Month: November 2022
Azul Joins the Effort of Improving Supply Chain Security by Launching Vulnerability Detection SaaS
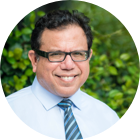
MMS • Olimpiu Pop
Article originally posted on InfoQ. Visit InfoQ
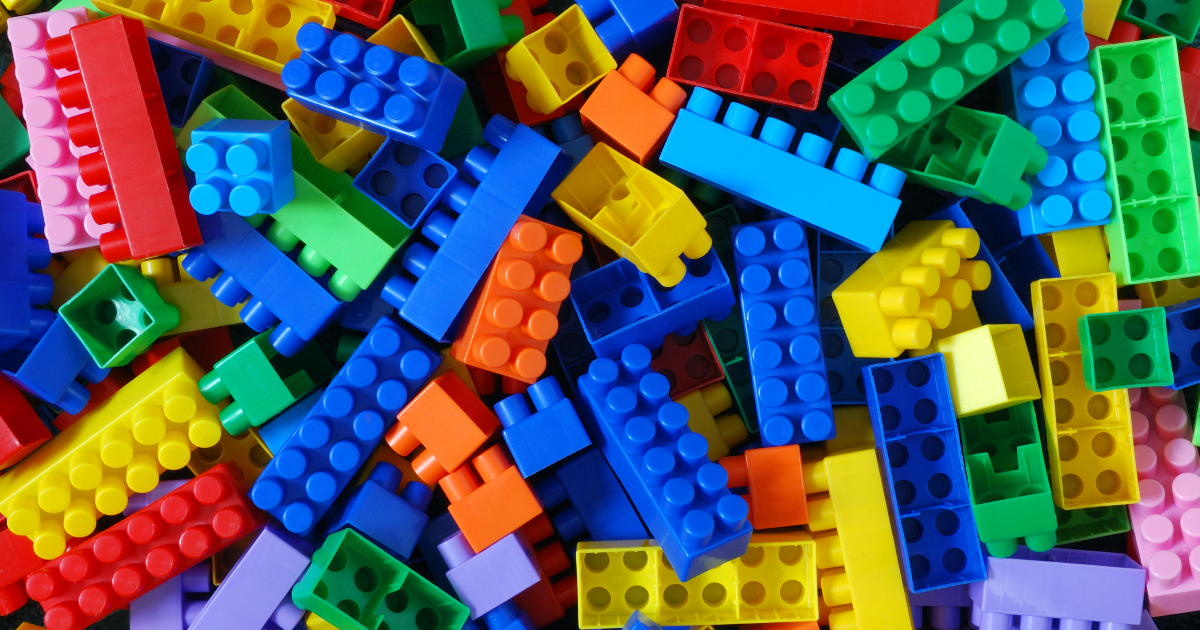
November, 2nd: Azul released a new security product that intends to offer a solution to the increased risk of enterprise software supply chain attacks, compounded by severe threats such as Log4Shell. Azul Vulnerability Detection is a new SaaS that continuously detects known security vulnerabilities in Java applications. In addition, they promise not to affect the application’s performance.
The Vulnerability Detection is a software composition analyzer(SCA), that intends to be Azul’s trial to take software supply chain security to the production environments. By doing so it allows organisations to presumably identify the actual point of use of vulnerable code, rather than just being present. In this way, it hopes to eliminate false positives and promises not to have any impact on the application’s performance.
The application doesn’t rely on agents for data collection but instead uses forwarders: a component designed to enable the communication between JREs on an internal network and the cloud vulnerability detection software.
Presumably, they were built to be easily configurable to move through firewalls and segmented networks and in this way be able to be used as the single control point for organisations to monitor traffic. By monitoring code executed based on real usage patterns recorded from any environment where its JVM is running (QA, development or production) an organisation should be able to compare its usage patterns. Once in the cloud, the information is compared against a curated CVE database containing Java-related vulnerabilities.
Azul considered that by gathering data at the JVM level it will be able to detect vulnerabilities in everything that runs on Java from built, bought or open-source regardless if they are frameworks (like Spring, Hibernate, Quarkus, Micronaut etc.), libraries or infrastructure (for instance Kafka, Cassandra, Elasticsearch).
More than just identifying vulnerable uses of the vulnerable code, the product comes with historical traceability forensics: the history of component and code use is retained, providing organisations with the forensic tool to determine whether vulnerable code was actually exploited prior to being known as vulnerable.
In order to make this happen, the Azul JVM is delivered with the Connected Runtime Service(CRS), which allows detection and communication with the Azul Vulnerability Detection Forwarder. It runs inside the Java process collecting information about the instance. Disabled by default, the CRS can be enabled either command line arguments or an environment variable. The successful connection will be reported in the log files: [CRS.id][info] CRS authenticated: YOUR_UUID, once the logs are enabled. Support for configuring JVMs at scale is also provided: rather than configuring each JRE individually, each enabled instance will look up two DNS entries for the other properties. The host could be either the cloud tool or a forwarder. All the JVMs in a common network will connect to the cloud.
