Month: February 2023
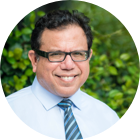
MMS • RSS
Posted on nosqlgooglealerts. Visit nosqlgooglealerts
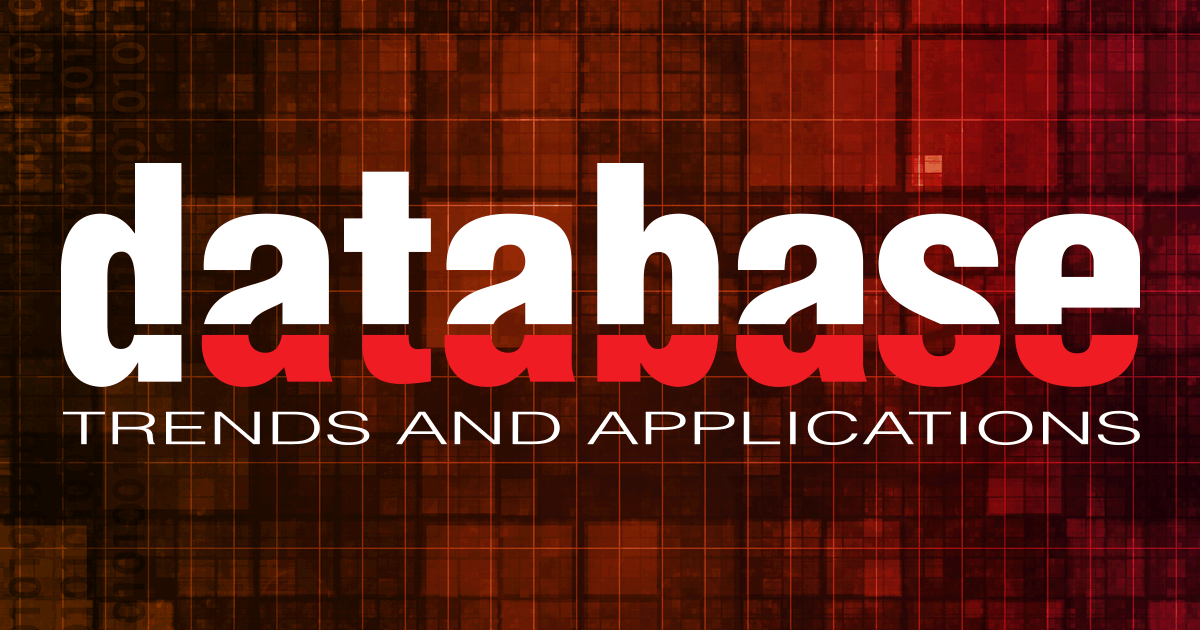
Progress, a provider of application development and infrastructure software, announced it has completed the acquisition of MarkLogic, a leader in complex data and semantic metadata management and a Vector Capital portfolio company.
“Our Total Growth Strategy consists of three pillars—Invest and Innovate, Acquire and Integrate and Drive Customer Success. The MarkLogic acquisition aligns with this approach by adding industry-leading products to our already-strong portfolio, new and meaningful customer relationships to our large customer base and significant revenue to our top line,” said Yogesh Gupta, CEO, Progress. “Expanding our ability to serve our customers propels their business success. This is an exciting opportunity, and we are thrilled to move forward together.”
The acquisition represents another major milestone in Progress’ Total Growth Strategy, according to the vendor.
MarkLogic extends Progress’ capabilities beyond structured data with a powerful NoSQL database, informed search, and semantic AI.
It enables users to connect, create and consume complex, contextual data and addresses a variety of high-value use cases such as complex customer data challenges, large data volumes, multiple data sources and types—yet manages it all natively from a single unified platform, according to the vendor.
As previously reported, Progress acquired MarkLogic for a purchase price of $355 million. The transaction is expected to be accretive to both non-GAAP earnings per share and cash flow, beginning in Q2 2023.
For more information about this news, visit www.progress.com.
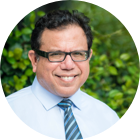
MMS • RSS
Posted on nosqlgooglealerts. Visit nosqlgooglealerts
Acquisition expands Progress’ industry-leading product portfolio and continues to deliver on Total Growth Strategy
BURLINGTON, Mass., Feb. 07, 2023 (GLOBE NEWSWIRE) — Progress (NASDAQ: PRGS), the trusted provider of application development and infrastructure software, today announced the completion of the acquisition of MarkLogic, a leader in complex data and semantic metadata management and a Vector Capital portfolio company. It is also another major milestone in Progress’ Total Growth Strategy.
“Our Total Growth Strategy consists of three pillars—Invest and Innovate, Acquire and Integrate and Drive Customer Success. The MarkLogic acquisition aligns with this approach by adding industry-leading products to our already-strong portfolio, new and meaningful customer relationships to our large customer base and significant revenue to our top line,” said Yogesh Gupta, CEO, Progress. “Expanding our ability to serve our customers propels their business success. This is an exciting opportunity, and we are thrilled to move forward together.”
MarkLogic extends Progress’ capabilities beyond structured data with a powerful NoSQL database, informed search and semantic AI. It enables users to connect, create and consume complex, contextual data and addresses a variety of high-value use cases such as complex customer data challenges, large data volumes, multiple data sources and types—yet manages it all natively from a single unified platform.
“MarkLogic presents a unique opportunity for Progress customers. By expanding our data capabilities, our customers gain more agility as to how and where they can leverage their data,” said John Ainsworth, Executive Vice President, General Manager, Application and Data Platform, Progress. “At the same time, MarkLogic customers gain access to a global leader with a proven track record in the software infrastructure space.”
As previously announced, Progress acquired MarkLogic for a purchase price of $355 million. The transaction is expected to be accretive to both non-GAAP earnings per share and cash flow, beginning in Q2 2023.
About Progress
Dedicated to propelling business forward in a technology-driven world, Progress (Nasdaq: PRGS) helps businesses drive faster cycles of innovation, fuel momentum and accelerate their path to success. As the trusted provider of the best products to develop, deploy and manage high-impact applications, Progress enables customers to develop the applications and experiences they need, deploy where and how they want and manage it all safely and securely. Hundreds of thousands of enterprises, including 1,700 software companies and 3.5 million developers, depend on Progress to achieve their goals—with confidence. Learn more at www.progress.com and follow us on LinkedIn, YouTube, Twitter, Facebook and Instagram.
