we build are fairly standard for how Meta builds web applications. We build on top of a lot of the core infrastructure that powers things like Facebook and Workplace.
The VR surfaces for the Quest, we use Unity as our delivery platform or our environment for building this app. We have to support the different capabilities across the Quest line of headsets. I think we support from the 2 onwards. Then all of our backend systems. There’s a lot of background processing and real-time clients and stuff like that. That’s, again, provided by a lot of the core infrastructure that is powering things like Facebook and Messenger and Work Chat.
The Architecture of Workrooms
Workrooms as an application in a very simplified way. It involves headsets and clients as the main interaction point. You can join via the www client, which is a way for you to use your video camera and microphone. You can engage in a video call. The headsets provide the more immersive experience. We use a variety of different real-time systems in the background to provide Voice over IP or the 360 audio state sync for avatars, and various bits and pieces to support whiteboards and other features of the application. A lot of the backend is in the core stack from Meta. It’s GraphQL and framework TAO.
Obviously, that’s got the support of the scale of Facebook behind it. Developing for web is a standard process, where it’s a well-worn path. In Meta, this is one of the most easy to get going ways of building something. It’s very mature. You can spin up apps. You can work really quickly, use on-demand environments. It’s very familiar to a lot of people. React, obviously, being the primary frontend framework that we use there. Developing for VR is a different story altogether, because it’s far less mature. There’s less support for it across Meta. Then, when you look at the headsets as well, you’ve got new challenges that you have to adapt to. Things like the frame rate. It becomes increasingly important to maintain that, otherwise you can actually cause physical symptoms for people. Things like motion sickness and feeling head-achy and other unwanted side effects. The six degrees of freedom.
When I started working on 3D, again, 6DoF was like, why are we talking about this? It’s 3D, isn’t it? The six degrees of freedom is about tracking the position of your head and the location of your headset in the room. It’s one of the reasons why sometimes you’ll find it funny if you put your headset on when you’re on a flight that things start to whizz around in a really weird way, unless you put it in flight mode. Underlying the stack here we’ve got Unity and the Android open-source platform, which is what VROS is built on top of, and all the XR tech that we’ve got in Meta uses. Essentially, this is an Android phone strapped to your face.
To give you some context about the kind of challenges that you might have when you’re actually thinking about building for this thing. Rendering UI in VR is an interesting problem to consider. The main element that we talk about when thinking about rendering here is the compositor, because when your app is generating its scenes, you’ll end up with an eye buffer similar to this. In fact, you’ll end up with two, because we have two independent displays within the headset itself. If you were to project this straight onto the display as it is, it would look really bad, because they’re so close to your eyes, there’s like a pincushion distortion that’s there.
Instead, the compositor will have a few extra steps in the processing pipeline that eventually applies this barrel distortion to make it so that when the images are presented to the user, they appear to be correct and flat. Obviously, this is not a free operation. There’s quite a bit of work that goes into doing that.
What are the Limiting Factors of Scaling?
How does that affect us? What does that limit? The scaling effects of this mean that we have to think about how many people we can have concurrently in meetings, how many features we add to our applications, whether we enable pass-through because AR adds extra processing requirements. The key thing to bear in mind here is that perhaps when we talk about web, we’re talking about hitting 60 frames per second, but for us, we’re targeting something a little bit higher res, so we want to hit 72, which gives us just under 14 milliseconds to do all the work per frame.
Ideally, we’d like to aim for 90, because it’s an even smoother experience. It helps to make people feel more comfortable within VR. If I break that out into what the render cycle looks like, you can see, there’s a whole bunch of VR tracking going on. The CPU does a lot of work to generate the scene and process all of the business logic that goes on and within the application. Then the GPU will render at the scene. The compositor steps in, and that generates the final displays to go to each eye. What happens when you don’t hit that buffer, you don’t hit that 14 millisecond? If our GPU extends beyond where we want to render, then we drop frames, we have latency there. This is where we increasingly find the experience becomes very jarring for people, and we have to be extremely mindful that if we don’t hit this, that this is where the real-world effects, the physical symptoms can start to affect people dramatically. We can monitor this.
Oculus tooling has loads of great performance debugging tools. This is one that you can see within the application, but there’s also other ones using the Developer Hub, so you can use Perfetto to grab traces from the headset and see what’s going on. Here you can see that frame drop is represented by latency, and it’s relatively low in this example. If you’ve got a particularly heavy workload, and as I mentioned before, AR makes the workload even heavier because of the amount of sensor data it’s processing, this is something that you have to be extremely careful to make sure you’re not introducing excessive latency.
Compositor Layers
One way that you can improve things, especially when you think about elements of your UI that maybe need to be crisper, is to use something called compositor layers. Compositor layers are a way to bypass some of the processing that happens within the compositor itself. They’re a way of saying, I’ve got a texture, and I want to render it to a shape, and I want it to appear at this point in the scene. You avoid having to do some of the sampling that would decrease the quality of the images that are presented to customers.
At a high level, what you see here is the overlay. The quad overlays is a compositor layer. The eye buffer then has holes punched in it, which is where the UI will eventually be displayed. This represents here as these small blue rectangles underneath the compositor. They have the benefit of operating largely independently of the application, so they are guaranteed to run at the full frames per second of the compositor, which is at least as good as the frame rate of the app.
Hopefully, you can see here the difference that this has on the quality of rendering. For things like text, it’s extremely important that we have the ability to drop into this layer to show that the text becomes readable. Because again, we have a limited resolution within the headset itself. It’s incredibly important that when you’re presenting stuff, especially in a work environment, people can read it. You can see here how the compositor layer on the left eye frame buffer leaves an alpha channel. Hopefully, when you flick between the two, you can start to see the difference in the aliasing and the quality of the text.
How We Use JavaScript Across Workrooms
I mentioned about web technology, so let’s have a think about how JavaScript is used across Workrooms. I’ll go back to my high-level diagram here, and pop in some of the technologies that you might find. We can see that JS, React, and Jest play a part in both our web and our headset stacks. That’s because we found that React is a really effective way for us to enable people to work on the apps and build these 2D panels without necessarily having to become deeply expert in building VR applications. If I zoom out to look at a reasonably high-level view of this, the Unity app has a bunch of C# code in it. It also has a bunch of native libraries and native code that we write for performance optimization reasons.
Then, there’s also a whole load of JS, and the JS is largely where the UI resides. You can have a look at a slightly more grown-up version that’s on the Oculus developer portal to see how these things come together. Then they ultimately all talk to the OpenXR runtime that sits within the VROS layer in the headset. React, and specifically React Hermes and Metro, how do we use this? If I look at the UI for Workrooms, the key thing that you’ll notice is there are some 2D flat elements, and these are all the prime elements that are going to be part of the React render case. We have multiple components here that get rendered in different places, and these will sometimes end up as overlay layers in the compositor, or sometimes they’ll be rendered in the application itself.