In a world that software development is more and more built by using open source components, Gartner( in its Emerging tech: A Software Bill of Materials is Critical to Software Supply Chain Management from September 6th, 2022) predicted that “by 2025 45% of the global organisations will have experienced attacks on their supply chain, a three fold increase from 2021”. Almost one year since log4shell happened, Azul Systems tries to provide a solution for the increasing threat that supply chain attacks can pose. Their newly released SCA software promises to detect vulnerabilities where they happen: in the JVM.
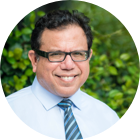
MMS • Oghenevwede Emeni
Article originally posted on InfoQ. Visit InfoQ
Key Takeaways
- Go is a statically typed open-source language that can be used for server-side programming, cloud-based development, command-line tool development, and game development.
- Continuous integration and deployment (CI/CD) enables organisations to release updates on a regular basis while maintaining quality.
- It is easier to test smaller changes using CI/CD before they are merged into the main application. This, as a result, encourages testability.
- Failures are easier to detect and resolve with CI/CD.
- Testing helps find error points in your application.
Introduction
In this article, we’ll look at how to use the gin framework to create a simple Golang application. We will also learn how to use CircleCI, a continuous deployment tool, to automate testing and deployment.
Go is a statically typed open-source programming language created by Google engineers with the sole purpose of simplifying complex software development processes and architectures. Its key features include high-performance networking, concurrency and ease of use. Goroutines are also extensively used in Go. A Goroutine is a function that runs alongside other goroutines in a program. Goroutines are useful when you need to do several things at once. Google, Cloudflare, MongoDB, Netflix, and Uber are a few companies that use Go.
Gin is a Go-based high-performance HTTP web framework that can be used to build microservices and web applications. The main advantage of Gin is that it allows developers to create scalable and efficient applications without having to write a lot of boilerplate code. It produces code that is easy to read and concise. It includes built-in routing, middleware for handling functionality, a logger, and a web server.
Building a simple CRUD API
We’ll create a simple API for a student management tool for a departmental club. The club president will have the ability to add new students and retrieve all students. To fully follow this tutorial, we will also require the following:
- Go installed, as well as a working knowledge of the language.
- Knowledge of tests and how to write them.
- An account on GitHub.
- An account on CircleCI.
- An Heroku account.
Please keep in mind that if you wanted to try this on a free tier account on Heroku, Heroku will be discontinuing its free tier plan soon. However, the procedures described here can be easily applied to the majority of other cloud hosting platforms.
Building the CRUD Application
Our simple departmental club API will only have two functionalities: adding students as members and viewing all members; nothing complicated! These will be POST and GET requests. We will not connect to any database such as MongoDB or MySQL. We will, however, use local storage and create a default student in the database. This student is automatically added whenever we restart the server.
Let’s get this party started. First, we’ll make a folder for our project. Let’s call it stud-api.
Within that folder, we will initialise our golang program and install all of the dependencies that we will need.
mkdir stud-api
cd stud-api
Next we will initialise our go.mod file and install all the needed dependencies.
go mod init stud-api
cd stud-api
go get -u github.com/gin-gonic/gin github.com/rs/xid github.com/stretchr/testify
Github.com/rs/xid is a library for creating unique identifiers. We will use it in this project to automatically generate IDs for each new student. The github.com/stretchr/testify package will be used to test our various endpoints.
Let’s get started on the API. For the sake of simplicity, we will only create one file called main.go
. This file will contain our struct
, as well as our API controllers, services, and routes. We will create three endpoints:
- A welcome function that sends a greeting message.
- A
CreateStudent()
function for adding a student to the database. - A
GetStudents()
function that returns all of the database’s registered students.
We will import three packages into our newly created main.go
file: an HTTP package, the xID package, and the gin package. Next, we’ll write a main()
function that will contain all of our API routes. Then we’ll make another function, which we’ll call WelcomeMessage()
. It will contain a simple message to be printed when we call the associated route.
package main
import (
"net/http"
"github.com/gin-gonic/gin"
"github.com/rs/xid"
)
func main() {
//setting up routes
router := gin.Default()
router.GET("/", WelcomeMessage)
router.Run()
}
//Welcome Message
func WelcomeMessage(c *gin.Context) {
c.JSON(http.StatusOK, gin.H{"message": "Hey boss!"})
}
To see what we have done so far, we are going to spin up our server using the command below:
go run main.go
When it runs successfully, the CLI will display the message “Hey boss!”. Now that we’ve created that simple function, let’s move on to our database and struct
.
We’ll build a simple Student struct
that takes three parameters: the student’s name, department, and level, and then generates an ID for the user when it’s successfully added to the database.
//Setting up student struct
type Student struct {
ID string `json:"id"`
Name string `json:"name"`
Department string `json:"department"`
Level string `json:"level"`
}
Let us now create our local database, that will be expecting the three values we are passing to the server as well as the generated ID. Our database will be called Students and will contain default data for one student, while any new students we create will simply be added to it.
