Month: April 2023
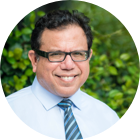
MMS • Almir Vuk
Article originally posted on InfoQ. Visit InfoQ
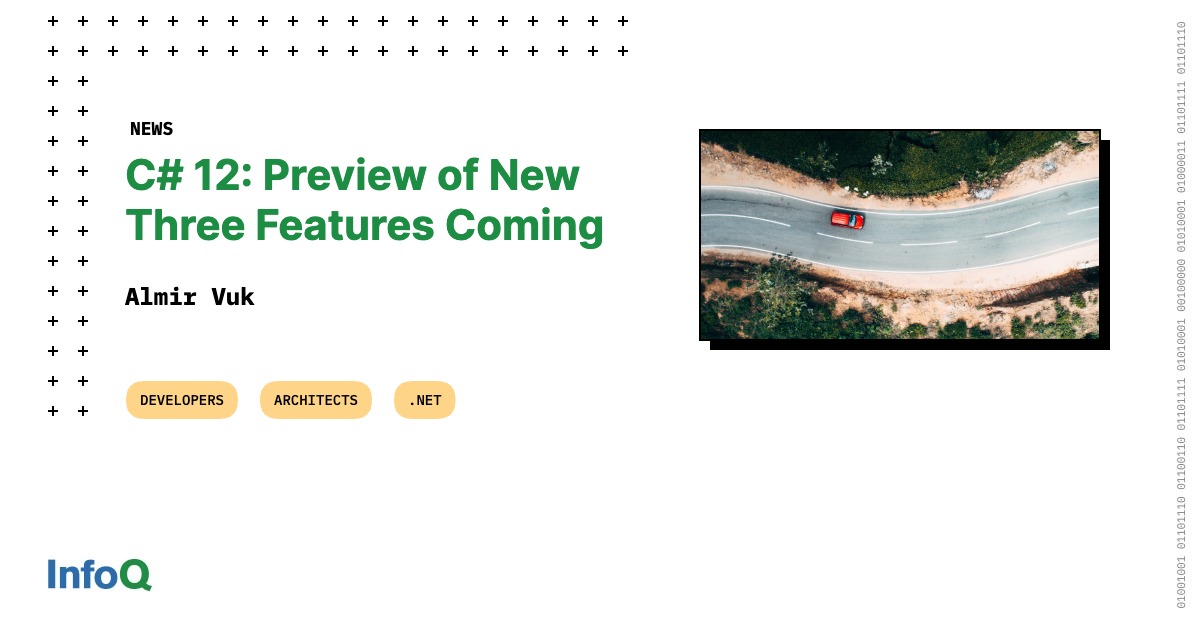
Microsoft has published a detailed release post that announces three new features that will be part of the upcoming release of C# 12. While still in the preview version, the C# 12 version introduces the features like primary constructors (for non-record classes and structs), using aliases for any type, and default values for lambda expression parameters.
The first highlight is the ability to use primary constructors which allows adding parameters to the class declaration and use them in the class body. This feature was previously only available for record type in C# 9 as part of the positional syntax for records, but it will now be extended to all classes and structs. With primary constructors, developers now can use the parameters to initialize properties or to include them in the code of methods and property accessors.
This feature is designed to simplify the process of creating and initializing objects in C#, allowing for more concise and readable code. The following code example shows how a primary constructor can be used in C# 12:
public class Student(int id, string name, IEnumerable grades)
{
public Student(int id, string name) : this(id, name, Enumerable.Empty()) { }
public int Id => id;
public string Name { get; set; } = name.Trim();
public decimal GPA => grades.Any() ? grades.Average() : 4.0m;
}
(Code sample provided by Microsoft devblogs.microsoft.com)
In addition to primary constructors, the default values for lambda expression parameters are another preview version feature. With this feature, developers can now specify and define default values for lambda expression parameters using the same syntax as for other default parameters. The default value will be emitted in metadata and is available via reflection as the DefaultValue of the ParameterInfo of the lambda’s Method property.
Before this preview release, if a developer wanted to provide default values for lambda expression parameters, they had to use local functions or a method called DefaultParameterValue from a specific namespace called System.Runtime.InteropServices.
Kathleen Dollard, Principal Program Manager, .NET, the author of the original blog post states the following:
These approaches still work but are harder to read and are inconsistent with default values on methods. With the new default values on lambdas you’ll have a consistent look for default parameter values on methods, constructors and lambda expressions.
Moving on to, the third highlight of C# 12 preview is a feature that enables the way to provide an alias to any type. Starting from this version, developers will be able to use using
directives to abstract actual types and provide friendly names for “confusing or long generic names”. By having these aliases, developers can improve the readability of their code and make it easier to understand.
This feature allows developers to give alias names to almost any type, including nullable value types and tuples, like in the following code sample:
using Measurement = (string Units, int Distance);
using PathOfPoints = int[];
using DatabaseInt = int?;
public void F(Measurement x){ ... }
(Code sample provided by Microsoft devblogs.microsoft.com)
In addition to this, a user named Muhammad Miftah wrote an interesting comment regarding the usage of aliases. On April 11, 2023, the user wrote the following:
using type aliases seem to only work within the file, unless you define it as a global using, but then the global scope is polluted.
I think you should introduce a namespace-wide equivalent, maybe introduce a type keyword for that? Also it would be extra cool to also have the option to reify the type alias into something that exists and is reflection-queryable at runtime. Also usable in generics and generic type constraints. TypeScript already has this!
Furthermore, the comments on the post indicate a significant level of interest in the C# 12 version and upcoming releases. Some commenters have requested further details and clarifications on the features, while others have provided suggestions for additional ones. However, there are also users who expressed their lack of interest and critics who express concern about the rapid development of this programming language.
Thus, it is recommended for readers to take a look in the comments section since it is very insightful and it brought a lot of discussion between the users and author, including a lot of code samples and information regarding the future evolution of this programming language.
Also, to test these out users need to download the latest Visual Studio 17.6 preview or the latest .NET 8 preview. Microsoft is calling the community members to provide their feedback about mentioned features: primary constructors, using aliases for any type, and default values for lambda expression parameters.
Lastly, interested users can track the implementation progress of C# 12 through the Roslyn Feature Status page and follow the design process of this programming language through the CSharpLang GitHub repository page.
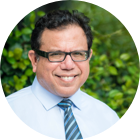
MMS • Peter Miscovich
Article originally posted on InfoQ. Visit InfoQ
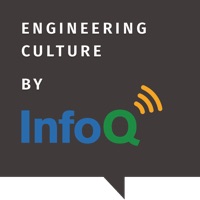
Subscribe on:
Transcript:
Hey folks, QCon New York is returning to Brooklyn this June 13 to 15. Learn from over 80 senior software leaders at early adopter companies as they share their firsthand experiences implementing emerging trends and best practices. From practical techniques to pitfalls to avoid, you’ll gain valuable insights to help you make better decisions and ensure you adopt the right organizational patterns and practices. Whether you’re a senior software developer, architect, or team lead, QCon New York features over 75 technical talks across 15 tracks covering ML ops, software architectures, resilient security, staff plus engineering, and more to help you level up on what’s next. Learn more at qconnewyork.com. We hope to see you there.
Good day, folks. This is Shane Hastie for the InfoQ Engineering Culture podcast. Today I’m sitting down with Peter Miscovich. Peter is one of the authors of a new book, The Workplace You Need Now: Shaping Spaces for the Future of Work. Peter, welcome. Thanks for taking the time to talk to us today.
Peter Miscovich: Shane, it’s a pleasure to be with you today.
Shane Hastie: My first question to my guests is almost always, who’s Peter?
Introductions [01:25]
Peter Miscovich: Well, thank you. I’ve been involved in hybrid workplace transformation for over 25 years. Began my early career in architecture and design, have an engineering background. I was former Andersen Consulting, Accenture partner and then PwC advisory partner, and then came to JLL 15 years ago to lead our global workplace transformation and strategy innovation practice. So I’ve helped to transform 1.5 billion square feet of corporate real estate. I’ve worked with almost every major financial institution and technology firm, and I’ll add life sciences to that mix and telecommunications and media. I’ve also served on a number of boards. I serve on the Accenture technology vision board under Accenture’s Chief Technology Officer Paul Daugherty and I serve on the series sustainability NGO board focused upon climate change risk and investments to mitigate climate change risk.
So over the past 25, 30 years, I’ve been focused upon workplace transformation at scale. I pioneered early programs in the 1990s with AT&T, IBM at the time, Accenture, Citigroup, PwC in really examining how technology can enable new levels of human performance and how the physical and digital workplace evolution can help enterprise performance and business performance. So I’m a bit of a hybrid myself, Shane, in having an engineering background, but yet focused on technology, focused on behavior, focused on human performance and then physical workplace design and the physical digital, if you will, blur that we’ve all been experiencing certainly in the post pandemic world.
The shift to hybrid and remote working has been going on for a long time [03:13]
Shane Hastie: Talking about that physical digital blur, is that has been so much of what has happened in the last few years where there was the radical shift for most technologists, not everyone, and certainly not every role in every company, but the vast majority of our audience will have experienced that radical shift from working predominantly or if not entirely in person to suddenly remote. And now we’re starting to see the hybrid workspace come back. What are the big challenges and the current state of the workplace when we look at that today?
Peter Miscovich: Yes, I’ll be glad to share maybe a bit of history, Shane, if that’s helpful,
Shane Hastie: Please do.
Peter Miscovich: Relative to the evolution. So if we go back to the 1990s and the early 2000s, the majority of the work, and this is from our work with Accenture and Gartner and Forrester and McKinsey and Brookings leading think tanks, but certainly from a historical perspective, work was office centric and location centric actually for the last 90 to 100 years. And it began shifting in that 1990s, 2000s timeframe as mobile technologies, the advent of wireless technologies. I had an early white paper Shane, where I predicted that wireless would come into the office environment, and I published that white paper in 1999 and four CIOs challenged me in stating that wireless will never come to the corporate workplace due to security issues and we’ll never see wifi or wireless in the workplace.
So even in those early days, 1990s, 2000s, there was a lot of resistance to the transformation that was already underway. In the 2010 to 2015 and as we approached 2020, we saw the advent of the cloud. I’ll use 2007 and 2010 as pivotal years, iPhone, iPad, mobile technologies, 4G now moving to 5G wireless. And so work was leaving the office 30 years ago when I began my journey in terms of hybrid and remote work. And I’ve been working in a hybrid or remote manner myself for over 25 years. What happened in March 2020, which is really fascinating at scale, is we had three billion people over a period of three to four weeks all go remote. And I think we had many clients, many of my clients for instance, that had frontline workers who never really went remote. It had to be on the front lines with our consumer products clients or other critical workforce cohorts.
The chasm in workplace design represents the societal shift that is happening as we move to more human centric working 05:53]
But then a majority of the office-based workers, knowledge workers in particular all went remote. And the chasm that that occurred from 2020 March to I’ll say even 2023 March, is that there’s a deepening divide between the historical office centric design that was based upon consistent work experiences. It enabled in place physical serendipitous collaboration, it required visibility based management. That 90 year ecosystem of office based work was disrupted at scale. And we’re moving now in 23 through 25 through 2030, certainly into a human centric model. And we’re leaving the location-based, office base work design model, but we have many leaders, many managers, many folks even in the tech sector that are still very much tied to that office centric work model. And so as the new model is emerging, providing flexible work experiences, intentional digital collaboration, empathy based management, human centricity, regenerative workplace, which we’ve written a lot about, it’s a huge shift.
It’s a societal shift. And many folks in leadership, many of the C-suite folks that I work with, senior leaders are very uncomfortable with the shift that’s occurring. And so there’s expectations today from management. I have multiple clients, I can’t name them, but you would know all of them. And the CEOs are mandating three days back, two days back, four days back in the office. And what will most likely evolve over time is that there’ll be hybrid centricity, human centricity as the go forward model and moving away from a place-based approach to work to probably a time-based and then I’ll add probably a virtual metaverse based hybrid approach to work.
And that transformation could take three years, could take seven years, could take 15 years. But clearly the transformation that started 30 years ago is now accelerating. The pandemic was an accelerant to the transformation of work. And those organizations that are embracing these human-centric hybrid and flexible work behaviors and management styles and cultural norms will be the big winners in the future in our view. But the tension and the conflicts and the continuous experimentation we see continuing for the foreseeable future, certainly over the next two to three, perhaps five, seven years.
