Month: March 2023
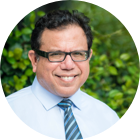
MMS • Sergio De Simone
Article originally posted on InfoQ. Visit InfoQ
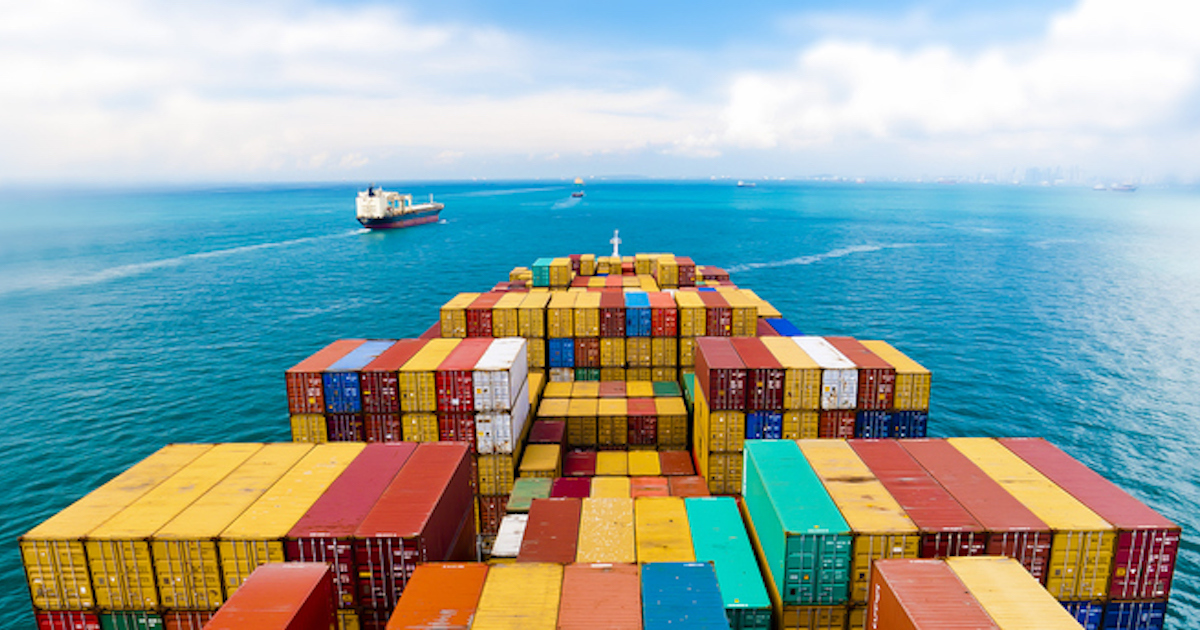
Docker has announced the second technical preview of Docker+Wasm, aiming to make it easier to run Wasm workloads and extending runtime support by including Fermyon’s spin, Deislabs’ slight, and Bytecode Alliance’s wasmtime runtime engines.
The three new Wasm engines in Docker+Wasm bring the total number of supported runtimes to four, including WasmEdge, which was already supported in Docker+Wasm technical preview 1. All of them are based on the runwasi
library, which recently joined the containerd
project.
runwasi
is a Rust library that enables running wasm workloads managed through containerd, thus effectively creating the abstraction of a new container type in addition to the Linux container originally supported by containerd. Runwasi as its name implies is based on WASI
, a modular system interface for WebAssembly providing a common platform for Wasm runtimes. This means that if a program is compiled to target WASI, it can be run on any WASI-compliant runtime.
A Wasm container typically only includes a compiled Wasm bytecode file, without requiring any additional binary libraries, which makes it much smaller. This also implies a Wasm container is usually much faster to startup and more portable than Linux containers. For example, as WasmEdge co-founder Michael Yuan noted on Twitter, while a “slim” Python container image for Linux is over 40MB, its Wasm container counterpart takes less than 7MB.
Being Wasm containers directly supported by containerd, in order to try out Docker+Wasm technical preview 2 in Docker Desktop latest release, the only required thing is enabling the “Use containerd” option under Settings > Features in development.
To run a Wasm container using wasmtime, you can then execute:
$ docker run --rm --runtime=io.containerd.wasmtime.v1
--platform=wasi/wasm secondstate/rust-example-hello:latest
Thanks to this, Wasm containers can be run side by side with Linux containers using Docker Compose or other orchestration platforms such as Kubernetes. Additionally, Docker Desktop is able to package a Wasm app into an OCI container by embedding a Wasm runtime in it to enable to share it through a container registry such as DockerHub and others.
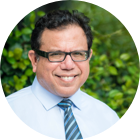
MMS • Steef-Jan Wiggers
Article originally posted on InfoQ. Visit InfoQ
Google recently announced the general availability of Google Distributed Cloud (GDC) Hosted, an offering for customers with the most stringent requirements, including classified, restricted, and top-secret data. It complements Google Distributed Cloud Edge and Google Distributed Cloud Virtual, which generally became available in 2022.
GDC Hosted provides a comprehensive managed cloud solution, which includes the hardware, software, local control plane, and operational tooling necessary for deployment, operation, scalability, and security. In addition, it seamlessly integrates with commonly utilized third-party software and systems, including hardware security modules for added protection, IT service management tools for built-in ticketing, observability tools for monitoring, and popular DevSecOps tools to boost developer, security, and operator productivity.
According to the company, financial services, healthcare, manufacturing, and utility customers can benefit from GDC Hosted. These benefits include:
- Full isolation: GDC Hosted is air-gapped and does not require connectivity to Google Cloud or the public internet at any time to manage the infrastructure, services, APIs, or tooling. This ensures that data remains secure and private.
- Integrated cloud services: GDC Hosted delivers Google Cloud services, including data and machine learning technologies.
- Data sovereignty: GDC Hosted allows customers to control their data entirely and meet strict data security and privacy requirements.
- Open ecosystem: GDC Hosted is designed around Google Cloud’s open cloud strategy. It is built on the Kubernetes API and uses leading open-source components in its platform and managed services.
- Flexibility: GDC Hosted offers customers the flexibility to deploy a completely managed cloud in their own data centers or other facilities while taking advantage of cloud services’ functionality, flexibility, and scale.
GDC Hosted can be compared to other hybrid cloud solutions such as AWS Outposts and Azure Stack. These solutions also aim to integrate on-premises resources with public cloud services and provide a common workload deployment process and APIs for both on-premises and cloud-based environments.
Regarding the GA release of GDC Hosted, Google also tweeted via their Google Cloud Tech account:
With this announcement, we also have a set of unique hardware options, which come in two form factors: (1) rack-based configurations and (2) GDC Edge Appliances.
Lastly, the GDC Hosted evaluation configuration pricing starts at $300,000 monthly. In addition, GDC Hosted offers additional flexible commercial models and provides cloud billing capabilities, including labeling of resources, enabling budgeting, and chargeback between different organizations.
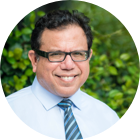
MMS • Renato Losio
Article originally posted on InfoQ. Visit InfoQ
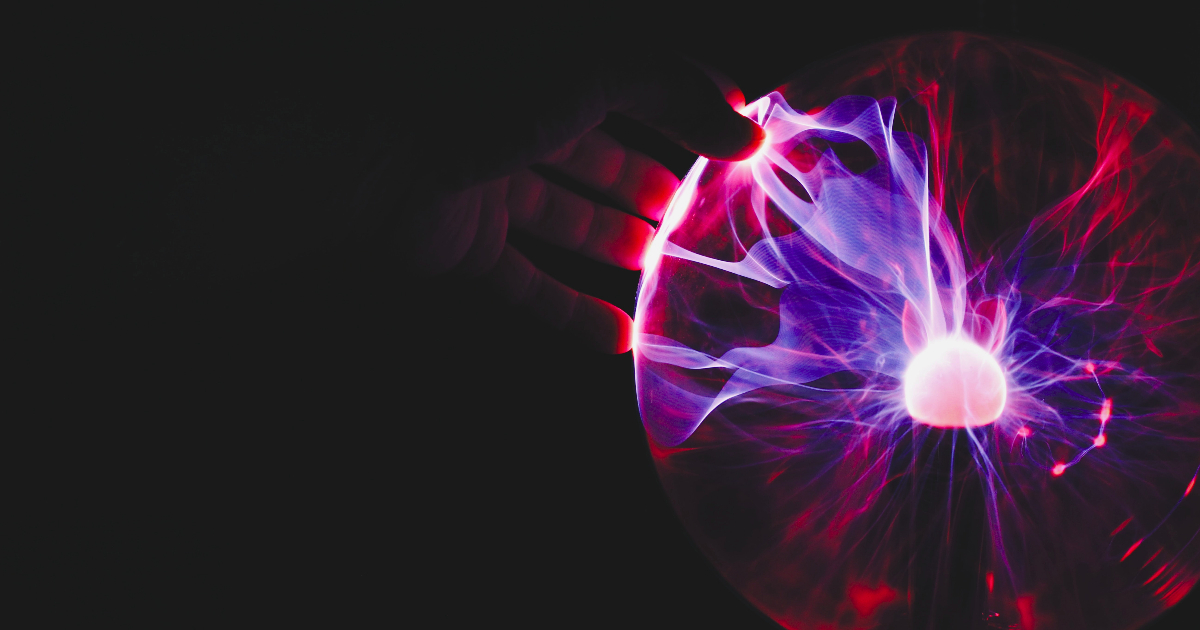
During the latest Pi Day, AWS announced Mountpoint for Amazon S3, an open-source file client to deliver high throughput access on Amazon S3. Currently in alpha, the local mount point provides high single-instance transfer rates and is primarily intended for data lake applications.
