Month: June 2023
NoSQL Database Market: A Systematic Review of Modern Threats, Trends and Emerging Challenges
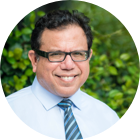
MMS • RSS
Posted on nosqlgooglealerts. Visit nosqlgooglealerts
What analysis has been conducted in the NoSQL Database market report to assess the implications of the COVID-19 impact, ongoing Russia-Ukraine war?
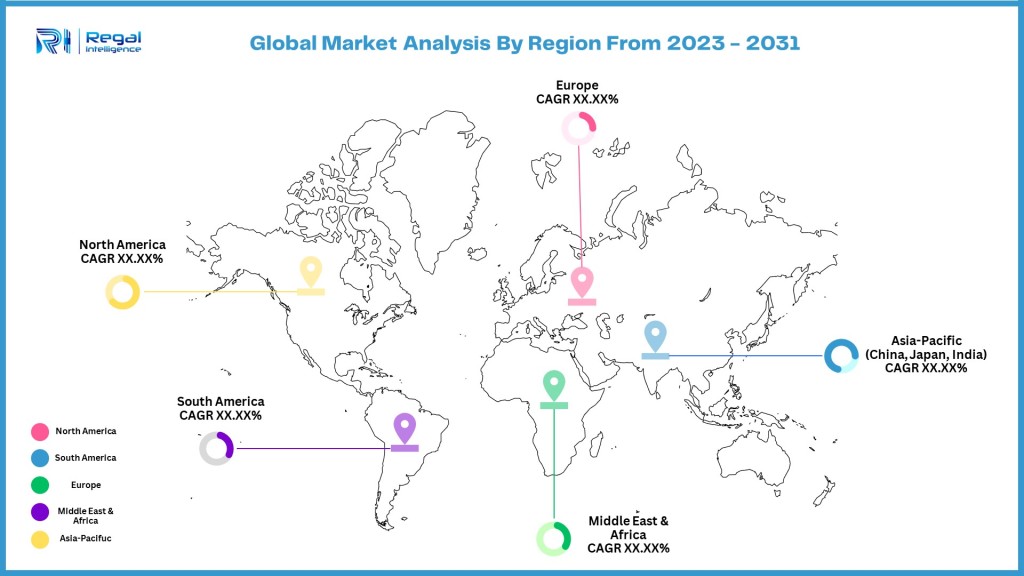
This report studies the NoSQL Database market, covering market size for segment by type (Key-Value Based Database, Document Based Database, etc.), by application (BFSI, Healthcare, etc.), by sales channel (Direct Channel, Distribution Channel), by player (Aerospike, Amazon Web Services, Apache Cassandra, Basho Technologies, Cisco Systems, etc.) and by region (North America, Europe, Asia-Pacific, South America and Middle East & Africa).
The NoSQL Database Market report thoroughly studies and analyzes the impact of the COVID-19 pandemic and the geopolitical tensions on the world economy and trade. Additionally, it assesses the implications of the Russia-Ukraine war on market dynamics. The report offers a comprehensive outlook on the NoSQL Database market industry, encompassing overview, challenges, opportunities, restraints, and future trends. It also includes a forecast for future trends and growth prospects in the industry for the year 2023. The report further analyzes key metrics such as CAGR, market share, market revenue, demand and supply, consumption patterns, manufacturing capabilities of industry leaders, regional analysis, consumer behavior, and competitive landscapes. These insights enable businesses to identify market potentials and facilitate informed decision-making.
Prominent players in the industry:
- Aerospike
- Amazon Web Services
- Apache Cassandra
- Basho Technologies
- Cisco Systems
- CloudDB
- Couchbase Server
- DynamoDB
- Hypertable
- IBM
- MarkLogic
- Microsoft
- MongoDB
- MySQL
- Neo Technology
- Objectivity
- Oracle
- PostgreSQL
Get a Sample of the Report @ https://www.regalintelligence.com/request-sample/242675
NoSQL Database market research report provides in-depth information and insights into the market for the forecast period of 2023-2031. Major players in the NoSQL Database market and their competitive landscapes are analyzed, as the players drive the market and get affected on the frontline. The report addresses key challenges faced by the market and provides effective solutions for its growth. Additionally, it examines the supply chain channels encompassing raw materials, distribution, and production operations of major market players.
How the market segmentation analysis benefits in terms of understanding market growth over the forecasted time frame?
The report contemplates different regions of the global NoSQL Database market based on end consumer type, item type, application and geographical analysis. The analysts altogether study these fragments of the market to give clear bits of information on various segments of the market. Various touchpoints like overall market share, revenue, regional development, cost of production, and income and cost evaluation, are considered while assessing the market segments. This segmentation analysis encourages users to comprehend the market development over the forecasted time frame in the context of segments and make the best knowledgeable decisions as needs be.
NoSQL Database Market Major Applications:
- BFSI
- Healthcare
- Telecom
- Government
- Retail
NoSQL Database Market Segment by Product Types:
- Key-Value Based Database
- Document Based Database
- Column Based Database
- Graph Based Database
For Any Query, Contact Us: https://www.regalintelligence.com/enquiry/242675
What are the secondary sources utilized and how were industry experts, such as CEOs, VPs, directors, and executives, involved in the research methodology?
The research methodology used to estimate and forecast this market begins by capturing the revenues of the key players and their shares in the market. Various secondary sources such as press releases, annual reports, non-profit organizations, industry associations, governmental agencies and customs data, have been used to identify and collect information useful for this extensive commercial study of the market. Calculations based on this led to the overall market size. After arriving at the overall market size, the total market has been split into several segments and subsegments, which have then been verified through primary research by conducting extensive interviews with industry experts such as CEOs, VPs, directors, and executives. The data triangulation and market breakdown procedures have been employed to complete the overall market engineering process and arrive at the exact statistics for all segments and subsegments.
Primary Objectives of NoSQL Database Market Report:
- To conduct a comprehensive analysis of the market landscape, including current trends, growth prospects, and future forecasts.
- To identify potential opportunities and assess the associated challenges, obstacles, and threats in the market.
- To develop strategic business plans that are aligned with industry and economic shifts, ensuring adaptability and long-term success.
- To evaluate the competitive landscape and devise strategies to gain maximum advantage over rivals.
- To provide actionable insights and data-driven recommendations for making informed business decisions.
What are the major areas that the report focuses upon?
- What will be the NoSQL Database market size in 2030 and growth rate?
- What are the key factors driving the market at global, regional and country level?
- Who are the key vendors in the NoSQL Database market and their market strategies?
- What are the restraints and challenges to NoSQL Database market growth?
- What are the NoSQL Database market opportunities and threats faced by the vendors in the global NoSQL Database market?
- What are some of the competing products in this NoSQL Database and how big of a threat do they pose for loss of market share by product substitution?
- What M&A activity has taken place in the past 5 years?
Get Full Report: https://www.regalintelligence.com/buyNow/242675
To summarize, the NoSQL Database market research report includes market analysis, strategic planning, and providing valuable insights for decision-making. Moreover, the report additionally considers a lot of critical factors like production and utilization patterns, supply and demand gap checks, market development factors, future patterns, trends, industry outlook, Cost and revenue study, and so on. This report likewise gives investigative bits of information by using tools like, SWOT, PESTEL and Porter’s Five Forces, investment return report is additionally included helping the readers and financial specialists to get appropriate assessment in regards to potential market development, growth factors and rate of profitability analysis.
For More Related Reports Click Here :
Human Machine Interface (HMI) Software Market
3-Phase Vacuum Circuit Breaker Market
1-Ethynyl-3,5-Dimethoxybenzene Market
About Us:
Regal Intelligence aims to change the dynamics of market research backed by quality data. Our analysts validate data with exclusive qualitative and analytics driven intelligence. We meticulously plan our research process and execute in order to explore the potential market for getting insightful details. Our prime focus is to provide reliable data based on public surveys using data analytics techniques. If you have come here, you might be interested in highly reliable data driven market insights for your product/service, reach us here 24/7.
Mention your Queries here to Get a Call from Our Industry Expert @ sales@regalintelligence.com
Contact Us:
Regal Intelligence: www.regalintelligence.com
Phone no: +1 231 930 2779 (U.S.)
LinkedIn: https://www.linkedin.com/company/regal-intelligence
Twitter: https://twitter.com/regalinsights
Pinterest: https://www.pinterest.com/regalintelligence/
2 Artificial Intelligence (AI) Growth Stocks That Are Just Getting Started | The Motley Fool
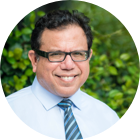
MMS • RSS
Posted on mongodb google news. Visit mongodb google news
Looking back, 2023 may well mark a turning point for artificial intelligence (AI). The latest developments in large language models have given birth to next-generation technology, including OpenAI’s ChatGPT — but that’s likely just the beginning. The capabilities of these AI systems have sparked imaginations, and the brightest minds in business are looking for ways to employ this groundbreaking technology to automate repetitive tasks, improve customer service, and create new opportunities.
The ongoing AI revolution has investors searching high and low to profit from the massive potential afforded by this state-of-the-art technology. While estimates vary wildly, one of the most bullish forecasts comes from Cathie Wood’s Ark Investment Management, which suggests that the global AI software market will grow at 42% annually, topping $14 trillion by 2030. Even if this estimate turns out to be overly bullish, it helps show that the market for AI-enabled software could grow at a blistering pace for years to come.
Let’s look at two high-flying stocks that are well positioned to benefit from the AI revolution.
Image source: Getty Images.
1. HubSpot
HubSpot (HUBS -0.14%) made its fortune by disrupting traditional advertising. It pioneered the concept of inbound marketing, which builds relationships with potential customers with compelling content offered online, via social media, and in blog posts.
The company has since expanded its empire to encompass the entire spectrum of customer relationship management (CRM), with a vast ecosystem of interconnected offerings. These include solutions for marketing, sales, service, content management, and operations teams, with tools that help to manage data, commerce, reporting, automation, content, messaging, and payments.
CEO Yamini Rangan laid out the case for what the latest advances in AI means to HubSpot and its customers in the company’s first-quarter earnings call, saying, “HubSpot is [a] powerful, yet easy to use … all-in-one CRM platform powered by AI.” He noted that the company is integrating generative AI across its offerings, going on to say that the company is differentiated by its “unique data and broad distribution.”
“HubSpot CRM data is unified and cohesive, making it easier for AI to ingest and drive relevance,” Rangan said. Finally, the chief executive points out that HubSpot customers “don’t have to become AI experts to reap the transformational benefits” available on its platform.
HubSpot’s first-quarter results provide a glimpse at its potential. Even in the middle of a challenging economy, revenue grew 27% year over year, while adjusted earnings per share (EPS) of $1.25 more than doubled. The results were fueled by solid customer gains, which grew 23%. Perhaps more importantly is the expanding relationships with existing customers, as 45% of the company’s annual recurring revenue is generated by clients using three or more hubs.
The stock is currently selling for 10 times next year’s sales, so it isn’t cheap in terms of traditional valuation measures. That said, in less than nine years, HubSpot stock has gained more than 1,600% — and is still well off its peak. Given its history of strong growth, is valuation seems much more reasonable.
2. MongoDB
MongoDB (MDB -1.43%) made a name for itself by disrupting the traditional database paradigm. While most databases are limited to rows and columns, MongoDB’s Atlas cloud-native platform can handle this and much more, including video and audio files, social media posts, and even entire documents, providing users with much more robust database solutions. This provides developers with a much greater degree of flexibility to create software applications.
When announcing the company’s first quarter of fiscal 2024 results, CEO Dev Ittycheria explained what the shift to AI means to MongoDB, saying: “We believe the recent breakthroughs in AI represent the next frontier of software development. The move to embed AI in applications requires a broad and sophisticated set of capabilities, while enabling developers to move even faster to create a competitive advantage.” He went on to say the company was “well positioned to benefit from the next wave of AI applications in the years to come.”
MongoDB’s results from the first quarter of fiscal 2024 help tell the tale. Revenue of $368 million grew 29% year over year — even in the face of economic headwinds — while its adjusted EPS of $0.56 soared 180%. Fueling the results was the most net new customer additions in more than two years. The results were led by Atlas, the company’s fully managed, database-as-a-platform service platform, which grew 40% year over year and now makes up 65% of MongoDB’s total revenue.
The stock might seem expensive at 14 times next year’s sales, but consider this: In just over five years, MongoDB stock has gained more than 1,000% — even after its downturn-induced drubbing — so its valuation shouldn’t be viewed in a vacuum.
As new customers seek out platforms offering the greatest capacity to build and run new AI applications, MongoDB’s Atlas is a top choice.
