Month: November 2022
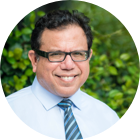
MMS • Sergio De Simone
Article originally posted on InfoQ. Visit InfoQ
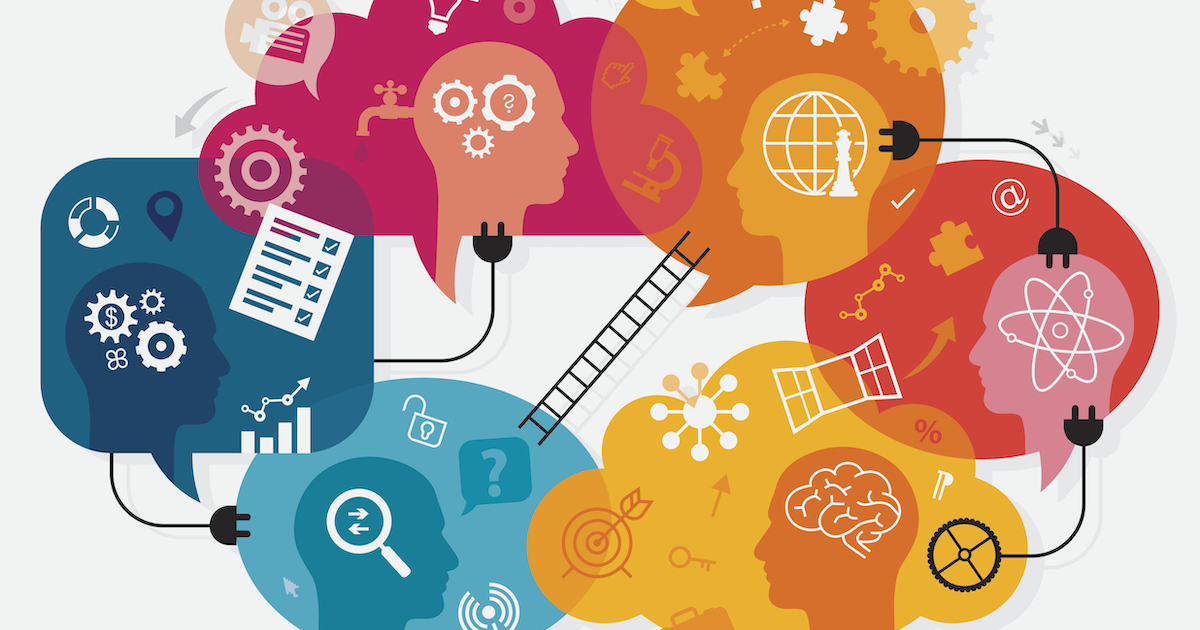
The Windows Subsystem for Linux has reached GA status in the Microsoft Store, adding support for Windows 10 in addition to Windows 11. Among the most notable features in WSL 1.0.0 are opt-in systemd support and the possibility of running Linux GUI applications on Windows 10.
WSL was originally included in Windows OSes as an optional component, known as “in-Windows” WSL, that users could activate to install and run a Linux distribution. WSL in the Store makes this process more straightforward and enables automatic updates and servicing without requiring a full OS update.
Adding support for systemd means WSL can be more similar to popular Linux distributions like Ubuntu and Debian, which use systemd by default. This will also enable running applications that require systemd under WSL, including microk8s and systemctl.
Supporting systemd required changes to the WSL architecture. As systemd requires PID 1, the WSL init process started within the Linux distribution becomes a child process of the systemd. […] Additional modifications had to be made to ensure a clean shutdown (as that shutdown is controlled by systemd now).
Due to these changes, Microsoft preferred making systemd an opt-in feature to avoid changing the behavior of existing installations, but will investigate making it the default option. To enable systemd, you need to edit the wsl.conf
file and add the systemd=true
option under the [boot]
section. Finally, you need to restart your WSL instance.
The possibility of running Linux GUI apps, already available on Windows 11, is now also available on Windows 10. This enables running Linux GUI apps from the Windows Start menu, as well as pinning them to the Windows task bar. Cutting and pasting across Linux and Windows apps is seamlessly supported, too.
With this announcement, Microsoft is not entirely sunsetting “in-Windows” WSL, which will indeed still receive critical bug fixes, but new features will only appear in the Store version.
The Store version of WSL is now the default that is installed by running the command wsl --install
on fully patched Windows 10 or 11 systems. Analogously, you can update an existing installation by running wsl --update
on fully patched systems.
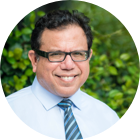
MMS • Steef-Jan Wiggers
Article originally posted on InfoQ. Visit InfoQ
Microsoft recently announced the public preview of Azure SQL Database External REST Endpoint Integration – an ability to call a REST endpoint natively from Azure SQL Database.
The public preview of Azure SQL Database External REST Endpoint Integration is a follow-up from this year’s earlier private preview. With the public preview, the new system stored procedure sp_invoke_external_rest_endpoint is available to everyone.
The integration massively reduces difficulties in integrating Azure services with Azure SQL Database. With only one line of code, according to the company, the Azure SQL database can integrate with a wealth of services such as Azure Functions, Cognitive Services, Event Hubs, and Azure containers.
The full syntax, according to the documentation, is as follows:
EXEC @returnValue = sp_invoke_external_rest_endpoint
@url
[ , [ @payload = ] N'json_payload' ]
[ , [ @headers = ] N'http_headers_as_json_array' ]
[ , [ @method = ] 'GET' | 'POST' | 'PUT' | 'PATCH' | 'DELETE' ]
[ , [ @timeout = ] seconds ]
[ , [ @credential = ] credential ]
[ , @response OUTPUT ]
The stored procedure accepts arguments like url (HTTPS REST endpoint to be called), payload (Unicode string in a JSON format), headers(as flat JSON), method(HTTP method calling the url GET, POST, PUT, PATCH, DELETE), timeout(time in seconds allowed for the HTTPS call to run), credential(inject authentication info), and response(response received from the called endpoint passed into a variable).
Developers can call a REST endpoint, like an Azure Function, for example:
declare @ret as int, @response as nvarchar(max);
exec @ret = sp_invoke_external_rest_endpoint
@method = 'GET',
@url = 'https://my-function.azurewebsites.net/api/say?message=hello world',
@response = @response output;
select @ret as ReturnCode, @response as Response;
Christopher Webb, a member of the Power BI CAT team at Microsoft, mentions another example on his personal blog:
And guess what, the Power BI REST API is one of the APIs you can call! This means, for example, you can run DAX queries via the Execute Queries endpoint (bearing in mind all the limitations) from TSQL.
Glenn F. Henriksen, a CTO at Justify, tweeted:
Finally, we can move all our app logic into SQL-stored procedures and triggers! This was the final missing piece!
And in addition, Jeff Taylor, a senior data engineer, tweeted:
This looks incredible! This should make obtaining certain datasets faster and simpler and in stored procedures no less! A great addition to an already-known language!
Lastly, a GitHub repository contains more examples, with additional ones coming soon.
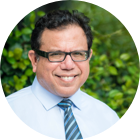
MMS • Ming Wen
Article originally posted on InfoQ. Visit InfoQ
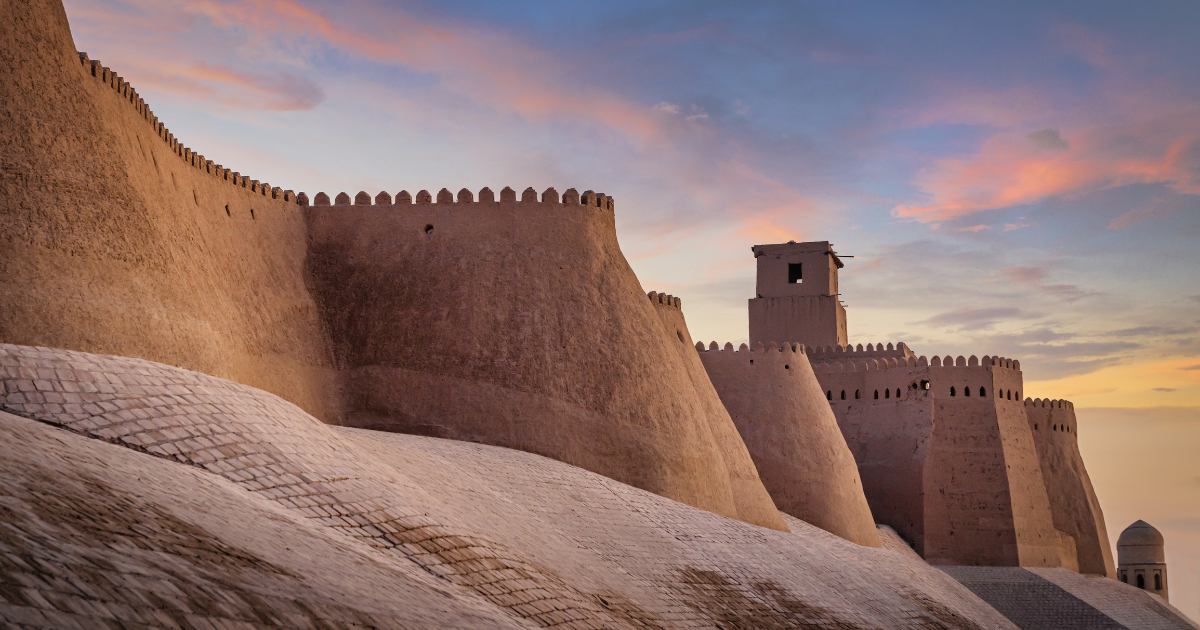
Key Takeaways
- Only a few companies have an API security policy that includes dedicated API testing and protection
- Defense-in-Depth is a multi-layered defense that provides different types of protection: boundary defense, observability, and authentication
- Authentication is the most important and can be achieved through password complexity, periodic modification, and two-factor authentication
- To improve efficiency in security management, one can change from “finding bad people” to “identifying good people”, by utilizing the allowlisting approach
- Zero Trust is the next level for API security, though it is not a silver bullet
Stats of API Security
According to Salt Security’s 2022 API Security Survey:
- 95% of the more than 250 survey respondents said they’ve experienced an API security incident in the past 12 months
- only 11% of respondents have an API security strategy that includes dedicated API testing and protection and 34% lack any security strategy at all for APIs
- shift-left tactics are falling short, with more than 50% of respondents saying developers, DevOps, or DevSecOps teams are responsible for API security while 85% acknowledge their existing tools are not very effective in stopping API attacks
- when asked about their biggest concern about their company’s API program, 40% of respondents highlighted gaps in security as their top worry
- 94% of API exploits are happening against authenticated APIs, according to Salt customer data
- stopping attacks tops the list of most valuable attributes of an API security platform
- 40% of respondents are grappling with APIs that change at least every week, with 9% saying their APIs change daily
From the survey, we could see that nearly all companies have experienced API security incidents. However, only 11% of companies have an API security policy that includes dedicated API testing and protection.
So, what kinds of protection should a company build to defend against these attacks? Security problems are not unique to the Internet age. Over the last thousands of years of human history, various countries have been exploring and practicing multiple defense strategies and fortifications. These experiences and ideas are also applicable to the field of network security.
For example, WAF (Web Application Firewall) is analogous to castle walls, identity authentication is equal to the commanders’ ID card, and honeypots are used to lure attackers away from high-profile targets. Among these war strategies, one of the most effective methods is Defense-in-Depth (DiD).
Defense-in-Depth
Defense-in-Depth is a multi-layered defense strategy that provides different types of protection at each line of defense.
DiD can be roughly divided into three different key areas of defense:
Defending the Borders
Boundary defense is the most basic and common type of defense. Almost all companies invest in boundary defenses, such as WAFs, which use regular expressions and IP denylists to defend against known attack methods and security vulnerabilities.
Most of the so-called attacks are initiated by “script kiddies”, relatively unskilled individuals who do not have strong technical backgrounds or hacker mindsets. They can only attack targets in batches by using pre-written scripts. In this scenario, boundary defense can well resist these indiscriminate attacks.
As the protection of the outermost layer, it has always been one of the necessary defense methods, though, from a technical point of view, WAF does not necessarily require strong technical skills.
In addition to WAFs, some defense tools are explicitly designed for bots. Using bots to carry out “credential stuffing” attacks is a standard method to attempt to steal high-value digital assets. The strategy is to buy login information, such as leaked emails/passwords, and then attempt to log in to other websites in batches. The most efficient defense method to combat credential stuffing is to identify the bot and intercept all requests made by the bot.
The basic strategy behind bot interception is a defense tool deployed between the server and client acting as an intermediary. When the server returns a response page, the tool inserts JavaScript into that response. It requires the client (browser or bot) to execute the JavaScript to calculate the token and put it into the cookie. After the tool receives the token, it will judge whether the other party is malicious based on the token value and take corresponding actions. It can also encrypt specified page contents (such as the URL on the page or the content of the form).
Although, in theory, this kind of encryption is not difficult for a determined cryptography enthusiast (because a perfect key establishment mechanism is not implemented between the client and the server), it is enough to stop hackers with penetration tools.
In an enterprise server-side architecture, the WAF, SSL gateway, traffic gateway, and API gateway are all connected in series, with upstream and downstream relationships. Each can be deployed separately or combined into one component. Take Apache APISIX as an example: it provides some standard features for boundary defense: IP allowlists and denylists, a WAF rules engine, fault injection, and rate limiting. When used in combination, it can block almost all indiscriminate attacks.
Detecting Intruders
In network security, the scariest event is not actually finding a security incident, but never finding one. The latter means that you may have been hacked many times without realizing it.
Being able to observe security risks is critical in combating targeted attacks. After a hacker has breached the outermost layer of defenses, we need observability mechanisms to identify which traffic is likely the malicious attack traffic.
Common means of implementing security observability are honeypots, IDS (Intrusion Detection System), NTA (Network Traffic Analysis), NDR (Network Detection and Response), APT (Advanced Persistent Threat), and threat intelligence. Among them, honeypots are one of the oldest methods. By imitating some high-value targets to set traps for malicious attackers, they can analyze attack behaviors and even help locate attackers.
On the other hand, APT detection and some machine learning methods are not intuitive to evaluate. Fortunately, for most enterprise users, simple log collection and analysis, behavior tracking, and digital evidence are enough for the timely detection of abnormal behaviors.
Machine learning is an advanced but imperfect technology with some problems like false or missed reports. However, most enterprises don’t currently need such solutions. They care more about logs collecting and tracking because they need to collect digital evidence. Digital evidence not only serves as evidence of the crime but also helps companies better understand the weaknesses of their internal systems.
For example, when a hacker attacks a system and steals data, a behavior tracking solution would track a number of things including: when the hacker attacked, which servers were accessed, which data has been exfiltrated, and how the hacker behaved within the environment. These access logs can be found and gathered as digital evidence.
At the observability level, Apache APISIX can do more things than tracing, logging, and metrics. It can use plugins to simulate high-value targets to confuse the attackers, utilize the traffic mirroring functionality to send partial requests to the blocking and non-blocking security detection tools for analysis, and make quick security decisions to stop the attack.
Preventing Lateral Movement
When the attacker breaks through the outer defense line and enters the intranet, it is time for authentication and access control to play a role. This defense method is similar to showing your ID cards to purchase alcohol and your passport at the airport checkpoint. Without the corresponding identity and authority, it is impossible to enter the corresponding system.
This line of defense reflects a company’s basic security skills and technical strength. Many companies’ internal systems do not have well-established authentication and authorization architecture, and implementing those solutions requires long-term and continuous investment.
For example, numerous systems have been used for years in financial institutions, such as banks, securities, and insurance companies. The significant number of employees and legacy systems requires a high cost in both time and money to unify the identity authentication by implementing SSO (Single Sign-On).
This line of defense essentially puts obstacles everywhere for the attackers, making it impossible for them to move laterally within the network.