React VR is a way for us, like I said, to take JS, build a texture and then integrate it within the Unity environment, and so that we can generate lots of UI quickly, and we can use all the familiar tooling that we have with React. It’s a really nice development experience as well, because we can enable things like hot reloading, where previously it would have been a bit more tricky, with something like C#. The renders, as with all these things, they look extremely familiar. If you’ve done any React development over the last however many years, you’ll recognize this instantly.
One of the nice benefits, although React VR is different, we have a different backend to React Native, we do have the ability to use some of the React Native components that are part of other applications, so things like animations can be brought in there. A key thing that’s made some of the things I’ll talk about later really easy to do is, building the render tree allows us to have addressable elements via test IDs, which is something that I’ll go to later on.
Obviously, there’s tradeoffs with this. Why would we choose React over Unity? The main thing is productivity. It is a lot quicker, and it enables familiarity. Engineers who aren’t necessarily native Unity engineers, or haven’t been working in 3D for long, they can onboard a lot more quickly. If we’ve got a UI that we want to share between parts of the web and some of the parts of the VR app, that’s possible too. Hot reloading, like I mentioned, there’s the component inspector. We can get the component hierarchy for test IDs, but it doesn’t completely absolve you of knowing about the underlying platform, so you still have to know a little bit about Unity and C# to be able to be effective.
One of the key nuances here is state management is a little bit different to how perhaps you would do this in a web application, where quite often we would keep state within components. We try and avoid that as much as possible, because of the need for rendering UI and the collaboration between different headsets, so multiple people using the app at the same time. Thankfully, the great John Carmack agrees that React is a good idea. After seeing it get deployed across the Oculus apps that we build, he came out and said that React definitely is a faster way of building UI than Unity. If John thinks it’s good, that’s fine by me.
It makes sense, because if you consider how things need to change and the rapid nature of building UI, they’re right at the fast, thrashy end of this spectrum. We don’t necessarily want to optimize for long, stable infra level stuff. We want to be thinking about how we can enable change as quickly as possible. Developer experience and ease of use definitely factor really highly in our decision making there. Perhaps some of the tradeoffs that we would get there, like performance, we can offset by the fact that we get much better productivity.
Creating a new panel is fairly straightforward. You write the component in JS, register it for Unity use. Then, because everything in Unity is a game object, we need to create one of those and use the component that we generate as an element on the game object, enable things like colliders so that we can have interactions, and then we position it within the scene. I mentioned about state management, and that’s where the React VR modules come in, because we don’t want to have too much of the state living within the JS code. We want to be able to communicate between JS and C# fairly easily. React VR modules allow us to do that in a really straightforward way. You can see here, within the application, you can choose the table layout for the room.
Obviously, this is something that you can’t have one layout and then another person in the meeting have a different layout. This is something that needs to be synchronized across everybody. We want to be able to maintain that state in a central place. Again, these modules are really simple to create. You define the type in JS, run some code generation, that’ll produce a JS class, and it will produce a C# interface. Then we implement that. It provides mocks so we can test and all sorts of other useful bits and pieces.
It’s a really straightforward way of making sure that we can enable easy interop between our C# and our JS layers. Performance is really important. You can see here, we have critical decisions to make around which UI is going to be rendered as a compositor layer, and which UI is going to be rendered as an application layer. We have a limit. There is only a certain number of compositor layers we can support, so we have to be really careful where we choose to use them. Typically, anything that’s going to be front and center, like notifications, we will try and make sure that they are rendered as crisply as possible. We also need to be mindful that we still want to have as much budget left for all the work that’s going on in the background.
Building in Quality
Quality, this is where things get quite interesting. Workrooms at a high level, like I say, is a multiplayer application. When you’re building these multiplayer applications, it seems difficult, it might be difficult to consider how you would test multiplayer scenarios. We also need to bear in mind that it’s not just VR, there’s VC users, there’s web users, and all of these different parts of the stack. They interact with each other, and they need to collaborate in a way that we can test in a useful manner. This is where we can look to some of the tooling that’s provided centrally by Meta. You might be familiar with this. This has been published about how Meta developer environments work, and how we build code and deploy apps. We rely as much as possible in a lot of this core tooling to make sure that we have full support for testability and managing our test environments and building apps that we can use in CI.
The key elements that I’m going to talk about are how we edit and how we test, and then how we review, and how we understand whether we’re shipping something that we think is of high enough quality. When we think about testing, we often talk about pyramids and how we want to balance between unit integration, end-to-end. Clearly, for us, end-to-end tests are going to be extremely important. However, they have some drawbacks, and we have to be really careful in our decision making to know whether we want to go really deep into the end-to-end area, or whether we would be beneficial just thinking about more integration unit tests. The trophy that you see here, has been put forward as a concept I think by Kent C. Dodds, as a way that web products can really benefit from the depth of coverage that you get from an end-to-end test.
If we think about this from a VR perspective, there are some drawbacks there that we have to be really careful to consider. Web, as I said, really mature. There’s loads of great tooling available, good browser support, battle hardened libraries like Puppeteer. There’s lots of value from your end-to-end tests, and they generally are not too flaky or too slow. The size and the scale of the www code base in Meta has proved that there’s lower reward for going for an integration approach here.
The end-to-end testing tends to win for us. VR, on the other hand, there’s less coverage here. It’s not something that’s so widely supported, because we’re quite a small group, really, compared to the web users. Because a lot of the developers are coming from a game development background, there’s still quite a heavy reliance on manual QA and play testing through people actually putting the headset on, creating meetings, joining them, and finding the issues in using human effort rather than automation. We don’t have wider support from a test framework, subset of features. Tests can be very slow. There’s also some really interesting challenges around the fact that they’re going to have to be on physical devices.
One of the problems that I’ve come across in the last few months was that when we bought new devices and they were put into our device labs, they were put at a different orientation. Bear in mind that we track where you are in physical space. That meant that when we were recording test videos and we were trying to assert on things in the environment, you were looking straight at the ceiling rather than at your desk or looking at the VC panel. Emulators are coming. That’s something that we’re investing heavily in, but they’re not quite there yet. One of the crutches that we have is that Unity is obviously a visual environment, so people tend to favor doing the quickest, easiest thing, which is sometimes writing the tests that run against the editor itself. Again, it’s not the same as the deployed artifact. It’s not the same as the thing that someone’s actually going to download and use on their headset.
I’m going to start this running in the background, because it’s a relatively long video. This is the sort of thing that you can see from a test when we write them. You can see we’ve got the two views here that are distorted, as mentioned before, from the compositor, and the environment loads up. We’ve joined the meeting as a single avatar, and then we’re using our test framework to cycle through the different parts of the UI and check that it’s working as expected, that things change, and we can assert on what’s present, what’s not present. We can check whether interactions that happen between JS and C# are working correctly. We can really dig into, is this thing doing what it’s supposed to?
