Month: June 2023
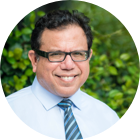
MMS • RSS
Posted on mongodb google news. Visit mongodb google news
The launch of OpenAI’s next-generation chatbot, ChatGPT, in November 2022 has proved to be a golden moment for artificial intelligence (AI) stocks. While the current investor interest in all things AI may seem to be bordering close to frenzy, it is not completely without substance.
According to Ark Investment Management’s Big Ideas 2023, AI software could generate up to $90 trillion in enterprise value in 2030. Given that AI is all set to be the next big thing for the coming decade, long-term investors may benefit by picking these three stocks now.
1. MongoDB
The first AI stock to buy now is cloud-native database specialist MongoDB (MDB -0.04%). The company’s document database continues to benefit from the increasing use of non-relational databases in various applications across industries.
Additionally, the increasing adoption of AI in our daily lives, coupled with more AI-driven coding tools for developers, is expected to dramatically boost the development and deployment of new software applications. Since many of these applications rely on large amounts of unstructured data stored across disparate sources, MongoDB’s scalable and distributed database design, combined with easy querying capabilities, will benefit from these tailwinds.
MongoDB has delivered an impressive performance in the first quarter of fiscal 2024 (ended April 30, 2023), with revenue and earnings crushing consensus analyst expectations. Revenue rose year over year by 29% to $368.3 million, while adjusted net income almost tripled to $45.3 million in the same time frame. Although not yet generally accepted accounting principles (GAAP) profitable, the company is gradually inching toward that goal.
MongoDB’s cloud-based subscription offering, Atlas (database-as-a-service), is the fastest-growing part of the company. In Q1, Atlas’ revenue was up 40% year over year and accounted for 65% of the company’s total sales. Atlas ended Q1 with 41,600 customers, up from 33,700 in the same quarter of the previous year. Despite the difficult macros, Atlas consumption trends were better than expected.
MongoDB is definitely not cheap at 17.7 times next year’s sales. However, considering the company’s rapidly improving financial metrics, robust consumption trend for Atlas, and AI tailwinds, the premium valuation seems justified.
2. Splunk
The second AI stock to buy now is machine learning (ML, a subfield of AI) software player Splunk (SPLK 0.04%). Long before AI was a buzzword, this leading security and observability company was deploying advanced ML models to ingest and analyze huge amounts of machine data to derive actionable insights. The company helps clients in areas such as information technology operations, search, analytics, and security. Splunk enables real-time performance monitoring and analysis of enterprise infrastructure and applications, as well as helps mitigate cyber risk by detecting, investigating, and responding to cyber threats rapidly.
Splunk delivered blowout results in the first quarter of fiscal 2024 (ended April 30, 2023), with revenue and earnings handily beating consensus estimates. Revenue jumped 11% year over year to $752 million, while cloud revenue grew at a much faster pace of 30% year over year to $419 million. Customers spending at least $1 million annually on Splunk’s cloud-based products grew year over year by 32% to 433, while total customers spending $1 million annually on the company’s products (cloud and on-premises) were up by nearly 17% year over year to 810.
On the other hand, operating expenses were down by 2% year over year in the first quarter. These numbers are impressive, considering that customers continued to delay cloud spending in the first quarter. Improving revenue mix toward higher-margin cloud business and optimal expense management can further push up profit margins in the coming quarters.
Additionally, Splunk reported a free cash flow of $486 million in Q1, up 253% on a year-over-year basis. Thanks to the solid business momentum, the company revised its revenue and free cash flow guidance higher for fiscal 2024.
Splunk estimates its total addressable market to be more than $100 billion. Considering the company’s solid financials and an annual run rate of only $3.7 billion, there is much scope for future growth for this ML stock.
3. Pinterest
The third AI stock to pick now is social media company Pinterest (PINS -0.29%). The company’s entire business model depends on the use of powerful AI algorithms for visual search (searching for images using other images instead of text) and for making personalized recommendations based on patterns associated with past user activity and the relationships between saved pins.
Thanks to an in-depth understanding of its user base, Pinterest has also been quite useful for marketers in crafting effective and targeted advertising strategies. Since users come to the platform in search of new ideas and with shopping intent, the relevant advertisements do not feel like an intrusion and instead help improve the overall user experience.
After struggling for the past few quarters, new CEO Bill Ready seems to have finally brought Pinterest back into growth mode. In the recent quarter (Q1 fiscal 2023 ended March 31, 2023), the company’s revenue climbed 5% year over year to $603 million, ahead of the consensus estimate of $592.6 million. The company also saw a 7% year-over-year increase in the number of monthly active users (MAUs) to 463 million.
Pinterest is now focusing on improving engagement and monetization per user by increasing overall actionability on the platform. To do that, Pinterest is increasing shopping advertisements and engagement on shoppable pins. The company has also partnered with Amazon to bring more products and an improved consumer buying experience to the platform. And the company recently introduced mobile deep linking to redirect users to the advertiser’s website.
Pinterest is not yet a profitable company. However, with management focusing on increasing average revenue per user, share prices may also grow rapidly in the coming quarters.
John Mackey, former CEO of Whole Foods Market, an Amazon subsidiary, is a member of The Motley Fool’s board of directors. Manali Bhade has no position in any of the stocks mentioned. The Motley Fool has positions in and recommends Amazon.com, MongoDB, Pinterest, and Splunk. The Motley Fool has a disclosure policy.
