Month: August 2022
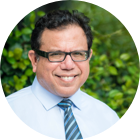
MMS • Sergio De Simone
Article originally posted on InfoQ. Visit InfoQ
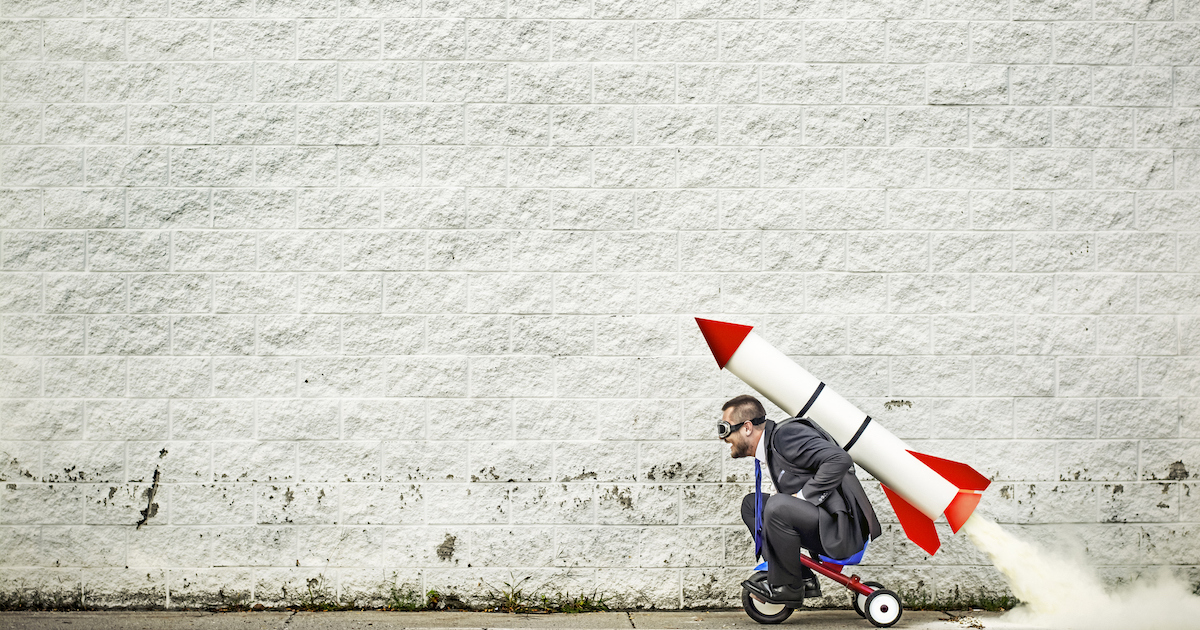
The open source vulnerability scanner Trivy has been recently extended to support cloud security posture management (CSPM) capabilities. While initially available only for AWS, Trivy will soon get support for other cloud providers, says Aqua Security.
Trivy is a scanner for vulnerabilities in Kubernetes images, file systems, and Git repositories. Additionally, it can detect configuration issues and hard-coded secrets. The new feature adds an aws
command to the trivy
CLI which enables scanning live AWS accounts for all the checks defined in the AWS CIS 1.2 benchmarks.
For example, according to the AWS CIS benchmark, a properly configured cloud system should avoid using the root user, ensure multi-factor authentication is enabled for IAM users, make sure that credentials unused for 90 days or longer are disabled, and many more. For each of those checks, a remediation procedure is also defined in the standard.
The first step to use trivy
with a live AWS account is authentication, followed by a scan. You can scan all resources in your default zone, as well as resources in a specific region or select a specific service. Additionally, you can filter scan results based on their severity:
$ aws configure
$ trivy aws
$ trivy aws --region=us-west-1
$ trivy aws --service=s3 --region=eu-east-1
$ trivy aws --severity=MEDIUM
The tool also supports several output formats including text tables, JSON, sarif, cosign-vuln, GitHub, and others. Furthermore, Trivy will cache its result for a configurable amount of time to allow you to inspect them without having to run the scan again.
Previous to the new release, Trivy could be used to scan the static configuration files of an AWS services, but not a live AWS account.
Interestingly, Aqua Security offers a paid CSPM SaaS enabling multi-cloud security posture management across AWS, Azure, Google Cloud, and Oracle Cloud. This solution is based on the open-source tool CloudSploit, which Aqua Security acquired in 2019. While the fact that CloudSploit has not been updated since August 2020 could lead to believe that Aqua Security is sunsetting it in favor of integrating its functionality into Trivy, the company has not disclosed any specifics about the relationship between the two tools.
Cloud security posture management helps organizations discover and remediate security risks, misuse, and misconfigurations in public clouds, with a specific focus on multi-cloud environments. Traced back to Gartner, CSPM is a moniker for a number of distinct approaches and practices to cloud security including risk assessment, incident response, compliance monitoring, configuration monitoring, and others.
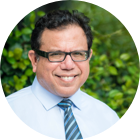
MMS • Juan Fumero
Article originally posted on InfoQ. Visit InfoQ
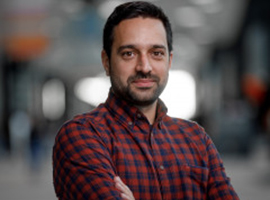
Transcript
Fumero: Heterogeneous devices such as CPUs are present in almost every computing system today. For example, mobile devices contains a multi-core CPU plus an integrated GPU. Laptops usually contains two GPUs, one that is integrated into the main CPU, and one that is dedicated, usually for gaming. Even data centers are also integrating devices such as FPGAs. All of these devices help to increase performance, and run more efficient workloads. Heterogeneous devices are here, and are here to stay. Programmers of current and future computing system need to handle execution on a wide and diverse set of computing devices. However, many of the parallel programming frameworks for these devices are based on C and C++ programming language, and so expanding execution from other the languages such as Java is almost absent. That’s why we introduced TornadoVM. TornadoVM is a high performance computing platform for JVM. Java developers can benefit from execution on GPUs, FPGAs, multi-core, in an automatic manner. I will focus on programmability and how developers can use TornadoVM to accelerate their applications.