The really cool thing about this is that we can have multiple devices at the same time in the same test doing the same thing, and we can assert that they’re all doing it in the way that we want to, which is critical when you consider that we’re also supporting multiple versions of the Quest headset. Some will have certain features. Some will have more power. The Quest 3, a much better device than the Quest 2 in many ways, but it also has different drawbacks and different things that we need to consider. The editor environment, like I said, is a standardized thing in Meta. This is generally where I spend most of my day. I look at Visual Studio code a lot. What you’ll notice is there’s an awful lot of stuff on the left-hand side, custom extensions. This is the key way that we can start to standardize and make testing easier and building quality easier.
As I said, we need to think about how we test on these physical devices. We’ve got a way now to avoid having people with local versions of the headsets, their instance, or whatever, their Quest 3, their Quest Pro, that they might have configured slightly differently to the way that it gets configured for a consumer. We have the labs available to us, and we can use a tool that we call RIOT, which stands for Run It Over There, and it allows us to lease devices very quickly using a visual workflow in Visual Studio code.
We can lease as many of these as we need to, to run our tests and actually start to use different versions of the device to validate our work. You can see here I’ve got some command line tooling as well. We’ve actually managed to completely inline this workflow. Now when you write a test, you can actually define the devices that you want within it and the application that you want within the test file. Everything is just a case of click and go, once you’ve got your device leased, which is amazingly streamlined.
The reason I’m talking so much about this is, again, this is another familiar tool. This is all built and operates using Jest, a framework that when I first started using it in 2014 felt like it was the worst testing framework in the world. It’s something that I now think is absolutely invaluable, and I spend pretty much my working life thinking about how we can make more of it, because it’s a really critical part of our quality arsenal. End-to-end testing looks a bit like this. As I mentioned, there’s a way for us to lease these devices and have different devices that we talk to. We don’t just limit ourselves to headsets, although that’s the primary focus of my work, is making sure that our tests are operating across multiple headsets at once. You can also have Android devices, iOS devices, and smart glasses, for example.
These tests, they’re very familiar to people, and they rely heavily on the Jest end-to-end framework that we’ve been working with internally. These tests will be familiar to anybody that’s written an end-to-end test for the web. It’s very straightforward. We have to pull our environment through to make sure that we’ve got access to the UI driver. Again, because we can have multiple devices leased for a test, we want to be able to have access to multiple UI drivers. This is the code that powers the test that I showed you in the video earlier. It’s a very straightforward, go find this thing, click it, go find this thing, click it. We can do things like pull back the text. We can assert that something is present, something isn’t present.
Again, this is where the power of the React component tree really helps us out and makes things so that we can understand the state that our application’s in. A little way down the middle there, you can see, I’ve got this sendSystemEvent. This is another way that we bridge between JS and C#. In the background, we have a small web server running on the device during tests. This allows us to say, can you please do this? Or, can you give me this state so I know what kind of seat layout I’ve selected, or have I managed to change the seat position that I’m in? If I zoom out one level and you look at the environment, you actually have access to the VR headset itself, which is another way that we can start to think about play testing in an automated fashion, all the things that we want to do that a user might do in the real world.
You can see here we have doff and don, which I find is quite quaint as an API. You can doff your headset, make sure that the device thinks it’s taken off, and then you can don it again. It’s a way for us to then assert things like, is the right UI appearing when someone puts the headset back on, is our app crashing, that kind of thing? You can see here, this lockView, this is something that I alluded to earlier. We noticed that certain headsets in certain configurations in the labs ended up pointing the wrong way, and so it was difficult to know whether something was actually working or not, because one thing would be perfectly set up, and another device would be pointing out the window or something.
Because we are building on top of Jest, that means we also get the full support of a lot of the developer tooling around the integration and CI part of the Meta life cycle. Here you can see, this is the analysis that we can pull back from a test to see what’s happening. We can put manual checkpoints in so that we know performance markers to see, is this thing getting faster or slower? What’s causing bottlenecks within our application? This is very high level, but you can also dive in to the device specifically. You can use something called dumpsys to go and really dig into the details of what processes we’re running and find out if there are any performance issues there. You can see as well we capture any crashes.
Unfortunately, this one looks like it did have a crash in it, though, weirdly enough, the test passed. There you go, a little hint at some of the interesting challenges around the flakiness of testing on VR. This then integrates further into our CI so that we can see the state of our application at any time and the state of our code that we’re writing. This is the Fabricator UI that’s part of everyone’s core workflow at Meta, no matter whether you’re working on VR, www, low-level infra systems. The key thing here, down at the bottom here, it says it’s landed, and we can see where it is in the deployment cycle. There’s also a whole bunch of really useful information here about which tests get selected and run. When you are deploying these apps and building them for different use cases, and we share a lot of code because we work in a monorepo, the ability for us to have our tests run on other people’s changes is massively important.
The fact that we’ve standardized this device leasing approach, we’ve standardized the way that we go through the process of running tests, and we’ve got the tooling, people will see these tests running on diffs that they didn’t even know were going to touch something to do with Workrooms. We get an awful lot of signals. You can see here, we’ve got 2500 different checks, and lints, and things that have run.
Key Takeaways
The key things from my learnings of VR, is that the React programming paradigm has really unlocked the power for us to bring people on board quickly. I’ve never really dug into game development. I’ve never really dug into C#. I’ve never really worked in any kind of 3D environment at any great depth, but I was able to onboard within several weeks and was productive writing tests, building code, and getting the app deployed. That’s partly because it was so familiar, because React allowed me to do that.
Jest as well, because of the familiarity of Jest and the way that you can leverage the existing experience you’ve had, maybe building for the web or mobile using it, it allowed me to get in and dive into how we can validate the quality of our application quickly. It means that there’s no overhead to onboarding. I can really make efficient tests. I can understand the state of things. I can treat it like a browser, and I can also interact with different devices at the same time. That doesn’t absolve us of learning about the different platforms, so there’s still a lot to do. You still have to understand how things work underneath, things like the compositor, compositor layers, the render pipeline, the problems that come with performance.
You gradually have to onboard to those as well and understand where React benefits you, but also where React maybe offers you some of the limitations. It’s amazing what you can do with the web platform, so investing in your skills in that area is definitely a worthwhile thing. Always bet on JS.
Questions and Answers
Participant 1: Do you use those Jest tests for any of the performance concerns, or is it just like, this button shows up and I can click it?
Thomas: Can we use the Jest tests to understand some of the performance concerns? Yes. The way that we have test suites set up is we have some that run specifically to validate the UI, some to understand how the multiplayer aspects work. We spawn lots of avatars and see what happens if we have loads of people trying to do the same thing at the same time. Then we also have tests that turn off as much of the debugging and insights as possible, and just hammer the environment.