Article originally posted on mongodb google news. Visit mongodb google news
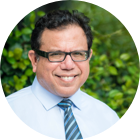
MMS • RSS
Posted on mongodb google news. Visit mongodb google news
This Bull Market Indicator Hasn’t Been Wrong in 67 Years, and It Has a Clear Message for Where Stocks Head Next
Social Security Has an Income Inequality Problem — and It’s Getting Progressively Worse
Where to Invest $5,000 for the Next 5 Years
Statistically Speaking, Nearly 50% of Future Retirees May Face an Unpleasant Social Security Surprise
Article originally posted on mongodb google news. Visit mongodb google news
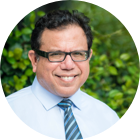
MMS • Matt Campbell
Article originally posted on InfoQ. Visit InfoQ
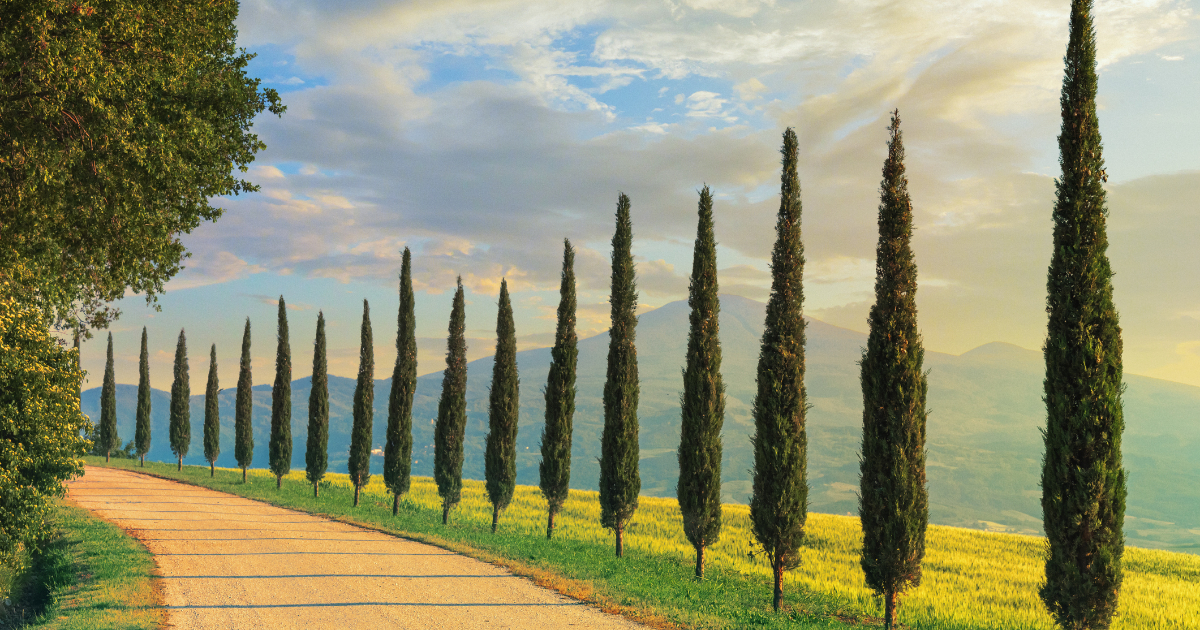
AWS has open-sourced Cedar, their language for defining access permissions using policies. Cedar is integrated within both Amazon Verified Permissions and AWS Verified Access. Cedar can also be integrated directly into an application via the provided SDK and language specification.
Cedar allows for expressing policies separate from the application code. This decoupling enables them to be independently authored, analyzed, and audited. Cedar supports role-based access control (RBAC) and attribute-based access control (ABAC) approaches.
The SDK can be used for authoring and validating policies as well as authorizing access requests. Cedar is written in Rust but also has both a Rust crate and a Java package to allow for using Cedar from Java.
Validating that a request is authorized can be done by invoking the Cedar authorization engine. The request information is translated into a Cedar request and passed into the Cedar authorization engine. The following example demonstrates this in Rust:
pub fn is_authorized(
&self,
principal: impl AsRef,
action: impl AsRef,
resource: impl AsRef,
) -> Result {
let es = self.entities.as_entities();
let q = Request::new(
Some(principal.as_ref().clone().into()),
Some(action.as_ref().clone().into()),
Some(resource.as_ref().clone().into()),
Context::empty(),
);
info!(
"is_authorized request: principal: {}, action: {}, resource: {}",
principal.as_ref(),
action.as_ref(),
resource.as_ref()
);
let response = self.authorizer.is_authorized(&q, &self.policies, &es);
info!("Auth response: {:?}", response);
match response.decision() {
Decision::Allow => Ok(()),
Decision::Deny => Err(Error::AuthDenied(response.diagnostics().clone())),
}
}
The Cedar authorization engine is invoked via the call self.authorizer.is_authorized(&q, &self.policies, &es)
. The arguments to the call include the access request, Cedar policies, and the entity set. The access request contains the principal, action, and resource information needed to confirm if the request is permitted. Based on the analysis, the call will return either Decision::Allow
or Decision::Deny
.
Policies can be created via the SDK as well. The following Java example creates a policy that permits the principal Alice to perform the action View_Photo on any resource that is a child of the Vacation resource:
private Set buildPolicySlice() {
Set ps = new HashSet();
String fullPolicy = "permit(principal == User::"Alice", action == Action::"View_Photo", resource in Album::"Vacation");";
ps.add(new Policy(fullPolicy, "p1"));
return ps;
}
In Java, a query can be performed using the isAuthorized method:
public boolean sampleMethod() throws AuthException {
AuthorizationEngine ae = new WrapperAuthorizationEngine();
AuthorizationQuery q = new AuthorizationQuery("User::"Alice"", "Action::"View_Photo"", "Photo::"pic01"");
return ae.isAuthorized(q, buildSlice()).isAllowed();
}
Permit.io followed the AWS announcement with the release of Cedar-Agent, an HTTP server that acts as a policy store and data store for Cedar-based policies. The store allows for creating, retrieving, updating, and deleting policies. The data store allows for in-memory storage of the application’s data. It integrates with Cedar-Agent to allow for authorization checks to be performed on the stored data. Authorization checks are performed on incoming HTTP requests.
User dadadad100, on a HackerNews post, commented that they saw Cedar potentially filling a gap in the application authorization space:
Cedar falls somewhere between OPA with its datalog (prolog) based search approach and a Zanzibar based approach. It’s not clear which direction will win out, but it is time that this problem got some attention.
Other users, such as Oxbadcafebee, expressed frustration that AWS didn’t lend their support to Open Policy Agent instead.
Cedar is open-source under Apache License 2.0 and is available via GitHub. More details can be found in the recent AWS blog and by joining the Cedar Policy Slack workspace.