Note Regarding Forward-Looking Statements
This press release contains statements that are “forward-looking statements” within the meaning of Section 27A of the Securities Act of 1933, as amended, and Section 21E of the Securities Exchange Act of 1934, as amended. Progress has identified some of these forward-looking statements with words like “believe,” “may,” “could,” “would,” “might,” “should,” “expect,” “intend,” “plan,” “target,” “anticipate” and “continue,” the negative of these words, other terms of similar meaning or the use of future dates.
Risks, uncertainties and other important factors that could cause actual results to differ from those expressed or implied in the forward looking statements include: uncertainties as to the effects of disruption from the acquisition of MarkLogic making it more difficult to maintain relationships with employees, licensees, other business partners or governmental entities; other business effects, including the effects of industry, economic or political conditions outside of Progress’ or MarkLogic’s control; transaction costs; actual or contingent liabilities; uncertainties as to whether anticipated synergies will be realized; and uncertainties as to whether MarkLogic’s business will be successfully integrated with Progress’ business. For further information regarding risks and uncertainties associated with Progress’ business, please refer to Progress’ filings with the Securities and Exchange Commission, including its Annual Report on Form 10-K for the fiscal year ended November 30, 2022. Progress undertakes no obligation to update any forward-looking statements, which speak only as of the date of this press release.
Press Inquiries: |
Investor Relations: |


NoSQL Market 2023-2028, Global Share, Size (US$ 35.7 Billion), Growth (CAGR of 29.92 …
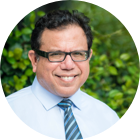
MMS • RSS
Posted on nosqlgooglealerts. Visit nosqlgooglealerts
The increasing user-created content has led to the generation of big datasets is primarily driving the NoSQL market.
SHERIDAN, WY, USA, February 6, 2023 /EINPresswire.com/ — The latest report published by IMARC Group, titled “NoSQL Market: Global Industry Trends, Share, Size, Growth, Opportunity and Forecast 2023-2028,” offers a comprehensive analysis of the industry, which comprises insights on the market share. The report also includes competitor and regional analysis, and contemporary advancements in the market. The global NoSQL market size reached US$ 7.3 Billion in 2022. Looking forward, IMARC Group expects the market to reach US$ 35.7 Billion by 2028, exhibiting a growth rate (CAGR) of 29.92% during 2023-2028.
Year Considered to Estimate the Market Size:
• Base Year of the Analysis: 2022
• Historical Period: 2017-2022
• Forecast Period: 2023-2028
Request a Free PDF Sample of the Report: https://www.imarcgroup.com/nosql-market/requestsample
Industry Definition and Application:
NoSQL represents a non-relational database (NRDB) that provides a mechanism to store and retrieve data. In terms of the database type, it can be categorized into key-value-based, document-based, column-based, graph-based, etc. NoSQL databases can support structured query language (SQL) and non-tabular, polymorphic, semi-structured, and unstructured data. They are constructed for particular data models and include flexible schemas that allow programmers to build and manage modern applications. NoSQL solutions prove highly effective when vast amounts of data are required to be retrieved and stored, when there is no requirement for constraints and joint support at the database level, or when data is dynamic and unstructured. Consequently, they find extensive utilization across numerous sectors, such as banking, healthcare, telecommunication, government, retail, etc.
NoSQL Market Trends:
The increasing user-created content, on account of the rising influence of social media, rapid penetration of high-speed internet, and easy access to smartphones, that has led to the generation of big datasets is primarily driving the NoSQL market. In addition to this, the growing need for improved management and analysis of data that are generally sparse and unstructured is acting as another significant growth-inducing factor. Besides this, the escalating integration of NoSQL systems with the industry 4.0 landscape to enhance the possibility of high scalability, distributed computing, lower cost, and schema flexibility is also positively influencing the global market. Moreover, the introduction of non-relational databases that are being increasingly employed in the financial sector to integrate data in a more useful and faster manner while incurring lesser costs is expected to propel the NoSQL market over the forecasted period.
NoSQL Market 2023-2028 Competitive Analysis and Segmentation:
Competitive Landscape With Key Players:
The competitive landscape of the NoSQL market has been studied in the report with the detailed profiles of the key players operating in the market.
Some of these key players include:
• Aerospike
• Amazon Web Services
• Apache Cassandra
• Basho Technologies
• Cisco Systems
• Couchbase, Inc
• Hypertable Inc.
• IBM
• MarkLogic
• Microsoft Corporation
• MongoDB Inc.
• Neo Technology Inc.
• Objectivity Inc.
• Oracle Corporation
Key Market Segmentation:
The report has segmented the global NoSQL market based on database type, technology, vertical, application and region.
Breakup by Database Type:
• Key-Value Based Database
• Document Based Database
• Column Based Database
• Graph Based Database
Breakup by Technology:
• MySQL
• Database
• Oracle
• Relational Database Management Systems (RDBMS)
• ACID
• Metadata
• Hadoop
• Others
Breakup by Vertical:
• BFSI
• Healthcare
• Telecom
• Government
• Retail
• Others
Breakup by Application:
• Data Storage
• Metadata Store
• Cache Memory
• Distributed Data Depository
• e-Commerce
• Mobile Apps
• Web Applications
• Data Analytics
• Social Networking
• Others
Breakup by Region:
• North America
• Asia Pacific
• Europe
• Latin America
• Middle East and Africa
Ask Analyst for Customization and Explore Full Report with TOC & List of Figures: https://www.imarcgroup.com/request?type=report&id=2040&flag=C
Key Highlights of the Report:
• Market Performance (2017-2022)
• Market Outlook (2023-2028)
• Market Trends
• Market Drivers and Success Factors
• Impact of COVID-19
• Value Chain Analysis
• Comprehensive mapping of the competitive landscape
If you need specific information that is not currently within the scope of the report, we will provide it to you as a part of the customization.