Mountpoint for Amazon S3 translates local file system API calls to S3 object API calls like GET and LIST. The client supports random and sequential read operations on files and the listing of files and directories. The alpha release does not support writes (PUTs) and the client is expected to only support sequential writes to new objects in the future.
James Bornholt, scholar at AWS and assistant professor at the University of Texas, Devabrat Kumar, senior product manager at AWS, and Andy Warfield, distinguished engineer at AWS, acknowledge that the client is not a general-purpose networked file system, and comes with some restrictions on file operations and write:
Mountpoint is designed for large-scale analytics applications that read and generate large amounts of S3 data in parallel but don’t require the ability to write to the middle of existing objects. Mountpoint allows you to map S3 buckets or prefixes into your instance’s file system namespace, traverse the contents of your buckets as if they were local files, and achieve high throughput access to objects.
The open-source client does not emulate operations like directory renames that would require many S3 API calls or POSIX file system features that are not supported in S3 APIs.
Mountpoint for S3 is not the first client presenting S3 as a filesystem, with Goofys and s3fs popular open-source options to mount a bucket via FUSE. While some developers question on Reddit the need for a new client and worry that it will be used outside the data lake space, Bornholt, Kumar and Warfield write:
Mountpoint is not the first file client for accessing S3—there are several open-source file clients that our customers have experience with. A common theme we’ve heard from these customers, however, is that they want these clients to offer the same stability, performance, and technical support that they get from S3’s REST APIs and the AWS SDKs.
Built in Rust on the Common Runtime (CRT) used by most AWS SDKs, the new client relies on automated reasoning to validate the file system semantics. Corey Quinn, chief cloud economist at The Duckbill Group, tweets:
Oh no, what has AWS done? I didn’t spend fifteen years yelling at people not to use S3 as a file system just to be undone by the S3 team itself!
Ben Kehoe, cloud expert and AWS Serverless Hero, warns:
Thinking about S3 using file concepts is going to mislead you about the semantics of the API, and you will end up making the wrong assumptions and being sad when your system is always slightly broken because those assumptions don’t hold.
Released under the Apache License 2.0, Mountpoint is not yet ready for production workloads. The initial alpha release and the public roadmap are available on GitHub.
Presentation: Blazing Fast, Minimal Change – Speed Up Your Code by Refactoring to Rust
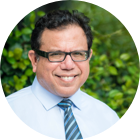
MMS • Lily Mara
Article originally posted on InfoQ. Visit InfoQ
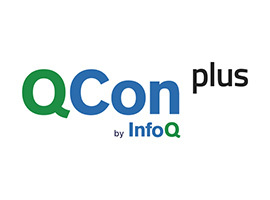
Transcript
Mara: My name is Lily Mara. I’m an engineering manager at OneSignal in San Mateo, California. I’ve been using Rust professionally on side of desk projects since about 2017. In 2019, I started at OneSignal, where it’s used as a primary language. I spoke at RustConf 2021, about the importance of not over-optimizing our Rust programs. I’ve spoken at many Rust meetup groups. I’m the author of the book, “Refactoring to Rust,” available at manning.com.
Rust – Full Rewrites
If you’ve heard of Rust before, you have probably heard one thing over again, you’ve probably heard that it’s pretty fast. It generally performs on par with something like C or C++. It’s way faster than some dynamic languages that we’re maybe using like Python or Ruby. If you have an older, monolithic application written in one of these languages, maybe with something like Django or Rails, then it might be tempting if you’re performance constrained to say, let’s throw this old thing out, and let’s start over with Rust. Rust is fast, we want to go fast, let’s rewrite it in Rust. This is a very tempting idea. The reality is often as they do, encroach on our perfect vision. Full rewrite projects can often be quite problematic for a number of reasons. Some of which, they can often take a lot longer than we expect. We think something’s going to take a month, it ends up taking three years. We think something is going to be really easy, we realize that the problem was so much more complicated than we realized. We can introduce new bugs, because different programming languages have different paradigms. When you’re trying to adapt old code into a new system, you can misunderstand the way the old thing worked. Full rewrites often also do not fix underlying architectural problems. This might be things like using the wrong database technology. It might also be things in the code. We think a piece of code looks ugly, because we don’t understand everything that’s going on, and we try and rewrite it in a simpler way. We realize that, there actually was a reason we did all those things in a very strange way in the original code. Full rewrite projects are often problematic.
Microservices, and FFI Refactoring
What else is available to us? There’s also microservices, of course. We can break out our monolith into multiple services. We can put the performance where it needs to be put, and leave the monolithic stuff in the monolith. If you’re working at a place that doesn’t really have a robust infrastructure for managing a bunch of microservices, and maybe you’re working at a place where there really is just one monolith rolled out on a couple of boxes, and you’re really not ready for the architectural shift of going to a bunch of microservices. What do you do in this case? We’re going to discuss the feasibility aspect in a little bit more detail later. The gist of it is that microservices are always the best option for everybody in every circumstance, of course. I would like to propose an alternative that we can use. For the purposes of having a term for it, I’m going to refer to it as FFI refactoring. FFI refactoring is where we take a little piece of the code, we rewrite it in a faster language, in this case, Rust, and we connect it to the original codebase using CFFI. This is something that’s going to be a little bit abstracted for us, and it’s going to be made a lot easier by some of the binding libraries that we’re going to be using. The underlying technology is CFFI. We’re going to refer to it as FFI refactoring. We’re also going to be using the terms host language to refer to the original programming language, and guest language to refer to the new programming language.
When is this an acceptable strategy for us to use? If you’re working at a place that has a really robust infrastructure for working with lots of microservices, then maybe consider using a microservice. If there’s an existing pattern, sitting there ready for you to use, maybe just use that existing pattern. It’s generally much easier to follow what’s already sitting there than to try and blaze a new trail. If you’re in a place where there isn’t a robust infrastructure for microservices, if architecturally shifting to microservices would be really difficult for you, or maybe you’re running code on an end user device and you don’t necessarily want to have a bunch of binaries talking to each other over local loopback networking, in order to run your program, then maybe FFI refactoring is a better option for you.
There are some unfortunate realities that we’re going to need to discuss when we’re going to FFI refactoring. Because we’re going to move to multiple languages for our program, we are probably going to be complicating our deployments a bit. Because we’re going to have to ship not just a bunch of Python and Ruby files to the servers, we’re going to have to compile Rust beforehand. We’re going to have to ship Rust dynamic library files to our servers. We’re going to have to make sure OS versions and compiler versions match up. This will get slightly more complicated. It’s possible that we can add bugs, just like with a full rewrite project, all we’re doing now is a rewrite on a smaller scale. Because we’re reimplementing code, we can of course create bugs. Because it’s on a smaller scale, the chance for that is maybe a little bit less. We will definitely have to watch out for translation bugs, because we’re moving between multiple programming languages. We don’t have to just worry about the quirks of Python, we also have to worry about the quirks of Python and Rust and the quirks of translating Python data structures to Rust data structures.