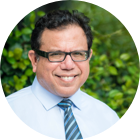
MMS • A N M Bazlur Rahman
Article originally posted on InfoQ. Visit InfoQ
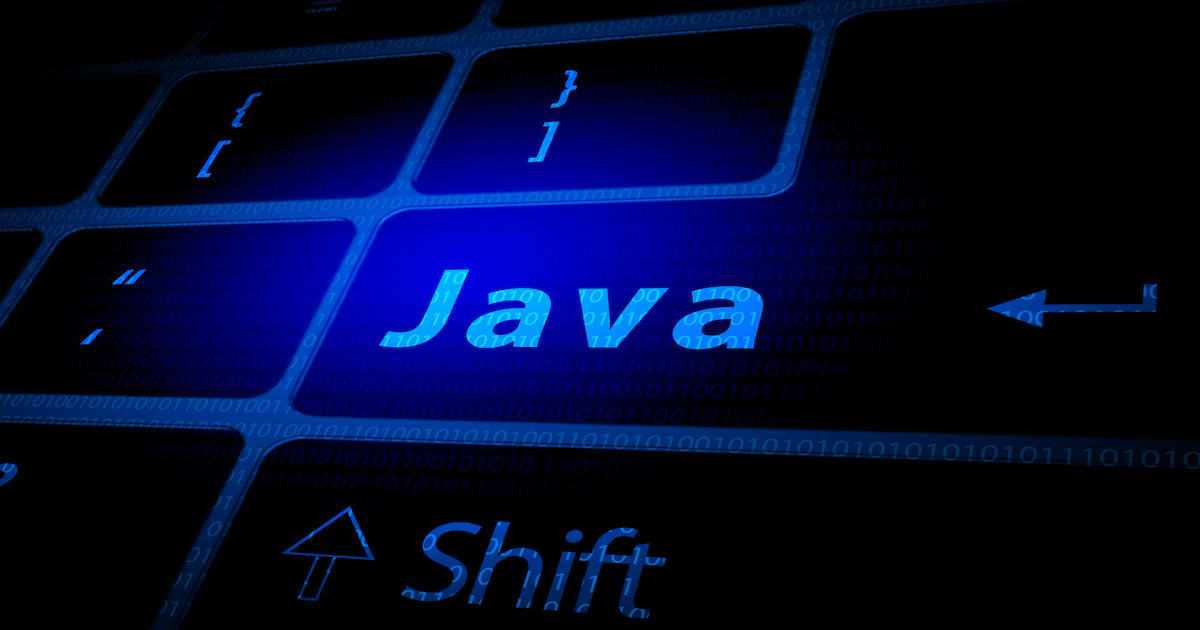
JEP 443, Unnamed Patterns and Variables (Preview), has been Completed from Targeted status for JDK 21. This preview JEP proposes to “enhance the language with unnamed patterns, which match a record component without stating the component’s name or type, and unnamed variables, which can be initialized but not used.” Both of these are denoted by the underscore character, as in r instanceof _(int x, int y)
and r instanceof _
. This is a preview language feature.
Unnamed patterns are designed to streamline data processing, particularly when working with record classes. They allow developers to elide the type and name of a record component in pattern matching, which can significantly improve code readability. For example, consider the following code snippet:
if (r instanceof ColoredPoint(Point p, Color c)) {
// ...
}
In this instance, if the Color c
component is not needed in the if block, it can be laborious and unclear to include it in the pattern. With JEP 443, developers can simply omit unnecessary components, resulting in cleaner, more readable code:
if (r instanceof ColoredPoint(Point p, _)) {
// ...
}
Unnamed variables are useful in scenarios where a variable must be declared, but its value is not used. This is common in loops, try-with-resources statements, catch blocks, and lambda expressions. For instance, consider the following loop:
for (Order order : orders) {
if (total < limit) total++;
}
In this case, the order
variable is not used within the loop. With JEP 443, developers can replace the unused variable with an underscore, making the code more concise and clear:
for (_ : orders) {
if (total < limit) total++;
}
Unnamed patterns and variables are a preview feature, disabled by default. To use it, developers must enable the preview feature to compile this code, as shown in the following command:
javac --release 21 --enable-preview Main.java
The same flag is also required to run the program:
java --enable-preview Main
However, one can directly run this using the source code launcher. In that case, the command line would be:
java --source 21 --enable-preview Main.java
The jshell option is also available but requires enabling the preview feature as well:
jshell --enable-preview
Let’s look at a few more advanced use cases of unnamed patterns and variables introduced in JEP 443:
Unnamed patterns can be particularly useful in nested pattern-matching scenarios where only some components of a record class are required. For example, consider a record class ColoredPoint
that contains a Point
and a Color
. If you only need the x
coordinate of the Point
, you can use an unnamed pattern to omit the y
and Color
components:
if (r instanceof ColoredPoint(Point(int x, _), _)) {
// ...
}
Unnamed pattern variables can be beneficial in switch statements where the same action is executed for multiple cases, and the variables are not used. For example:
switch (b) {
case Box(RedBall _), Box(BlueBall _) -> processBox(b);
case Box(GreenBall _) -> stopProcessing();
case Box(_) -> pickAnotherBox();
}
In this example, the first two cases use unnamed pattern variables because their right-hand sides do not use the box’s component. The third case uses the unnamed pattern to match a box with a null component.
Unnamed variables can be used in lambda expressions where the parameter is irrelevant. For example, in the following code, the lambda parameter v
is not used, so its name is irrelevant:
stream.collect(Collectors.toMap(String::toUpperCase, _ -> "No Data"));
In try-with-resources statements, a resource represents the context in which the code of the try block executes. If the code does not use the context directly, the name of the resource variable is irrelevant. For example:
try (var _ = ScopedContext.acquire()) {
// No use of acquired resource
}
Unnamed variables can be used in catch blocks where the name of the exception parameter is irrelevant. For example:
try {
int i = Integer.parseInt(s);
} catch (NumberFormatException _) {
System.out.println("Bad number: " + s);
}
Notably, the underscore character was previously valid as an identifier in Java 10. However, since Java 8, the use of underscore as an identifier has been discouraged, and it was turned into a compile-time error in Java 9. Therefore, it is assumed that a minimal amount of existing and actively maintained code uses underscore as a variable name. In instances where such code does exist, it will need to be modified to avoid using an underscore as a variable name.
Given this, JEP 443 is a significant step towards making Java code more readable and maintainable. This is particularly beneficial in complex data structures where the shape of the structure is just as important as the individual data items within it. By allowing developers to omit unnecessary components and variables, it reduces code clutter and makes the code easier to understand. As developers gain more experience with this feature, it is expected to become an integral part of Java programming.