It is worth mentioning that there are not many companies that do well in seemingly simple password management. Password complexity, mandatory periodic modification, and mandatory two-factor authentication (SMS or dynamic password) for critical systems are easier said than done. Only companies who understand network security well can implement them well. There are a number of reasons why this is true but the key reasons include:
- Many companies pay insufficient attention to password management because they believe that they are only effective within the internal network, which is relatively safe.
- It will burden the IT department if companies conduct regular secret changes because there are many employees whose secrets are different, which is hard to manage.
- Unless the policy is required at the company level, implementing consistent and effective secret management is challenging.
This third line of defense is the most important one. Boundary protection and security threat observation, to a large extent, exist to help the third line of defense. If an application system itself is not safe, no matter how strong the first two lines of defense are, there will be attacks that slip through the net.
This line of defense is where an API Gateway comes in strong as it provides a number of key authentication features:
- Various authentication methods, such as JWT and key auth
- Integrating with multiple authentication systems such as OKTA and Auth 2.0
- TLS and mTLS encryption of traffic
- Automatic rotation of keys
In summary, these three defense layers require inter-department cooperation to block security attacks effectively. These three defense layers are typically controlled by different departments: WAF by the Security Operations team, observability by the R&D teams, and authentication by the IT department. Each area will use different tools, such as WAFs, OpenTelementry, and Keycloak, to implement their respective solution. This split of responsibility across many teams is why effective blocking requires inter-department cooperation.
So, is there a more efficient way, the so-called “silver bullet,” to solve all security problems?
From “Denylisting” to “Allowlisting”
The network security community has been thinking about this issue for a long time. The strategy for most security defense and detection methods is to look for a small number of security threats within a massive quantity of data, similar to finding a needle in a haystack. There will inevitably be both false positives and false negatives.
What if we change our thinking and turn “finding bad people” into “identifying good people”? Will it help us shine a new light on the problems?
Over ten years ago, some antivirus software companies began to make such an attempt. Their logic was to add the commonly used software to an allowlist, identifying the executable programs one by one, and then the rest would be viruses. In this case, any new virus could not escape the detection of antivirus software. This plan was quite ideal. Nevertheless, it took four or five years for the software companies to implement it, and it was not entirely allowlisting but hierarchical management.
The allowlisting approach is equally applicable in API security. For example, a company provides a payment API interface, which requires a token to access. If there is no token or the token cannot properly be accessed, then it must be a malicious request and needs to be rejected directly.
Ideally, all APIs should have similar authentication and access controls, and only authenticated access is allowed. Although this cannot defend against internal threats, social engineering, and 0-day attacks, it will significantly increase the threshold of attacks, making the cost high and indiscriminate attacks impossible.
For attackers, when the ROI (Return on Investment) becomes unreasonable, they will immediately turn around and look for other easy-to-break targets.
Based on the ideas of defense in depth and allowlisting, a “zero trust” security framework has gradually evolved, hoping to solve network security problems once and for all.
Is Zero Trust the Silver Bullet for API Security?
What is Zero Trust? Put simply: there is no trusted client in the system, so you will need to verify your identity everywhere.
Whether it is an external request or internal access, a bastion or a springboard, a mobile phone or a PC, an ordinary employee or a CEO, none can be trusted. Access to systems and APIs is allowed only after authentication.
It seems that there are no loopholes except that it is troublesome to verify the identity everywhere. So is zero trust the silver bullet for cybersecurity?
Simply put: no, zero trust is not a silver bullet.
Zero trust is a comprehensive security framework and strategy. It requires adding strict and unified identity authentication systems to all terminals, BYODs (Bring Your Own Devices), servers, APIs, microservices, data storage, and internal services. Think of zero trust as a safety air cushion. We can understand the zero trust model by using the Wooden Bucket Theory, which states that a wooden bucket can only hold as much water as its shortest plank. Translating this to security, our defenses are only as good as our weakest spot. This implies that the hacker will always attack the weakest part. If the bucket leaks, it doesn’t matter how much water it could hold. However, we also can’t say, “90% coverage of a zero trust model is not substantially better than 0% coverage” because the measures can increase the cost of hackers’ attacks. If we can realize 90% coverage of a zero trust model, then almost 80%-90% of vicious attacks could be intercepted.
The implementation of zero trust is complicated. Imagine adding identification equipment in all transportation hubs, such as airports and high-speed railways. It is incredibly costly in terms of time and money.
In a large enterprise, there will be hundreds of systems, tens of thousands of APIs and microservices, and hundreds of thousands of clients. It takes great effort and cost to build a complete zero-trust system. Therefore, zero trust is mainly implemented in government, military, and large enterprises. For most enterprises, it is wiser to learn from the idea of zero trust and build a security defense system with a higher ROI.
There are two core components of Zero Trust:
- Identity and Access Management
- API Gateway with integrated security
The focus on these two components can make the implementation of zero trust more realistic.
Note here that zero trust is not flawless. Zero-trust solutions cannot fully defend against zero-day or social engineering attacks, although they can greatly reduce the blast radius of those attacks.
Conclusion
However, security is a never-ending game of cat and mouse because attackers are always hoping to find means to acquire high-value digital assets or to achieve their destructive outcomes. Defense alone cannot avoid the attacker’s guns and arrows.
Consequently, it is also necessary to improve the security awareness of developers and reduce exposure to vulnerable surfaces as much as possible.
Developers stand in the middle of code and application. On the left is the “code”, while on the right is the “application”. Hence we need to pay more attention to the left side as the code is the root of all the problems. We should adopt the “shift left” method, which means a DevOps team needs to guarantee application security at the earliest stages in the development lifecycle. The vulnerable exposure can be significantly reduced if the developers improve their security awareness.
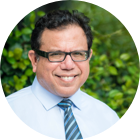
MMS • Kevlin Henney
Article originally posted on InfoQ. Visit InfoQ
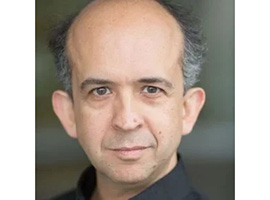
Transcript
Henney: My name is Kevlin Henney. I’m here to talk to you about six impossible things. That idea of six impossible things comes from one of the Alice books, “Alice in Wonderland,” is the first book. “Through the Looking-Glass” is the second book by Lewis Carroll. The White Queen is asking Alice to believe in something that Alice is like, “I can’t believe that. It’s impossible.” The Queen just dismisses that, says, sometimes I’ve believed as many as six impossible things before breakfast. I want to emphasize this idea of impossibility, because we are often taught that nothing is impossible, just takes more time, more effort. Anything is possible. At the same time, we also sometimes brand something as impossible, when actually it’s just ridiculously hard and not feasible. That’s not the same thing. Somebody says, that’s impossible, it would take 1000 years. Then they’ve just told you the circumstances for its possibility. There is a distinction here. I’m actually interested in the things that are challenges that we can’t meet directly, we may work around them. We may use our ingenuity. We may pull back and innovate in other ways. There are certain limits, limits defined by mathematics and physics that every now and then hit in software development.
Representations Can Be Infinite
I’m going to count these down, let’s start at six. Representations can be infinite. This is impossible. You’ll say, how? Surely I can represent infinity, in my floating-point numbers, I can do that, plus or minus infinity. Yes, you’re representing, it’s a stand-in for infinity. It’s not actually infinity. There are no infinities in the physical universe. Infinity is a mathematical concept, not a physical one. There’s a distinction here. We are using a placeholder to say, this thing is infinity. It’s like infinity, it doesn’t behave like infinity. It is not itself infinity, it is much more bounded. There are also other concepts that lie beyond finiteness. For example, not a number, is not a finite numeric concept. This is perhaps one of the most familiar to people in their day to day use of applications in the web, we get thrown back with NaN, it’s not a number. Sometimes it’s as frustrating as not being able to complete a flight booking. Other times it can be a little more dramatic. This happened in 2020. Driverless race car drives into a wall. What had happened is during the initialization lap, something had happened which caused the steering control signal to go to NaN. Subsequently, the steering locked to the maximum value to the right. This was a bit of a state management issue. It was interesting when I tweeted this originally, somebody had pointed out to me, are you saying that these applications that they use JavaScript? I was like, no, that’s not at all the same. NaN is used outside the realm of JavaScript. It comes from IEEE-754, and IEEE-854 standards. This is the 1980s. We see NaN errors all over the place. JavaScript, thanks to its long standing idea that there is only one numeric type, and it’s a very atypical numeric type, floating-point type, is its only way of expressing numbers historically. It’s a limitation to do with that, and that therefore, is very public facing.
When it comes down to floating-point representations, there was a piece in “97 Things Every Programmer Should Know,” the piece by Chuck Allison, called floating-point numbers aren’t real. He makes this observation. This is important because real numbers have infinite precision, and are therefore continuous and nonlossy. In fact, real numbers are uncountably infinite. Between zero and one there are an infinite number of numbers, and we are not able to represent them all. What we are ever going to do is approximation. Floating-point numbers are about approximation. They have limited precision so they are finite, and they resemble badly behaved integers. These are badly behaved integers. They don’t behave as you would expect. It’s a little more than that, because it is not simply that they are badly behaved integers. Integers are not always that well behaved either.
One of the things that we learn is that most languages present us with integers that are unlike true integers. True integers are unbounded, they are countably infinite. A 32-bit integer is not countably infinite, it is countably finite. We see it thrust into our faces every now and then. Here’s one from a few years ago, Visual Studio telling me that my license will expire in about 2 billion days. That number, if you come from outside software development that looks just like an arbitrarily large number. If you’re a software developer, you look at that and you go, yes, that’s 2^31 minus 1. That is the maximum value of a signed 32-bit int. That’s a little bit suspicious. We can also see there’s something else going on here as well. Somehow, something got set to int max value. Also, notice your license has gone stale and must be updated. Surely that’s not right. My license is good for a few 100,000 years. I’m good here. Clearly, the number that is being used for comparison is different. This is likely to be something that manifests in the presentation layer rather than in the core. It does confront us with the boundedness and the limitations. As it were in errors, we tend to find we confront the finiteness of our machines.
We might be tempted to try and prove things. There are limits to proofs, and there are limits to the practicality of proofs. There’s also something else when we talk about the limits of proof. This was inadvertently highlighted in a piece by Jon Bentley, 1983. During the ’80s, Jon Bentley had a column in Communications of the ACM, Programming Pearls. These were collected in a number of books, Programming Pearls, more Programming Pearls. Then there was a second edition of Programming Pearls in the ’90s. This one, what you find in the first edition, writing correct programs is interesting, because he presents a binary search algorithm. He presents a binary search algorithm and proves that it is correct. Here’s the pseudocode that he presents for it. Of note there is a three-way partitions. In that case, there’s a three-way partition in terms of the comparison, then he annotates this more completely. All of those things in curly brackets that say MustBe, basically MustBe is an assertion because it’s an invariant, a thing that must be true at that point. He uses this to demonstrate the correctness of this algorithm. One of the major benefits of program verification is that it gives programmers a language in which they can express that understanding. We should always be on the lookout for opportunities to improve our vocabulary, our ways of expressing and understanding certain problems, more different points of view can be very helpful.
He also observes, this is not the be all and end all. These techniques are only a small part of writing correct programs. Keeping the code simple is usually the way to correct this. He also highlights another aspect where our emphasis is drawn, where our attention is drawn. Several professional programmers familiar with these techniques have related to me an experience that is too common in my own program, when they construct a program, the hard parts work first time, while the bugs are in the easy parts. You’ve probably had this experience. You know it. You’re so focused on the bit that you know is hard, you put so much attention. You manage the detail, and you get it right. You completely overlook something else that’s been invalidated or something else that should have been changed in conjunction with a more complex thing. This demonstrates to us that we have a blind spot, but that blind spot is in fact deeper than what he’s described.
Here’s code from the Java libraries. This is in the binary search method that is found in the collections utility classes developed by Josh Bloch. Josh Bloch was a student of Jon Bentley, and Josh is using the three-way partition in there. Josh Bloch is using the approach that Bentley proved was correct. This works just fine until you use a very large array. This is the point, very large arrays did not really exist in the 1980s and the 1990s. This is why this bug was only found and reported in 2006. There was a problem here. In any binary search, no matter how you’re doing it, you’re going to end up with trying to establish the midpoint. Binary search is about searching between midpoints and halving the distance appropriately. The intuitive way that works with integers is that you take the low point you’ve been searching, and the high point, and you find the midpoint, you add the two together and you divide by two. It’s the arithmetic mean. That’s fine. There’s only one problem. This is Java. Java doesn’t have integers, it has ints. Ints are truncated, they are not countably infinite. They are countably countable. They are countably finite.
If you have a large value that is low, and a high value that is low, when you add the two together, they won’t make a very large number, they’ll make a very negative number. That’s the point. You simply didn’t have arrays that were going to be this size. You did not have arrays that were going to have 2 gigs worth of entries, until you did. What we end up with is this assumption. This assumption was that ints behave like integers, and they don’t. The one thing we know is that ints are not integers, they actually follow a different form of arithmetic. Majority of the operations most of the time behave like integers, but they’re not integers. We fooled ourselves. It’s so easy to follow that habit. The fix is relatively simple, what you do is you find the distance between the low and the high. You halve that, and then you add that to the low point. We can see in the original proof that the assumption is here. He is assuming that he is using integers, but he’s not. He’s using integer division on integers, except that that’s not what’s actually going on.
We find other confrontations with the boundedness of numbers, and again, back to infinity. This is the USS Yorktown. It’s a U.S. Navy cruiser, that’s since been decommissioned. In 1998, it basically was dead in the water for about 48 hours. Source of the problem? They made a change from a Unix to Windows installation. This was originally published under the issue of problems with Windows. Actually, it was not a problem with Windows specifically. The source of the problem on the Yorktown was the data contained a zero where it shouldn’t have done. When the software attempted to divide by zero, which is a big no, remember, there are no infinities. A buffer overrun occurred, yes, big time, crashing the entire network and causing the ship to lose control of its propulsion system. Divide by zero is something that is not going to crash Windows. Most likely what this is, given that this was networking software, this is probably the driver and it runs in kernel mode. That is what caused the problem. This was a custom driver and Windows do not have sufficient defenses, with the driver for dealing with this. As Shakespeare observed, this is the monstrosity in love, that the will is infinite and the execution is confined, the desire is boundless and the act a slave to limit. There are no infinite representations.
Every Question Has an Answer
Coming to number five, not every question has an answer. It turns out, we can ask more questions than we can get answers to. To demonstrate this, many years ago, back before Facebook was busy destroying democracy. I submitted a bug report to Facebook and I was told that my feedback would be used to improve Facebook. That did not apparently happen. Thanks for taking the time to make a report. How much time? That took me back quite away, 31st of December 1969. That seems familiar, that’s really close to another number. What we need to understand is that if you’re a real full stack developer, not a JavaScript developer who does frontend and talks to a database. If you’re a full stack developer, you know how to program in C. The full stack is really deep. Everything is ultimately built on C at that level. The time function in C, what does it measure? What is it responding? On most platforms that use time, or have time, the implementation is based on POSIX. The POSIX standard says the time function shall return the value of the time in seconds since epoch. When is epoch? This is really easy to find. It’s actually quite a popular class of errors. You can actually find that out. What is time when it is zero? It’s the 1st of January 1970. A stroke of good luck leads you into that. It’s fairly unlikely that Intel were distributing drivers on the 1st of January 1970, for Windows operating systems. This is a classic zero initialization fault. That is what I thought would go wrong, a zero initialization fault. Then, a time zone shift. I’m based in the UK, Facebook is American, therefore, that’s West and I was negative time-wise from where I am, so therefore I assumed a negative time adjustment. That would give you zero initialization, and then back into 31st of December.