Workrooms as a product is one of the most performance intensive applications that you can put on the Quest platform, because we’ve got video streams coming in. We’ve got multiple avatars that have got real-time data being synced between them. You’ve got remote desktop, so you can bring your laptop or your desktop computer into the environment as well, screen sharing. Then, you can also turn on pass-through. There are all these different things that really stress you out. Being able to set that up using some of the tooling that you have with the VR device API allows us to really go, let’s just run this for 10, 15 minutes and see what happens. How many crashes do we see? Do we see much in the way of drop-offs in the frame rate?
Participant 2: How far do you think we as an industry are able to have VR as the first-class citizen as we have with mobile? How far we are from this? If you believe that it will be possible to render my React Native apps in devices like Quest automagically, like we do with iOS and Android.
Thomas: Can we use React Native to render apps for Quest? How far are we from this being as prolific as mobile?
When you go onto the Quest store and you see various apps, a lot of the panel apps that you see are React apps, and they’re built with React Native. Yes, that’s going to be possible. I don’t know that we have the same capability at the moment that you might think with the Vision Pro, where they can take an iPad app and they can put it into the VR space. It’s not wholly impossible, but yes, from a React Native point of view, we definitely do that. A lot of the 2D panel apps that you will interact with do use that a lot.
VR as a First-Class Citizen
In terms of the proliferation, probably one of the biggest challenges from a product experience perspective is the friction of actually putting the device on and the time it takes you to get into the meeting. If you’re comparing to like a VC call, it’s pretty quick. You click the link and it spins up and your camera comes on and off you go. Matching that, I think, is a key element of making it more of a ubiquitous experience. I think that will apply broadly to different types of applications as well. Once you’re in the environment, and as long as the comfort level is there, the heat in the device isn’t too high, yes, there’s a lot of things that you can do in there that actually make you think, yes, this is my first choice. An example of that is one of the features in Workrooms.
When you launch the app, you go into what we call the personal office, and in there, you can connect using the remote desktop software, and you can have three screens. Those three screens offer you much more real estate, perhaps, than you would have just working on a laptop or whatever. Because the devices are pretty small that you take it to a coffee shop or take it somewhere on holiday. I’ve been using it in my hotel room while I’ve been in London. I’ve now got this really expansive thing. For me, the benefit of working in that means it becomes a natural choice. Over the coming years, as I’m sure, hardware will get better and the displays have higher resolution, that will become more appealing and you’ll think, this is the sort of thing that I will definitely reach for my headset first, and maybe not my laptop, or maybe a combination of both, or mobile.
Participant 3: I’m really curious about how closely related Meta’s teams are around the software and the hardware pieces. For example, as you’re building stuff in React VR, as you’re building Workrooms, how much influence does your group have on in terms of what comes next for Quest v next, or insights you have about what might be available in the future? How closely that tracks.
Thomas: How closely do the software teams work with the hardware teams within Reality Labs? Can I share any insights about the future?
I can’t really share any insights about the future, but if you go onto The Verge, they somehow found all the information about our hardware roadmap last year. Maybe have a look at that.
In terms of the collaboration between software and hardware teams, like most software now, there’s multiple layers of abstraction. The teams that we engage with the most are the VROS teams and some of the teams in the XR world who are looking at the core system libraries to support things like spatial audio, guardian boundaries. We have less influence on the hardware roadmap. Even internally that’s quite well protected and guarded. We do have to know quite early doors what’s coming. If you look at the move from the Quest Pro to the Quest 3, one of the things that we gained was really superior pass-through, but we lost the eye tracking support and the face tracking support.
Which for us as Workrooms, that’s a really great feature, because part of the draw of being in that product and using it as a meeting is the body language and the feeling that you’re actually connected with somebody in the same area, but you’re not necessarily physically located together. The facial aspect of that was really powerful. Knowing what’s coming on the hardware roadmap and maybe understanding the implications that has for us, that’s critical, but it tends to be more of a feed-in rather than us influencing it.
Participant 4: What do you see as the key limiting factor in achieving what you’d like to be able to achieve at the moment. What do you think about what Apple are doing in this space, because it seems to be a different direction to where you’re going.
Thomas: What’s the limiting factor for our products? What are my thoughts on Apple and the Vision Pro and what they’re doing?
I think, personally, the big limiting factor is still the performance, because it is still a fairly low power device that you wear. We’re trying to get them as light as possible. We don’t want to have the battery pack that you clip to your boat, for example. The heat generated makes it uncomfortable and all that stuff. Seeing performance improvements as will naturally happen with hardware evolution is good. The quality of optics as well, making the lenses better and being able to support higher resolution, that will also help. Like I said before, it’s the time it takes for you to get into the environment, because they take a lot of compute to make happen these experiences, not just from the rendering point of view, but just the whole managing state and the real time and data aspect. Any performance gains we can get there to reduce the barrier to entry, I think is the critical thing.
In terms of Apple, I try to use one. I haven’t actually managed to get my hands on one yet. I think it’s really interesting the way that they’ve positioned their apps in their ecosystem to be more around individual usage. I know that there’s a bit more coming now with the personas and FaceTime. It did feel much more like an individual consumption device. I’m not sure I’m 100% on board with the wearing it for family gatherings and taking videos and stuff, because I think that’s a bit weird and intrusive. You might see, I’ve got my Meta Ray-Bans on.
I think this is a far more nice form factor for, “I’m at a family gathering. I’m going to take a quick photo, and I don’t want to get my phone out and be intrusive” .There’s obviously potential here, and there’s a lot we can do. I think the more competition there is, the more people are exploring this area, the more beneficial it will be for everybody. I’m really keen to see where it goes.
Participant 5: How much time do you spend in Workrooms, productive?
Thomas: How much time do I spend in Workrooms, productive? I probably use it for an hour or two a day, depending on the meetings that I’m in. Obviously, there’s an added incentive, because it’s the product that I work on, so I tend to want to dogfood it a bit more. Workrooms as a collaboration platform is widely used across Meta. A lot of it is still video calling, but it is heavily used. We have work from VR Days, where they count as in-office days, so people can have an extra day at home, and they can use their headsets, and they can join meetings in VR. What we found through that is actually people are starting to use the personal office a lot more as well, for reasons like I said, you don’t necessarily have to have three massive physical monitors in your workspace. You can sit there and you can be productive quite easily with it.
The thing that I enjoy about using the product is that I’m more present in the meeting. When you’re on a video call, it’s quite easy to get distracted, change tabs, fiddle with emails, go on to workshops, and the thing, whereas when someone can actually see you and your body language is there, you just are more present. The 360 audio, the spatial audio, I think that was the biggest thing. You can’t really see it in a video or understand it to actually experience it, but because it’s there in those specific places within a room, there’s less crosstalk and that awkward, “I’m sorry, you go”. That just kind of is gone. It’s much more efficient as a collaboration medium as well. Just need the widespread adoption of the devices and people to think about it as their first choice now.