What makes a good project for an FFI refactor? As I’ve kept hammering home, it’s very similar to the discussion that we’ve been having for several years now of microservices versus monoliths. Do we want to make our big deploy even bigger or do we want to split it out into a bunch of stuff? Microservices can be great. You can scale independently. You can upgrade independently. You can deploy independently. One thing going down doesn’t necessarily take everything else down. There’s lots of reasons to use microservices. There’s also reasons to consider doing an FFI refactor. As I’ve said, if you have a monolith, and it will be difficult for you to go to a microservices based approach, maybe do FFI refactoring. Or, it may also be the case that you need to do a very slight performance bump and that could be maybe dwarfed by networking overhead. If you use an FFI refactor, you can keep everything within memory, within a single process, and you can get some serious performance benefits by doing that.
You should also consider what language you’re using as your host language. If we take a look at this little compass here, we can see there are some languages that are going to be slower than Rust, where doing an FFI refactor to Rust will probably improve performance. C and C++ generally are like on par with Rust as far as performance goes, maybe slightly faster, so doing an FFI refactor to Rust might actually decrease performance a little bit. You should also consider how the tooling is for your language. Some languages, Ruby, Python, Node.js, have really good tooling for integrating with Rust. Lua also has quite good tooling for integrating with Rust. Languages in this upper right quadrant right here are going to be really good choices for us to use for an FFI refactor.
If you’re dealing with something that has poor tooling, or something that’s really not going to get much of a performance benefit from refactoring to Rust, then you should maybe consider other options. Go is a pretty interesting choice, because Go is at a similar performance level to Rust. Rust is generally faster because it doesn’t have a garbage collector, it doesn’t have quite as heavy of a runtime. However, the tooling is not great, because if we wanted to integrate Go with Rust, we have to rely on the CFFI. Go developers can tell you that once you have to invoke the CFFI in Go, it slows down a lot. There hasn’t been a whole lot of development work on building out great Go bindings that I’m aware of at least, because people are aware that there’s this huge performance penalty that will have to be paid, if you want to do an FFI linking between Go and Rust. Generally speaking, a language like Ruby, Python, Node, Lua is going to be a really good choice, and others not so good.
Example
For the purposes of having a concrete example to talk through in the Rust, of this talk, we’re going to imagine that you’re a developer, you’re working on a Flask HTTP server application that’s written in Python. We’re just going to take a look at this one handler just so that we have something really small and concise that we can deal with. This handler, it takes in a list of numbers in a JSON request POST body. It computes several statistical properties about those numbers. It computes the range, which is the difference between the maximum and the minimum values. It computes the quartiles, which are the 25th, 50th, and 75th percentiles. It computes the mean, the average of all the numbers. It computes the standard deviation, which is something that I don’t exactly know the definition of, but statisticians tell me it’s important. Let’s see how we can do an FFI refactor, let’s see how we can redo this in Rust. Let’s go.
There is a free resource at doc.rust-lang.org/book. This is the book, “The Rust Programming Language,” written by Steve Klabnik, and Carol Nichols, Goulding. It’s available for free online. There’s a few places where I’m going to be calling out which chapter in “The Rust Programming Language” you should read through if you would like to get some more information on one of these subjects. If you are interested in Rust more generally, I would highly recommend reading through the book because it’s a pretty good book.
We’re going to get started by creating a new Rust project by running, cargo new –lib rstats. This is going to create a couple new files for us. The first one is carg.toml, which is like the package manager’s registry file, sort of like package JSON in a Node project. It’s going to create a lib.rs file. This is the entry point for our crate. Let’s open up that cargo.toml file, and we’re going to add a couple of dependencies. The first one is we’re going to add a statistics crate version 0.15 of a crate called statrs. If you noticed, in the Python code, we were actually using the statistics module from Python’s standard library. Rust has a much smaller standard library than Python’s. It basically only includes OS primitives, things like files, threads, basic timer functionality, and some networking code, as well as some generic data structures. Python has a very large standard library by comparison. We’re bringing in this statistics crate so that we have access to some statistical functions.
We’re also going to be bringing in version 0.16 of the pyo3 crate. Pyo3 is going to be used to generate the bindings that talk between Rust and Python. We’re also going to need to enable the extension module feature. This is required for making an extension module, making something that compiles Rust code into something that Python knows how to deal with. There are other features available for doing different things. You can, for example, write Rust code that runs Python code. Lots of different options available to us. We’re also going to add a little bit of metadata further up in the cargo.toml. We’re going to set the crate type to be a cdylib. Normally, when we compile Rust, we’re actually compiling code that is only useful to the same version of the Rust compiler on the same hardware architecture. Setting the crate type to cdylib will actually cause us to use C calling conventions, and this is necessary so that the Python interpreter knows how to call our functions.
Now, the general architecture of what we’re going to do here. We have our Python code. We have our Rust code. The Flask library is going to call into our Python HTTP handler, which is going to deserialize the JSON request body. It’s going to send that over across the FFI boundary into Rust, which is going to compute the statistics. Then we’re going to send that back across the FFI boundary to Python. That is going to be serialized back into JSON, which is then going to go back out to the HTTP client. We could more easily have the JSON deserialize and serialize steps happen inside of Rust. I didn’t want to do that because it goes against the spirit of this talk. The idea is that we can take one piece of functionality, and we can rewrite that one piece of functionality in Rust. In my mind, the JSON serialize, deserializing is some extra piece of work that needs to stay in Python for some reason. We’re going to keep that in Python. It’s also going to give us the opportunity to see how we can parse structured data back and forth between these two languages. Because if we were doing the JSON parsing and serializing in Rust, then we would actually just be parsing strings back and forth. That’s a little bit less interesting.
Now let’s go ahead and jump into the code. We’re going to open up the lib.rs file in the source directory, and there’s going to be a bunch of starter code in there. We’re just going to go ahead and delete all that. We’re going to create a new function called compute_stats. It’s going to take in a Vec of f64s, that is a growable array of 64-bit floating point numbers that lives on the heap. We’re going to call that numbers. What is our return type going to be on this function?
Let’s look back to the Python code. The Python code returns a JSON object that has these four properties. It has a range, quartiles, means, and stddev, standard deviation. In Rust, we generally don’t parse around anonymous dictionaries that have complex types for the values. Generally speaking, we use structs that have well typed fields. We’re going to create a new struct in our Rust code, we’re going to call it StatisticsResponse. It’s going to have those expected four fields in it. It’s going to have three f64 values for the range, the mean, and the standard deviation. It’s also going to have a quartiles field that has an array of three f64 values. This is going to match the structure of our Python code. Then we’ll set the return type of our compute_stats function to be that StatisticsResponse type. We’re now going to need to bring in a couple of types from the statrs library. These are all necessary, and I know there’s a lot of them. We’re going to bring in data, distribution, max, min, and OrderStatistics. Some of these are types. Some of these are traits, but we need to bring all of them in so that we can compute the statistics that we need to.
Jumping down, back into our compute_stats function, we’re going to take that vector of numbers and we’re going to put it into a data which is a type that we just pulled out of the statrs crate. This is necessary because a lot of the traits that we just pulled in, they can only be called on a data instance and not on a vector directly. You can also see that we marked our data as being mutable. That is because in order to compute some of these statistics, statrs is actually going to shuffle some of the elements in our data structure around. If you’re used to coming from a language like Python, or Ruby, or Java, then this might seem a little bit strange to you, because, normally, I think in those languages, if items need to be shuffled around, it’s pretty common for the library to actually make a defensive copy of whatever your input buffer is, so that as a user of that library, you’re not going to have your data changed around. Generally speaking, Rust takes the exact opposite approach, where if things need to be mutated under the hood, that will be exposed to the users so that if the original order of your data buffer was not strictly required, you don’t have to do any defensive copies at all. Your code can be just a teensy bit little faster. If you have a really big set of numbers that you’re computing statistics on, you don’t need to copy those at all, you could have a multi-gigabyte vector of numbers to compute statistics on and they’ll just be shuffled around in memory as required, instead of needing to be copied just to preserve ordering that we don’t necessarily care about.
Now we can get to filling in our StatisticsResponse. We’ll put an instance of StatisticsResponse at the end of our function, and we’ll start filling in the fields. Computing the range is relatively straightforward, very similar to what we did in Python. We’ll subtract the Max from the mean. Computing the quartiles is also pretty straightforward. We can use the lower quartile median and upper quartile functions on our data instance. Computing the mean is very straightforward, but it does have one little extra trick on it. Notice that the call here is data.mean.unwrap. What is this unwrap telling us? For that, we’re going to need to jump to the definition of the distribution trait. We can see that the mean function does not actually return a value directly, it returns an Option value. This is something that’s unique to Rust and some other ML type languages. If you’re used to coming from a different language, you’re probably used to dealing with null values. Null values are a special value that can generally be assigned to variables of any type. If you want to write code that correctly handles null values, you basically need to pepper checks all over your code. You need to repeat those checks, because any string instance or array instance or HashMap instance, might actually secretly be holding a non-value.