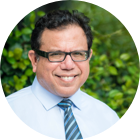
MMS • RSS
Posted on mongodb google news. Visit mongodb google news
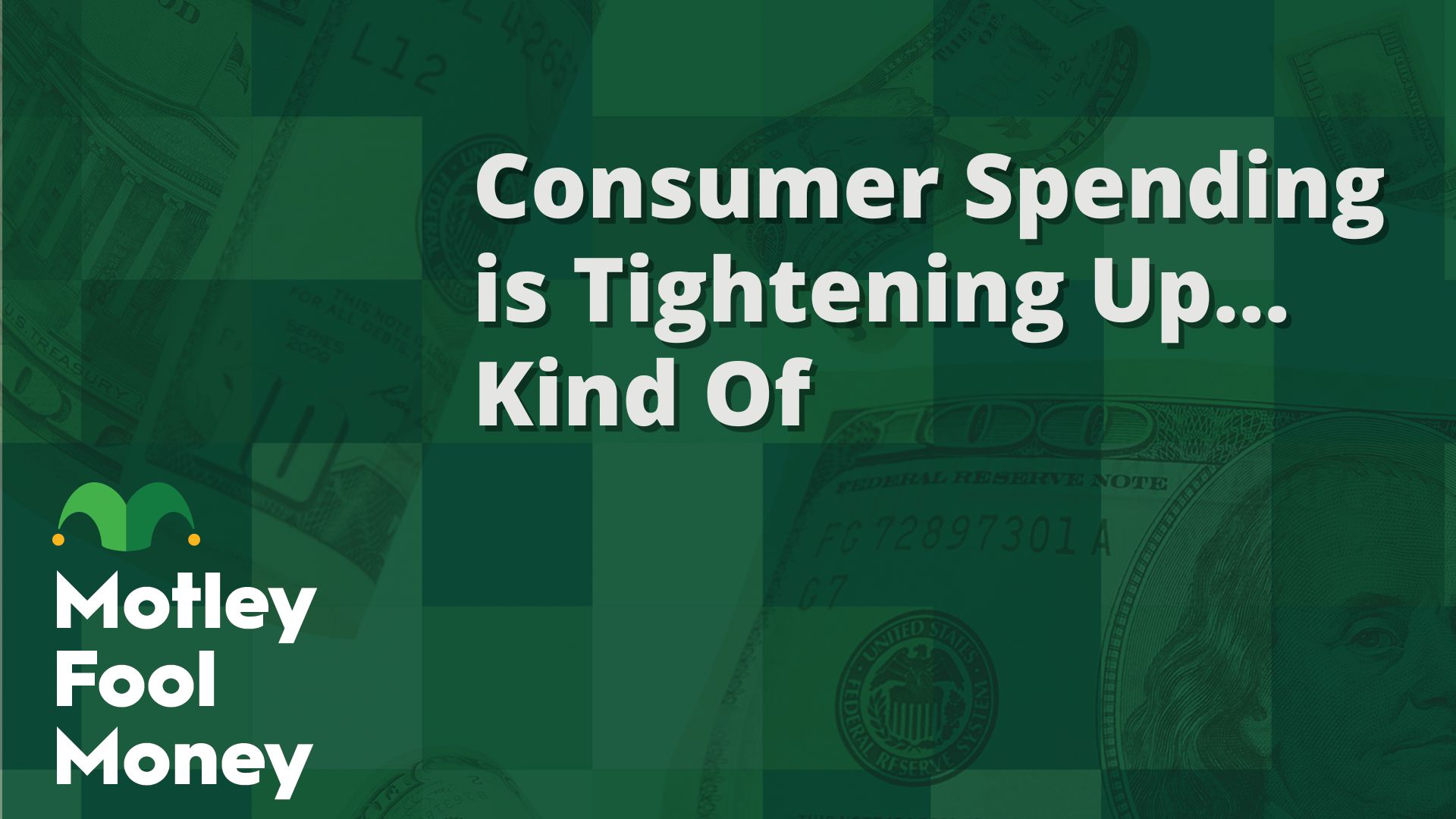
In this podcast, Motley Fool senior analysts Ron Gross and Emily Flippen discuss:
- The debt ceiling resolution, and updates on employment and consumer debt.
- Why Chewy and Five Below continue to report strong results while other retailers are seeing consumer spending slow down.
- The numbers behind MongoDB‘s blowout earnings release.
- Lululemon‘s strong earnings report.
- Two stocks on their radar: Compass Minerals and Veeva Systems.
In addition, The Motley Fool’s Scott Kassing talks through three high-conviction stock ideas with Sandhill Investment Management’s Richard Ryskalczyk.
To catch full episodes of all The Motley Fool’s free podcasts, check out our podcast center. To get started investing, check out our quick-start guide to investing in stocks. A full transcript follows the video.
video-js.video-js.vjs-fluid:not(.vjs-audio-only-mode) {padding-top: 56.25%;}
10 stocks we like better than Chewy
When our analyst team has a stock tip, it can pay to listen. After all, the newsletter they have run for over a decade, Motley Fool Stock Advisor, has tripled the market.*
They just revealed what they believe are the ten best stocks for investors to buy right now… and Chewy wasn’t one of them! That’s right — they think these 10 stocks are even better buys.
*Stock Advisor returns as of May 30, 2023
This video was recorded on June 02, 2023.
Dylan Lewis: We’ve got plenty of news on the big picture and where consumer spend is and isn’t going. You’re listening to Motley Fool Money.
It’s the Motley Fool Money radio show. I’m Dylan Lewis, joining me in studio, Motley Fool senior analysts Emily Flippen and Ron Gross. Great to have you both here.
Ron Gross: How are you doing, Dylan?
Emily Flippen: Hey.
Dylan Lewis: We’ve got stock ideas and some earning surprises, but we’re kicking off with the big macro, got the debt ceiling deal, a fresh jobs report, and an update on consumer debt. Ron, where do you want to start?
Ron Gross: Let’s go to the debt ceiling because I think it’s on everyone’s mind, and I’ll say phew. Phew. How about that? Yeah, we got it done. I honestly didn’t think it was in jeopardy of not getting done, although there were a few days here and there, where it seemed like our politicians might not be able to come together. But it was literally a necessity. When something is literally a necessity, I think it’s fair to bet on that happening. It was a compromise, as most things in politics are, that’s fine. Some give-and-take. We live to fight another day whether there should be a debt ceiling or not, it’s a political question, not really an investing one so I will leave that for other pundits. But I personally, I’m happy it got done. Friday’s stock market is strong. I would think partly as a result of that being accomplished.
Dylan Lewis: I’m going to speak for the market and investors everywhere and say, I’m just happy that the government will have the ability to pay its bills for the foreseeable future. Emily, apart from that, anything you’d add to the debt ceiling?
Emily Flippen: I love this game of chicken we play with our financial systems. Lots of fun for investors in the market, but in all seriousness, we do get some interesting developments out of these occasionally. I think we saw one today with the debt deal, the deal that raise the debt ceiling actually cut IRS funding that was granted as part of the Inflation Reduction Act by upwards of $20 million. It was an $80 billion deal. That could impact the development of a free federal tax filing system, $20 billion, excuse me. So cutting that by $20 billion, maybe the IRS doesn’t come in, doesn’t develop their own tax system which had been previously a priority of that government agency, which could be a net positive for some of the free tax filers that already exist out there, like Intuit‘s TurboTax. Just seeing where the federal government spending is going to shift potentially over the next couple of years, that’s one of the things that was standing out to me.
Dylan Lewis: Sticking with the big picture, we’ve also got a fresh jobs report Friday morning. U.S. employers added a seasonally adjusted 339,000 jobs last month, and unemployment currently sits at 3.7%, up slightly from recent months. Ron, what stood out to you in the report?