See more presentations with transcripts
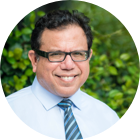
MMS • RSS
Posted on mongodb google news. Visit mongodb google news
Avior Wealth Management LLC trimmed its position in MongoDB, Inc. (NASDAQ:MDB – Free Report) by 90.2% during the fourth quarter, according to its most recent filing with the Securities and Exchange Commission. The institutional investor owned 134 shares of the company’s stock after selling 1,240 shares during the quarter. Avior Wealth Management LLC’s holdings in MongoDB were worth $31,000 at the end of the most recent reporting period.
Several other institutional investors have also modified their holdings of the company. Hilltop National Bank raised its stake in MongoDB by 47.2% during the 4th quarter. Hilltop National Bank now owns 131 shares of the company’s stock worth $30,000 after acquiring an additional 42 shares in the last quarter. Quarry LP lifted its holdings in shares of MongoDB by 2,580.0% in the 2nd quarter. Quarry LP now owns 134 shares of the company’s stock valued at $33,000 after purchasing an additional 129 shares during the last quarter. Brooklyn Investment Group acquired a new stake in shares of MongoDB in the 3rd quarter valued at about $36,000. GAMMA Investing LLC grew its stake in MongoDB by 178.8% during the 3rd quarter. GAMMA Investing LLC now owns 145 shares of the company’s stock worth $39,000 after buying an additional 93 shares during the last quarter. Finally, Continuum Advisory LLC increased its position in MongoDB by 621.1% during the third quarter. Continuum Advisory LLC now owns 137 shares of the company’s stock worth $40,000 after buying an additional 118 shares during the period. 89.29% of the stock is owned by hedge funds and other institutional investors.
MongoDB Price Performance
MDB stock traded down $7.26 during midday trading on Thursday, hitting $271.07. The stock had a trading volume of 2,240,897 shares, compared to its average volume of 1,653,422. MongoDB, Inc. has a 12-month low of $212.74 and a 12-month high of $509.62. The firm has a market cap of $20.19 billion, a PE ratio of -98.93 and a beta of 1.25. The company’s 50-day moving average price is $274.45 and its 200 day moving average price is $269.62.
MongoDB (NASDAQ:MDB – Get Free Report) last released its earnings results on Monday, December 9th. The company reported $1.16 EPS for the quarter, beating the consensus estimate of $0.68 by $0.48. MongoDB had a negative return on equity of 12.22% and a negative net margin of 10.46%. The firm had revenue of $529.40 million during the quarter, compared to analyst estimates of $497.39 million. During the same period in the prior year, the firm posted $0.96 earnings per share. The business’s quarterly revenue was up 22.3% compared to the same quarter last year. As a group, sell-side analysts anticipate that MongoDB, Inc. will post -1.79 EPS for the current year.
Insider Buying and Selling
In related news, CEO Dev Ittycheria sold 8,335 shares of MongoDB stock in a transaction on Tuesday, January 28th. The stock was sold at an average price of $279.99, for a total value of $2,333,716.65. Following the transaction, the chief executive officer now owns 217,294 shares in the company, valued at approximately $60,840,147.06. This trade represents a 3.69 % decrease in their ownership of the stock. The sale was disclosed in a legal filing with the Securities & Exchange Commission, which can be accessed through the SEC website. Also, insider Cedric Pech sold 287 shares of the company’s stock in a transaction dated Thursday, January 2nd. The stock was sold at an average price of $234.09, for a total value of $67,183.83. Following the sale, the insider now owns 24,390 shares of the company’s stock, valued at $5,709,455.10. This represents a 1.16 % decrease in their ownership of the stock. The disclosure for this sale can be found here. Over the last three months, insiders have sold 42,491 shares of company stock valued at $11,554,190. Insiders own 3.60% of the company’s stock.
Analysts Set New Price Targets
A number of equities analysts have recently issued reports on the stock. China Renaissance assumed coverage on shares of MongoDB in a research report on Tuesday, January 21st. They set a “buy” rating and a $351.00 target price on the stock. The Goldman Sachs Group raised their price objective on shares of MongoDB from $340.00 to $390.00 and gave the stock a “buy” rating in a research report on Tuesday, December 10th. Stifel Nicolaus upped their target price on shares of MongoDB from $325.00 to $360.00 and gave the company a “buy” rating in a report on Monday, December 9th. Rosenblatt Securities initiated coverage on MongoDB in a report on Tuesday, December 17th. They set a “buy” rating and a $350.00 price target on the stock. Finally, Mizuho upped their price objective on MongoDB from $275.00 to $320.00 and gave the company a “neutral” rating in a research note on Tuesday, December 10th. Two analysts have rated the stock with a sell rating, four have assigned a hold rating, twenty-three have issued a buy rating and two have assigned a strong buy rating to the company. According to MarketBeat.com, the stock currently has an average rating of “Moderate Buy” and a consensus price target of $361.00.
Read Our Latest Research Report on MongoDB
MongoDB Company Profile
MongoDB, Inc, together with its subsidiaries, provides general purpose database platform worldwide. The company provides MongoDB Atlas, a hosted multi-cloud database-as-a-service solution; MongoDB Enterprise Advanced, a commercial database server for enterprise customers to run in the cloud, on-premises, or in a hybrid environment; and Community Server, a free-to-download version of its database, which includes the functionality that developers need to get started with MongoDB.
Featured Articles
Before you consider MongoDB, you’ll want to hear this.
MarketBeat keeps track of Wall Street’s top-rated and best performing research analysts and the stocks they recommend to their clients on a daily basis. MarketBeat has identified the five stocks that top analysts are quietly whispering to their clients to buy now before the broader market catches on… and MongoDB wasn’t on the list.
While MongoDB currently has a “Moderate Buy” rating among analysts, top-rated analysts believe these five stocks are better buys.