Article originally posted on mongodb google news. Visit mongodb google news
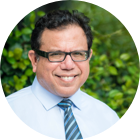
MMS • Michael Redlich
Article originally posted on InfoQ. Visit InfoQ
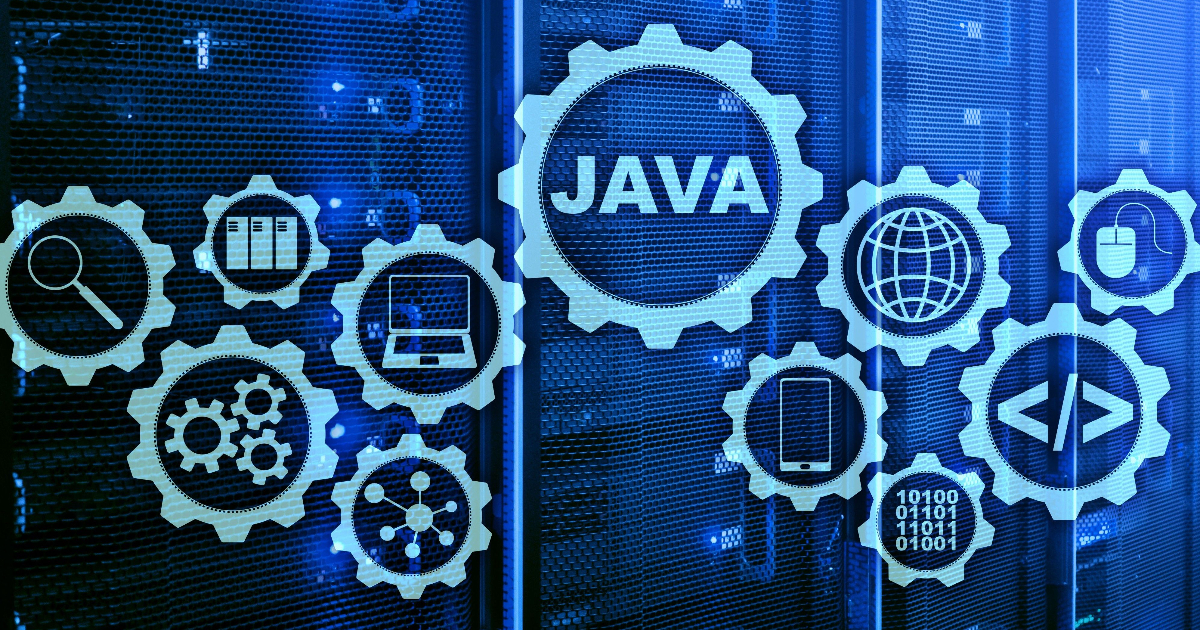
Day Two of the 9th annual QCon New York conference was held on June 14th, 2023 at the New York Marriott at the Brooklyn Bridge in Brooklyn, New York. This three-day event is organized by C4Media, a software media company focused on unbiased content and information in the enterprise development community and creators of InfoQ and QCon. It included a keynote address by Alicia Dwyer Cianciolo and presentations from these four tracks:
- Designing Modern Reliable Architectures
- Hosted by Silvia Esparrachiari, Software Engineer at Google.
- Provides a diverse collection of reliability strategies covering finance applications, gaming platforms and other real world segments of the tech industry.
- MLOps – Production & Delivery for ML Platforms
- Hosted by Bozhao (Bo) Yu, Founder at BentoML.ai.
- Explores the latest trends, best practices, and case studies in MLOps, and discusses how they can be applied to improve the reliability, scalability, and efficiency of machine learning systems.
- Next Gen Fintech: Performance, Complexity & Privacy
- Hosted by Neha Sardana, Vice President and Senior Developer at Morgan Stanley.
- Provides solutions to the challenges of data breaches, privacy, security, and performance, and stays on top of the latest trends and best practices in Fintech.
- Optimizing Teams for Fast Flow – Surviving in the Post-Agile Aftermath
- Hosted by Katharine Chajka, Senior Product Manager at Planview Flow Methodology.
- Focuses on understanding how to identify the specific issues slowing down flow and finding changes that resolve those impediments to make the most of the time and money spent on change.
There was also one sponsored solutions track.
Danny Latimer, Content Product Manager at C4Media, kicked off the day two activities by welcoming the attendees and introduced Daniel Bryant, InfoQ News Manager, who discussed InfoQ news activities and the InfoQ core values: information robin hoods; best, not (necessarily) first; facilitators, not leaders; and content that can trusted. Pia von Beren, Project Manager & Diversity Lead at C4Media, discussed the QCon Alumni Program and the benefits of having attended multiple QCon conferences. The aforementioned track leads for Day Two introduced themselves and described the presentations in their respective tracks.
Keynote Address
Alicia Dwyer Cianciolo, senior technical lead for Advanced Entry, Descent and Landing Vehicle Technology Development at the NASA Langley Research Center, presented a keynote entitled, NASA’S Return to the Moon: Managing Complexity in the Artemis Program. Cianciolo started her presentation with the famous quote from John F. Kennedy’s speech at Rice University in 1962: “We choose to go to the moon in this decade and do the other things, not because they are easy, but because they are hard.” With than in mind, she introduced the Artemis program, considered the “sister” to the Apollo program, as a collection of projects, namely:
Artemis = Space Launch System + Orion Spacecraft + Human Landing System (HLS) + Extravehicular Activity and Human Surface Mobility Program (EHP) + Gateway
Cianciolo currently works on the Human Landing System. Artemis and its component projects were designed as a collaboration for space missions. After introducing each of these projects, she provided background and orbit information on previous and upcoming Artemis launches. Artemis I, launched on November 16, 2022 and splashed down on December 11, 2022 featured the Space Launch System and Orion projects. Artemis II, scheduled to launch at the end of 2024, will feature the Space Launch System and Orion projects and include a flight crew: Reid Wiseman (commander), Victor Glover (pilot), Christina Hammock Koch (Mission Specialist) and Jeremy Hansen (Mission Specialist). The plan for Artemis III is to land on the moon and will feature the Space Launch System, Orion, HLS and EHP projects and include another flight crew that is still to be determined. She described the complex of operations related to the HLS that includes mission segments, contracts, landing requirements and gateway orbit. Apollo 11 through Apollo 17 landed on or near the moon’s equator. The landing plan for Artemis III is to land within 6° latitude and less than 10° surface slopes on the moon’s south pole due to the rough terrain. Another challenge is the amount of daylight that often changes. The crew will need a six-day window of daylight and to be in constant communication with Earth. “What could go wrong?” Cianciolo asked. The hardest part of going to the moon is talking to people, Cianciolo said, as she recalled her experience in which it took six months to resolve this seemingly simple issue related to sharing a bathroom space. The plan for Artemis IV is to land on the International Habitation Module and will feature the Space Launch System, Orion, HLS, EHP and Gateway projects and include a flight crew to be determined.
Highlighted Presentations
Maximizing Performance and Efficiency in Financial Trading Systems through Vertical Scalability and Effective Testing by Peter Lawrey, CEO at Chronical Software. Lawrey kicked off his presentation by discussing how allocating objects may have an overhead of 80x than that of collecting them. He maintained that the loss of objects can be allocated, but recommends against this strategy that has been a practice. As the legendary Grace Hopper once said: “The most dangerous phrase in the English language is: “‘We’ve always done it that way.'” He provided various analyses and benchmarks on why allocations don’t scale well. Allocations can spend approximately 0.3% of the time in the garbage collector. The concept of accidental complexity is complexity that is not inherent in a problem, but rather in a solution that can be removed or reduced with a better design or technology choice. Lawrey provided many examples and analyses of accidental complexity that included memory usage analysis from Chronical Queue where most of the allocation activity was from the JDK Flight Recorder. In many applications, especially in the financial industry, selecting a “source of truth” can significantly impact the latency and complexity of the application. The concept of durability guarantees identifies critical paths for performance, but are often considered the largest bottlenecks. Examples include: a database; durable messaging that is guaranteed to be on disk; redundant messaging; and persisted messaging that will eventually be on disk. Lawrey introduced Little’s Law, a founding principle in queueing theory, as L = λ, such that:
- L = average number of items in a system
- λ = average arrival rate = exit rate = throughput
- W = average wait time in a system for an item (duration inside)
Little’s Law is applied in many aspects of system design and performance enhancement. The higher the latency, the higher the inherent parallelism required to achieve a desired throughput. On the opposite end of that spectrum, the lower the latency, the inherent required parallelism is minimized. Traditional object allocation in Java can impede performance, especially in high throughput scenarios, creating a bottleneck that hinders vertical scalability. By minimizing accidental complexity and using an event-driven architecture, vertical scalability can be achieved.
Performance and Scale – Domain-Oriented Objects vs Tabular Data Structures by Donald Raab, Managing Director and Distinguished Engineer at BNY Mellon, and Rustam Mehmandarov, Chief Engineer at Computas AS. Raab and Mehmandarov started their presentation with a retrospective into the problems with in-memory Java architectures using both 32-bit and 64-bit memory circa 2004. In the 32-bit world, it was challenging for developers to place, say, 6GB of data, into 4GB of memory. The solution was to build their own “small size” Java collections. The emergence of 64-bit provided some relief, but total heap size became an issue. Compressed Ordinary Object Pointers (OOPS), available with the release of Java 6 in late 2006, allowed developers to create 32-bit references (4 bytes) in 64-bit heaps. Solutions in this case include; building their own memory-efficient mutable Set
, Map
and List
data structures; and building primitive collections for the List
, Set
, Stack
, Bag
and Map
data structures. Raab and Mehmandarov then described the challenges developers face today where, for instance, large CSV data needs to be processed in-memory. They asked the questions: “How can that efficiently be accomplished in Java?,” “How can memory efficiency of data structures be measured?,” “What decisions affect memory efficiency?,” and “Which approach is better: row vs. columns?” To measure the cost of memory in Java, Raab and Mehmandarov introduced the Java Object Layout (JOL), a small toolbox to analyze object layout schemes in JVMs, and how to use it within an application. Using a large CSV data set as an example, Raab and Mehmandarov provided a comprehensive look into the various memory considerations: boxed vs. primitive types; mutable vs. immutable data; data pooling; and row-based vs. column-based structures. They also explored three libraries: Java Streams, introduced in Java 8; Eclipse Collections, invented by Raab; and DataFrame-EC, a tabular data structure based on the Eclipse Collections framework. Three Java projects: Amber, Valhalla and Lilliput, are working to improve productivity, value objects and user-defined primitives, and reduce the object header to 64 bits, respectively.
A Bicycle for the (AI) Mind: GPT-4 + Tools by Sherwin Wu, Member of the Technical Staff at OpenAI, and Atty Eleti, Software Engineer at OpenAI. In 1973, efficiencies in cycling started to emerge which were compared to a condor bird. Wu and Eleti coined the phrase “A bicycle for the mind” and used this as a metaphor for Steve Jobs and the creation of Apple. In 2023, the emergence of ChatGPT has evolved the phrase to “A bicycle for the AI mind.” They discussed large language models (LLMs) and their limitations followed by an introduction to new function calling capability that improves their gpt-4
and gpt-3.5-turbo
applications. Wu and Eleti provided numerous demos for: converting natural language into queries; calling external APIs and multiple functions; and combining advanced reasoning with daily tasks. They maintained that technology is still in its infancy and they are excited to see how this technology will evolve in the future.
Implementing OSSF Scorecards Across an Organization by Chris Swan, Engineer at atsign.
Swan introduced the Open Source Security Foundation (OSSF or OpenSSF), a “cross-industry organization that brings together the industry’s most important open source security initiatives and the individuals and companies that support them.” The OpenSSF Scorecard project, just one of the projects under OpenSSF, helps open source maintainers improve their security best practices and to help open source consumers judge whether their dependencies are safe. A number of important software security heuristics are measured against an open source software project and assign each heuristic with a score of 0-10. A badge is generated containing the heuristic and score that may be placed on the GitHub repository. This provides a visual representation that the maintainers of the open source repository care about security, and a feeling of safety and security. Swan explored the five holistic security practices: code vulnerabilities, maintenance, continuous testing, source risk assessment and build risk assessment. He encouraged developers who are new to OpenSSF to use Allstar, another OpenSSF project, as a starting point for assessing security for their own open source projects. Swan provided a comprehensive introduction on how to get started by exploring tools such as: GitHub Insights, a tool that can guide developers to create a good quality open source repository; and Terraform, an Infrastructure as a Service (IaaS) resource that provides scripts to improve a GitHub repository. The process also includes a very long questionnaire in which developers should budget at least one hour. The 80:20 rule in OpenSSF states that 20% of effort is required to obtain 80% of the Scorecard score. However, Swan commented that it gets more difficult from there and that it is really hard to achieve high scores.
Summary
In summary, day two featured a total of 27 presentations with topics such as: reliable architectures, machine learning, financial technology (fintech) and optimizing engineering teams.
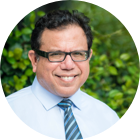
MMS • RSS
Posted on mongodb google news. Visit mongodb google news
Voya Investment Management LLC recently announced a decrease in its position within MongoDB, Inc. by 17.7% during the fourth quarter of the fiscal year. According to the company’s most recent Form 13F filing with the U.S. Securities and Exchange Commission (SEC), Voya Investment Management LLC owned approximately 1.83% of MongoDB with 1,265,042 shares valued at $249,011,000 as of its last SEC filing. This comes after a successful second quarter for MongoDB that saw positive results in earnings per share and revenue growth.
MongoDB is a database platform provider that offers various solutions to enterprise customers worldwide such as Atlas, Community Server and Enterprise Advanced, which can be run on-premise or in the cloud. The company has been making headway in its quest for innovation by providing free-to-download versions of their databases with all features included, enabling developers to utilize MongoDB more conveniently.
The second quarter results showed a promising future for MongoDB given that they beat the consensus estimate of earnings per share significantly. The firm made an impressive $0.56 per share compared to anticipated $0.18 cents which translated into revenue of $368 million compared to project estimates of $347 million.
Moreover, research analysts are forecasting negative earnings per share for the current fiscal year from MongoDB indicating that losses will be incurred as opposed to profits – it remains to be seen whether these projections are accurate but it cannot be denied that this may have played a part in Voya’s recent decision.
In conclusion, Voya’s latest Form 13F filing and increased interest within the company has brought us closer to understanding how institutional investors are viewing tech stocks like MongoDB and what it could mean for potential investors in similar enterprises moving forward into Q3/Q4 of this year.
Institutional Investors and Analysts Show Strong Interest in MongoDB Inc.
Institutional investors and hedge funds have either added to or reduced their stakes in MongoDB, Inc, according to recent reports. Notable players among them include Vanguard Group Inc with a 1% increase in holdings, 1832 Asset Management LP who increased their holdings by a whopping 3283771%, Franklin Resources Inc who raised theirs by 6.4%, State Street Corp with a 1.8% increase, and Geode Capital Management with a 4.5% rise. As of now, institutional investors hold almost 85% of the company’s stock.
MDB stock opened at $385.40 on Friday and has a market capitalization of $26.99 billion. The company offers general-purpose database solutions such as MongoDB Atlas – a multifaceted database solution intended for use on the cloud; MongoDB Enterprise Advanced – a robust database server intended for enterprise-grade customers; and Community Server – an entry-level free-to-download version providing users with basic MongoDB functionalities.