Browse Related Reports:
Tax Automation Software Market 2023-2028
Anti-Money Laundering (AML) Software Market 2022-2027
About Us
IMARC Group is a leading market research company that offers management strategy and market research worldwide. We partner with clients in all sectors and regions to identify their highest-value opportunities, address their most critical challenges, and transform their businesses.
IMARC’s information products include major market, scientific, economic and technological developments for business leaders in pharmaceutical, industrial, and high technology organizations. Market forecasts and industry analysis for biotechnology, advanced materials, pharmaceuticals, food and beverage, travel and tourism, nanotechnology and novel processing methods are at the top of the company’s expertise.
Our offerings include comprehensive market intelligence in the form of research reports, production cost reports, feasibility studies, and consulting services. Our team, which includes experienced researchers and analysts from various industries, is dedicated to providing high-quality data and insights to our clientele, ranging from small and medium businesses to Fortune 1000 corporations.
Elena Anderson
IMARC Services Private Limited
+1 631-791-1145
email us here
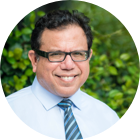
MMS • RSS
Posted on nosqlgooglealerts. Visit nosqlgooglealerts
Versie 22.3.4 van DBeaver is uitgekomen. Met dit programma kunnen databases worden beheerd. Het kan onder andere query’s uitvoeren en data tonen, filteren en bewerken. Ondersteuning voor de bekende databases, zoals MySQL, Oracle, DB2, SQL Server, PostgreSQL, Firebird en SQLite, is aanwezig. Het is verkrijgbaar in een opensource-CE-uitvoering en drie verschillende commerciële uitvoeringen. Deze voegen onder meer ondersteuning voor verschillende nosql-databases toe, zoals MongoDB, Apache Cassandra en Apache Hive, en bevatten verder extra plug-ins en jdbc-drivers. De changelog sinds versie 22.3.2 ziet er als volgt uit:
Changes in DBeaver version 22.3.4:
- ChatGPT integration for smart completion and code generation (as optional extension)
- Accessibility:
- Text reader for entity editor was improved
- Text reader for data grid was improved
- SQL editor:
- Query generation from human language text was added
- Server output log levels configuration was improved
- Global metadata search was fixed
- Variables resolution is fixed in strings and comments
- Issue with queries with invalid line feeds was resolved
- Data editor:
- Grouping panel messages were improved
- Datetime calendar editor was fixed (in panel and inline editor)
- Database navigator: issue with rename/refresh was resolved
- Dashboards were fixed for inherited databases
- Project import now sets DBeaver nature (thanks to @froque
- ERD: custom diagram editor was fixed (issue wth missing notes and connections was resolved)
- Databricks: table DDL reading was fixed, extra SQL keywords were added (thanks to @mixam24)
- DB2 BigSQL: table with RID_BIT columns data reading was fixed (thanks to @bkyle)
- MySQL: numeric identifiers quoting was fixed
- Netezza: tables/views search query was improved
- PostgreSQL:
- Procedures invocation was improved (thanks to @plotn)
- Filter by enum was fixed (thanks to @plotn)
- Redshift: triggers DDL reading was fixed
- Snowflake: table constraints reading was fixed
- We switched to Java 17 so now DBeaver supports all newest JDBC drivers
Changes in DBeaver version 22.3.3:
- SQL editor:
- Output logs viewer now respects log levels and supports log search
- Auto-completion for mixed case schema name was fixed
- Result tabs count message was fixed
- Auto-completion of cell values is now configurable
- Query execute time is now populated in statistics (thanks to @bob27aggiustatutto)
- Mixed-case variables resolution was fixed
- Execute statistics UI was fixed (redundant info was removed)
- NPEs during auto-completion were fixed
- Data editor:
- Column name ordering in record mode was fixed
- Chart type configuration added to the context menu
- Issue with column focus in context menus was fixed
- General UI:
- Database navigator now respects font size customization
- Dashboard graphs now support dark themes
- DB2: client application name population was fixed
- Exasol: privileges reading was fixed for SaaS (thanks to @allipatev)
- MySQ:/MariaDB: schema privileges save was fixed (issue with UI refresh)
- Oracle: complex data types resolution was fixed for resultsets
- PostgreSQL:
- Full backup with roles and groups is now supported
- View triggers DDL was added
- Procedure debugger was fixed
- Issue with URL-baes connections backup was resolved
- Vector data types support was fixed
- Aggregate functions DDL was fixed
- Redshift: incorrect schemas info read in different databases was fixed
- Snowflake:
- Java 17 support has been added
- Complex DDL statements parser was improved
- SQL Server: procedures and functions rename was fixed
- Vertica: comments read can e disabled for all metadata (for performance)
Versienummer | 22.3.4 |
Releasestatus | Final |
Besturingssystemen | Windows 7, Linux, macOS, Windows 8, Windows 10, Windows 11 |
Website | DBeaver |
Download | https://dbeaver.io/download/ |
Licentietype | Voorwaarden (GNU/BSD/etc.) |
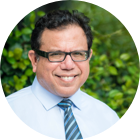
MMS • Alex Soto
Article originally posted on InfoQ. Visit InfoQ
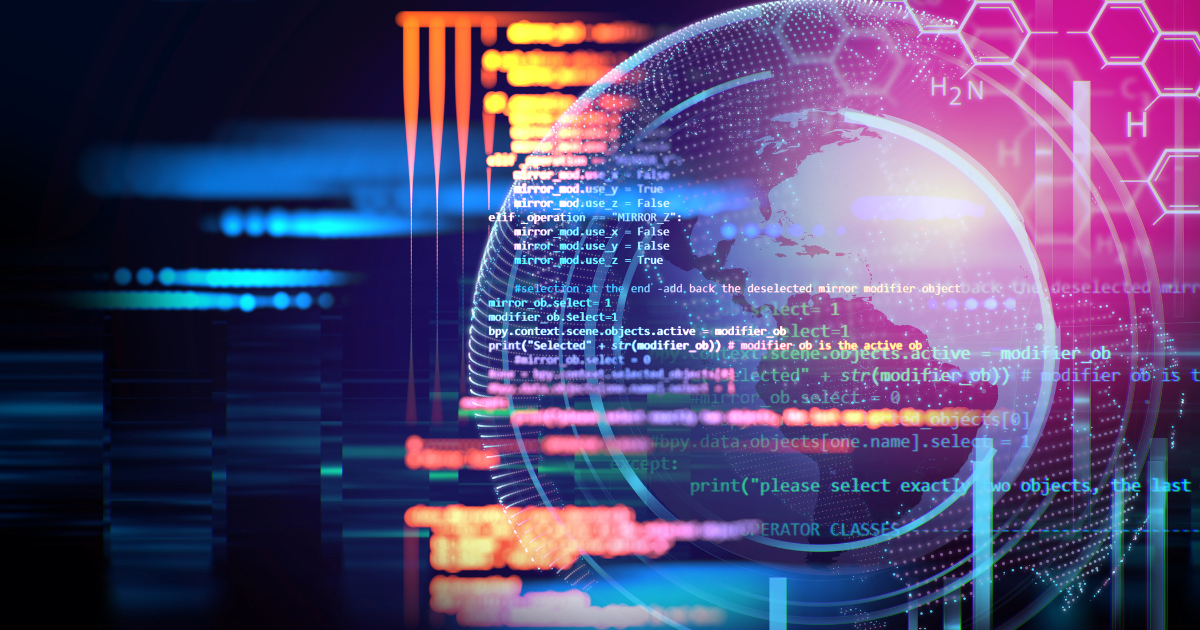
How data is processed/consumed nowadays is different from how it was previously practiced. In the past, data was stored in a database and it was batch processed to get some analytics. Although this approach is correct, more modern platforms let you process data in real-time as data comes to the system.