Background
I’m Juan Fumero. A research fellow at the University of Manchester. I’m the lead developer and architect of the TornadoVM project.
Outline
I’m going to talk about the following topics. I’m going to introduce some terminology, and I’m going to motivate the project a bit further. Then I’m going to dive in into the programmability. TornadoVM has currently two ways of programming, or two APIs. One is called Loop Parallel API, and the second is called Parallel Kernel API. We will explain each of them and I will show you some performance results. Then, I will explain how Tornado translates from the Java code to the actual parallel hardware, how Tornado maps the application to parallel hardware. Finally, I will show how TornadoVM is being piloted in industry, with some use cases.
Fast Path to GPUs and FPGAs
The question is how to access heterogeneous hardware right now. At the bottom, I show different hardware: CPUs, GPUs, FPGAs. At the top level, I show different high level programming languages. We will stay with Java, but similar situation applies for all the programming languages. If you choose Java, Java is executing on top of a virtual machine, and OpenJDK is an implementation of the virtual machine, but also GraalVM, Corretto, JDK, all of them work in a similar way. Essentially, the application is translated from the Java source code to bytecode. Then the VM executes the bytecode. If the application is executed frequently, the VM can optimize the code by compiling the methods that run frequently into optimized machine code only for CPU. However, if you want to access heterogeneous devices, such as GPUs, or FPGAs, you have to do it through a JNI library or JNI call. Essentially, the programmer has to import a library and make use of that library through a JNI call. In fact, the programmer might have an optimized application for one particular GPU, but if the application of the GPU changes, he might have to redo it again, or might have to retune some parameters. This happens also with different vendors of FPGAs, or different models even of GPUs. There is no full JIT that works in the same way that works for CPU. In the sense that it can frequently execute methods or frequently execute code and get optimized code for that architecture. There is no such thing for heterogeneous devices. That’s where TornadoVM sits. That’s the place of TornadoVM. TornadoVM works in combination with an existing JDK. TornadoVM is a plugin to JDK that allow us to run applications on heterogeneous hardware. That’s what we propose.
Hardware Characteristics and Parallelism
Let’s introduce some terminology I need. There are three different architectures here, one CPU, and a GPU, and an FPGA. Each architecture is optimized for different type of workloads. For example, CPUs are really optimized for low latency applications, while GPUs are really optimized for high throughput. FPGAs is a mixture between them. You can get very low latency and very high throughput. The FPGA, the way it works is that it’s physically wiring your application into hardware. You have exactly the pieces you need to run the application, in hardware. That’s why you can get very low latency. You can get higher throughput just by replicating units. I want to map these architectures to existing type of parallelism. In the literature, you can find three main types of parallelism, task parallelization, data parallelization, and pipeline parallelization. CPUs are quite optimized for task parallelization. Any of these architecture actually can use any type of parallelism. Let’s say that CPUs are quite optimized for task parallelization, meaning that each core can run different tasks. In contrast, GPUs are quite optimized for running data parallelization, meaning that the code you’re going to run is the same, the functions you’re going to run is the same, but take in different inputs. That’s where data parallelization comes from. Then you have FPGAs that are quite suitable for representing pipeline parallelization. In fact, with some instructions, you can enable a destruction level pipeline of instructions. That’s a very good target. Ideally, we want a framework that can express different type of parallelism to maximize performance for each type of device. I will show how TornadoVM does it.
TornadoVM Overview
I’m going to explain TornadoVM. TornadoVM is a plugin to JDK that allows Java developers to execute programs on heterogeneous hardware, fully automatic. It has an optimized JIT compiler, and it’s specialized for different types of hardware. The code that is generated for GPUs is different from the code generated for FPGAs or multi-core. Tornado can run also on multi-core systems. TornadoVM can also perform task migration between architectures, between devices. For example, TornadoVM can run the application on a GPU for a while, and later on migrate the execution without restarting the application to another GPU, or FPGA, while multi-core, back and forth. TornadoVM, the way it’s programmed is fully hardware agnostic. The input application, the source code of the application to be executed on heterogeneous hardware is the same for running on GPUs, CPUs, and FPGAs. TornadoVM can run with multiple JDK vendors, can run with OpenJDK, GraalVM, Red Hat Mandrel, Amazon Corretto, and Windows JDK. It’s open source. It’s available on GitHub. If you want, you can explore on GitHub.
TornadoVM System Stack
Let me show an overview of the system stack of TornadoVM. At the top level, we have an API. This is because TornadoVM exploits parallelism, it doesn’t detect parallelization. Tornado needs a way to identify where the parallel coordinates are located in the source code. That’s done through an API. I have an example of the API here in the slide. Don’t worry about the details, because I will show, step by step, how to build an application for TornadoVM. In a sense, what TornadoVM provides is tasks. Each task is a method. Tornado compiles at the method level, same as JDK, or JVM, compiles from the method level, to efficient code for GPUs and FPGAs. To indicate where the parallelism is, apart from the method level, it also provides some annotations. In fact, Tornado provides two annotations, @Parallel, and @Reduce. Here I show an example with @Parallel.
With Tornado, you can also create a group of methods, which means a group of tasks that are going to be compiled together in one compilation unit, and that’s what we call Task-Schedule. An example of a Task-Schedule is here. We have a Task-Schedule, we give it a name. Then we have a set of tasks. In this case, just one task. You can have as many as you want. Don’t worry about the rest of the details, because we will go through an example. That’s at the API level. Then we have the TornadoVM engine, which takes the input expressions from the bytecode level and automatically generate code for different architectures. Right now, Tornado has two backends, generate code for OpenCL and CUDA. It has two backends. The user can select which one to use, or sometimes Tornado can just pick one best to choose and run there.
Example – Blur Filter
For the rest of the presentation, I’m going to focus on the API level. We will discuss how TornadoVM can be used to accelerate Java applications. I’m going to start with an example, I’m going to use the blur filter. The blur filter is a filter for photography. Essentially, you have a picture and you want to make a blur effect in that picture. All the examples I show in this presentation are available on GitHub, so check out the code, and follow the code along with the explanation here.