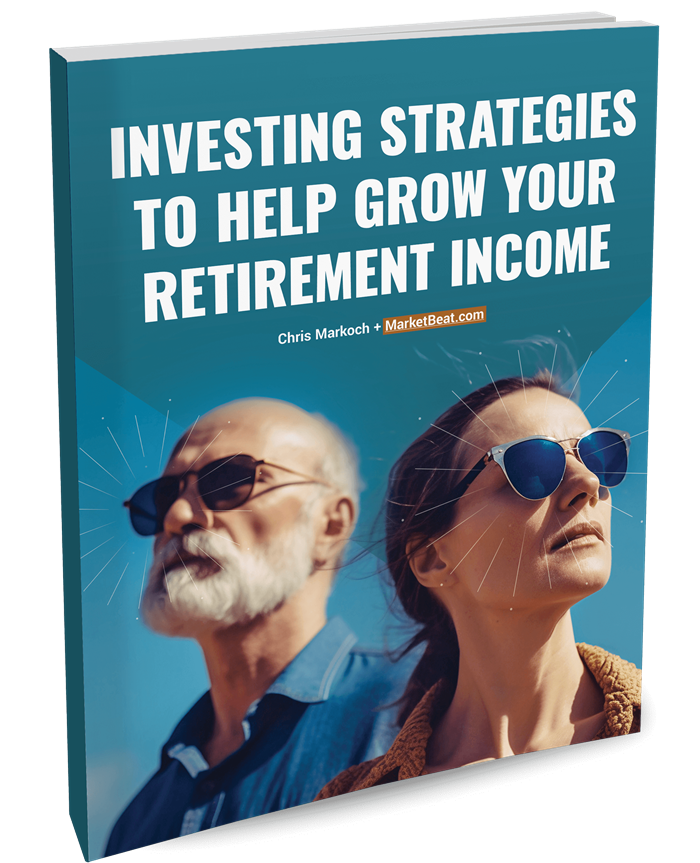
Need to stretch out your 401K or Roth IRA plan? Use these time-tested investing strategies to grow the monthly retirement income that your stock portfolio generates.
Article originally posted on mongodb google news. Visit mongodb google news
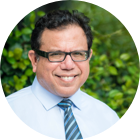
MMS • RSS
Posted on mongodb google news. Visit mongodb google news
Avior Wealth Management LLC trimmed its position in MongoDB, Inc. (NASDAQ:MDB – Free Report) by 90.2% during the fourth quarter, according to its most recent filing with the Securities and Exchange Commission. The institutional investor owned 134 shares of the company’s stock after selling 1,240 shares during the quarter. Avior Wealth Management LLC’s holdings in MongoDB were worth $31,000 at the end of the most recent reporting period.
Several other institutional investors have also modified their holdings of the company. Hilltop National Bank raised its stake in MongoDB by 47.2% during the 4th quarter. Hilltop National Bank now owns 131 shares of the company’s stock worth $30,000 after acquiring an additional 42 shares in the last quarter. Quarry LP lifted its holdings in shares of MongoDB by 2,580.0% in the 2nd quarter. Quarry LP now owns 134 shares of the company’s stock valued at $33,000 after purchasing an additional 129 shares during the last quarter. Brooklyn Investment Group acquired a new stake in shares of MongoDB in the 3rd quarter valued at about $36,000. GAMMA Investing LLC grew its stake in MongoDB by 178.8% during the 3rd quarter. GAMMA Investing LLC now owns 145 shares of the company’s stock worth $39,000 after buying an additional 93 shares during the last quarter. Finally, Continuum Advisory LLC increased its position in MongoDB by 621.1% during the third quarter. Continuum Advisory LLC now owns 137 shares of the company’s stock worth $40,000 after buying an additional 118 shares during the period. 89.29% of the stock is owned by hedge funds and other institutional investors.
MongoDB Price Performance
MDB stock traded down $7.26 during midday trading on Thursday, hitting $271.07. The stock had a trading volume of 2,240,897 shares, compared to its average volume of 1,653,422. MongoDB, Inc. has a 12-month low of $212.74 and a 12-month high of $509.62. The firm has a market cap of $20.19 billion, a PE ratio of -98.93 and a beta of 1.25. The company’s 50-day moving average price is $274.45 and its 200 day moving average price is $269.62.
MongoDB (NASDAQ:MDB – Get Free Report) last released its earnings results on Monday, December 9th. The company reported $1.16 EPS for the quarter, beating the consensus estimate of $0.68 by $0.48. MongoDB had a negative return on equity of 12.22% and a negative net margin of 10.46%. The firm had revenue of $529.40 million during the quarter, compared to analyst estimates of $497.39 million. During the same period in the prior year, the firm posted $0.96 earnings per share. The business’s quarterly revenue was up 22.3% compared to the same quarter last year. As a group, sell-side analysts anticipate that MongoDB, Inc. will post -1.79 EPS for the current year.
Insider Buying and Selling
In related news, CEO Dev Ittycheria sold 8,335 shares of MongoDB stock in a transaction on Tuesday, January 28th. The stock was sold at an average price of $279.99, for a total value of $2,333,716.65. Following the transaction, the chief executive officer now owns 217,294 shares in the company, valued at approximately $60,840,147.06. This trade represents a 3.69 % decrease in their ownership of the stock. The sale was disclosed in a legal filing with the Securities & Exchange Commission, which can be accessed through the SEC website. Also, insider Cedric Pech sold 287 shares of the company’s stock in a transaction dated Thursday, January 2nd. The stock was sold at an average price of $234.09, for a total value of $67,183.83. Following the sale, the insider now owns 24,390 shares of the company’s stock, valued at $5,709,455.10. This represents a 1.16 % decrease in their ownership of the stock. The disclosure for this sale can be found here. Over the last three months, insiders have sold 42,491 shares of company stock valued at $11,554,190. Insiders own 3.60% of the company’s stock.
Analysts Set New Price Targets
A number of equities analysts have recently issued reports on the stock. China Renaissance assumed coverage on shares of MongoDB in a research report on Tuesday, January 21st. They set a “buy” rating and a $351.00 target price on the stock. The Goldman Sachs Group raised their price objective on shares of MongoDB from $340.00 to $390.00 and gave the stock a “buy” rating in a research report on Tuesday, December 10th. Stifel Nicolaus upped their target price on shares of MongoDB from $325.00 to $360.00 and gave the company a “buy” rating in a report on Monday, December 9th. Rosenblatt Securities initiated coverage on MongoDB in a report on Tuesday, December 17th. They set a “buy” rating and a $350.00 price target on the stock. Finally, Mizuho upped their price objective on MongoDB from $275.00 to $320.00 and gave the company a “neutral” rating in a research note on Tuesday, December 10th. Two analysts have rated the stock with a sell rating, four have assigned a hold rating, twenty-three have issued a buy rating and two have assigned a strong buy rating to the company. According to MarketBeat.com, the stock currently has an average rating of “Moderate Buy” and a consensus price target of $361.00.
Read Our Latest Research Report on MongoDB
MongoDB Company Profile
MongoDB, Inc, together with its subsidiaries, provides general purpose database platform worldwide. The company provides MongoDB Atlas, a hosted multi-cloud database-as-a-service solution; MongoDB Enterprise Advanced, a commercial database server for enterprise customers to run in the cloud, on-premises, or in a hybrid environment; and Community Server, a free-to-download version of its database, which includes the functionality that developers need to get started with MongoDB.
Featured Articles
Before you consider MongoDB, you’ll want to hear this.
MarketBeat keeps track of Wall Street’s top-rated and best performing research analysts and the stocks they recommend to their clients on a daily basis. MarketBeat has identified the five stocks that top analysts are quietly whispering to their clients to buy now before the broader market catches on… and MongoDB wasn’t on the list.
