Month: August 2022
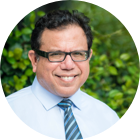
MMS • Jordan Bragg David Van Couvering Dave Copeland
Article originally posted on InfoQ. Visit InfoQ
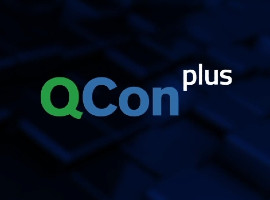
Transcript
Schuster: In this track, we were trying to learn, how can we become a better developer? How can we de-sacrifice ourselves from our terrible state of being? Let’s start off with the sins of our youth. To introduce yourself, I’d like to ask you each, if you could go back, let’s say 20 years in the past, can you tell us what would you tell your junior developer self to start doing or stop doing?
Copeland: I will probably tell myself to be nicer to everyone, and not to feel so empowered and entitled. Because I think a lot of us maybe remember the power of learning how to program, it’s amazing. Sometimes you cannot be so nice. Because getting along with everyone is a really great way to get good software written. I will probably say something like that. I also might say, don’t over-engineer things, so just keep things simple. Something like that.
Schuster: Why were you so mean to people?
Copeland: I was allowed to be. I have a very direct personality. You feel very powerful when you learn how to program, you can make the computer do stuff and other people can’t and they think you’re really smart. Then if you’re really direct and a little bit unkind, they let you get away with it, which is not good. I got smartened up eventually in my career to not do that, and that it was not ok. I wish I had gotten told that way earlier in my career.
Schuster: How would you say that to yourself? Because I can see myself hearing that, “Be nicer, other people are necessary too.” I can see myself saying, “No, I’m a genius. Why would I listen to you, old man?”
Copeland: I would have to frame it as a selfish benefit to my younger self, like you will get further if you’re nicer to other people, and not even nicer, just kinder and respect other people’s positions. I think I could have landed it if I had made it a self-benefit. I was 22 years old, so who knows?
Schuster: Just tell them, you catch more honey badgers with honey than with vinegar.
Van Couvering: I think I used to send too many soapbox emails, saying this all has to change, and we need to do this right away. This is a big mistake. That was fine when I was a junior engineer. When I became a little more senior, it had a real impact on morale. I couldn’t believe what people told me that people care what you say and take it seriously. I’m like, what? Learning how to think about the impact of my words. There’s a quote I have up here that says, “Your speech should pass through four gates. Is it true? Is it kind? Is it necessary? Is it appropriate to time and place?” I try to do that more, and be more thoughtful about the words I use, and also, when to pull the big trigger to bring in leadership to say something needs to be done here. I used to do it way too much.
Schuster: Was that ok when you were a junior, would you say, or was it not ok when you were a junior? I understand that you’re saying as a senior, you have an outside view.
Van Couvering: Even as a junior, I would send those emails to my manager, for example. I always felt safe saying what I felt, that as an engineer we were expected to say what we feel and damn the consequences. Like you said, as I was younger, I was given more permission to be that way. Maybe people just had compassion. I think it was something I needed to learn from the beginning even if it was back in the old days, and all I had was email, we were allowed to do these group emails and start a big Reply All chain, and things like that.
Schuster: Your soapbox messages in hindsight, were some of those warranted? Did you say stop using technology X and you were right? What do you think?
Van Couvering: You can be right and still be wrong. If you don’t bring the group along in the right way, then was it really a success? I was laughing because even recently, I still have this thing, I still have to be careful. I sent an email to the CEO at WeWork that we didn’t have any snacks. That wasn’t really necessary or useful. It’s not enough to be right. What I’ve learned the most is that, yes, it’s about technology but it’s even more about people being in software. That’s what it ultimately comes down to, is that it’s a bunch of people creating something, not just the technology.
Schuster: I’m hearing that being a better developer might actually be about people skills and understanding people, which is annoying, because I like the tech. Maybe Jordan, you can tell us it’s all about the tech. What would you tell your younger self, learn more Rust?
Bragg: I’ll try and learn more languages. If it was technology wise, I think that I wish I spent more time being mentored by people and seeking out people who had a lot more knowledge, when I didn’t at the time. I think I’ve been trying to course correct that over the years. David has actually been a great mentor to me. I think that you’re hungry and this is warranted, David, that CEO email. Outside of that, I think I wanted things perfect too much early on. You waste a lot of time like that. That’d be something I’d probably course correct. One of the things that I think I was fortunate in, was that I started off as actually a tester, not a developer, just because the job market was tough at the time. I feel like that built a lot of those soft skills of empathy and things. I do think it’s highly valuable. I always say that people should spend some time in another role to get empathy for those roles. Yes, I’m still in the soft skill bucket.
Where to Get Training on Personal Skills
Schuster: I do see now that apparently, personal skills are important. Once we’ve sold the personal skills to people, where can people train this stuff? Knowing that lots of developers are introverts, and they just hang out in darkened rooms, poring through IGs. Where can they get these skills?
Copeland: I got some appreciation by, I had this job, and for the first two years we were forbidden from ever talking to a user. The program manager was like, “The users are terrible. You don’t want to waste your time with them.” Then someone above him decided we were going to have a user group and they came in to use the software, and we’re going to watch them. It was amazing, because you could see the pain we were inflicting on these poor people with our software design choices. You can understand what they’re trying to do, and you start to empathize with them. Then that sort of, if I just think about what it’s like to use this software or to do the job, then I can start to empathize and interact with this person, and be a partner to them, and not be adversarial or anything like that. You can learn a lot. I’ve learned so much in that one day of watching people use this piece of software that I didn’t in two years.
Schuster: That’s a really hard one, when actually you write something that’s not a developer tool and you write it for non-developers, and you actually see your friends and family struggle through this thing that you thought this is ingenious, this is a perfect UI. I designed it. Then they just struggle. You feel so embarrassed. It’s, why do I not understand people apparently? You have to talk more to people.
Copeland: It turns out if you talk to them, you can start to figure things out.
Schuster: They’re not just a nuisance on the other end of a credit card. It’s a service role as a developer in a certain way.
Van Couvering: For me, similarly, I did engineering support for three years, when bugs came through, I was on the team that addressed those issues. That was really educational for me. I still get hackles in the back of my neck when someone catches an exception, and then just moves on without logging anything, because so many times I’ve been in this horrible situation where it’s like, what is going on? Three days, four days later, I find out that this exception had been silently swallowed. It really helped me understand that perspective of building code that is maintainable and high quality and has the right observability. I do agree with what both Jordan and Dave said, which is finding ways to put yourself in the shoes of other roles. Like I talked about talking like a suit, trying to put yourself in the shoes of the business person is also really helpful. I think there are also some really good courses on helping you learn how to communicate better, that are often offered by your organization, or LinkedIn Learning, or Udemy, and things like that, that if you’ve realized that maybe this is something that’s hampering you, as a developer, go seek out those courses.
Bragg: In that same world, networking across teams is also pretty important. I feel like spending a lot of time with product people and things and going deep with some of their events and things also helps you get context and empathy. Because I’ve been in multiple places where the engineering and product are so adversarial. They’re as happy as you are to have somebody seeing their view.
Van Couvering: It’s funny, I have to tell this story. I always try to get to be with customers, so I told the people at Castlight I wanted to meet with some of our customers, and we’re at a healthcare company. It turns out, they were just desperate to find anyone to fill in shoes for stuff they were trying to get done, and so I ended up being sent to South Carolina to a turkey slaughtering facility and sit in the cafe, and meet people who needed to sign up for Castlight. It was the strangest, weirdest experience I ever had sitting in this little dimly lit fluorescent cafe with people with all their butcher aprons on coming by. It did teach me that people had trouble logging in, but it was like, I don’t know if that’s the best way for me to learn it. I just always remember going there, like 4:00 in the morning when everyone starts piling in and having to walk through the sanitization pool and sit in the cafe.
Big Force Multipliers for Developers
Schuster: This was looking at the past, basically, let’s move on to maybe a bit more technical stuff. In your current job or maybe your recent jobs, what do you think are the big force multipliers for developers, maybe that you’ve seen happen or that you think will happen? Jordan, you were talking about code reading. Is that a really important thing? Do you see people struggling reading files, taking forever to understand code bases? Is there something new that’s coming or that you can recommend that people can become very efficient?
Bragg: I think what I learned from my talk was that there was not enough tools for code learning and code reviewing. There’s a lot of tools for reviewing commits and things, but not a lot to have readability. I think that it is a common problem. I think, more and more we see people who just do Stack Overflow. I think there’s a name they call those people. Then it’s just like people who it’s too daunting to jump into this code base, and so they have to move fast. I think Stack Overflow was a big help for a lot of people. I don’t know if that’s the end all be all though.
Copeland: The last few years, like all the developer tools for just doing something more expediently, like Terraform makes it so much easier for me who’s not an operations person to get some basic things done quickly. There’s just tons of things like that, that make everybody more efficient, like design systems. One of the apps I work on was internally facing, so it doesn’t need a handcrafted design. I can just use Bulma, which is like Bootstrap. I could use CSS and make a nice looking UI if I wanted to, but I don’t have to, I can use this design system, and it is just way easier and makes it way faster. It eliminates all these things I have to think about. It reduces what I’m thinking about, to like what is a special problem that I’m trying to solve and not all these ancillary, like how much padding does this button need or things that don’t really matter that just need to be done.
GitHub’s Copilot
Van Couvering: I keep reading about how the new GitHub AI tool, Copilot, is actually really impactful, by people who I really trust in the community. Someone said, I wrote the method histogram, or a structure for a histogram, and it automatically generated the add method and the summarize method for me, not just a signature, but all the code for it. I suspect that we’ll see that grow. Getting rid of boilerplate work is always a good thing, and then in some cases is actually writing the code for you.
Bragg: I’d love to see Copilot be a good review tool for you too.
Van Couvering: What do you mean?
Bragg: Meaning like, you could give it your code, and it can optimize your code for you. It’s like, this is poorly formatted, or the names are bad, or whatever, because we’re all great at names. I know I’m pretty terrible at naming things.
Is Copilot Going to Replace Developers?
Schuster: Is Copilot going to end our profession? I hear that’s what you’re saying.
Van Couvering: No. I thought about this, but I see so much of what we have to do are these value judgment decisions about the right way to approach something and think about something and design something. I don’t see how an AI can figure that kind of thing out. We talked about having empathy for the user, understanding the best flows, things like that. I can see it getting rid of the more just banging on a keyboard stuff, just to get something working. I can see it getting rid of that.
Bragg: I could see it getting rid of a lot of the highly technical stuff, and you do more of the soft skills and designing to tell it what to create.