Rust does not have the concept of null, it doesn’t have a secret variable that can be assigned to variables of any type. Instead, Rust has a special type called Option. Instead of being a special value that can be assigned to variables of any type, an Option is a wrapper that goes around a variable. If you have an f64, for example, if you have that 64-bit floating point number, that is always guaranteed to be initialized to something, if you have a Vec, that is always guaranteed to be initialized to something. If you have an Option Vec, or an Option f64, then you have to write the code that deals with the possibility that that thing is not initialized. That could look something like this. We can use a match statement. We need to deal with the case that there’s nothing there, if we want to deal with the thing that is inside of the Option.
Comparing the two, Option versus null. Option is strongly typed. You can’t get away with forgetting to check something. You can also centralize your checks, which is really nice and really powerful. Because like I said, when you’re dealing with null values, you don’t necessarily know that the input value to a function, or the return value from a function isn’t null. Because according to the type system, it’s theoretically possible for any function in Java, or Ruby, or Python to return null. We end up repeating null checks all over the place. With Option, because it’s strongly typed, you can convert an Option back into a Vec. Then, as long as you write the rest of your code to deal with Vec, you know that it’s initialized and you never have to do that check again. It’s very convenient, and it leads to great peace of mind knowing that things are initialized.
Let’s jump back to our code and see what that one line that we made all that fuss, was about. On this line, we have data.mean, which returns an Option f64. Then we call unwrap on it. Unwrap is a function for dealing with Options. It will look at the Option, and if there’s a value present, it returns the value. If there’s no value present, it will actually panic the whole thread and make the thread unwind up to a point where there’s a panic handler. Generally speaking, in production code, you don’t want to be using unwrap, you want to be using proper handling of our Options with a match statement like we had previously. This is quick and dirty, so we’re going to use an unwrap. If you’d like some more information on using Options, you can read chapter six of “The Rust Programming Language.”
Similarly, when we calculate the standard deviation, this function also returns an Option, so we’re also going to need to use unwrap on it. Now we have our StatisticsResponse. It’s got all the fields in it, but it’s actually not very useful to us yet, because it’s a Rust function that returns a Rust data type, and we need a Python function. We need something that we can run from Python and call from our Flask HTTP handler. We don’t have it yet. Let’s do that. We’re going to need to import some more types from, this time, pyo3. Pyo3 is the Rust crate that allows us to write bindings between Python and Rust. We’re going to bring in pyo3::prelude::*. A prelude is a convention, but not necessarily a requirement for Rust crates. If there’s a lot of types and traits and macros and things that need to be brought in, in order for your crate to be really useful, it can be common for crate authors to include a module called prelude that includes all the most commonly needed things. You can use a glob import like this, as we’re doing here.
Before we can make a Python function, we actually need to make a module first, a Python module. A thing that can be imported in Python. In order to do that, we need to write a function that has the same name as our crate, which is rstats. Write a function called rstats, and we’re going to add this little annotation above it, PyModule. This is coming from the prelude of pyo3, and it is going to automatically expand at compile time into a bunch of C stuff that the Python interpreter knows how to read and knows how to turn into a module. This is going to require us to add a couple of parameters to this function that are not both going to be used, but they’re both required, based on the definition of the PyModule macro. The first one is just called Python. This is the type that comes from pyo3. It represents taking the GIL, the Global Interpreter Lock of the Python interpreter. A lot of times, if you’re constructing a Python type, you need access to the Python type. This is to prove to pyo3 that you are holding on to the GIL, because it’s easy to misuse the GIL when you’re writing Python C code. Since we’re not actually using it in this function, we’re going to prefix it with an underscore so that the Rust compiler doesn’t complain and say, you have an unused parameter on this function. Next up, we are going to add a parameter called m, and this is going to be a reference to a PyModule type. As implied, this is a reference to an empty Python module. Inside the body of this function, we are going to add our new compute_stats function to the Python module. We also need to set a return type for our PyModule function, for our rstats function, and that return type is going to be PyResult(). There’s a couple of interesting things going on in here. We’re going to jump through them real quick.
The Result type is the way that we handle errors in Rust. Rust does not have an exception system that bubbles values up and lets you catch exceptions with handlers. Instead, much like with Options, we have a result type that has two branches. It has an Ok branch, which also contains a success value inside of it. There’s an error branch that also contains an error value inside of it. These are strongly typed. If you want to assume that your function returned a successful result and get the successful result out, you have to deal with the possibility that your function returned an error. That code generally looks like this. Just like with Option, we would use a match statement. I’m going to say if it’s ok then pull the value out and do something with it, if there was an error, pull the error out and do something with it. That is Result. If you’d like more information on using the result type for error handling, you can read chapter nine of “The Rust Programming Language.” We also had something inside of the result type, we had that open parenthesis, close parenthesis. This is something called the unit type, which is an empty tuple. It’s an interesting thing that’s somewhat unique to Rust. This is somewhat similar but not exactly similar to a null value. A null value can generally be assigned to values of any type, but the unit type is actually a type in and of itself. You cannot actually assign the unit value to anything other than a variable of the unit type. It represents nothing.
If we jump back to our function, it has a PyResult return type which is actually just a wrapper type, an alias type that comes from the pyo3 crate, and it has its error side always set to being a pyo3 Python error. You just have to fill in the success side. We have our success side set to the unit type, because a result is going to communicate either a success or an error. We really only have side effects in this function. We have the side effect of defining a function in here, putting a function onto our module. There’s not like a value that we can return. We’re not fetching something from a database that might fail. There’s not a great sentinel value that we could return, so we’re going to use the unit type instead. The body of this function, we’re just going to put that Ok, that success case with the unit value inside of it. This isn’t going to define our compute_stats function in a way that Python knows how to deal with but it is going to define a Python module called rstats.
Let’s try and use it. We’ll jump back over to the Python code, we will add import rstats to the top, and we’ll try to run our Python code. We’re going to get a giant error because there’s no module named rstats that Python knows how to import. If you nested your rstats folder directly under the folder where the Python code is, this is actually going to work but it’s not actually going to be importing the module that we care about. It’s just going to be importing the directory in a way that Python can default to sometimes. The Python module system is a little confusing. We want to write something that is actually going to be importing our Rust code, not just the directory. Still on the CLI, we’re going to install a developer tool that’s created by the pyo3 team called maturin. We’re going to jump into our rstats folder, the folder with our Rust code, and we’re going to run maturin develop. This is going to compile our Rust code and generate some Python bindings for it. Now, if we run flask run, it’s going to start up successfully. There’s not going to be an error, because that Python module is going to be present. It is going to know how to import rstats.
Let’s jump back over to our Rust code and see what we can do. Let’s bring in our compute_stats function. On top of the compute_stats function, we’re going to add this pyfunction annotation. That is going to add some extra code at compile time. Once again, that is going to transform the input types and the output types into something that Python knows how to deal with. If we actually tried to compile this Rust code right now, it’s actually going to give us a huge compiler error. It’s going to say it doesn’t actually know how to turn a StatisticsResponse into something that Python knows how to deal with. We got to fix that. We can do that by adding some more of these little decorators onto our Rust code. We’re going to add the pyclass attribute macro on top of our StatisticsResponse struct. We’re going to add the pyo3(get) attribute macro on top of all the fields of our StatisticsResponse. This is necessary so that we can access all these individual fields. Otherwise, they would just be hidden from the Python side.
Next, we’re going to jump down into our module definition function, and we are going to put in this somewhat complicated line of code. I know it’s a lot to look at, but it is well documented and all those steps are necessary. We’re going to call the add_function function on our module. We’re going to parse that the results of the wrap_pyfunction macro on our compute_stats function, and that also needs access to the module. Then these question marks that are here at the end are error handling. Those are actually going to be doing an early return if those expressions fail, if they evaluate to error responses. We’re almost there. We’re so close. We have reimplemented the functionality. We have generated the Python bindings. We have exposed those bindings to Python. We have generated a Python class that we can use in order to get access to our fields. Let’s recompile our Rust code. We’ll run cargo build from the command line. We don’t need to run maturin develop again, because of the symlinks that were created. We can just recompile normally, and this is going to regenerate everything that’s required.