Blur Filter Performance
Before going into the details of how it’s programed, I want to show you performance of this application running on a heterogeneous hardware. Here I show four different implementations. The first one, the red using parallel streams, so it’s going to run on CPU, and the implementation is using streams, Java streams. There is no GPU underneath. There is nothing. It’s just Java with parallel streams. The number represent the speed-up against Java sequential, so the higher the better. I run this on my laptop. I have 16 cores on my laptop, and the speed I get is 11.4. It’s quite good. It’s not linear, but quite close. If I run this with Tornado on a multi-core, I can get better speed-up. Still, I’m using 16 cores, but I get 17x performance compared to Java. This is because Tornado generates OpenCL for CPU. OpenCL is very good at vectorizing code. It’s using vector units. Maybe those vector units are not easily accessible, when you compile from Java streams. That’s why you can get a better performance. If we run the application on integrated graphics, we can get up to 19x performance. If we run the application on the GPU I have on my laptop, we can get up to 340x performance. It’s quite high. I now run the image. It’s an image of 5K pixels by 4K pixels. I think it is pretty standard for any camera nowadays. If we compare the speed-ups we get against the parallel version of the Java streams, which is what you can get right now in Java, we can get up to 30 times faster if we run on the GPU.
Blur Filter (Sequential)
How is this implemented? The blur filter has the following pattern. It has two loops to iterate over the x axis of the picture and the y axis of the picture, so x and y coordinates. Then you apply the filter. For the specific of the filter, just check the code online. It’s basically a map operator. For every pixel, I apply a filter, a function. This is data parallelization, essentially, because every pixel can be computed independently of any other pixel. The first thing to do in Tornado is to annotate the code.
Blur Filter – Defining Data Parallelism
As I say, because the pixel can be computed in parallel, what we do is to add annotation @Parallel for these two loops. Meaning that we tell Tornado, these two loops can be fully computed in parallel, and we have two level of parallelization here, to the kernel or to the parallel loop. In fact, this is quite common on GPUs. In contrast with CPU architectures, which we have one level of parallelization, we have 10 threads, 16 threads, or 50 threads. On GPUs, we can define 2D level of parallelization, even 3D level of parallelization. It’s because GPUs are mostly created for rendering graphics, and graphics are image of pixels, x pixels, and y pixels. The first thing to do is to annotate the code. By annotating the code, we define the data parallelization.
Blur Filter – Defining the Task Parallelism
The second thing is to define the tasks. Because this is a picture, we can split the task in three channels. The picture is represented with three channels, RGB: red, blue, green. What we’re going to do is to compute each of the channels in parallel, red channel, green channel, and blue channel. For that, we need to create three tasks. We have a TaskSchedule. It’s an object that Tornado provides. You need to provide a name for the object, a name for the TaskSchedule. In this case, we say blur filter, could be any other name. Then you define which data you want to copy in. Meaning that Tornado is expecting to play with this data, that’s because usually GPUs, CPUs, and FPGAs, they don’t share memory. We need a way to tell Tornado which memory, which regions you want to copy in the device and copy out. That’s done through the StreamIn and the StreamOut.
Then you have a set of tasks, tasks for red filter, tasks for the green channel, and tasks for the blue channel. The tasks are defined as follows. You parse a name, and this is useful because in the terminology, you want to launch task, you can say these tasks run on one device, this task runs on another device. You can refer that by the name. Then the second parameter is a function pointer or method pointer, essentially, the first is the class.method. We want to accelerate the method inside this class called blurFilter. The rest are the normal parameters for the method call. In fact, the signature of the method was 6 parameters, and those are the parameters expressed here as in any other method call. Then we call execute. Once we call execute, it will run in parallel on the device.
How TornadoVM Launches Java Kernels on Parallel Hardware
How TornadoVM selects the threads to run because the Java application is single thread, we just annotate sequential code with parallel annotations. What is happening is the following. When this call executes, so in our case will be filter.execute, it will start optimizing the code. It will compile the code from an intermediate representation. Tornado extends Graal, so the optimization happens in the intermediate representation level. It will optimize the code, and then will translate from the optimized code to efficient PTX or OpenCL code. Then it will call execute. When it call execute, it will launch hundreds or thousands of threads. How does Tornado know how many threads to run? It depends with the input application. Remember our kernel, the blur filter, we have two parallel loops, and each loop iterates over the dimensions of the image. X dimension and y dimension of the image. Tornado gets this information because it compiles at runtime, and create a grid of threads. It’s going to launch 2D grid of threads with the number of pixels in the x axis and the number of pixels in the y axis. Then it will compute the filter. Each pixel will be mapped to one thread.
Enabling Pipeline Parallelism
Let me talk about how Tornado enables pipeline parallelization. We talk about task parallelization, we can define many tasks to run, and data parallelization. Each task is a data parallel problem. TornadoVM can also enable pipeline parallelization. This is done especially on FPGA. When we select an FPGA to run, or when Tornado selects the FPGA to run, it will automatically insert information in the generated code to pipeline instructions. By using this strategy, we can increase performance 2x over the previous parallel code. It’s quite good.
Understanding When to Use this API
Let me explain the pros and cons of using this style of API. As an advantage, this API based on annotations, allows the user to annotate sequential code. The user has to reason about sequential code, provide a sequential implementation, and then think about where to parallelize in the loop. In one way, this is fast for development, because if I have existing Java code, sequential code, I can just add annotations and get a parallel code. This API is very suitable for non-expert users. You don’t need to know GPU compute. You don’t need to know the hardware to run there. It doesn’t require the user to have that knowledge. As a limitation, this API, the loop annotation API, we call it parallel loop API, is limited in the number of patterns to run. We can run the typical map application, which is the filter. For each pixel, you compute a function, that’s the map pattern. Other patterns like scan or complex stencil, is hard to get from this API. Also, this API doesn’t allow the developer to have control over the hardware. It’s totally agnostic. Some developers need that control. Also, if you have an existing OpenCL and CUDA code, and you want to port it to Java, it might be hard. To solve these limitations, we introduce a second API that we call the parallel kernel API. I will explain how the API looks like.