Actually, there is another explanation that in more recent years I’ve come to consider as more plausible. Going back to the C Standard, the value of minus one is returned from time if the calendar time is not available. That might seem initially, how can time not be available? Surely, time is always available. That’s the shortfall here. No, it isn’t. Time is a service, it can fail just like anything else. When you involve time in your application, it’s not some global variable, it’s asynchronously updated that you can call from a static method anywhere. APIs that do that are slightly misleading, you’re actually coupling to an external dependency. Like anything that involves that, like accessing anything across the network, that’s subject to failure. It’s not common that you’ll get that failure. That’s not what we’re discussing here. We’re not discussing frequency, we’re discussing possibility. It is quite possible that minus one is returned. If minus one is returned, then that will be interpreted as one second before midnight, which will give you 1969.
Another area of interest, when we talk about this stuff is algorithms. Algorithms for people who’ve done computer science degrees, they’ve done algorithms to death. What is it? Because the word is widely misused these days in association, and widely misused and over-generalized as something intrinsically nefarious relates to machine learning. It’s nothing more complex, and it’s quite innocent. It’s a process or set of rules to be followed in calculations or other problem solving operations, especially by a computer. To make things a little more exciting, if you are sick to death of computer science and sorting algorithms, let’s have fun with esoteric algorithms. I’ve been writing about esoteric algorithms on my blog post on and off for a while. Sleep sort, drop sort, and this one’s permutation sort. Permutation sort, the complexity of this thing is grossly inefficient. It has factorial time complexity. It’s shocking. We can consider it as a systematic but unoptimized search through the permutations of the input values until it finds the one arrangement that is sorted. For 10 elements that’s potentially 3 million comparisons that it’s going to perform. This is hugely inefficient. It’s also great fun. It’s also a useful provocation. It’s not something you’d ever put into production code, except perhaps if you ask them, what are your performance requirements? We have no performance requirements. Use permutation sort. If you use permutation sort, that person will discover they do indeed have performance requirements, it’s just they didn’t know what they were. They didn’t know the boundary. Demonstrate where the boundary is.
Let’s do this in Groovy. I need to work out whether or not something is sorted. I’ve got a simple predicate function here. That figures out that’s correct. Now, here’s permutation sort. Permutation sort, I can just use the permutation generator. I use the permutation generator that will systematically return me an iterator, permutation generator is iterable. All I do is I just keep on going. If it’s sorted, then I return the permutation. Otherwise, keep on going. There’s a thought here that surely there can be nothing worse than permutation sort in terms of performance. Yes, don’t be so sure. I recently wrapped up on writing about bogosort. Bogosort is interesting, because bogosort is not systematic. Bogosort takes a slightly different approach. It just randomly shuffles, not systematically, it randomly shuffles and checks whether they’re all sorted. We might naively write it like this while it’s not sorted, then shuffle the values. That does give a free pass of the values that are already sorted. We’re going to do a shuffle first. Here’s where we definitely get terrible performance. Because this is interesting, we would just randomly shuffle it. Is it good? No. It’s like throwing a deck of cards up into the air, and does it land, is it sorted? No. Ok, throw it again. That gives you a sense of the possibility that this is not the most efficient way to do things.
We might still have some objections and concerns. Why don’t you, Kevlin, technically you are systematically generating it because you’re using pseudo random numbers rather than real random numbers. That’s a fair objection, but is not one sustained for very long. We can have access to true random numbers through the entropy of your hardware. The way this works on Java platform, you SecureRandom, and that uses a pseudo random number generator that is seeded off. There’s one time where we use a number that is truly random. We’re not doing this, people often use time, but time is not random. There are days it feels like that. Time is not random, but you’re guaranteed that SecureRandom will give you something that is seeded in something that is as truly random as can be got from the hardware.
We have a potential objection. Is this now an algorithm? Is it still an algorithm? Why would I question that? Because an algorithm is a specific procedure. I’ve been very nonspecific, randomness is not specific. We’ve actually said there is a completely nonspecific part here. There is actually a deeper and more subtle objection. Procedure which always terminates is called an algorithm. It’s not guaranteed to terminate. This could actually genuinely never ever terminate, which is interesting. There is no guarantee that the sorted sequence, it will appear. Of course, the probability is so close to zero, that it probably isn’t even actually representable in a floating-point number. Every time I’ve ever implemented bogosort, it has always terminated. Then, how would I wait for the end of the universe or beyond? Here’s a question, how do we test all this?
This is the origin of a very popular quote on Structured Programming, 50 years ago, this was published. Dijkstra has a popular quote, “Program testing can be used to show the presence of bugs but never to show their absence.” It does demonstrate that we have this challenge, how do I demonstrate that this will always be correct, or rather, will always terminate? Here’s a very simple example based test case. Every time I’ve ever run it, it certainly passes. I’ve taken a sequence of values, I tell you what the expected ones are, I bogosort it and I test the values I get are the expected ones. How do I guarantee? I want to be able to guarantee that this terminates, how can I do that? I can’t do it in the algorithm, maybe I can have the test enforce that, and basically say, fail the test if it runs forever. Do we do it like this? That’s not going to work either, because it turns out, it’s not just a case that there is no end of time. It’s not just the case that we don’t have that constant available to us in JUnit. It doesn’t actually mean anything. If we reach the end of time, then fail the test because the algorithm didn’t terminate. Realize that doesn’t really make a lot of sense. We just choose pragmatism, 1000 milliseconds. Yes, and that runs typically within a second. That’s fine.
What we’ve done there is we’ve been pragmatic. We’ve also demonstrated, another reason there are no infinities. It’s not just that physics doesn’t tolerate them. It’s that our patience won’t. It also teaches us how to solve things like the halting problem that is related to termination. We can fix that very easily by putting timeouts in it. Indeed, that is how we address these problems. Where we are not guaranteed to ever receive an answer, what we do is we exchange the non-determinism of not knowing whether we receive an answer for the non-determinism of we receive an answer or we get told it timed out. In other words, we offer certainty and time, but we trade it for certainty and result. That’s why timeouts exist.
Every Truth Can Be Established Where It Applies
Related is another impossibility. Every truth can be established where it applies. It’s related to Godel’s incompleteness theorems. Adrian Colyer did a really nice summary of this in a piece on fairness in machine learning. He highlights that, in common with a number of other things, the beginning of the 20th century there was this optimism that physics and mathematics will be completely known. Particularly in the light of the proof from Bertrand Russell and Alfred North Whitehead in Principia Mathematica. They have the goal of providing a solid foundation for mathematics. Twenty years later, Kurt Godel shattered the dream, showing for any consistent axiomatic system there will always be theorems that cannot be proven within that system. In other words, to prove those theorems, you have to step outside that. In other words, there are statements that are true that cannot be proven to be true within that context.
Summarized differently, Godel Escher Bach and perhaps a little more formally, “All consistent axiomatic formulations of number theory include undecidable propositions.” Many people might say, that’s great, Kevlin, fine, undecidable propositions, fine, axiomatic formulations and number theory, fine, but I’m dealing with code here. It turns out, code is equivalent to such a formulation. Everything that applies here applies to our code. There are undecidable propositions in code dependent on the context. Let me demonstrate that. Let’s try and determine how long a piece of string is. To be precise, let’s measure the length of a string in C. A standard function for this in C is strlen, back in the days before we had vowels and sufficiently long identifiers. What we’ve got is size_t, that is an unsigned integer type that is used for sizes. Char *, this is a pointer to the beginning of the sequence of characters. A string in C is delimited by a null. When we reach that null, we are done. We have measured the whole length of the string. We go ahead, we measure it. We set it up. We start with our result, it’s zero. While the nth position from s does not equal null character, increment the count, return the count, we are done. This implementation is actually very similar to the one that you’ll find in Kernighan and Ritchie’s C Programming Language, and retain most of the naming conventions from there.
What truths can we establish? What must be necessary for this to work? Here’s one, that pointer cannot be null. See, I can assert that. Here’s another one. For this to work in a way that is defined, there must exist an n such that there is a null, and that every point between the beginning and that position n, are valid, and are well defined in the context of C. As long as you’re not wandering across garbage memory and techy stuff that is undefined and inaccessible. You will notice that this is in gray, and also uses a bunch of symbols that are not native to C. That is because you cannot actually write this in C. It is not actually something you can assert on. It is not possible within the context of strlen, to prove that it can behave correctly. That’s a simple way of looking at it.
We can actually see this in practice, we can change the context. I can demonstrate by changing the context, stepping outside strlen, into a test case here. Here, I’m going to present it with a valid string, “Be excellent to each other.” I’m going to print out how long it is. I’m going to print the string and how long it is. Here we go. Be excellent to each other, 26 characters. I can demonstrate the correctness of that just by inspection and stepping outside, and by execution. Here, we’re not going to provide enough space. I’m only going to provide five characters in space. In other words, not enough space for the null. Actually, C will allow you to write it in this form. We’re not going to get null. When I run it, actually, there’s a reasonable chance it will show five because memory may be null, but the fact that it did work is not a guarantee that it should work. Equally well, you could be cast into the void, heading towards the galaxy M87. Similarly, there’s the idea that actually maybe the pointer itself is uninitialized. That’s just garbage, and there you are inside a black hole in M87. The point there is not defined. There is no way to demonstrate the correctness of this inside the context of strlen. I can do it by inspection, with static analysis outside that.
Why is this relevant? Because a lot of people work in managed languages, and they’re thinking, I don’t need to worry about that undefined behavior. There are cases you can demonstrate that kind of Godel problem in other cases, but actually just always remember that in any large system, there is always going to be an element that is touching the void in this respect. In this piece by Thomas Ronzon in “97 Things Every Java Programmer Should Know,” that Trisha Gee and I edited a couple years back. Thomas looks through and says, how can I crash my JVM? How can I crash my managed environment? In most cases, it’s by stepping outside those, stepping outside context. As he says, write some native code, all the syntax of C, all safety of C. This is bigger than just that. As Adrian observes, one premise of many models of fairness in machine learning is that you can measure, almost prove fairness in a machine learning model from within the system, from the properties, the model itself, or perhaps the data it’s trained on. However, we know we can’t. To show a machine learning model is fair, you have to step outside. You need information from outside the system. This is also important for many of the metrics that we fool ourselves with.
This is a wonderful demonstration of this. What you’re looking at is 99 secondhand phones that are running Google Maps. In 2020, in the first wave of lockdowns, Simon Weckert in Berlin, wandered around Berlin, and created virtual traffic jams. Notice, I’m using the word virtual traffic jam, just as is reported there on his website, virtual traffic jam, because that’s not a traffic jam, is it? Google Maps can’t tell you whether it’s a traffic jam. It’s not possible for Google Maps to do that. What it’s possible for it to do is to try and establish and correlate between traffic jams and the presence of phones. Therefore, to determine, there’s a lot of phones moving very slowly. They’re all in navigation mode. There’s a lot of phones moving slowly. Yes, therefore, that correlates with a traffic jam. Notice, I’m using the word correlation, it’s not causal. What they’re doing is they’re doing it there. A lot of the time it’s going to show you where the traffic jams are. Some of the time, it’s not.
This is a reminder that many people are measuring engagement. I know what engagement is, my dictionary knows what engagement is. It’s the state of being engaged. It’s emotional involvement or commitment. Is that what people are measuring? You’ve got whole marketing departments trying to nudge up an engagement value. They’re not measuring engagement, they need to stop using that word. We don’t know whether or not people are engaged. In fact, the correlations are actually far weaker. This is engagement. We don’t know how long people are spending actually looking at a screen. You can tell when somebody’s moved off to another screen, but you don’t know what they were doing before that unless you’re doing eyeball tracking. That’s a security issue. The point there is, I had this exactly yesterday. I was on a web page that I was on for a few seconds and I was going to go to another web page, but the doorbell went, I received a delivery. For 2 minutes, I was not on my screen. There is an engagement statistic somewhere that tells people that I was on that page for 2 minutes and 30 seconds. No, I wasn’t. I was on that page for 30 seconds. The minute I got back down, I moved on to another page.
People are measuring clicks and shares, and that’s what they need to understand. They’re not measuring engagement. Engagement should always appear in quotes. We must be careful not to confuse data with the abstractions we use to analyze them. We do not always know the answers to the questions in the context in which they are answered. We do not know that that is a traffic jam. We do not know that that is engagement. We do not know whether or not this is actually a valid call to a particular method in a particular context. We cannot necessarily prove this machine learning system is fair by using all of the assumptions that we put in to that machine learning system. We need to step outside that to demonstrate that, see the bigger picture.
The Future Is Knowable Before It Happens
When we look to the bigger picture, let’s also look to the future. Here is something that’s impossible, the future is knowable before it happens. That seems obvious. We trip over that. Often, we do so because we don’t appreciate the degree to which software development is actually an exercise in applied philosophy. It’s about epistemology. It is about the nature of knowledge, as Grace Hopper observed, “To me programming is more than an important practical art. It is also a gigantic undertaking in the foundations of knowledge.” In essence, a code base is a codification of knowledge. It’s codification of the knowledge that we have about the problem domain and the solution domain bound together and expressed in a way that is formally executable. It’s a formal specification of this knowledge. Therefore, knowledge is the heart of everything that we do. That means we have to have good models of what we know and what we don’t know. Likewise, what correlates versus what is causal? What we know versus what we don’t know. There are things that we know, we know, there are things that we know that we don’t know.
Then it starts getting a little more exciting. There are things we don’t know we don’t know, these are often assumptions. Those are the things that are hidden until you have them contradicted, “I had assumed that.” At that moment you discovered you had an assumption. You had the assumption all along, but if anybody had asked you before, what are your assumptions? That thing, whatever it was that was contradicted in future was not known to you. You did not know you had the assumption. Then, you cannot find out, you have no process for knowing. These things are unknowable until they happen. Unknowable unknowns are the challenge here. The halting problem, for example. I cannot know whether or not something is going to terminate until it doesn’t terminate, but I do have a process for that. I can’t tell that future. We have this observation that prediction is very difficult, especially about the future. It’s probable that Niels Bohr actually said this, but there are other contenders. I find that interesting and fascinating, because this is in the past, this quote was made. We don’t know who said that. If we don’t know this about the past, how on earth are we going to be able to know about the future?
We try and tackle the future in various ways. Here’s a roadmap. In fact, this is a template for a roadmap. You can go online, you can go to Google and find out PowerPoint templates for roadmaps. There’s many of them, and lots of them use road images. Invariably, they suffer one problem. I don’t have a problem with the roadmap. I have a problem with the fact that people don’t use it correctly. Let me show you a real roadmap. This is Bristol. This is where I live. There’s more than one road. That’s important, because that’s the point of a roadmap. If I only have one road, I don’t really need a roadmap. People are misusing the metaphor. The metaphor is much more exciting when you show the different branches and possibilities. Because if you don’t show those, it means apparently you know how to predict the future. I’m guessing that this particular PowerPoint template dates back to 2018. Of particular interest here is 2020. Somebody here had a roadmap that included 2020. How many of you people out there had roadmaps for 2020 back in 2018? How many of them say global pandemic changes everything about the nature of our work, and how we work, and how our clients interact with us, and how global markets work, and even the business of going to the shops? I’m pretty sure that nobody had that down. That was not knowable until it happened.
It doesn’t take a pandemic to highlight this. People are making this mistake all the time. I hear often when people are talking about the requirements. They’re talking about prioritizing by business value. That sounds great. Sounds very positive. We are trying to focus on the business. Does anyone have a problem? You can’t do it. I’m not saying you shouldn’t do, I’m saying you can’t do it. That’s a very different statement. You can’t do it because it’s impossible. You don’t know what the business value of something is, unless you travel into the future, and then travel back. It’s the traveling back that is hard. We’re traveling into the future all of the time. It’s the traveling back that’s the hard part. That’s why you can’t prioritize by business value. You are always using an estimate. It is prioritizing by estimated business value. You might say, “Kevlin, you’re just picking on words. It’s just semantics.” You’re right. It is just semantics. Semantics is meaning. If you don’t say what you mean, how are people supposed to know? If you’re in the business of confusing estimates with actuals, we need to have a serious conversation. Because you cannot prioritize by business value, you can prioritize by estimated business value. That’s a little more interesting. Ask me to change the way you work, as the great Grace Hopper said, “Humans are allergic to change. They love to say, ‘We’ve always done it that way.’ I try to fight that. That’s why I have a clock on my wall that runs counter-clockwise.” Grace Hopper is the reason I have a clock on my wall that does this. It’s good for messing with people’s minds, but it breaks an assumption.