Copeland: I don’t know how to describe this notion, but it’s like you get to a certain level of experience where writing code to solve some problem gets to be straightforward within the scope of some set of problems. Once you’re there, you can start thinking about those higher level things you all were just talking about. For me, a library that did things would be great. If Copilot spits out a bunch of code that does things, that’s cool, that helps make that like, just getting the code written better. I would agree. The real meat of being really, truly effective is thinking about the higher, 1, 2, 3 levels up from that, which I’m sure some AI person can come up with some way they think will solve that wrongly. I don’t think it’s quite yet there.
Coding Automation Tools
Schuster: The thing I wonder about some of the Copilot examples, because I’m not sure if Copilot is just copy pasting existing code. I don’t think it is generating new code. If you Google for, how do I measure the length of a string in C? The first five pages are wrong, because they just tell you to use a strlen. I wonder how much crap is automated, is copied into our code, and how much can we trust this magic, Jiminy the cricket that sits in our IDE and writes our code for us? What countering tools do we need for that? Is it linting? Is it code review? These bugs sitting in our code?
Van Couvering: I think you still need to have code review. Garbage in, garbage out is what you’re saying. All I’m saying is I haven’t used it myself. I don’t know. As people who are using it, who are people I know and respect are saying, this is a game changer for me. They say it is very similar to code completion and the stuff that IDEs came up with 10 years ago, as that step function level of productivity for them. Yes, it could be a virus of bad code spreading everywhere. It’s a good point.
Copeland: Or it could learn how each of us code individually and produce code like we would produce, like amplifying the problems that we have, and making it harder for us to solve our problems by just reinforcing all of us.
How to Maintain Focus as a Developer
Schuster: I love Dave’s comment about making sure you’re focusing on the right problem to solve. Given that you have that clarity, any suggestions for maintaining focus as a developer to stay in flow, so organizational stuff, time management?
Copeland: I do test-driven development, some version of that, which is, I write a test first on some way. I try not to get up or lose focus unless I have a failing test or a passing test. Because then if I forget what’s happening, I can just run the test suite and figure out where I was. Run the test suite, look at Git and figure out, where did I leave things off, if my brain gets completely empty. Then, if I’m not in those states, then I try to just shut out everything and just get to some form of interruptibility. I plan for being interrupted by structuring my work around getting to states where interruption is ok, and trying really hard in between to not do that.
Van Couvering: It’s like running mini sprints in your own head.
Copeland: If I had the test just pass, and then I could look at the code and be like, that’s terrible, but it’s passing, so now I’m in the refactor parts, so now I can make it better, something like that. That gets hard when you got 10 branches going. There’s a limit to how much that will work. That’s the basis for what I would do.
Van Couvering: The thing I often work on is applying lean principles to my own personal backlog. You’re talking about as like doing things incrementally.
The other thing is I really make sure that I haven’t taken on too many things at once. That I don’t have, like you said, seven branches going on at once, I really try to avoid that, and minimize my work in progress. That’s really helped me not feel crazy and have fewer interruptions, fewer meetings, actually, because I’m less involved. I’m involved in fewer initiatives, so I don’t have to be in as many meetings. That’s really helped me is being willing to say no to myself, as it were.
Bragg: I think that time management is like my enemy at all times. It goes by too fast. I try to do a lot of things like the Pomodoro Technique and things like that. Every day look and be like, what am I going to do? What’s my goal? Keep myself honest. Then there’s so many distractions, like identifying whatever things seemingly break you from concentration. To me, it’s Slack, I think drives me crazy. Dedicate time to completely be off there. I think for the Pomodoro thing, it’s like, if you can do 20 minutes dedicated like 3 or 4 times a day, that’s considered a productive day, which is funny. It’s one of those things where, when you’re reading code, you can just get down in like the depth and four hours later you still haven’t written anything, but you just have to keep yourself in check with something to keep you.
Copeland: All these developer tool things come back to this, because if you do need to get distracted to go do something, and you can do that really quickly and expediently without thinking a lot, then you’re losing less context. If it’s super urgent that marketing needs this button on some page, and I just have to stop what I’m doing and go do it, if I can just write one line of code to fix that, then I haven’t emptied my brain too much. If I got to open up some giant CSS file and figure out what is going on and learn everything from scratch, then forget about it.
Server-side IDEs
Schuster: I think that’s one of the interesting developments recently also is things like GitHub Codespaces and all of these server-side IDEs that really make it easy to just jump into a project. Everything’s set up. There’s no 5-hour yak shaving session to get MySQL installed and so on. What do you think of that? I think that’s a big change. Fifteen years ago if you had told me I would be writing code in a browser, I would have told you you’re on something, you had a bit too much Sherry, but it’s here now. I think all GitHub developers work in Codespaces now.
Van Couvering: I haven’t had the opportunity to do that yet. Every now and then, about every two years or so, I see someone demonstrate an in-browser IDE, and I’m like, “Yes, it’s a great idea. Not there yet.” It sounds like we’re there yet. I haven’t had a chance to work with Codespaces. Docker was the same idea, is like everything’s set up for you ahead of time, you don’t need to set it up. I think we’re talking about things that get rid of all the overhead and let you focus on doing your work. All those things can help you become a better developer.
Copeland: I think Codespaces, there is a non-browser way to interact with it because I think the underpinnings of it are some container VM Docker thing. I was talking to someone at Salesforce because they’re working on some write code in the browser thing, too. I was like, why? Who wants that? They’re like, developers that work at a big company where they’re not allowed to install software, can then get VS Code, which is probably better than whatever they’re allowed to install. That absolutely makes sense. I don’t know that that’s the right solution. I get why someone would do that. I think it’s the underpinning of everything is set up, and you can just go and a bunch of decisions are made, and you got to be comfortable with those decisions. If someone’s like, you have to write VS Code in the browser, I would be very unhappy. I suppose over time, I would learn to love it. We all have our preferences about what we want. If your team has 20 different preferences, it’s hard to leverage that to take advantage of consistency.
Schuster: That’s the interesting thing about all these browser based tools using VS Code, because I think VS Code is the fastest growing IDE over the last couple of years. They snuck it in by the backdoor, because VS Code is already the browser. In Electron, it weighs 500 gigabytes. There we are, going to the browser is not much of a difference, which is a move I didn’t see coming. Microsoft is a developer company, it turns out. Who knew?
Bragg: I think they’re trying to put a lot of their tools in the browser. I think that’s a lot of the Microsoft move. One of my previous jobs, we actually had to do all of our development through a VM that we SSH’d into, or remote desktop’d into. As a developer, it can be very frustrating when you’re trying to type and it lags on just creating a method name. I will be curious to see if we’re past that, like it is pretty smooth. I do think that the containers and Docker and stuff made it a lot better, at least for me. You get into this environment where they don’t trust you, but at least they let you have Docker. Then it’s like, I can spin up MySQL, I can spin up all these things without having to beg.
The Qualities of a Bad Developer worth Improving or Removing
Schuster: What are some of the top few qualities of a bad developer that would have the most impact to improve or remove? We’ve already talked about your bad habits, maybe something that you see in others.
Bragg: I think people who just sit in the silo, they just run off and do little. There are some of these projects where you don’t hear from them, and all of a sudden, they have a fully built thing, or they don’t have any incremental releases of anything. You’re waiting two years for something that they told you was a big deal. I think that is like being kind of practicing incremental and not having perfect stuff.
Van Couvering: Yes, the massive PR.
Copeland: We also see the, “That couldn’t possibly happen. I’m having this problem. There’s no way.” It is like, “Did you check x?” “No, but that wouldn’t be it.” There’s some sort of you got to get over this attitude that things are impossible that like a lot more things are possible, and that things are explainable. Literally everything can be explained in the computer that’s like, what’s going on. You just have to get that mindset. It’s just really hard, especially when you’re starting out, to not just go straight into, “That could never happen. I don’t think that would be it.” You should check. Trust me.
The Happy Path Mindset of Designing
Van Couvering: I think another one that came to mind for me is the happy path way of designing. It’s just like, I wrote these few tests, it works. We’re good, and the design is good. I work a lot with junior engineers like, think of all the horrible things that could go wrong here, and how are you planning to address them. You may decide that you’re not going to address them right away, but at least you’ve thought of them and your design is prepared to address them if and when you need to. Especially messaging systems.
Schuster: “Dave, that’s really boring, I want to move on to the next feature.”
Copeland: It is like getting out of the happy path mindset. There is no happy path at all. Let that concept just go. What could possibly happen? Did you handle everything that could happen? If you didn’t, it’s cool, but like you said, if you thought it out, that’s fine. How could my code get interrupted in this one line? Trust me, it will happen.
Bragg: Even when you think it through, you’re still going to be surprised. There’s still going to be things that happen. You’re like, I never thought that would happen.
Embracing Continuous Learning
Schuster: It’s a motivational problem, as a developer, because you just want to finish your code and have the cool stuff work. You don’t want to find the flaws. Is that a job of QA? I always call it the bastard’s mindset. You have to think in some way that makes your own tool break and just say, what if I hit the backspace button like this?
Copeland: If you’re on-call to fix it when it breaks, you’ll change your mindset. That’s not necessarily pleasant, and we can all get in an on-call situation that really sucks. I learned a lot by being on-call for something that there was no one else to ask to fix. I learned a lot about designing things by having to figure it out. Which, I don’t know if that was the right way to teach me that, but I definitely learned.
Schuster: I hear you saying that DevOps is a good thing.
Copeland: Yes.
Van Couvering: Yes. It is frustrating, you feel like you have to know the entire stack up and down from CSS down to Kubernetes, and how the network works and what it means to have a multi-core CPU, and all the different levels of caching. Plus, you have to think like a suit, and so on. Over time, you do have to learn all those things, but you don’t have to learn them in depth.
Copeland: Be willing to learn when needed. You don’t have to know it all when you started but be willing to dig in, growth mindset.
Van Couvering: I think that’s a really great thing that I had to learn is not being afraid of something I don’t know, and being willing to dig in. It’s a really great attitude to pick up, and be less afraid.
Schuster: This developer job sounds like quite a nuisance. It’s a lot of learning, lots of stepping out of your comfort zone, but it is fun.
Chaos Testing
Talking about breaking your own stuff, what do you think about chaos testing, and that sort of thing?
Van Couvering: I always want to do it, I never get to do it. No one’s ever ready for it. That and pair programming. Everyone is like, yes, we should totally do that later.
Bragg: I think we’ve also moved into where developers own their own stuff, and are on PagerDuty call. They don’t want to send chaos monkeys in production, because it’s going to call them in the night. It’s a good technique. I haven’t got to do it yet.