On the research front, the Goldman Sachs Group raised its price target on MDB from $280 to $420 while Needham & Company LLC upped theirs from $250 to $430. Tigress Financial reaffirmed its buy rating on MDB shares while analyst firm KeyCorp analyzes the stock as ‘overweight.’ Additionally, Oppenheimer rated MDB highly enough to increase their price target from $270 to $430. Every analyst concludes that this remains an exemplary choice for investment.
There has been insider trading activity, with CEO Dev Ittycheria selling over $11 million worth of stocks recently while CAO Thomas Bull sold shares valued at over $138 thousand dollars.
To sum up –
MongoDB is currently offering cutting-edge general-purpose databases worldwide while securing vast participation amongst institutional investors around the world due to the strategic investments made in spreading awareness about invaluable offerings like MongoDB Atlas and Community Server among others. Moreover, analysts predict substantial growth opportunities for this company, rendering MongoDB shares as an excellent investment opportunity.
Article originally posted on mongodb google news. Visit mongodb google news
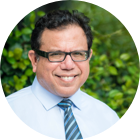
MMS • RSS
Posted on mongodb google news. Visit mongodb google news
Kovack Advisors Inc. trimmed its position in MongoDB, Inc. (NASDAQ:MDB – Get Rating) by 26.3% during the 4th quarter, according to its most recent disclosure with the Securities and Exchange Commission. The fund owned 1,576 shares of the company’s stock after selling 563 shares during the quarter. Kovack Advisors Inc.’s holdings in MongoDB were worth $310,000 at the end of the most recent reporting period.
Several other large investors have also recently made changes to their positions in MDB. Bessemer Group Inc. bought a new stake in shares of MongoDB in the fourth quarter worth about $29,000. BI Asset Management Fondsmaeglerselskab A S bought a new stake in shares of MongoDB in the fourth quarter worth about $30,000. Lindbrook Capital LLC increased its holdings in shares of MongoDB by 350.0% in the fourth quarter. Lindbrook Capital LLC now owns 171 shares of the company’s stock worth $34,000 after purchasing an additional 133 shares during the last quarter. Y.D. More Investments Ltd bought a new stake in shares of MongoDB in the fourth quarter worth about $36,000. Finally, CI Investments Inc. increased its holdings in shares of MongoDB by 126.8% in the fourth quarter. CI Investments Inc. now owns 186 shares of the company’s stock worth $37,000 after purchasing an additional 104 shares during the last quarter. 84.86% of the stock is owned by institutional investors.
Analysts Set New Price Targets
Several research firms recently commented on MDB. Tigress Financial reiterated a “buy” rating and issued a $365.00 price target on shares of MongoDB in a research report on Thursday, April 20th. Royal Bank of Canada lifted their price target on MongoDB from $235.00 to $400.00 in a research report on Friday, June 2nd. The Goldman Sachs Group lifted their price target on MongoDB from $280.00 to $420.00 in a research report on Friday, June 2nd. JMP Securities lifted their price target on MongoDB from $245.00 to $370.00 in a research report on Friday, June 2nd. Finally, Wedbush dropped their price target on MongoDB from $240.00 to $230.00 in a research report on Thursday, March 9th. One analyst has rated the stock with a sell rating, two have issued a hold rating and twenty-one have issued a buy rating to the stock. Based on data from MarketBeat, the stock presently has a consensus rating of “Moderate Buy” and an average target price of $328.35.
MongoDB Stock Performance
MongoDB stock opened at $379.90 on Friday. The company has a 50 day moving average price of $279.41 and a 200-day moving average price of $230.20. The company has a debt-to-equity ratio of 1.44, a quick ratio of 4.19 and a current ratio of 4.19. MongoDB, Inc. has a fifty-two week low of $135.15 and a fifty-two week high of $398.89. The company has a market capitalization of $26.61 billion, a PE ratio of -81.35 and a beta of 1.04.
MongoDB (NASDAQ:MDB – Get Rating) last posted its quarterly earnings results on Thursday, June 1st. The company reported $0.56 earnings per share for the quarter, beating the consensus estimate of $0.18 by $0.38. MongoDB had a negative net margin of 23.58% and a negative return on equity of 43.25%. The firm had revenue of $368.28 million during the quarter, compared to the consensus estimate of $347.77 million. During the same quarter in the previous year, the firm posted ($1.15) earnings per share. The company’s revenue was up 29.0% on a year-over-year basis. As a group, equities analysts forecast that MongoDB, Inc. will post -2.85 earnings per share for the current year.
Insider Activity
In related news, CAO Thomas Bull sold 605 shares of the business’s stock in a transaction that occurred on Monday, April 3rd. The shares were sold at an average price of $228.34, for a total value of $138,145.70. Following the completion of the transaction, the chief accounting officer now owns 17,706 shares of the company’s stock, valued at $4,042,988.04. The sale was disclosed in a legal filing with the Securities & Exchange Commission, which is accessible through this link. In related news, CRO Cedric Pech sold 720 shares of the business’s stock in a transaction that occurred on Monday, April 3rd. The shares were sold at an average price of $228.33, for a total value of $164,397.60. Following the completion of the transaction, the executive now owns 53,050 shares of the company’s stock, valued at $12,112,906.50. The sale was disclosed in a legal filing with the Securities & Exchange Commission, which is accessible through this link. Also, CAO Thomas Bull sold 605 shares of the business’s stock in a transaction that occurred on Monday, April 3rd. The stock was sold at an average price of $228.34, for a total transaction of $138,145.70. Following the completion of the transaction, the chief accounting officer now directly owns 17,706 shares of the company’s stock, valued at approximately $4,042,988.04. The disclosure for this sale can be found here. Over the last quarter, insiders sold 106,682 shares of company stock valued at $26,516,196. Corporate insiders own 4.80% of the company’s stock.
MongoDB Profile
MongoDB, Inc provides general purpose database platform worldwide. The company offers MongoDB Atlas, a hosted multi-cloud database-as-a-service solution; MongoDB Enterprise Advanced, a commercial database server for enterprise customers to run in the cloud, on-premise, or in a hybrid environment; and Community Server, a free-to-download version of its database, which includes the functionality that developers need to get started with MongoDB.
See Also
Receive News & Ratings for MongoDB Daily – Enter your email address below to receive a concise daily summary of the latest news and analysts’ ratings for MongoDB and related companies with MarketBeat.com’s FREE daily email newsletter.
Article originally posted on mongodb google news. Visit mongodb google news
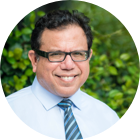
MMS • RSS
Posted on mongodb google news. Visit mongodb google news
Kovack Advisors Inc. reduced its stake in MongoDB, Inc. (NASDAQ:MDB – Get Rating) by 26.3% during the fourth quarter, according to the company in its most recent 13F filing with the SEC. The firm owned 1,576 shares of the company’s stock after selling 563 shares during the quarter. Kovack Advisors Inc.’s holdings in MongoDB were worth $310,000 at the end of the most recent quarter.
A number of other institutional investors also recently bought and sold shares of the stock. 1832 Asset Management L.P. raised its holdings in MongoDB by 3,283,771.0% during the fourth quarter. 1832 Asset Management L.P. now owns 1,018,000 shares of the company’s stock valued at $200,383,000 after acquiring an additional 1,017,969 shares during the period. Renaissance Technologies LLC raised its holdings in MongoDB by 493.2% during the fourth quarter. Renaissance Technologies LLC now owns 918,200 shares of the company’s stock valued at $180,738,000 after acquiring an additional 763,400 shares during the period. Norges Bank bought a new stake in MongoDB during the fourth quarter valued at about $147,735,000. William Blair Investment Management LLC raised its holdings in MongoDB by 2,354.2% during the fourth quarter. William Blair Investment Management LLC now owns 387,366 shares of the company’s stock valued at $76,249,000 after acquiring an additional 371,582 shares during the period. Finally, Marshall Wace LLP raised its holdings in MongoDB by 87.4% during the third quarter. Marshall Wace LLP now owns 696,998 shares of the company’s stock valued at $138,396,000 after acquiring an additional 325,136 shares during the period. 84.86% of the stock is owned by institutional investors.
Analyst Ratings Changes
A number of equities research analysts have commented on MDB shares. William Blair reissued an “outperform” rating on shares of MongoDB in a report on Friday, June 2nd. Barclays lifted their target price on MongoDB from $280.00 to $374.00 in a report on Friday, June 2nd. Credit Suisse Group reduced their target price on MongoDB from $305.00 to $250.00 and set an “outperform” rating on the stock in a report on Friday, March 10th. JMP Securities lifted their target price on MongoDB from $245.00 to $370.00 in a report on Friday, June 2nd. Finally, Wedbush reduced their target price on MongoDB from $240.00 to $230.00 in a report on Thursday, March 9th. One analyst has rated the stock with a sell rating, two have issued a hold rating and twenty-one have assigned a buy rating to the company. According to data from MarketBeat, the company currently has a consensus rating of “Moderate Buy” and a consensus price target of $328.35.
Insider Activity
In other news, CAO Thomas Bull sold 605 shares of MongoDB stock in a transaction that occurred on Monday, April 3rd. The shares were sold at an average price of $228.34, for a total transaction of $138,145.70. Following the completion of the sale, the chief accounting officer now owns 17,706 shares of the company’s stock, valued at $4,042,988.04. The transaction was disclosed in a legal filing with the Securities & Exchange Commission, which is accessible through this hyperlink. In other news, CRO Cedric Pech sold 720 shares of MongoDB stock in a transaction that occurred on Monday, April 3rd. The shares were sold at an average price of $228.33, for a total transaction of $164,397.60. Following the completion of the sale, the executive now owns 53,050 shares of the company’s stock, valued at $12,112,906.50. The transaction was disclosed in a legal filing with the Securities & Exchange Commission, which is accessible through this hyperlink. Also, CAO Thomas Bull sold 605 shares of the business’s stock in a transaction that occurred on Monday, April 3rd. The stock was sold at an average price of $228.34, for a total transaction of $138,145.70. Following the completion of the transaction, the chief accounting officer now directly owns 17,706 shares of the company’s stock, valued at approximately $4,042,988.04. The disclosure for this sale can be found here. Over the last quarter, insiders sold 106,682 shares of company stock valued at $26,516,196. Corporate insiders own 4.80% of the company’s stock.
MongoDB Stock Down 1.4 %
Shares of NASDAQ:MDB opened at $379.90 on Friday. MongoDB, Inc. has a 1 year low of $135.15 and a 1 year high of $398.89. The business has a 50 day simple moving average of $279.41 and a two-hundred day simple moving average of $230.20. The firm has a market capitalization of $26.61 billion, a PE ratio of -81.35 and a beta of 1.04. The company has a debt-to-equity ratio of 1.44, a current ratio of 4.19 and a quick ratio of 4.19.
MongoDB (NASDAQ:MDB – Get Rating) last announced its earnings results on Thursday, June 1st. The company reported $0.56 earnings per share (EPS) for the quarter, beating the consensus estimate of $0.18 by $0.38. MongoDB had a negative return on equity of 43.25% and a negative net margin of 23.58%. The business had revenue of $368.28 million during the quarter, compared to analyst estimates of $347.77 million. During the same quarter in the previous year, the business earned ($1.15) earnings per share. The company’s revenue for the quarter was up 29.0% compared to the same quarter last year. On average, equities analysts predict that MongoDB, Inc. will post -2.85 EPS for the current year.
MongoDB Profile
MongoDB, Inc provides general purpose database platform worldwide. The company offers MongoDB Atlas, a hosted multi-cloud database-as-a-service solution; MongoDB Enterprise Advanced, a commercial database server for enterprise customers to run in the cloud, on-premise, or in a hybrid environment; and Community Server, a free-to-download version of its database, which includes the functionality that developers need to get started with MongoDB.
See Also
Receive News & Ratings for MongoDB Daily – Enter your email address below to receive a concise daily summary of the latest news and analysts’ ratings for MongoDB and related companies with MarketBeat.com’s FREE daily email newsletter.
Article originally posted on mongodb google news. Visit mongodb google news
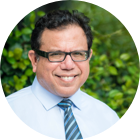
MMS • Renato Losio
Article originally posted on InfoQ. Visit InfoQ
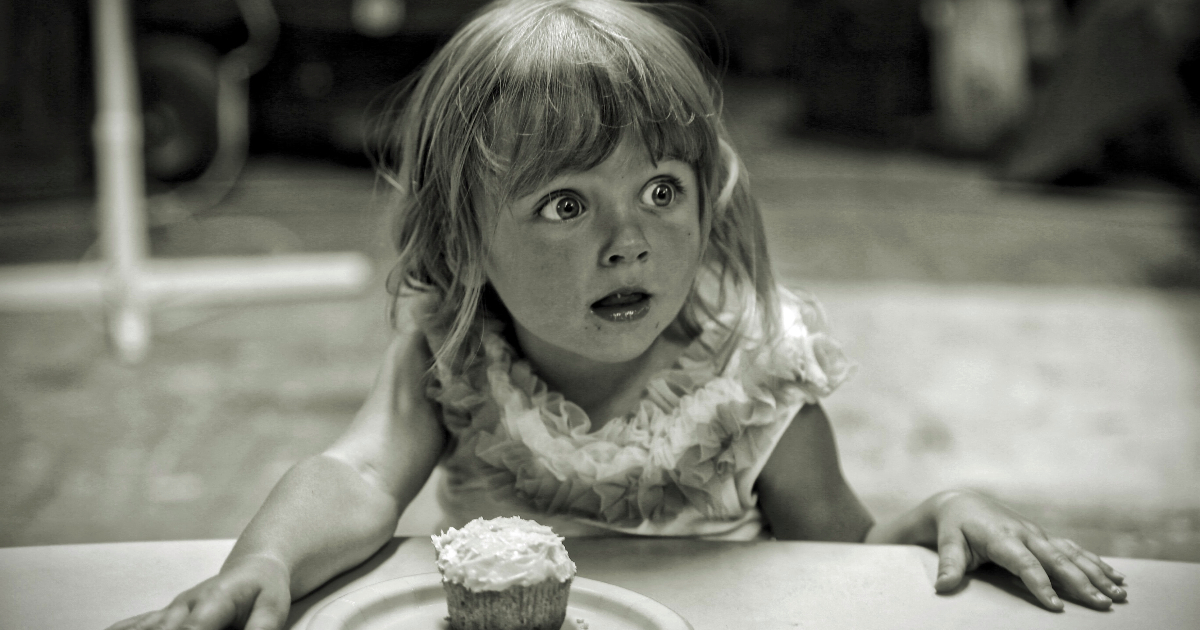
Microsoft recently announced the general availability of Azure Linux container host for AKS. Available on GitHub under the CBL-Mariner project codename, the lightweight Linux distribution includes only the packages needed to run workloads on a cloud environment.