Blur Filter Using the Kernel API
Let’s go back to our previous example, the blur filter. We have two parallel loops that we iterate over the x dimension and y dimension of the image, and compute the filter. We can translate this to our second API. Instead of having two loops, we will have implicit parallelism by introducing a context. The context is a Tornado object that the user can use. That object will give you access to the thread identifier for each dimension. For the x axis, we can get the information through context.globalIdx, and for the y axis, we can get information from the context.globalIdy. Then we compute the filter as usual. This is closer, if you’re familiar with CUDA and OpenCL, with those programming models. In fact, you might think about 2D grid, and then to identify in a unique way a thread, you just need to access to that thread in particular through x position and y position. Then you do your computation as usual.
Tuning the Amount of Threads to Run
How do we know the threads to run in this case? We need something else. Before, remember that Tornado will analyze the expressions at runtime, and will get the threads at runtime. That’s fully transparent for the user. In this case, the threads are not set. The user needs to set up that for us. In this particular example, because it’s a 2D grid, the user can create a 2D grid called worker 2D, and parses the x threads to run in the x dimension, and the y threads to run in the y dimension. Then it will set up the name of the function with that workerGrid. Then when the user calls execute, it will need to parse the grid. That’s the only difference you need to know. Through the Kernel Parallel API, the user, apart from manipulating at the thread level, can access local memory. For example, GPUs have different memories, global memory, local memory, present memory, so the user can program that memory, or can even synchronize a block of threads. It’s very close to what you might find with CUDA and OpenCL.
Strengths of TornadoVM
If we have this API that is coming from CUDA and OpenCL, why do we want to use Java, instead of having the application in OpenCL and PTX, or CUDA and PTX? TornadoVM also has other strengths, for example, live task migration, or code optimization, so we specialize the code depending on architecture. Also, it will run on FPGA. The workflow to run on FPGA is fully transparent, fully integrated with Tornado. Meaning that you can use your favorite IDE, for example IntelliJ, Eclipse, or any other editor, and you can just run on the FPGA if you have it. It can also be deployed easily on Amazon instances, for example, on cloud deployment. You will get that for free by porting that code into Java and TornadoVM.
Performance
I’m going to show some performance results because Tornado can be used for more than just applying filters for photography. In fact, it can be used for other types of applications. For example, for FinTech, or math simulations like the Monte Carlo or Black-Scholes that we see here. It can be used for computer vision applications, for physics simulation, for signal processing, and so on. Here I show a graph. The x axis shows different types of applications, and different implementations for running on the multi-core. The implementation is the same, it is different executions for different devices. The blue represents the multi-core. The green represents the FPGA. The violet, purple one represents the execution on GPU. The bars represent speed-ups. Again, Java sequentials, so the higher the better. As we can see for some applications running on the FPGA, it’s not worth it, so you don’t get any speed-up. For other types of applications like physics simulation, or signal processing, it’s very good at it. You can achieve very high speed-ups, for example, for signal processing, or physics simulation, you can get thousands of speed-ups compared to Java. These results are taken from one publication that we have. If you’re interested, just check out our website. It’s listed there.
TornadoVM Being Piloted in Industry
I’ll show how TornadoVM is being piloted in industry. I show two different use cases here, that we are working on. One with Neurocom Company in Luxembourg. They run natural language processing algorithm. So far what they have achieved is 30x performance by running their hierarchical clustering algorithms on GPUs. We have another use case, in this case, from Spark Works Company, a company based in Ireland. What they do is they have information from IoT devices, they want to post-process that information. They use a very powerful GPU, GPU100, to do the post-processing. They can get up to 460x performance compared to Java, which is quite good.
Remarks & Resources
TornadoVM is open source. It’s available on GitHub. You can download it. You can contribute if you want to. You can make suggestions. We are open to suggestions from the community. In fact, some of the features I have been talking about are coming from the community. We also have Docker images. You can run Tornado with Docker. It’s very easy, assuming you have the driver already installed. It’s just pull and run, essentially. There is a team behind TornadoVM. This is not created by just one person. This is an academic project. We are in academia. We are interested in collaborations, either academic collaborations or industry collaboration.
Takeaways
I have shown that heterogeneous devices are now pretty much in almost every computing system. There is no escape. Programmers of current computing systems as well as future computing system need to handle somehow, with the complexity of having a wide and diverse set of devices, such as GPUs, FPGAs, or any other hardware that is coming. Along with that, I have shown a strategy, a proposal to program those devices through TornadoVM. TornadoVM can be seen as a platform, high performance computing platform for Java and JVM that works in combination with existing JDKs. For example, with OpenJDK. We have discussed an application, for example, the blur filter. I have shown two different ways of implementing a blur filter, one using the parallel loop API that is well suited for non-experts in parallel computing. The parallel Kernel API that is suitable for people or developers that know CUDA and OpenCL already, and want to pour existing code into Tornado.
Why Debugging Is Tricky
Debugging is very tricky, because if anyone has been experimenting with GPU compute, and FPGA, debugging is a very frustrating task. What TornadoVM can do right now is logging information, can give you the compilation time. Meaning Tornado can compile from Java bytecode to OpenCL or PTX. We time those times, meaning from Java bytecode, using Graal compiler to the PTX, and then a second step of compilation is from the PTX or OpenCL via the driver, to actual binary. I can give you that. As well as data transfer time, for example, how much time does it take to send data back and forth? How much actual compute time it takes, along with how many threads Tornado runs on the actual platform, decide what to do with your application. All this information can be enabled with the profile option in Tornado. It’s not fully debugged, so you cannot do this by step by step execution on a GPU, as far as I know. I don’t know if there is any project on that. I think it’s complicated because you usually have thousands of threads running on that platform. What you usually do is to have a small set of threads to run on there, and then debug the application from that point. That’s one step.