Copeland: Same. It sounds great. It seems like it is advance band. Like you have to have a lot in place even to figure out why is your code breaking. Even if you know how you broke your code to know what actually broke, like you need a lot of other stuff there. Even getting that and understanding that can be really hard. To come back to what we were talking about in the beginning. If you have that, then you can figure out what your software is doing more quickly and feel more effective and feel more in control. That’s good. Getting there is a journey. It’s also an expensive journey.
How to Get Unstuck from a Blocker during Development
Schuster: How soon should the developer ask for help when they struggle with an issue? How important is the early communication of a blocker during a development phase? How do you get yourself unstuck, more or less?
Copeland: It depends on how much experience. This is really hard. Because if I say as soon as you know your blocks, because we’ve all worked with developers who they literally won’t try. The second they had a problem, they’ll go ask. Then you got the opposite, which is like they will never ever ask. It’s really hard. I think as the person who might get asked, you maybe need to be proactive and see what’s going on with them more often. I think never asking is always wrong. You have to be ok asking at some point.
Van Couvering: There’s got to be a way to measure enough is enough. The Pomodoro Technique is nice in a way because if you spend one Pomodoro trying to figure something out, and you still haven’t figured it out, or maybe two, you could gauge it that way. It’s like time box it in some way. It’s like, ok, maybe I’m stupid, fine. Maybe I’m just missing something obvious, but I’m wasting time at this point. I’m thinking about, for me, probably a day. If I was at it for a day and still hadn’t figured it out, then I would probably reach out. Yes, try for 15, 30 minutes before asking for help. It depends on the size of the problem.
Schuster: I always think, I should apply myself more. If I just loaded that problem deeper into my brain some pattern metric will find the solution. If I give up then I’ve given up. Then, on the other hand, there’s always the shower solution. You just get yourself showered, and there it is, there’s the problem. What am I doing? That’s one thing I learned is that usually going for a walk is my shower solution. It’s astonishing. I go for like a 2-hour walk every day, and every day, within 20 minutes, I have to stop and take out a notebook and say, this is solved. Why didn’t I think of this before? It’s a remarkably low-cost solution, you don’t even have to buy a Pomodoro Timer.
Bragg: Walking is good. Actually, that’s a great way that I’ve done in the past, where I get really stuck and I just walk away. It seems like you had a good idea to bring a notepad with you, because for me I get like a mile away, and I’m like, “No, write this down.”
Van Couvering: I still remember telling my son who is programming in Minecraft, it’s like, “You need to take a break,” he’s like, “No.” I was like, “You have to take a break.” It can be so hard.
See more presentations with transcripts
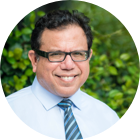
MMS • Erin Schnabel
Article originally posted on InfoQ. Visit InfoQ
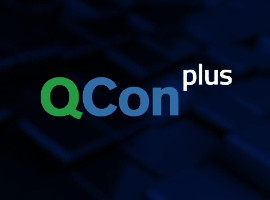
Transcript
Schnabel: This is Erin Schnabel. I’m here to talk to you about metrics.
One of the things that I wanted to talk about in the context of metrics when I talk about metrics, people are starting to use metrics more for SRE purposes when they’re measuring their applications. It’s changed recently, especially in the Java space where we’re used to profiling and we’re used to application servers that run for a long time, and how you measure those. That’s changing a little bit. How we approach measuring applications is changing. That’s part of what I cover in this talk.
I’m from Red Hat. I build ridiculous things. It’s how I learn. It’s how I teach. The application I’m going to use as background for this talk is something called Monster Combat. I started in 2019, I started running a Dungeons & Dragons game for my kids. It just happened that I needed to have a crash course in all of the combat rules for D&D, because I didn’t want to be the one that didn’t know how this was supposed to work. At the same time that I was learning a lot about metrics, and of course being a nerd, I was like, let’s just write an app for that. What this application does, is it takes 250 monsters, and it groups them in groups of 2 to 6, and it makes them fight each other. The general idea is they fight until there’s only one left. Then we see how long that takes. It’s a different kind of measurement. I deliberately went this way when I was trying to investigate metrics, because almost all the time when you see articles or tutorials on using Prometheus, it goes directly into HTTP throughput, which is one concept of performance. It’s one aspect of observability that you want to know when you’re in a distributed system. It’s not the only one. I felt like because everybody goes to throughput, you’re not actually learning what it does, you’re just copying and pasting what’s there. I needed to get into something else, so that it would really force me to understand the concepts.
Observability: Which for What?
This is from a performance perspective, there’s even another leg on here that we’re starting to explore. I include health checks in the notion of observability, as we get to bigger distributed systems, because how automated systems understand if your process is healthy or not, is that’s part of making your individual service observable, so that something else can be able to tell your service is alive, or it’s dead, or whatever. Metrics is what we’re going to talk about, which is one leg. Metrics are the proverbial canary in the coal mine, in a way. You’re not going to do incident diagnostics with them, because as we will explore, what you’re looking at when you’re looking at metrics, you’re always dealing with numbers in the aggregate. You’re way far away from the individual instance. They can be very useful. They can really help you find trends. I will show you in some cases, they helped me identify bugs. They’re useful numbers, you just have to bear in mind what it is that you’re looking at, and what you’re not looking at when you’re working with metrics.
Log entries are where all of your detailed context stuff is. Distributed trace is starting to tie all these pieces together. With distributed tracing, that’s when you have your span. This method calls this method calls this method. Here’s where the user entered the system. Then here’s the chain of things that happened that are all related to that. You can get some really good end-to-end context of how stuff is flowing through your system, using tracing. What we’re starting to see with tracing, if you’ve followed metrics lately, is you’re getting the notion of exemplars that show up in metrics. You’re starting to see span IDs or something showing up in your log entry. You’re starting to get the mythical universal correlation IDs that we wanted back in the 2000s. You’re actually now starting to see it with the advent of a distributed trace. Especially since we are starting now at the gateway to say, “There’s not a span ID here. Let me attach one.” Then we have better semantics for when the parent span is, where child spans are, how you establish and maintain those relationships. Which means you can now leave those breadcrumbs in all of these other pieces, in your metrics and your log entries, which can help you chain everything together. It’s a really good story.
The piece that’s not on here, obviously, if you are used to doing performance is profiling data. Profiling data is a different animal altogether, in terms of how you turn it on, how you gather data, where it goes after that. Interestingly, we are starting to have some conversations in the OpenTelemetry space about whether or not you can push profiling data over the OTLP protocol too, for example. If you had the case where in your cloud, pick your runtime in the cloud, if you had profiling enabled, would you be able to send that profiling data wherever it needs to go? We’re also starting to play with, from a Java application perspective. We’re starting to talk about, can we have libraries like Micrometer, for example, pick some metrics up from JFR (Java Flight Recorder) traces, rather than having to produce it themselves. Can JFR emit data itself and send it along? We’re just at the nascent beginning of how things like that where JFR is profiling data. How we can integrate JFR into these observability systems as we otherwise have them so that we can take advantage of the richer data that the Java flight recorder has, from a performance perspective. It’s not quite what we’re going to be talking about in this talk, but the big picture is there, and how we tie all these things together. It’s an evolving space. If you’re interested in that, just join the OpenTelemetry community. It’s a very open space. They have all their community documents out there. There’s one for each language. If you prefer Golang, there’s a community for you. If you like Java, there’s a community for you.
Time-series Data for Metrics
With metrics, as opposed to older school application performance metrics spaces, we’re working with time-series data. I’m going to use Prometheus mostly in this example, but other observability providers that are working with time-series data, they might have you do the aggregation in different ways. You’re still generally working with the time-series data and with time-series datastores. What we’re talking about there is just a string key, and one numeric value. The trick with this numeric value is how you identify it, how you aggregate it, what you do with it later, but you’re still taking one measurement with one identifier. That’s the general gist. They keep getting appended right to the end of the series. That’s how these series grow. It’s just you keep adding to the end over time. Theoretically speaking, you want these gathered at regular intervals.
The way I think about this is based on my own experience, as a young person. I spent two summers in the quality control office of a manufacturing plant. My dad’s company was a third-tier supplier in the automotive world. I was in the quality control office and I was measuring parts. That was my summer intern job. You would have a lot, basically. It’s like a big bin of raw materials that would be a big bin of parts that would then go to the next supplier in the chain. They would take every 100, every 50, I don’t remember the interval. I just remember measuring a lot of parts. I don’t know how often they were grabbing them from the line. The general gist was fixed cadence every x parts, you pick one off, it goes into me and I measure it. The general premise was, you watch the measurements and the tolerances will start to drift, and you get to a point you’re like, time to change the drill bit or whatever the tool is, because it’s getting worn out. You’re watching the numbers to start seeing where they drift so that you catch when you’re at a tolerance before you start making parts that are bad.
This is the same thing. When you’re thinking about metrics and what they’re for, you’re not talking about diagnostics. What you really want to start getting is that constant stream of data that is feeding into dashboards and alerts, such that you understand the trends and you can maybe start seeing when things are happening because the trend starts going bad before everything goes bananas. That’s the general idea. That’s why you want it at a regular cadence. You want to have a thing that’s very regular, so that when things start getting out of alignment, you can see because the pattern is breaking. If you’re just being sporadic and haphazard about your collection, it’s harder to see when the pattern breaks. That’s the general premise for what we’re trying to do with metrics.
Example – Counter
This is an example of code, uses the Micrometer library in Java. A lot of other ones look very similar. With the application that I’m using for my exploration of metrics, this is Dungeons & Dragons space, so of course there’s dice rolls. This is the most basic metric type as a counter. I’ve got my label, my dice rolls. I have now tags, I’ve got a die. I’ve got a face. They have a value, so like that die which is parsed in as a key is going to be like a d10, d12, d20, whatever the dice is. Then I can see what label I’m going to use, and the label by that is it’s doing zero padding. If I roll a 1, I get a 01. That’s just to make sure the labels are nice and read well. Then I increment that counter.
Dimensions and Cardinality
When we’re looking at the tags and labels, this is where aggregation across dimension starts to be really interesting. You do see this when you see regular Prometheus documentation, when they’re looking at HTTP endpoints, and your throughput traffic, and all that stuff. I wanted to explore that in a different way, so that you could get a better feel for what is actually happening. We talk about dimensions, we have the dice. We have the die that was rolled. We have the face. Because I’m using multiple instances, I’ll show you in a second, we do still have an instance and a job. I could look at dice rolls within one of the instances or across the instances, it’s up to me. This is what we mean by filtered aggregation. I can decide which of those four dimensions, which of those four tags or labels, I think are interesting or important, or that I want to do any kind of view on.