The Azure Linux container host for AKS is an open-source Linux distribution available as a container host on Azure Kubernetes Service (AKS). The new distribution is designed to provide reliability and consistency across the AKS, AKS-HCI, and Arc products. Azure Linux node pools can be deployed in a new cluster or added to existing Ubuntu clusters. It is also possible to migrate existing Ubuntu nodes to Azure Linux nodes.
The distribution is designed to be lightweight, both for performance and security, with a 400MB core image and around 300 packages. Jim Perrin, principal program manager lead at Microsoft, writes:
Azure Linux is designed with a minimalist view and a cloud focus. Window managers and other graphical interfaces are removed, resulting in a lower attack surface, reduced dependencies, and a generally smaller footprint. This ultimately helps reduce update interruptions and improves reboot speeds.
The general availability follows last year’s preview announcement and Azure Linux is the same distribution as CBL-Mariner. The distribution has the primary purpose of serving as the container host for AKS (AKS), running as a VM on Hyper-V but bare metal installations on x64 or ARM64 are also possible. Perrin adds:
Getting started with the Azure Linux container host is as easy as changing the OSSku parameter in your ARM template or other deployment tooling.
User cpressland on Reddit writes:
We’ve been running Mariner Linux in Production for just over a month and it’s been absolutely rock solid. Nice to see this get an official name (…) and for our non-AKS workloads I hope this becomes an option there too.
While the general availability is recent, Microsoft claims to be running Mariner as the internal Linux OS since last year, with products such as Xbox, Playfab, Minecraft, and many Azure services deployed on Mariner.
The famous Linux is a cancer quote from Steve Ballmer has been mentioned by many since the announcement. Peter Zaitsev, founder at Percona and open source advocate, tweets:
A couple of decades ago you could hardly imagine there would be such a thing as Microsoft Linux.
Microsoft is not the only cloud provider developing a lightweight distribution for cloud deployments, with Amazon Linux 2023, an option with long-term support on AWS, and Container-Optimized OS from Google, based on the Chromium OS project, other popular choices.
The CBL-Mariner documentation is available on GitHub.
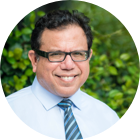
MMS • RSS
Posted on nosqlgooglealerts. Visit nosqlgooglealerts
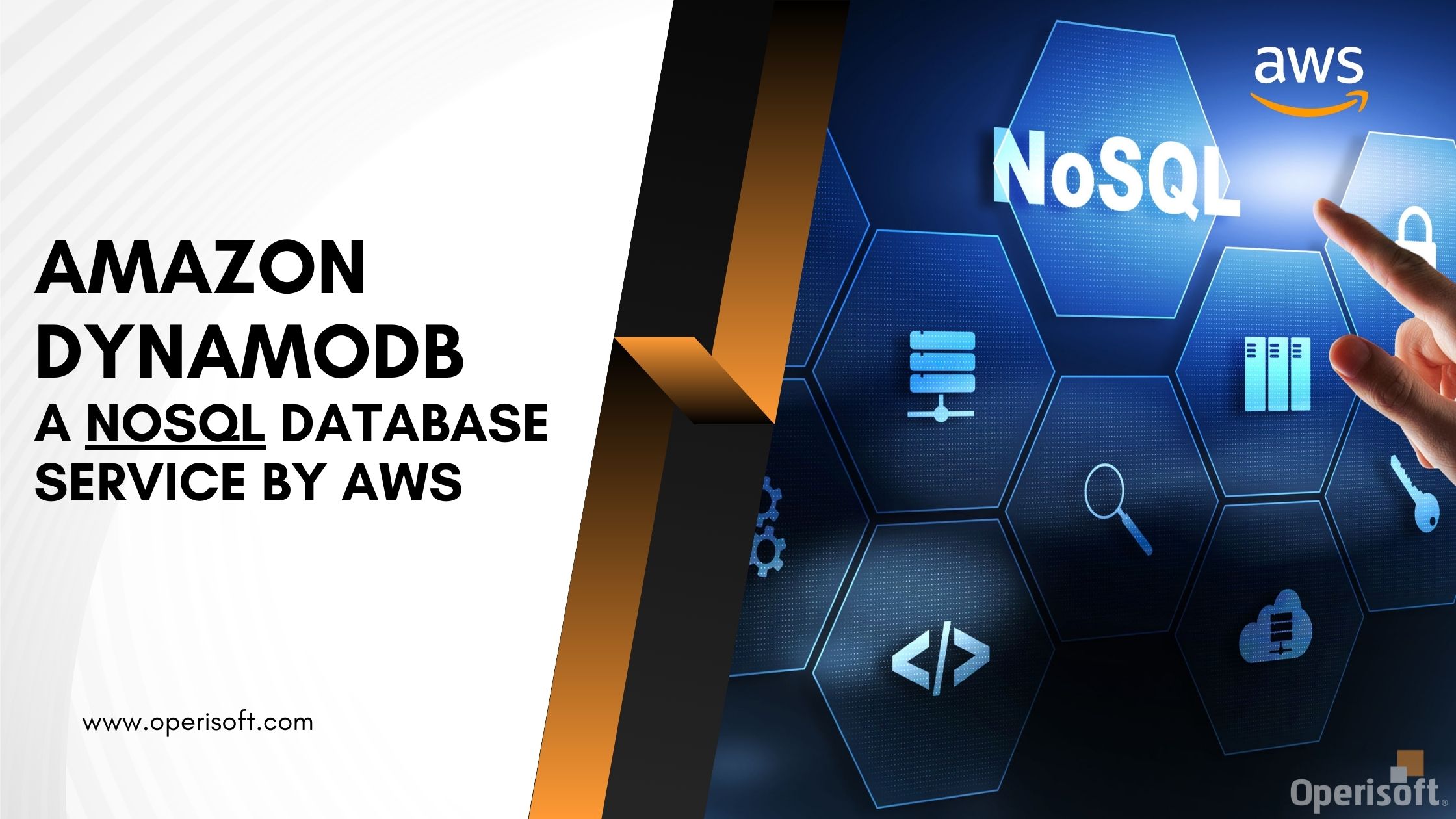
DynamoDB is a managed NoSQL database service offered by Amazon Web Services (AWS). It has gained immense popularity as a highly available, scalable, and reliable data solution among cloud computing infrastructures. DynamoDB is a fully managed service that eliminates the need for AWS customers to manage their own database servers and provides extraordinary flexibility in terms of data access, storage, and management.
What is DynamoDB?
DynamoDB is a popular NoSQL database offering from AWS that provides high availability and scalability. It eliminates the need for manual scaling, managing, and monitoring of data infrastructure. It provides an efficient and reliable data storage service in a managed environment. DynamoDB can handle big data, and it helps users to store and retrieve any amount of data with automated and optimal performance allocation and dynamic provisioning capacity.
The platform is designed to serve adaptable workloads, and its flexibility to handle varying data demands makes it ideal for real-time applications. DynamoDB supports JSON-based document storage and semi-structured data models. It enables users to access and manage their data across multiple regions globally and provides secure and fast access to data with advanced features like encryption, backup, and restore capabilities.
DynamoDB Architectural Overview
The DynamoDB architecture allows for partitioned scaling, load balancing, and multi-region support. Every item in the table is automatically distributed across multiple partitions, and the partitions are distributed uniformly to maximize storage operations and cache utilization. The platform operates on a master-slave data replication configuration, with writes directed to a master node that forwards the data to its corresponding slaves.
The write propagation is performed for both local and global scenario, where writes are attempted entirely on local nodes before being distributed globally to all affected nodes. This design helps to reduce response time latency for local and regional reads and actions. DynamoDB offers high-performance querying via its well-designed indexing system. The platform employs Local Secondary Index (LSI) and Global Secondary Indexes (GSI) to support fast querying operations.
Features of DynamoDB
Scalability and High Availability
DynamoDB is a highly available, scalable, and distributed data storage service, making it an ideal solution for enterprises with fluctuating workloads. It was designed for scale from its inception and can be scaled up or down based on business demands automatically. Scaling is performed through partitioning; a technique that divides data into smaller units and allocates storage and processing resources to each partition. The platform provides multiple built-in features, such as auto scaling, capacity on-demand, read/write provisioning, and more, all aimed at providing scale and elasticity.
Flexible Data Models
DynamoDB supports a flexible data model that allows users to store unstructured, semi-structured, and structured data in its service. Its data models can be categorized as key-value, document, and more. The platform supports data types such as numbers, strings, binary data, sets, and document data format, including list and maps. Users can choose from any of these data models and formats based on their data needs and access or modify their data in real-time to suit their use cases.
Security and Availability
DynamoDB provides an enhanced level of security and availability for users and their data. It provides automated backups, point-in-time recovery, multi-copying, and encryption of data-at-rest and in-transit. These features provide data protection and privacy, making it ideal for businesses that require compliance and regulatory compliance. AWS also provides users with tools to manage and monitor access to their data and network traffic in real-time, including encryption for access keys, data encryption, and data access control management.
Low Latency and High Performance
DynamoDB provides a low read and write latency data access process through its global and multi-region availability, partitioning, and load balancing features. It ensures that all read and write actions are performed quickly and efficiently, irrespective of the volume or changing patterns of traffic. DynamoDB also supports caching and indexing, which enables the application to easily store and retrieve frequently used data records. Its caching feature helps reduce the overall response time for frequently accessed data records, leading to optimized performance and lower costs.
Use Cases for DynamoDB
Internet of Things Sensors and Devices
DynamoDB can handle IoT data as sensor data, device telemetry data, and more. IoT devices generate massive amounts of data generated in real-time, which may require immediate processing, querying, and analysis to identify anomalies, optimize performance, and reduce downtime. It is a perfect solution for data storage with high availability, capacity, and fast access speeds to support IoT device data management and analytics.
Gaming
DynamoDB provides gamers with a scalable, efficient, and high-performance solution for managing their data, including user profiles, game data, and game metadata. The platform is designed to handle high traffic and sudden spikes in usage demands, providing low latency and high throughput read and write actions, with automatic scaling and capacity provisioning.
High-Speed and Scalable Web Applications
DynamoDB is perfect for high-traffic web applications, chat applications, and social media networks. It is designed to deliver fast response times and high throughput, providing low latency, read and write actions with high scalability. Its support for multiple data models, flexible schema, and rich querying options makes it an ideal solution for web applications with various data requirements.
Real-Time Analytics
DynamoDB is a perfect solution for real-time analytics in the cloud. It can store and process large datasets and provide developers with a flexible, cost-effective, and highly available solution for running large-scale data analytics and machine learning models. Its stateless architecture, support for various data models, and built-in indexing make it a good platform for real-time data processing and querying operations.
DynamoDB is a powerful managed NoSQL database service that is designed to handle any size workload and data model with high scalability, availability, and performance. It eliminates the need for manual database management, provisioning, scaling, and monitoring and allows the users to focus on their business logic and application development. It provides a robust solution for multiple use cases, including IoT, gaming, web applications, and analytics, and has advanced security and data protection features.
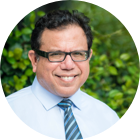
MMS • Brandon Byars
Article originally posted on InfoQ. Visit InfoQ
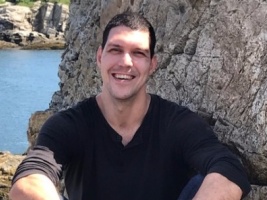
Transcript
Byars: I want to talk about the journey of evolving an API without versioning. I like to start this with a little bit of a story. Just for background context, when I say API, a lot of folks immediately jump to something like a REST or a GraphQL API, and those certainly fit the criteria. So do Java APIs that operate on process, or event-based APIs, they still have some agreement that needs to be made between their consumers and the producers. A lot of the talk is dedicated to the complexity of that agreement. This is based on a real story, but it has been changed, because the story itself isn’t public.
The context, we want to book a cruise, ourselves and a partner for a vacation. This sequence diagram is an overly simplified view of what happens from a systems standpoint, when you book a cruise. It might be that we have a reservation system. It’s basically a two-phase commit. You hold the room. You pre-reserve the room until payment has been collected through a payment gateway. Then the reservation itself is confirmed in the underlying reservation system. When this works well, everybody is happy. What happens in this real scenario that I’ve abstracted is there was a significant spike in load. What that spike in load did is it forced the reservation system to send back an unexpected error on that last step, at confirming the reservation. An error that the team that developed the booking service had never seen before. Normally, you run into unexpected errors in systems, you get some unpredictable behavior. In this case, the unpredictable behavior was fairly catastrophic for the organization. Because what they had done is they’d built a retry loop around unexpected errors in the booking service for the entire workflow.
Under load, at peak volume, I might try to book a service. I’d pre-reserve the room. My credit card would be charged, getting their own confirmation. Retry, pre-reserve another room, charge my credit card, get in there, preauthorize or pre-reserve another room, charge my credit card again, and so on. What happens is that that loop continued until either a customer had pre-reserved every room on the ship, or they had maxed out their credit card, and the payment gateway itself returned an error, or there was some fraud-based error alert from the payment gateway. That was obviously a big PR disaster for the organization. It caused a lot of consternation. It was very CNN visible headline. All based on the fact that the agreement between the API producer of the reservation system and the API consumer of the booking service did not completely cover the surface area of responses available.