The second step is that we can speed the code that Tornado generates. Meaning that I want to see what Tornado generates with OpenCL code, and then I can debug it myself. This is especially useful on FPGAs, because FPGA world is another area. Related to that with the debugging, we also have a debug mode for FPGA. The FPGAs will add a lot of extra overhead in the creation of the application, debugging and run. Usually, compilation on FPGA takes two or three hours of compiling that code. This is because if we generate OpenCL, that’s what OpenCL driver gives us. Tornado can run with the debug option and is fully integrated with the low level tools. For example, if we’re using the Intel FPGA with Intel tools, you can run your debug mode from your IntelliJ editor, for example, or whatever editor you use. Just run debug and you simulate that application on FPGA. It’s not running actually on FPGA, it’s running on your host. It’s a quick try that what Tornado generates can actually run on FPGA. That’s one thing on feedback.
Questions and Answers
Beckwith: Recently, I was looking at an OpenCL stack, and there were some differences that I found out based on the operating system and the enablement that happens, right from the underlying hardware architecture up to the OS. Have you found TornadoVM to generate a different optimized stack depending on the OS and the underlying hardware architecture?
Fumero: In fact, it depends even the driver we use, on the same operating system. I can tell you of an example we had a few months back. What Tornado does inside is a bit complicated. It’s not only about the full JIT, it’s also managing data buffers for you. Essentially, the code that is generated by the architecture is different. One of the things we do for reductions, when you have a reduction it’s a special case when you run in parallel. A reduction means that you have a list of values and you want to reduce all of them to scalar values. This is fully data dependent. To run the next iteration you need to complete the previous one. There’s an algorithm to make this in parallel. To make this in parallel you need to play with workgroups, in the GPU world. Meaning, I split my problem into small problems, and because those block of threads is a subset of the whole iteration space, can share memory, but sharing memory on GPUs is not coherent. You need to insert values. We had this a few months back that we have these reductions working. Then in one platform, Intel, actually, it didn’t work. It was because one of the drivers they put something that Tornado didn’t realize, then the next version we have to [inaudible 00:35:13], then Tornado can pick up and magically reductions continue working again. Yes, even the same platform with the same OS, it’s just different driver implementation. You can get different behaviors. Usually not. Usually, you get the same thing. Actually, we cross-validated between them, so we have a full unit test suite. We run it in different architecture. We run it on Intel integrated graphics, Intel CPU, NVIDIA GPUs. We run a subset on FPGAs as well. We cross-validated that everything is going well.
Beckwith: It’s very smart to build it on top of OpenCL, and CUDA, and everything. I like the idea of cross-validation and unit testing as well.
Fumero: You can have the AoT, so you can run Java code, and then compile the code ahead of time. You can even make your own modifications. If you’re an expert in OpenCL, or CUDA, you can just throw your new modifications, new optimizations, and then pre-compile it and run it in TornadoVM. That’s fully integrated. For TornadoVM, I just save compilation time, and that’s all. On FPGAs, it’s more complicated, because this is long term. Especially, we design this ahead of time because of the FPGA workgroup. The way we usually go for the FPGA is that we first do debugging mode. We try on CPU, on your local host. Then we do the full JIT mode. You have your application. You run it. Then you wait two hours to get your binary. If you’re running on a server that runs on the application for months, or years, within two hours, it’s fine. As soon as the application is ready, Tornado will switch devices. For many users, they want instant performance, so for that, you can plug in your FPGA, your bitstream, which is the configuration file for actual architecture for the FPGA. We can get that binary directly from the OS.
See more presentations with transcripts
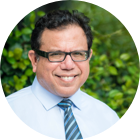
MMS • Steef-Jan Wiggers
Article originally posted on InfoQ. Visit InfoQ
Recently, AWS announced the general availability of a new capability for their Trusted Advisor service with Trusted Advisor Priority, allowing Enterprise Support customers prioritized and context-driven recommendations manually curated by the AWS account team based on their knowledge of the customer’s environment and the machine-generated checks from AWS Services.
AWS Trusted Advisor is a service that continuously analyzes customers’ AWS accounts and provides recommendations to help them follow AWS best practices and Well-Architected guidelines. The service currently implements over 200 checks in five categories: cost optimization, performance, security, fault tolerance, and service limits. Depending on the level of support (AWS Basic Support, AWS Developer Support, AWS Business Support, or AWS Enterprise Support), customers either have access to core security and service limits checks, or access to all checks.
With the Priority capability, Enterprise Support customers have a prioritized view of critical risks, which shows prioritized, contextual recommendations and actionable insights based on the business outcomes. In addition, it also surfaces risks proactively identified by the customers’ AWS account team to alert and address critical cloud risks stemming from deviations from AWS best practices.
The capability was in preview earlier this year. The GA release now includes new features such as allowing delegation of Trusted Advisor Priority admin rights to up to five AWS Organizations member accounts, sending daily or weekly email digests to alternate contacts in the account, and allowing customers to set IAM access policies for Trusted Advisor Priority.
Sébastien Stormacq, a principal developer advocate at Amazon Web Services, explains in an AWS news blog post:
Trusted Advisor uses multiple sources to define the priorities. On one side, it uses signals from other AWS services, such as AWS Compute Optimizer, Amazon GuardDuty, or VPC Flow Logs. On the other side, it uses context manually curated by your AWS account team (Account Manager, Technical Account Manager, Solutions Architect, Customer Solutions Manager, and others) and the knowledge they have about your production accounts, business-critical applications, and critical workloads.
Source: https://aws.amazon.com/blogs/aws/aws-trusted-advisor-new-priority-capability/
Other public cloud providers, such as Microsoft, offer similar services as AWS Trusted Advisor. Microsoft’s Azure Advisor analyzes customers’ configurations and usage telemetry and offers personalized, actionable recommendations to help customers optimize their Azure resources for reliability, security, operational excellence, performance, and cost. The recommendations stem from the Azure Well-Architected Framework.
AWS Trusted Advisor Priority is available in all commercial AWS Regions where Trusted Advisor is available, except the two AWS Regions in China. It is available at no additional cost for Enterprise Support customers.