While MongoDB currently has a “Moderate Buy” rating among analysts, top-rated analysts believe these five stocks are better buys.
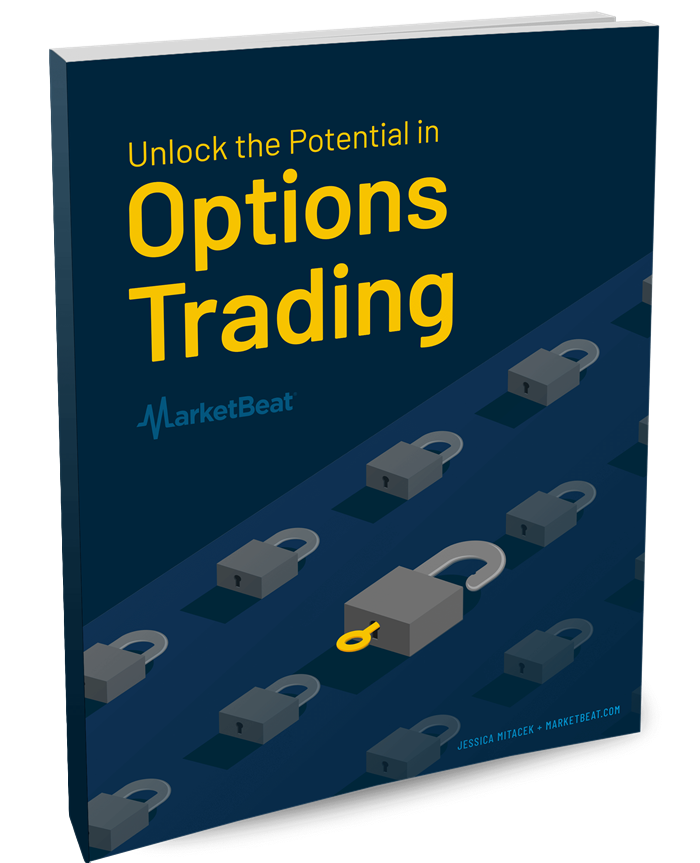
Options trading isn’t just for the Wall Street elite; it’s an accessible strategy for anyone armed with the proper knowledge. Think of options as a strategic toolkit, with each tool designed for a specific financial task. Get this report to learn how options trading can help you use the market’s volatility to your advantage.
Article originally posted on mongodb google news. Visit mongodb google news
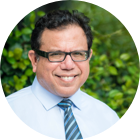
MMS • Sergio De Simone
Article originally posted on InfoQ. Visit InfoQ
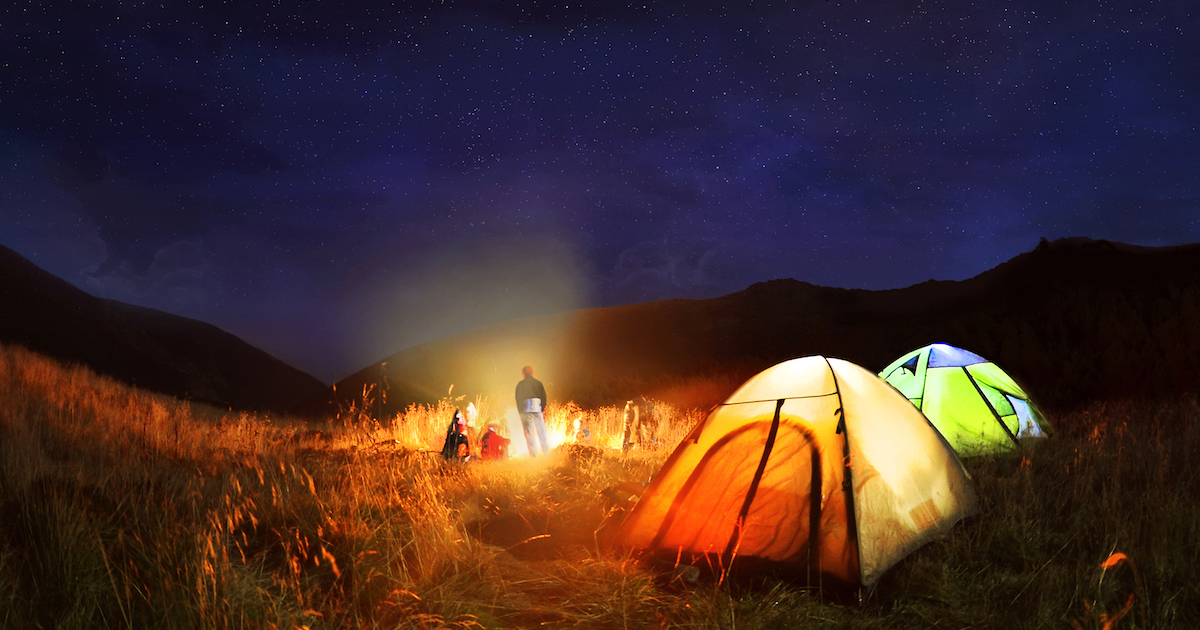
Apple’s new Advanced Commerce API provides iOS developers more flexibility to dynamically manage large content catalogs, creator experiences, and subscriptions with optional add-ons, such as premium features. For a developer to be allowed to use the new API, they must request access to Apple.
The Advanced Commerce API enables apps adopting it to host and manage their catalogs of In-App Purchases and SKUs outside of the App Store. However, they use the App Store commerce system to handle end-to-end payment processing, global distribution, tax support, and customer service. In other words, while the StoreKit In-App Purchase APIs require apps to configure all of their product identifiers in advance, the new Advanced Commerce API makes it possible to manage the catalog of products an app offers dynamically, at runtime.
Advanced Commerce API features are available through requests you make using StoreKit in your app and endpoint requests from your server. To authorize these requests, you generate JSON Web Tokens (JWTs).
To simplify generating JWTs to authorize calls and adopting the App Store Server API, Apple has also open-sourced the App Store Server Library. The library provides an API client to encode requests to and decode responses from the App Store Server and to verify that Apple signed the transaction data coming in responses. It is available in four languages, including Swift, Java, Python, and Node.
Examples of apps that could be eligible to use the new API are apps that provide a large catalog of one-time purchases, such as courses or games; apps that provide a platform for third-party creators to offer and sell their creations; and apps that want to sell add-ons to an existing subscription service, so users can buy a subscription and later add or remove any additional features they wish.
Last year, Apple changed the App Store rules to have all apps use the App Store for their In-App Purchases. This change affected apps like Patreon, Instagram, and others, for which Apple started applying their 30% App Store fee last November. The new Advanced Commerce API will not change this status of things but aims to provide more value to developers to justify that fee.