//Students database
var students = []Student{
{
ID: "10000xbcd3",
Name: "Alicia Winds",
Department: "Political Science",
Level: "Year 3",
},
}
Now that this is in place, let’s write the CreateStudent() function and the route that will interact with it.
//Create a new student account
func CreateStudent() gin.HandlerFunc {
return func(c *gin.Context) {
var newStudent Student
if err := c.BindJSON(&newStudent); err != nil {
c.JSON(http.StatusBadRequest, gin.H{
"Status": http.StatusBadRequest,
"Message": "error",
"Data": map[string]interface{}{"data": err.Error()}})
return
}
//Generate a student ID
newStudent.ID = xid.New().String()
students = append(students, newStudent)
c.JSON(http.StatusCreated, newStudent)
}
}
Now we will add the route needed to communicate with the function to our main() function.
func main() {
-------------
router.POST("/createStudent", CreateStudent())
-------------
}
To test what we have done so far, if we spin up our server, and test our endpoint (localhost:8080/createStudent)
in Postman or any other environment, passing the name, department, and level in the body, a new user will be generated automatically with a unique ID. Please note that this is a non-persistent database.
We now need to create our last function which we will use to get all the students in the club database. This request is a simple GET function, which will search the students database and return all of its content.
func GetStudents() gin.HandlerFunc {
return func(c *gin.Context) {
//Fetch all students in the DB
c.JSON(http.StatusOK, students)
}
}
Finally, we’ll create the route that will interact with our newly created function. It will be added to our main function, along with the other routes.
func main() {
------------------
router.GET("/students", GetStudents())
router.Run()
}
Let’s also test this one on postman! To accomplish this, we will need to launch our server and access this endpoint: localhost:8080/students. All we need to do is use the HTTP verb GET. There is no need to include any body or query parameters. It will return all of the students in the database after a successful run. And with that, our simple CRUD API is finished!
Writing Simple Local Tests
In this section, we will run unit tests on the endpoints we have created. The goal is to ensure that each function works as expected. To test these functions, we will use the testify package. In addition, we must create a new file called new_test.go. This file will contain the various tests that we will write. We’ll need to import a few packages after we’ve created the new file in our root in our main directory.
func main() {
------------------
router.GET("/students", GetStudents())
router.Run()
}
Testify makes it easy to perform simple assertions and mocking. The testing.T object is passed as the first argument to the assert function in go. The assert function then returns a bool indicating whether the assertion was successful or not. The testify mock package offers a method for quickly creating mock objects that can be substituted for actual objects when writing test code.
Now we will set up a router and write a simple test for our welcome message. The assert
function in the simple welcome message test below will use the equality variant to determine whether the test argument matches the mock response.
func SetRouter() *gin.Engine {
router := gin.Default()
return router
}
func TestWelcomeMessage(t *testing.T) {
mockResponse := `{"message":"Hey boss!"}`
r := SetRouter()
r.GET("/", WelcomeMessage)
req, _ := http.NewRequest("GET", "/", nil)
w := httptest.NewRecorder()
r.ServeHTTP(w, req)
responseData, _ := ioutil.ReadAll(w.Body)
assert.Equal(t, mockResponse, string(responseData))
assert.Equal(t, http.StatusOK, w.Code)
}
Next, we’ll write a simple test for the createStudent()
function using mock data. We will continue to use the xID package to generate the Student ID, and we will receive a bool indicating whether or not the test was successful.
func TestCreateStudent(t *testing.T) {
r := SetRouter()
r.POST("/createStudent", CreateStudent())
studentId := xid.New().String()
student := Student{
ID: studentId,
Name: "Greg Winds",
Department: "Political Science",
Level: "Year 4",}
jsonValue, _ := json.Marshal(student)
req, _ := http.NewRequest("POST", "/createStudent", bytes.NewBuffer(jsonValue))
w := httptest.NewRecorder()
r.ServeHTTP(w, req)
assert.Equal(t, http.StatusCreated, w.Code)}
Finally, we’ll write our final test for the GetStudents() function.
func TestGetStudents(t *testing.T) {
r := SetRouter()
r.GET("/students", GetStudents())
req, _ := http.NewRequest("GET", "/students", nil)
w := httptest.NewRecorder()
r.ServeHTTP(w, req)
var students []Student
json.Unmarshal(w.Body.Bytes(), &students)
assert.Equal(t, http.StatusOK, w.Code)
assert.NotEmpty(t, students)
}
We have completed all of the tests and can now run them locally. To do so, simply run the following command:
GIN_MODE=release go test -v
And here’s our final result:
Automating Tests with Continuous Development
CircleCI is a platform for continuous integration and delivery that can be used to integrate DevOps practices. We will use this CI/CD tool in this article to automate our tests and deploy our code to our server but let’s start by automating tests with CircleCI first.
Make sure you have a CircleCI account, as specified in the prerequisites, and that you have successfully pushed the code to GitHub. Check your CircleCI dashboard to ensure that the project repository is visible.
Now, in the project directory, we need to create a .circleci folder and a config.yml file, which will contain the commands needed to automate the tests.