Apache Kafka (or Kafka) is a distributed event store and stream-processing platform for storing, consuming, and processing data streams.
One of the key aspects of Apache Kafka is that it was created with scalability and fault-tolerance in mind, making it appropriate for high-performance applications. Kafka can be considered a replacement for some conventional messaging systems such as Java Message Service (JMS) and Advanced Message Queuing Protocol (AMQP).
Apache Kafka has integrations with most of the languages used these days, but in this article series, we’ll cover its integration with Java.
The Kafka Streams project helps you consume real-time streams of events as they are produced, apply any transformation, join streams, etc., and optionally write new data representations back to a topic.
Kafka Streams is ideal for both stateless and stateful streaming applications, implements time-based operations (for example grouping events around a given time period), and has in mind the scalability, reliability, and maintainability always present in the Kafka ecosystem.
But Apache Kafka is much more than an event store and a streaming-processing platform. It’s a vast ecosystem of projects and tools that fits solving some of the problems we might find when developing microservices. One of these problems is the dual writes problem when data needs to be stored transactionally in two systems. Kafka Connect and Debezium are open-source projects for change data capture using the log scanner approach to avoid dual writes and communicate persisted data correctly between services.
In the last part of this series of articles, we’ll see how to provision, configure and secure an Apache Kafka cluster on a Kubernetes cluster.
Series Contents
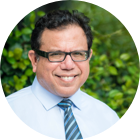
MMS • Andrea Messetti
Article originally posted on InfoQ. Visit InfoQ
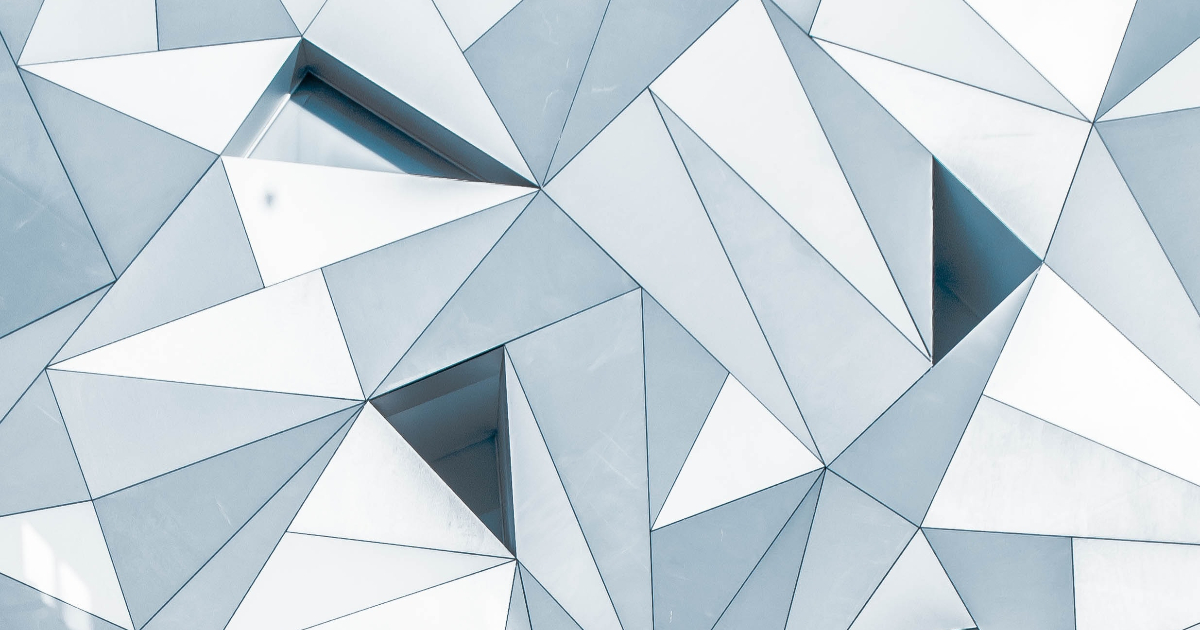
JobRunr, a Java library designed to handle background tasks in a reliable manner within a JVM instance, released the new version 6.0 after a year of development since the release of version 5.0 in March 2022.
With the latest update, JobRunr introduces several new features, including JobBuilders which allow developers to configure all aspects of a job using a builder API:
jobScheduler.create(aJob()
.withName("My Scheduled Job")
.scheduleAt(Instant.parse(scheduleAt))
.withDetails(() -> service.doWork()));
And JobLabels which allow tagging of jobs with custom labels:
@Job(name="My Job", labels={"fast-running-job", "tenant-%0"})
void myFastJob(String tenandId) {
// your business logic
}
The server name is now also visible in the dashboard, along with MDC Support for logging during the success and failure of a job. It also comes with the support of Spring Boot 3 AOT with a new starter.