Now, we can do the Python refactoring. Over in the Python code, we can change up our handler a little bit. We can call rstats.compute_stats, that’s the function that we wrote and we exposed. We’ll parse it our numbers, just the normal numbers that come straight out of the requests JSON body. Then we are going to parse all of the fields from our response, from the StatisticsResponse into Flask’s jsonify function. We actually do have to work all of the fields here individually, unfortunately. Pyo3 does not automatically generate JSON deserializable Py classes, we could do it with a little bit of extra work. We’re just going to work all the fields manually. We can now boot up our flask application, and we can try running it. We can try running our HTTP handler. Let’s use curl. We’ll hit that stats endpoint, and we do get some numbers back. It’s all working, everything’s flowing great.
Let’s compare the results from our Python handler, the original Python handler that was 100% Python, as well as our refactored handler. There are actually some differences in here. These values are not the same. The quartiles fields are different between Python and Rust. In my research, I learned that there are some differences in statistical libraries and how they compute quartiles of large data series. What do we do? I’m not an engineer anymore, but let me think back on my time as a staff engineer, and give you a great answer to that question. It depends. That’s right. You actually have to use your brain. You have to think about the needs of your system. You have to figure out exactly what you need to do. There’s a number of strategies that we can take to fix this problem depending on needs.
Strategies
What can we do? There’s two broad things we can do. We can maintain the existing behavior exactly, or we can figure out if there’s a way we can deal with it. Maybe this change is acceptable for your system, for some reason. I don’t know why it might be, but maybe it is. Maybe you can update your client so that they can deal with this change. Maybe it is possible for you to deal with it. If you want to maintain behavior, you need your code to return exactly the same stuff. What can we do? There’s a couple strategies we can explore. We could try using a different library. Maybe there’s something other than statrs that has the same return values for quartiles as the Python code. What if that’s not an option? Maybe we could reimplement Python statistics library in Rust. Maybe it’s not quite as fast as statrs, but even just rewriting the exact same code can often be much faster, because Python is going to have a lot more copying, a lot more GC overhead than something like Rust will. There’s another option too. Because we’re taking an incremental approach here, we could actually leave the quartile calculation within Python completely. We don’t need to do everything in Rust. It’s just something that we can do. Based on what your needs are, based on your specific situation, you need to explore one of these options. I left this error in here on purpose, so that we could discuss this. It’s very important.
Testing
Now that we have our functionality written, let’s talk about how we could test it. I know everybody loves writing tests. Everybody loves having super long test suites, but tests are super important, especially when we’re going between multiple languages. We’re going to write some subunit tests in our Rust code so that we can do automatic validation. We’re going to create a new module at the bottom of our Rust code called tests. The name of the module isn’t strictly important, it’s just convention. We’re going to add an attribute macro on top of the module. This is going to do conditional compilation for us. The test code is not going to be included in any production builds. It’s only going to be compiled when we write test code. We are going to import the compute_stats function from the root of the crate into our tests module. We’re going to write a new function called test_9_numbers. Adding the test attribute macro on top of our function is going to give us the ability to have our function picked up by Rust’s automated test harness, and it will run the function and give us an alert if the function panics, which is going to happen if any of our assertions fail.
Let’s pop in some known numbers. Let’s calculate the statistics for that set of known numbers. We’re going to add in some assertions. These are easy to calculate because it’s only 9 numbers. We can run our tests by using cargo test. This is going to compile our code for us. It’s going to tell us that we had one test function and it ran successfully. One unit test is obviously not enough to deal with a whole big refactor like this. We’re going between multiple languages and we really need to be careful with our testing. You should be leveraging existing tests. You should be leveraging the tests that already exist in Python. You should be updating those so that they’re capable of testing, not just the Python code, but the Rust code as well. Because compute_stats is just a normal Python function, you can call it from either place. You can rely on dependency injection as well, so that you can test more code paths with both the Python code and the Rust code. Something else you can do is actually do randomized testing between the old code and the new code. Generate a random input, feed it into the old code, see what it gets you back. then feed it into the new code and compare those two results. They should match up.
Performance
Let’s also talk about performance. We did this whole thing with the goal of making our code faster? Did we do it? Let’s see. We can use Python’s timeit module to do some microbenchmarking. For the purposes of this microbenchmark, stats_py is a function that has the original code of our Python HTTP handler in it. We’re going to feed in those 9 numbers, and we’re going to run this 10,000 times. We’re going to do a very similar thing with our Rust code. We’re going to take those 9 numbers, and we are going to compute those stats 10,000 times. Let’s see what we got. What happened. We can see that the Rust code ran a little more than 100 times faster than the Python code. This seems really promising. This is very cool. This is a benchmark that’s running through Python, so we’re not just getting the faster code because it’s all in Rust, but there is a certain amount of overhead that comes from it being in Python too. This is somewhat fair. There’s a little bit of trickery going on in here, because we ran the test 10,000 times. This is actually the total time. Once we add in the average time, it starts to get a little bit less impressive. Because you divide those numbers by 10,000, and you realize, the Python code was pretty quick on its own already. This is not an extremely slow problem. I was feeling a little bit uncreative when I came up with this problem. If you started with something that was taking 500 milliseconds, a second, 5 seconds in Python code you might expect to see significantly more impressive results from refactoring to Rust. We did make the thing 100 times faster. That did actually happen. However, it was already operating at a relatively quick speed in Python, to begin with.
We also have to consider macrobenchmarking. It’s really tempting to just want to do a microbenchmark, like just put the teeniest bit of code on the bench and test that. That’s going to give you the best-looking results, at least. If we do a macrobenchmark that compares the HTTP performance of the old code and the new code, we can see that it’s about a 15% performance difference between Python and Rust. That’s because a lot of the time that is spent in our HTTP endpoint is going into the Flask library itself, and its HTTP handling, and the JSON serializing, deserializing. Once again, we should be picking something that is pretty CPU bound, where we’re spending a lot of our time in one place, and we should be pulling that into Rust. Microbenchmarks and macrobenchmarks are both super important. They both have their uses. It is important to do macrobenchmarks, and to make sure you know how your system is actually performing under load.
Summary
We looked at FFI refactoring and what it is. We looked at how to think about the feasibility of an FFI refactoring project. We learned how we can use the pyo3 library to do an FFI refactoring project. We learned a little bit about how we can test systems using Rust’s testing framework. We looked at how we could do some benchmarking strategies for an FFI refactoring project.
See more presentations with transcripts
AWS and NVIDIA to Collaborate on Next-Gen EC2 P5 Instances for Accelerating Generative AI
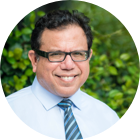
MMS • Daniel Dominguez
Article originally posted on InfoQ. Visit InfoQ
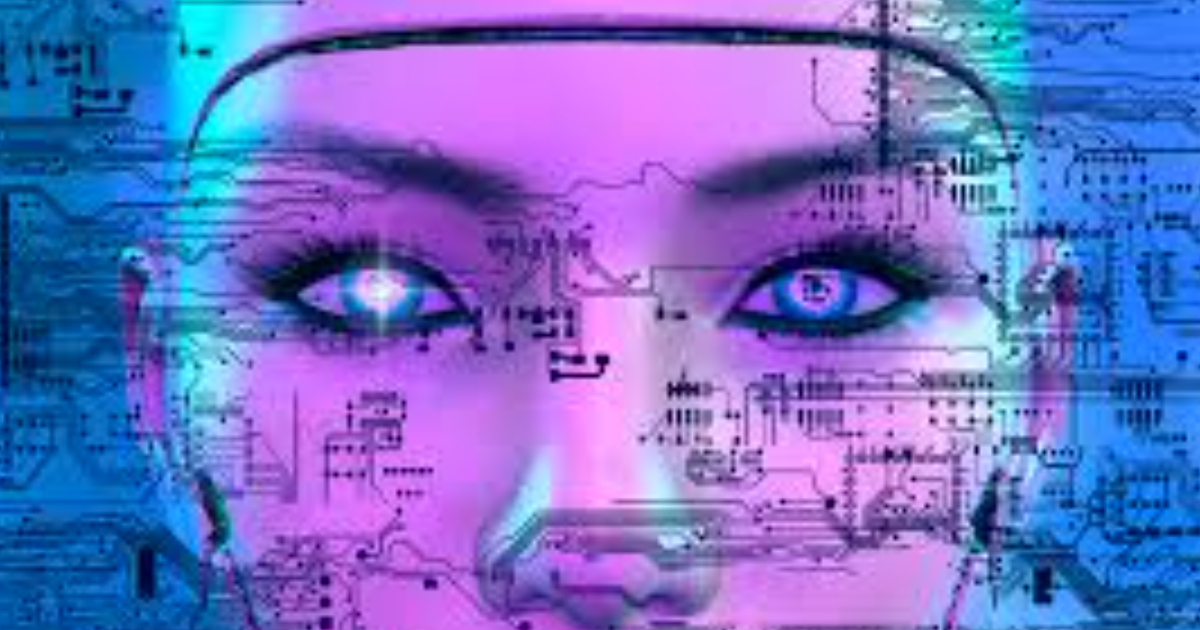
AWS and NVIDIA announced the development of a highly scalable, on-demand AI infrastructure that is specifically designed for training large language models and creating advanced generative AI applications. The collaboration aims to create the most optimized and efficient system of its kind, capable of meeting the demands of increasingly complex AI tasks.