A Distributed System is Knowable
Another impossibility that people often don’t realize they’re making, but they often do it when they’re talking about data consistency, and indeed many things about distributed systems. When people assume, we want the data that we have here in Europe to be the same as the data that we have in Hong Kong and in Singapore, within a few milliseconds. Not realizing that the speed of light means that that’s actually not going to be possible. We have limits to what is knowable within a distributed system. Leslie Lamport captured the essence of what it is to have a distributed system many years ago. A distributed system is one in which the failure of a computer you didn’t even know existed can render your own computer unusable. It reminds us of the physicality and limitations of various systems.
It goes further than just a little bit of humor. Eric Brewer formulated this, originally was a principle, it eventually got proven. It’s better known these days as the CAP theorem. It basically identifies three things, three qualities, three behaviors that we are interested in: consistency, availability, and partition tolerance. Consistency, it’s about the data. Consistency is the idea that every time you request a value for a piece of data, you will receive the most recent value, or you will receive an error. We all receive the same answer or we receive, “I’m sorry, that wasn’t available right now.” Availability is every request for such data will receive an answer, but you’re not guaranteed it’s the latest answer. Partition tolerance. Partition is a fancy way of saying, I started with one network, now I’ve got two. In other words, basically, message loss, for whatever cause. The point there is, you can have two out of three of these, but never all three. That was proven 20 years ago. You can have things be consistent. I’m going to give you the right answer, or I’m going to give you an error status in the event of any failures. I can always give you an answer. I can give you the last cached version in the event of failure. There’s also the interesting case that actually I can demonstrate, you can have the right answer, and everybody else has the right answer for once, but there is no tolerance for failure. That’s actually the limiting case of, it is viable when you’re running in a single process.
The CAP theorem is Heisenberg’s Uncertainty Principle for Distribution. All of these things are captured at a level by Douglas Adams, “We demand rigidly defined areas of doubt and uncertainty.” Indeed, we have these, but we need to understand where these are. Because there are a number of cases where we find that we are being given inconsistent things. There’s no reason for it. You often get this. You have a message that says you have four messages in your inbox, or you have four things in your scheduled post queue, or you have whatever it is, you have four of them. Then you look at the screen, it shows five. Clearly, one of these numbers is wrong, and it’s clearly the four. There are clearly five. All you have to do is take the length of that. How do we end up with such a mess? Because that’s nothing to do with distribution or eventual consistency. That’s to do with the fact that we’ve got frontends. In a frontend, you are on a system that can be consistent. This is maybe a side effect of people using micro frontends or whatever, but this has nothing to do with the limitations of a distributed system. It’s just the limitations of poorly designed client-side code. This bit is solvable.
Technical Debt Is Quantifiable as Financial Debt
The last point that I want to focus on is technical debt, that it’s actually quantifiable as financial debt. People often do this. It’s not possible. It’s not just that it’s not right, it’s also not right. In other words, its intent is actually also not possible. We understand that systems can become more complex through compromises through the nature of time. Meir Lehman captures elegantly enough in 1980, “As an evolving program is continually changed, its complexity, reflecting deteriorating structure, increases unless work is done to maintain or reduce it.” There are lots of different ways of talking about our systems and the quality, the abstract natures, we use different metaphors. The metaphor here that Martin Fowler helped popularize, came originally from Ward Cunningham, “Technical debt is a wonderful metaphor developed by Ward Cunningham to help us think about this problem.” Ward came up with it in 1992. He didn’t actually call it technical debt, he just said, we can imagine this is basically like debt. There’s a parallel here, “Like financial debt, the technical debt incurs interest payments, which come in the form of the extra effort that we have to do in future development, because of the quick and dirty design choice.” It doesn’t have to be quick and dirty, it can be quite appropriate, but it’s limited by the extent of our knowledge.
We need to remind ourselves, it’s a wonderful metaphor. It’s a metaphor. I find people taking it a little bit too literally. I found myself cautioning against the category of treating the technical debt metaphor, literally and numerically: converting code quality into a currency value on a dashboard. That’s a disastrous thing to do. It’s like the bad stats we talked about earlier. First of all, you cannot know what that value is financially. The best, you’re only ever going to end up with an estimate of the debt. There are reasons even this is not the right way of looking at it, because it is based on a fallacy and a misunderstanding. If you can find the conversion rate, well done for you. I have had people tell me, no, we do that. If you’ve got a currency value, and there are tools that will give you a currency value, there are people, you can pay them money, and they will tell you what your future is. Don’t be taken in by this. It’s nonsense. It is bad science, answered based on a deep misunderstanding. When people have said, no, we have an estimate, and they’ve used the word estimate, well done then. We have an estimate, and it’s not in currency values, it’s in hours, hours of work, in order to repay that debt. They’ve made a slight category error there. They’re assuming technical debt is the cost of repaying the debt. The problem is, technical debt is not the cost of repaying the debt. Technical debt is the cost of owning the debt. That was all of the wording that Martin, Ward, and many other people added. It’s been lost in the excitement of, maybe we can use a number for this. Be careful.
The message of the technical debt metaphor is not simply a measure of the specific work needed to repay the debt. It is the additional time and effort added to all the past, present, and future work that comes from having the debt in the first place. The burden of debt is not momentary, it’s not now. It is across all of these spans of time. How much did it cost you in the past? Then, again, in the future, how much will it cost you in the future? That value may be large or it may indeed turn out to be a zero, something you can write off. Which brings us to the end of six impossible things. These are not the only impossible things, there are other impossible things that I have hinted at. I have not fully explored the halting problem. I have not fully explored the question of the speed of light limiting certain behaviors in distributed systems, and so on. It should give you a taster of this idea that sometimes we need to step outside a little bit and look at things from a different angle that may spur on innovation. It may allow us to be creative, but it may also give us creative questions.
See more presentations with transcripts
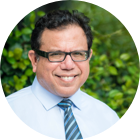
MMS • Grant Barra
Article originally posted on InfoQ. Visit InfoQ
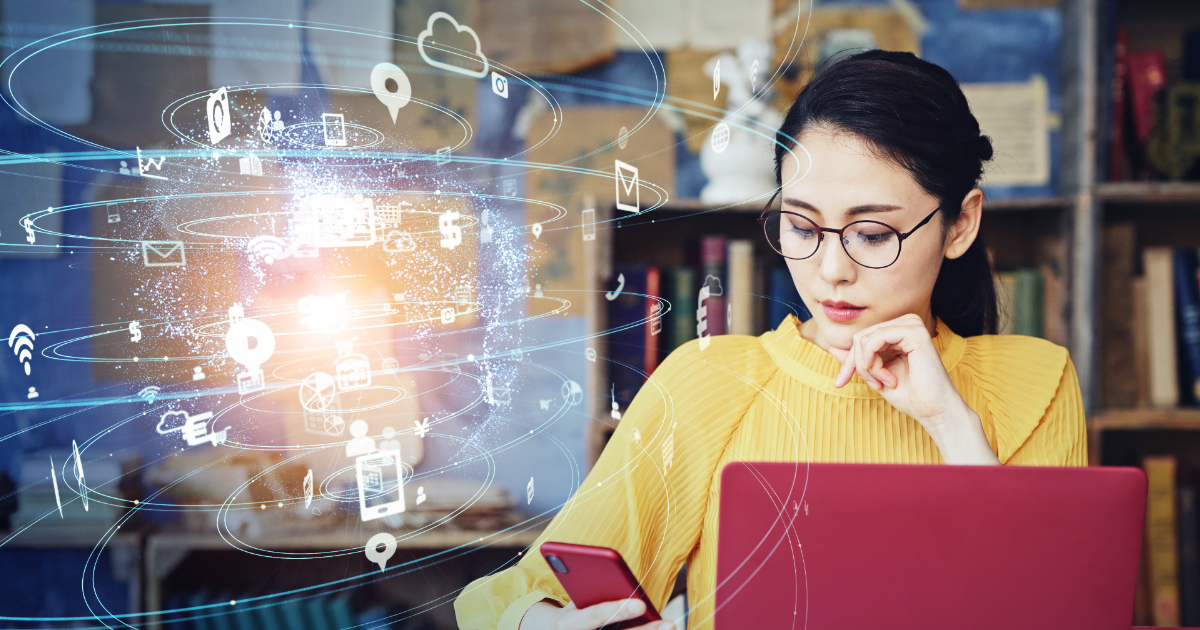
Key Takeaways
- A recent survey shows a significant number of workers would rather be fired than return to the office.
- Entrepreneurs set on working from home can start their own businesses if they find the right SaaS platform for the industry they want to work in.
- The best business-targeted SaaS platform will utilize artificial intelligence and automation to improve efficiency and customer satisfaction.
- Developers wanting to tap this trend may want to design their business-targeted platforms with these characteristics: white-label, proprietary, sales- and retention-focused, and win/win.
- Here are some inspirations to get SaaS developers started on developing successful platforms that tap this work-from-home trend for entrepreneurs.
Innovation springs from many avenues, including unexpected events like the COVID-10 pandemic, changes in industries, markets, or demographics, and problem-solving. The reaction to the pandemic drove some significant changes to the way we do business. As a result, this unexpected event presented many opportunities to start new businesses—and design the tech that drives those businesses.
When the pandemic began, it seemed the whole world was shut down almost instantly, shuttering businesses of every kind and putting people out of work. All those closures left people around the globe with ample time on their hands. The closures also led to growing creativity around how to make a living when going into the office, store, or any other place of business became an impossibility.
Many are willing to risk getting fired by refusing to return
As people’s fears over COVID-19 infection started to decline, many companies called on their employees to return to the office or other place of business. However, a significant percentage of workers just aren’t having it.
A recent survey conducted by RELI Exchange found that 26% of those who worked remotely during the pandemic are refusing to comply with their employer’s mandate to return to the office at least part time. These employees often fit the popular category of “quiet quitter,” which means they are refusing to do anything more than the bare minimum required of them.
Interestingly, the RELI Exchange survey also found that the percentage of workers who plan to start a business if they get fired is just as high as the percentage of those who simply plan to find another job—at 40% for each. Only 11% plan to ask for their job back, while just 8% plan to take an extended break.
It may seem strange that so many people are willing to risk getting fired, especially amid aggressively increasing interest rates and the threat of a recession. However, a recent Gallup poll uncovered just how widespread this quiet-quitting trend is.
It found that at least half of the workforce in the U.S. is now made up of quiet quitters, and as it turns out, the threat of so-called “quiet firing” is very real. Quiet firing involves making work uncomfortable—or even toxic for these quiet quitters, until they fold and either find another job or, as the RELI Exchange survey found, start their own business.
SaaS platforms make it easy for remote workers to start a business
These sizable percentages of people potentially getting fired or quitting and then starting their own business mean there is ample opportunity for entrepreneurs and software-as-a-service (SaaS) developers alike.
One thing that sprang from the pandemic-era lockdowns has been the spark that caused people with an entrepreneurial mindset to look for ways to move old-school business models online. The innovations that resulted from this spark have made the possibility of starting a business accessible to a wider audience.
However, those innovations require developers with the know-how and prescience to determine which types of businesses can move online without losing the key elements involved in doing business in that space. SaaS developers who understand how a wide variety of businesses work can find some incredible opportunities designing the platforms that enable remote workers to have their own business.
To be successful, these developers will have to draw from the best that today’s technology has to offer: artificial intelligence and automation.
Using AI and automation to run a business
One of the biggest challenges with starting a small business is being able to compete with the massive companies that have dominated the market share in that space for years. However, SaaS developers who know how to tap artificial intelligence and automation to drive success in any area of business will enable entrepreneurs to compete with the biggest players in their industry.
For example, artificial intelligence and automation can make businesses operate more efficiently and improve customer satisfaction. Both of these areas often rank highly when customers are searching for a company to do business with.
As a result, entrepreneurs can be nimble and compete with international corporations that have more overhead. For example, RELI Exchange is an insurtech platform that allows entrepreneurs to start their own independent insurance agency.
It utilizes SaaS technology to automate things like gathering customer information and making recommendations. This reduces the time required to serve a customer significantly, thereby creating a scalable business with very little overhead. Through automation, agents can be more productive and spend more time focusing on growing their business than on the busywork.
What to consider when creating business-targeted SaaS platforms
SaaS developers can look to a wide variety of industries for inspiration on which areas can be the most profitable — both for them as the developer and for the business owners who pay for the rights to use their platforms. The key is to look for industries with traditional or antiquated business models that haven’t seen serious innovation or been updated since technology started to grow and revolutionize multiple industries.
Real innovation requires SaaS developers to truly think outside the box and look at what technology makes possible. An easy way to go about selecting industries to revolutionize is to consider which ones primarily operate offline.
Virtually any of these traditionally offline industries are ripe for the picking by SaaS developers with the foresight to imagine what’s possible and the skill to develop a platform that every business owner in the space will want to have.
Of course, the most successful business platforms will share some key characteristics. They will be:
- White-label
- Proprietary
- Sales-focused
- Win/ win/ win
Why white-label platforms are so attractive to entrepreneurs
The first characteristic on this list is something the average SaaS developer might not think about because they are focused on the coding and other skills necessary to get the job done. However, from a small business owner’s perspective, utilizing a white-label SaaS platform to start and run their business is quite attractive.
A white-label platform enables them to establish their own brand and use their own name to sell or offer whatever services they specialize in. For business owners, branding is everything because customers often opt for the brand names they know and trust. A white-label SaaS platform gives them a firm foundation upon which to build their business.
Think of a SaaS platform as a turnkey business platform, but by making it white-label, the entrepreneur does business under their own name. Entrepreneurs buy into a platform for multiple reasons, one of which is having a proven model, systems, processes, and coaching, which increases their chances of success.
Laying a firm foundation is critical to success, as any business owner would tell you. However, the added bonus of a white-label platform enables the entrepreneur to truly make the business their own. They’re creating their own brand, but they aren’t starting from scratch.
A white-label SaaS platform upon which business owners can establish their company offers the best of both worlds: the firm foundation of a proven platform with an already-successful business model paired with the opportunity to build their own brand.
Why proprietary platforms are essential
Next, SaaS developers need to build a moat around the business because they’re making money on licensing it out. By making their platform proprietary, developers show business owners why theirs is the best option on the market. In fact, developers who get into platform design with a focus on building ready-made business models for entrepreneurs can corner the market and have a first-mover advantage.
They may find that no one else has thought up ways to revolutionize a particular industry yet. Of course, if their platform is successful, other people will attempt to replicate it. As a result, these developers will need to continue innovating on their proprietary platform to stay ahead of the competition.
Focus on sales and retention
Developers should also consider what it takes to capture the most sales in whatever industry they are targeting with the platform they’re developing. Business owners won’t go for any tech-driven model that doesn’t enable them to maximize their sales.
In some cases, it might be a good idea to collaborate with one or more business owners in the space, especially for developers who don’t have extensive knowledge about the industry they’re targeting. Seasoned professionals already know what it takes to get sales and can offer insight that could enable developers to create an online or tech-driven version of those sales methods.
An area related to sales is retention. Building a mentorship program into the model will go a long way toward retaining customers because they get real-time training to help them improve their skills and learn how to use the platform more effectively.
Make it win/ win for everyone
Finally, SaaS platforms must offer solutions that allow everyone to win. Those that don’t will not be successful. There are three groups that all must win through the use of that platform: the business owner, their customer and the developer that created it.
Business owners must reap multiple benefits from using the platform, including increases in sales, efficiency, and productivity and a decrease in expenses. It’s no secret that large corporations turn to automation and technology to accomplish these tasks. Thus, a SaaS platform that packages everything into one product for small business owners enables them to take on their much larger competition more effectively.