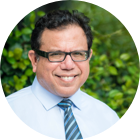
MMS • Sergio De Simone
Article originally posted on InfoQ. Visit InfoQ
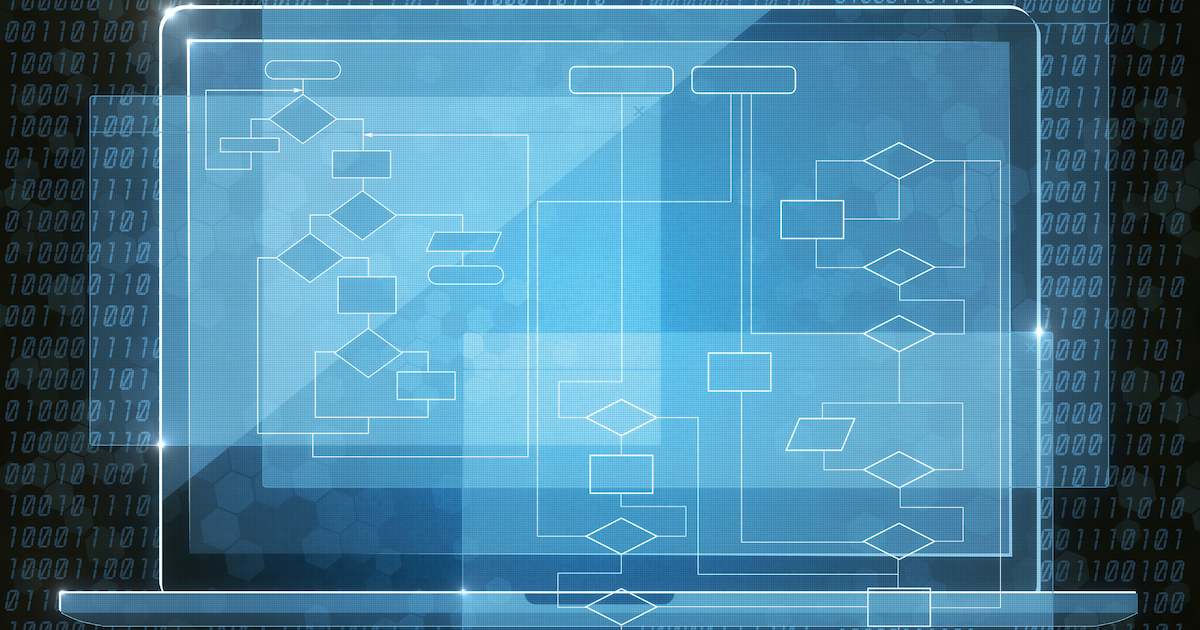
Now available in beta, CameraX 1.2 brings out-of-the-box integration with some of MLKit vision APIs and a new feature aimed to reduce shutter button lag when taking pictures.
To make it easier for developers to use an Android camera with MLKit, Camera 1.2 introduces MlKitAnalyzer
to handle much of the required setup. MLKitAnalyzer
is a wrapper around MLKit detectors which forwards them all the camera frames so they can be processed. At the end of the analysis, it will invoke Consumer.accept(T)
to send on the aggregated results.
The following snippet shows how you can use MLKitAnalyzer
for barcode scanning:
val options = BarcodeScannerOptions.Builder()
.setBarcodeFormats(Barcode.FORMAT_QR_CODE)
.build()
val barcodeScanner = BarcodeScanning.getClient(options)
cameraController.setImageAnalysisAnalyzer(executor,
new MlKitAnalyzer(List.of(barcodeScanner), COORDINATE_SYSTEM_VIEW_REFERENCED,
executor, result -> {
...
});
Besides barcode scanning, CameraX 1.2 supports face detection, text detection, and object detection.
Another new feature in CameraX 1.2 aims to reduce the delay between pressing the shutter button and the actual frame being captured. This feature, dubbed zero-shutter lag, does not actually reduce the intrinsic latency of the device, rather it uses a circular buffer to store the most recent frames captured by the camera. Then, when the user presses the shutter button, it picks the buffered frame with the closest timestamp to the moment when takePicture(OutputFileOptions, Executor, OnImageSavedCallback)
is invoked.
Zero-shutter lag is enabled using CAPTURE_MODE_ZERO_SHOT_LAG
with ImageCapture.Builder.setCaptureMode()
. This feature may not work on all devices, since it requires more memory to store the circular photo buffer. The CameraInfo.isZslSupported()
API can be used to query device capabilities. Additionally, zero-shutter lag cannot be used when capturing video or with vendor extensions implementing special effects.
As a last note, CameraX 1.2 adds a new API to set location metadata for saved videos and includes a number of bug fixes, such as incorrect Exif metadata, crashing when recording video with not microphone available, and others.
CameraX is part of Android Jetpack, a suite of libraries aiming to simplify the creation of Android apps by adopting best practices, reducing boilerplate, and writing code working across Android versions and devices.
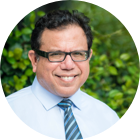
MMS • Steef-Jan Wiggers
Article originally posted on InfoQ. Visit InfoQ
AWSGoat is a vulnerable-by-design infrastructure on AWS featuring the latest released OWASP Top 10 web application security risks (2021) and other misconfiguration based on services such as IAM, S3, API Gateway, Lambda, EC2, and ECS. It mimics real-world infrastructure with additional flaws and uses a black-box approach, including multiple escalation paths.
The INE team developed the AWSGoat project and presented it at the recent Black Hat 2022 conference, and before the OWASP Singapore chapter. The team also developed AzureGoat targeted for Microsoft Azure. Both projects contain the following vulnerabilities and misconfigurations:
- XSS
- SQL Injection
- Insecure Direct Object reference
- Server-Side Request Forgery on App Function Environment
- Sensitive Data Exposure and Password Reset
- Storage Account Misconfigurations
- Identity Misconfigurations
Pentester Academy stated in a tweet:
Written for the infosec community, AWSGoat is a realistic training ground for #AWS exploitation techniques.
To start with AWSGoat, a user needs an AWS account (like AzureGoat, an Azure account) and AWS Access Key with Administrative Privileges. To use the AWSGoat repo, users can fork it, add their AWS Account Credentials to GitHub secrets, and run the Terraform Apply Action. This workflow will deploy the entire infrastructure and output the URL of the hosted application. Alternatively, there is a manual process.