Shane Hastie: What are some of the experiments that our listeners could consider trying?
Experiments and rapid learning to find what works best for your ecosystem [08:37]
Peter Miscovich: The experimental landscape is quite vast, and I will share several. So for example, I have an R&D technology group, even during the pandemic and post pandemic that’s been experimenting with a 8:00 AM to 12 noon, 1:00 PM three days a week, sometimes two days a week, hybrid patterning whereby they get together in their scrum teams or innovation teams or R&D teams for a period of time, usually in the mornings eight, 9:00 AM to 12, one, sometimes they’ll have lunch together and then they disperse in the afternoon to do their other work either at home or co-working sites or wherever. And that’s an example of looking at a time-based solution to hybrid versus a place-based solution. And they’ve been highly effective. It’s very intentional. They look forward to their time in the office together. And that time together is orchestrated in a very intentional and successful synergistic way.
And it’s built upon itself. It’s actually proven very successful for this firm, both in terms of their innovation cycles, their product development cycles and the like. I have another client organization that’s highly remote digital first. They have built a large retreat center. They have 80,000 employees, primarily tech and engineering, and they’re bringing those folks through that retreat center at a pretty strong episodic cadence. And groups of cohorts of R&D, engineering, software development will get together. There’s opportunities for brainstorming. You can go for a hike and have a meeting. It’s community building, but it’s not office space. It’s strictly innovation slash retreat, regenerative, resort if you will, almost environments. And so that’s another example of where innovation and new ways of working can be orchestrated outside of the traditional or typical corporate office environment.
Experimenting with the metaverse [10:43]
And then I’ll use a third experimentation which has been published from our work, for example, with Accenture, 120,000 folks. We’ve exercised for the last two years, metaverse training, metaverse socialization, lots of experiments in the virtual mixed reality world at Accenture and with Accenture clients that we’re partnering with in using the Metaverse, not as a sole means of hybrid workplace experimentation, but an opportunity for immersion in some cases for socialization, in some cases for onboarding, training and engaging employees perhaps in a new and unique way relative to collaborative, socialization, behavioral shifts, upskilling. And we do think again, by 2026, 27, we’ll start seeing more and more of the extended reality, mixed reality Metaverse, virtual reality environments. We’ll all be in the Metaverse probably one to two hours a day by 2027, and it may be bumpy and a ways until we get there relative to the technologies. But those are three examples of both time-based, if you will, hybrid experimentation as well as place-based and technology-based experimentation, pilot programs that are scaling well and showing good promise for future success.
Shane Hastie: So three distinctly different but very interesting experiments.
Becoming learning organisations [12:09]
Peter Miscovich: Yeah. And I think Shane, I’ve been in the trenches of hybrid work for many years and I’ve always been a big believer in experimentation. And what’s fascinating, it’s great to hear, for instance, we have Microsoft as a client. Satya Nadella, CEO of Microsoft, one of his pillars of Microsoft’s vision and mission is having this learning mindset and a mindset for experimentation. We were doing a lot of this hybrid piloting 15, 20 years ago. The technology hadn’t quite matured then. But what’s fascinating today, Shane, is that our insurance clients, our consumer products clients, our financial clients, our governmental clients, our educational clients, certainly our technology clients are all experimenting. And we’re hoping that the experimentation continues.
As the economic headwinds develop and uncertainty continues as we go into 2023, there are those who believe that we’ll retrench back to old ways of working, but our sense is that this level of experimentation will continue. The talent war, if you will, especially for tech talent and digital talent will continue to be a challenge for many organizations. And the only way to engage talent and to innovate and to innovate both business model product innovation is through experimentation. And as workplace and hybrid work, experimentation becomes part of the DNA of any leading organization, those that experiment more and embrace experimentation and that learning mindset per Satya Nadella will be the leaders and will take market share. They’ll be the success stories of 2026, 2027, 2030 and beyond.
Shane Hastie: Looking to those leaders, looking to that future, what are the challenges and the opportunities for organizations? So if they want to become one of these leaders, if they want to attract great talent, and if we’re talking to our listeners on this podcast who are typically the technical influencers, technical leaders, what can they do?
Leaders need to embrace the changes that are underway [14:17]
Well, the one thing that we share with our executive leadership teams consistently is that they need to embrace the present and embrace the changes that are underway. And that requires sometimes intentional forgetting and intentional letting go of historical work behaviors and work norms. And then I would add to really start listening to your people. And we will soon have potentially five if not six generations in the workplace. And Google just announced this morning a very interesting hybrid workplace pilot, and they’re piloting the program slightly differently for their cloud engineers and some of their other engineering groups. But what I found fascinating about this Google pilot, and I would recommend again, commend Google for their ability to really listen to their employees and understand what do their people want and to take an empathy based listening approach for every group, for every cohort in your organization.
Work-life balance doesn’t really exist today – the need is for effective work-life integration [15:22]
And the question and the challenge will be, and it is for many of our clients right now, technology clients and our clients in general, is that you may not be able to provide a human-centric individualistic program and cultural norm for everyone, but you’ve got to be able to meet people at least halfway and show with good intent, with good transparency, with mission-driven authenticity, that you’re really listening to your people. You want to give them the tools and capabilities and practices and policies that will make them successful and to meet their life-work balance or life-work integration, I should say. And for years, people have, many organizations, many HR, think tanks and the like have professed the need for work-life balance, which doesn’t really exist today, especially in our whole accelerated post pandemic world of ambiguity, complexity, disruption, acceleration. But the majority of employees today want life-work integration.
And the majority that I know want to work for organizations that will enable that in a way where they can have a healthy balance between their lifestyle, their work style, our research in the regenerative workplace to have the balance between mental wellbeing, social wellbeing, and physical wellbeing. And if you’re a leader out there of a large tech team, if you’re listening to those employees and to your people and you have empathy for them, and as individual managers, you show enough caring and enough ability to flex and meet them at least halfway, I think you’re going to have committed employees who are going to be exceptional performers. And I think that’s a major societal shift in organizational shift, Shane, that I think is a very positive outcome from the pandemic.
The challenge with it is that most leaders and many CEOs and executive leadership teams find that level of engagement either to be threatening or they’re not comfortable with that level of transparency, openness, and empathy. And so here in lies a challenge that we have from a management and behavioral and policy perspective that will probably continue over the next two to three years or longer. And it might require the next generation of managers who will have all of these great values and capabilities, who will probably be the next leaders to really actualize what we’re describing.
Shane Hastie: So that sounds to me like a generational shift. Are we teaching this next generation of leaders to be more humanistic or are they just following in the footsteps?
The generational shift in leadership attitudes [18:03]
Peter Miscovich: Well, it’s a great question. I have nine godchildren and they’re all pretty much Gen Z. They range in age from let’s say 15 years old to 32 years old. So maybe young Gen Y, mid-gen Y. I think they have a level of digital literacy and awareness of the world and awareness of the globalized interconnected world that I think surpasses any previous generation. So I think their mindsets and their values are already quite advanced and prepared for what is coming. I think where we need to do a better job, those of us that are more senior to Gen Y or Gen Z or Gen Alpha that will soon be following Gen Z is that we’ve got to mentor and enable and share with them some of the good humanistic lessons that we’ve learned. And this is where time spent in personalized intentional mentoring and engagement is really important.
And it’s in all the surveys, Gallup surveys, all the HR global workforce surveys show that Gen Z, they very much appreciate in-person engagement. It’s really interesting. There’s also a survey for Gen Alpha and Gen Z, which is fascinating, that they find that in-person engagement is so unique that it’s sort of like for those of us we were raised where going on a vacation was something unique and kind of experiential. They feel like they’re so connected and so accustomed to their digital literacy and their digital lifestyles that’s sort of in-person, human to human in real life, IRL engagement is considered sort of an interesting novelty to them and they really treasure it.
And so I think we have to think about how we make those in-person moments that matter meaningful, both from a learning, mentoring and perhaps even reverse mentoring perspective. And I think the challenge today at a generational level with social media, I have my issues about social media and its negative impact at a societal level and how we need to perhaps engage differently, especially with our younger generations as they manage through all the complexities and all of the mental and emotional and psychological challenges that social media presents to them as an entire generation. So I think there’s a lot of great generational exchange and partnership that will help that next generation. And we do need to make the time and the effort and that intentionality and again, strong empathy to understand what they’re experiencing and what they’re going through and how we can help them better navigate.
So I know that’s a rather lengthy response, but I think there’s a lot of complexity to the generational evolution that’s occurring. And if we have five or six generations in the workforce, virtual, digital, physical, digital, whatever, combination thereof, it’s going to require a lot more good communication, authenticity, transparency, empathy to make that multi-generational workforce high performing. So I think the effort definitely needs to be made. And I think there’s a bit of fatigue Shane, post pandemic for all of us. And I think the ability to refresh and regenerate right now is really important as we move into 23 and 2024 and beyond, as we still need to heal our psyches from the post-traumatic stress of the pandemic at a societal level. And I don’t know if everyone agrees with that, but I think there’s a lot of post pandemic, post-traumatic stress from the pandemic that we’ve never really recognized at a societal level that we do need to recognize and heal from.
Shane Hastie: How do we start? How do we start the healing? How do we start the changes towards the human-centric workplace?
Start with quiet [22:03]
Peter Miscovich: Well, I always start with quiet. And I’ve known Cal Newport who many in your audience may know around deep work and deep thinking, and I’ve been a practitioner of meditation for 24 years, 25 years. But we have to start with quiet, and we have to start with the quietness in terms of listening to ourselves. And I think that healing and that regenerative process begins with the quietness of our own consciousness and tuning into that quietness and getting grounded and healed, if you will, in terms of our own sense of self and sense of wellbeing and sense of wellness. And then I think the next step in the process is the listening, the listening and deep listening and listening without judgment, listening unconditionally if you will, and listening that will hopefully lead to understanding. And then with the understanding, I think we can begin to co-create, co-partner at a generational level or cross-generational level, some of the potential solutioning that is required.
And what I just described, I think will heal political divides. I think it will heal racial divides. I think it will heal cultural divides. I mean, if we think of the war in Ukraine to the politics of the US, to the rise of dictatorships and authoritarian regimes, a lot of it’s around the fact of sort of a non-humanistic, non-listening, non-quiet, non-empathy based approach to life. And so I think the pandemic in a very, I mean, as horrific as the pandemic was, it was also a wake-up call to tune into our deeper selves, and to find that quiet within ourselves, and to listen to ourselves and to be guided to listen deeply to others, and hopefully then gain that empathy and understanding to co-create and partner together collectively at a societal level to the healing and I think the path forward that we’re all seeking as we move ahead.
Shane Hastie: Shifting context slightly to just talk about the book, there are three key themes in there, the personalized workplace, the responsible workplace, and the experiential workplace. What do you mean by personalized workplace?
The book: The workplace you need now [24:26]
Peter Miscovich: We wrote the book, our CEO and our head of research and myself in early 2021 in three months during the middle of the pandemic. And at the time we divided the book up into three sections. And as you name them, the personalized section, the responsible section, and then the experiential section. And the personalized section of the book is really about the individual and it relates to some of what I just shared relative to how to make the workplace and the hybrid work environment work for the individual at a personalized level. And so if we think about personalization today, whether it’s retail or vacationing or personalized learning, the ability to have a personalized work experience is one of the key tenants in the book. And it all centers around what we call this win-win win theme. The future of work needs to be a win for the individual in terms of personalized work.
It needs to be a win for society in terms of responsible and sustainable workplace and real estate practices. And then it needs to be a win for the organization in terms of experiential outcomes and experience. So the personalized section begins sort of that individualistic journey. How do we make the hybrid workplace inclusive of technology enablement work for the individual? And then how do we make the hybrid workplace ecosystem in the second section work for society in terms of responsible workplace practices, responsible technology, real estate practices? And then finally the third section is focused on the win for the organization in terms of delivering these experiential outcomes. And the experiential outcomes are enabled both by technology, by physical space, by services, by human interaction, all of the things that we experience every day. How do we make those meaningful moments that matter every day for our people?
So the book was a bit prescient, if that’s the right word. And we were not sure in January 2021, for instance, whether hybrid work would stick. I was a strong believer that it would stick, having practiced hybrid work for many years and seeing that over time as any exponential growth curve can relate to that slow growth, slow growth, slow growth, and then exponentially, it really begins to take off. And I don’t know if hybrid work follows an exponential growth pattern, but as we look at personalized work, responsible work, experiential work, all of that certainly seems to be moving forward in 2023 post the book being published in late 2021. And we believe that all of the trends we predicted will probably continue as we go to 2027 and beyond.