In terms of performance improvements, JobRunr has optimized the enqueueing of jobs and integrated MicroMeter for metrics tracking of enqueued, failed and succeeded jobs on each BackgroundJobServer
but this is now opt-in. Additionally, the library has made stability improvements to handle exceptions and prevent job processing in case of a database outage.
Created by Ronald Dehuysser, JobRunr was first introduced to the Java community in April 2020. It provides a unified programming model for creating and executing background jobs with minimum dependencies and low overhead. Jobs can be easily created using Java 8 lambdas, which can be either CPU or I/O intensive, long-running or short-running.
JobRunr guarantees execution by a single scheduler instance using optimistic locking, ensuring that jobs are executed only once. For persistence, it supports both RDBMS (Postgres, MariaDB/MySQL, Oracle, SQL Server, DB2, and SQLite) and NoSQL (ElasticSearch, MongoDB, and Redis). The library has a minimal set of dependencies, including ASM, slf4j, and either Jackson, gson, or a JSON-B compliant library. It also includes a dashboard to control the tasks running in the background and see the job history.
The Pro version adds the capability to define priority queues and to create background jobs in batch.
In conclusion, JobRunr is a simple and effective library for handling background jobs in Java applications. Its ability to support different storage options, optimized performance, and enhanced stability makes it a suitable choice for a wide range of use cases. JobRunr can manage the execution of background tasks, whether they are deployed in the cloud, on shared hosting or in a dedicated environment.
Java News Roundup: Helidon 4.0-Alpha4, Spring, GlassFish, Quarkus, Ktor, (Re)Introducing RIFE2
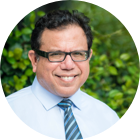
MMS • Michael Redlich
Article originally posted on InfoQ. Visit InfoQ
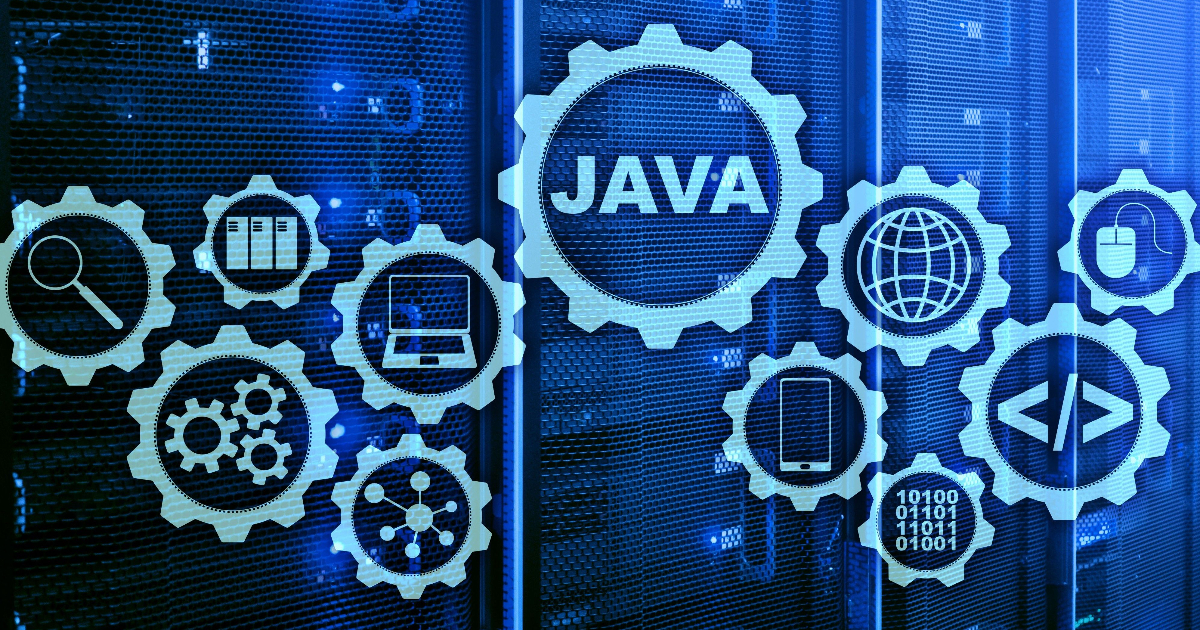
This week’s Java roundup for January 30th, 2023 features news from JDK 20, JDK 21, Spring Tools 4.17.2, GlassFish 7.0.1, Quarkus 2.16.1, Helidon 4.0.0.-ALPHA4, Hibernate Search 6.1.8 and 5.11.12, PrimeFaces 11.0.10 And 12.0.3, Apache Commons CSV 1.10.0, JHipster Lite 0.27.0, Ktor 2.2.3 and (re)introducing RIFE2 1.0.
JDK 20
Build 34 of the JDK 20 early-access builds was made available this past week, featuring updates from Build 33 that include fixes to various issues. More details on this build may be found in the release notes.
JDK 21
Build 8 of the JDK 21 early-access builds was also made available this past week featuring updates from Build 7 that include fixes to various issues. More details on this build may be found in the release notes.
For JDK 20 and JDK 21, developers are encouraged to report bugs via the Java Bug Database.
Spring Framework
The release of Spring Tools 4.17.2 delivers bug fixes and improvements such as: a NullPointerException
from the OpenRewrite Java Parser; update the generated parser for Java properties with latest version of ANTLR runtime; provide more information about the definition of “Java sources reconciling;” and execution of the upgrade recipe for Spring Boot 3.0 throws an exception. More details on this release may be found in the release notes.
GlassFish
The Eclipse Foundation has released GlassFish 7.0.1 featuring: dependency upgrades; an overhaul of some class loader mechanics to speed up operations; and a more reliable monitoring of server shutdown. GlassFish 7 is compatible with Jakarta EE 10 with JDK 11 as a minimal version. However, it compiles and runs on JDK 11 to JDK 19 with success of initial tests on Build 30 of the JDK 20 early-access builds.
Quarkus
Less than a week after the release of Quarkus 2.16.0, Quarkus 2.16.1.Final, a maintenance release that was made available to the Java community. This release ships with bug fixes, improvements in documentation and dependency upgrades. The format for Micrometer metrics has migrated to Prometheus. More details on this release may be found in the changelog.