Setting up the config.yaml
This file contains all of the configurations required to automate Heroku deployment and testing. For the time being, we won’t be focusing on the Heroku section because we’re more interested in the code that helps automate our tests. The file contains the Go orb and Jobs that checkout and run the test. We’ll need to re-push to GitHub after adding the code below to our config file.
workflows:
heroku_deploy:
jobs:
- build
- heroku/deploy-via-git:
requires:
- build
filters:
branches:
only: main
jobs:
build:
working_directory: ~/repo
docker:
- image: cimg/go:1.17.10
steps:
- checkout
- restore_cache:
keys:
- go-mod-v4-{{ checksum "go.sum" }}
- run:
name: Install Dependencies
command: go get ./...
- save_cache:
key: go-mod-v4-{{ checksum "go.sum" }}
paths:
- "/go/pkg/mod"
- run:
name: Run tests
command: go test -v
After this step, we can return to our CircleCI dashboard and choose our project. Then we need to click the Setup button next to it and select the branch we’re working on. When we click the Setup button, our program will begin to run. A successful build would look like the image below (when we scroll down to the run tests section)
That’s it! We were able to successfully build a simple API, create local tests, and automate the testing process. The entire automation process here means that the pipeline attempts to run the test every time a push is made to that branch on the github repository.
Automating Deployment to Heroku using circleCI
First, we must configure Heroku. If you do not already have a Heroku account, you will need to create one and connect your GitHub profile to your Heroku account for easy deployment and automation. Once that is completed, we will need to create a Procfile (yeah, with no extensions) within our project folder. Within the Procfile we will add the following:
web: app
After we do that we will make a push to GitHub. Now let’s take a quick look at the config.yaml file we created earlier. We can now analyse the first section. We can see that we imported the Heroku orb and we have a workflow that contains a job that builds and deploy the code in the main repository.
Returning to our Heroku dashboard, we must first create a project on Heroku and obtain our API keys, which can be found in the account settings. We Will need to add this key to our CircleCI project. To do this, navigate to our existing project on CircleCI and select Project Settings. Then go to the environment variables section and add two things:
HEROKU_APP_NAME
, with the valuestud-api
(the name of our application)HEROKU_API_KEY
with the key we just obtained from Heroku.
We have successfully configured our circleci project for continuous deployment to Heroku. If this was successful, we should see a successful build message on our CircleCI dashboard. Something like this:
To see what we’ve done, we can return to our Heroku dashboard and retrieve our project URL. This is the URL in this case: https://stud-app-api.herokuapp.com/. All of the functionalities can be tested by appending the routes you want to test to the end of the url. For example testing the endpoint that fetches all students:
Conclusion
Continuous development enables developers to create better and faster products. Continuous integration and development tools have simplified the entire process by automating operations that would otherwise have required more time or expertise. CI/CD tools have gradually helped to improve product quality by automating everything from testing to quick application deployment.
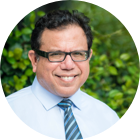
MMS • Steef-Jan Wiggers
Article originally posted on InfoQ. Visit InfoQ
Recently AWS announced the general availability (GA) of a new deployment option for Amazon Neptune, providing automatic scaling capacity based on the application’s needs. The deployment option is called Amazon Neptune Serverless.
Amazon Neptune is a fully-managed graph database service that makes it easy to build and run applications that work with highly connected datasets. The service has various features and ways to provision a database, which now includes a Serverless option.
Daniel Dominguez, a software program manager, explains in his blog post the serverless deployment option:
If you have unpredictable and variable workloads, Neptune Serverless automatically determines and provisions the compute and memory resources to run the graph database. Database capacity scales up and down based on the application’s changing requirements to maintain consistent performance, saving up to 90% in database costs compared to provisioning at peak capacity.
Developers can create a Neptune Serverless cluster from the Amazon Neptune console, AWS Command Line Interface (CLI), or SDK, with support for AWS CloudFormation soon. They can use the database service available and popular graph query languages (Apache TinkerPop Gremlin, the W3C’s SPARQL, and Neo4j’s openCypher) to execute powerful queries that are easy to write and perform well on connected data. The service is suitable for use cases such as recommendation engines, fraud detection, knowledge graphs, drug discovery, and network security.
Swami Sivasubramanian, vice president of Databases, Analytics, and Machine Learning at AWS, said in a press release:
Now, with Amazon Neptune Serverless, customers have a graph database that automatically provisions and seamlessly scales clusters to provide just the right amount of capacity to meet demand, allowing them to build and run applications for even the most variable and unpredictable workloads without having to worry about provisioning capacity, scaling clusters, or incurring costs for unused resources.
Maciej Radzikowski, a software developer at Merapar, tweeted:
After Aurora, welcome Amazon Neptune “Serverless”…
✅ Yes, I’m glad there is an auto-scaling capability.
✅ Yes, I’m aware scaling to zero is difficult for DBs.
❌ No, I don’t like putting “serverless” label on things that are not serverless.