When we talk about cardinality, which is another word you’ll hear often, we’re talking about every time you have a unique combination of those labels, you get effectively a new time series, a new series of measurements that have that exact matching combination of labels. We might also hear the word cardinality explosion. That happens when you create a tag or a label that has an unbounded set as a value, usually, because someone made a mistake with tracking HTTP requests, for example, and in the path, they used a user ID. That’s the most common example because you know the user ID is unique all the time, or they use the order ID and that shows up in the label. That means you have like 80 bajillion unique entries. It is way too much data, your data explodes, they say cardinality explosion, and that’s where that term comes from.
Here’s my dice rolls total. I’m playing a bit of a baker’s game in the kitchen. I have six servers running. One that’s Spring. One that’s Quarkus with Micrometer. One that’s Quarkus with Micrometer in native mode. One that’s Quarkus with MP Metrics, and one that’s working with MP Metrics in native mode. I’ve got five servers running. They’re all collecting data, you can see in the job. The instance and the job is going to be the same, I don’t have multiple instances of the same server. I only have one of each. The instance and the job will always be the same. You can see here’s all the d10s, here’s the d12s. You can see the face. That’s the kind of data that it looks like. I’m getting my single number measurement afterwards.
If I look at that as a dashboard, we try to make a dashboard for this, and I just put in dice rolls total. This is Grafana. It’s going to tell me some helpful things firstly, theoretically speaking. There we go. This is the last six hours because again, this has been running. You see this counter, it’s a mess. I’m not getting anything useful out of it. You can see it’s an incrementing value forever. Grafana is going to help you say, yes, this is the counter, you should be looking at a rate. We know counters always go up. In the case of Prometheus counters specifically, it will adjust for, like if I was going to go take one of my servers and reset it, that could chunk, that counter resets to zero. If I was relying on this graph, you would see it as a big chunk in the data. Prometheus understands with its rate function, how to account for that, so we can do a rate. Grafana further has a special interval that it can use for rate, where it’s saying, I’ll do the interval that’s also smart, because I know about the interval that Grafana is using to scrape the Prometheus metric. I will take that scrape interval into account when I’m calculating a rate interval. That one’s nice. This should flatten out our data, theoretically speaking. Maybe I should make it think about it less. There we go.
I think it’s thinking too hard because it’s trying to chug six hours’ worth of data, let’s go back to just one hour. You can see this is still like real chaos. What am I even looking at? I don’t even understand. We have tags. This is where we start to aggregate across dimensions. How can we take this massive data and make some sense out of it? There’s a couple things we could do that we could find interesting. We’re within Dungeons & Dragons space here. I can take a sum of these counters. I can take the sum by die. This shows me the comparative, like a frequency of rolls across the different dice types. There’s an interesting thing we can do, because in some cases these cumulative values for this particular use aren’t necessarily valuable. We can flip this view to, instead of showing time, left to right, we can say, just show me the summation of the series right here. We can see, here’s our dice types. You can tell at a glance now that d20s are used way more often than d4s, which is expected. When I was learning, it was like, I didn’t know. It gave me a feel for what was going on. D20s are used for every tag, for every save, for everything. It’s not really particularly surprising. D12s and d4s are probably the rarest used, obviously. The rest are d8s, d6s, d10s are more standard damage types. That’s what we can learn from that.
Because these are dice rolls, the other thing we might want to know is, are we getting an even distribution of rolls? Have I done something wrong with my random function that’s trying to emulate a nice even probability of values? If we want to look at that, for example, I might pin the die, and then sum that by the face. Here, I can say, for d10, here’s what all the faces look like that I’m rolling. You’re like, they’re all over the place. This is when Grafana does a kind of persnickety thing. It’s taking that y-axis, and it’s changing the scale on you. If we set the Y-Min as 0, we can see that these are actually quite even. It’s a nice distribution across just in the last hour, right across all of the different numbers. When we take that min off, it really tries to highlight the difference. It looks big, but if you look at that scale over on the left, it’s not very big. We can tell, our dice rolls are behaving the way we expect. That’s amazing.
You May Find More than Just Numeric Trends
When I first wrote this, I’m like, “That’s not interesting. Why are you doing that?” When I was first writing this application, I actually did something wrong, when I was just setting up all the dice rollers, and I forgot to do something with the d12. It was when I was producing this kind of dashboard that I was like, I really messed that up. I didn’t get any of the numbers. It helped me find a bug, because I had tested a bunch of things, I just hadn’t tested that thing, and it fell through the cracks. I found it because I was setting up dashboards trying to count to make sure that my dice were rolling evenly. Useful.
Attacks: Hits and Misses
When I was writing this application, part of what I was trying to do was learn the combat rules for Dungeons & Dragons. That involves different kinds of hits. You have a hit against armor class, you have a hit against difficulty class. How the rolls and damage and stuff is calculated is a little bit different. There’s special things that happen when you roll a 20 and when you roll a 1, which is also interesting to know. In this little example, I have two standard misses in here, two standard hits. I have a critical miss which is a 1, and I have a critical hit which is a 20, when you roll a 20. Then that damage is doubled when you roll a 20.
Micrometer: Timer and Distribution Summary
To measure this thing, again, when I was trying to do this, I was trying to just get a better understanding of what an average battle would be like between monsters when I didn’t know what I was doing, so that I would have some idea of what normal was. Because player characters they have all kinds of other special abilities that makes what they can do in combat more interesting. I just wanted to have a feeling for what combat should be like usually. I created this measurement where I’m looking at the attacks per round. Then I’m measuring, was it a hit or a miss? You could see there’s a little more than just hit or miss. There’s, is it a hit, or is it a miss, or is it a critical hit, or is it a critical miss? Then there’s an attack type. That’s if it’s that armor class based attack or a difficulty class based attack. A good example of a difficulty class based attack is a dragon that just breathes poison breath everywhere, it’s then up to the opponents to not be poisoned. That’s the difference. Then there’s damage types, like poison, or bludgeoning, or slashing, or fire, or cold, and they all do different kinds of damage at different levels. It was all stuff I was trying to learn. Then I record the damage amount at the end, so I could do some amount of comparison about how much damage different kinds of attacks do, so I could get a better feel for how combat was going to work.
In the case of Micrometer, it provides me a distribution summary, which basically just gives me a count and a sum and a max. It’s not just a counter, I also have the sum, and then I have this max value within a sliding time window. The max was not particularly useful for me, you see that more often in your normal web traffic measurement where you’re trying to find the outlier, this request took x amount of seconds. You’re still within this sliding window, but Prometheus would record it. You’d be able to see, something happened and there was huge latency in this window, we should probably go look and follow the downstream breadcrumbs and figure out what happened.
If we go back now to our dashboard, I have some definitely going to the oven for this one. Here’s the finished duck, I’m going to pull out of the oven for you, because I’m going to look at a couple of these. The attack dashboard. Let’s look at this. I still have my dice rolls. I have that nicely pulled out, that was the final dashboard of all of my dice. I’m taking the hit and the miss. We’re just looking at hit or miss. This one is not particularly interesting, although you should be able to see that a hit is the most common. The normal armor class style attack that misses is the next common. Critical hits and critical misses are less than that. You can see the difficulty class hits just don’t happen very often. It’s important to understand that relative infrequency, because as we got to see some other graphs, you’ll be like, but this graph is really choppy. It’s because it doesn’t happen that much. You have to realize that almost all observability systems will do some approximation, they’ll do some smoothing of your graph. When you have a real sparse data source, like these DC attacks, you might see some things in your graph. You just have to be aware that that’s what’s happening, that there’s some smoothing activity happening.
When you’re looking at these attack types, this was something that I wanted to understand, how much damage am I getting from hits or misses? Within this graph, for example, you can see an armor class, an AC style hit. The average is around 12. It’s about normal. Happens most often. It’s a nice steady even line. Remember, critical hit, the damage is doubled. That’s your green line. That looks about right, it’s about doubled. It depends on which one got doubled, and it doesn’t happen all the time. You’ve got some smoothing, it’s not exactly double, but it’s about a double. That makes sense. Everything makes sense. The difficulty class based attacks, I had some aha moments that I learned while making all this stuff. You’ll notice the red line is really all over the place. The blue line is really all over the place. The thing with difficulty class attacks is if the dragon breathes poison breath everywhere, it does bajillion d8 tours of damage. It’s up to then the opponents to roll to save. If they save, then they take half of the damage. Which means out of the gate, that red line is these DC style attacks. They’re critical hits out of the gate, basically. Then it’s up to the opponent to save it to make it a normal hit. The level of creature and all that stuff, like the values for DCs are all over the place, and that’s exactly what this data is showing you. They’re infrequent, so there’s graph smoothing. They’re just a lot less consistent value. You also still see generally the same relationship where the red line is, roughly, if you really wanted to smooth that curve, it is roughly double where that darker blue line is. That makes sense.
The other thing you’ll see with the average damage by attack type is that these DC based attacks, on average, do a lot more damage than your normal melee weapon style, let me hit you with my hammer, Warhammer attack, which also makes sense. That’s why when someone says, you have to save for a DC, you’re always rolling way more dice. It’s way more fun, way more dangerous. That’s all from that one measurement. I’m looking at this measurement, I’ve taken now hit or miss, and the attack type. I’ve analyzed them to pieces, but I’m just still using that one damage amount measuring point. I’m just re-splicing these dimensions to learn different things. The other thing we can start looking at is the type of damage. This again, you start getting into really sparse data, and you get into more approximations of what’s going on. What’s interesting to me here, and this is consistent across the board, poison and lightning will wreck you every time. Don’t neglect your constitution score because poison is awful. Slashing, piercing, bludgeoning relatively low amounts of damage, but they are the most frequently used. Poison and lightning, they will kick your butt.
Then if I scroll down, I can pull out more. If I have an eighth just a poison or a lightning attack against armor class, you can see these are sparse datasets, so there are some gaps. They’re pretty low when you have these DC based attacks, and even lightning usually does start pulling ahead on the DC based attacks. That’s just within the last 30 minutes. If I take it with the last six hours, you still see like super choppy. It just depends on which monster is fighting which monster, how high those values are. You can see, it’s still lightning and poison are up there at the top. Everybody else is down below. It’s consistent. When I make my Dungeons & Dragons characters now I do not neglect my constitution score. That was what I learned.
Other Discoveries
“Erin, what does this have to do with my application? I am not writing Dungeons & Dragons. What am I learning about this?” Here’s another case where I was writing the application, late nights with always extra credit assignment. When I was just writing metrics, of course, I’m having like 250 to 500 monsters fighting each other, I’m not going to write unit tests to cover all possible permutations of combat options where some have the armor class attacks, and some have difficulty class attacks. I caught errors in my code, because I was looking at this from an aggregated point of view. I was just letting, just like I am now. These guys are just battling the crap out of each other in the background, and I’m just looking at the result. I found a critical saved hit. It should not have ever happened when I added the difficulty class style, when I added handling for these DC based attacks, I just did something wrong. That ended up being like 3:00 in the morning, Erin wrote that code. We’re just going to try that again.