Helidon
Oracle has released Helidon 4.0.0-ALPHA4 that delivers support for Helidon MP on Helidon Níma, a microservices framework based on virtual threads, and provides full support of MicroProfile 5.0-based applications working on virtual threads. Other notable changes include: a more efficient web server shutdown strategy; a deprecation of the MicroProfile Tracing specification; and enhancements to the Helidon builders. More details on this release may be found in the release notes.
Hibernate
Versions 6.1.8.Final and 5.11.12.Final of Hibernate Search were made available this past week.
Version 6.1.8 features: automatic reindexing will no longer be skipped when changing a property annotated with @OneToOne(mappedBy = ...)
@IndexedEmbedded
; regular testing of Hibernate Search 6.1 for compatibility with Hibernate ORM 6.2; and dependency upgrades to Hibernate ORM 5.6.12.Final and Jackson 2.13.4.
Version 5.11.12 features an updating/deleting of entities in one tenant will no longer remove entities with the same ID from the index for other tenants.
PrimeFaces
PrimeFaces 12.0.3 and 11.0.10 have been released delivering fixes such as: an implementation of between
and notBetween
values for the filterMatchMode
property within the JpaLazyDataModel
class; the cookie name that violates the Open Web Application Security Project (OWASP) Rule 941130; and the convertToType()
method defined in the JpaLazyDataModel class throws a FacesException
for java.util.Date
; More details on these releases may be found in the list of issues for version 12.0.3 and version 11.0.10.
Apache Software Foundation
Apache Commons CSV 1.10.0 has been released with notable changes such as: the get(Enum)
method defined in the CSVRecord
class should use the name()
method instead of the toString()
method from the Enum
class; the toList()
method defined in the CSVRecord
class does not provide write access to a newly-created List
; and identify duplicates in null, empty and blank header names in the CSVParser
class. More details on this release may be found in the release notes.
JHipster
JHipster Lite 0.27.0 has been released featuring: a refactor of the bootstrapping; support for Apache Cassandra; a new inject()
function and self-closing component tags defined in the Angular frontend; and a number of dependency upgrades, the most notable of which is Angular 15.1.3.
The JHipster team has completed a migration to the authorizeHttpRequests()
method defined within the HttpSecurity
class of Spring Security 6.0 that migrates from an allow-by-default to a deny-by-default behavior for increased security.
JetBrains
JetBrains has released version 2.2.3 of Ktor, the asynchronous framework for creating microservices and web applications, that include improvements such as: the FileStorage
function throws a FileNotFoundException
when the request path is long; the HttpRequestRetry
retries on the FileNotFoundException
thrown by FileStorage
; and a multipart File
doesn’t upload the whole file and throws an “Unexpected EOF: expected 4096 more bytes” for larger files. More details on this release may be found in the what’s new page.
RIFE2
Geert Bevin, software engineering and product manager at Moog Music, has revamped and reintroduced his original RIFE framework, active from 2000-2010, with version 1.0.0 of RIFE2, a full-stack framework to create web applications with modern Java. Version 1.0.0 is the initial stable release that includes: a redesign and rework of the continuations workflow engine; internal concurrency fixes and improvements; a safety check to prohibit routing changes after deployment; and a new MemoryResources
class that offers capabilities from implementations of the ResourceFinder
and ResourceWriter
interfaces for resources that are stored in a memory. InfoQ will follow up with a more detailed news story.
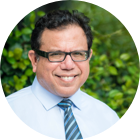
MMS • Aditya Kulkarni
Article originally posted on InfoQ. Visit InfoQ
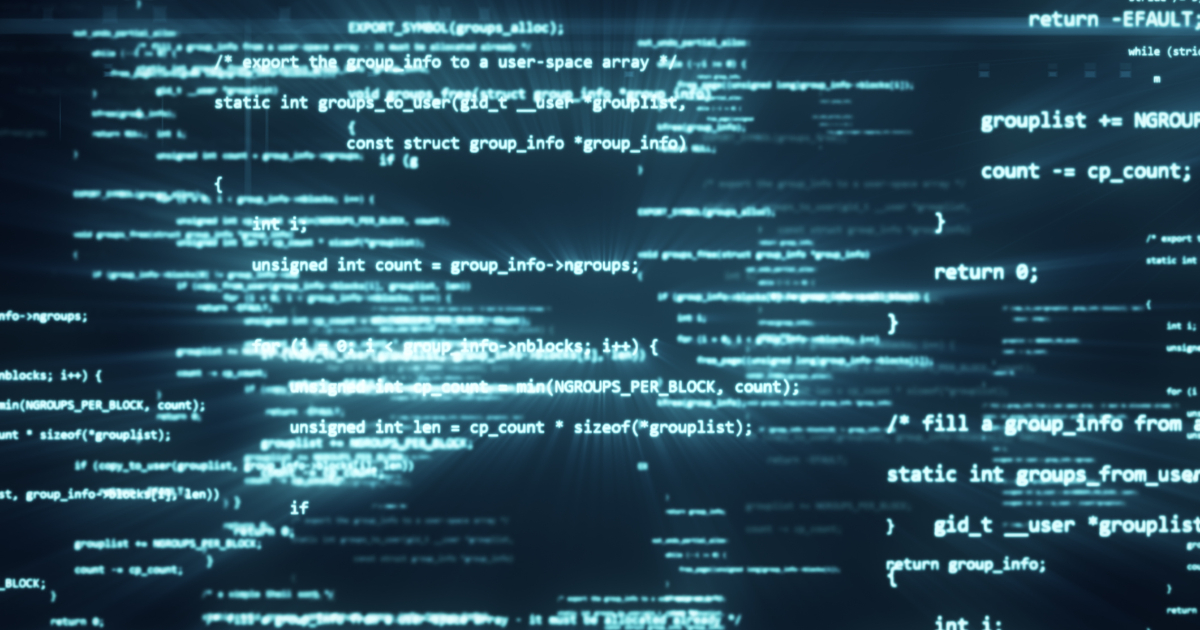
Octosuite, an open-source intelligence (OSINT) framework, recently released its latest version 3.1.0. Octosuite provides a wide range of commands to investigate publicly-visible GitHub accounts and repositories through GitHub’s Public APIs.