In addition, Sebastian Bille, founder & software engineer at Elva, summarized the limitations in his tweet:
Neptune’s “Serverless” release
- No CloudFormation support
- Only a few regions
- Still requires a VPC
- Doesn’t scale to zero
Currently, Neptune Serverless is now available in the following AWS Regions: US East (Ohio), US East (N. Virginia), US West (N. California), US West (Oregon), Asia Pacific (Tokyo), Europe (Ireland), and Europe (London).
Lastly, more details on the serverless deployment option are available on the documentation page. Additionally, pricing details can be found on the pricing page.
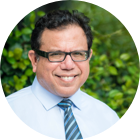
MMS • Anthony Alford
Article originally posted on InfoQ. Visit InfoQ
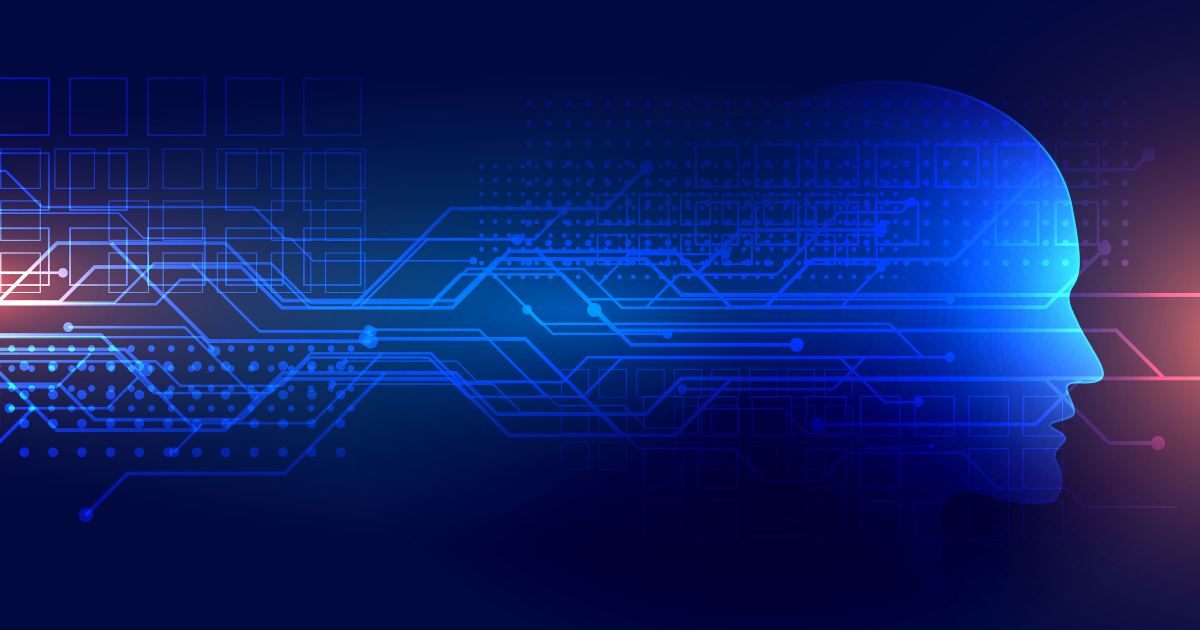
Anaconda, makers of a Python distribution popular among data scientists, recently published a report on the results of their State of Data Science survey. The report summarizes responses from nearly 3,500 students, academics, and professionals from 133 countries, and covers topics about respondent demographics and jobs as well as trends within the community.
The report was announced on the Anaconda blog. The survey ran from April 25 until May 14 this year, with respondents gathered from social media, the Anaconda website, and the Anaconda email database. The survey begins with demographics questions, then moves to workplace topics, including job tasks, tools, and the future of work. The survey also drills deep into the use of open-source software (OSS) in the enterprise, with particular focus on contribution as well as security concerns. The report concludes with several key takeaways, such as concerns in industry about a data-science talent shortage.
According to Anaconda,
As with years prior, we conducted a survey to gather demographic information about our community, ascertain how that community works, and collect insights into big questions and trends that are top of mind within the community. As the impacts of COVID continue to linger and assimilate into our new normal, we decided to move away from covering COVID themes in our report and instead focus on more actionable issues within the data science, machine learning (ML), and artificial intelligence industries, like open-source security, the talent dilemma, ethics and bias, and more.
The report is organized into the following sections:
- The Face of Data Science: demographic data about the respondents
- Data Professionals at Work: data about the work environment
- Enterprise Adoption of Open Source: use and contribution to OSS and concerns about the OSS “supply chain”
- Popularity of Python: data about the adoption of various programming languages by the respondents
- Data Jobs and the Future of Work: data about job satisfaction, talent shortages, and the future of the workforce
- Big Questions and Trends: sentiments about innovation and government involvement
The section on enterprise adoption of OSS revealed a concern about the security of OSS projects. In particular, the recent Log4j incident was a “disruptive and far-reaching example” that caused nearly a quarter of the respondents to reduce their OSS usage. Although most companies represented in the responses still use OSS, nearly 8% do not, and of those more than half said it was due to security concerns, which is a 13% increase from last year. The results also showed a 13% year-over-year decrease in the number of respondents whose companies encourage them to contribute to OSS.