Shane Hastie: So what is a regenerative workplace?
What is a regenerative workplace? [27:23]
Peter Miscovich: I spoke at several conferences in the fall on this topic, and the challenge at a societal level right now at an organizational level is that anxiety, depression, loneliness, fear, insecurity, I mean adolescent suicides, all of those mental health crises are at an all time high. And as we look at how we orchestrate work and workforce policies and workplace transformation, the regenerative workplace begins to address in again three very specific areas, how do we enable and help with mental health and mental health interventions both in terms of work, work practice, management styles, engagement levels? Again, going back to empathy and listening. So the first element of the regenerative workplace is really the mental wellness and wellbeing element. And then the second element is the social wellbeing and socialization, having a sense of community, feeling that you’re part of not only an organization, but you’re part of your community, part of your network of community members, whether that’s a virtual community, could be an in-person community, but socialization and social wellbeing is the second pillar of the regenerative workplace.
And then the third pillar is physical wellbeing, and that involves everything from not being on Zoom or Microsoft Teams calls 15 hours a day. The ability to work in a manner where your physical health, both from things like ergonomics, from sleep patterns to eye strain, to the ability to exercise and engage and take care of your body as best we can in again, this very accelerated period of disruption and accelerated change. What’s fascinating is we have several clients now that have global wellness executives within their organizations, often reporting up to the CEO or chief human resource officers. And we’re finding at the enterprise level, companies are beginning to understand that if they take care of their workers and really provide this regenerative workplace approach, mental, social, physical, that the rewards from a commitment perspective, a performance perspective, a wellbeing perspective, a reduction in healthcare cost perspective are going to be considerable.
I’ve been in the trenches, Shane, on sustainability for 25 years and in the trenches of integrative wellness for 20 years, and some of these things just take a long time. I was hybrid working for 25 plus years. So we believe in the next 10 years, regenerative workplace practice will become the practice, but it’s going to take perhaps again, another generational shift to fully embrace it, to endorse it, and to practice it at scale. But the pandemic was a big wake-up call, especially as it relates to mental wellness and mental wellbeing. So we think it has a future and we strongly believe in it, and we’re helping advise our clients to embrace the regenerative workplace leading practices.
Shane Hastie: Peter, some really, really interesting and deep thoughts there. If people want to continue the conversation, where do they find you?
Peter Miscovich: Well, they’re welcome to reach out to me via LinkedIn or I’m glad to share as a follow-up from your podcast, my email address, it’s Peter, P-E-T-E-R dot Miscovich, M-I-S-C-O-V-I-C-H@jll.com. And I welcome technologists and the technology sector very openly. I think the human centricity of the technology sector is really key and critical to our future. Shane, I’ve been working with ChatGPT and had about 10 years of immersion, and I have a couple of articles focused on artificial intelligence and work automation, and I do think the human centricity and the humanistic values of the technologists who are listening to this today, they are critical to the future of all of us in terms of how we navigate and how we move forward with all of the incredible technological change that is just beginning. So I embrace your audience in a big way and would be glad to be a resource to you or to your audience in any way that I can be helpful.
Shane Hastie: Thank you so much.
Mentioned:
.
From this page you also have access to our recorded show notes. They all have clickable links that will take you directly to that part of the audio.
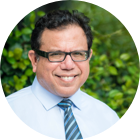
MMS • Steef-Jan Wiggers
Article originally posted on InfoQ. Visit InfoQ
Microsoft recently announced a new data mapper for Azure Logic Apps available as a Visual Studio Code extension. The mapper capability is currently in public preview.
Azure Logic Apps is a cloud platform where developers can create and run automated workflows with minimal code. It has two plans: Consumption and Standard. The Azure Logic App Consumption plan runs in the multi-tenant Azure Logic Apps. In addition, it supports mapping through an integration account, where a developer can upload schema and mappings to use within an action in a Logic App flow.
On the other hand, Standard supports local development on Windows, Linux, and Mac, providing a new layout engine that supports complex workflows and enables custom connector extensions. Standard doesn’t rely on the integration account, and schemas and maps are part of the flow. However, a graphical mapper was not available until just now.
The Data Mapper extension provides a graphical way to map data from a source schema to a target schema using direct mappings and functions, handling any translation between supported schema types in the backend.
Alex Zúñiga, a Program Manager of Azure Logic Apps at Microsoft, explains in a Tech Community blog post:
Data Mapper provides a modernized experience for XSLT authoring and transformation that includes drag and drop gestures, a prebuilt functions library, and manual testing. In addition, you can use the extension to create maps for XML to XML, JSON to JSON, XML to JSON, and JSON to XML transformations. Once created, these maps can be called from workflows in your logic app project in Visual Studio Code and deployed to Azure.
Kent Weare, a Principal Program Manager for Azure Logic Apps, tweeted in response to the availability of a data mapper in the consumption plan:
For now, we are prioritizing the General Availability (GA) of LA Standard for VS Code. Following that, Azure Portal (LA Standard). Too early to commit either way for consumption.
With the data mapper, developers building workflow have one tool for mapping and transformations. They can inspect the underlying code for a mapping, use pre-built functions like Conversion, Date and time, Logical comparison, Math and String, test the map with a sample message, and have an intuitive user experience with zoom options, mini-view, and map checker.
When asked by InfoQ about what is driving this investment from Microsoft, here is what Weare had to say:
Developer productivity and reducing friction when building integrated solutions are very important for us. In addition, we wanted to have a one-stop shop to solve data transformation needs without introducing additional or external tools. The Public Preview release of the new Data Mapper is the first step in this journey.
We are looking forward to more customer feedback to help us drive the next level of investments in this area.
Lastly. the documentation pages and walkthrough video show more details and guidance of the data mapper.
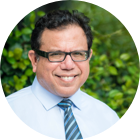
MMS • Matt Campbell
Article originally posted on InfoQ. Visit InfoQ
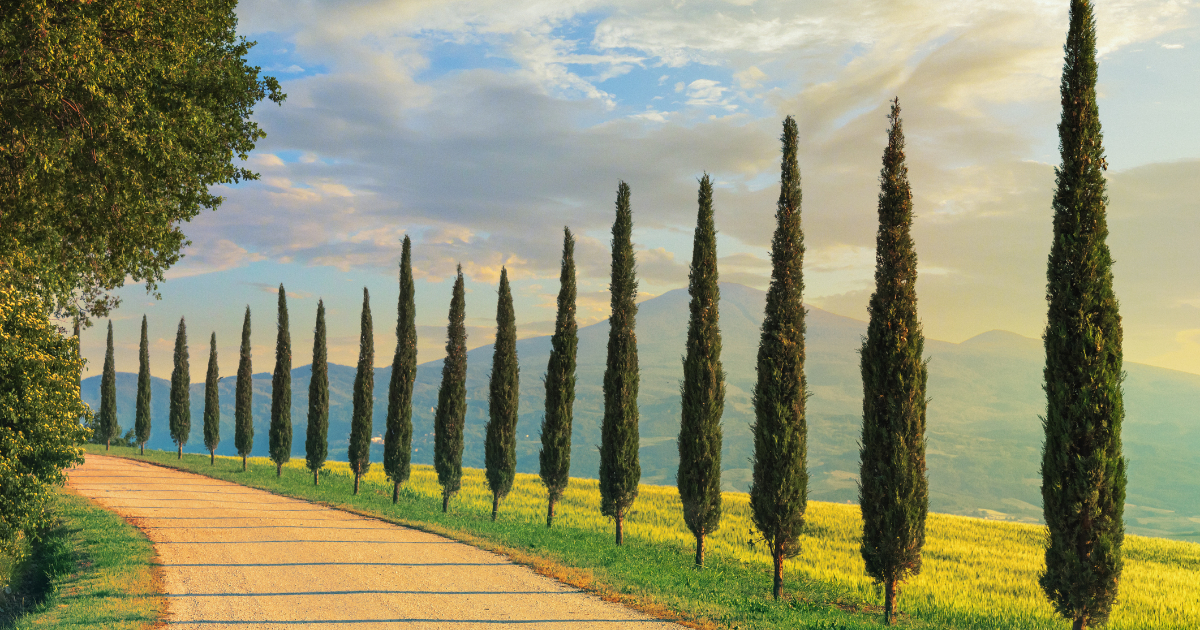
HashiCorp has released a number of new improvements to the CDK for Terraform (CDKTF). These improvements include enhanced type coercion, iterators support, and function support. Other improvements target the experience of working with CDKTF within Terraform Cloud or Terraform Enterprise. This includes improvements to plan and apply and the automated creation of Terraform workspaces.
The CDK allows for writing Terraform configurations in a number of programming languages including C#, Python, TypeScript, Go, and Java. It includes support for all existing Terraform providers and modules. The CDKTF application code synthesizes into JSON output that can be deployed with Terraform directly.
The 0.16 release improves type coercion by updating the convert
command to match the type of value being assigned to an attribute. The convert
command is used to convert pre-existing Terraform HCL code into a CDKTF-compatible language. The command now compares the type being generated from HCL and matches it to the provider schema. In the event of a type mismatch, the incoming value will be coerced into the correct type. This change prevents errors that could occur in previous releases as Terraform automatically converts primitive types to match the resource schema.
The release also improved the conversion of meta-arguments such as count
and for_each
. Now the count meta-attribute can be represented as an iterator via an escape hatch. In addition, convert is able to use iterators for for_each
, count
, and dynamic blocks without an escape hatch.
The following TypeScript example uses the new TerraformCount
function to create the specified number of instances as defined within servers.numberValue
:
const servers = new TerraformVariable(this, "servers", {
type: "number",
});
const count = TerraformCount.of(servers.numberValue);
new Instance(this, "server", {
count: count,
ami: "ami-a1b2c3d4",
instanceType: "t2.micro",
tags: {
Name: "Server ${" + count.index + "}",
},
});
HashiCorp recommends using iterators when referencing dynamic data that will not be known until after Terraform applies the configuration. For static data, they recommend using loops within the selected programming language. Escape hatches are needed when referencing a specific index within the list. This is because CDKTF implicitly converts lists to sets when iterating over them.
Conversion can also now convert Terraform functions to the appropriate CDKTF function. This improves the readability of code and permits autocomplete to function properly. With this change, the following HCL expression:
replace("hello-${22+22}", "44", "world")
Would be converted to:
cdktf.Fn.replace("hello-" + cdktf.Token.asString(cdktf.Op.add(22, 22)), "44", "world")
The 0.15 release made a number of improvements to how CDKTF interacts with both the Terraform Cloud and Terraform Enterprise environments. In previous releases, during stack configuration with CDKTF, the interaction with Terraform inside Terraform Cloud was hidden. It could only be viewed through a URL.
This release replaced the Terraform Cloud API-based implementation with one that makes calls directly to the Terraform CLI. This allows for the full plan to be displayed with details. Additionally, CDKTF now supports additional features such as cost estimations and Sentinel policies when run within Terraform Cloud.
The release also introduced automatic workspace creation when running plan and diff or apply and deploy. This improves the previous behavior where workspaces would have to be manually created through the Terraform Cloud or Enterprise UI.
More information about the contents of the release can be found in the blog post and upgrade guide. HashiCorp has a discussion forum for questions. The CDK for Terraform tutorials are recommended for users new to CDKTF.