For customers, moving the business online can be quite beneficial. For example, the insurance industry was dramatically changed with the advent of online platforms that enabled customers to review multiple quotes all in one place.
Moving other types of businesses online can have similar effects, depending on what types of customer-centric innovations are best suited to each industry. Essentially, developers must figure out what kinds of tech-driven improvements customers want most in that particular industry.
The value of a technical mindset
Of course, SaaS developers bring their own value to the table when it comes to designing platforms for entrepreneurs wanting to start their own tech-driven business. For example, problem-solving is a primary technical skill needed by any developer wishing to design a SaaS platform for entrepreneurs. Successful developers will have to solve the problems associated with creating a platform that fits the needs of entrepreneurs in a particular industry.
Security is also a critical issue. Many entrepreneurs are handling sensitive data like payment information and even, in some cases, Social Security Numbers. Successful SaaS developers will have to build security into their platforms in an unobtrusive way that still allows for the characteristics listed above to be included.
Other considerations developers will have to think about when designing their platforms include compliance for the industry they are targeting, data management, digital marketing, and more. A technical mindset brings value to each of these areas.
Potential pitfalls when developing SaaS platforms for entrepreneurs
Of course, there are always potential pitfalls when working on any kind of technology. Every platform must be completely secure with no loopholes or backdoors. A survey conducted by Archive360 and Pulse found that 58% of global technology leaders say that many SaaS platforms don’t meet all their security requirements.
Since these business-targeted SaaS platforms are designed for use by a large number of entrepreneurs, developers must aim for the security requirements that fit the standard of whatever industry they’re targeting.
Additionally, developers must remember that the entrepreneurs who buy into their platforms will depend on them if things go wrong. When a serious problem occurs, multiple entrepreneurs may contact them all at once, creating problems for developers who don’t have a communications infrastructure in place to deal with such incidents.
Other potential pitfalls include problems with software integration from other vendors, redundant or ineffective tools or apps, and the so-called “noisy neighbor” effect, which is caused by multiple entrepreneurs using the same SaaS platform.
Tap the “-tech” trend to create solutions for entrepreneurs
With all these other factors in mind, SaaS developers may be looking for a bit of inspiration on what kinds of businesses can benefit the most from revolutionization. They need only look to the trend that has added “-tech” to multiple industries.
Perhaps one of the most well-known is fintech. There have been multiple innovations in the world of finance that have changed the industry forever. Decentralized finance (DeFI) is one of the newer innovations, and there have been many others within that DeFi umbrella. Fintech has been a particularly hot area for investment, so many fintech companies have received sizable valuations, even during the startup phase.
Education was also ripe for revolutionization, resulting in numerous edtech firms that have changed the industry forever. Students no longer need to go to a physical classroom to learn, although even those who do have multiple innovations within the classroom. The point is that more people than ever before have been able to learn in ways that have improved their educational outcomes.
Some other industries that innovation has changed forever include insurance (insurtech), real estate (proptech), law (legaltech), healthcare (healthtech), and food or dining (foodtech).
SaaS startup stories for inspiration
At this point, some developers may have some ideas for which industry you can disrupt with your next SaaS platform. However, some might need a little more to get the creative juices flowing. In fact, some developers may already be sitting on something that would make a great SaaS platform for entrepreneurs—even though they built it for something else.
For example, Slack’s founders only built the platform as a tool for themselves while working at a gaming company called Tiny Speck. They didn’t realize its commercial use until later. Some developers can find some stellar SaaS ideas based on what their customers need, which is what Mail Chimp’s founders did.
You might not even need to have a totally new idea. All you need is a platform that greatly improves what’s already available. Plaid’s founders were successful because they felt they could improve upon the other fintech platforms that connected users’ bank accounts to fintech companies. Like Slack’s founders, they only built Plaid because they needed it for something else.
In the case of RELI Exchange, the insurtech platform was built to remove the major barriers for starting an independent agency. Agency partners are provided everything they need to create a future-proof, tech driven, individually branded, independent insurance agency of their own.
Tap into the innovation
Developers need only look at current events for additional ideas. While the response to the pandemic created many problems, it also caused widespread innovation across nearly every industry. Entrepreneurs have more options for starting a business than ever before.
Quiet quitters who vehemently oppose returning to the office suddenly have the ability to start their own businesses online — without the need to establish an office or pay overhead expenses like hiring office staff. In fact, online businesses enable these quiet quitters to start their side hustle part time and then switch to full time when the business is healthy and capable of supporting them without additional income.
Finally, SaaS developers can tap into this trend by creating platforms of their own that are designed to revolutionize an industry in life-changing ways. The success of platforms like the well-known fintech Plaid and the insurtech RELI Exchange demonstrates just how lucrative this opportunity can be for developers.
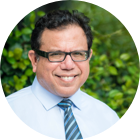
MMS • Johan Janssen
Article originally posted on InfoQ. Visit InfoQ
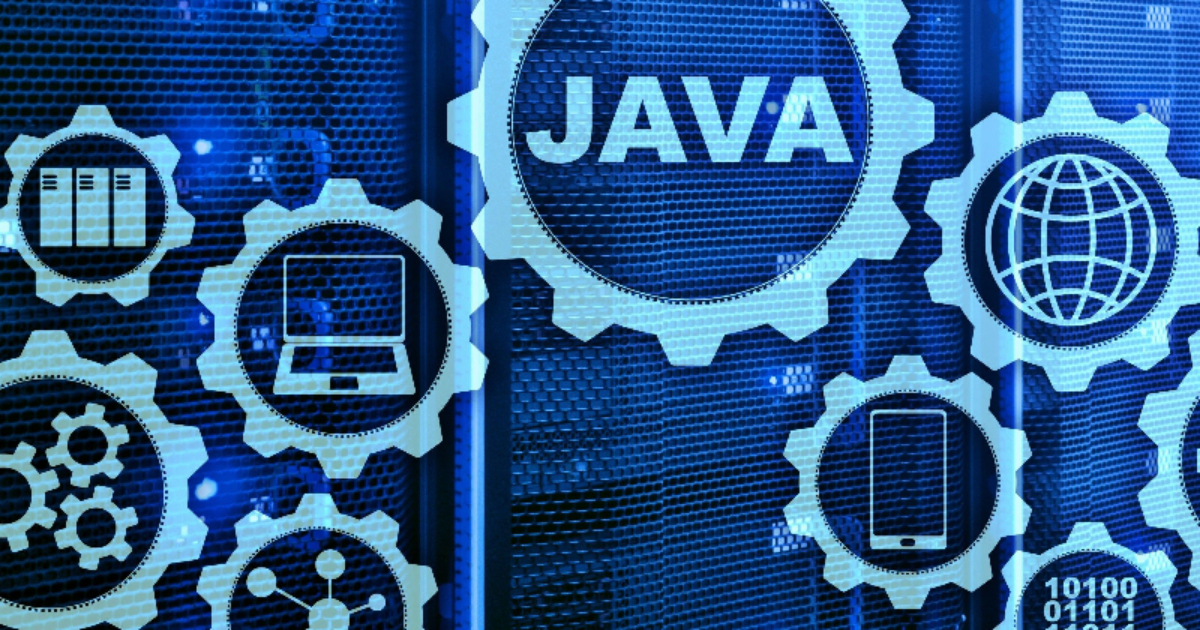
Lightbend has released Akka 22.10, the first release using the recently implemented Business License (BSL) 1.1 license model. New features include support for Java 17, Scala 3.1 and the new Projections over gRPC module.
The Akka distribution contains the modules Akka (core) 2.7.0, Akka HTTP 10.4.0, Akka gROC 2.2.0, Akka Management 1.2.0, Alpakka Kafka 4.0.0, Alpakka 5.0.0, Akka Persistence R2DBC 1.0.0, Akka Persistence JDBC 5.2.0, Akka Persistence Cassandra 1.1.0 and Akka Projections 1.3.0 combined in one umbrella release. This release uses Ubuntu style versioning while previous versions, such as Akka 2.6.20, used semantic versioning. The current version,22.10, was released in October 2022. All modules from this release are binary compatible with the previous, Apache 2 licensed, release.
Akka now includes the new Projections over gRPC module, which allows publish-subscribe communication by using gRPC instead of a message queue or broker. gRPC uses an event journal on the producer side and Akka Projections event processing together with offset tracking on the consumer side. At least once, or exactly once, delivery is supported depending on the type of Projection handler.
Java 17 is now officially supported together with Java 8 and 11. Scala 3.1 is now supported for Akka core together with Scala 2.12 and 2.13. The Akka Persistence R2DBC plugin now supports PostgreSQL and the open source cloud native Yugabyte database.
Dependencies, especially the ones with security vulnerabilities, have been updated to newer versions. Also, Lightbend, the company behind Akka, is now SOC 2 compliant and work is in progress on becoming certified by an external auditor. For the SOC 2 certification, an auditor assesses whether the vendor complies with the five trust principles: security, availability, processing integrity, confidentiality and privacy.
Work continues on Akka Edge, for which Projections over gRPC is seen as the first step. Kalix, Lightbend’s Platform as a Service, which enables developers to build distributed systems without worrying about the underlying architecture, already uses Projections over gRPC for its service to service communication. Replicated Event Sourcing for reliable multi cluster and multi region event sourcing is planned for the next Akka release.
More details may be found in the release notes.
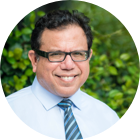
MMS • Jim Barton
Article originally posted on InfoQ. Visit InfoQ
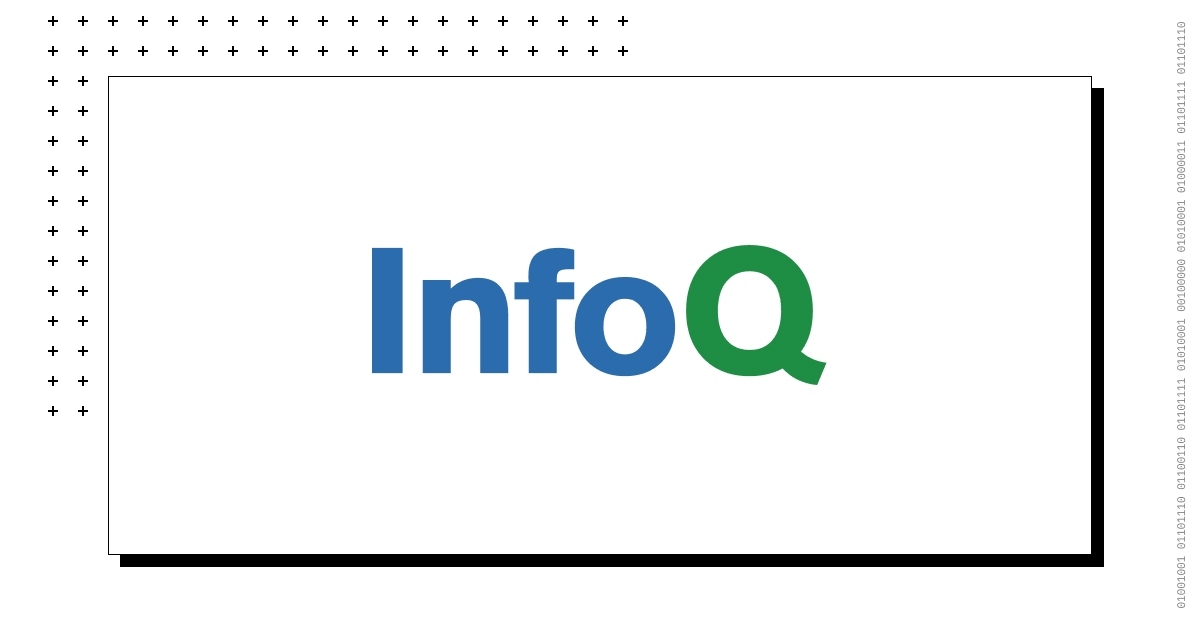
Subscribe on:
Introduction [00:01]
Thomas Betts: Hi everyone. Today’s episode features a speaker from our QCon International Software Development Conferences. The next QCon Plus is online from November 30th to December 8th. At QCon, you’ll find practical inspiration and best practices on how to implement emerging software trends directly from senior software developers at early adopter companies to help you adopt the right patterns and practices. I’ll be hosting the modern APIs track so I hope to see you online. Learn more at qconferences.com.
When building a distributed system, we have to consider many aspects in the network. This has led to many tools to help software developers improve performance, optimize requests, or increase observability. Service meshes, sidecars, eBPF, layer three, layer four, layer seven, it can all be a bit overwhelming. Luckily, today I am at QCon San Francisco and I’m talking with Jim Barton, who’s presenting a talk called Sidecars, eBPF and the Future of Service Mesh.
Jim Barton is a field engineer for Solo.io whose enterprise software career spans 30 years. He’s enjoyed roles as a project engineer, sales and consulting engineer, product development manager, and executive leader of Techstars. Prior to Solo, he spent a decade architecting, building, and operating systems based on enterprise open source technologies at the likes of Red Hat, Amazon, and Zappos.
And I’m Thomas Betts, cohost of the InfoQ Podcast, lead editor for architecture and design at InfoQ, and an application architect at Blackbaud. Jim, welcome to the InfoQ Podcast.
Jim Barton: Thank you.
Fundamentals of service mesh [01:17]
Thomas Betts: Let’s start with setting the context. Your talk’s about the future of service mesh, but before we get to the future, can you bring us up to the present state of service mesh and what are we dealing with?
Jim Barton: Sure. So service mesh technology has been around for a few years now. We’ve gained a lot of experience over that time and experimented with some different architectures, and are really getting to a point now where I think we can make some intelligent observations based on the basic experience we have and how to take that forward.
Thomas Betts: How common are they in the industry? Are everybody using them? Is it just a few companies out there? What’s the adoption like?
Jim Barton: When I started at Solo a few years ago, it was definitely in the early adopter phase, and I think now what we’re seeing is we’re beginning to get more into, I’m going to say, mid adopters. It’s not strictly the avant garde who are willing to cut themselves and bleed all over their systems, but it’s beginning to be more mainstream.
The use of sidecars in service mesh [02:08]
Thomas Betts: So sidecars was another subheading on your talk. Where are they at? Are they just a concept like a container? It’s just where you run the code next to your application. And are there common products and packages that are intended for deployment as a sidecar?
Jim Barton: If you survey the history of service mesh technology, you’ll see that sidecars is a feature of just about all of the architectures that are out there today. Basically, what that means is we have certain crosscutting concerns that we’d like the application to be able to carry out. For example, very commonly security, being able to secure all intra-service mTLS communication or secure all communication using mTLS. And so sidecars are a very natural way to do that because we don’t want each application to have to do the heavy lifting associated with building those capabilities in. So if we can factor that capability out into a sidecar, it gives us the ability to remove that undifferentiated heavy lifting from the application developers and externalize that into infrastructure. So sidecars are probably the most common way that’s been implemented since the “dawn of service mesh technology” a few years ago.
Thomas Betts: They really go hand in hand. Most of the service meshes have the sidecar, and that’s just part of what you’re going to see and part of your architecture?
Jim Barton: Absolutely. I’d say if you survey the landscape, the default architecture is the sidecar injection based architecture.
What do application developers need to know about service mesh? [03:26]
Thomas Betts: I’ve had a few roles in my career, but network engineer was never one of them. So I know that layer seven is the application layer and layer one is the wire, but I have to be honest, I would’ve to look it up on Google, whatever is in between. You talked about the whole point of the service mesh is to handle the things that the engineers and the application developers don’t have to deal with. If they have the service mesh in place, what do they need to know versus what do they not need to know?
Jim Barton: And that’s part of the focus of this talk is beginning to change the equation of what they need to know versus what they don’t need to know. Ideally, we want the service mesh technology to be as transparent to application developers as possible. Of course, that’s an objective that we aspire to but we may never 100% reach, but it’s definitely something where we’re moving strongly in that direction.
Thomas Betts: And the other part of my intro with the layers is where does all this technology operate? Where does the service mesh and the sidecar fit in? Where does eBPF fit into this? And does the fact that they operate at different levels affect what the engineers need to know?