The collaboration will make use of the most recent Amazon Elastic Compute Cloud (Amazon EC2) P5 instances powered by NVIDIA H100 Tensor Core GPUs as well as AWS’s cutting-edge networking and scalability, which will provide up to 20 exaFLOPS of compute performance for creating and training the largest deep learning models.
Accelerated computing and AI have arrived, and just in time. Accelerated computing provides step-function speed-ups while driving down cost and power as enterprises strive to do more with less. Generative AI has awakened companies to reimagine their products and business models and to be the disruptor and not the disrupted, said Jensen Huang, founder and CEO of NVIDIA.
Customers can scale up to 20,000 H100 GPUs in EC2 UltraClusters for on-demand access to supercomputer-class performance for AI thanks to P5 instances, which will be the first GPU-based instance to benefit from AWS’s second-generation Elastic Fabric Adapter (EFA) networking, which offers 3,200 Gbps of low-latency, high bandwidth networking throughput.
AWS and NVIDIA have collaborated for over a decade to create AI and HPC infrastructure, resulting in the development of P2, P3, P3dn, and P4d(e) instances. The latest P5 instances are the fifth generation of NVIDIA GPU-powered AWS offerings and are optimized for training complex LLMs and computer vision models for demanding generative AI applications such as speech recognition, code generation, and video/image generation.
Amazon EC2 P5 instances are deployed in powerful hyperscale clusters called EC2 UltraClusters, which consist of top-performing compute, networking, and storage resources. These clusters are among the most powerful supercomputers globally and enable customers to execute complex multi-node machine learning training and distributed HPC workloads. With petabit-scale non-blocking networking powered by AWS EFA, customers can run high-level inter-node communication applications at scale on AWS. EFA’s custom OS and integration with NVIDIA GPUDirect RDMA boost the performance of inter-instance communications, reducing latency and increasing bandwidth utilization. This is essential for scaling deep learning model training across hundreds of P5 nodes.
In addition, both companies have begun collaborating on future server designs to increase the scaling efficiency with subsequent-generation system designs, cooling technologies, and network scalability.
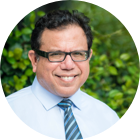
MMS • Almir Vuk
Article originally posted on InfoQ. Visit InfoQ
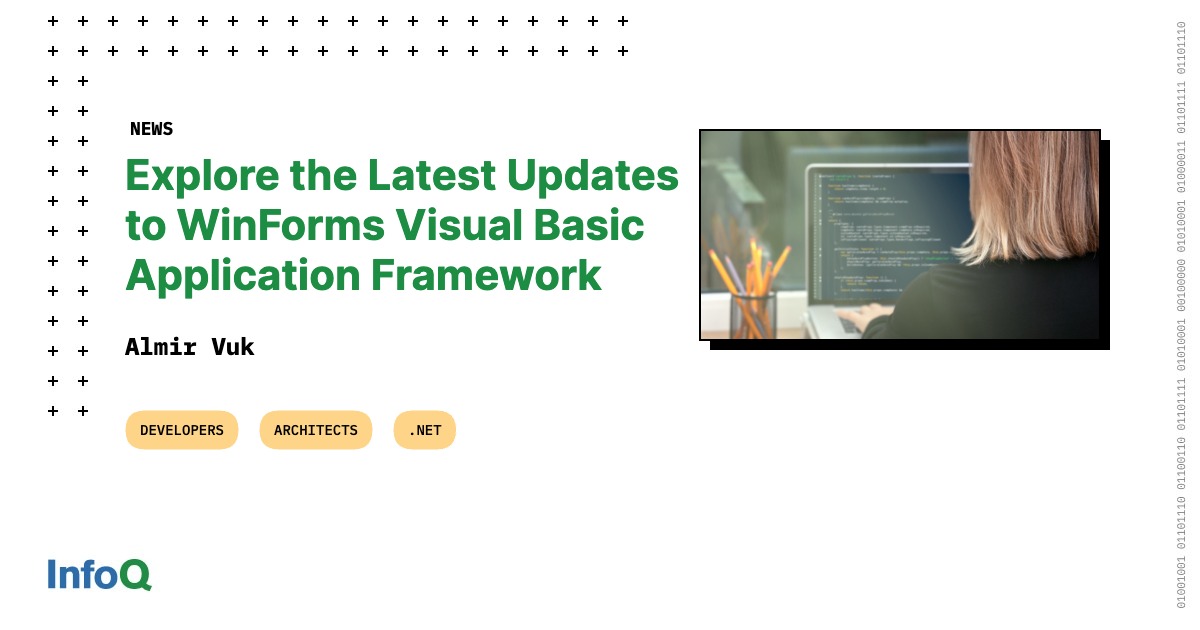
Recent updates to the WinForms Visual Basic Application Framework are covered in-depth in a blog post published by Microsoft. The original blog post explains the advantages users can expect to experience by updating their applications to the most recent .NET versions and provides comprehensive information on the new features and improvements.
One of those is the ability to convert older .NET Framework-based Visual Basic Apps to .NET 6, 7, or 8+ is one of the update’s key benefits. This enables developers to benefit from the vast array of new features and performance upgrades that come with the updated runtime.
In addition to the migration benefits, the new Windows Forms Out-of-Process Designer for .NET has undergone changes and improvements. The designer now features better support for Object Data Sources and offers a more streamlined user experience.
Upgrading to newer frameworks creates opportunities to support modern technologies that were not previously compatible with the .NET Framework. One such example is Entity Framework Core, a modern data access framework that enables .NET developers to handle database backends using .NET objects. Despite not being natively supported by Microsoft for Visual Basic, EFCore is constructed to facilitate its expansion by the community, which can provide code generation support for additional languages such as Visual Basic.
With the introduction of .NET 5, several enhancements were made to WinForms, including the addition of the TaskDialog control, improvements to the ListView control, and enhancements to the FileDialog class. In addition, there was a significant improvement in performance and memory usage for GDI+. Furthermore, in .NET Core 3.1, the default font for WinForms Forms and UserControls was changed to Segoe UI, 9pt to give the traditional WinForms UI a more contemporary appearance.
Building on these improvements, .NET 6, allows users to set any desired font as the default font for the entire WinForms Application. This is achieved in the Visual Basic Application Framework through an additional Application Event called “ApplyApplicationDefaults”, which was introduced during the .NET 6 timeframe. This event enables developers to set values for HighDpiMode, the application’s default font, and the minimum splash dialog display time.
The latest stable version of .NET 7, and the introduction of Command Binding in WinForms, .NET 7 makes it simpler to implement a UI Controller architecture based on the MVVM pattern for WinForms Apps and include Unit Tests in WinForms Apps. Additionally, WinForms received improvements such as rendering in HighDPI per Monitor V2 scenarios and have repeatedly enhanced accessibility support from .NET 5 to .NET 7 through Microsoft UI Automation pattern to make it work better with accessibility tools like Narrator, Jaws, and NVDA.
Regarding the Visual Basic Application Framework Experience, Microsoft has introduced changes for a new project properties UI in Visual Studio, which is now in line with the project properties experience for other .NET project types. The updated UI offers theme and search features, with a focus on improving productivity and providing a modern look and feel. Also, the original blog post authors are suggesting that users who are new to the updated project properties experience are encouraged to read the introductory blog for more information.