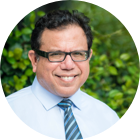
MMS • Renato Losio
Article originally posted on InfoQ. Visit InfoQ
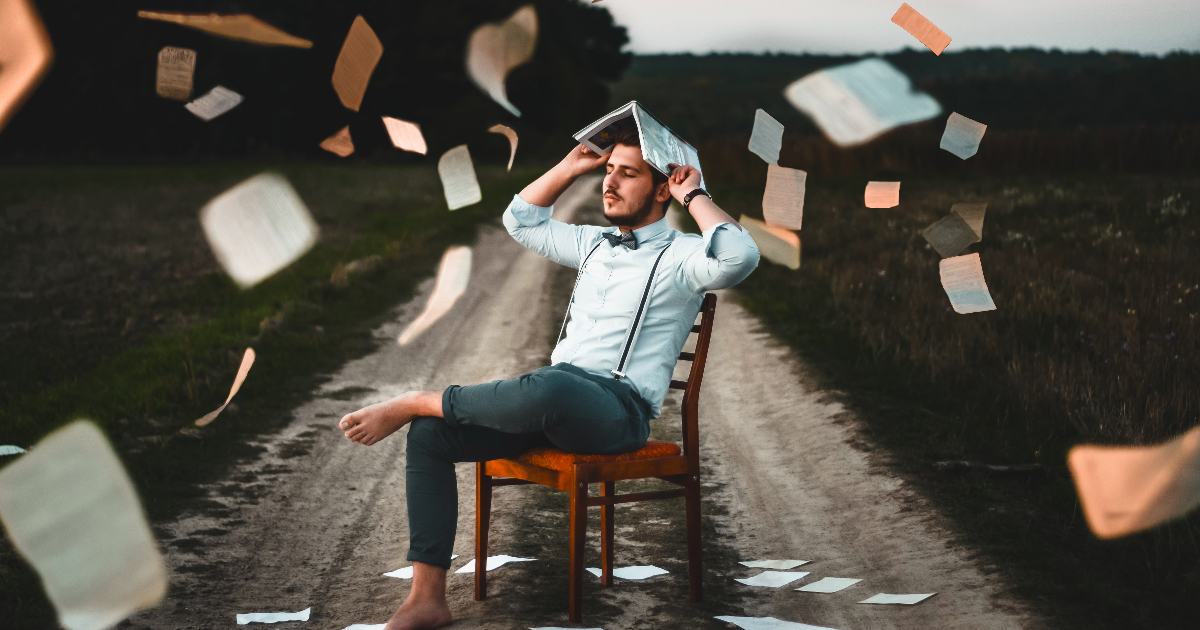
AWS recently released an update to the Well-Architected Framework. The new version strengthens prescriptive guidance with over 100 best practices updated across all six pillars of the framework.
According to the cloud provider, the enhanced prescriptive guidance offers new and updated best practices, implementation steps, and architectural patterns that help customers better identify and mitigate risks in cloud deployments.
The updates and improvements focus on providing better coverage across the available services, almost all for the ones released in the last couple of years. Services that were added or expanded in coverage include Elastic Disaster Recovery, Trusted Advisor, Resilience Hub, Config, Security Hub, GuardDuty, Organizations, Control Tower, Compute Optimizer, Budgets, CodeWhisperer, and CodeGuru. Announcing the updates to the framework, Haleh Najafzadeh, senior solutions architecture manager at AWS, writes:
From a total of 127 new/updated best practices, 78% include explicit implementation steps as part of making them more prescriptive. The remaining 22% have been updated by improving their existing implementation steps. These changes are in addition to the 51 improved best practices released in 2022 (18 in Q3 2022, and 33 in Q4 2022), resulting in more than 50% of the existing Framework best practices having been updated recently.
The Well-Architected Framework is a collection of best practices that allow customers to evaluate and improve the design, implementation, and operations of workloads on AWS. The framework is focused on six pillars: operational excellence, security, reliability, performance efficiency, cost optimization, and sustainability. As reported separately on InfoQ, AWS recently added as well a new container lens.
AWS is not the only cloud provider offering a set of guiding tenets for cloud architects: the Google Cloud Architecture Framework describes best practices and provides recommendations on Google Cloud, while Microsoft offers the Azure Well-Architected Framework (WAF), with the recently added sustainability guidance.
Jess Alvarez, AWS training architect at A Cloud Guru, writes about the benefits of the framework and highlights the main differences between the providers:
There are some key differences between AWS and Azure’s take on the Well-Architected Framework. You’ll notice a difference in pillars and a difference in depth. AWS includes best practices for each pillar both for your environment AND your organization (…) Azure has a hard focus on best practices for your environment and does NOT go into the business side of each pillar. Some businesses don’t need that level of support and may prefer a framework that cuts to the chase.
The new version of the AWS framework is available within the AWS Well-Architected whitepapers and in the AWS Well-Architected tool in a subset of AWS regions.
AI, ML & Data News Roundup: HuggingGPT, AWS Bedrock, Microsoft Visual ChatGPT, and StableLM
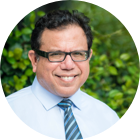
MMS • Daniel Dominguez
Article originally posted on InfoQ. Visit InfoQ
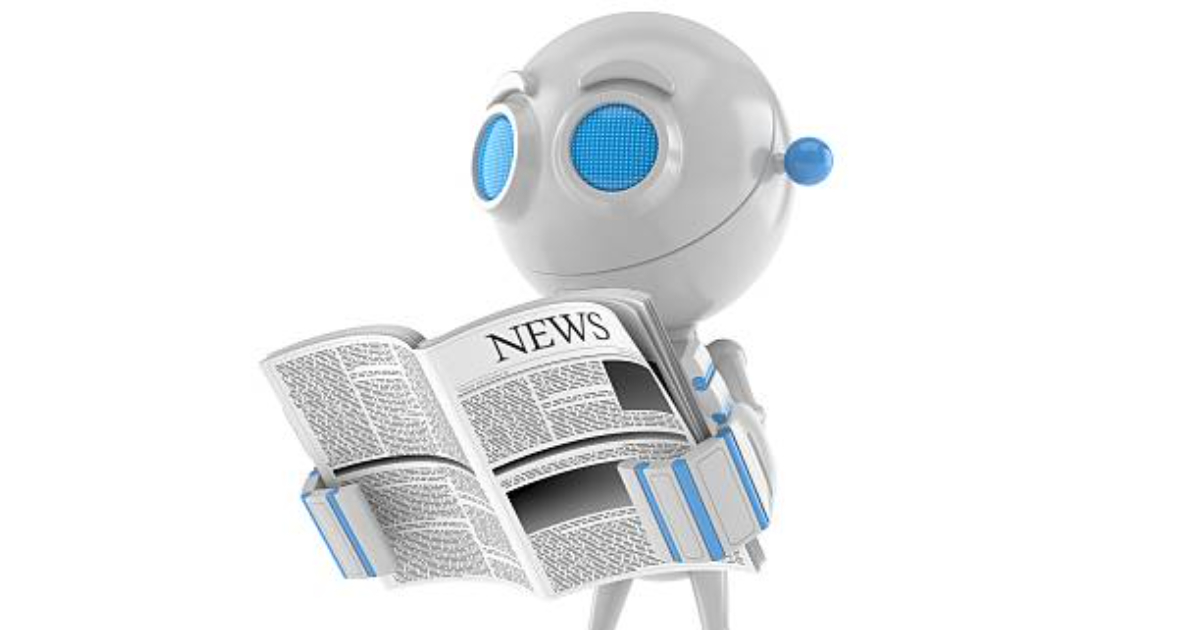
The latest update for the week of April 17th, 2023, includes the recent advancements and announcements in the domains of data science, machine learning, and artificial intelligence. The spotlight this week is on top players like Hugging Face, AWS, Microsoft, and Stability AI, who have introduced groundbreaking innovations.
HuggingGPT: Leveraging LLMs to Solve Complex AI Tasks with Hugging Face Models
A recent paper by researchers at Zhejiang University and Microsoft Research Asia explores the use of large language models (LLMs) as a controller to manage existing AI models available in communities like Hugging Face.
AWS Enters the Generative AI Race With Bedrock and Titan Foundation Models
AWS announced their entry into the generative AI race with the launch of Amazon Bedrock and Titan foundation models. Amazon aims to democratize access to generative AI technology, catering to customers across various industries and use cases. This groundbreaking development positions Amazon as a formidable competitor in the rapidly growing AI market.
In addition AWS announced the general availability of Amazon EC2 Trn1n instances powered by AWS Trainium and Amazon EC2 Inf2 instances powered by AWS Inferentia2, the most cost-effective cloud infrastructure for generative AI.
Microsoft Open-Sources Multimodal Chatbot Visual ChatGPT
Microsoft Research recently open-sourced Visual ChatGPT, a chatbot system that can generate and manipulate images in response to human textual prompts. The system combines OpenAI’s ChatGPT with 22 different visual foundation models (VFM) to support multi-modal interactions.
Stability AI Launches the First of its StableLM Suite of Language Models
Stability AI released a new open-source language model, StableLM. The Alpha version of the model is available in 3 billion and 7 billion parameters, with 15 billion to 65 billion parameter models to follow. Developers can freely inspect, use, and adapt our StableLM base models for commercial or research purposes, subject to the terms of the CC BY-SA-4.0 license.
Global NoSQL Database Market Size Business Growth Statistics and Key Players Insights 2023-2030
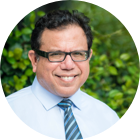
MMS • RSS
Posted on nosqlgooglealerts. Visit nosqlgooglealerts
New Jersey, United States – Our report on the Global NoSQL Database market provides a comprehensive overview of the industry, with detailed information on the current market trends, market size, and forecasts. It contains market-leading insight into the key drivers of the segment, and provides an in-depth examination of the most important factors influencing the performance of major companies in the space, including market entry and exit strategies, key acquisitions and divestitures, technological advancements, and regulatory changes.
Furthermore, the NoSQL Database market report provides a thorough analysis of the competitive landscape, including detailed company profiles and market share analysis. It also covers the regional and segment-specific growth prospects, comprehensive information on the latest product and service launches, extensive and insightful insights into the current and future market trends, and much more. Thanks to our reliable and comprehensive research, companies can make informed decisions about the best investments to maximize the growth potential of their portfolios in the coming years.
Get Full PDF Sample Copy of Report: (Including Full TOC, List of Tables & Figures, Chart) @Â https://www.verifiedmarketresearch.com/download-sample/?rid=129411
Key Players Mentioned in the Global NoSQL Database Market Research Report:
In this section of the report, the Global NoSQL Database Market focuses on the major players that are operating in the market and the competitive landscape present in the market. The Global NoSQL Database report includes a list of initiatives taken by the companies in the past years along with the ones, which are likely to happen in the coming years. Analysts have also made a note of their expansion plans for the near future, financial analysis of these companies, and their research and development activities. This research report includes a complete dashboard view of the Global NoSQL Database market, which helps the readers to view in-depth knowledge about the report.
Objectivity Inc, Neo Technology Inc, MongoDB Inc, MarkLogic Corporation, Google LLC, Couchbase Inc, Microsoft Corporation, DataStax Inc, Amazon Web Services Inc & Aerospike Inc.
Global NoSQL Database Market Segmentation: Â
NoSQL Database Market, By Type
• Graph Database
• Column Based Store
• Document Database
• Key-Value Store
NoSQL Database Market, By Application
• Web Apps
• Data Analytics
• Mobile Apps
• Metadata Store
• Cache Memory
• Others
NoSQL Database Market, By Industry Vertical
• Retail
• Gaming
• IT
• Others
For a better understanding of the market, analysts have segmented the Global NoSQL Database market based on application, type, and region. Each segment provides a clear picture of the aspects that are likely to drive it and the ones expected to restrain it. The segment-wise explanation allows the reader to get access to particular updates about the Global NoSQL Database market. Evolving environmental concerns, changing political scenarios, and differing approaches by the government towards regulatory reforms have also been mentioned in the Global NoSQL Database research report.
In this chapter of the Global NoSQL Database Market report, the researchers have explored the various regions that are expected to witness fruitful developments and make serious contributions to the market’s burgeoning growth. Along with general statistical information, the Global NoSQL Database Market report has provided data of each region with respect to its revenue, productions, and presence of major manufacturers. The major regions which are covered in the Global NoSQL Database Market report includes North America, Europe, Central and South America, Asia Pacific, South Asia, the Middle East and Africa, GCC countries, and others.
Inquire for a Discount on this Premium Report @ https://www.verifiedmarketresearch.com/ask-for-discount/?rid=129411
What to Expect in Our Report?
(1) A complete section of the Global NoSQL Database market report is dedicated for market dynamics, which include influence factors, market drivers, challenges, opportunities, and trends.
(2) Another broad section of the research study is reserved for regional analysis of the Global NoSQL Database market where important regions and countries are assessed for their growth potential, consumption, market share, and other vital factors indicating their market growth.
(3) Players can use the competitive analysis provided in the report to build new strategies or fine-tune their existing ones to rise above market challenges and increase their share of the Global NoSQL Database market.
(4) The report also discusses competitive situation and trends and sheds light on company expansions and merger and acquisition taking place in the Global NoSQL Database market. Moreover, it brings to light the market concentration rate and market shares of top three and five players.