Once installed, users can leverage a module included in the project, which features a serverless blog application utilizing AWS Lambda, S3, API Gateway, and DynamoDB. The module comprises various web application flaws and allows for the exploitation of misconfigured AWS resources. Furthermore, there is a playlist available on YouTube.
Source: https://github.com/ine-labs/AWSGoat
Similarly, the AzureGoat also contains a module featuring a serverless blog application utilizing Azure App Functions, Storage Accounts, CosmosDB, and Azure Automation. Both projects will receive more modules in the future.
In an INE blog post on AWSGoat, Jeswin Mathai, INE’s chief architect (Lab Platform) and one of the contributors, said:
Although at its infancy, the team has ambitious plans for AWSGoat. The next (second) module is already under development and will feature an internal HR Payroll application utilizing the AWS ECS infrastructure. Future additions include defense/mitigation aspects, including Security Engineering, Secure Coding and Monitoring, and Detecting attacks.
In addition, he said:
This will be a massive project in years to come, and it’s open-source, so anyone can contribute too.
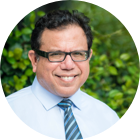
MMS • Ben Linders
Article originally posted on InfoQ. Visit InfoQ
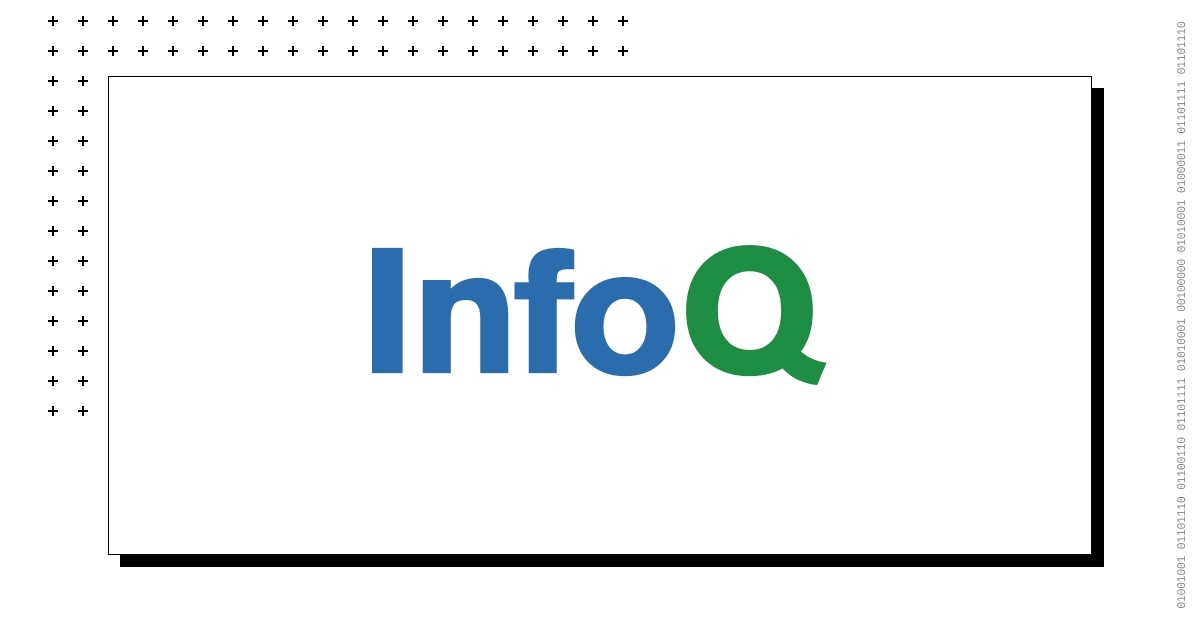
Observability can be programmed and automated with observability as code. A maturity model can be used to measure and improve the adoption of observability as code implementation.
Yury Niño Roa, cloud infrastructure engineer at Google, spoke about programming observability at InfoQ live August 2022.
Niño Roa referred to Thoughworks who in 2019 recommended treating the observability ecosystem configurations as code and adopting infrastructure as code for monitoring and alerting infrastructure:
They motivated us to choose observability products that support configuration through version-controlled code and execution of APIs or commands via infrastructure CD pipelines.
She suggested treating observability as code as a technique for automating the configuration of the observability tools, in a consistent and controlled way, specifically using infrastructure as code and configuration as code.
In her talk Observability is Also Programmed: Observability as Code, Niño Roa presented an Observability as Code Maturity Model that can be used as a way to benchmark and measure its adoption.
The model can be used as a reference for knowing at which level you are:
The model provides criteria to determine the status of an organisation in two axes: sophistication and adoption. For sophistication, it uses four stages: elementary, simple, sophisticated and advanced, and for adoption, there are another four levels: in the shadows, In Investment, In Adoption and in Cultural Expectation.
For example, an organisation is in an advanced stage if it has an automation workflow for observability as code implemented and it’s running on production. The idea is that you identify in which stage you are, reviewing the criteria of each stage and questioning yourself about your implementations and achievements, Niño Roa mentioned.
InfoQ interviewed Yury Niño Roa about observability as code.
InfoQ: How would you define observability?
Yury Niño Roa: Observability is a broad concept whose definition has been controversial between the industry and academy. Some vendors in the industry insist that observability does not have a special meaning, using the term without distinction of telemetry or monitoring. I think the proponents of this definition relegate observability when they use it as another generic term for understanding how the software operates. Monitoring is a part of observability since it allows us to anticipate the system’s health based on the data it generates (logs, metrics, traces).
InfoQ: Why should we do observability as code? What benefits can it bring?
Niño Roa: That is an excellent question since I think the benefits have not been unlocked totally. Some of them include:
- Reducing toiling required for provisioning dashboards for monitoring.
- Having repeatable, replicable and reusable configurations required in the configuration dashboards, alerts and SLOs.
- Documenting and generating context using infrastructure as code to configure monitoring platforms.