Background
My name is Brandon Byars. I am head of technology for Thoughtworks North America. This talk is based on a significant amount of experience that I’ve had in API development throughout my career. I’ve written an open source tool that will be the baseline of this talk following the API bit over nearly a decade, called mountebank. A few articles on martinfowler.com. This one is based on one that I haven’t yet published. It’s actually long been in my queue to finish, and this talk is a little bit of a forcing factor for me to do that. This is all based on real world experience. I have led a number of platform engagements. We consider these API platforms a really good way of skilling development inside organizations. One of those articles, the enterprise integration using REST, is quite dated, maybe 10 years old at this point.
This adaptation of Jamie Zawinski’s quote here on regular expressions is something that I wrote in that article. “Some people when confronted with a problem think, I know I’ll use versioning. Now they have 2.1.0 problems.” Versioning is oftentimes seen as the standard de facto approach to evolving an API in a way that makes sure that the agreement on backwards incompatible changes, on breaking changes is made explicit, forcing the consumers to upgrade for the new functionality, but in a very managed way. That’s a very architecturally sound strategy. There’s a reason that’s used so widely. You see here on the left, an adaptation of the old famous Facebook slogan of moving fast and breaking things. As you see from the quote that I put on the right, I like to challenge the idea of versioning as the default strategy, because I think it does cause a lot of downstream implications. The fact that all consumers do have to upgrade is itself a point of inconvenience for many of those consumers. This talk is really dedicated to exploring alternative strategies that produce more or less the same results, but with different tradeoffs for the consumers and different tradeoffs for the producer as well.
Contract Specifications as Promises
When we talk about APIs, again, REST APIs, GraphQL, Java APIs, it doesn’t matter, events, we have something like a contract, the specification. Of course, in the REST world, OpenAPI tends to be the 800-pound gorilla. There are of course alternatives, but this is a pretty widely used one. It’s easy to fall into the trap as technologists to think of that specification as a guarantee. I really like the word promise. Mark Burgess came up with this promise theory. He was big on the configuration management world, CFEngine, and Puppet, and Chef, and so forth that led to infrastructure as code techniques that we use today. He has a mathematical basis for his promise theory in the infrastructure configuration management world. For more lay audiences, he wrote a book on promise theory, and this quote came out of it. “The word promise does not have the arrogance or hubris of a guarantee, and that’s a good thing.” Promises fundamentally are expressions, communication patterns that demonstrate an intent to do something, but promises can be broken. As we saw in the reservation system example, promises can sometimes be broken in unexpected ways that lead to cascading failures.
Following the Evolution of a Complex API
I’d like to explore that idea of making best effort attempts to solve customer’s needs through some storytelling. I mentioned this open source product that I’ve managed for nine years now called mountebank. It’s a service virtualization. If you are familiar with mocks and stubs that you might use to test your Java code, for example, service virtualization is a very similar construct. It just exists out-of-process instead of in-process. If your runtime service depends on another service, if you’re put in the booking service, you depend on the reservation service, and you want to have black box tests, out-of-process tests against your booking service. In a deterministic way, where you’re not relying on certain tests to be the test data to be set up in the reservation systems, you can virtualize the reservation system. Mountebank allows you to do that. It opens up new sockets that will listen, and that will listen for certain requests that match certain criteria, and respond in a way that you the test designer set up. It’s a very deterministic way of managing your test data.
There’s more to it than this picture on the bottom. In the book that I wrote, I had to draw a number of diagrams that described how mountebank worked. This one covers more or less the back part of the process, generating the response. Mountebank gets a call. It’s a virtual service that needs to respond in a specific way, returning the test data relevant to your scenario. What it does is it grabs a response. There’s multiple types of ways of generating a response, we’ll look at a couple. Then the bulk of the storytelling is going to be around this behaviors box. Behaviors are post-processing transformations on those response. We’ll look at some examples because there has been a significant evolution of the API in sometimes backwards incompatible ways in that space, all done without versioning. Then the core construct of mountebank is virtual servers. Mountebank just holds an imposter, but it’s the same idea as the virtual stub.
Evaluating Options from a Consumer’s Perspective
As we look at some of the different options, where versioning wins hands down is implementation complexity. When you version an API, you can simply delete all of the code that was there to support a previous version. You can manage the codebase in a more effective way if you are the API producer. I’m not going to look at implementation complexity as a decision criteria because I’ve already experienced that versioning wins on that front. Instead, as I look through a number of alternatives to versioning, I’m going to look at them from the consumer’s perspective. These three criteria are the ones I’m going to focus on. When I say obviousness, think the principle of least surprise. Does it do what you expect it to in a fairly predictable way? Does it match your intuitive sense of how the API should work? Elegance is another proxy for usability. When I think elegance, is it easy to understand? Does it use the terms and the filters in a consistent way? Is the language comprehensible? Does it have a relatively narrow surface area because it’s targeted to solve a cohesive set of problems? Or does it have a very broad surface area and therefore hinder the ramp-up to comprehension, because it’s trying to solve a number of different problems in an infinitely configurable way? Then stability is, how often do I as the API consumer have to change to adapt to the evolution of the API?
Evolution Patterns – Change by Addition
A few patterns all in real-world patterns that came out of my experience, maintaining mountebank. This snippet of JSON is an example of how you might configure a response from the virtual service. This is HTTP. Mountebank supports protocols outside of HTTP. This is, I think, a pretty good one. All this is doing is saying how we’re going to return 500 with that text that you see in the body. You can also set up things like headers, for example. One of the first requests for a feature extension after releasing mountebank was, somebody wanted to add latency to the response. They wanted to wait half a second or three seconds before mountebank responded. The easiest thing in the world would have just been to add that quite directly, some latency to the JSON, which is pretty close to what I did. I added this behaviors element with a little bit of a clumsy underscore, because I was trying to differentiate the behaviors from the types of responses, represents generation of a canned response, like you see here. There’s two others. There’s ways of record and replay, and it’s called proxy. There’s ways of programmatic configuration of response, it’s called inject. Since those are not underscore prefixed, I thought I would do the underscore on the behaviors.
More importantly, I thought that having a separate object, even though I only had one use case for it right now on this latency, was a point of extension. I think that’s just a foundational topic to bring you up. We talk a lot about backwards compatibility. There is a little bit of forward thinking that allows us to cover up at least some forward compatibility concerns. If we can do something as simple as ensure, for example, that our API doesn’t respond with a raw array. Because as soon as you need to add paging information, and you have to add an object wrapper, you’ve made a breaking change. Adding an object for extensibility is a pretty popular forwards compatibility pattern. This is an example, even though I wasn’t quite sure what I would use it for when I wrote this. This works pretty well. This was just your simple addition to an API. This is Postel’s Law, where you should be able to evolve an API in a way that doesn’t change or remove elements and only adds to them. When I think about how that fits against the rubric that I mentioned earlier, I think this is as good as it gets. We should always feel comfortable as API producers, adding new elements, being a little bit thoughtful about how to do that in a forwards compatibility way. This covers obviousness, elegance, and stability quite well.
Evolution Patterns – Multi-typing
That worked great. Then somebody said, I want the latency to be configurable. I mentioned that mountebank has this inject response type, which lets you programmatically configure a response. I thought maybe I would take advantage of that same functionality to let you programmatically configure the latency. What I did is I kept the wait behavior, but I just had it accept either a number or a string that represents a JavaScript function. I call that multi-typing. It worked well enough. It allowed me to fit within the same intention of adding latency, which are two different strategies of how to resolve that latency through a number of milliseconds or a JavaScript function. It’s not as obvious. It’s not as elegant. I have not done this since that initial attempt. If I were to run into the same problem today, I’d probably add a separate behavior, something like wait dynamic. I think that’s a little bit less elegant because of experience the surface area, because you have to understand the API, but it’s a bit more obvious. I think obviousness and making sure that it makes it easy, for example, to build a client SDK, that doesn’t have to have some weird translation. Because you need different maybe subclasses, or functions, or properties to describe the API in a way that gets translated to how the API works, because it’s polymorphic in sometimes unhelpful ways. It works. I wouldn’t recommend it. It certainly involves not having to release a new version to fix the API itself.
Evolution Patterns – Upcasting
This third pattern is really my favorite. It’s upcasting. It’s a pretty common pattern. You see a lot in the event driven world, for example, but it really works for a number of different kinds of APIs. A subsequent behavior that was added to the list was this one around shellTransform. The idea was, mountebank has created this response, this status code, this body, but sometimes I want to post-process that JSON, to change it to add some dynamic information. I want to be able to use a shell program because I don’t want to parse in a JavaScript function. I want maybe to use Ruby, in this example, to do something dynamic. It was relatively easy to build that. Then what people asked for was, actually, I want a pipeline of shell programs. I want to have very small targeted shell programs that did one thing and be able to compose multiple of them to generate the post processed response. What I had to do was change shellTransform, originally a string into an array. It would execute each of those shell programs in order in the array. This one, assuming that both the string and the array can be parsed, is a little bit less obvious, because it does have some components of that multi-typing that we just looked at. It’s actually managed in a much more productive way. I think this is a very elegant and very stable approach. I think this is one of the first approaches that I generally reach for when I try to evolve an API without breaking their consumers. Let me show you how it works.
First of all, just to acknowledge, this is a breaking change. We changed the API from a string to an array. The new contract, the new specification of the API lists only the array. It does not advertise that it accepts a string. I could have simply released a new version, changed the contract to the array, and asked any consumers who had the string version to update themselves. That would have been at their inconvenience. The upcasting allows me a single place in the code that all API calls go through. I have this compatibility module, and I follow the upcast function on it, parsing in the JSON that the consumer is sending in the request. You can see the implementation of that upcast function, or at least a portion of it down below. I have this upcastShellTransformToArray, and there’s a little bit of noise in there. It’s basically just looking for the right spot in the JSON and then seeing if it is a string. If it is, it’s wrapping the string with an array so it’s an array of one string. It is managing the transformation that the consumers would have had to do in the producer side. It’s adding a little bit of implementation complexity, although quite manageable, because it’s all managed in one spot in the code, at the core of the tradeoff of not having to inconvenience any consumers.
Another reason I really like the upcasting pattern is that it works a bit like Russian dolls, you can nest them inside of each other. This is another example over time, the behaviors, these post-processing transformations of the response, added a bit more functionality. You see several here, wait, we mentioned that adds 500 milliseconds. ShellTransform, now a list of shell programs that can operate on the JSON and the response. Lookup also has a list. Copy has a list. Decorate is just a string transformation that you can run. Then it has this repeat directive that allows you to return the same response to the same request multiple times in a row. Normally, it works like a circular buffer, it rotates through a series of responses, but you can ask it to hold back for three times on the same response before cycling to the next one.
I wanted to do in a much more composable way because it allows the consumer to specify the exact order of each transformation, which isn’t possible on the left. On the left, there’s an implicit order encoded inside mountebank, not published, not advertised. While some transformations operate one time, at most, like decorate or wait, some can operate multiple times, like shellTransform and lookup. Repeat, it turns out doesn’t really belong there, because it’s less of a transformation on the response and more a directive on how to return responses when there’s a list of them from mountebank standpoint. What I wanted to do was have a list where every single element in the list is a single transformation, and you can repeat the transformations as much as you want. If you want to repeat the wait transformation multiple times, that’s on you, you can do it. It’s very consistent. This actually allowed me to make the API, in my opinion, more elegant, and more obvious, because it works more like consumers would expect it to work rather than just demonstrating the accidental evolution of the API over the years. I rank this one quite high, just like testing in general, but like all non-versioning approaches, it does require a little bit of implementation complexity.
The good news is that the implementation complexity for nested upcasting is trivial. All I have to do, I have the exact same hook in the pathway of requests coming in and being interpreted by mountebank, you can call this compatibility module, and all I have to do is add another function for the additional transformations after the previous one. As long as I execute them in order, everything works exactly as it should. We did the upcastShellTransformToArray, so took the string, made an array. The next instance, all I have to do is make the other transformation. If you have a very old consumer that only has the original contract, it’ll upcast it to the next internal version of that contract. Then the upcastBehaviorsToArray, we’ll update it to the published contract as it exists today at mountebank. The implementation was pretty trivial. It was just looking for the JSON elements in the right spot and making sure that if there was an array, it would unpack each element of the array in order. If it was a string, it would keep it as is but it’ll make sure that every single element in the behaviors array had a single transformation associated to it.
Evolution Patterns – Downcasting
The next instance of a breaking change managed without a version was far more complex. This one is going to take a little bit of a leap of faith to understand. I don’t want to deep dive into how to use mountebank, or the mountebank internal mechanics too much. This one does require a little bit more context. I mentioned that mountebank allows you, as we’ve already seen, to represent a canned response that it’ll return. For HTTP, we had the 500 status code in the body text. An alternative is this way of programmatically generating a JSON response, instead of, is, you parse in inject in a JavaScript function as a string, as you see here. The function at first just had the original request that the system under test made to mountebank as a virtual service. There is a way of keeping state so that if you were to programmatically generate the response, and maybe you wanted to add how many times you’ve done that, you could keep a counter, and you could attach the result to that counter as part of the response that you generated, and a logger. That was the original definition of the JavaScript function. You could parse it. Pretty early on, people wanted to be able to generate the response in an asynchronous way. Maybe they wanted to look something up from the database or have a network hop, so I had to add this callback. Then, a little bit later after that, it turns out that the way I’d implemented state was too narrowly scoped. Somebody made a very good pull request to add a much better way of managing state. It was certainly inelegant because I had these two state variables and the JavaScript function. While I tried to do my best in the documentation to explain it, that certainly did not aid comprehension for a newcomer to the tool, that required having to follow along the accidental evolution of the tool.
Anybody who’s done a lot of refactoring in dynamic languages, languages, in general, know that one of the most effective ways to simplify that type of interface is to use this idea of a parameter object. As you have parameters start to explode, you can replace it with a single object that represents the totality of the parameters. Then, of course, that makes a very easy extension point, because if I need to add a sixth parameter down the line, it’s just a property on that config object. This is the new published interface for mountebank. Again, a breaking change, because for people who passed on that JavaScript function on the left, they now have to be transformed into that JavaScript function on the right. However, assuming mountebank can do that transformation for you, through this technique called downcasting, it’s a pretty elegant way of managing the complexity in a producer, instead of passing it on to the consumers. It’s not quite as obvious because there is a little bit of magic that happens underneath the hood. It’s not quite as elegant, because you do have this legacy of these old parameters that somehow have to be passed around. If done well, it can be very stable.