(5) Readers are provided with findings and conclusion of the research study provided in the Global NoSQL Database Market report.
Key Questions Answered in the Report:
(1) What are the growth opportunities for the new entrants in the Global NoSQL Database industry?
(2) Who are the leading players functioning in the Global NoSQL Database marketplace?
(3) What are the key strategies participants are likely to adopt to increase their share in the Global NoSQL Database industry?
(4) What is the competitive situation in the Global NoSQL Database market?
(5) What are the emerging trends that may influence the Global NoSQL Database market growth?
(6) Which product type segment will exhibit high CAGR in future?
(7) Which application segment will grab a handsome share in the Global NoSQL Database industry?
(8) Which region is lucrative for the manufacturers?
For More Information or Query or Customization Before Buying, Visit @ https://www.verifiedmarketresearch.com/product/nosql-database-market/Â
About Us: Verified Market Research®Â
Verified Market Research® is a leading Global Research and Consulting firm that has been providing advanced analytical research solutions, custom consulting and in-depth data analysis for 10+ years to individuals and companies alike that are looking for accurate, reliable and up to date research data and technical consulting. We offer insights into strategic and growth analyses, Data necessary to achieve corporate goals and help make critical revenue decisions.Â
Our research studies help our clients make superior data-driven decisions, understand market forecast, capitalize on future opportunities and optimize efficiency by working as their partner to deliver accurate and valuable information. The industries we cover span over a large spectrum including Technology, Chemicals, Manufacturing, Energy, Food and Beverages, Automotive, Robotics, Packaging, Construction, Mining & Gas. Etc.Â
We, at Verified Market Research, assist in understanding holistic market indicating factors and most current and future market trends. Our analysts, with their high expertise in data gathering and governance, utilize industry techniques to collate and examine data at all stages. They are trained to combine modern data collection techniques, superior research methodology, subject expertise and years of collective experience to produce informative and accurate research.Â
Having serviced over 5000+ clients, we have provided reliable market research services to more than 100 Global Fortune 500 companies such as Amazon, Dell, IBM, Shell, Exxon Mobil, General Electric, Siemens, Microsoft, Sony and Hitachi. We have co-consulted with some of the world’s leading consulting firms like McKinsey & Company, Boston Consulting Group, Bain and Company for custom research and consulting projects for businesses worldwide.Â
Contact us:
Mr. Edwyne Fernandes
Verified Market Research®
US: +1 (650)-781-4080
UK: +44 (753)-715-0008
APAC: +61 (488)-85-9400
US Toll-Free: +1 (800)-782-1768
Email: sales@verifiedmarketresearch.com
Website:- https://www.verifiedmarketresearch.com/
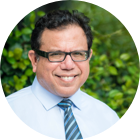
MMS • Artenisa Chatziou
Article originally posted on InfoQ. Visit InfoQ
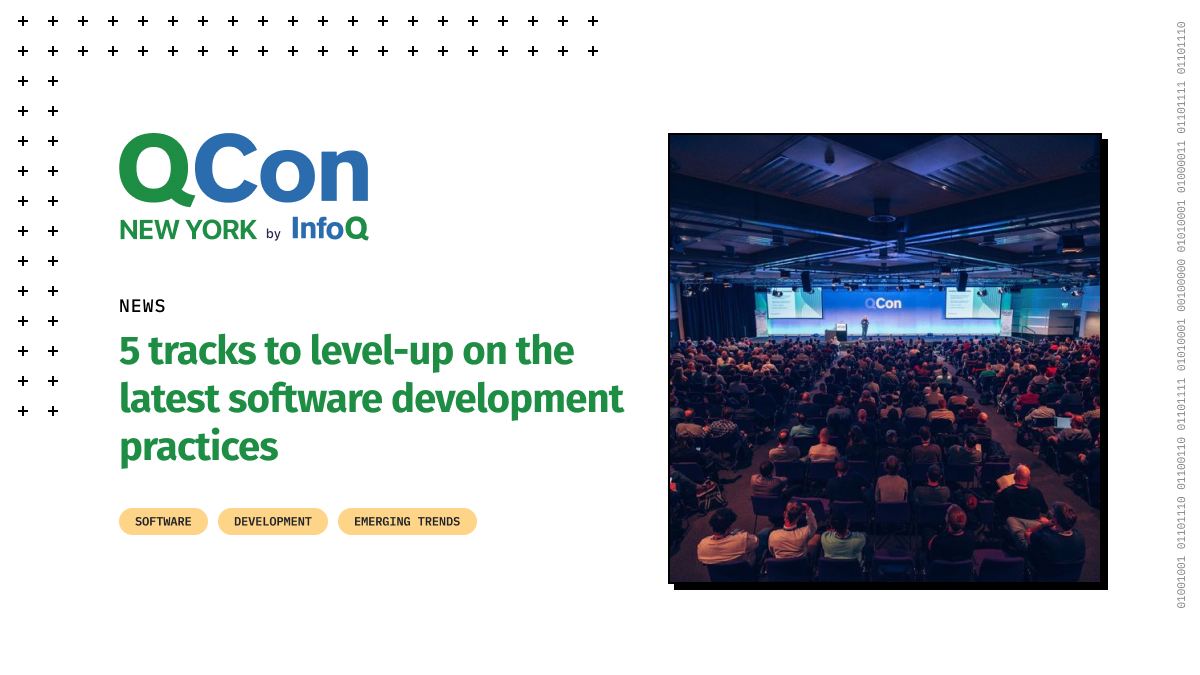
The 2023 edition of the QCon New York (June 13-15) software development conference, hosted by InfoQ, is set to bring together over 800 senior software developers. The three-day conference will feature over 80 innovative senior software practitioners  from early adopter companies sharing how they are solving current challenges, providing new ideas and perspectives across multiple domains to provide clarity on emerging tech decisions. You will learn from real-world practitioners who are applying emerging trends and practices to help you solve common problems.
With 15 tracks to choose from, these are 5 essential tracks that senior software developers, software architects, and tech team leads won’t want to miss:
-
“Modern Data Architecture & Engineering,” led by Allen Wang, Senior Staff Engineer at DoorDash, will focus on the evolving landscape of data technologies and SaaS platforms, and the core engineering principles that can be leveraged to build efficient and scalable data architectures.
-
“Architectural Blueprints Of Real World Machine Learning Systems,” will be led by Nikhil Garg, CEO of Fennel, and will explore the architectural components of real-world machine learning systems, such as recommendation systems and fraud detection, and delve into the challenges and performance considerations of these complex distributed systems.
-
“Connecting Distributed Systems: APIs Done Right,” led by Daniel Bryant, Head of DevRel at Ambassador Labs & InfoQ News Manager, will provide insights from leading organizations on designing and operating distributed systems and APIs, sharing lessons learned and best practices.
-
“Architectures You’ve Always Wondered About,” led by Wes Reisz, Technical Principal at Thoughtworks & Creator/Co-host of #TheInfoQPodcast, will delve into the architectures of well-known tech companies and explore patterns, anti-patterns, best practices, and challenges behind delivering exceptional user experiences and supporting massive user bases.
-
“Staff+ Engineering: New Skills, New Challenges,” hosted by Leslie Chapman, Engineering Fellow at Comcast, will focus on the skills and challenges that come with leading large projects and setting the technical direction for organizations, providing practical advice from experienced high-level individual contributors.
Early bird tickets for QCon New York are available until May 9, 2023, offering a chance to save on registration. Don’t miss this opportunity to level-up on the latest software development practices, connect with a global developer community, and gain insights from senior software practitioners, and InfoQ Editors.
Boosting Quarkus Native Performance: Should You Stick with Space/Time or Switch to Adaptive GC?
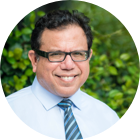
MMS • A N M Bazlur Rahman
Article originally posted on InfoQ. Visit InfoQ
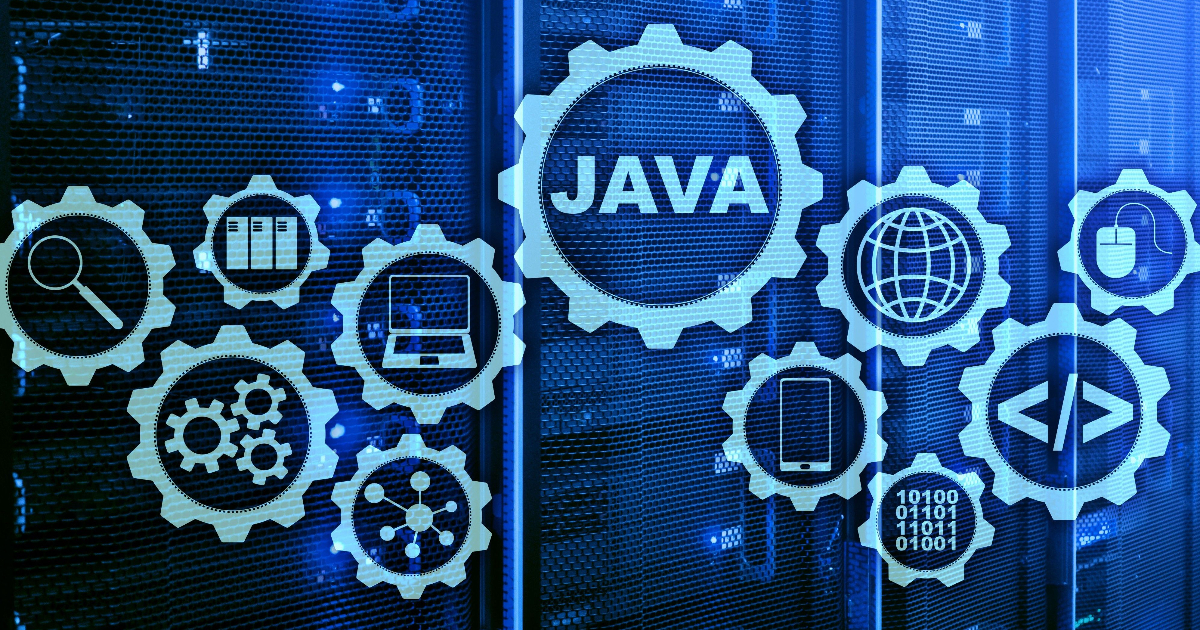
Quarkus, the Kubernetes Native Java Framework, has switched its default garbage collection (GC) policy from space/time to adaptive GC for native runtime in version 2.13.6.Final. Adaptive GC is designed to aggressively trigger GCs in order to keep memory consumption low. This makes it more effective in situations where a low memory footprint is important, and it appears to perform better under heavy stress.
This is in response to concerns about the impact of the space/time policy on memory consumption and performance in some situations. While space/time is generally effective in balancing young and full GCs and is currently the default for Quarkus, it can cause odd memory consumption when physical memory is over three gigabytes, and no maximum heap size (-Xmx
) is configured.
Adaptive GC is GraalVM CE’s default GC policy for serial GC. It is based on HotSpot’s ParallelGC adaptive size policy but with a greater focus on memory footprint. This means that, unlike space/time, it aggressively triggers GCs to keep memory consumption down. Adaptive GC tracks the pause time in consecutive minor collections and schedules a full GC if a threshold is passed, making it more effective at mitigating potential GC nepotism issues.
However, Quarkus may still choose to retain space/time as an option. The lack of survivor spaces in space/time means that young-lived objects can easily build up in the old generation until full GC, leading to potential spikes in memory consumption. Furthermore, some developers may still prefer the predictability of space/time in certain situations.
There are potential risks associated with both policies. The space/time policy requires the passing of a non-public API (-H:
) to set up, which may not be sustainable in the long term. On the other hand, switching to adaptive GC could generate fewer full GC events, which is one of space/time’s main features. The serial GCs old generation could also switch from copying to mark-sweep-compact, which would require further evaluation of the cost of full GCs.
Quarkus’ potential switch to the adaptive GC policy underscores the importance of selecting the right GC policy for the situation. Developers must consider factors such as physical memory, workload stress levels, and the need for a low memory footprint. A deep understanding of GC policies can help developers optimize application performance and minimize memory consumption.
Developers who are interested in learning more about the investigation into native memory usage in Quarkus can refer to the original GitHub issue for further details. Quarkus has also made it easier for developers to optimize how their apps use memory by adding a new section to their Reference Guide called “Native Memory Management.” By taking advantage of these resources, developers can gain a deeper understanding of how their applications use memory and make more informed decisions to improve performance.