Build Resilient Systems with Insights on AI, Multi-Cloud, Leadership & Security at QCon London 2025
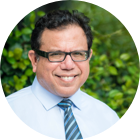
MMS • Artenisa Chatziou
Article originally posted on InfoQ. Visit InfoQ
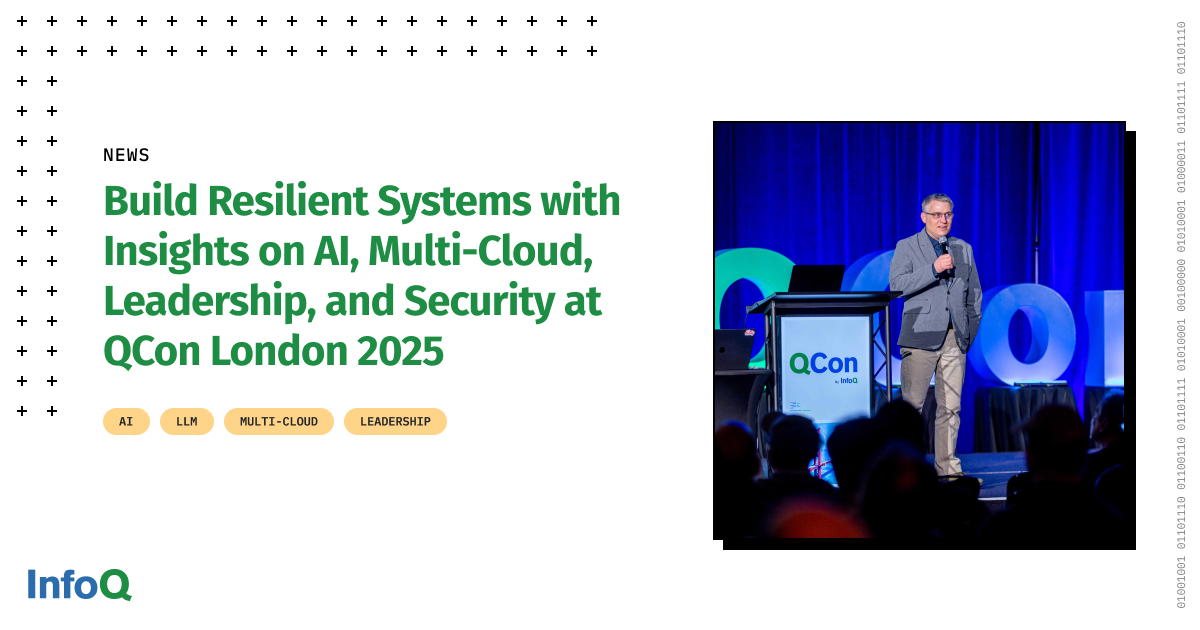
QCon London returns on April 7-11, 2025, bringing together 125+ senior software practitioners to share actionable insights across 15 practitioner-curated tracks focusing on emerging trends in software development.
Designed for senior software engineers, architects, and team leads, QCon will help you adopt the right technologies and practices. Discover how senior software practitioners are tackling modern challenges, from scaling architectures and enhancing developer productivity to securing supply chains and navigating AI integration.
Top Tracks at QCon London 2025: Architecture, Staff+, AI, and More
Architectures You’ve Always Wondered About
This track explores real-world examples of innovative companies pushing the limits with modern software systems. Speakers will share their stories of scaling their systems to handle large amounts of traffic, data, and complexity. QCon London 2025 Track Host: Ian Thomas, software engineer @Meta, with a background in Computer Science with a detour into UX and design, and QCon London 2024 PC Chair.
The Path to Senior Engineering Leadership
What does it take to reach senior leadership in tech? Gain insights into the journey toward senior engineering leadership, including strategies for managing teams, driving technical decisions, and fostering growth. QCon London 2025 Track Host: Leandro Guimarães, senior engineering manager @ClassPass, former chair of QCon in Brazil, working in software development for over 24 years.
AI and ML for Software Engineers: Foundational Insights
Deep dive into the foundational concepts of AI and ML and discover practical ways to integrate these technologies into software development. QCon London 2025 Track Host: Hien Luu, senior engineering manager @Zoox, leading the Machine Learning Platform team, & author of MLOps with Ray.
Engineering Productivity and Developer Experience
Learn how to enhance engineering productivity and create developer experiences that empower teams to deliver high-quality software efficiently. QCon London 2025 Track Host: Blanca Rojo, distinguished engineer and executive director at UBS, specializing in cloud engineering, and AI champion.
Modern Data Architectures
In this track, software leaders will share the actionable insights, cutting-edge strategies, and real-world case studies you need to navigate this evolving landscape. QCon London 2025 Track Host: Fabiane Nardon, data expert, Java Champion by Sun Microsystems & data platform director @totvs, Brazil’s largest tech company.
Connecting Systems: APIs, Protocols, Observability
Explore best practices for designing APIs, implementing protocols, and achieving observability in distributed systems. QCon London 2025 Track Host: Daniel Bryant, platform engineer, co-author of “Mastering API Architecture”, Java Champion, and InfoQ news manager.
Health Tech
Discover how technology is transforming healthcare with insights on optimizing patient outcomes, resilience, and innovations at the intersection of health and technology. QCon London 2025 Track Host: Dr. Jack Kreindler, founder and CEO @Wellfounded Health, chair of Leaders in Health, Extreme Environments Physiologist, & Medical Technology Entrepreneur, Researching the Limits of Human Resilience & Technology for Optimizing Health & Patient Outcomes.
Resilient Engineering Practices for Security against Modern Threats
Learn how to secure your software supply chain by embedding proactive strategies into your workflows to tackle modern vulnerabilities.QCon London 2025 Track Host: Sonya Moisset, staff security advocate @Snyk, a mentor for women in tech, a cybersecurity writer for FreeCodeCamp publications, 4x GitHub Stars, and an active member of the tech community in the UK.
Multi-Cloud and Hybrid Cloud Architectures
In this track, speakers will share real-world examples of multi-cloud adoption, focusing on integration, security, automation, orchestration, and analytics to optimize performance and cost-efficiency. QCon London 2025 Track Host: Dio Rettori, multi-cloud strategy and product @JPMorgan Chase & Co, ambassador for Cloud Native Computing Foundation, advisor for InfinyOn Inc., previously @Solo.io, @Red Hat, and @ Pivotal Software.
Emerging Trends in the Frontend and Mobile
This track will explore cutting-edge trends and best practices for building high-performance, responsive, and engaging frontend and mobile applications that meet the evolving needs of modern users. QCon London 2025 Track Host: Sareh Heidari, senior software engineer at F1 Arcade®, the intersection of Formula 1® racing simulations in a hospitality setting.
Presented by InfoQ, QCon London 2025 shares talks you can trust, free from vendor bias and marketing agendas. Attendees gain actionable insights from real-world experiences shared by practitioners adopting emerging trends and practices.
For more details, visit QCon London 2025. Early bird pricing ends on February 11, 2025.