Lastly, developers interested in learning more about the updates to WinForms Visual Basic Application Framework can visit Microsoft’s official developer blog for more information and very detailed documentation about updates, changes, and new features around the WinForms Visual Basic Application Framework.
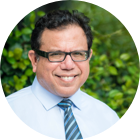
MMS • RSS
Posted on nosqlgooglealerts. Visit nosqlgooglealerts
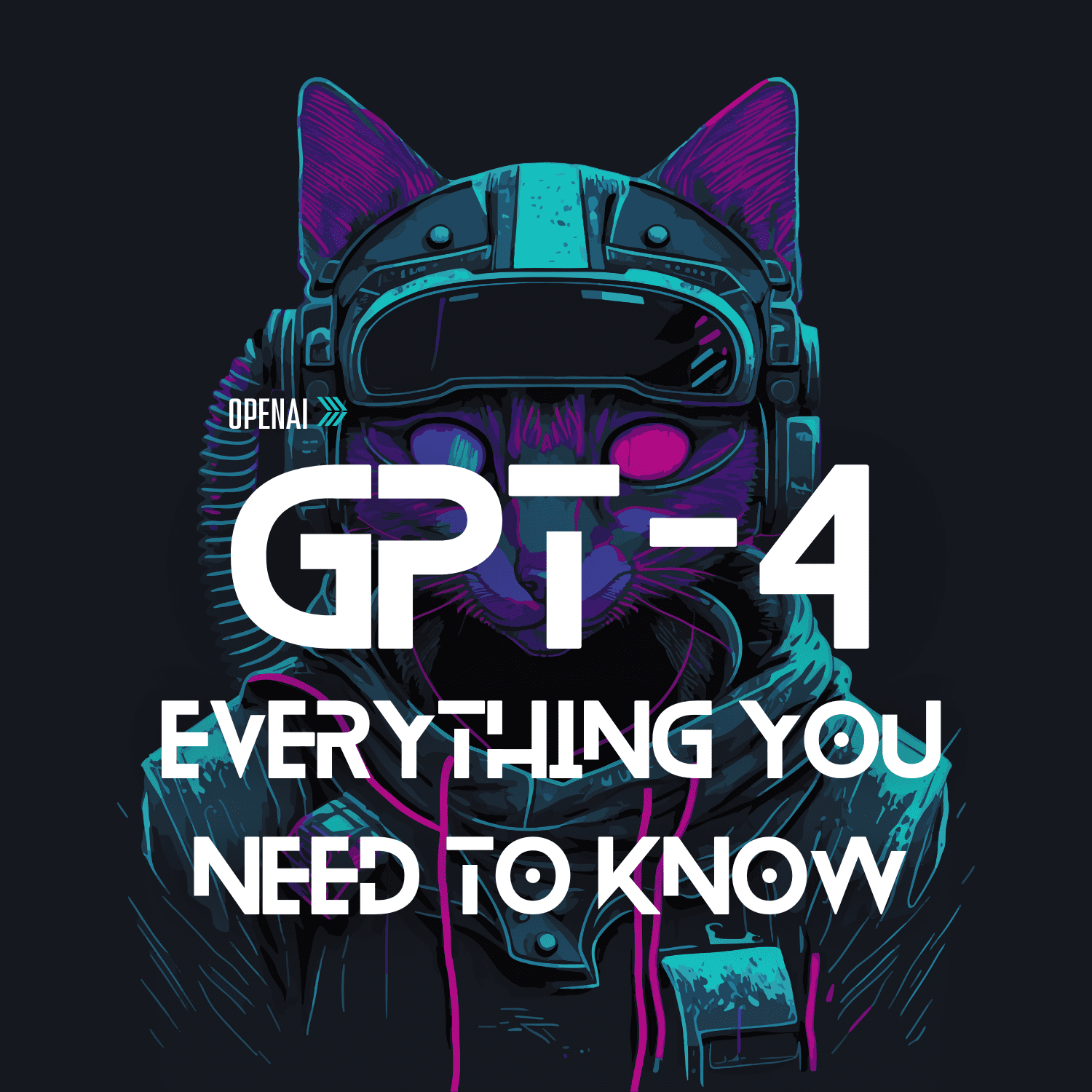
GPT-4: Everything You Need To Know • OpenChatKit: Open-Source ChatGPT Alternative • Introduction to __getitem__: A Magic Method in Python • NoSQL Databases and Their Use Cases • 7 Must-Know Python Tips for Coding Interviews
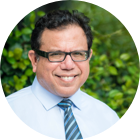
MMS • RSS
Posted on mongodb google news. Visit mongodb google news

MongoDB Inc. (NASDAQ: MDB)’s stock price has increased by 5.23 compared to its previous closing price of 211.13. However, the company has experienced a 0.42% gain in its stock price over the last five trading sessions. Barron’s reported on 09/01/22 that MongoDB Stock Falls Sharply as Fiscal-Year Forecast Disappoints
Is It Worth Investing in MongoDB Inc. (NASDAQ: MDB) Right Now?
while the 36-month beta value is 1.06.Analysts have differing opinions on the stock, with 17 analysts rating it as a “buy,” 4 as “overweight,” 6 as “hold,” and 0 as “sell.”
The average price point forecasted by analysts for MongoDB Inc. (MDB) is $244.22, which is $26.74 above the current market price. The public float for MDB is 66.20M, and currently, short sellers hold a 5.40% ratio of that floaft. The average trading volume of MDB on March 23, 2023 was 1.72M shares.
MDB’s Market Performance
MDB’s stock has seen a 0.42% increase for the week, with a 4.02% rise in the past month and a 11.01% gain in the past quarter. The volatility ratio for the week is 4.53%, and the volatility levels for the past 30 days are at 5.21% for MongoDB Inc. The simple moving average for the last 20 days is 4.64% for MDB stock, with a simple moving average of -3.95% for the last 200 days.
Analysts’ Opinion of MDB
Many brokerage firms have already submitted their reports for MDB stocks, with Bernstein repeating the rating for MDB by listing it as a “Outperform.” The predicted price for MDB in the upcoming period, according to Bernstein is $282 based on the research report published on February 17th of the current year 2023.
Guggenheim, on the other hand, stated in their research note that they expect to see MDB reach a price target of $205. The rating they have provided for MDB stocks is “Neutral” according to the report published on January 27th, 2023.
Wedbush gave a rating of “Outperform” to MDB, setting the target price at $240 in the report published on December 15th of the previous year.
MDB Trading at 4.97% from the 50-Day Moving Average
After a stumble in the market that brought MDB to its low price for the period of the last 52 weeks, the company was unable to rebound, for now settling with -52.93% of loss for the given period.
Volatility was left at 5.21%, however, over the last 30 days, the volatility rate increased by 4.53%, as shares surge +4.76% for the moving average over the last 20 days. Over the last 50 days, in opposition, the stock is trading +22.81% upper at present.
During the last 5 trading sessions, MDB rose by +1.07%, which changed the moving average for the period of 200-days by -18.56% in comparison to the 20-day moving average, which settled at $212.82. In addition, MongoDB Inc. saw 12.87% in overturn over a single year, with a tendency to cut further gains.
Insider Trading
Reports are indicating that there were more than several insider trading activities at MDB starting from Bull Thomas, who sale 5,000 shares at the price of $202.93 back on Mar 15. After this action, Bull Thomas now owns 16,203 shares of MongoDB Inc., valued at $1,014,650 using the latest closing price.
Ittycheria Dev, the President & CEO of MongoDB Inc., sale 40,000 shares at $207.86 during a trade that took place back on Mar 01, which means that Ittycheria Dev is holding 190,264 shares at $8,314,576 based on the most recent closing price.
Stock Fundamentals for MDB
Equity return is now at value -53.80, with -14.80 for asset returns.
Conclusion
In a nutshell, MongoDB Inc. (MDB) has experienced a better performance in recent times. The stock has received mixed “buy” and “hold” ratings from analysts. It’s important to note that the stock is currently trading at a significant distance from its 50-day moving average and its 52-week high.