The section on big questions and trends looked at several topics, including blockers to innovation. A majority said that insufficient talent and insufficient investment in engineering and tooling were the biggest barriers in the enterprise. Perhaps related to this concern about talent shortages, respondents said the most important role of AutoML would be to enable non-experts to train models, and nearly 70% of respondents thought their governments should provide more funding for STEM education.
In a discussion on Twitter about the survey responses on the role of government in tech, industry analyst Lawrence Hecht pointed out:
[Respondents] mostly want the government to give them money. Only 35% want regulation of Big Tech. A follow-on chart shows that even more clearly that there is no desire for specific actions re: AI and tech regulation — more of a general angst.
Earlier this year, InfoQ covered Stanford University’s AI Index 2022 Annual Report, which identifies top trends in AI, including advances in technical achievements, a sharp increase in private investment, and more attention on ethical issues. More recently, AI investors Nathan Benaich and Ian Hogarth published their fifth annual State of AI Report, covering issues related AI research, industry, politics, and safety. At the 2022 Google Cloud Next conference, Kaggle presented the results of their annual State of Data Science and Machine Learning survey of 23,997 respondents from 173 countries; the survey questions covered areas related to respondent demographics, programming language and tools, machine learning, and cloud computing.
The Anaconda survey response raw data is available on the Anaconda Nucleus website.
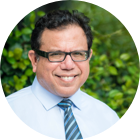
MMS • Bruno Couriol
Article originally posted on InfoQ. Visit InfoQ
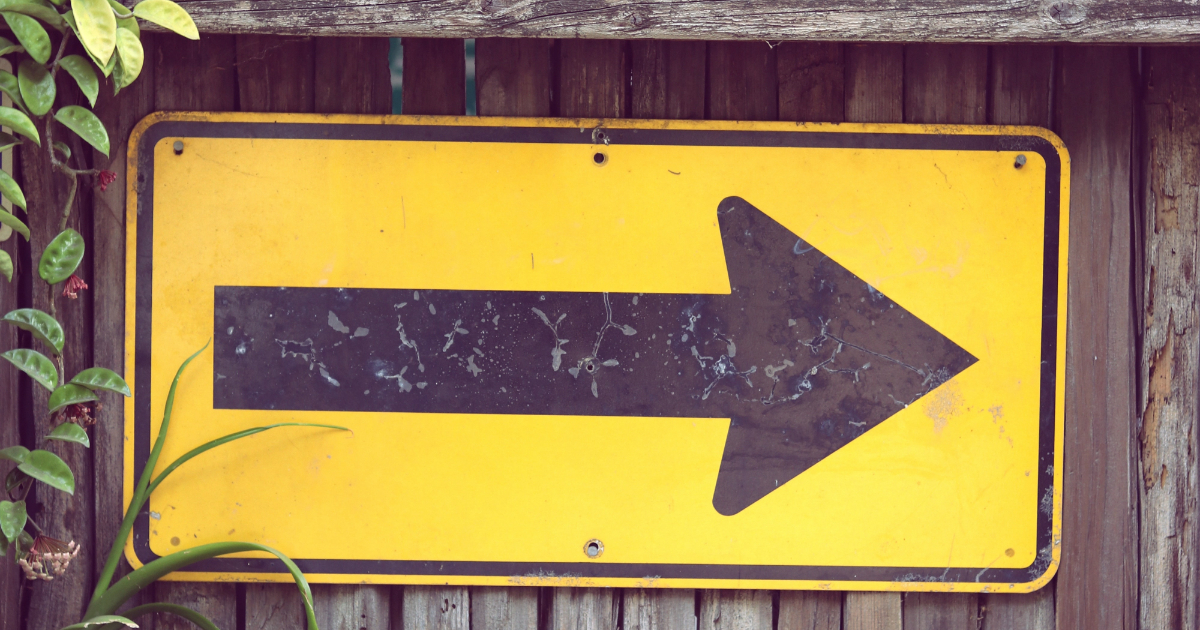
The Next.js conference recently introduced Next.js 13, the latest version of the React application framework. Next.js 13 wants to enable developers to make “dynamic without limits” applications through innovative features, many of which are still in alpha or beta. The new features update the compiler, routing, and rendering infrastructure, and improve the component toolkit.
The Next.js team explained in a recent keynote the rationale behind the latest release of Next.js:
Next.js had its origins as a React framework for dynamic server-rendered sites. Instead of optimizing for single-page applications, we designed Next.js for teams building ambitious, complex applications. But being dynamic has always come with a lot of limits.
You’ve wanted to be dynamic, but it’s meant at the expense of costly, always-on infrastructure, requiring manual provision and extensive operations.
You’ve wanted to be dynamic, but it’s meant juggling two sets of runtime APIs, no JS in the server, and web standard APIs in the browser.
You’ve wanted to be dynamic, but often only in a single region origin, depending on legacy, static, CDN caching to try to perform and scale.
[…]
Today, we’re releasing Next.js 13 to enable you to be dynamic without limits.