In the other case with misses, I realized, I’m like, you should not be having more critical hits than you have normal misses, that’s wrong. That one actually was sneaky, because I didn’t write any code wrong in all of the battle code that I would have tested, but that was all fine. It took me a long time to figure out that this was actually a data entry problem. When I was interpreting, like reading in the statistics for all of these monsters and reading in all their stuff I had missed a plus in the regular expression for their armor class, and so everybody had an armor class of 9, which meant they were hitting all the time. You get other benefits from turning on metrics, which is not related to performance, but it’s still useful. One of the things I have seen a lot of people say is that when they turn on metrics for their application, they find blatant bugs that they didn’t know that they had.
Questions and Answers
Printezis: Do you have a version of it using Warhammer instead of Dungeons & Dragons?
Schnabel: No, I haven’t played Warhammer yet. I should. This has inadvertently taken over my life. I only wanted to do it to do something with my kids, my son and his friends. I didn’t want them to lose the ability to pretend. I was like, I’m going to do this thing. I’m going to be this great mom. Now it’s like, I’m watching Critical Role, I got like a library this big. Metrics are hard, because I work on Micrometer now. I watch the forum in Slack and stuff, and people come in, and the hardest part people have is they want to measure precise numbers. People don’t get the smoothing part. You’re always dealing with a rate, which is already several measurements smooshed into a measurement across a sliding time window, so like, but I need to know the exact. It’s like, you get the alert and you can go look somewhere else.
Printezis: That’s challenging, though. Metrics are helpful, but then you need to have some log entries or something that you need to go and look at details. I don’t know how you find that tradeoff about what you store where?
Schnabel: Tradeoffs with data storage, and how long?
Printezis: Exactly.
Schnabel: The nice thing about metrics is there is a very consolidated datastore. If you get your tags and labels right, you can take that one measurement, and really go to town trying to understand all of the meanings. Depending on the application that you’re writing, there’s so much more you can do with metrics than just throughput and memory usage, which is what most of the examples show. I really wanted to be like, “No.” “Yes, ok, you need that. Sure.” You can do really cool other things to make sure your application is actually doing what it’s supposed to be doing and is actually meeting the requirements. Why are you writing this app to begin with? Because you want users to choose their favorite color. How many users are choosing chartreuse? We have too many colorblind people on here. Nobody is picking this color. Something’s wrong. I feel like people are still wrapped around what you can do with the metrics. It’s fun.
If you start using metrics and you want to come find, the Micrometer has a Slack workspace, you can come join and ask questions. OpenTelemetry has a project, to your point earlier about logs and metrics and traces and all the datastore and all this stuff. OpenTelemetry is really trying to solve the problem of how you collect all that stuff. The OTLP protocol, how the collectors work, where all the data goes, whether or not you can just have all of your services emitting that information. Then have the collectors sort and sift it and send it to all of the places that it needs to go, so that you can manage that collection retention policy stuff, is really great.
Printezis: One more observation, so you did mention that metrics typically have time as the primary axis. Have you seen any cases where that’s not the case? I have definitely needed a few times to correlate to different numbers instead of time and the metric.
Schnabel: When you’re collecting metrics, the point of that time-series data is that it is over time. That’s what gives you the two points. When you’re looking across spans, there’s other things that you can do once you get into tracing. That’s part of why OpenTelemetry is trying to figure out how to bring tracing and metrics closer together so that you have some tracing data in your span, or metrics data in your span. Like, this span took x long, and this span took x long. Then when you’re trying to look across the span, or between two spans, you can start to understand why they may be performed differently or behave differently, because there’s a lot more information in the span. The spans have a lot more context anyway. If you have the measurements also there, then you can start doing a lot more correlation between them.
Printezis: What advice can you give to a company that use log Splunk instead of proper metrics. This can give me some good space to use to argue for pervasive metrics? My opinion would be, you have to use both. They’re actually useful for different reasons.
Schnabel: They’re useful together. Splunk is very active in OpenTelemetry also. Splunk is getting to the perspective, they’re taking the view where they just are the dragnet and collect everything, and you post process all that stuff later. If you have the ability, because you’re running in Kubernetes, for example, to set up metrics and to have Prometheus, if you have Splunk, then later you can keep the amount of data that you’re collecting in Prometheus a lot thinner, so you can still see. You have to have this attitude that the metrics are just your early warning, they’re just your early live signal. Then you still fall back to Splunk. Splunk is absolutely essential. You’re saying I want a little bit more early warning of what’s going on in my system, and that’s where metrics can be useful. If you instrument what your application is doing, you do catch stupid things, like bugs that you didn’t think, behaviors that are not supposed to be happening.
See more presentations with transcripts
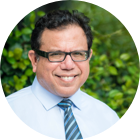
MMS • Steef-Jan Wiggers
Article originally posted on InfoQ. Visit InfoQ
Recently, Microsoft announced its Azure Fluid Relay service’s general availability (GA). This managed cloud offering enables web developers to use the Fluid Framework for building enterprise-grade collaborative applications.
Azure Fuild Relay service is built upon WebSockets, a full-duplex communication channel supported by modern web browsers. The innovation in Fluid Framework announced in 2019 at Microsoft’s Build developer conference is that it supports distributed and synchronized shared state – so that creating a collaborative application is simplified. The only supported language currently for the Fluid Framework is TypeScript or JavaScript.
Developers can set up and run their applications with Azure Fluid Relay by provisioning the Azure Fluid Relay service in their Azure account and configuring the azure-client SDK package to connect their applications to the Azure Fluid Relay instance.
The azure-client package has an AzureClient class that allows developers to interact with Fluid. The class contains the logic of connecting the Fluid container to the service. In addition, developers need to update their app’s configuration to connect to the Azure service with the correct configuration values.
import { AzureClient, AzureConnectionConfig } from "@fluidframework/azure-client";
const clientProps = {
connection: {
type: "remote",
tenantId: "YOUR-TENANT-ID-HERE",
tokenProvider: new AzureFunctionTokenProvider(
"AZURE-FUNCTION-URL"+"/api/GetAzureToken",
{ userId: "test-user",userName: "Test User" }
),
endpoint: "ENTER-SERVICE-DISCOVERY-URL-HERE",
},
};
const azureClient = new AzureClient(clientProps);
Note that Microsoft also offers WebSocket-based services with Azure Web PubSub, a fully-managed service that supports native and serverless WebSockets and Azure SignalR, which allows developers to add real-time web functionality to applications. The latter is not designed to abstract distributed state in the same way as with Azure Fluid Relay. Furthermore, with Azure Web PubSub, similar to SignalR, without client or protocol requirements developers can bring their own WebSocket library.
In a Microsoft Developer blog post, Mathangi Chakrapani and Pranshu Kumar, both senior product marketing managers at Microsoft, wrote:
The service enables developers to build and deploy enterprise-ready collaborative applications that perform at industry-leading speed and scale. Azure Fluid Relay takes care of all details of Fluid collaboration while you as developers focus your attention on your app and end-user experience.
Currently, the Azure Fluid Relay service is available in around 10 Azure regions – half of which are in the US – on a pay-as-you-go basis. More details on pricing are available on the pricing page. In addition, developers can find more information and guidance through the documentation landing page.
Amazon Comprehend Announces the Reduction of the Minimum Requirements for Entity Recognition
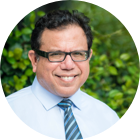
MMS • Daniel Dominguez
Article originally posted on InfoQ. Visit InfoQ
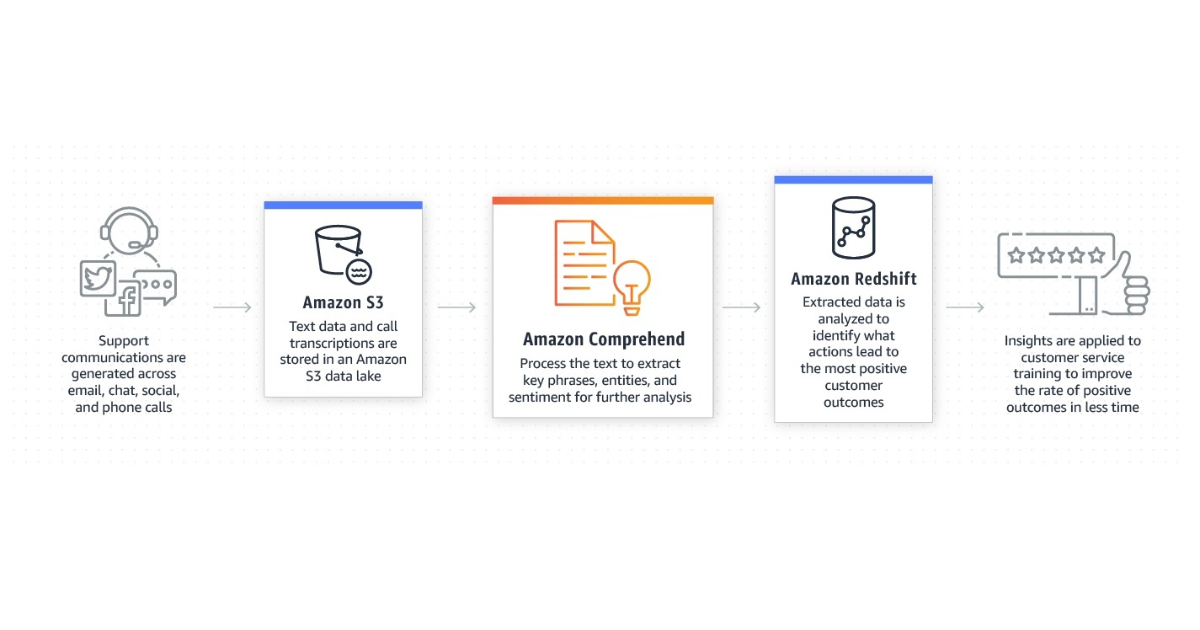
Amazon is announcing that they lowered the minimal requirements for training a recognizer with plain text CSV annotation files as a result of recent advances in the models powering Amazon Comprehend. Now, you just need three documents and 25 annotations for each entity type to create a unique entity recognition model.
You can use Amazon Comprehend, an NLP service, to automatically extract entities, key phrases, language, sentiments, and other information from documents. For instance, using the Amazon Comprehend console, AWS Command Line Interface, or Amazon Comprehend APIs, you may start detecting entities right away, such as persons, places, commercial products, dates, and quantities.