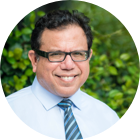
MMS • Ian Thomas
Article originally posted on InfoQ. Visit InfoQ
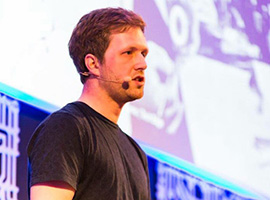
Transcript
Thomas: Welcome to my talk about orchestration versus choreography, patterns to help design interactions between our services in a microservices architecture. To start off, I’m going to take us on a little tangent. I want to talk to you about footballs, specifically this ball that’s on screen right now, which is the official match ball for the World Cup 2022. Footballs consist of many different panels that are normally stitched together to make a sphere. In modern footballs, they’re not stitched together using traditional methods anymore. They’re thermally bonded. The panels can be of all sorts of different shapes and sizes. This allows manufacturers to make balls as round as possible, which contributes to different properties and makes them fly faster and straighter, and give players more options for giving the ball bend and stuff like that. Just, park that idea for a second because I’m going to take you on another tangent, which is about the bit that’s inside this football. This football is incredible. It’s got a sensor in the middle that it will be measuring different aspects of the ball 500 times a second, so that FIFA can make better refereeing decisions. They can determine how fast the ball is moving, how often it’s been kicked, where it is on the pitch. It can help inform decisions around offsides, and other things like that. Also, they’re going to use it to produce AI graphics so that you can see at home exactly what’s going on at any time, with absolute millimeter precision.
This isn’t what I wanted to talk to you about footballs for. Remember, I was talking to you about those panels. I think the panels on a football, especially old footballs, bear a striking resemblance to how our software systems look. Take this, for example, this ball has been well and truly used to within an inch of its life. You can see that it’s worn, and the seams are starting to show. In certain places bits are falling off it. This is a bit similar to how our systems age, if we don’t look after them properly. This one here you can see has been pushed even further, and it’s no longer even round. It’s debatable whether this will roll properly, whether it will fly straight through the air. It’s really in a bad way. If we let our systems get in this state, there’s normally only one course of action left for us, and that’s to throw it away and start again with something new. If we don’t take action soon enough, perhaps we’ll see something like this, where bits of our football will explode and the innards will pop out and it becomes completely unusable. It might still have a chance of being fixed, but it’s a serious incident. Or if it’s just too far gone, and everything’s exploded, and there’s no chance of fixing it, we literally are left without any choice but to start again. A very costly mistake.
Background
I’m Ian. I’m a software architect from the UK. I want to talk to you about quite a few things that, unlike the footballs, help us to maintain our systems to keep them in good working order over time. Specifically, I want to look at this idea of change and how the decisions that we make when we’re designing our architectures, and the way that we implement our architectures affects our ability to effect it. Within that context, I want to think about coupling and complexity and various other aspects of how we put our systems together, including the patterns that we might use, such as orchestration and choreography that help us to effect long term evolvable solutions. As we go through this talk, I’d like you to keep in mind these five C’s that I’ve called out on screen right now. I want you to think about them not just from a technology perspective, but also from a human and organizational perspective. Because ostensibly, this is a talk about decision making in design, and that involves people as well as systems.
Lehman’s Laws of Software Evolution
If we start at the point of change, a good reference, I think to help us understand why change is necessary is a paper by a man called Manny Lehman who was a professor at Imperial College London. Before that, a researcher at IBM’s division in Yorktown Heights, New York. His paper programs life cycles and laws of software evolution, lays out some laws that I think are quite relevant for this discussion. He’s got eight laws in total, and I’m not going to go through all of them in individual detail. I just want to call out four in particular that all relate to various things around how we will build and have to maintain our systems. Change, complexity, growth, and quality, they’re all affected and describe the need for change. Without change, our systems become less satisfactory. Without growth, they become less satisfactory. By adding change and adding things as new features, without intentional maintenance or intentional work to manage and reduce complexity, the effort to change in the future is also made harder, so we slow our rate of change possible. Then this idea at bottom left of declining quality is a really interesting one, because it’s saying that, essentially, stuff’s changing all around our system, and if we don’t keep up with it, then we’re also going to have a perception of reduced quality.
That leads on nicely to this other framing, which is thinking about where change comes from and what stimulus triggers it. The orange boxes are fairly obviously things that happen outside of our system. Either user needs or feature requirements or wanting to adapt to modern UI standards, whatever. These are things that would cause us to have to update our systems. The purple ones, they reflect more the problem that if we’re not careful and deliberate in the way that we want to work with our systems and put the effort in to maintain them properly, we will self-limit our ability to impose change on the systems. The two that are in the middle, the organizational stability and conservation familiarity laws are really interesting from a sociotechnical perspective, because they are effectively talking about the need for teams to remain stable. They can retain a mastery at a decent enough level to make change efficiently. Also, they need to be able to keep the size of the system to a relatively stable level. Otherwise, they can’t hold all the information in their heads. It’s an original source of talking about cognitive load. Useful things to think about.
In his paper, Manny Lehmann calls out how complexity and how the growth of complexity within a system leads to a slowdown in change. This graph really clearly shows how, over a number of years, the ability to effect change is slowed quite rapidly. The amount of change that happens at each release is reduced. Typically, when we think about microservices, it’s in the context of our organizations wanting to scale, and that’s not just scaling the systems themselves, it’s also scaling the number of people that can work on them. We’d like to think that adding more people into our business would lead to an acceleration in the rate of features that we deliver. Actually, I’ve seen it more often than not where without proper design and without proper thought into how we carve up our systems and the patterns that we use, actually adding more people slows us down. Just take a minute to think which of these charts most closely matches your situation, and perhaps have a reflection thinking why it is that you are in the situation you’re in.
Workflows
We’re talking about interaction patterns between our services. That probably means we’re thinking about workflows. It’s useful to introduce the concept of what a workflow actually is. I’ve got this rather crude and slightly naive version of an e-commerce checkout flow to hopefully highlight to you what I would consider a workflow. You can see there are various states and transitions in this diagram. If you go along the top, you’ve got the happy path. If you also look, there are quite a few states where we’ve had a problem, and we need to recognize that and handle it. Going through this, you can see there are lots of different things to consider not least the fact that some of these stages don’t happen straightaway. Clearly, once we’ve processed the payment, the product isn’t going to immediately jump off a shelf and into a box and into a wagon to deliver it to your house. Equally, if there are problems, some of them are completely outside of our control, and people end up getting stuck in quite horrible ways.
One of the useful things about having diagrams like this, when we’re thinking about designing systems, is that we can start to look at meaning behind them. Here I’ve drawn some boxes around related areas and related states. This is where we start to see areas that we could perhaps give to teams, individual ownership. A lot of the terminology that I’m going to use to talk about these workflows will relate to domain driven design. Just as a quick recap, to help make sure everyone’s on the same page with what the words mean, I’m going to use, the blobs in the background, they represent bounded contexts. A bounded context is a way of thinking about a complete and consistent model that exists strictly within the bounds of that bounded context. People from different aspects of the business will have shared understanding of what all the terms and models mean, and that is our ubiquitous language. It’s important to think that within a bounded context, we’re not just saying it’s one microservice. There could be many services and many models in there. The key thing is that they’re consistent. We could further group things within a bounded context by creating subdomains. The last thing to call out is the bit between all of these bounded contexts is really important because, ultimately, these things have to work together to provide the value of the whole system. That bit in between is where we define our contracts. It’s the data layer. It’s the interchange context that allows us to transfer information between our bounded contexts in a understandable and reliable way.
With that being said, we’ll just zoom back out to the overall workflow, and look at this in a different way too, because, again, it’s helpful to think about the meaning when you see the lines between the states. Here, we can identify when something might be considered an external communication. We’re going to have to think about our interaction patterns and our interchange context, or whether it’s an internal communication, and we’ve got a lot more control. We don’t have to be quite as rigorous and robust in our practices, because we have the ability within the team to control everything.
Interaction Patterns – Orchestration
Let’s move on to interaction patterns. I’m going to start by talking about orchestration, because perhaps it’s the slightly simpler of the two to understand. Orchestration typically is where you would have a single service that acts as an orchestrator or a mediator, and that manages the workflow state. You would probably have a defined workflow that it knows about and has modeled properly. It involves organizing API calls to downstream services that are part of bounded context. There’s generally no domain behavior outside of the workflow that it’s mediating, it’s generally just there to effect the workflow and all the domain knowledge is in the bounded context themselves. One of the more naive ways of thinking about orchestration is that it’s a synchronous approach. That’s true to an extent, because a lot of the times the orchestrator will be calling into these services using synchronous API calls. It doesn’t necessarily mean that the overall workflow is synchronous. Because we’re using these synchronous calls, it does mean that there might be a tendency for there to be some latency sensitivity, especially if things are happening in sequence. If the workflow is particularly busy, and is a source of high traffic, that scale is obviously going to cascade too, which might also lead to failure cascading down.
Clearly, this is a very naive view of what an orchestration workflow might look like. One of the things that’s quite interesting to consider is that actually, orchestration does have informal implementations too. You might have quite a few of these without really thinking about it within your code bases. Where we have bounded contexts before that just looked like they were the end of the request from the orchestrator, actually, they could act as informal orchestration systems themselves. We had such a system like this in a backend for frontend application that we designed when I was working with PokerStars sports. Here you can see, it’s a fairly straightforward pattern to implement, because we’re only going to go to three services to build up data. The end result that a customer will see relies on calling all three of these systems. Now this UML sequence diagram is a little bit dry. Some of the problems with this are probably better called out if we think about it in slightly more architectural diagram manner. The key thing to show here is that we kind of see this representation of a state machine in this flow. One of the things that’s missing is all the error handling. This is the problem with this approach. Because in a general-purpose programming language, you might find that these ad hoc state machines exist in a lot of places. Essentially, these workflows aren’t just state machines or statecharts, or however you want to call it. If we’re not careful, and we don’t think about them, and design them properly, and do the due diligence that you might do if you were defining a workflow using an actual orchestrator system, such as Step Functions or Google Workflows, then it’s easy to miss some of the important nuances in error handling scenarios.
Human-in-the-Loop and Long Running Workflows
Another thing to think about, and I mentioned it before, is that there’s this simplification about orchestration being synchronous, and choreography is asynchronous. Actually, if you think about it, a lot of the workflows that you will be modeling in your architectures often have human in the loop. What I mean by that is that there’s some need for some physical thing to happen in the real world before your workflow can progress. Think about a fulfillment in our e-commerce example. Perhaps closer to home a better way to emphasize what I mean here is to think about something that most developers will do. Whether you work in e-commerce or not, this will hopefully bear some resemblance to your working patterns, and that is the development workflow. Because here we’ve got a good example of something that needs to have both human and automation aspects. There are systems that need to talk to each other to say, yes, this has happened or, no, it hasn’t, in the case of maybe a PR being approved and that triggering some behavior in our CI pipeline to go and actually build and deploy to a staging environment, for example. Then you can also see how there’s needs for parts of this workflow to pause, because we’re waiting for a manual approval or the fact that a PR review is not going to happen instantaneously, there are things happening in the real world that need to be waited on.
Handling Failure
The other thing to think about because we’re using APIs a lot with these orchestration patterns is that there’s significant things like failures that we have to consider in our application design. Thankfully, I think we’ve got quite a mature outlook on this now as an industry. Many of these patterns are very well known and often implemented in our technology by default. With the rise of platforms and platform teams, and the way that they think about abstracting the requirements for things like error handling and failure handling, you often find that many of these requirements are now pushed to the platform themselves. For example, if you’re using something like a service mesh. In the cases where the failure and/or errors are expected, and they’re going to be part of the workflow themselves, so they’re not unexpected things like network timeouts, or similar problems. The nice thing about having a central orchestration system is that when a thing goes wrong, you can model the undos, the compensatory actions that you need to be taking to get the previous steps in your workflow back into a known good state. For example, here, if we had a problem where we couldn’t fulfill an order and we needed to cancel the payment and reallocate stock or update our inventory in some way, it’s easy for the orchestrator to manage that because it has knowledge of the whole workflow. The last thing to say is that because of the way you can build some of these workflows using cloud systems, like Step Functions, and Google Workflows, or Azure Logic Apps, it’s very trivial to have a single instance of an orchestrator per workflow. Rather than one single thing for all workflows, you can build them similar to how you would build your microservices, so you have one per specific use case.