The new release features improvements in the toolkit (improved Link component, new Image component, and new @next/font
library). Additional alpha/beta features provide a glimpse of the future of server-side rendering, as envisioned by Vercel.
The new Image component is designed to improve user experience, as driven by native lazy loading, less client-side JavaScript being shipped, and the absence of layout shift. On the developer experience side, the new component strives to be easier to style and configure.
The improved Link component no longer requires an anchor tag (i.e., ) as a child. The following is now valid Next.js code for an anchor link:
<Link href="/about">
About
</Link>
@next/font
(released in beta) will automatically optimize fonts (including custom fonts) and remove external network requests for improved privacy and performance. The documentation details:
@next/font
includes built-in automatic self-hosting for any font file. This means you can optimally load web fonts with zero layout shift, thanks to the underlying CSSsize-adjust
property used.
Next.js 13 introduces Turbopack (released in alpha), intended as a Rust-based replacement to Webpack that provides developers with orders of magnitude improvements in speed. Like Parcel before it, Turbopack incrementally builds and bundles source files. The Next.js team touts:
Turbopack only bundles the minimum assets required in development, so startup time is extremely fast. On an application with 3,000 modules, Turbopack takes 1.8 seconds to boot up. Vite takes 11.4 seconds. Webpack takes 16.5 seconds.
Turbopack has out-of-the-box support for Server Components, TypeScript, JSX, CSS, and more.
Evan You, the creator of Vite, recently challenged the Vite vs. Next/Turbo benchmark, finding similar speed when the benchmark is performed using comparable configurations. As of the day of publication of this article, Vercel’s benchmark methodology and results have been published and corrected some mistakes but remain a topic of debate.
While the improvement in the developer experience is expectedly positively valued by many developers, one developer pointed to possible downsides and limitations:
There’s a massive ecosystem of Webpack plugins that will likely make migration very hard for existing apps. Vercel will likely need to count on community contributions with some kind of plug-in system and that might be difficult because JavaScript developers like me are too dumb and lazy to learn Rust.
Furthermore, […] Vite with esbuild is already fast enough for most projects and it’s got an awesome developer experience that’s hard to beat.
You should also know that Vercel has an angle to make money on this by remotely caching builds in the cloud.
Next.js 13 also brings major changes to the routing and rendering infrastructure of the framework, some of which have been worked on in direct collaboration with the React core team to leverage React’s Server Components, Suspense, and streaming. The documentation explains:
The new router includes support for:
- Layouts: Easily share UI between routes while preserving state and avoiding expensive re-renders.
- Server Components: Making server-first the default for the most dynamic applications.
- Streaming: Display instant loading states and stream in units of UI as they are rendered.
- Support for Data Fetching:
async
Server Components and extendedfetch
API enables component-level fetching.
For more detail, developers may refer to the release note.
While there are plenty of positive reactions to the release from developers, one developer noted:
The rules related to how server components can be used may be unintuitive and hard to teach. React already has a reputation for being too complicated, I don’t think this is going to help. Dealing with client-side JS and Node runtimes in the same codebase is already a bit of a dance, but in the old paradigm at least there was only one interaction point between the two worlds (
getServerSideProps
/getStaticProps
), now it could be at every component boundary.
Another developer warned against the bleeding-edge nature of some of the new features:
Next.js embraces some experimental, not yet stable React features, which the React team is researching, like server-side components, or async/await support in those server-side components.
And therefore, NextJS also embraces potential future React concepts. But what’s really important to understand, and what can’t be stressed enough is that we’re talking about unstable, not yet finished APIs, which are still being researched and fleshed out.
For that reason, if you explore the beta docs for this new/app
folder and this new way of building Next.js applications, you will find a lot of warnings and notes about features still missing, not being finished, likely to change, and so on.
Next.js is open-source software available under the MIT license. Contributions are welcome via the Next.js GitHub repo following the Next.js contribution guidelines and code of conduct.