Here is what it looked like, in this instance in mountebank. What we basically did was we had the new parameter object, this config parsed in, and we continue to parse the subsequent parameters, even though we don’t advertise them, we don’t call them out explicitly on the contract. You can’t go to the mountebank documentation today, and see that these parameters are being parsed in. The only reason they are is for consumers who have never updated to the publish contract using the old contract. Those older parameters will still be parsed in. That solves everything beyond the first parameter, the parameter object. It doesn’t solve what happens with the parameter object itself, because that still needs to look like the old request that used to be parsed in. That’s why we call this downcastInjectionConfig call down here. That takes us back to the compatibility module. All of my transformations that manage breaking changes in the contract, I can centralize in this compatibility module. I can go to one place and see the history of breaking changes through the API. When I say breaking changes, they are breaking changes to the publish contract, but mountebank will manage the transformation from old to new for you. The consumer doesn’t have to.
In this case, what I had to do to make that config parameter object that had to have state, had to have the logger, had to have the done callback on there so that people using the new interface, it would work as expected. For people using the old interface, it had to look like the old request. That’s what this bolded code down below is doing. There’s a little bit of internal mechanics that I mentioned. Mountebank has multiple protocols, there’s method and data, or ways of sensing for, in this case, HTTP and TCP. Then what it would do is it would take all of the elements of the request, none of which I knew conflicted with the names of state and logger and the done callback. I had to just have that expert knowledge as the person who architected the code to know I wasn’t going to run into any naming conflicts, but it would add all of the elements like the request headers, the request body, the request query string, to the config object. While it was a parameter object that only had state and the logger and callback for most consumers, if you happened to have your code use the old function interface, it would also have all the HTTP request properties on it as well. It continued to work. That way, it was downcasting the modern code to the old version in a way that would support both old and new in a way that was guaranteed to not run into any naming conflicts.
Evolution Patterns – Hidden Interfaces
This next pattern is, I think, where things get really interesting and really explore the boundaries of what is a contract, and what is a promise that I hinted at early. Getting back to the shellTransform. I gave a little bit of a brief description of it. It allows you to build a shell program written in the language of your choice, that would receive the JSON encoded request and response. It would allow you to spit out a JSON encoded response. It allows programmatic transformation. If you were writing this in JavaScript, for example, the way it was originally published, your code would look something like this. The request and the response would be parsed as command line arguments to your shell program, voted the right way. You would have to interpret those in your code. That had all kinds of problems, especially in Windows. It has to do with the maximum length of the command line, which is actually more variable than I understood when I wrote this code between operating systems and shells. In Windows it’s quite limited. It’s maybe 1048 characters or something like that. Of course, you can have very heavyweight HTTP requests or responses. If you are inputting that JSON, and it’s a 2000-character body, you’ve already exceeded the limit on the shell. That’s the character limit itself.
There are also a number of just polling complexities to quote the JSON the right way and escape internal quotes for the different shells. I figured it out on Linux based shells. The variety of polling mechanisms on Windows-based shells, because there’s more than one, you have PowerShell, you have the cmd.exe, you have the Linux Cygwin type ports, was more complexity than I realized when I went with this approach. What I had to do was have mountebank as the parent process, put these things in environment variables that allow the child process to read the environment variables, very safe, very clean. I don’t know why I didn’t start there from the beginning, but I didn’t. That’s the reality of API developments, you make mistakes. I wanted this to be the new published interface. Of course, I still had to leave this in there. I just removed it from the documentation. That’s what I mean when I say a hidden interface. It’s still supported, it’s just no longer part of the published contract. If it worked, I think it’s a reasonably safe way of moving forward. I downgraded stability a little bit. I think the reason is into that with the description I gave you of the character limitations of the shell.
What happened was by still publishing stuff to the command line, and this code down here was more or less the code that let me do it in this quoteForShell, manage the complexity of trying to figure out if they’re on Windows and how to quote it exactly right. Unfortunately, even if you weren’t using the old interface, even if you weren’t using the command line interfaces, if your shell program was using the environment variables, it still introduced scenarios where it would break mountebank, because it would put the command line variables as part of the shell invocation. Sometimes in certain shells, in certain operating systems, that invocation would exceed the character limit supported by the shell itself. Even though you had no intention of using them, even though you didn’t know they were being parsed, mountebank would throw an error, because it exceeded the shell limitation.
For a while, what I tried to do was say, let me be clever, and if you’re on Windows, do this, if you’re on Linux, do that. It was too much complexity, I don’t know that I’m smart enough to figure out actually how to do it all. Even if I was, you would still run into edge cases. No matter how big the character limit of the shell is, there is a limit. It’s possible to exceed that limit, especially if you’re testing very large bodies for HTTP, for example. My first attempt was to just truncate it by shells, but pretty soon I realized that was a mistake, so I had to truncate it for everybody. This was a real tradeoff. I think, probably the pivotal moment in this talk, because there was no way for me to guarantee that I could do this without a version without breaking people. If I truncated it for people who were on a Linux shell that had hundreds of thousands of characters as a limit, and I truncated it for Windows, which maybe had 1000 or 2000-character limit. There may be people who used the old interface on Linux, post-truncation, that they would get an error. I was unaware of any. I had zero feedback that that was the case. It was certainly a possibility, even if it was somewhat remote. Because the way of publishing on the command line wasn’t around for very long before it switched to the environment variable approach.
Releasing a new version would have been the safest option by far to satisfy all of the constraints around stability in that scenario. However, it would have also forced consumers to upgrade. It would have been very noticeable to consumers. They would have had to read the release notes, figure out what they need to change, and do around the testing associated with that. If alternatively, I took the approach that I did, which was to just truncate in all cases, publish only the environment variable approach, and rely on the fact that it was unlikely to break anybody. If it did, the error message would exactly specify what they needed to do to fix it until you switch to the environment variables. Then I was optimizing for the masses. I was optimizing for what would support most people in a very frictionless way with a clear path of resolution for what may be zero people who are affected by the breaking change.
How To Think About API Evolution
That’s uncomfortable, because that forces us to rethink API evolution away from an architectural pattern that guarantees stability, to thinking about it as the users would think about it. I was really inspired by this thing called Hyrum’s Law. Hyrum worked at Google. With a sufficient number of users on the API, it doesn’t matter what you promised in the contract, because consumers will couple themselves to every part of the API. I remember for a while, Microsoft Windows, when they would update, they would have to add code to the updated operating system because they would test not just the operating system itself, but they would test third-party applications using the operating system. Third-party developers had done all kinds of very creative things with unpublished parts of the Windows SDK for a long time. Windows, as they changed these unpublished parts of the SDK, maybe we were doing something clever with this eighth bit that was unused in a byte, which was a real scenario that happened sometimes. They would have to detect that and write code in the new operating system that would continue to support the same behavior, even though there’s never something that guaranteed.
Hyrum’s Law
There’s a famous xkcd comic out there where users are complaining about their Emacs was taking advantage of the fact that when you held the spacebar down, it overheated the computer to create some side effect. The developer was like, no, I just fixed the overheating problem. The Emacs user was like, no, can you change it back to the old behavior. Hyrum’s Law is a really humbling law for an API producer. Especially as one who has had a public API available for most of a decade now, I really relate to how frequently I find myself surprised at how people have hacked an API to do something that I didn’t anticipate they could do in a way that I wasn’t intending to support, but now is oftentimes supported. Mountebank is primarily a RESTful API, but some people embedded it in JavaScript, and I never really meant to support it. Some people did that because it solves the startup time, it’s just part of your website instead of a separate website. Now I have this accidental complexity of supporting a JavaScript API that you could embed in an Express application as well. That’s an example of Hyrum’s Law. Mentioned in this book, “Software Engineering at Google,” which is why I put it there. I think I got a lot of value from some of the patterns of what Google’s had to do to scale to 50,000 engineers.
API Evolution Is a Product Management Concern
We talk a lot about API as a product nowadays, usability, feasibility, viability being common descriptions of the tradeoffs in product management. I think that rethinking backwards compatibility evolution or breaking change evolution, from an architecture concern to a product management concern, is a much healthier position to think about how to manage the evolution of your API. I think that the tradeoffs that are represented by product thinking are more nuanced than the tradeoffs represented by architecture thinking. I think versioning is a very solid architectural pattern that guarantees stability in the case of breaking changes. There are always needs for that pattern. Mountebank itself has enough debt underneath it. One of these days, I would like to release a subsequent version that allows me to remove a lot of the cruft, a lot of the things I really no longer want to support, but have to because of some of these backwards compatible transformations that I’m doing.
If we think about viability, we’re solving problems that our users have, an API context, I really liked the idea of cognitive load that the authors of “Team Topologies” talk about. When I think about any product, what I really want to do is simplify the underlying complexity, I really have no idea how my phone works. I have no idea how it connects to a cell tower. I don’t understand the underlying mechanics, physics, material design. I barely understand the software. It simplifies an interface for me to be able to use it. Same as driving a car. It’s a couple pedals and a steering wheel. We have mirrors in the right places. I can drive without having to understand the underlying complexity of the system that I’m driving. I want my APIs to do the same thing. Usability really has been the focus of this talk. How do I manage evolution to that system, or to that interface in a way that provides the most usable and stable experience for my users? Then, feasibility is very much an architectural concern. How do I do that in a way that is technically feasible, that protects downstream systems and it satisfies the non-functional requirements of the overall ecosystem at large? Rethinking API evolution as product management, I think, for me has been a pretty profound way of understanding the needs and empathizing with the needs of the consumers of mountebank. It’s something that I’d recommend you consider as you’re evolving your own API, versioning is always an option that you can reach for. Upcasting and some of these others, I think, would be valuable additions to your toolbox.
Questions and Answers
Betts: How do you hide the complexity and keep it from being too bloated?
Byars: A large part of that, in my context, was trying to centralize it. Almost all of the code in mountebank only knows how to respond to the newest interface that is documented and supported behind the contract. Most of the code doesn’t have this legacy behind it. For upcasting, there’s one hook in the request processing pipeline that causes compatibility module. That’s where all the transformations happen that convert from old to new. The exceptions are downcasting. A few downcast calls have to be sprinkled in certain strategic areas of your code. That is a little bit of debt that I’d love to clean up someday with the new version. For most of the transformations, it’s pretty straightforward.
Betts: There was a question about returning a string instead of other data types. That made me wonder, a lot of your patterns you talked about are how you handle changes to the request to support different inputs. How do you evolve the response that you give to the consumer?
Byars: I don’t think there is a path that I see for the producer managing backwards incompatible changes on the response without a version. In fact, this is one of the driving forces that I would love to someday create a version for mountebank on, because there are some responses that I look on now, and it’s like, “I wish I hadn’t had done that.”
Betts: Sometimes these changes happen, and you have to evolve because there are just new features you want to add. Sometimes it’s a mistake in the original design. What drove you to make the change? Does that influence your decision?
Byars: Ideally, you’re trying to be thoughtful in the API design to make plugging in new features an addition. That has been the norm. It’s not universal. Generally speaking, that’s an easier process. Sometimes covering up mistakes requires more thought on the API design change in my experience. There are simplistic ones where I really wish I hadn’t created this endpoint or accepted a PR with this endpoint that has a specific name, because it doesn’t communicate, where I’m really hoping that feature communicates to users. It actually conflicts with some future features that I want to add. That actually happened. What I did in that case was, there’s a little bit of hidden features going on, and I just changed by addition. I created the new endpoint with a name that wasn’t as elegant as what I originally wanted to. I just compromised on that because it was more stable. My criteria is less elegant, but more stable. I just accepted that. There’s a tradeoff. Sometimes you can’t get the API exactly the way you want because of the fact that you have real users using it. That’s not necessarily a bad thing, especially if you can keep those users happy. Until there’s an attempt at deprecating an old endpoint, create a new one I want to communicate, but still having a little bit of compromise in the naming of fields. Then, of course, some of these other patterns that you see here are other strategies that do require more thought, more effort than just adding a feature in most cases.
Betts: With your centralized compatibility module, how do you actually stop supporting deprecated features? With versioning you can delete the code that’s handling version, whatever of the API, as long as it’s in a separate module. Does this stuff live around forever?
Byars: Yes, I’ve never deprecated those features. As soon as I release something, and it’s hard for an open source product sometimes to know who’s using what features. I don’t have any phone home me analytics, and I don’t intend to add any. You have to assume that you’re getting some users of that feature. The good news is that with the centralized compatibility module, especially with upcasting, which is most of what I’ve done, it’s relatively easy to adjust. I’ve been able to take one of these other patterns that doesn’t require too much fuss. Downcasting is the hardest. One of these days, especially for the response question that you asked, because that’s where I have the most debt, that I haven’t been able to use these strategies to resolve, I would love to do a version. That would be the opportunity to do a sweep through the code that I no longer want to maintain.
Betts: I’m sure mountebank v2 will be really impressive.
Byars: The irony is I did release a v2, but it was a marketing stunt. I looked at the [inaudible 00:48:21] spec and they say, if it’s a significant release, you can use a major version. I felt pedantically validated with what they said. It was really just a marketing stunt, and I made sure in the release notes to say, completely backwards compatible.
Betts: There’s no breaking changes.
See more presentations with transcripts
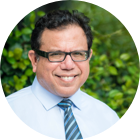
MMS • Akshat Vig
Article originally posted on InfoQ. Visit InfoQ
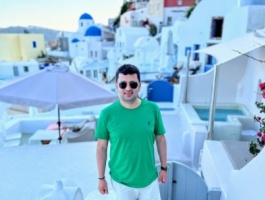
Transcript
Vig: How many of you ever wanted a database that provides predictable performance, higher availability, and is fully managed? What I’m going to do is talk about evolution of a hyperscale cloud database service, which is DynamoDB. Talk through the lessons that we have learned over the years, while building this hyperscale database. I am Akshat Vig. I’m a Principal Engineer in Amazon DynamoDB team. I’ve been with DynamoDB right from its inception.