Ron Gross: This one is interesting to me because the jobs report came in hotter or better than expected. But there was a mixed bag because unemployment did tick up to 3.7%. Now that’s due to a sharp decline in self-employed data, people who identify as self-employed. That’s interesting a little bit in and of itself. But interestingly, the markets reacted positively to the strong jobs report. Now that is counterintuitive to the usual counterintuitiveness. It’s the same as double negatives make a positive. Usually, you would expect the market to sell off on a strong report because that gives the Fed cover to continue to raise rates if they feel they need to. Strong labor market leads to higher wages, wage inflation that keeps inflation persisting. But just when you thought you had these things figured out, the market goes ahead and pulls a 180 on you, trades higher, maybe it’s the debt ceiling, or maybe it’s that some of Friday’s data did show a moderating in wage inflation, and that is good news. Maybe not for the person earning the wage, but for the economy as a whole and what the Fed is trying to do to slow the economy. But we had a very strong Friday on this hot jobs data.
Emily Flippen: I will say this, I think part of it could also be the presumption that the federal government is not going to raise rates despite what a strong jobs report this was. I know that sounds counterintuitive because the Federal Reserve has said time and time again, no, we’re going to do what’s best for the economy regardless of how the market reacts, regardless of how other pundits react, we’re going to do what we think is best, but I think part of their job is managing expectations. So many consumers, Americans, investors, and the market, they’ve already baked into their expectations. No near-term rate increases. Part of me wonders if they’re taking that for granted and expecting things to stay the same even if the jobs report is strong.
Dylan Lewis: We generally don’t factor the interest rate movements too much into our individual investment decisions. Is there anything in here, Ron, that you would look at and say, this is what I expect. I know the jobs report can be a bit of a gamble as we look at it. But is there anything that you would say, yeah, this is where I’m leaning with where the Fed might be going.
Ron Gross: Well, I think the economy looks strong enough right now where I don’t expect a recession to be imminent. The big R word keeps rearing its ugly head as the Fed continues to raise. I’m not necessarily sure the Fed is actually done raising completely, maybe there’ll be a pause, maybe we will get 25 basis points here or there, or maybe the economy will slow as a result in 2024, but I don’t see an imminent. I think we’re going to be relatively OK, even if a recession happens, I think it will be shallow. At least that’s the way the numbers seem to be shaping up right now.
Dylan Lewis: One more data point that might have gotten lost a bit in the shuffle this week, consumer credit and revolving debt continues to rise and is nearing $1 trillion, according to the Fed. This has been an interesting metric to follow over the past few years. It is currently clocking in at all-time highs, Emily, up 25% since 2021. Does that have you concerned at all?
Emily Flippen: Well, it doesn’t have me shocked. That’s for sure we’ve seen record-high inflation, we’ve seen a pandemic, we’ve seen just the general lives, of I think, average Americans be really negatively impacted by the cost of living. So, I’m not surprised to hear that a number of those Americans are increasingly putting necessities on their credit card bill, which is what I think this probably is, people, trying to pay for things like groceries or phone bills, stuff that is no longer discretionary but necessary. It’s good to see the economy somewhat being strong, hopefully, that number comes down, but with inflation the way that it is, it’s not a surprise to see credit card debt rise.
Ron Gross: Let’s be careful when we say consumers are strong because if it’s at the expense of their savings or at the expense of a higher credit card bill, that’s really not the definition of strong to me. I think we can talk about this a little bit later, we’re starting to see that show up in some of the retailers that focus on lower-income shoppers. The Fed appears to be maybe getting what it wants, which is a slowing down of the economy and spending, but consumers maybe making up for that by dipping into savings or debt.
Dylan Lewis: Let’s zoom into that retail space a little bit and start examining that because I think as we start to see consumers get stretched, you wonder where dollars start to go. We looked at some results like those from Macy’s this past week. Really a seemingly solid quarter meeting expectations from this business, but it cut the forecast for the top and bottom line for the rest of the year. Reading through the call, Ron, it seems like they are being cautious as they look out and a lot of retailers are as they’re planning the rest of the year.
Ron Gross: They are, and it depends on where you’re focused. Macy’s is seeing weakness in discretionary categories, as I think will be a theme as we go across, whether it’s TVs at Target or some discretionary items at Macy’s. That’s where they’re being cautious, appropriately so. This stock is still off 40% from its 52-week high, so even though perhaps some of this data from this quarter met expectations, it’s still relatively weak with sales down 7%, brick-and-mortar down 6%, digital sales down 8%. There’s some real weaknesses continuing in this business. You did have a slight increase in gross margins, but that was offset by higher operating expenses. It all boils down to earnings per share being down almost 50%. This is a weak business currently in this environment causing them to lower guidance. Again, demand trends weakening further in discretionary categories is what the CEO said. They’re going to have to continue to take markdowns, they’re going to have to continue to adjust product mix, deal with high inventory levels, and hopefully have it worked through the system. They maintained their dividend, 4.8%, it’s actually trading 4 times updated earnings. If you want to take a shot on a retailer that’s been around for quite some time, getting their act together, that could be interesting, but things currently are weak.
Dylan Lewis: You mentioned management’s comments. They said something to the effect of consumers are pulling back in retail spend, and reallocating to food, essentials, and services. As we look to earnings from other companies, looking at Chewy here, pet supplier, it seems like Emily, people are still very comfortable spending on their pets.
Emily Flippen: I have a bone to pick with you, Dylan. Always seem to want to give credit to Chewy’s success on factors outside of their control. Like you just said, oh, well pet spend is up, so Chewy is doing well. But this quarter, the past couple of weeks have been a great example for why that is exactly not the case because you can look at Petco. Petco reported earnings, I believe last week and the market was extremely disappointed because of management’s commentary around the fact that consumers aren’t making this discretionary spend, their margins are weak. The economic environment is getting worse and meanwhile Chewy comes out and it’s almost the exact opposite story. Maybe the discretionary spending has fallen, but they’re saying, look, people aren’t trading down on their pets. They’re still buying the premium items that have slightly higher margins. The premium foods, the premium toys, because that’s how much they care about their animals, and that’s a deep relationship that Chewy has with their customers is that they understand the value of Chewy’s platform in a way that I think Petco doesn’t quite understand. Chewy knows. Look, I have a cat. I also recently bought a house. Do you know how many ads I’m getting from Chewy about cat towers? I have like three cat towers coming to me in the mail. Petco doesn’t know that about me, despite the fact that I can shop at a Petco and I have bought food at Petco before. Chewy just has a better understanding of its customer than other pet retailers. That’s what we saw showing up in this quarter. Just incredible results, some expansion of margins despite the fact that the economic environment is weaker.
Dylan Lewis: One of the things I wanted to dig into was that relationship with customers. I think one of the ways that it shows up in the results we saw from Chewy was those Autoship sales, those were up 19% year over year, outpacing overall revenue growth for the business. I have to think if you’re a retailer, having that type of relationship with your customer has to be the dream.