Written in Python, Octosuite provides a secure and user-friendly interface to easily search and explore data related to a repository, organization, or user. The search feature also looks for topics, commits, and issues to quickly locate relevant data. All the results of searches are exported in a comma-separated value (CSV) readable format.
Source – Octosuite: A New Tool to Conduct Open Source Investigations on GitHub – bellingcat
Users can get started with Octosuite through a command-line interface (CUI) or graphical user interface. While CLI is more flexible in processing the batch processing of data, GUI allows users to search commands from a dropdown menu. The installation guide for Octosuite is available here.
Once Octosuite is installed, the user needs to run octosuite
in the terminal. At the time of launch, Octosuite will attempt to create three directories – .logs
for storing logs of each session, output
to save CSV files, and download
folder where the source
code from the source command will be saved.
To use different capabilities like getting user profile or organization profile info, search, log, and CSV management, Octosuite has subcommands. Some subcommands in the context of searching users are provided below:
Search Users
------------
octosuite --method users_search --query
Search Issues
-------------
octosuite --method issues_search --query
Search Commits
--------------
octosuite --method commits_search --query
Search Topics
-------------
octosuite --method topics_search --query
Search Repositories
-------------------
octosuite --method repos_search --query
We are seeing that the Open Source Intelligence market is expected to experience significant growth over the next five years, with around 26% of organizations using open-source investigation tools already. As a side, readers can also refer to this list of OSINT resources.
Octosuite is an important tool for open-source investigators, security researchers, and anyone who needs to analyze and investigate data stored on GitHub quickly. For example, Octosuite can be used to investigate incidents like the 2022 GitHub Malware Attack, where more than 35,000 repositories were affected by a single user account.
Bellingcat Tech Team, creator of Octosuite has encouraged feedback from the community about the tool. Users can fill out this form to share how they’ve used Octosuite in their research or investigation.
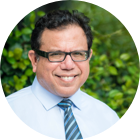
MMS • Sergio De Simone
Article originally posted on InfoQ. Visit InfoQ
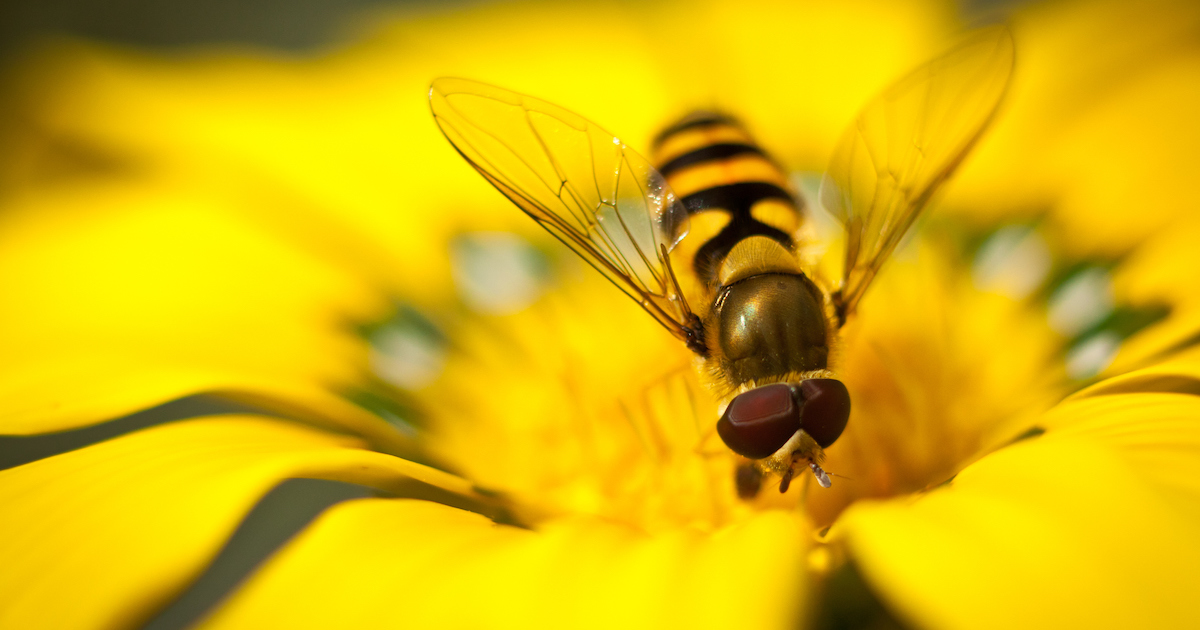
The latest release of the Go language, Go 1.20, improves compiler performance, bringing it back in line with Go 1.17. Additionally, the language now supports conversion from slice to arrays and revises struct
comparison.
The introduction of generics in Go 1.18 made compiler performance worse in comparison with Go 1.17. This was due mostly to changes in front-end processing, including the generics type checker and the successive parsing phase.
Go 1.18 and 1.19 saw regressions in build speed, largely due to the addition of support for generics and follow-on work. Go 1.20 improves build speeds by up to 10%, bringing it back in line with Go 1.17.
With this improvement, the process to introduce generics in Go can now be considered complete, going from an initial, functionally complete, generics implementation in Go 1.18, to runtime performance improvements in Go 1.19, to this final step to bring compile performance back in line with pre-generics Go’s.
It is worth to observe that generics may have an impact in runtime performance depending on their usage. This is more directly related to the specifics of Go’s generics implementation and is mostly noticeable when passing an interface to a generic function. The workaround for this performance hit is using pointers instead of interfaces as generic function arguments.
At the language syntax level, Go makes it easier now to convert a slice into an array. A slice in Go is a special variant of an indexed, contiguous collection that is not typed by the number of elements it contains, as Go arrays are. Converting a slice into an array, thus just requires augmenting the type associated to the slice with the number of slice items you want to use in the array. This was previously possible by applying an intermediate conversion from a slice into an array pointer into an array. Both ways are shown in the following snippet:
var aSlice = []int{ 1, 2, 3 }
var anArray = [2]int(aSlice)
var anotherArray = *(*[2]int)(aSlice) //-- old way
In Go 1.20, struct
value comparison takes into account the order of the fields and stops at the first mismatch between fields, which are compared one at a time. This explicitly rules out any possibility of confusion present in the previous specification that could lead to consider that all fields should be compared, even beyond the first mismatch.