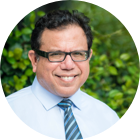
MMS • Srini Penchikala
Article originally posted on InfoQ. Visit InfoQ
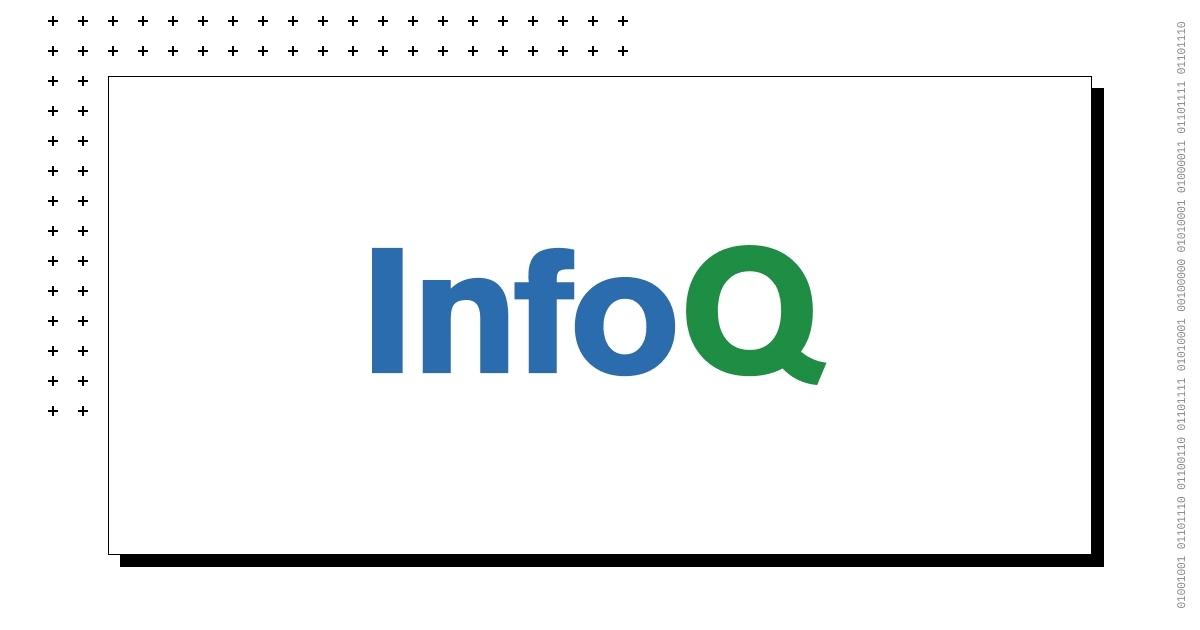
CloudEvents specification can help solve the challenges associated with cloud event management lifecycle, like discovery of event producers, setting up subscriptions and event verification. Doug Davis from Microsoft spoke on Thursday at KubeCon CloudNativeCon North America 2022 Conference about how CloudEvents project has been focused on eventing-related painpoints that might benefit from some standardization.
Currently there is no consistency in how the events are managed in the cloud, which is causing the application developers to have to write new event handling logic for each event source.
A typical messaging based solution in the cloud consists of different event producers going to a middleware message broker like Kafka which in turn is subscribed by different event consumers. The challenge is that the events and messages can be very diverse in terms of formats, schemas, and business logic. These architectures also need to support different messaging protocols like MQTT and HTTP.
CloudEvents, organized via the CNCF’s Serverless Working Group, is a specification for describing event data in a common way and seeks to simplify event declaration and delivery across services and platforms. It’s a simple specification that defines common event related metadata independent of business logic. The project goals are to improve interoperability, enable automation, runtime generation & validation, and development time tooling.
Davis discussed how cloud event specification looks like with the help of a JSON example. CloudEvents defines extra metadata with four additional properties: specversion, event type, event source, and event ID. He also showed the difference in message formats like HTTP binary vs HTTP structured format.
CloudEvents context attributes include: required parameters like the ones mentioned above, optional parameters like subject, time, datacontenttype, and dataschema. There are also extensions like dataref, distributed-tracing, traceparent, tracestate, partitionkey, severity etc.
He talked about how CloudEvents can help with rest of the lifecycle, including the following use cases:
- discovery of event producer endpoints,
- consumption/subscription mechanism,
- discovery of event consumer endpoints,
- how to push to an event consumer,
- discovery of event types, formats, and attributes,
- discover supported endpoints, and
- event validation.
Davis described how Discovery Service Endpoint works in sending or receiving messages. Event producer registers itself with Discovery Service. Event consumer talk to the producer after retrieving the details from the Discovery Service. He showed examples of message definitions with a mixture of s3-events using different formats and schemas. The Subscriber side of the communication needs more than the basic specification attributes. The team is looking to create a brand new spec just for subscribers with additional options like filtering, pull or push model etc. Message delivery portion takes care of tasks like transfer of messages, validation of messages, retrieval of metadata from Discovery Service including message schema.
Schema Registry specification helps with standardization for storing/managing schema itself. Its goals are to keep the spec simple, protocol neutral and scenario/schema/messaging neutral. Schema registry structure includes attributes like schema groups, grouping / access control, and schema versions.
The new specs for Discovery API, Subscriptions API, and Schema Registry don’t need to be used together, they can be used independently.
CloudEvents integrations include several popular cloud event, data and security technologies like Adobe I/O Events, Alibaba Cloud EventBridge, Argo Events, Azure Event Grid, Debezium, Falco, Google Cloud Eventarc, IBM Cloud Code Engine and Knative Eventing.
Davis concluded the presentation saying that CloudEvents is a new effort and is still under active development. Its working group has received a lot of industry interest, ranging from major cloud providers to popular SaaS companies.
The plan is to have a release candidate version by EOY 2022 with support for different languages and different protocols. The working group is looking for end-user feedback on any new use cases on discovery that are missing in the current specification.
If you are interested in more information on Cloud Events spec, checkout the following resources: CloudEvents website, specifications repo, and weekly calls on Thursdays @ 12pm ET (see repo for dial-in info & slack info). If you are interested in contributing, checkout the CloudEvents Github project, join their weekly call via Zoom, check the meeting notes, and review the Governance model to familiarize with the contribution process.