Emily Flippen: Yeah. The relationship is great, I will say as an investor, it makes me a little bit nervous because as you mentioned, nearly three-fourths of all sales are coming from their Autoship program, which is great. That’s a deep relationship, a deep understanding of what those consumers need. But they don’t exactly have a lot of room for improvement from those levels. There is still going to be a portion of their sales that are happening spontaneously. I realized that I need something, I need it to be delivered to me quickly. Chewy has the best offer, I’m going to buy it quickly. Maybe that’s off-cycle from when I would normally get an Autoship. If that’s a key metric that investors are looking at, I wouldn’t be surprised to see that tick down at some point, even marginally in future quarters, just because it’s already so high. The same is true for their net sales per active customer. For the first time in Chewy’s history, it surpassed $500 per customer, which is incredibly high. We’ve seen that grow as the relationship deepens over time, but we’ve also seen incredible levels of pet inflation in particular. That’s also potentially artificially inflated by the price of the goods that people are buying. I wouldn’t be surprised if the economic inflation comes down to see both of these metrics come down as well.
Dylan Lewis: After the break, we’ve got more retail news and updates from tech companies. Stay right here. This is Motley Fool Money. Welcome back to Motley Fool Money. I’m Dylan Lewis here in studio with Emily Flippen and Ron Gross. We were talking retail earlier and the theme of slowing consumer spend is very present right now, but it seems to be hitting retailers differently. Ron, we look at results from discount retailers, Dollar General, and Five Below, we see slightly different narratives with these two companies in their earnings reports.
Ron Gross: Yes, and it’s a little bit surprising because you would assume they have a similar client base, but it’s not exactly the same. Dollar General is indicating that due to the macroeconomic environment, business has been quite challenging. Their customer base, which is lower-income shoppers are shifting their spending to basic goods and cutting back on discretionary purchases. Not different than we saw in Macy’s or Target or any of the other retailers that have been reporting. That shows up in their numbers. But more importantly, it shows up in their guidance and the stock got slammed as a result of that guidance because it is relatively weak. Now with Five Below, even though a similar customer base, it’s a little bit different. They have a slightly higher price point. They have some stores that are even higher than the typical stores’ price points. They’re very good at creating the merchandise mix that the customer is looking for. As a result, the metrics that they put forth in this earnings report are really strong and they were able to tighten their guidance, not raise it, but tighten it because they have a better outlook into the future which is also interesting because this is a very uncertain environment. Their numbers continue to look strong, including opening 200 plus stores and continue to expand. They’re two very different reports and you may not have guessed they would be at the outset.
Dylan Lewis: You mentioned the store opening plans, and that was another place where these two businesses diverge a little bit. We saw in commentary from management Dollar General say they are going to be a little bit more opportunistic with real estate and may slow some of their expansion plans, believing that perhaps there may be some real estate deals for them in the future. Ron, I wanted to ask you, is that something that you buy as a commentary for management or do you think that is a nice way to say we are altering our plans for the rest of the year?
Ron Gross: Well, for sure there are altering their plan for the rest of the year. Dollar General is going to slow the spend, which by the way, is what you want your company to do if things are not going that well, despite your desire for a company to grow, if the environment is not right, then you do want them to slow. On the other hand, Five Below is going through their Five Beyond concept, which is a little higher price point. That’s where they’re focusing on a little bit of the more high-end consumer where they think the opportunity lies. It will be interesting to see the next report and the report after that, how things are going.
Dylan Lewis: Switching over to tech, MongoDB posted some eye-popping results in their first-quarter report this week, revenue for the database software company was up nearly 30% year over year to $370 million and net losses came in ahead of expectations. Emily, I was looking at this report and to me, it seemed like one of those earnings results where pick a metric and the company did a pretty great job?
Emily Flippen: It was eye-popping to everyone including management themselves. [laughs] If you listen to the call, management almost sounds confused about why they did. They said, we had all these expectations for what the first quarter could look like, but man, did it really do better? If you haven’t read through yet, virtually every metric across the board for was performing well. That’s great for a business like MongoDB because they’re competing with other database solutions from cloud titans like Amazon, Google, Microsoft, Oracle. They need to have those customer wins. We saw that customer wins, especially in the large enterprise side, come through with this most recent quarter. But what I found really interesting was the fact that they attributed some of their unexpected success to actually AI and machine learning users, so shocking, exactly. Well, actually it’s interesting, though, because their NoSQL database, which is better for unstructured data, plays really well into the hands of the unstructured data that you typically get from things like AI programs. But 10% or so of the customers that they acquired in the most recent quarter were specifically using their solutions for AI or machine learning, which is a large portion. Probably responsible for that unexpected win, so depending on your opinions about AI or machine learning, that could probably factor into how you expect MongoDB is going to do in terms of eye-popping results and future quarters on the plus side or the downside.
Dylan Lewis: One of the things that I wanted to dig into, the comments from management in this report was the CEO said they’re seeing customers continue to scrutinize technology spend and they are looking to separate the must-haves from the nice-to-haves. From everything I’m seeing the results here it seems like MongoDB is firmly on the must-have side for a lot of businesses.
Emily Flippen: That’s what management want you to think. They spent virtually the entire call explaining all the different use cases they have, especially with some Chinese companies as well like Alibaba and how they’ve implemented their solutions to decrease their cost structure, improve efficiency, and it’s great. They do seem to have a lot of wins. Now whether or not something was actually necessary the amount of consumption that happens on the platform. We’ve seen that decline as consumption has declined just across the board because of the broader economy. It might be a must-have, but consumption is still a big question mark.
Dylan Lewis: Ron, one of the things I wanted to ask you about was, we know customers are going through this mindset and this approach to looking at where they’re spending their money. Is it fair for investors to take a similar approach? They look at some of the names, especially the tech names in their portfolio.
Ron Gross: Omega has declared this the year of efficiency and then we’re seeing that across the board, whether it’s becoming meaner or doing layoffs. You do want your companies reacting to the current environment, even if that means selling off an underperforming subsidiary and as investors to hone hopefully a diversified portfolio, you want to make sure you own the stocks that you’re most comfortable with that are taking the steps necessary to outperform.
Dylan Lewis: Emily Flippen, Ron Gross. We’ll see you a little bit later in the show. But up next we’ve got some high-conviction ideas to keep an eye on.
Welcome back to Motley Fool Money. I’m Dylan Lewis. We love getting stock ideas here at The Motley Fool and getting other perspectives on the companies we follow. The Fool’s Scott Kassing sat down with Richard Ryskalczyk, the chief equity analyst at Sandhill Investment Management, to get a bit of both. The two talked about some of Sandhill’s highest-conviction ideas right now in cybersecurity and healthcare and how their team is analyzing high-growth companies in the current environment.
Scott Kassing: If you don’t mind, there’s one name on your high-conviction list that I wanted to start with, and that is Palo Alto Networks, ticker symbol PANW. The reason being is the industry it lives in, cybersecurity, and the consensus around that. I haven’t met an equity analyst who’s bearish on the space. Everyone believes five years from now, it’s more relevant. Where I do see non-consensus is who the winner or winners will be. In a space like this, I’m so tempted to just buy an ETF or a basket of cybersecurity stocks. From your lens, why is that not the best approach? Why Palo Alto?