Additionally, you can build a custom entity recognition model if you need to extract entities that aren’t included in the built-in entity types of Amazon Comprehend. This will enable you to extract terms that are more pertinent to your particular use case, such as product names from a product catalog, domain-specific identifiers, and so forth.
Creating an accurate entity recognizer on your own using machine learning libraries and frameworks can be a complex and time-consuming process. Amazon Comprehend simplifies your model training work significantly. All you need to do is load your dataset of documents and annotations, and use the Amazon Comprehend console, AWS CLI, or APIs to create the model.
You can send Amazon Comprehend training data in the form of entity lists or annotations to train a custom entity recognizer. In the first scenario, you offer a set of documents together with a file that has annotations indicating the locations of entities inside the set of documents. As an alternative, entity lists allow you to supply a list of entities together with a label designating the entity type for each one, as well as a collection of unannotated documents that you anticipate containing your entities. Both methods can be used to successfully train a bespoke entity recognition model, however in some cases one can be preferable.
Up until now, you needed to provide a collection of at least 250 documents and a minimum of 100 annotations for each entity type in order to begin training an Amazon Comprehend custom entity recognizer. Amazon is revealing that the minimal requirements for training a recognizer with plain text CSV annotation files have been lowered as a result of recent enhancements to the models underpinning Amazon Comprehend. With as few as three documents and 25 annotations for each entity type, you can now create a unique entity recognition model.
Given that both the quality and quantity of annotations have an effect on the resulting entity recognition model, annotating documents can take a significant amount of time and effort. Poor outcomes may stem from insufficient or inaccurate annotations. Tools like Amazon SageMaker Ground Truth, can be used to annotate documents more rapidly and create an augmented manifest annotations file, to assist in setting up a procedure for collecting annotations.
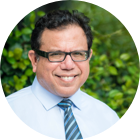
MMS • Steef-Jan Wiggers
Article originally posted on InfoQ. Visit InfoQ
Recently, Google announced the general availability of Bigtable federated queries with BigQuery allowing customers to query data residing in Bigtable via BigQuery faster. Moreover, the querying is without moving or copying the data in all Google Cloud regions with increased federated query concurrency limits, closing the longstanding gap between operational data and analytics, according to the company.
BigQuery is Google Cloud’s serverless, multi-cloud data warehouse that simplifies analytics by bringing data from various sources together – and Cloud Bigtable is Google Cloud’s fully-managed, NoSQL database for time-sensitive transactional and analytical workloads. The latter is suitable for multiple use cases such as real-time fraud detection, recommendations, personalization, and time series.
Previously, customers had to use ETL tools such as Dataflow or self-developed Python tools to copy data from Bigtable into BigQuery; however, now, they can query data directly with BigQuery SQL. The federated queries BigQuery can access data stored in Bigtable.
To query Bigtable data, users can create an external table for a Cloud Bigtable data source by providing the Cloud Bigtable URI – which can be obtained through the Cloud Bigtable console. The URI contains the following:
- project_id is the project containing the Cloud Bigtable instance
- instance_id is the Cloud Bigtable instance ID
- (Optional) app_profile is the app profile ID to use
- table_name is the name of the table for querying
Once the external table is created, users can query Bigtable like any other table in BigQuery. In addition, users can also take advantage of BigQuery features like JDBC/ODBC drivers and connectors for popular Business Intelligence and data visualization tools such as Data Studio, Looker, and Tableau, in addition to AutoML tables for training machine learning models and BigQuery’s Spark connector to load data into their model development environments.
A big data enthusiast Christian Laurer explains in a medium article the benefit of the new approach with Bigtable’s federated queries:
Using the new approach, you can overcome some shortcomings of the traditional ETL approach. Such as:
• More data freshness (up-to-date insights for your business, no hours or even days old data)
• Not paying twice for the storage of the same data (customers normally store Terabytes or even more in Bigtable)
• Less monitoring and maintaining of the ETL pipeline
Lastly, more details on Bigtable’s federated queries with BigQuery are available on the documentation page. Furthermore, Querying data in Cloud Bigtable is available in all supported Cloud Bigtable zones.
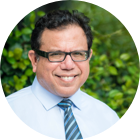
MMS • Sergio De Simone
Article originally posted on InfoQ. Visit InfoQ
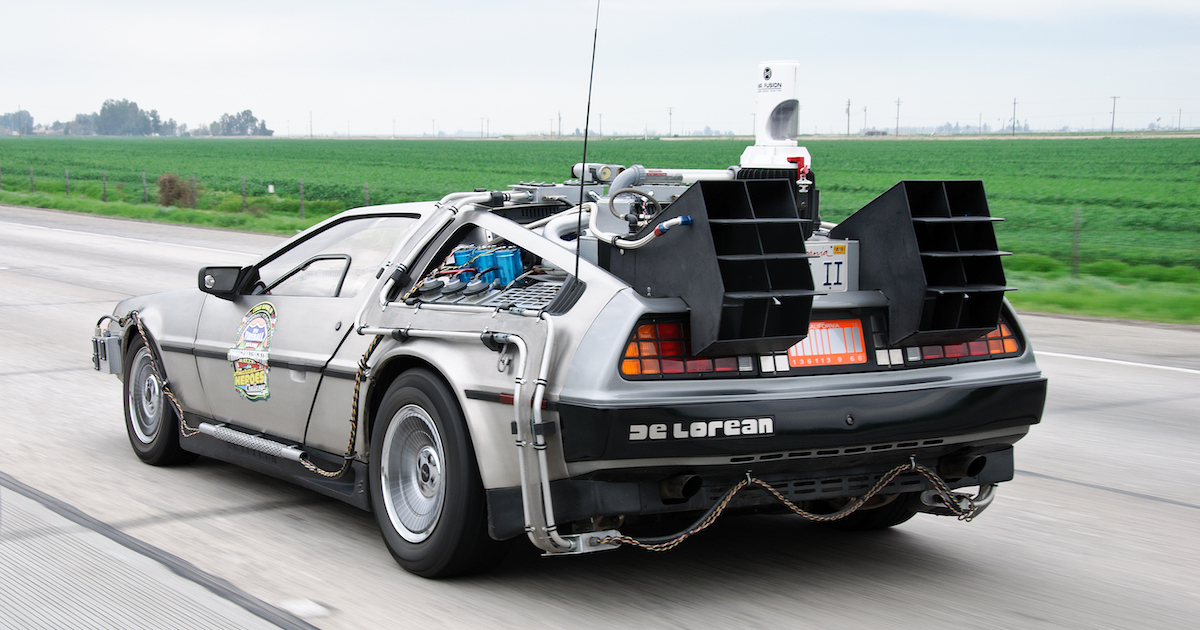
Go 1.19 focuses on improving the implementation of the toolchain, runtime, and libraries, especially for generics performance, the language memory model, and garbage collection.
After the introduction of generics in Go 1.18, the Go team has been working on improving their implementation to increase performance and to address subtle syntax corner cases. Generics performance can be up to 20% higher in some programs, says the Go team, although no benchmarks have been released yet. The syntax correction affects the scope of function type parameters, which makes it possible to write declarations like the following:
type T[T any] struct {}
func (T[T]) m() {}
In Go 1.18, instead, the T
inside the [T]
function parameter is shadowed by the outer type
definition, producing an error.
The Go memory model, which defines the way Go ensures variables read in one goroutine are guaranteed to reflect values written to that variable in a different goroutine, has been revised introducing a new definition of the happen-before relation which brings its semantics closer to that of other mainstream languages, including C, C++, Java, Rust, and Swift. This change does not impact existing programs, says Google, and should not concern most developers. In addition, a couple of new atomic types have been added to the sync/atomic
package, specifically atomic.Int64
and atomic.Pointer[T]
, mostly to simplify their usage.
Go 1.19 also introduces a few changes to its garbage collector that should help optimize Go programs so they run more efficiently in containers with dedicated amounts of memory.
Until Go 1.19, GOGC was the sole parameter that could be used to modify the GC’s behavior. While it works great as a way to set a trade-off, it doesn’t take into account that available memory is finite.
In particular, the possibility of transient spikes, which require new memory allocation proportional to the live heap, pose a limit to the maximum value that can be set for the live heap size (GOGC). For example, if the transient spike requires a new heap of the same size as the live heap, GOGC must be set to half the available memory, even if an higher value could provide better performance.
To better handle this, Go 1.19 introduces a configurable memory limit, controlled through the GOMEMLIMIT
environment variable. Knowing the maximum heap size, the Go garbage collector can set the total heap size in a more efficient way.
As a last note, Go 1.19 introduces a number of performance and implementation improvements including dynamic sizing of initial goroutine stacks, automatic use of additional Unix file descriptors, jump tables for large switch statements on x86-64 and ARM64, and many others. If you are interested in the full detail, make sure you read the official release notes.
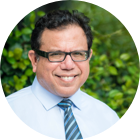
MMS • Edin Kapic
Article originally posted on InfoQ. Visit InfoQ
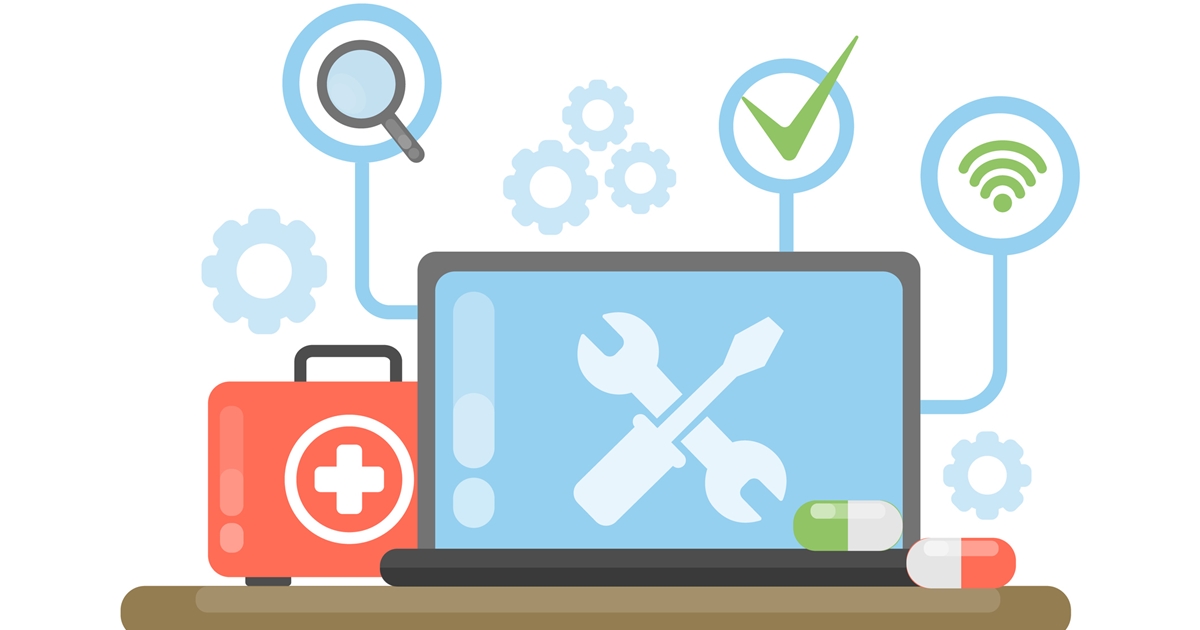
Microsoft has released version 8.0.0 of .NET Community Toolkit (NCT), a collection of helpers and APIs that make it easier to use patterns like MVVM (model-view-viewmodel) independently of the underlying platform. In this version, developers can benefit from reduced boilerplate code and streamlined API methods.