Orchestration, Pros and Cons
Orchestration, pros for it. There’s a lot you can do to help with understanding the state and managing the state of your workflows. That means that you can do quite a lot of stuff regarding error handling and complex error handling in a more straightforward and easily understood way. Lots of platform tooling exists to help you remove some of the complexity around unexpected failures and how you’d handle them. Ultimately, there’s probably a slightly lower cognitive load when working with highly complex workflows. On the negative sides, though, because you have the single orchestrator at the heart of the system, you do have a single point of failure, which can cause some issues. That partly might lead to an issue with scalability or additional latency because the orchestrator itself is acting as the coordination point, and all requests have to go through it. You might also find there are some problems with responsiveness. Generally, because of the way you’re coupling things together with the APIs and the knowledge of the workload being encoded into the orchestration system, there is a higher degree of coupling between the orchestrator and services.
Interaction Patterns – Choreography
Let’s move on to choreography, and think about how that might differ a bit to orchestration. The easiest thing to say, and the most obvious thing to say outright is that there is no single coordinator that sits at the heart of this. The workflows are a bit more ad hoc in their specification. That normally is implemented using some intermediate infrastructure like a Message Broker, which is great because it gives us some nice properties around independence and coupling, and allowing us to develop and grow and scale our systems in a slightly more independent way. Because of that, as well, it might mean that we’re slightly less sensitive to latency, because things might be able to happen in parallel. There are additional failure modes to consider, and there’s other aspects to managing the overall workflow that will become apparent as we go through in the next few slides.
Again, this is a pretty naive view. If we look at something that’s a bit more realistic where there might be several events being emitted by different bounded contexts or many more bounded contexts involved in the overall workflow, you can see that there are some problems come in the form of understanding the state of things, and also the potential to introduce problems like infinite loops. It’s not beyond the realms of possibility to think that the event coming out of our bottom blue bounded contexts could trigger the start of a workflow all over again, and you could end up going round and round. Decisions that you have to make when you’re working in this way, include thinking, I’m not going to use an event or I’m not going to use a command. A command would imply that the producer knows about what’s going to happen in the consumer. That’s perhaps not necessarily the most ideal scenario because one of the things we’re trying to get out of this approach and one of the benefits that we get is having weaker coupling. Maybe that’s inferring a bit too much knowledge about the downstream systems. If we do use events, they’re not just plain sailing either. There’s quite a few things to consider there, which we’ll talk about. I think on balance, I would generally prefer to use an event over a command, because it would allow us to operate independently as a producing system and allow many consumers to hook into our events without necessarily having to infer that knowledge onto the producer. Some words of warning, though, issues can arise if events are rebroadcast, or if you have multiple different systems producing the same event. Something to keep in mind, it should be a single source of truth.
When we have got events, Martin Fowler’s article about being event driven, provides four nice different categorizations of the types of event that you might want to consider using. Starting from the top and the most simple is event notification, where you’re basically just announcing a fact that something has changed. There’s not much extra information passed along with that notification. If there is a downstream system that’s interested in that change, it probably means it’s going to have to call back to the source of the event to find out what the current state of the data is. If you’re not happy with that, and that’s a degree of coupling and chattiness that you’re not wanting to live with, then you could use the event carried state transfer approach, which would reduce that chattiness. It would infer that there’s going to be more data being passed within our events. It does allow you to build up your own localized view of an event or an entity. That might mean that the downstream systems are more responsive, because they’re able to reply without having to coordinate with an upstream system if they get a request, which is a nice property to have.
Event sourcing takes that a step further, where all the events are recorded in a persistent log. That means that you can probably go back in time and replay them to hopefully get you back to a known good state. That’s assuming that when you are processing these events in the first place, there’s no side effects to going off to other systems that you would have to think about and account for when you were doing a replay. Another thing that needs to be considered in this approach is how you would handle schema changes and the need for consumers to be able to process old events produced with one format and current events that might have a new, latest schema. The last pattern to talk about here is CQRS. The only thing I’m really going to say about this is it’s something that you should perhaps consider with care and have a note of caution about, because while on the face of it, it sounds like a really nice idea to be able to separate out your patterns for reading and writing, actually, there’s quite a lot of nuance and stuff that can go wrong there that might get you into trouble further down the line. If you are interested in that pattern, do go away and have a read, and find out more for yourself and think about the consequences of some of the design choices.
Once we’ve decided on what type of event we’re going to use, we have to then think about what message pattern we’re going to use. Because again, we’ve got quite a lot of stuff to think about when we’re designing. They have implications on the performance of our system and the coupling between the components. If we’re going to look at a standard AMQP style approach, where you’ve got different types of exchanges, and queues, we could look at something like this and say, we’ve got a direct exchange and we’re going to have multiple queues bound to different routing keys. Here, I’ve called out a couple of different decisions that you’re going to have to make. Because again, it’s not as straightforward as it might first appear, you’ve got to think whether you’re going to have durable or transient exchanges or queues, whether you’re going to have persistent or transient messages. Ultimately, you’re going to have to think a lot about the behaviors you want to have if the broker goes down or if a consumer stops working. One of the things that you might find here is that while messages will be broadcast to all queues that are bound to the same routing key, so every consumer, every queue will get all messages that it’s interested in. If you bind multiple consumers to a queue, you end up with what’s known as a worker pool. This is great if you have no real need to worry about ordering. If precise ordering is important, then this can have implications on the outcomes of your system. Again, it’s another consideration you have to take into mind when you’re thinking about implementing things.
Other types of exchanges that you can have include fanout. While this is great, because it means that you can broadcast all messages to all consumers in a really efficient way. If you choose to use event carried state transfer, then it might mean that you’re sending an awful lot of data because each queue receives a copy of each event. That might have implications on your bandwidth and the cost of bandwidth. Depending on your world, it might make it more expensive or it might saturate and you have a limit to the throughput you can achieve. The last type of exchange you can have in this setup is a topic exchange where your consumers can define queues that bind to patterns. They can receive multiple different types of messages through the same queue, which is great. There’s a lot of great things you can do with this. Again, if you have a high cardinality, in the set of types of messages you’re going to receive or lots of different schemas, in other words, this can have serious implications for the ability of your consumers to keep up with change.
Other approaches that you might have for these systems include something like this, which is a Kafka implementation, so it works slightly differently to the previous ones. In that it’s more akin to the event sourcing model where each record is produced to a topic and stored durably in a partition. There’s no replication, there’s no copies of events going on here, it’s a slightly more efficient way to allow multiple consumers to consume the same data. Because of the way that partitions work, a nice property is that as you scale your consumers, assuming you don’t scale beyond the number of partitions in your topic, you’ll be able to manage to process things really efficiently, because you can assign more resources, so a consumer per partition, but you also retain your ordering within the partition. We’re not having the same issues as we would do with the round robin approach from before. Another nice property of using something like Kafka is that your consumer groups basically keep a record of where they are independently of each other. You’re not worried about queue backup or anything like that, because ultimately, it’s just the same data being read. It’s an offset that’s being updated by the consumer itself, by the group itself. Again, things to consider here is how you want to handle offset lag. Because if your offset lag grows too much, and the retention policy of your topic is set such that it will start to delete messages before they’ve been consumed, then you’ve got big problems.
Choreography Considerations
Some of the considerations that I’ve called out there just summarized in one place, and a couple of extras to think about as well. Two, in particular, on the left-hand side, that I think people need to be aware of are delivery semantics. Are you going to go for at-least once or at-most once delivery. I would imagine properly, at-least once is the preferable option there to make sure that you’re going to actually receive a message. Then that means you’ve got to answer the question of what you’re going to do if you receive an event twice. That’s where idempotency comes in. On the right-hand side, the key differences there between orchestration and choreography, relate more to the error handling, which I’ll touch on. Also, how you’re going to handle a workflow level timeout, or something where you want to see the overall state of a workflow. They become much harder in this world because there is no central organizing element that’s going to be able to tell you the answers or coordinate a timeout in that way.
I mentioned error handling. If we go back to this original view of how things might go wrong, you can see how if we’ve got a system that’s choreographed, and we’re using events to say all the different transitions that should happen, if we get to a point where we have payment that fails, we might already have allocated stock. At that point, we’ve got to work out how we’re going to make a compensatory action. You can see for that slightly simplified view, it’s probably ok, you could probably work out a way to emit an event and make your stock system listen to the event and then know what to do when it hears it. If you’ve got a more complicated or an involved workflow, having to do those compensatory actions in an event driven system becomes increasingly difficult.
Choreography, Pros and Cons
In summary, then, choreography, you have lots of good things to think about because you get a much weaker coupling between your services if you do it right, which leads to greater ability to scale things, more responsiveness in your services. Because you’ve got a single point of failure, you have a decent chance of making more robust fault tolerance happen. You could also get quite high throughput. There’s a lot of good things to think about here. On the con side, if we have a particularly complicated workflow, especially if you’ve got complicated error handling requirements, then the complexity of that implementation grows quite rapidly. Other things that you need to bear in mind is that it might not be possible to version control the end-to-end workflow, there’s no single representation of it, which is something that is again, in a complicated workflow, quite a nice property to have.
Choosing Your Patterns
How would we go about choosing between these different approaches? If you’ve read about this or seen any content on the internet, you might have come across this blog post from Yan Cui, where he basically says, as a rule of thumb, say, orchestration should be used within a bounded context of a microservice, but choreography between bounded contexts. To put that into our diagram from before, that would mean that within inventory and payment, we’re all good to use our orchestration systems, but between the bounded context, we’re going to just send events. I think actually, that’s quite problematic, especially when you consider what I was talking about with complicated error handling.
How Formally Do You Need to Specify your Workflow?
If we think about how we might want to handle coordination and understand the overall workflow, that’s where we can start to think about this continuum of how formally specified or informally specified, our workflows might be. On the far-left hand end we’ve got something that’s a very specific orchestration service, say like a Step Functions, where we can use a DSL or a markup language like YAML, or JSON, to define the various stages of our workflows. We can be very declarative in explaining it, and we can version control it. In some cases, you can even use visual editors to build these workflows too. Stepping inward and thinking about the example I gave with the backend for frontend approach, you could use a custom orchestrator that’s defined using a general-purpose programming language, but then you’re taking on the responsibility of ensuring you’ve handled all the errors properly yourself. It’s perhaps a little bit less robust overall, for more complicated approaches.
Go into the other end, as I mentioned, choreography doesn’t necessarily have the same ability to specify your workflows in one place. There is an approach you can take where you would use something called a front controller where the first service in a workflow has a burden of knowledge of the rest of the workflow. It can be used to query all the systems involved, to give you an accurate update of the state. There is a way that you can semi-formally specify the workflow in some regards. Typically, right at the very right-hand end you would have what would be a more stateless approach. There is no central state management. In this case, the best you can hope for is documentation. Possibly, you’re only going to have the end-to-end understanding of the workflow in a few people’s heads. If you’re unlucky, you probably don’t have anybody, you might not have anybody in your business that knows how everything works in one place. There’s a bit of a gap in the middle there. If that is a SaaS opportunity, and we’re in the right place in San Francisco, for the brains to think about an opportunity to build something there, if you do do something and you make money off it, please keep me in mind.
CAP Theorem
Distributed systems talks wouldn’t be complete without a reference to the CAP theorem. This is no different. There’s a slightly different lens to this that I want to broach, which is thinking about the purpose of microservices from the perspective of an organizational view. Traditionally, we think about this in terms of the technology and saying that in the face of a network partition, you can either choose to have consistency or availability, but not both. If we flip this around and say, how does this apply to our teams, bearing in mind that now we have very distributed global workforces? If we’ve distributed our services across the world, we need to think about how the CAP theorem might apply to our teams. Instead of partition tolerance, if we replace that with in the face of a globally distributed workforce, we can either optimize for local consistency, so teams are co-located, and they’ve got a similar time zone, so they can make rapid decisions together. They can be very consistent within themselves. Obviously, they can’t be awake 24 hours, so they’re not going to be highly available. On the other hand, you might say, actually, it’s important the teams are available to answer questions and support other teams around the world, so we’ll stripe the people within them across different time zones. In that case, yes, you’ve improved your availability, but again, people can’t always be online all the time. The ability for the team to make consistent decisions and have local autonomy is reduced. Perhaps it’s a rough way of thinking about it, but it makes sense to me.