Richard Ryskalczyk: From the highest level, I agree completely. I fully understand that there is consensus long this entire area and for good reason. There’s just so many continued cyber threats, whether that be from foreign entities or smaller hackers to large governments, that’s not going to stop. Then when you layer on top of that, just the continued larger attack surface that’s out there. You not only now have your internal network. You have cloud-hosted data, you have all of your employees working remotely on multiple devices. You have a massive long-term secular growth opportunity in this industry. It’s a $100 billion-plus market growing 14%, so I understand why everybody likes this area. To your point, there’s a lot of fragmentation there. There’s a lot of competition in the space. You’ve got the Ciscos of the world, the Junipers, the Symantecs, the Check Points. All of these, I guess I’d call them the old guard, if you will, much slower growing. You’ve got some of these newer-guard platforms like a Palo Alto or a Fortinet. Obviously, Microsoft is huge in the space too. Then I think your point is there’s a lot of other smaller point solutions like a Zscalar or a CrowdStrike or SentinelOne, so why not just own a basket of all of them? It goes down to, what is the valuation on each of these? What’s the valuation relative to the growth rate?
What is their free cash flow look like? We generally stay away from some of the ultra high-growth that are not yet profitable just because it’s so difficult to figure out exactly when will they hit profitability. Specifically on some of those point solutions, it’s hard to see how in the long run they’re able to hold onto their niche or their competitive advantage because there’s always the next threat and the next piece of software to come out. What we’re really seeing and one of the reasons we like Palo Alto specifically is on top of this just general cybertrend, and we’re seeing this throughout all of software, is you’ve got all these multiple points solutions that these CTOs have at these large enterprises. Basically, their heads are spinning trying to manage all of these solutions, trying to stitch them altogether where there are holes in that. What we see is this continued consolidation down and the number of vendors that these larger enterprises are utilizing, and they’re shifting more toward these broader platform solutions. Not only do you have the broad trend, but you’ve got a player like Palo Alto who’s built a really phenomenal platform solution to take care of really all of the main issues within the cybersecurity space today. That’s why we’re pointing specifically at Palo Alto.
Scott Kassing: Maybe an unofficial prediction, there could be some M&A activity in the space in the future and Palo Alto’s, I guess, one-stop-shop reputation gives it an advantage over maybe an Okta which is very specialized, meant to be a complement. Is that fair?
Richard Ryskalczyk: No, I think that’s a great characterization. This has been a trend we’ve followed for many years, as I’m sure many have. Palo Alto went through a period of time where they actually were the consolidators in the industry and they bought up a handful of businesses and then worked to recode and retool everything onto this one platform. They were a consolidator, but yes to your point, like an Okta for instance, that point solution, that can be replicated and when you can replicate that and you toss that onto a broader platform, I think some of those moats that maybe Okta might’ve had when they first came out, start to erode a little bit.
Scott Kassing: One company, in particular, I’m really excited to talk about that I think is probably the least familiar to our listeners, and that is TransMedics. I came across this company when I saw a stat that said, I believe only around 20%-25% of hearts and lungs that are donated are actually transplanted. That level of inefficiency just boggles my mind. Could you give the listeners just a brief breakdown into what exactly the TransMedics business is and your thesis behind it.
Richard Ryskalczyk: Sure. Yeah. TransMedics, it’s definitely our earliest-stage investment as far as the life cycle of the business is concerned. They’re still very early on in trying to accomplish what they are looking to accomplish. So the company is, as you pointed out, they’re looking to revolutionize organ transplantation. As you mentioned, I think when you look between heart, lung, and liver, maybe only about a third of those overall organs that are donated are actually transplanted. For heart and lung, yes, it is about 20% or so. You check that organ donor on your card, but very low likelihood that those organs actually get donated. A big piece of that is just the limitations of the current system being that you have limited time to transport, you have limited distance because of that, that you can transport these organs. The standard of care right now is that these organs are basically shipped in an Igloo cooler, sitting there on ice essentially. You see all these other advancements in the healthcare industry and you say, how is this possible when you have heart surgeons who have spent a decade in school learning how to do these transplants, essentially going and putting the hard on ice and taking it into a plane and getting it from patient A to patient B. It’s pretty astounding that that’s the level of this industry. What TransMedics is looking to do is really take, they’re now FDA approved, what they call their organ care system, this OCS medical device, which keeps the lungs breathing, the heart beating, the liver going, and allows the transplant team to monitor the vitals of these organs and it allows them to keep these organs basically alive. One, you see what the vitals are, which is a huge piece of it. But two, you can keep them alive for much longer, so you can take them on longer flights for longer distances. It’s really looking to completely revolutionize this whole marketplace.
Scott Kassing: For sure. Well, it’s got such a good for humanity mission that it’s tough to root against. Now I will say the market certainly has been paying attention. I think if I look at it today, the stock is up 37% year to date, so the question I have in my mind is, I love the business, but do I love the stock? How do you go about valuing a company that is fast-growing but it’s not yet profitable and we could be entering a recession in late 2023? How are you balancing the risk and the opportunity there?
Richard Ryskalczyk: Sure. Well, when you step back and you look at it from the 40,000-foot view and you say, what’s the opportunity here? When they say there are about 17,000 organs between the heart, lung, and liver that are transplanted per year, and TransMedics has a goal of essentially doubling that over time. If they can go and do that, right now, they’ve got about a 10% share of the current market. They want to become the standard of care. Not only do they want to take a large share of the current transplants that are being done, but they then want to go and double the overall marketplace. If they can even work their way anywhere near toward that, they’re almost at a level of profitability now. In the most recent quarter, I think their revenue was up over 160% and they only burned through, I think, I believe about $6 million dollars in cash. They’re well on their way to getting toward profitability here. They’re not going to keep growing 162% by any means, but if they can keep the growth rate up and hit some of the levels that they want to hit as far as penetrating this marketplace and growing the overall market, there’s a ton of upside is still be had from here. Yes, it’s had a nice run throughout 2022, and thus far into 2023. But if they’re able to, looking a few years out, get to the penetration rates that they think they can from here and get to some profitability I think you could still see the stock substantially higher from here in the not-too-distant future. Now you’re starting to talk about not just a price-to-sales metric, you’re starting to talk about actual free cash flow at a P/E multiple. We still think there’s a lot of upside here.
Scott Kassing: Well, you touched on it at the end. I would say there’s a renewed respect for valuation among our listener base post the growth-stock collapse of 2022. When you look at TransMedics, price-to-earnings isn’t going to tell you anything. It’s in the negatives. It’s price-to-sales. A multiple metric that you would encourage an investor who has this on their watch list to focus on or is there a better multiple metrics to keep their attention toward?