Jim Barton: Wow, there’s a lot to unpack in that question there. So I would say transparency, as I said before, is an ultimate goal here. We want to get to the point where application developers know as little as possible about the details of the mesh. Certainly, if you look at the way that developers would have solved these kinds of requirements five years ago, there would’ve been an awful lot of work required to integrate the various components. Let’s take mTLS as an example to integrate the components into each individual application to make that happen, so there was a very high degree of developer knowledge of this infrastructure piece. And then, as we’ve begun to separate out the cross-cutting concerns from the application workloads themselves, we’ve been able to, over time, decrease the amount of knowledge that developers have to have about each one of those layers. There’s still a fair amount, and that’s one of the things that we’re addressing in this talk and in the service mesh community in general is getting to a point where the networking components, whether it’s layer four or layer seven, will be isolated more distinctly from the services themselves.
mTLS is the most common starting point for service mesh adoption [05:32]
Thomas Betts: You mentioned mTLS a few times. What are some other common use cases you’re seeing?
Jim Barton: Sure, yeah. I mentioned mTLS just because we see that as probably the most common one that people come to a service mesh with. You look at regulated industries like financial services and telco, you look at government agencies with the Biden administration’s mandate for Zero Trust architectures, and mTLS security, and so forth. So we see people coming to us with that requirement an awful lot but yeah, certainly there are a lot of others as well.
The three pillars of service mesh are connect, secure, and observe, and so we typically see things falling into one of those three buckets. So security is a central pillar and, as I said before, is probably the most common initial use case that users come to us with.
But also just think about the challenges of observability in a container orchestration framework like a Kubernetes. You have hundreds of applications, you have thousands of individual deployments that are out there, something goes wrong. How do you understand what’s happening in that maze of applications and interconnections? Well, in the old world of monolithic software, it wasn’t that difficult to do. You attach a debugger and you can see what’s happening inside the application stack. But every debugging problem becomes a murder mystery that we have to figure out who done it, and where they were, and why. It just adds completely new sets of dimensions to the problems where you’re throwing networking into the mix at potentially every hop along the way. So I’d say observability is also a very common use case that people come to us with, with respect to service mesh technology. What’s going on? How can I see it? How can I understand that? And so service meshes are all about the business of producing that information in a form that it can be analyzed and acted upon.
Also, from a connectivity standpoint, so the third pillar, connect, secure, observe, from a connectivity standpoint, there’s all kinds of complexities that can be managed in the mesh that in the past, certainly when my career started, you would handle those things in the application layer themselves. Things like application timeouts, failovers, fault injection for testing, and so forth. Even say at the edge of the application network, being able to handle more, let’s say, advanced protocols, maybe things like gRPC, or OpenAPI, or GraphQL, or SOAP, those are all pretty challenging connectivity problems that can be addressed within service mesh.
Service mesh and observability [08:01]
Thomas Betts: So going back to the observability, since this is something that’s at the network layer, is it just observability of the network or are we able to listen for things that are relevant to the application’s behavior? Is that still inside the app code to say I need this type of observability, this method was called, or can you detect all that?
Jim Barton : With the underlying infrastructure that’s used within the service mesh, just particularly if you look at things like Envoy Proxy, there are a whole lot of network and even some application level metrics that are produced as well. And so there’s a lot of that information that you really do get for free just out of the mesh itself. But of course, there’s always a place for individual applications publishing their own process-specific, application-specific metrics, and including that in the mix of things that you analyze.
Thomas Betts: And is that where things like OpenTelemetry, that you’d want to have all that stuff linked together so that you can say, “This network request, I can see these stats from service mesh and my trace gets called in with this application.”?
Jim Barton: Absolutely. Those kinds of standards are really critical, I think, for taking what can be a raft of raw data and assembling that into something that’s actually actionable.
The role of eBPF in service mesh [09:01]
Thomas Betts: So the observability, I think, is where eBPF, at least as I understand it, has really come into play, is that we’re able to now listen at the wire effectively and say, “I want to know what’s going on.” What exactly does that mean? Does it eliminate the need for the sidecar? Does it augment it? And does it solve a different problem?
Jim Barton: eBPF has gotten a ton of hype recently in the enterprise IT space, and I think a lot of that is well deserved. If you look at open source projects like Cilium at the CNI networking layer, it really does add a ton of value and a lot of efficiency, additional security capabilities, observability capabilities, that sort of thing. I think when we think about that from a service mesh standpoint, certainly there’s added value there, but I don’t think, at least from a service mesh standpoint, most of what we see is eBPF not being so much a revolution, but more of a evolution and an improvement in things that we already do.
Just to give you an example, things like short circuiting certain network connections. Whereas before, without eBPF, I might have to traverse call going from A to B, I might have to traverse a stack, go out over the network, traverse another layer seven stack, and then send that to the application. With eBPF, there are certain cases where I can short circuit some of that, particularly if it’s intra-node communication, and can actually make that quite a bit more efficient. So we definitely see enhancements from a networking standpoint as well as an observability and a security standpoint.
Roles of developers and operations [10:29]
Thomas Betts: When it comes to implementing the eBPF or a service mesh in general, is that really something that’s just for the infrastructure and ops teams to handle? Developers need to be involved in that? Is that part of the devops overlapping term or is there a good separation of what you need to know and what you should be capable of doing?
Jim Barton: That’s the great insight and a great observation. I think certainly our goal is to make the infrastructure as transparent as possible to the application. We don’t want to require developers, let’s say an application developer who’s using service mesh infrastructure to be required to understand the details of eBPF. eBPF is a pretty low level set of capabilities. You’re actually loading programs into the kernel and operating those in a sandbox within the kernel. So there’s a lot of gory details there that a typical application owner, developer would rather not have to be exposed to, and so one of our goals is to provide the goodness that that kind of infrastructure can deliver, but without surfacing it all the way up to the application level.
Thomas Betts: So are the ops teams able to then just implement service mesh without having to talk to the dev teams at all, or does it change the design decisions of the application to say because we have the service mesh in place and we know that it can handle these things, I can now reduce some of the code that I need to write, but they need to work hand in hand to make those decisions?
Jim Barton: Definitely ops teams and app dev teams, they obviously still need to communicate. I see a lot of different platform teams, ops teams in our business and I think the ones that are the most effective, it’s a little bit like an official at a sports event, at a basketball game, they’re most effective when you’re not aware of their presence. And so I think a good platform team operates in a similar way, they want to provide the app dev teams the tools they need to do what they do as efficiently as possible within the context of the values of the organization that they represent, but the good ones don’t want to get in the way. They want to make the app dev teams be as effective, and efficient, and as free flowing as possible without interfering.
Thomas Betts: Building on that, how does this affect the code that we write, if at all?
Jim Barton: There are some cases today where occasionally the service mesh can impact what you do in the application itself, but those are the kinds of use cases that the community is moving as aggressively as it can to root those out, to make those unnecessary anymore. And I think with a lot of the advances that are coming with the ambient mesh architecture that we’re going to talk about a fair amount in the talk tomorrow, that plays a big role in removing some of those cases where there does have to be a greater degree of infrastructure awareness on the application side.
Thomas Betts: Yeah, I think that’s always one of those trade offs that back when you were just doing a monolith and there was probably small development team, you had to know everything. When I started out, I had to know how to build the server, and write the code, and support it. And as you got to larger companies, larger projects, bigger applications, distributed applications, you started handing off responsibilities, but it also changed your architectural decisions sometimes, that you got to microservices because you needed independent teams to work independently. This is a platform service. You don’t have a service mesh for just one node of your Kubernetes cluster, this seems to be something that has to solve everyone’s problems. So who gets involved in that? Is it application architects? Is it developers on each of the teams to decide here’s how our service mesh path is going to go forward?
Jim Barton: So how do we separate responsibilities across the implementation of a service mesh? I would definitely say in the most effective teams that I see, the platform team frequently takes the lead. They’re often the ones who are involved earliest on because they’re the ones who have to actually lay down the bits, and set down the processes and the infrastructure that are going to allow the development teams to be effective in their jobs. That being said, there’s clearly a case where the application teams need to start onboarding the mesh, and so the goal is to make that process as easy as possible and the operational process there as easy as possible. There definitely needs to be a healthy communication channel between the two, but I would say, from my experience at least, if the platform team does its job well, it should be a little bit like unofficial at a basketball game that’s being well officiated, they shouldn’t be top level of my consciousness every day as an app developer.
Who makes decisions regarding service mesh configuration? [14:39]
Thomas Betts: I think the good deployment situations I’ve run into personally have been I just write my code, check it in, the build runs, it deploys, and look, there’s something running over there, and there’s a bunch of little steps in the build pipeline and the release pipeline that I don’t need to understand. And I know that I can call out to another service because I know how to write my code to call their services. How does that little piece change? Because I have my microservices and I know I have all these other dependencies. Is that another one of those who gets to decide how those names and basically the DNS problem, the DNS is always the problem, that we’re offloading the DNS problem to the service mesh in some ways to embrace that third pillar of connectivity. Where does that come into play? Is it just another decision that has to be made by the infrastructure team?
Jim Barton: I would say a lot of those decisions, at least in my experience, it can vary from team to team where those kinds of decisions get made, but definitely there are abstractions in the service mesh world that allow you to specify, “Hey, here is a virtual destination that represents a target service that I want to be able to invoke.” From an application client standpoint, it’s just a name. I’ve used an API, I’ve laid down some bits that basically abstracts out all that complexity. Here’s a name I can invoke, very easy, very straightforward way to invoke a service. Now, behind the scenes of that virtual destination, there may be all kinds of complexity. I may have multiple instances that are active at any point in time within my cluster, I may have another cluster that gets failed over to. If cluster one fails, I may fail over to another cluster that has that same service operational. In that case, there’s a ton of complexity behind the scenes that the service mesh ideally will hide from the application developer client. At least that’s the place that the people I work with want to get to.
OpenAPI and gRPC with service mesh [16:22]
Thomas Betts: And then I want to flip this around. So I deal with OpenAPI Swagger specs all the time, and that’s how I know how to call that other service. We’ve talked about how my code should be unaware of the service mesh underneath. Does the service mesh benefit if it knows anything about how my API is shaped? And is there a place for that?
Jim Barton: I would say ideally, not really. There are definitely a variety of API contract standards out there that have different levels of support, depending on what service mesh platform you’re using. OpenAPI, to take probably the most common example in today’s world, is generally pretty well supported. I certainly know that certainly in our context with Istio, that’s a very common pattern. And so there are others. Some of the more emerging standards, for example GraphQL, I think the support for that is less mature because it’s a newer technology and it’s more complex. It’s more about, whereas OpenAPI tends to take a service or a set of services and provide an interface on top of that, GraphQL, when you get to a large-scale deployment of that, potentially provides a single graph interface that can span multiple suites of applications that are deployed all over the place. So there’s definitely an additional level of complexity as we move to a standard like GraphQL that’s less well understood than say something that’s been around for a while, like OpenAPI.
Thomas Betts: The third one that’s usually thrown around with those two is gRPC. And so that’s where gRPC is relying on a little bit of different, it’s HTP2, it’s got a little bit more network requirements. And so just saying that you’re going to use gRPC already implies that you have some of that knowledge of the network into your application. You’re not just relying on it’s HTTP over Port 80 or 443, everything’s different underneath that. Does that change again or is that still in the wheelhouse of service mesh just handles that, it’s fine, no big deal?
Jim Barton: I see gRPC used a lot internally within service meshes. I don’t see it used so much from an external facing standpoint, at least in the circles where I travel. So you’re absolutely correct, it does imply some underlying network choices with gRPC. I’d say because it is a newer, perhaps slightly less common standard than OpenAPI in practice, there’s probably not quite as pervasive support for it in the service mesh community, but certainly in a lot of cases it’s supported well and doesn’t really change the equation, from my standpoint, vis-a-vis something like OpenAPI.
Sustainability and maintainability [18:49]
Thomas Betts: So with all of the service mesh technology, does this help application long term sustainability and maintainability? Having good separation of concerns is always a key factor. This seems like it’s a good way to say, “I can be separated from the network because I don’t have to think about connectivity and retries because that’s no longer my application’s problem.” Is that something you’re seeing in the industry?
Jim Barton: Absolutely. I’d say being able to externalize those kinds of concerns from the application itself is really part and parcel of what service mesh brings to the table, specifically the connect pillar of service mesh value. So yeah, absolutely. Being able to externalize those kinds of concerns into a declarative policy, being able to express that in YAML, store it in a repo, manage it with a GitOps technology like an Argo or a Flux, and be able to ensure that policy is consistently applied throughout a mesh, there’s a ton of value there.
Service mesh infrastructure-as-code [19:43]
Thomas Betts: Your last point there about having the consistency, that seems to be very important. Microservices architecture, one of the jokes but it’s true, is that you went from having one problem to having this distributed problem, and your murder mystery is correct. You don’t even know that there is another house that the body could be buried in. So is this a place where you can see that service mesh comes in because it is here’s how the service mesh is implemented, all of our services take care of these things, we don’t have to worry about them getting out of sync, and that consistency is really important to have a good microservices architecture?
Jim Barton: Yeah, let me tell you a story. I spent many years at Red Hat and we did some longterm consulting engagements with a number of clients. One of them was a large public sector client whose name you would recognize, and we were working with them on a longterm, high touch, multi-week engagement. And we walked in one Monday morning and something happened, I still don’t know what it is, but our infrastructure was gone. Basically, it was completely hosed. And so in the old world, we would’ve been in a world of hurt. We’d had to go debug, and so we start going down this path of all right, what happened? That’s our first instinct as engineers, we want to know what happened, what was the problem?
And we investigated that for a while and finally someone made the brilliant observation, “We don’t necessarily have to care about this. We’ve built this project right, we have all of the proper devops principles in place here, all we really have to do is go press this button. We can regenerate the infrastructure and by lunchtime we can be back on our development path again.” And so that was the day I became a believer, a true dyed-in-the-wool believer in devops technology, and it was just that it was the ability to produce that infrastructure consistently based on a specification, and it’s just invaluable.
Thomas Betts: I’ve seen that personally from one service, one server, one VM, but having it across every node in your network, across your Kubernetes, all of that being defined, and infrastructure as code is so important for all those reasons. That applies as well to the service mesh because you’re saying the service mesh is still managed in YAML stored in repo.
Jim Barton: It’s basically the same principles you would apply to a single service and simply adopting them at scale, pushing them out at scale.
Ambient Mesh [21:53]
Thomas Betts: We spent a lot of time talking about the present. I do want to take the last few minutes and talk about what’s the future? So what is coming? You mentioned ambient mesh architecture. Let’s just start with that. What’s an ambient mesh?
Jim Barton: Ambient mesh is the result of a process that Google and Solo have collaborated on over the past year, and it’s something we are really, really excited about at Solo. And so we identified a number of issues, again, from our experience working with clients who are actually implementing service mesh, things that we would like to improve, things that we would like to do better to make it more efficient, more repeatable, to make upgrades easier, just a whole laundry list of things. And so over the past year, we’ve been collaborating on this, both Google and Solo are leaders in the Istio community, and just released in the past month, the first down payment, I’m going to say, an experimental version of Istio ambient mesh into the Istio community. And so what that does is it basically takes the old sidecar model that’s the traditional service mesh model, and gives you the option of replacing that with a newer data plane architecture that’s based on a set of shared infrastructure resources as opposed to resources that are actually attached to the application workloads, which is how sidecar operates.
Benefits of shared service mesh infrastructure [23:12]
Thomas Betts: What are the benefits of having that shared infrastructure? Is it you only need to deploy 1 thing instead of 10 copies of the same thing?
Jim Barton: The benefits that we see are threefold, really. One is just from a cost of ownership standpoint, replacing a set of infrastructure that is attached to each workload, and being able to factor that out into shared infrastructure. There are a lot of efficiencies there. There’s a blog post out there that we produce based on some hopefully reasonable assumptions that estimate reduction in cost and so forth on the order of 75%. Obviously, your mileage varies depending on how you have things deployed, but there’s some significant savings from just an infrastructure resource consumption standpoint. So that’s one, is just cost of ownership.