Why DynamoDB?
AWS offers 15-plus purpose-built database engines to support diverse data models, including relational, in-memory, document, graph, time-series. The idea is that you as a customer can choose the right tool for the use case that you’re trying to solve. We are zooming in into DynamoDB, which is a key-value database. The first question that comes to mind is, why DynamoDB? Let’s go back to history. During 2004-2005 timeframe, amazon.com was facing scaling challenges caused by the relational database that the website was using. At Amazon, whenever we have these service disruptions, one thing we do as a habit, as a culture, is we do COEs, which are basically correction of errors. In that COE, we ask questions, how can we make sure that the issue that happened does not happen again? The use case for which that particular outage happened was related to a shopping cart. One of the questions that we asked in the COE was, why are we using a SQL database for this specific use case? What are the SQL capabilities that are actually needed? It turns out, not many. Choosing the right database technology is the key to build a system for scale and predictable performance. At that time, when we asked this question, if not an SQL database, what exactly would we do? At that time, no other database technology existed that met the requirements that we had for the shopping cart use case.
Amazon created Dynamo. It was between 2004 to 2007, where Dynamo was created. Finally, in 2007, we published the Dynamo paper after letting it run in production, and used by not just the shopping cart use case but multiple amazon.com services. Dynamo was created in response to the need for a highly available, scalable, and durable key-value database for the shopping cart, and then more teams started using it. Dynamo was a software system that teams had to take, run the installations on resources that were owned by them, and it became really popular inside Amazon within multiple teams. Hearing this one thing we heard from all these teams is that, Dynamo is amazing, but what if you make that as a service, so that a lot of teams who are trying to become experts in running these Dynamo installations, it becomes easier? That led to the launch of DynamoDB.
Dynamo and DynamoDB, they are different. Amazon DynamoDB is a result of everything we have learned about building scalable, large-scale databases at Amazon, and it has evolved based on the experiences that we have learned while building these services. There are differences between Dynamo and DynamoDB. For example, Dynamo, it was single tenant. As a team, you would run an installation, you would own the resources that are used to run that service. DynamoDB is multi-tenant. It’s basically serverless. Dynamo provides durable consistency. DynamoDB is opinionated about it and provides strong and eventual consistency. Dynamo prefers availability over consistency, versus DynamoDB prefers consistency over availability. In Dynamo, routing and storage are coupled. We’ll see, routing and storage in DynamoDB are decoupled. Custom conflict resolution was something that was supported in Dynamo. In DynamoDB we have last writer wins. There are differences between Dynamo and DynamoDB.
Coming back to the question of, why DynamoDB? If you ask this question today, 10 years later, a customer still will say they want consistent performance. They want better performance. They want a fully managed serverless experience. They want higher availability on their service. We are seeing that, like consistent performance at scale, this is one of the key durable tenets of Dynamo. Key properties that Dynamo provides that as Dynamo is being adopted by hundreds of thousands of customers, and as the requests are increasing, even the request rates are increasing, customers who are running mission critical workloads on DynamoDB, the performance they’re seeing is consistent. They’re getting consistent performance at scale. Proof is in the pudding. One of the customers, Zoom, in early 2020 when they saw unprecedented usage that grew from 10 million to 300 million daily meeting participants, DynamoDB was able to scale with just a click of a button and still provide predictable performance to them.
DynamoDB is fully managed, what does it mean? DynamoDB was serverless even before the term serverless was coined. You pay for whatever you use in DynamoDB. You can scale down to zero essentially. If you’re not sending any requests, you don’t get charged for whatever you’re doing. It is built with separation of storage and compute. As a customer, in case you run into logical corruptions where you accidentally deleted some of the items or deleted your table, you can do a restore. Dynamo also provides global active-active replication where you have use cases where you want the data closer to the user, so you can run DynamoDB table as a global table.
On availability, Dynamo offers an SLA of four nines of availability for a single region setup. If you have a global table, then you get five nines of availability. Just talking about magnitude of scale, to understand that, like amazon.com being one of the customers of DynamoDB, 2022 Prime Day, amazon.com and all the different websites generated 105.2 million requests per second. This is just one customer. This can help you understand the magnitude at which DynamoDB runs. Throughout all this, they saw predictable single digit millisecond performance. It’s not just amazon.com, hundreds of thousands of customers have chosen DynamoDB to run their mission critical workloads.
DynamoDB, Over the Years
Introduction of DynamoDB. How is it different from Dynamo? What properties are the durable tenets of the service? Let’s look at how it has evolved over the years. DynamoDB over the years, it was launched in 2012, working backward from customer, that’s how Amazon operates. It started as a key-value store. We first launched DynamoDB in 2012, where you as the customer can do Put, Gets, and it scales. Foundationally, very strong. Then we started hearing from customers, we want more query capabilities, serverless capabilities in DynamoDB, and we added indexing. Then customers started asking about JSON documents, we added that so that they can now preserve complex and possibly nested structures inside DynamoDB items. Then, 2015, a lot of customers are asking us, can you provide us materialized views? Can you provide us backup, restore? Can you provide us global replication? We said, let’s take a step back, figure out what common building block we need to build all these different things that customers are asking. We launched DynamoDB Streams so that by the time we build all these native features inside Dynamo customers can innovate on their own, and a lot of customers actually used the basic scan operation and streams to innovate on their own. Most recently, we launched easier ingestion of data into Dynamo or easier export of data from Dynamo. Over the years, the ask from customers around features, predictable performance, availability, durability, that has been constant.
How Does DynamoDB Scale and Provide Predictable Performance?
How does DynamoDB scale and provide predictable performance? Let’s try to understand this particular aspect of Dynamo by understanding how exactly a PutItem request works. As a client, you send a request, you might be either in Amazon EC2 network or somewhere on the internet, it doesn’t matter. As soon as you make a request, you do a PutItem, it lands on the request router. Request router is the first service that you hit. As every AWS call, this call is authenticated and authorized using IAM. Once the request is authenticated and authorized, then we look at the metadata. We try to figure out, when exactly do we need to route the request? Because the address of like where exactly the data this particular item has to finally land, is stored in a metadata service, which is what the request router consults. Once it knows the answer, where to route the request, next thing it does is it basically verifies whether the table that the customer is trying to use, has enough capacity. If it has enough capacity, the request is admitted. In case the capacity is not there, request is rejected. This is basically admission control done at the request router layer. Once all that goes through, request is sent to the storage node. For every item in Dynamo, we maintain multiple copies of that data. DynamoDB storage nodes, one of the storage node is the leader storage node. The other two storage nodes are follower storage nodes. Whenever you make a write request, it goes to the leader, gets written on at least one more follower before the write is actually acknowledged back to the client.
We don’t have, not just a single request router, and not just three storage nodes, the service consists of many thousands of these components. Whenever a client makes a request, the request is routed to a specific storage node and sent to the request router, and then sent to the storage node. AWS just like a well-architected service, DynamoDB is also designed to be fault tolerant across multiple availability zones. In each region, there are basically request router and storage nodes which are in three different availability zones. We maintain three different copies of data for every item that you store in the DynamoDB table. Request router essentially does a metadata lookup to find out where exactly to route the request. It takes away the burden from the clients to do the routing. When I said storage and routing is decoupled, that’s what I meant, that the clients now don’t have to know about where to route the request. It is all abstracted away in the request router.
Wherever the request router gets a request, it finds out the storage nodes that are hosting the data, it will connect to the leader storage node. The leader storage node submits the request, acknowledges, and finally once it gets an acknowledgment from one more replica, it acknowledges it back to the client.
Data is replicated at least to two availability zones before it is acknowledged. DynamoDB uses Multi-Paxos to elect a leader, and leader continuously heartbeats with its peers. The reason it is doing it is that so that if a peer fails to hear heartbeats from a leader, a new leader can be elected so that availability is not impacted. The goal is to reduce the failure detection and elect a new leader as soon as possible in case of failures.
Tables
Now we understand the scale at which Dynamo operates, we understand how the request routing logic works. Let’s look at the logical construct, the table, and how exactly DynamoDB automatically scales as your traffic increases, as your data size increases in the DynamoDB table. As a customer, DynamoDB, you create a table, and each table, you specify a partition key. In this particular example, each customer has a unique customer identifier, and we are storing customer information in this table. Customer ID is your partition key. Then you also store other customer information like name, city, in the item as other attributes. DynamoDB scales by doing partitioning. How exactly that happens is, behind the scenes, whenever you make a call to DynamoDB with the customer ID or whatever is your partition key, Dynamo runs a one-way hash. The reason for doing that one-way hash is that it results in random distribution across the total hash page associated with that table. One-way hash, it cannot be reversed, it’s not possible to essentially determine the input from the hashed output. The hashing algorithm, it results in essentially highly randomized hash values, even for inputs that are very similar.
A table is partitioned into smaller segments based on the overall capacity that you have asked or the size of the table. Each partition, it contains a contiguous range of key-value pairs. For example, in this case, we have a green partition that has values roughly from 0 to 6. Similarly, we have the orange partition, which has values from 9 to B, and then you have the pink partition, which has values from E to F. Essentially, given a hashed value of an item partition key, a request router can determine which hash segment that particular item falls into, and from the partition metadata service, it can find out the three storage nodes, which are holding the copy of that particular item, and then send the request to that particular set of nodes.
As I explained previously, we have three copies of data in three different availability zones. If we have these three partitions, essentially, we have three green partitions in three different zones, three orange partitions, and then three pink partitions. All these partitions, the metadata about where exactly these partitions are, is stored in a metadata service. That particular metadata is called a partition map. What a partition map looks like, it essentially is the key ranges that that partition store supports, and then green1, green2, green3, these are essentially the addresses of the three storage nodes where that partition is actually hosted. Think about it, when Zoom comes and asks for 10 million read capacity unit table, we would essentially add more partitions. If suddenly they increase their throughput to 100 million, corresponding to that we would add more partitions, update the metadata, and that’s how DynamoDB scales.
Predictable Performance and Data Distribution Challenges
What challenges are there? DynamoDB is a multi-tenant system. What are the different challenges that come into picture that we have to solve? What are the lessons that we have learned to provide predictable performance? One of the common challenges in a multi-tenant system is workload isolation, because it’s not just one customer that we have, we have multiple customers. These customers, their partitions are installed on the storage nodes which are multi-tenant. If isolation is not done right, it can cause performance impact to these customers. Let’s jump into how exactly we solve that. In the original version of DynamoDB that was released in 2012, customers explicitly specified the throughput that the table required in terms of read capacity units and write capacity units. Combined, that is what is called as provisioned throughput of the table.
If a customer is essentially reading an item, which is up to 4 KB, that means it has consumed one read capacity unit. Similarly, if a customer is doing a write of a 1 KB item, that would mean the write capacity unit is consumed. Recall from the previous example for the customer’s table, we had three partitions. If a customer asks for 300 read capacity units in the original version of Dynamo, what we would do is we would assign 100 RCUs to each of the partitions. You have basically 300 RCUs in total for your table. Assuming that your workload is uniform, essentially your traffic is going to three different partitions at a uniform rate. To provide workload isolation, DynamoDB uses token bucket algorithm. Token bucket is to track the consumption of tokens of the capacity that that particular table has, and a partition has, and enforce basically a ceiling for that.
Looking at one of these partitions, we had token buckets at a partition level in the original version of Dynamo. Each second, essentially, we are refilling tokens, at the rate of the capacity assigned to the partition, which is the bucket in this particular case. When RCUs are used for read requests, we are continuously deducting them based on the consumption. If you do one request, and we basically consume one token from this bucket, the bucket is getting refilled at a constant rate. If the bucket is empty, obviously, we cannot accept the request and we ask customers to try again. Overall, let’s say that a customer is sending request, and if there are 100 tokens, the request will get accepted for the green partition. As soon as the consumed rate goes above 100 RCUs, in this particular example, as soon as it reaches 101 RCUs, your request will get rejected, because there are no tokens that are left in that token bucket. This is a high-level idea of how token buckets could work.
What we found out when we launched DynamoDB, is that uniform distribution for the workloads is very hard. Essentially getting uniform workloads across for the full duration of when your application is running, your table exists, it’s very hard for customers to achieve that. That’s because the traffic tends to come in waves or spikes. For example, let’s say you have an application which is for serving coffee. You suddenly will see that spike happening early in the morning, and then suddenly your traffic will increase. As most of the customers get the coffee they go to their office, what you would see is the traffic suddenly drops. Traffic is not uniform. It basically changes with time. Sometimes it is spiky, sometime there is not much traffic in the system. If you create a table with 100 RCUs, and you see a spike of traffic greater than 100 RCUs, then whatever is above 100 RCUs will get rejected. That’s what I mean by non-uniform traffic over time. Essentially, what’s happening is, maybe your traffic is getting distributed across all the partitions, or maybe it’s getting to a bunch of partitions, but it is not uniform across time. Which means if you have provisioned the table at 100 RCUs, any request that is being sent above the 100 RCU limit, it will all get rejected.
Another challenge that we saw was, customers solving this problem of seeing that they are getting throttled. To solve this problem of getting throttled, what they did was they started provisioning for the peak. Instead of doing 100 RCUs, they would ask for 500 RCUs, which means that it is able to handle the peak workload that they’ll see in the day, but at the same time for rest of the day, you are seeing a lot of waste in the system. This meant a lot of capacity unused and wasted, which incurred cost to the customers. Customers asked us, can you solve this problem? We said, what if we let the customers burst? What is the capacity of the bucket? To help accommodate the spike in the consumption, we launched bursting, where we allow customers to carry over their unused throughput in a rolling 5-minute window. It’s very similar to how you think about unused minutes in a cellular plan. You’re capped, but if you don’t use minutes in the last cycle, you can move them to the next one. That’s what we called as the burst bucket. Effectively, the increased capacity of the bucket was able to help customers absorb their spikes. This is the 2013 timeframe when we introduced bursting, unused provision capacity was banked to be used later. When you exercised those tokens, your tokens will be spent. Finally, that particular problem of non-uniform workload over time, we were able to solve.