Complexity and Coupling
Complexity and coupling are clearly going to be key elements that allow us to build these systems and have successful outcomes in terms of both the effectiveness of how they work, but also the long-term ability to change them. One thing to say is that you can have a degree of coupling that is inevitable, a domain will have necessary coupling and it will have necessary complexity. That’s not to say that you can’t push back and say, actually, this thing you’re asking me to do, is going to be almost impossible to build and is there a simpler way we can do it. Generally, there’s a degree of coupling and complexity that we have to accept, because it’s mandated by the domain requirements of the solution. However, what we can find as architects and tech leads, is that how we choose to implement our workflows can have a significant impact on how much worse we make things. We need to really consider coupling in quite a deep level of detail.
Michael Nygard offers his five different types of coupling, which I think are a really nice way of thinking about it. In particular, I’m really interested in the bottom one, incidental coupling, because especially when you think about event driven systems, this is where things can go bang or go wrong. You say, how on earth are these things linked? Another way to look at this is to consider whether coupling is essential, as I mentioned, or accidental. In this frame, I think you could say the essential stuff is that this is the necessary coupling for the domain that you’re working with. Whereas accidental, is probably down to not doing enough design, or the right design up front. This can manifest itself in slightly more subtle ways, it might not necessarily be that you’re being called out every night for incidents, it might be that you see something like this appearing. This is from when I was working at Sky Bet back in about 2018, and we had a situation where we’d decomposed our systems into smaller microservices or smaller services, and teams owned them nominally with a you build it, you run it mindset. We were finding increasingly that the amount of work it required another team to do some work on our behalf was growing all the time. Our delivery team put together this board, so we could map up the dependencies and talk about and have a standup every week. I can guarantee you after a short amount of time, there wasn’t an awful lot of whiteboard left, it was mainly just all Post-it notes.
To really emphasize just how bad this thing can be if you don’t get your coupling right, let’s have a think about this example from “Making Work Visible,” where Dominica DeGrandis introduces the idea of going for a meal at a restaurant where you’re only going to get seated if everyone arrives on time. If you’re both there on time, and it’s two people going for a meal, great, you’re going to get seated. If the other person’s late or you’re late, or you’re both late, you’re not going to get seated on time, so you’ve got a 1 in 4 chance. If a third person is added to the list of attendees, then your chances decrease quite rapidly. Instead of being 1 in 4, it’s now 1 in 8, or 12.5% chance of being seated. Adding more people over time, it quickly drops your chances of being seated on time to effectively zero because the probability is 1 over 2 to the n. To put this in a slightly more visual format. If we think about our coupling and the number of dependencies in the system, that model shows that if we have 6 dependencies, we’ve only got a 1.6% chance of success. If we have 10, it’s less than 0.1%. Clearly, dependencies are very problematic. Coupling can be very problematic. We have to be very deliberate in how we design things.
Another example from a company at a slightly different scale to us when I was at Sky Bet, is this from “Working Backwards” by Colin Bryar, and Bill Carr. They talk about this NPI approach where you would have to submit your prioritization request to say, I need another team to work on this thing, because it’s important for my thing that I’m trying to deliver. At its worst case, what happened was you would submit your requests, nothing would be prioritized for you. Instead, you’d be told to stop working on your projects that you thought were important because you were being prioritized to work on somebody else’s stuff. You were literally getting nothing for something. With all that being said, I’m going to add a sixth thing onto our list of types of coupling that we have to bear in mind when we’re deciding on these patterns. That is, what’s the level of organizational coupling that we’re going to be putting into our system? That’s when progress can only be achieved together. The last thing to say about coupling is that often when we think about microservices, we talk about decomposition and taking our monolith and breaking it up. Again, if we’re not deliberate in our design, and we don’t put enough thinking into it, we can get into a position where we’re actually adding coupling rather than removing it, so decomposition does not necessarily equal decoupling.
Complexity in our systems is something I’ve touched on and I mentioned that Manny Lehman had some of his laws that touched on thinking about cognitive load. I think this model helps to show a way of thinking about this and how we might want to be concerned and addressing cognitive load when we’re thinking about our patterns. This is an example from, I think it’s Civilization 4. It’s typical of most of real time strategy games where you have a map that you basically can’t see anything in apart from a bubble that’s your immediate area, and you go off exploring to find what’s there. The dark bits are the bits you haven’t yet seen. The slightly faded bits are areas that you’ve visited, but you no longer have vision of. You have an idea of what’s there, but realistically anything could be happening there. You don’t actually have visibility. You just have a false hope that you do know what’s going on. I think this maps nicely to thinking about an architecture. If we consider the whole map to be our city map, or whatever that you would describe your system design, your system diagram, the size of a team’s vision bubble is restricted by the complexity of the system. Rather than trying to make the vision bubble bigger, what we ultimately need to aim to do is reduce the size of the map to match the size of their vision bubble. Depending on whether you’re choosing orchestration, or choreography, or event driven versus synchronous, and more standardized API driven approach with a coordinator, that will have impacts as to just how much stuff they need to think about at all times.
As mentioned, I think a better way of framing the choice and to boil it down to a nice snapshot snappy view is to think if I have a particularly complicated workflow, something with notably complex error scenarios, that’s probably a good case to say, I’m going to put some orchestration pattern in place. Whereas if I need highly scalable, responsive, and there’s generally not too much in the way of error handling needed, and there’s no directly high complexity, error handling cases in there, choreography is probably a good fit.
Making It Work
We’ve chosen our patterns, what are some of the things that we need to consider about when we’re actually going to go and implement them? If I explode out from my example from before, with the backend for frontend, here, you can see a nice example of actually, you can probably use both effectively within your overall architecture, and you’re going to need to use both. Where we had the orchestration in the backend for frontend, you’ve also got the choreography between the trading system and the data processing pipeline, that’s going to build up a view of the world that’s going to ultimately provide our catalog of events for our systems. Some key things to call out here, though, is that that intermediate infrastructure that I said, this isn’t in here, was a set of different Kafka topics. There’s things here that aren’t obviously owned, don’t really belong to one bounded context or another, you now have something, some infrastructure that sits in the interchange context. There’s a lot of things to consider in this area. How a scheme is going to be evolved. How we’re going to manage ACLs. What do we need to do about certificates if we want to implement mutual TLS, that kind of thing?
If we take this diagram and think about it in a bit more detail, we want to consider first, what’s inside the boxes? How can we make them more effective when we’re building these patterns? I’m going to reframe something that was published as part of a series of blog posts by Confluent, where they were talking about four pillars of event streaming. These four pillars map nicely to thinking about these patterns and how we can make them successful. The systems themselves, obviously need to have some business value. We also need to ensure they’ve got appropriate instrumentation, so we can tell they’re working. The other two things I think are often overlooked, especially when we’re designing event driven systems. That’s probably because they’re a little bit harder to implement. These are the things that will help us to stop processing if we need to, to make it so that we’re not going to compound any problems once they’re detected. We can inspect the state of systems and understand what’s going on before maybe implementing a fix or providing some compensatory actions, and getting us back into a known good state. That operational plane on the far-right hand end, this is the thing where I’ve spoken to ops managers in the past and told them that we were moving to using Kafka for our state management. They started to sweat a little bit because they were like, how on earth am I going to go about rectifying any data inconsistencies or broken data that I would have ordinarily just been able to manage through logging into a database server and correcting it with SQL commands. Lots to think about within the boxes.
Perhaps more importantly, and something that I think doesn’t get enough attention, because of the implied simplicity of diagrams is what’s between the boxes. If we think about what’s in the line that we often see between these services, there’s quite a lot of things that we have to consider. These are just the technical aspects. If we add in all of the social and organizational aspects, you can see that that small thin line is holding an awful lot of weight, and it’s enough to cause us to have some severe overload. We have to think when we’re doing our designs, when we’re implementing our patterns, we have to think how we’re going to handle all this stuff, and everything else that won’t fit on this slide. In particular, some things that’s called out here that I think are really important, especially again, with our event driven approaches, is making sure we’ve taken the time to be explicit in how we’re handling our schemas. Because the worst thing that can happen is that you can get started quite quickly with event driven architectures. Before you know it, you’ve got yourself into a situation, you’ve got many different types of events flowing around, lots of ad hoc schema definitions. When you try and change something, you notice there’s a ripple effect of failure across your systems.
To counteract that, you can be deliberate in how you’re going to manage your compatibility. This guideline from again, Confluent from their schema, and Avro schema compatibility guide for their schema registry is a good way of framing the thinking you need to have. Rather than just saying I’m going to be backwards or forwards compatible, we also need to consider whether we’re going to have transitive compatibility. That means when you publish an update, you’re not just checking against the previous version of a schema, you’re checking against all of the previous versions. That will mean that if you do ever have to replay data, you’re going to make sure that your consumers are going to be able to process that because you’ve guaranteed that all versions of your schemas are going to be compatible.
Equally, we have to think about what format we’re going to send our data in. Because again, depending on our choices, for things like events, whether our event carried state transfer, or notifications, or whatever, these can have significant impacts on the operational capabilities of our system. If we have something that is great for human readability, but doesn’t compress particularly well, we might find that we have issues if we’re sending lots of messages with that data in, or if there are lots of updates that try and read something back from the system. We found out this to our horror on a high traffic day, Boxing Day, it was I think, in 2014, when a document that was used in our system, it was a notification pattern. A notification or an event would come through and this document will get pulled out of Mongo, and it will be used to determine what updates to make. It grew so large that we actually saturated our network, and we were unable to handle any requests from customers. Other things to think about if we do want to reduce the size, and serialization is clearly important, and thinking about balance and tradeoff between compression and efficiency of serialization is going to be important. There’s lots of documentation. You can do experiments, such as the one that Uber have done here, to identify what’s most appropriate for your scenario. The other thing to think about is whether you want to have an informal schema, or whether you want to have something that’s a bit more firm, something that’s going to be documented and version controlled, like in protobufs or Avro.
Instrumentation
One of the pillars of the event streaming systems and overall systems is instrumentation. I would firmly encourage people to ensure they put distributed tracing at the heart of their systems, because this is going to make working with these patterns and these workflows significantly more straightforward. Especially if you want to try and find things in logs, when the logs are coming from lots of different systems and telling you about all sorts of different things that are happening at once. Having a trace ID and correlation in there is such an effective way of helping you to diagnose problems or understand the state of a system. It’s probably the only realistic way you’ve got of understanding how things are working in a truly event driven distributed system.
There’s lots of other tools that you can use. One thing I would call out is that in the more synchronous, RESTful HTTP API space, I think Swagger or OpenAPI as it’s now known, is a fairly well-understood piece of technology that helps people with managing their schemas and testing and all that stuff. There is a similar approach coming about from AsyncAPI to help with event driven systems. I would encourage people to check that out. Equally, while we’re thinking about all these patterns, key and foremost for them is thinking about how our teams are going to work. Definitely, I encourage people to go away and think about how team topologies might apply to help them with thinking about the design of their systems and who’s going to own what.
All That Was Decomposed, Must Be Recomposed Again
The last thing to really touch on here before finishing up, is this idea that actually, when we’ve decomposed our systems into microservices, ultimately, at the end of the day, we’re going to have to recompose them again. That again, is where we might find a use for our orchestration pattern. From this reference architecture from AWS, you can see we’ve got this example here of AppSync, which is a GraphQL layer that’s orchestrating requests to the rest of the system. I’ve sneakily called this in here, because it’s not a coincidence that I’ve used a reference architecture from AWS, because one of the things that I think the bits between boxes, the lines are difficult is because they’re often striped between multiple different technology teams, because teams are aligned at the technology level, not at a domain level. Cloud gives us an opportunity to redress that and to think about it in a different way, and to give whole ownership to our teams. That’s because IAC is the magic glue that helps us bind teams, the infrastructure together for our teams and manage that complexity between the boxes.
Summary
I think that the title was a bit cheeky. Obviously, I don’t want you to have a monolith but we need to think about things working holistically together. A system is the sum of its component parts, so we need to make sure that they all work together well and they provide us the ability to change over time. Whether we have one system or many that work together, the same rules apply, we need to be deliberate in our design. To that mind, I want to encourage you, please do design for evolution, consider changing the reasons for it upfront. Be thorough and deliberate when you’re choosing the patterns for implementing your workflows, because how you implement things is the way that you will make things harder for yourselves in the future. The one thing that I hope everybody will go away and remember is that you have to remember the bits between the boxes, and that they all need people too.
See more presentations with transcripts