- Generally, the teams store the code for observability in repositories, so auditing the history of changes is easier.
- Providing security, because observability as code allows us to have stricter controls while we use continuous integration and deployment.
InfoQ: What are the challenges of observability as code?
Niño Roa: I think they are aligned to the challenges of other practices such as Infrastructure as Code and Configuration as Code. Specifically,
- Reaching a real adoption of engineering teams, leveraging automation wherever possible to accelerate observability delivery across environments.
- Defining clear KPIs to measure the impact of observability-as-code maturity in the organisations.
- Establishing and communicating the current state before implementing new observability-as-code capabilities.
- Documentation is a big challenge in any field, since automating the generation of documentation in an automatic way requires sophisticated techniques such as machine learning and processing of unstructured text.
InfoQ: What’s your advice for starting with observability as code?
Niño Roa: My first advice is that you should know about Infrastructure as Code, specifically about Observability as Code. After that, it is very important to get sponsorship for its implementation. I talk about this in the first stages of the Observability as Code Maturity Model. In these early stages, the organisations have decided to implement Observability as Code, so they have started to collect metrics and officially have practitioners who are dedicating resources to the practice.
Amazon Announces New Capabilities on Local Environments for SageMaker Canvas and Pipelines
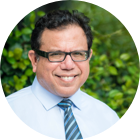
MMS • Daniel Dominguez
Article originally posted on InfoQ. Visit InfoQ
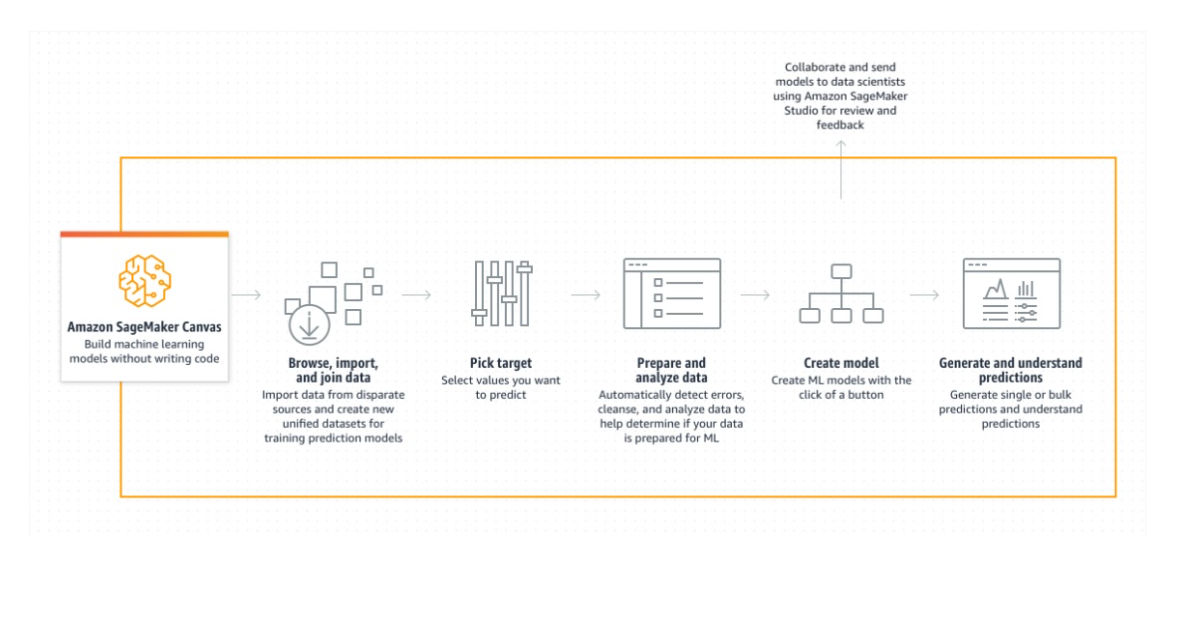
Amazon is announcing multiple capabilities for SageMaker, including expanded capabilities to better prepare and analyze data for machine learning, faster onboarding with automatic data import from local disk in SageMaker Canvas, and the testing of machine learning workflows in local environments for SageMaker Pipelines.
Business analysts can create reliable ML forecasts on their own with SageMaker Canvas, a visual point-and-click interface, without any prior machine learning knowledge or coding knowledge. SageMaker Canvas makes it simple to access and merge data from various sources, clean data automatically, and create ML models to get precise predictions with only a few clicks.
Users of SageMaker Canvas can import data from a number of sources, including local disk, Amazon S3, Amazon Redshift, and Snowflake. As of right now, users can upload datasets from their local disk directly to SageMaker Canvas without consulting their administrators because the necessary permissions are already enabled. When creating a domain, SageMaker can connect a cross-origin resource sharing (CORS) policy to the built-in Amazon S3 bucket for local file uploads by enabling the “Enable Canvas permissions” setting for administrators. If administrators don’t want domain users to upload local files automatically, they can choose to disable this feature. In all AWS areas where SageMaker Canvas is supported, faster onboarding with automatic data onboarding from local disk is now possible.
Additional features for data preparation and analysis in Amazon SageMaker Canvas include the ability to specify multiple sample sizes for datasets as well as the ability to replace missing values and outliers. With just a few clicks, SageMaker Canvas makes it simple to access and mix data from many sources, automatically clean data, and create ML models that produce precise predictions.
Finally using SageMaker Pipelines, you can create machine learning pipelines that directly integrate with SageMaker. SageMaker Pipelines now allows you to build and test pipelines on a local computer. With this release, you may locally check the compliance of your Sagemaker Pipelines scripts and parameters before executing them on SageMaker in the cloud.
The following steps are supported by Sagemaker Pipelines Local Mode: processing, training, transform, model, condition, and fail. You have the freedom to specify different entities in your machine learning workflow thanks to these stages. You may quickly and effectively troubleshoot script and pipeline definition issues by using Pipelines local mode. By upgrading the session, you may easily transition your workflows from local mode to Sagemaker’s managed environment.
The new capabilities add to the range of data preparation capabilities and advanced data transformations supported by Amazon SageMaker.