The sprawl of Microsoft UI frameworks in the last years (WPF, UWP, WinUI, Xamarin and MAUI) has made their .NET developers’ life harder if they wanted to build shared, platform-independent code in their applications. Microsoft and other developers in the community started sharing libraries and extensions for these frameworks in an effort to save developers’ time and reduce the implementation difficulty. Among other initiatives, a small MVVM library called MvvmLight developed by Laurent Bugnion saw significant adoption.
NCT is derived from a fork of Windows Community Toolkit (WCT) at version 7. WCT has extensions for WinUI and UWP, both being Windows-only frameworks. NCT inherits parts of code contributed to WCT that could be safely reused and it focuses on the platform-agnostic features that .NET application developers can benefit from. It includes a fast MVVM library that has been influenced by the design of MvvmLight. Microsoft mentions that NCT is used in their own developments such as Microsoft Store and Photos app.
Programming MVVM pattern leads involves creating commands and observable properties that drive UI updates. A basic command and observable property using NCT MVVM library looks like this:
Observable property Name
private string? name;
public string? Name
{
get => name;
set => SetProperty(ref name, value);
}
Command SayHello
private void SayHello()
{
Console.WriteLine("Hello");
}
private ICommand? sayHelloCommand;
public ICommand SayHelloCommand => sayHelloCommand ??= new RelayCommand(SayHello);
Version 8.0.0 of NCT simplifies this repetitive code by annotating properties and methods with attributes. These attributes get compiled, behind the scenes, into the code equivalent as the one seen before, using the Roslyn compiler source generator feature.
Observable property Name with annotations:
[ObservableProperty]
private string? name;
Command SayHello with annotations:
[RelayCommand]
private void SayHello()
{
Console.WriteLine("Hello");
}
Another source of boilerplate code in Microsoft MVVM frameworks is the implementation of the INotifyPropertyChanged
interface. It signals to the client code that a property has changed in the model and that it should probably redraw the UI to reflect the change. The cumbersome syntax of notifications using INotifyPropertyChanged
has prompted developers to create workarounds that encapsulate this behaviour as an abstraction, but as .NET doesn’t allow for multiple inheritance, the model class can’t inherit from any data parent class. NCT adds an annotation attribute that will generate the source code for this interface while still allowing the model to inherit from any other class, reducing the code verbosity.
A view model inheriting from a base type while still handling INotifyPropertyChanged behaviour
[INotifyPropertyChanged]
public partial class MyViewModel : SomeOtherType
{
}
This source generation feature of the new version has been hailed as a major improvement by some .NET developers.
Other NCT components that developers can use are helpers for parameter error validation or guard clauses. There is a set of helpers for leveraging high-performance .NET APIs such as Memory and Span. Microsoft recommends studying the source code of the MVVM sample application to get familiarised with the improvements in the new release.
Starting from version 8, the source code of all community toolkits is hosted under a single GitHub organisation under the umbrella of .NET Foundation. The NCT 8.0.0 is available as a NuGet package and it will run on any .NET Standard 2.0 platform, making it compatible with the legacy .NET Framework, but it will target the most recent .NET runtime to benefit from the performance improvements in them.
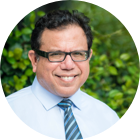
MMS • Olimpiu Pop
Article originally posted on InfoQ. Visit InfoQ
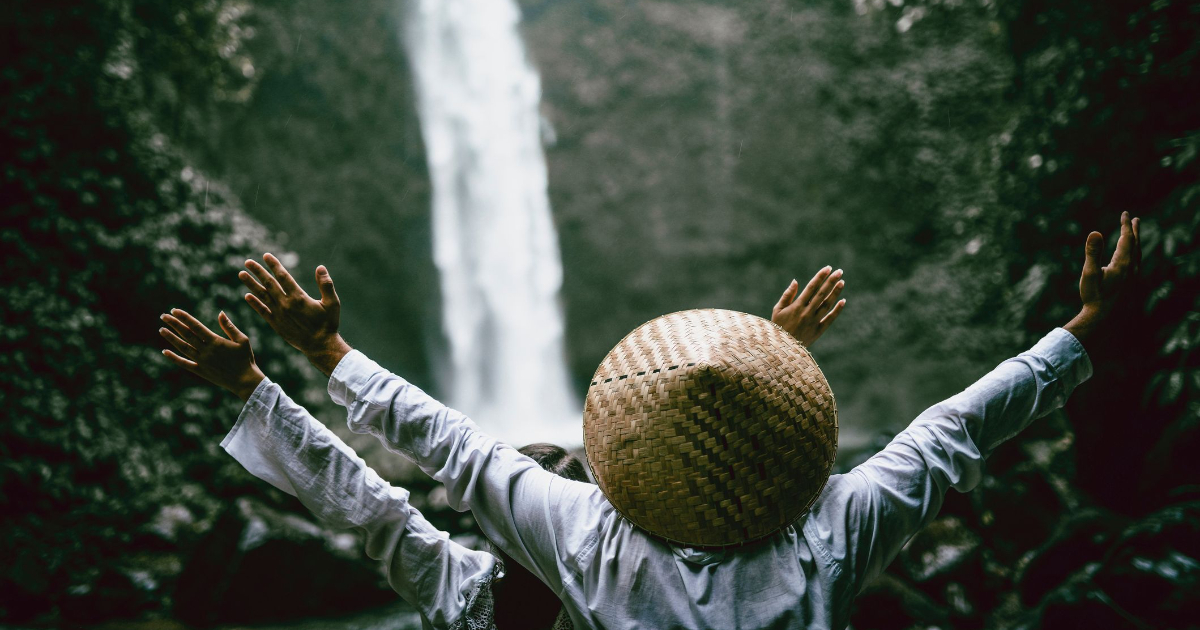
In 2015 Alexander Kondov, principal engineer at News UK, adopted Node.js as he considered its JavaScript’s ability to run both in the browser and the server an important feature that could enable his team. Based on his learning, he created the Tao of Node, which “contains proven rules and guidelines to build better applications.”
Node’s permissiveness to reuse libraries, logic, and types across front-end and back-end applications gave rise to the “full-stack developer” archetype: an engineer skillful enough to work on any part of the application they are needed. Its focus on freedom and flexibility, not imposing strict coding standards or application structures, brought it to point that each node application seems to follow a different approach.
Building on his experience building various applications, he laid down a series of principles for an improved experience while writing node applications.
InfoQ reached out to him, to find out more about these principles.
InfoQ: Hello, Alex. Thank you for taking the time to respond to the questions for our readers. Can you describe your responsibilities and what motivated you to write the Tao Of Node?
Kondov: I’m currently a principal engineer at News UK, leading two of our enabler teams that are building libraries, developer tools, and infrastructure for the rest of the engineers. My day-to-day responsibilities are not that different from that of any other developer, but I read and review more code than I write.
The goal behind Tao of Node was to formalize some of the common patterns that I’ve used successfully in my career and save some time for the engineers who are just starting. The Node ecosystem values freedom and choice but sometimes having a starting set of principles helps. Making good moves puts you in good positions, much like in chess.
InfoQ: Nice read, but lengthy. Top five takeaways for the reader? Any plans of making them enforceable through linters?
Kondov: First, I think application structure is critical, so I’d advise people to always organize their Node services around components and domain entities rather than technical responsibilities.
Second, co-locate related functionality and create nested modules.
Third, establish layers between the transport, domain, and data access logic.
Fourth, for performance, make sure you’re not blocking the event loop with intensive operations and you’ll solve most of your speed worries.
Last, don’t worry about database changes when it comes to abstractions – if you ever need to change the storage of an application in production the data in it should be your biggest worry.
Even though I considered creating an ESlint plugin, in the end, I preferred to keep them as guidelines. In that way, I don’t limit people’s creativity, as many of the rules are context-dependent.
InfoQ: How would you recommend bringing the theory into practice? How to implement it on existing projects?
Kondov: Piece by piece. Start by improving structure, it involves the least refactoring. Codebase reorganization requires mostly just import path changes. With a better structure, you can tackle each module at a time, separating layers and splitting logic. Similarly to a messy shelf, everything needs to go out before you can rearrange it.
A possible side effect of refactoring might be the decrease in the code’s quality. Once the mess is cleared up, you’ll have a more organized and productive application to work with.
InfoQ: What would be your biggest concern when looking at the JS ecosystem? What would you need?
Kondov: Node.js is a powerful tool that I would use for any application that doesn’t require heavy computation or manipulation of large data structures. It particularly shines when it comes to high-volume IO, but it can easily get winded if used for a problem that it cannot solve. Its community is quite resourceful, but it definitely needs some standardization, especially for tooling.
Much new functionality was added to JavaScript lately. That is not inherently bad, but bloating the standard library is not the way to do it. The need for backward compatibility results in a language that keeps growing. JS’ support for both functional and object-oriented paradigms, makes it appealing for engineers, but it can be very confusing for newcomers.
Tao of Node is my attempt to formalize some of the development patterns, but the JS community, in general, can benefit from a single tool that manages the toolchain – TypeScript, testing, linters, etc.
Seven years after node’s 1.0.00, Alexander Kondov celebrated this anniversary by publishing The Tao Of Node, an opinionated set of principles that should create a uniform approach for developing backend applications in JavaScript.
In the meantime, the once browser-only language grew in popularity and was awarded the silver medal by DataDog’s state of serverless report, while GitHub’s language statistics point to it as being one of the main languages being used in the first quarter of 2022.
In accordance with its popularity, JavaScript’s responsibility also grew, The Tao Of Node trying to normalize the various approaches that node’s flexibility allowed to appear.