We talked about the non-uniform distribution over time, let’s talk about non-uniform distribution over keys. Let’s say that you’re running a census application for Canada, and the data of the table is partitioned based on ZIP codes. You can see in this map, 50% of Canadians live below that line, and 50% of Canadians live north of that line. What you will see is that most of your data is essentially going in a bunch of partitions, which means your traffic on those partitions will be higher as compared to your traffic on some partitions. In this example, we have 250 RCUs going to the green partition, and 10 RCUs going to orange and pink partition. Overall, the partitions, they’re not seeing uniform traffic. The takeaway that we had from bursting and non-uniform distribution over space was that we had essentially tightly coupled how much capacity a partition will get to how physically we are basically landing these partitions. We had essentially coupled partition level capacity to admission control, and admission control was distributed and performed at a partition level. What that resulted in, just a pictorial picture of that, is you would see all the traffic go into a single partition. Then, since there is not enough capacity on that partition, the request will start getting rejected.
The key point to note here is that even though a customer table has enough capacity, for example, in this case, 300 RCUs, but that particular partition got only assigned 100 RCUs, so that’s why the requests are getting rejected. Customers were like, I have enough capacity on my table, why is my request getting rejected? This particular thing was called as throughput dilution. The next thing we had to do was solve throughput dilution. To solve throughput dilution, what we did was we launched global admission control. DynamoDB realized it would be going to be beneficial to remove the admission control from partition level and move it up to the request router layer and let all these partitions burst. Still have maximum capacity that a single partition can do for workload isolation, but move the token buckets from the partition to a global table level token bucket. In the new architecture, what happens is, we introduced a new service called as GAC, global admission control as a service. It’s built on the same idea of token buckets, but the GAC service centrally tracks the total consumption of table capacity, again, in terms of tokens. Each request router maintains a local token bucket to make sure the admission decisions are made independently and communicate with GAC to replenish the tokens at regular interval. GAC essentially maintains an ephemeral state computed on the fly from the client requests. Going back to the 300 RCU example, now customers could drive that much traffic to even a single partition because we moved the token bucket from the partition level to a global level, which is the table level bucket. With that, no more throughput dilution. A great win for customers.
That particular solution was amazing, so we had essentially launched bursting, and we did global admission control. It helped a lot of use cases in DynamoDB. Still, there were cases where a customer, due to maybe non-uniform distribution over time or space, could still run into scenarios where traffic to a specific partition is reaching its maximum. If a partition can do 3000 RCUs maximum, and a customer wants to do more on that partition, requests greater than 3000 RCUs would get rejected. We wanted to solve that problem as well. What we did was as the traffic increases on the partition, we actually split the partition. Instead of throttling the customer, we started doing automatic splits. The idea behind automatic splits was to identify the right midpoint, which will actually help to redistribute the traffic between two new partitions. If customers send more traffic to one of the partitions, we would again further split that into smaller partitions and route the traffic to the new partitions.
Now you have these partitions that are equally sized, or they’re balanced, essentially. You as a developer, did not have to do any single thing. AWS literally is adjusting the service to fit your custom needs on the specific usage pattern that you are generating for the service. All this magic happens to solve both the problems, even if you have non-uniform traffic over time, or non-uniform traffic over space. This is not something that we got right from day one. As more customers built on top of DynamoDB, we analyzed their traffic, understood the problems that they were facing, and solved these problems by introducing bursting, split for consumption, and global admission control as solutions for all these different problems. Going back to the picture, where if the customer is driving 3000 requests per second to the green partition, we would automatically split, identify where exactly is the right place to split that, and split it so that 1500 RCUs, 1500 RCUs, the traffic splits between the two. This was again, amazing. We essentially did a bunch of heavy lifting on behalf of the customers.
One thing we still were hearing from customers that, DynamoDB has figured out a lot of things for us, now, for us coming from the world where we always have been thinking about servers, now you’ve started asking us to think in terms of read capacity units, write capacity units. Can you further simplify that? Instead of asking customers to specify provisioning at the time of table creation, what we did was we launched on-demand, where you don’t even have to specify that. All the innovations that we did around bursting, split for consumption, global admission control, all of those enabled us to launch something which is basically on-demand mode on your tables where you just create a table and start sending requests, and you pay per request for that particular table.
Key Lesson
The key lesson here is that designing the system which adapts to the customer traffic pattern is the best experience that you can provide to the customer while using the database, and DynamoDB strives for that. We did not get this right in the first place. We launched with the assumption that traffic will be uniformly distributed but realized there are actually non-uniform traffic distribution based on time and space. Then, analyzing those problems, making educated guesses, we evolved the service and solved all these problems so that all the heavy lifting, all the essential complexity is moved away from the customer into the service, and customers, they just get a magical experience.
How Does DynamoDB Provide High Availability?
DynamoDB provides high availability. Let’s look at how DynamoDB does that. DynamoDB has evolved, and a lot of customers have moved their mission critical workloads into DynamoDB. AWS, there are service disruptions that happen. 2015, DynamoDB also had a service disruption. As I said in the beginning, whenever there is a service disruption that happens, we try to learn from them. The goal is to make sure that the impact that we saw doesn’t repeat. We want to make sure the system weaknesses are actually fixed, and we have a more highly available service. When this issue happened, one of the learnings that we had from that particular COE was that we identified a weak link in the system. That link was related to caches. These caches are essentially the metadata caches that we had in the DynamoDB system. One thing about caches is that the caches are bimodal. There are essentially two routes that a cache code can take. One is, when there is a cache hit, your requests are served from the cache. In the case of metadata, all the metadata that request routers wanted, it was being served from the cache. Then, you have a cache miss case where all the requests actually go back to the database. That’s what I meant by the bimodal nature of the caches. Bimodality in a distributed system is a volcano waiting to erupt. Why do I say that?
This is going back to our PutItem request, so whenever a customer made a request to DynamoDB to put an item or get an item, the request router is the first service where that request lands. Request router has to find out where to route the request. What are the storage nodes for that particular customer table and partition, so it will hit a metadata service? To optimize that, DynamoDB also had a partition map cache in the request routers. The idea is that since the partition metadata doesn’t change that often, it’s a highly cacheable workload. DynamoDB actually had about 99.75% cache hit ratio from these caches, which are on the request router. Whenever a request lands on a brand-new request router, it has to go and find out the metadata. Instead of just asking the metadata for a specific partition, it would ask the metadata for the full table, assuming that next time customer makes a request for a different partition, it already has that information. Maximum, 64 MB requests you can get.
We don’t have just one request router, as I said, we have multiple request routers. If a customer creates a table with millions of partitions, and start sending that many requests, they’ll probably hit not just one request router, they’ll hit multiple request routers. All those requests, then request routers would start asking about the same metadata, which means you have essentially reached a system state where you have a big fleet talking to a small fleet. The fundamental problem is that, either there is nothing in the caches, that is, the cache hit ratio is zero, you have a big fleet driving so many requests to a small fleet, and a sudden spike in traffic. In steady state it was 0.25%, if the cache hit ratio becomes 0, caches become ineffective. Traffic jumps to 100%, which means 400x increase in traffic, and that would further lead to cascading failures in the system. The thing that we wanted to solve is remove this weak link from the system so that the system can always operate in a stable manner.
How did we do that? We did two things. One is, as I said previously, in the first version of DynamoDB, request router, whenever it finds out that there is no information about the partition which it wants to talk to, it would load the full partition map for the table. First change it did was, instead of asking for the full partition map, just ask for that particular partition which you’re interested in. That was the one change that we did then, which was a simpler change. We were able to do it faster. Then we also, secondly, built an in-memory distributed datastore called MemDS. MemDS stores all the metadata in-memory. Think of it like an L2 cache. All the data is stored in a highly compressed manner. The MemDS processes on a node encapsulates essentially a Perkle data structure. It can answer questions like, for this particular key, which particular partition it lands into, so that MemDS can respond back to the request router, the information, and then request router can route the request to the corresponding storage node.
We still do not want to impact performance. We do not want to make an off-box call for every request that customer is making, so we still want to cache results. We introduced a new cache, which is called the MemDS cache on these request routers. One thing, which is different and critical, the most important thing which we did different here on these caches is that even though there is a cache hit on the MemDS cache, we would still send all the traffic to the MemDS system asking for the information, even though we have a cache hit on these MemDS nodes. What that is doing is essentially the system is generating always constant load to the MemDS system, so there is not a case where suddenly caches become ineffective, your traffic suddenly rises. It is always acting like the caches are ineffective. That’s how, essentially, we solve the weak link in the system of metadata getting impacted by requests landing from multiple request routers onto the metadata nodes. Overall, the lesson here is that designing systems for predictability over absolute efficiency improves the stability of a system. While system like caches can improve performance, but do not allow them to hide the work that would be performed in their absence, ensuring that your system is always provisioned to handle the unexpected load that can happen when the cache has become ineffective.
Conclusions and Key Takeaways
We talked about the first and second conclusion. The first one being adapting to customer traffic patterns improve their experience. We looked at it with how different problems we solved by introducing global admission control, bursting, and finally on-demand. Second, designing systems of predictability over absolute efficiency improve system stability. That’s the one we just saw with caches. Caches are bimodal. How it’s important to make sure that system is doing predictable load. The failure scenarios are tamed by making sure that your system is provisioned for the maximum load that you have to do. Third and fourth, these are two more things that we talk about in much detail in the paper. The third being DynamoDB is a distributed system. We have multiple storage nodes, multiple request routers. Performing continuous verification of data at rest is necessary. That’s the best way we have figured out to ensure we meet high durability goals. Last, maintaining high availability as system evolves, it might mean that you have to touch the most complex part of your system. In DynamoDB, one of the most complicated most complex part is the Paxos protocol, the Multi-Paxos protocol. To improve availability, we had to do some changes in that protocol layer. The reason we were able to do those changes easily was because we had formal proof of these algorithms that are being written there, that were written there right from the original days of DynamoDB. That gave us quite high confidence since we had a proof of the working system, we could tweak it and make sure that the new system still ensures correctness, all the invariants are met.
Questions and Answers
Anand: What storage system does metadata storage use?
Vig: DynamoDB uses DynamoDB for its storage needs. Just think about it, the software that is running on the storage nodes, that’s the same software which is running on the metadata nodes as well. As we have evolved, as I talked about MemDS being introduced, basically think of it like an L2 cache on top of the metadata that is being used to serve, like partition metadata for all the learnings that we had and scaling bottlenecks with the metadata.
Anand: You’re saying it’s just another Dynamo system, or it’s a storage system? Does the metadata service come with its own request router and storage tiers?
Vig: No, it’s just the storage node software. Request router, basically has a client, which talks to the storage nodes. It uses the same client to talk to the metadata nodes so that whenever the request comes, it’s exactly the same code that we run for a production customer who would be accessing DynamoDB.
Anand: Is MemDS eventually consistent? How does it change to a cached value addition of a new value of 1 MDS node get replicated to other nodes?
Vig: Think of MemDS like you have an L2 cache, and that cache is updated, not a write through cache, but a write around cache. Whenever a write happens on the metadata fleet, which is very low throughput, partitions, they don’t change often. What happens is, whenever a write happens on the partition table in the metadata store, those writes through streams, they are basically consumed by a central system, which sends all the MemDS nodes to have the updated values. This whole process happens within milliseconds again. As the change comes in, it just gets replicated on all the boxes. The guy which is in the center is responsible for making sure all the MemDS nodes are getting the latest values. Until it is acknowledged by all the nodes, that system doesn’t move forward.
Anand: Do you employ partitioning in master-slave models there, or are there agreement protocols? Does every MemDS have all of the data?
Vig: MemDS has all the metadata. It’s basically vertically scaled. Because the partition metadata, as I said, is not that big, it’s tiny. The main thing there is the throughput that we have to support. That’s what is very high. That’s why we just keep on adding more read replicas there. It’s not the leader-follower configuration. It’s like every node is eventually consistent. It’s a cache. They are scaled for specifically the reads that that metadata requests have to be served for whenever customers are sending requests, or any other system which wants to access the partition metadata.
Anand: There’s some coordinator that’s in the MemDS system, requests are send with a new write request, that coordinator is responsible for ensuring that all of the entire fleet gets that data.
Vig: Exactly.
Anand: It’s the coordinator’s job, it’s not each peer-to-peer gossip type protocol to link. That coordinator is responsible for durable state and consistent state. Each node, once they get it, they’re durable, but that’s just for consistent state transfers.
Vig: Yes. Durable in the sense it’s a cache, so when it crashes, it will just ask from the other node in the system to get up to speed and then start serving requests.
Anand: That coordinator, how do you make that reliable?
Vig: All these writes are idempotent. You could have any number of these guys running in the system at any time, and writing to the destination. Since the writes are idempotent, they always monotonically increase. It never goes back. If a partition has changed from P1 to P2, if you write again, it will say, “I have the latest information. I don’t need this anymore.” We don’t need a coordinator. We try to avoid that.
Anand: You don’t have a coordinator service that’s writing to these nodes?
Vig: We don’t have the leader and follower configuration there.
Anand: You do have a coordinator that all requests go to, and that keeps track of the monotonicity of the writes, like a write ahead log. It’s got some distributed, reliable write ahead log. It’s just somehow sending it.
When the partition grows and splits, how do you ensure the metadata of MemDS cache layers get updated consistently?
Vig: It’s eventually consistent. The workflows that are actually doing partition splits and all these things, they wait until MemDS is updated before flipping the information there. The other thing is, even if the data is not there in MemDS, storage nodes also have the protocol to respond back to the request router saying that, “I am updated. I don’t host this partition anymore, but this is the hint that I have, so maybe you can go talk to that guy.” For the edge cases where this information might get delayed, we still have mechanisms built in the protocol to update the coordinator.
Anand: Each partition also has some information of who else has this data?
Vig: MemDS node, yes.
Anand: Also, it’s a little easier because, unlike DynamoDB, this can be eventually consistent. DynamoDB, people care about consistency over availability and other things. Essentially, we’re saying that, when you split, it doesn’t have to be replicated everywhere immediately. It’s done as time.
See more presentations with transcripts