A second benefit is just operational impact. And so we see, particularly for customers who do service mesh at scale, let’s pick a common one, let’s say that there’s an Envoy CVE at layer seven, and that requires you to upgrade all of the proxies across your service mesh. Well, that can become a fairly challenging task.
If you’re operating at scale, you have to schedule some kind of downtime that can accommodate this rolling update across your entire service network so that you can go and then apply those envoy changes incrementally. That’s a fairly costly, fairly disruptive process, again, when you’re operating at scale. And so by separating that functionality out from the application sidecar into shared infrastructure, it makes that process a whole lot easier. Now, the applications don’t have to be recycled when you’re doing an upgrade like that, it’s just a matter of replacing the infrastructure that needs to be replaced and the applications are none wiser, they just continue to operate. It goes back to the transparency we were talking about before. We want the mesh to be as transparent as possible to the applications who are living in it.
We talked about cost of ownership, we talked about operational improvements, and third, we should talk about performance. One of the things we see when operating service meshes at scale is that all of these sidecar proxies, they add latency into the equation. And the larger your service network is and the more complex the service interactions are, the more latency that gets added into the process with each service call. And so part of that is because each one of those sidecars is a full up layer seven Envoy proxy, so it takes a couple of milliseconds to traverse the TCP stack typically. And if you’re doing that both at the sending end and the receiving end on every service call, you can do the math on how that latency adds up.
And so by factoring things out with the new ambient mesh architecture, we see, for a lot of cases, we can cut those numbers pretty dramatically. Let’s just take an mTLS use case because, again, that’s a very common one to start with. We can reduce that overhead from being two layer seven Envoy traversals at two milliseconds each, we can reduce that to being two layer four traversals of a secure proxy tunnel at a half millisecond each. And so you do the math on a high scale service and it cuts the additional latency, it improves performance numbers quite a bit.
Thomas Betts: And obviously, that’s what you’re getting into, it makes sense at scale. This isn’t a concern that you have with a small cluster, when you’re talking about the two milliseconds to half millisecond makes a difference when you’re talking about tens of thousands of them.
Jim Barton: The customers we work with, I mean we work with a variety of customers. We work with small customers, we work with large customers. Obviously, the larger enterprises are the more demanding ones, and they’re the ones who really care about these sorts of issues, the issues of operating at scale. And so we think what we’re doing is by partnering with those people, we’re driving those issues out as early in this process as possible so that when people come along with more modest requirements, it’s just they’re non-issues for them.
Future enhancements for GraphQL and service mesh [27:06]
Thomas Betts: Was there anything else in the future of service mesh besides the ambient mesh?
Jim Barton: First of all, the innovation within the ambient mesh itself is not done. Solo and Google wanted to get out there as quickly as possible something that people could get their hands on, and put it through its paces, and get us some feedback on that.
But we already know there are innovations within that space that need to continue. For example, we discussed eBPF a bit earlier, optimizing some of the particular new proxies that are in this new architecture, being able to optimize those to use things like eBPF, as opposed to just using a standard Envoy proxy implementation, to really drive the performance of those components just as hard as we possibly can. So there are definitely some, I’m going to say, incremental changes, not architectural changes, but incremental changes, that I think are going to drive the performance of ambient mesh.
So that’s certainly one thing, but we also see a lot of other innovations that are coming along the data plane of the service mesh as well. One that we are really excited about at Solo has to do with GraphQL technology. GraphQL to date has typically required a separate server component that’s independent of the rest of API gateway technology that you might have deployed in your enterprise. And we think fundamentally that’s a curious architecture. I have an API gateway today, it knows how to serve up OpenAPI, it knows how to serve SOAP, it knows how to serve gRPC maybe. Why is GraphQL this separate unicorn that needs its own separate server infrastructure that has to be managed? Two API gateway moving parts instead of one.
And so that’s something that we’ve been working on a lot at Solo and have GraphQL solutions out there that allow you to absorb the specialized GraphQL server capabilities into just another Envoy filter on your API gateway as opposed to have it being this separate unicorn that has to be managed separately. That’s something that we see again in the data plane of the service mesh that’s going to become even more important as GraphQL, again, my crystal ball, I don’t know if I brought it with me today, but if I look into my crystal ball, GraphQL’s adoption curve looks to be moving up pretty quickly. And we’re excited about that, and we think there are some opportunities for really improving on that from an operational and an infrastructure standpoint.
Thomas Betts: So the interesting question about that is most of the cases I hear about GraphQL, it’s that front end serving layer. I want to have my mobile app be as responsive as possible, it’s going to make one call to the GraphQL server to do all the things and then the data’s handled. That’s why it’s got that funny architecture you described. But I see service mesh is mostly on the backend between the servers. Is this a service mesh at the edge then?
Jim Barton: Service mesh has a number of components. Definitely, with the ambient mesh component that we’ve been talking about, we’re talking, to a large extent, about the internal operations of the service mesh, but an important component of service mesh and, in fact, one of the places where we see the industry converging is in the convergence of traditional standalone API gateway technology and service mesh technology. For example, let’s take Istio as an example in the service mesh space, there’s always been a modest gateway component, Istio ingress gateway, that ships with Istio.
It’s not that full featured, it’s not something that typically, at least on its own as it comes out of the box, is something that people would use to solve complex API gateway sorts of use cases. And so Solo has actually been in this space for a while and has actually brought a lot of its standalone API gateway expertise into the service mesh. In fact, we just announced recently a product offering that’s part of the overall service mesh platform that’s called Gloo gateway that addresses these very issues, being able to add a sophisticated, full-featured API gateway layer at the north south boundary of the service mesh.
Thomas Betts: Well, I think that’s about it for our time. I want to thank Jim Barton for joining me today and I hope you’ll join us again for another episode with the InfoQ podcast.
References
.
From this page you also have access to our recorded show notes. They all have clickable links that will take you directly to that part of the audio.
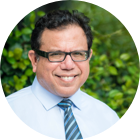
MMS • Matt Saunders
Article originally posted on InfoQ. Visit InfoQ
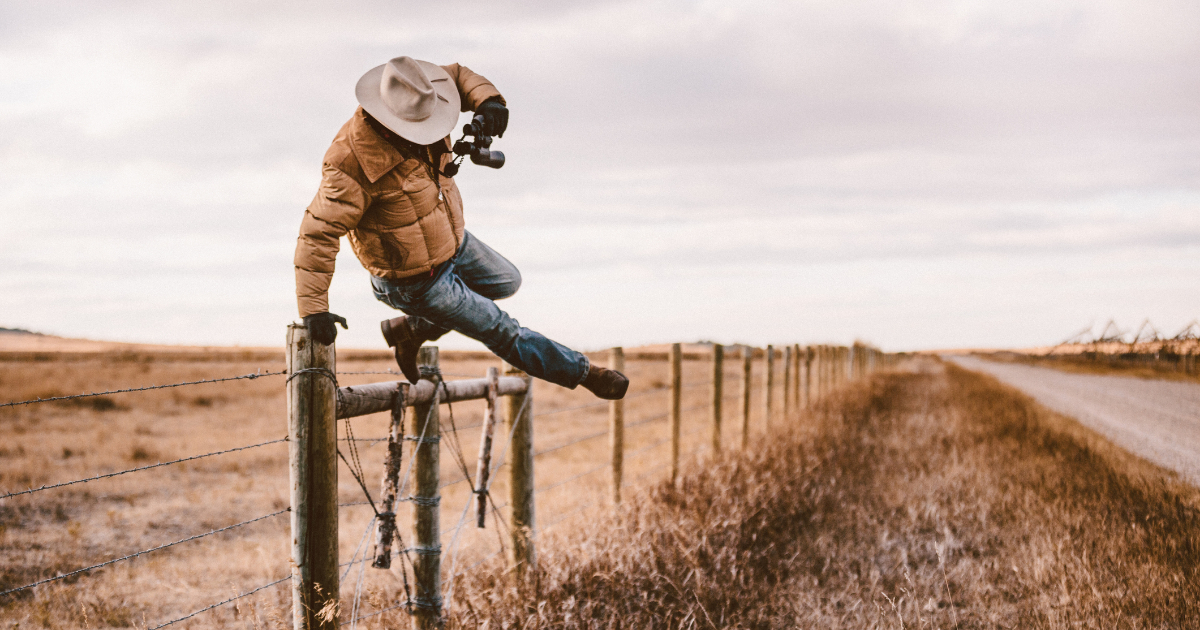
Following a successful beta trial, Hashicorp have announced the general availability of Boundary on their cloud platform HCP. This adds a key new aspect to Hashicorp’s managed solution for zero-trust security.
First released in October 2020, Boundary is an open-source project providing identity-based access to an organization’s applications and critical systems with fine-grained authorization. It does this without managing credentials, nor by exposing an organization’s network externally.
Many organizations moving to the cloud are adopting zero-trust security postures, with the new default being to trust nothing and no-one, and to authenticate and authorize everything. Existing software-defined perimeter solutions such as VPNs and PAM tend to be IP-driven and laboriously manual, whereas Boundary gives transparent fine-grained access to users and hosts. Hashicorp’s approach caters multiple clouds, on-premises and hybrid environments, reducing attack vectors and protecting data at every stage. HCP Boundary provides the third leg of Hashicorp’s zero-trust security solution – alongside HCP Consul and HCP Vault – providing automated workflows for users to access critical cloud-based infrastructure. Seamless onboarding of new users and managed resources also means that the day-to-day configuration overhead of a growing cloud infrastructure is minimized.
“At HashiCorp, we have always believed that identity is the foundation for zero trust security for applications, networks, and users. With HCP Boundary, companies now have a modern solution for privileged access management, securing access in dynamic, ephemeral environments for their workforce. We think we’ve reached an important milestone for our customers by delivering a security solution built for today’s threat and infrastructure landscape.” – Armon Dadgar, co-founder and CTO, HashiCorp
Boundary has been available in HCP (Hashicorp Cloud Platform) as a beta since June 2022, and now provides a production-ready managed service for remote access to cloud-based installations. HCP Boundary builds on the open-source version by adding enterprise functionality, such as dynamic credential injection through an integration with Vault. This allows users to access Boundary-managed hosts with single-use passwordless authentication, minimizing the possibility of credential leaks.
Boundary integrates with existing identity providers supporting OIDC, such as Microsoft Active Directory and Okta, and can also automatically discover services using dynamic service catalogs from Microsoft Azure and AWS, and can also integrate with Terraform to discover resources to manage. Finally, sessions authorized with Boundary are logged and auditable, to provide usage insights into sessions and events.
As HCP Boundary is a fully-managed service, Hashicorp take care of maintaining, managing and scaling production Boundary deployments, relieving admins from this responsibility. HCP Boundary is available for signup now.
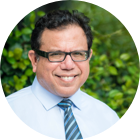
MMS • Omer Hamerman
Article originally posted on InfoQ. Visit InfoQ
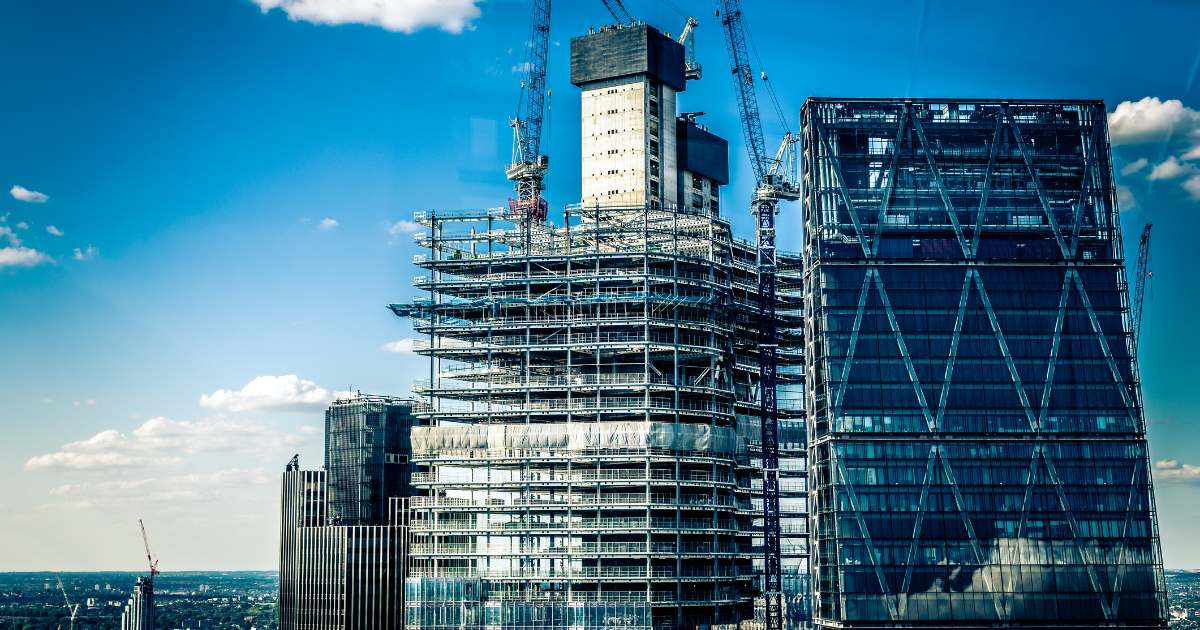
Key Takeaways
- While both CloudFormation and Terraform have the concept of modules, Terraform’s is better defined
- State storage in Terraform requires special care as the state file is needed to understand the desired state and can contain sensitive information
- CloudFormation excels at deploying AWS infrastructure whereas Terraform is best suited for dynamic workloads residing in multiple deployment environments where you want to control additional systems beyond the cloud
- Many organizations choose to use Terraform for databases and high-level infrastructure and CloudFormation for application deployment
- Since Terraform uses HCL, this can create beneficial segregation between Ops and Dev as Dev may not be familiar with this language.
While both CloudFormation and Terraform are robust IaC platforms that offer efficient configuration and automation of infrastructure provisioning, there are a few key differences in the way they operate. CloudFormation is an AWS tool, making it ideal for AWS users looking for a managed service. Terraform, on the other hand, is an open-source tool created by Hashicorp which provides the full flexibility, adaptability, and community that the open-source ecosystem has to offer. These differences can be impactful depending on your specific environment, use cases, and several other key factors.
In this post, I’ll compare CloudFormation and Terraform based on important criteria such as vendor neutrality, modularity, state management, pricing, configuration workflow, and use cases to help you decipher which one is the best fit for you.
But first, I’ll provide a bit of background on each platform and highlight the unique benefits that each of them brings to the table.
What is CloudFormation?
AWS CloudFormation is an Infrastructure as Code (IaC) service that enables AWS cloud teams to model and set up related AWS and third-party resources in a testable and reproducible format.
The platform helps cloud teams focus on the application by abstracting away the complexities of provisioning and configuring resources. You also have access to templates to declare resources; CloudFormation then uses these templates to organize and automate the configuration of resources as well as AWS applications. It supports various services of the AWS ecosystem, making it efficient for both startups and enterprises looking to persistently scale up their infrastructure.
Key features of CloudFormation include:
- Declarative Configuration with JSON/YAML
- The ability to preview environment changes
- Stack management actions for dependency management
- Cross-regional account management
What is Terraform?
Terraform is Hashicorp’s open-source infrastructure-as-code solution. It manages computing infrastructure lifecycles using declarative, human-readable configuration files, enabling DevOps teams to version, share, and reuse resource configurations. This allows teams to conveniently commit the configuration files to version-control tools for safe and efficient collaboration across departments.
Terraform leverages plugins, also called providers, to connect with other cloud providers, external APIs, or SaaS providers. Providers help standardize, provision, and manage the infrastructure deployment workflow by defining individual units of infrastructure as resources.