Article originally posted on mongodb google news. Visit mongodb google news
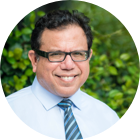
MMS • RSS
Posted on nosqlgooglealerts. Visit nosqlgooglealerts
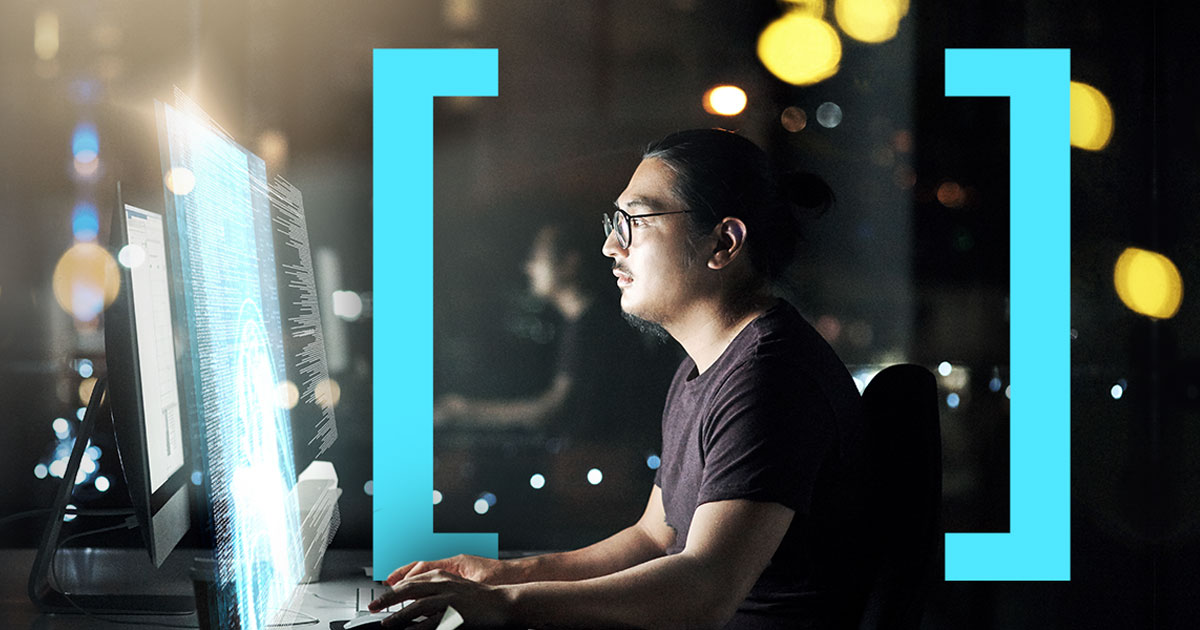
@media screen and (min-width: 1201px) {
.ccfsg641fe95f9cd3a {
display: block;
}
}
@media screen and (min-width: 993px) and (max-width: 1200px) {
.ccfsg641fe95f9cd3a {
display: block;
}
}
@media screen and (min-width: 769px) and (max-width: 992px) {
.ccfsg641fe95f9cd3a {
display: block;
}
}
@media screen and (min-width: 768px) and (max-width: 768px) {
.ccfsg641fe95f9cd3a {
display: block;
}
}
@media screen and (max-width: 767px) {
.ccfsg641fe95f9cd3a {
display: block;
}
}
TodayMarch 23, 2023
Microsoft has set itself the task of Azure Database for PostgreSQL the best destination for migrating or modernizing your open source enterprise workloads. For this reason, new features are now available to you that can be of great importance for the development of mission-critical applications.
The new features will help better protect your data, improve credential management, and gain better control over your databases.
Azure Active Directory
You can now improve database security by delegating the management and authentication of your database credentials to a central identity provider – Azure Active Directory in Azure Database for PostgreSQL – Flexible Server is now generally available for this purpose.
Customer Managed Keys
With the availability of Customer Managed Keys, you gain an additional layer of control and can encrypt your data to meet specific security and compliance requirements, for example.
Improvements in observability
New powerful tools such as improved metrics, PgBouncer monitoring, Azure Monitor Workbooks or Performance Insights ensure better monitoring of your database and optimization of app query performance.
@media screen and (min-width: 1201px) {
.xrjdh641fe95f9cd95 {
display: block;
}
}
@media screen and (min-width: 993px) and (max-width: 1200px) {
.xrjdh641fe95f9cd95 {
display: block;
}
}
@media screen and (min-width: 769px) and (max-width: 992px) {
.xrjdh641fe95f9cd95 {
display: block;
}
}
@media screen and (min-width: 768px) and (max-width: 768px) {
.xrjdh641fe95f9cd95 {
display: block;
}
}
@media screen and (max-width: 767px) {
.xrjdh641fe95f9cd95 {
display: block;
}
}
Related
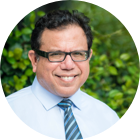
MMS • RSS
Posted on nosqlgooglealerts. Visit nosqlgooglealerts
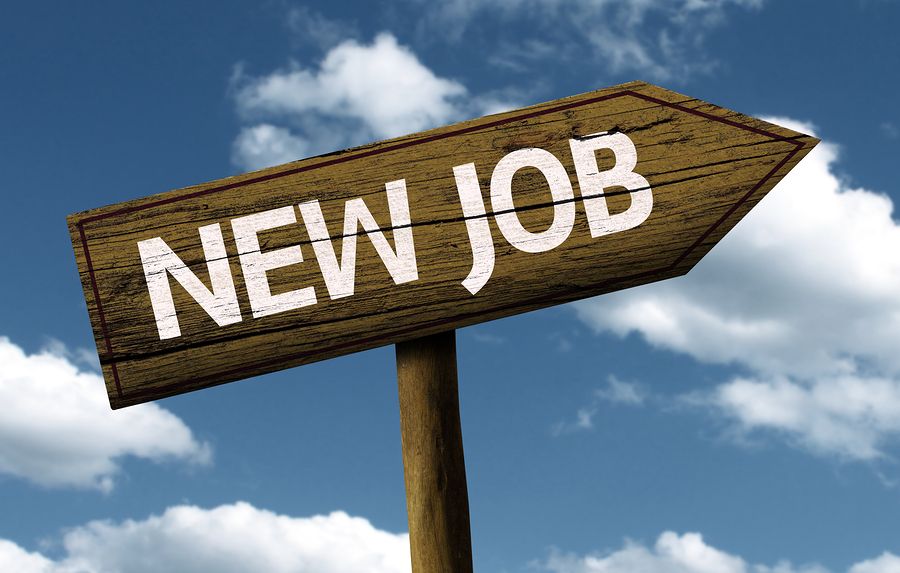
Tek Experts provides the services of a uniquely passionate and expert workforce that takes intense pride in helping companies manage their business operations. We care about the work we do, the companies we partner with and the customers they serve.
By delivering unrivaled levels of business and IT support, we make sure nothing gets in the way of our clients leaving their mark on the world. Our experience and expertise enable companies to focus on their core objectives, expand their service offering and exceed their customer expectations.Responsibilities
Develop modules for applications within a micro-service-based framework using JavaScript, React.js, Node.js, and other similar languages.
Employ Agile software methodology to liaise with customers and the development team and define and acquire requirements.
Develop front-end and back-end services for web-based applications.
Integrate UI and API microservices with NoSQL and SQL databases including MongoDB and PostgreSQL.
Actively troubleshoot and resolve performance issues as reported by customers and Support teams.
Offer nuanced insight in support of performance test strategy across multiple products
Design, create, and maintain load and performance test scripts using NeoLoad and other performance test tools.
Qualifications
Develop high-quality software design and architecture
Identify, prioritize and execute tasks in the software development life cycle
Develop tools and applications by producing clean, efficient code
Experience in software development, scripting, Agile and Scrum methodology
C-Descendant Languages: C-Sharp, Java Development Stack, Python, Flutter, Development Stack
Automate tasks through appropriate tools and scripting
Review and debug code
Perform validation and verification testing
Collaborate with internal teams and vendors to fix and improve products
Document development phases and monitor systems
Ensure software is up-to-date with the latest technologies
Develop flowcharts, layouts and documentation to identify requirements and solutions
Write well-designed, testable code
Preferred skills
Proven experience as a Full stack Software Engineer (5 years minimum)
Extensive experience in software development, scripting and Agile methodology
Ability to work independently
Experience using DevOps tools such as Azure DevOps and Automated Testing Frameworks
Knowledge of selected programming languages (e.g., Asp.net Core, C#).
In-depth knowledge of relational databases (e.g. MySQL, PostgreSQL, MySQL) and NoSQL databases (e.g. Cosmos DB, MongoDB)
Familiarity with various operating systems (Windows and Unix)
Analytical mind with problem-solving aptitude
Ability to work independently
Excellent organizational and leadership skills
BSc/BA in Computer Science or a related degree