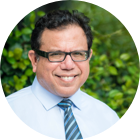
MMS • Ben Linders
Article originally posted on InfoQ. Visit InfoQ
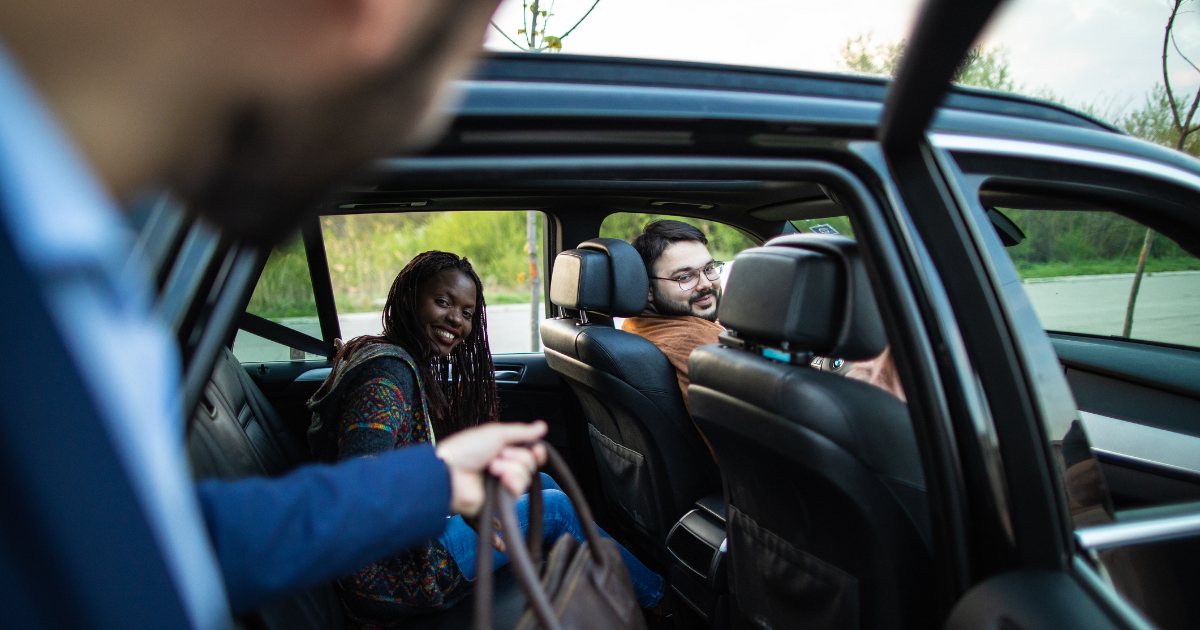
Getting and using feedback from colleagues who are also customers using your product can improve the quality of the product and help to improve the way of working. In this situation, it’s easier to receive feedback, but you can get overloaded by it.
Ada Pohl will speak about getting feedback when your customers are your colleagues at Agile Testing Days 2022. This conference will be held November 21-24 in Potsdam, Germany.
At MOIA, a ride sharing company, all parts of developing and running the service as well as the vehicles and drivers are internal. According to Pohl, there are upsides and downsides to getting and using feedback when your customers are also your colleagues:
The biggest upside is that you have easy access to feedback. You can approach colleagues directly or send out emails or surveys. Either way, you know where and who your customers are which makes getting feedback much easier.
A downside can be that you get too much feedback, unfiltered and maybe even unwanted. Which means you need to work through it to find the pieces that you can work with.
According to Pohl, the whole team benefits from having a feedback culture, as they develop their application very closely together with the drivers who are their customers; the biggest benefits were the rise in quality of the product they work on, the processes with which they work, and also the happiness of the team members.
InfoQ interviewed Ada Pohl about getting feedback.
InfoQ: What purposes can feedback serve?
Ada Pohl: Feedback helps you understand how your user is using your product, what works for them, what doesn’t work & what might be missing. Working agile we want to continuously learn and develop what we release. Ideas can look good on paper, but only reality shows how well they hold up and what needs or should be tweaked.
InfoQ: What methods do you use for collecting feedback? What are the advantages and disadvantages of these methods?
Pohl: Working on the navigation app for a ride pooling service we send out monthly surveys to our drivers with repeated questions for standing features as well as questions for new features.
We do interviews with individual drivers e.g. when we are about to release a bigger feature and want to know how it is perceived.
Another way of collecting feedback is a BugInbox Jira Board, through which our Hub Service Center Agents can send us any problem that gets reported by the drivers during their work. And we have bi-weekly exchanges with them for deeper feedback.
On top of this, we log actions & events of our application for monitoring and debugging. A lot of the time we might even start with just logging a new event to see if it gets sent when we expect it, and thereby learning from our data first, before implementing huge new features.
And we do test drives in which developers who implemented the feature are the driver. This has a huge impact on quality because putting on the driver’s shoes and experiencing the application as close to the real world usage as possible helps the team to get a better feeling of what might and might not be a good solution.
Especially for products like ours, testing interactions with a navigation system at your desk is just so different compared to testing it in the car.
InfoQ: How do you use the feedback that you get in your daily work?
Pohl: In my role as a quality specialist, I ask lots of questions. Having all the knowledge that feedback brings me means I can ask many more questions.
InfoQ: What have you learned?
Pohl: So much, but I think the most significant learning is that you need more than one way to collect feedback. Only with different ways and methods can you learn as much as you need to create a service or product that not only works for yourself, but also for your customer, especially in the situation where your customer will use your service or product.
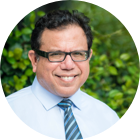
MMS • Karsten Silz
Article originally posted on InfoQ. Visit InfoQ
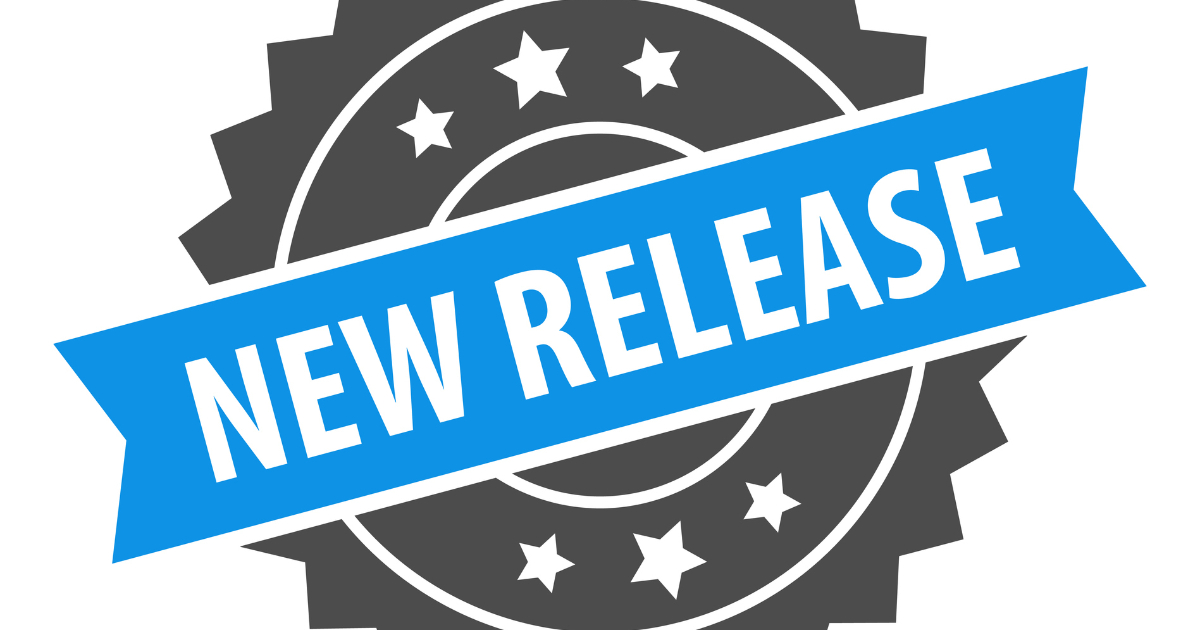
GraalVM is known for compiling Java into small native executables that start much faster than traditional Java programs. Release 22.2 by Oracle Labs addresses a long-standing pain point by introducing a configuration repository for Java libraries. Native Java compilation uses less memory, and the GraalVM distribution runs better on Apple Silicon and is smaller.
Native compilation makes Java more competitive in the cloud. Quarkus, Micronaut, and Helidon support GraalVM in production today. Spring 6 and Spring Boot 3 plan to do so by the end of this year. InfoQ published an article series on this topic.
Why does GraalVM need a configuration repository? Unlike regular Java, native Java executables can’t dynamically load new code when running. That’s why GraalVM’s native compiler “Native Image” needs to know all classes, methods, and fields used at runtime (“closed-world assumption”). Native Image detects that automatically with a reachability analysis. But dynamic Java features such as reflection and proxies hide some code from that analysis. Applications and libraries with these dynamic features must provide configuration hints to Native Image, or they won’t work at all in native Java.
Until now, Java developers had to provide these hints for libraries that didn’t ship with their GraalVM-supporting Java framework. The GraalVM Reachability Metadata Repository promises to remove that burden: GraalVM 22.2 reads hints (renamed into “reachability metadata”) from this new central repository. It is a collaboration between GraalVM, Micronaut, Spring Boot, and Quarkus and welcomes contributions.
Native Image now uses less RAM during compilation. For example, building the Spring PetClinic application uses just 2 GB. Memory-constrained CI environments or cloud services, like GitHub Actions, benefit from this resource reduction.
Native Image compiles not only JVM languages like Java, Scala, or Kotlin but also JavaScript, Python, Ruby, R, and WebAssembly. Python got an experimental bytecode interpreter with faster startup and better performance in this release. JavaScript interoperability with objects from other languages has improved.
The GraalVM distribution is smaller because it is more modular. It no longer includes the runtimes for JavaScript and LLVM or VisualVM. On Linux, this reduces the Java 17 GraalVM distribution size by 42% – from 431 MB to 251 MB.
With release 22.2, native Java executables can dump the memory heap into a file, as traditional Java applications can. That’s possible in three ways: By calling a runtime API, sending an OS signal to the application, or on exit. The release also includes faster escape analysis during compilation, improved debugging on Linux, and experimental strip mining optimization for counted loops.
The commercial GraalVM Enterprise Edition now has experimental Apple Silicon support, which was included in the Community Edition with the release of GraalVM 22.1 in April this year. Other GraalVM components now also support Apple Silicon in both editions, such as JavaScript, LLVM, Ruby, Java on the Truffle VM, and WebAssembly. The Enterprise Edition can also include a Software Bill of Materials (SBOM) in the CycloneDX format in the native executable.
OpenJDK manages the evolution of the Java language. But GraalVM is not part of OpenJDK as it belongs to Oracle Labs. Still, GraalVM will remain the only option for native Java in the coming years, as OpenJDK delayed its native Java plans in Project Leyden.