Key features of Terraform include:
- Declarative configurations via Hashicorp Configuration Language (HCL)
- The support of local and remote execution modes
- Default version control integration
- Private Registry
- Ability to ship with a full API
Comparing CloudFormation and Terraform
Vendor Neutrality
The most well-known difference between CloudFormation and Terraform is the association with AWS. While you can access both tools for free, as an AWS product, CloudFormation is only built to support AWS services. Consequently, it is only applicable for deployments that rely on the AWS ecosystem of services. This is great for users who run exclusively on AWS as they can leverage CloudFormation as a managed service for free and at the same time, get support for new AWS services once they’re released.
In contrast, Terraform is open-source and works coherently with almost all cloud service providers like Azure, AWS, and Google Cloud Platform. As a result, organizations using Terraform can provision, deploy, and manage resources on any cloud or on-premises infrastructure, making it an ideal choice for multi-cloud or hybrid-cloud users. Furthermore, because it is open source and modular, you can create a provider to wrap any kind of API or just use an existing provider. All of these capabilities have already been implemented by various vendors which offer users far more flexibility and convenience, making it ideal for a greater variety of use cases.
However, on the downside, Terraform often lags behind CloudFormation with regard to support for new cloud releases. As a result, Terraform users have to play catch-up when they adopt new cloud services.
Modularity
Modules are purposed for reusing and sharing common configurations, which renders complex configurations simple and readable. Both CloudFormation and Terraform have module offerings, however, CloudFormation’s is newer, and therefore not as mature as Terraform’s.
CloudFormation has always offered features to build modules using templates, and as of 2020, they now offer out-of-the-box support for modules as well. Traditionally, CloudFormation leverages nested stacks, which allow users to import and export commonly used configuration settings. Over the past few years, however, CloudFormation launched both public and private registries. As opposed to Terraform, CloudFormation offers its private registry right out-of-the-box which enables users to manage their own code privately without the risk of others gaining access to it. Furthermore, CloudFormation’s public registry offers a wide array of extensions such as MongoDB, DataDog, JFrog, Check Point, Snyk, and more.
Despite the fact that CloudFormation has come a long way in its modularity, Terraform has innately supported modularity from the get-go, making its registry more robust and easier to use. The Terraform registry contains numerous open-source modules that can be repurposed and combined to build configurations, saving time and reducing the risk of error. Terraform additionally offers native support for many third-party modules, which can be consumed by adding providers or plugins that support the resource type to the configuration.
State Management
One of the benefits of CloudFormation is that it can provision resources automatically and consistently perform drift detection on them. It bundles AWS resources and their dependencies in resource stacks, which it then uses to offer free, built-in support for state management.
In contrast, Terraform stores state locally on the disk by default. Remote storage is also an option, but states stored remotely are written in a custom JSON file format outlining the model infrastructure and must be managed and configured. If you do not manage state storage properly, it can have disastrous repercussions.
Such repercussions include the inability to perform DR because drifts have gone undetected, leading to extended downtime. This occurs if the state is impaired and can’t run the code, which means the recovery has to be done manually or started from scratch. Another negative repercussion is if the state file for some unexpected reason becomes public. Since state files often store secrets such as keys to databases or login details this information is quite dangerous to your organization if it gets into the wrong hands. If Hackers find state files it’s easier for them to attack your resources. This is an easy mistake to make since generally speaking, Terraform users that manage their own state on AWS store the files in an S3 bucket, which is one-way state files can be exposed publicly.
To combat this challenge, Terraform also offers an efficient deployment of self-managed systems that take care of the state by leveraging AWS S3 and DynamoDB. In addition, users can also purchase Terraform’s Remote State Management to automatically maintain state files as a service.
Pricing, License & Support
CloudFormation is a free service within AWS and is supported by all AWS pricing plans. The only cost associated with CloudFormation is that of the provisioned AWS service.
Terraform is open-source and free, but they also offer a paid service called Terraform Cloud which has several support plans like Team, Governance, and Business that enable further collaboration. Terraform Cloud offers additional features like team management, policy enforcement, a self-hosted option, and custom concurrency. Pricing depends on the features used and the number of users.
Language
CloudFormation templates are built using JSON/YAML, while Terraform configuration files are built using HCL syntax. Although both are human-readable, YAML is widely used in modern automation and configuration platforms, thereby making CloudFormation much easier to adopt.
On the other hand, HCL enables flexibility in configuration, but the language requires getting used to it.
It’s also worth mentioning some IaC alternatives that offer solutions for those who prefer to use popular programming languages. For example, in addition to CloudFormation, AWS offers CDK which enables users to provision resources using their preferred programming languages. Terraform users can enjoy the same benefits with AWS cdktf which allows you to set HCL state files in Python, Typescript, Java, C#, and Go. Alternatively, Pulumi offers an open-source IaC platform that can be configured with a variety of familiar programming languages.
Configuration Workflow
With CloudFormation, the written code is stored by default locally or in an AWS S3 bucket. This configuration workflow is then used with the AWS CLI or the AWS Console to build the resource stack.
Terraform uses a straightforward workflow that only relies on the Terraform CLI tool to deploy resources. Once configuration files are written, Terraform loads these files as modules, creates an execution plan, and applies the changes once the plan is approved.
Use Cases
While both CloudFormation and Terraform can be used for most standard use cases, there are some situations in which one might be more ideal than the other.
Being a robust, closed-source platform that is built to work seamlessly with other AWS services, CloudFormation is considered most suitable in a situation where organizations prefer to run deployments entirely on AWS and achieve full state management from the get-go.
CloudFormation makes it easy to provision AWS infrastructure. Plus, you can more easily take advantage of new AWS services as soon as, or shortly after, they’re launched due to the native support, compliance, and integration between all AWS services. In addition, if you’re working with developers, the YAML language tends to be more familiar, making CloudFormation much easier to use.
In contrast, Terraform is best suited for dynamic workloads residing in multiple deployment environments where you want to control additional systems beyond the cloud. Terraform offers providers specifically for this purpose whereas CloudFormation requires you to wrap them with your own code. Hybrid cloud environments are also better suited for Terraform. This is because it can be used with any cloud (not exclusively AWS) and can, therefore, integrate seamlessly with an array of cloud services from various providers–whereas this is almost impossible to do with CloudFormation.
Furthermore, because it’s open source, Terraform is more agile and extendable, enabling you to create your own resources and providers for various technologies you work with or create.
Your given use case may also benefit from implementing both–for example, with multi-cloud deployments that include AWS paired with other public/private cloud services.
Another example where both may be used in tandem is with serverless architecture. For example at Zesty, we use Serverless which uses CloudFormation under the hood. However, we also use Terraform for infrastructure. Since we work with companies using different clouds, we want to have the ability to use the same technology to deploy infrastructure in multiple cloud providers, which makes Terraform the obvious choice for us. Another unplanned benefit this adds for us is the natural segregation between Dev and Ops. Because Ops tend to be more familiar with the HCL language, it creates barriers that make it more difficult for another team to make a mistake or leave code open to attacks.
In general, many organizations choose to use Terraform for databases and high-level infrastructure and CloudFormation for application deployment. They often do this because it helps to distinguish the work of Dev and Ops. It’s easier for developers to start from scratch with CloudFormation because it runs with YAML or JSON which are formats every developer knows. Terraform, on the other hand, requires you to learn a different syntax. The benefit of creating these boundaries between Dev and Ops is that one team cannot interfere with another team’s work, which makes it harder for human error or attacks to occur.
It’s worth noting that even if you don’t currently use both IaC platforms, it’s ideal to learn the syntax of each in case you wind up using one or the other in the future and need to know how to debug them.
Summary
As we have seen, both CloudFormation and Terraform offer powerful IaC capabilities, but it is important to consider your workload, team composition, and infrastructure needs when selecting your IaC platform.
Because I’m partial to open-source technologies, Terraform is my IaC of choice. It bears the Hashicorp name, which has a great reputation in the industry as well as a large and thriving community that supports it. I love knowing that if I’m not happy with the way something works in Terraform, I can always write code to fix it and then contribute it back to the community. In contrast, because CloudFormation is a closed system, I can’t even see the code, much less change something within it.
Another huge plus for Terraform is that it uses the HCL language which I prefer to work with over JSON/YAML. The reason for this is that HCL is an actual language whereas JSON and YAML are formats. This means when I want to run things programmatically, like running in a loop or adding conditionals, for example, I end up with far more readable and writable code. When code is easier to read, it’s easier to maintain. And since I’m not the only one maintaining this code, it makes everyone’s life easier.
Another reason I prefer to use Terraform is due to our extensive use of public modules, which we needed to leverage prior to CloudFormation’s public registry offering.
While CloudFormation may be quicker to adopt new AWS features and manage the state for you for free, all things considered, I prefer the freedom that comes with open source, making Terraform a better choice for my use case.
Hope you found this comparison helpful!
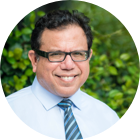
MMS • Edin Kapic
Article originally posted on InfoQ. Visit InfoQ
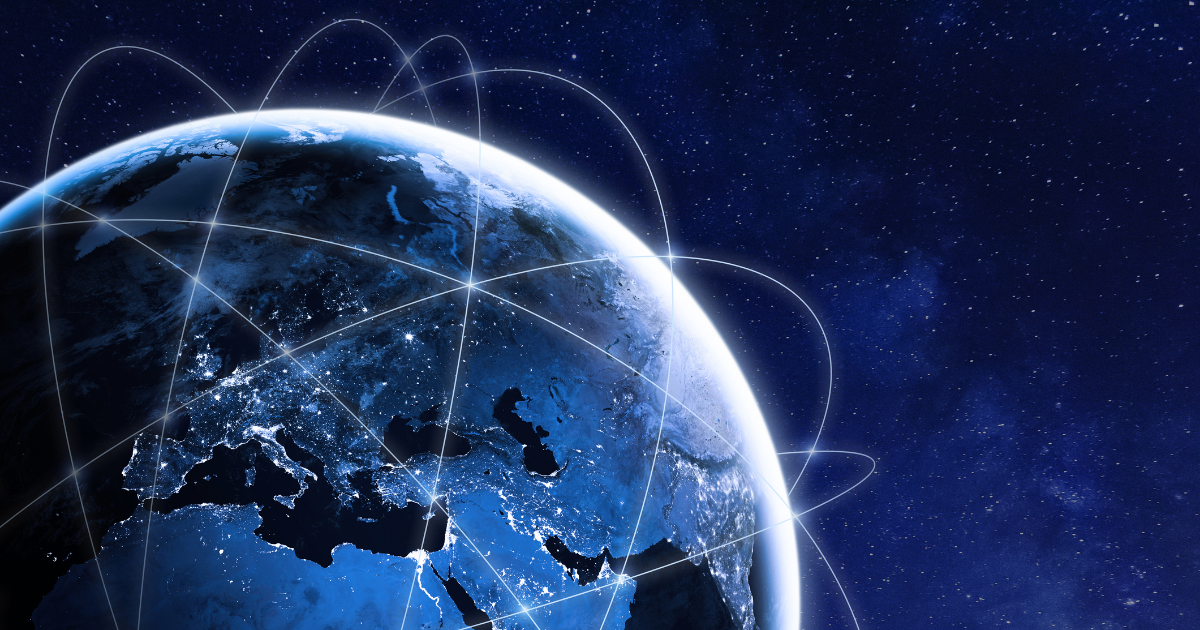
Sam Spencer, Microsoft’s .NET Core team program manager, announced on November 4th 2022 that the Upgrade Assistant .NET tool now includes a preview of an extension that migrates WCF (Windows Communication Foundation) service code from .NET Framework to .NET Standard targeting .NET 6 and later versions. The WCF code is migrated to the CoreWCF library, an open-source port of WCF for .NET Core.
The WCF migration extension for the Upgrade Assistant can convert single ServiceHost
instances. It reads the original configuration file and creates a new one for CoreWCF. It moves the System.ServiceModel
class references to CoreWCF
ones. It migrates the ServiceHost
instance to ASP.NET Core hosting model with the UseServiceModel
extension method.
Windows Communication Foundation (WCF) was launched with .NET Framework 3 in 2006 to unify existing SOAP and RPC communication protocols into a single .NET protocol. The original WCF code usually relies heavily on configuration XML files for setting the endpoints, protocols, and communication attributes.
The migrated CoreWCF code moves those configuration settings into the startup code. The configuration file for CoreWCF is minimal, stating only the correspondence between the service contract and implementation classes.
Sample CalculatorService console application Main method after migration:
using System;
using CoreWCF;
using CoreWCF.Configuration;
using CoreWCF.Description;
using CoreWCF.Security;
// Host the service within console application.
public static async Task Main() {
var builder = WebApplication.CreateBuilder();
// Set up port (previously this was done in configuration,
// but CoreWCF requires it be done in code)
builder.WebHost.UseNetTcp(8090);
builder.WebHost.ConfigureKestrel(options => {
options.ListenAnyIP(8080);
});
// Add CoreWCF services to the ASP.NET Core app's DI container
builder.Services.AddServiceModelServices()
.AddServiceModelConfigurationManagerFile("wcf.config")
.AddServiceModelMetadata()
.AddTransient();
var app = builder.Build();
// Enable getting metadata/wsdl
var serviceMetadataBehavior =
app.Services.GetRequiredService();
serviceMetadataBehavior.HttpGetEnabled = true;
serviceMetadataBehavior.HttpGetUrl = new Uri("http://localhost:8080/CalculatorSample/metadata");
// Configure CoreWCF endpoints in the ASP.NET Core hosts
app.UseServiceModel(serviceBuilder => {
serviceBuilder.AddService(
serviceOptions => {
serviceOptions.DebugBehavior.IncludeExceptionDetailInFaults = true;
});
serviceBuilder.ConfigureServiceHostBase(
serviceHost => {});
});
await app.StartAsync();
Console.WriteLine("The service is ready.");
Console.WriteLine("Press to terminate service.");
Console.WriteLine();
Console.ReadLine();
await app.StopAsync();
}
.NET Upgrade Assistant is an automated command-line tool that migrates .NET Framework project files to the latest versions of .NET. It supports C# and Visual Basic projects and migrates different legacy technologies such as ASP.NET MVC, Windows Forms, Windows Presentation Foundation (WPF), Universal Windows Platform (UWP), and Xamarin.Forms. It can be run in analysis or upgrade mode. However, Microsoft warns that manual steps will still be needed to migrate the application code fully and recommends the users of the tool be familiar with the official .NET porting guidance.
CoreWCF project was officially released in April 2022, although it began already in 2019. Its aim is to provide a subset of the most frequently used functionality from WCF service on the .NET platform. It is .NET Standard 2.0 compatible, allowing it to be migrated in place on .NET Framework 4.6.2 or above. It covers HTTP and TCP transport protocols with mainstream WCF bindings.
CoreWCF is not to be confused with WCF client libraries project, which provides support for consuming WCF services from .NET applications, implementing the client part of the original WCF framework.
The features that aren’t implemented at the moment in CoreWCF are named pipes transport protocol (there are indications that it will be released later), legacy bindings such as WS2007HttpBinding
or NetPeerTcpBinding
, advanced messaging security configuration, and support for queues and distributed transactions. Due to the multi-platform nature of modern .NET, it is understandable that the Windows-specific functionality of WCF is not migrated, except for the Windows Authentication mechanism support.
While Microsoft now supports CoreWCF as part of the general .NET platform, it recommends using modern communication protocols such as gRPC for new projects and leaving CoreWCF with the task of modernising server applications running on .NET Framework that have strong dependencies on WCF and SOAP.
Matt Connew, a member of CoreWCF team, states that the long-term intended goal of CoreWCF is to become the umbrella communication protocol by also incorporating gRPC, protobuf, and other protocol support, by getting rid of SOAP architectural decisions in the library core. It seems to reflect the belief among the .NET developers that WCF promised to be a generic communication framework with different supported protocols. However, it was bogged by the complexity of configuration and tooling support.