As a final note, Go 1.20 extends code coverage support to whole programs instead of just unit tests, as it was previously. This option can be enabled with the -cover
flag, which instruments a build target for code coverage. Previous versions of Go required to create a dummy unit test and execute main
from it to attain this same result.
Go 1.20 includes many more improvements and changes than what can be included here. Do not miss the official release notes for the full detail.
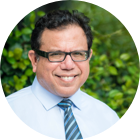
MMS • Claudio Masolo
Article originally posted on InfoQ. Visit InfoQ
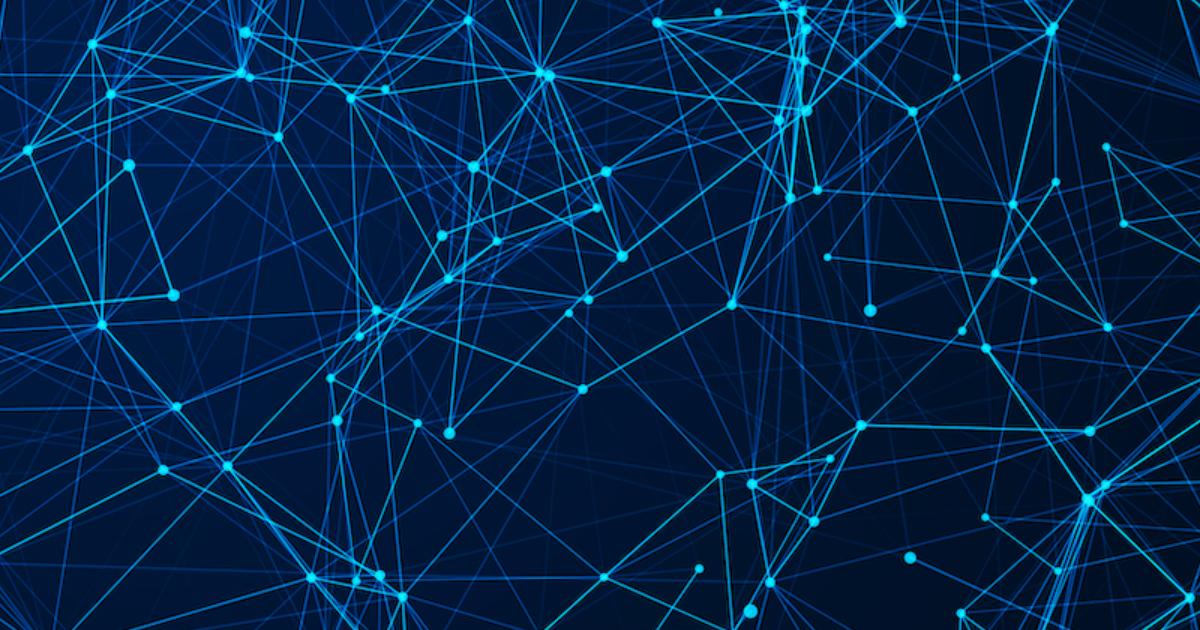
Infrastructure-from-Code (IfC) is an approach that creates, configures, and manages cloud resources understanding a software application’s source code, without explicit description. There are 4 primary approaches to Infra-from-Code: SDK-based, code annotation based, a combination of these two, and a new programming language that defines the infrastructure explicitly.
The SDK-based approach allows developers to use their code and, at deployment time, the tools analyze how the service code uses the SDK and generates the infrastructure. The SDK-based approach makes inferring usage from code more predictable but the SDK is always a step behind in leveraging the new cloud features. An example of SDK-based tools are Ampt and Nitric.
import { api } from '@nitric/sdk';
const helloApi = api('main');
helloApi.get('hello/:name', async(ctx) => {
const {name} = ctx.req.params;
ctx.res.body = 'Hello ${name}';
})
Nitric example of exposing endpoint to the Internet
The pure annotations approach is based only on in-code annotation. This approach is focused on understanding the developer’s use of framework and tools. The leading tool of this approach is Klotho which is more of an Architecture-from-code tool. Klotho introduces the capabilities (key annotations) like expose
, persist,
and static_unit
, that make existing programming languages cloud native.
const redis = require("redis");
/**
* @klotho:persist{
* id = "UserDB"
*}
*/
const client = redis.createClient();
Example of Klotho data persist for redis client
With the annotations and SDK approach, the developer annotates the code and the tools incorporate those into the framework. The principal tools for this category are Encore and Shuttle. These tools can be hosted on the IfC vendor’s platform or may be integrated with third-party cloud providers like GCP, AWS, or Azure. Another interesting tool is AWS Chalice which allows the creation and deploys applications that use AWS Lambda in python.
// encore:api public method=POST path=/url
func Shorten(ctx context.Context, p *ShortenParams)(*URL, error){
id, err := generateID()
if err != nil {
return nil, err
}
return &URL(ID: id, URL: p.URL), nil
}
Encore example for API request/response. The annotation specifies the URL path
The language-based approach introduces new programming languages that aim to be cloud-centric. Wing and DarkLang are the two most used programming languages. This approach allows the introduction of concepts that would be difficult to achieve in existing programming languages. A new programming language has some tradeoffs: software developers need to first learn it and then integrate it into existing tools and services. In addition, finding and hiring developers with expertise in a new programming language can take time and effort.
bring cloud;
let bucket = new cloud.Bucket();
new cloud.Function(inflight (_: str): str => {
bucket.put("hello.txt","world");
}
Wing example of cloud function definition
Chef, Ansible, Puppet, and Terraform were some of the first tools for Infrastructure-as-Code (IaC) and started to enable the creation and management of cloud infrastructures. The second wave of IaC used existing programming languages (Python, Go, TypeScript), to express the same idea as the tools of the first wave. Puluni and CDK are tools of this second generation.
For more details on the current state of Infrastructure from Code, readers are directed to Klotho’s State of Infrastructure from Code 2023 report.