Richard Ryskalczyk: Yeah, I mean when we talk about it here internally, we look at it a handful of different ways, so even though they’re not currently profitable, we still have gone and built out our model on where we think they can get to as far as penetration rates and growing the overall marketplace, and then you take a look at where they think over the medium term the gross margin can be and work down from there to get toward a profitability level, so you can look at potentially putting earnings multiple on a handful of years out from now and then discounting that back. You can look at maybe it’s at 12 times sales right now, but in a few years from now, if they can get to $500 million in revenue in 2027, that sales multiple will contract, maybe that contracts down to 10 times and that’s $150 stock and at 20% CAGR from here and based on some of the assumptions we’ve made, so a certain level of profitability in that maybe that’s 50 times earnings multiple for something that’s still only maybe at that point 30% penetrated and still has room to substantially grow the overall marketplace as well from there which I think is more than reasonable. There are a lot of different ways to look at it from multiples on current year’s sales to, let’s do a DCF based on expected profitability too. Let’s look a few years out in the more medium-term and put a multiple on it and discount that back, so we’ve looked at it a handful of different ways.
Dylan Lewis: Coming up after the break, Emily Flippen and Ron Gross return with a couple of stocks on their radar. Stay right here. You’re listening to Motley Fool Money. As always, people on the program may have an interest in the stocks they talk about and The Motley Fool may have formal recommendations for or against, so don’t buy or sell stocks based solely on what you hear. I’m Dylan Lewis joined again by Emily Flippen and Ron Gross. We have one more earnings story before we get over to stocks on our radar. Ron, a strong update from Lululemon this week that the leisure company posted 24% revenue growth and raised its outlook for 2023. This seems like good news for a company that probably needed some good news.
Ron Gross: They’ve had some stumbles of late, but overall a very strong company and you’re seeing the real divergence here between the high-end consumer and the lower-income shopper that we discussed earlier where we’re seeing some weakness in companies like Dollar General. Lulu, a very strong report as you mentioned, strength internationally up 60% and 79% increase in sales in China as China continues to open up. Comp sales up 13%, and direct-to-consumer up 16%. That’s 42% of sales now which is interesting because it’s down from 45%, so more in-store sales of late, just something to keep an eye on. Gross margins are up, operating income was up, earnings per share up 54% really strong. On the back of a little bit of a flub in their Mirror business that they acquired relatively recently for $500 million, wrote that down, looking to sell that, moving that business to an app-based solution which I think makes good sense, so they needed to work through that, put up some good numbers to have investors focused on some other metrics and not the Mirror business.
Emily Flippen: Uh, relatively recently, Ron? That was three years ago. That was peak pandemic hype, not surprised at all. I don’t think anybody wants to see them when they get out of that home fitness business. Peloton is just continuing to be a dumpster fire, so it’s a great example of why it’s probably not advantageous for Lululemon to hold onto that, especially when their core business is performing so well. But my big question mark is, how far does the pricing power for this company go? I mean I love my Lululemon leggings. They are expensive but I still have a hard time justifying the purchase, but I do justify it to myself. I mean do they have Apple-level pricing power or is it more like Chipotle where at some point the Chipotle burrito’s not worth it now, can they hike the prices? Yes. Will I pay up for Chipotle? Yes, but it doesn’t go as high as say my iPhone, so that’s my question mark with Lululemon long-term.
Dylan Lewis: Let’s get onto our radar stocks. Our man behind the glass, Dan Boyd, is going to hit you with a question. Ron, you’re up first, what are you watching this week?
Ron Gross: Go Danny Boy, you’re going to love Compass Minerals, CMP. Roots go all the way back to 1844, leading producer of salt for highway deicing. That’s about 80% of sales. The rest comes from plant nutrition sulfate of potash, SOP if you will. Now it’s not all peaches and cream, and weather conditions have hurt the business. They have a rough time passing on inflationary price increases because contracts are done well in advance, but new leadership is making some really strong moves to improve operations, shore up the balance sheet, improve profitability and they’ve got some new lines of business around lithium and some other new items that build on their core competencies that should spur growth into the future. It’s somewhat of a turnaround play, but it’s worth keeping an eye on.
Dylan Lewis: Dan, a question about Compass Minerals.
Dan Boyd: Yeah. Ron, how many cars do you think that Compass Minerals is responsible for completely rusting out in the Midwest, Upper Midwest, and Northeast?
Ron Gross: I’d rather have rust than be unsafe, Dan.
Dylan Lewis: It’s a good point. Yeah, I think listeners are used to hearing AI, perhaps not as used to hearing potash. Am I saying that right, Ron?
Ron Gross: Potash.
Dylan Lewis: Potash. On the show, it’s nice to work that in, I’m sure there are some folks out there that are happy to hear it. Emily, what is on your radar this week?
Emily Flippen: I’m looking at Veeva Systems. They’re a software business aimed at the life sciences industry and they had an unusual quarter this week, mostly because the stock is up something like 15%, which is very unusual for a company that is incredibly stable as Veeva has proven to be, but seasonally strong quarter for them which is wonderful, unexpectedly wonderful. This is a business that has been expanding its margins or looking to expand its margins, growing sales rapidly, but there’s a big question mark right now about how much money they’re spending on building out the functionality of their core CRM products and with their migration away from Salesforce to their own servers hoping that by 2025 they can really start expanding margins, but there’s a good argument to be made that the valuation looks really frothy right now.
Dylan Lewis: Dan, a question about Veeva Systems.
Dan Boyd: In February they became a public benefit corporation and since then their stock is down $90. Emily, is this altruism damaging their business?
Dylan Lewis: Look at that.
Emily Flippen: Shareholders are not happy about the full stakeholder relationship. Now, this is the broader slowdown in software that’s causing the reaction we’re seeing from Veeva plus their valuation. I’d actually argue that their move to become a public benefit corporation think about all their stakeholders is probably going to be a net benefit for this company over the long term.
Dylan Lewis: Dan, you’re really getting two different ends of the spectrum here.
Dan Boyd: You think?
Dylan Lewis: Ron, is giving you earth materials to work with and Emily’s having you focus a bit on the future with software, which company are you putting on your watch list?
Dan Boyd: I want to go with Minerals because I just think it’s more fun, like salt mines and potash, who doesn’t love that stuff? But I think I’m going to go with the public benefit corporation and Veeva this time around, Dylan.
Emily Flippen: A wise choice.
Dylan Lewis: Appreciate it. Emily Flippen and Ron Gross, thanks so much for being here.
Ron Gross: Thanks, Dylan.
Dylan Lewis: That’s going to do it for this week’s Motley Fool Money radio show. The show is mixed by Dan Boyd. I’m Dylan Lewis. Thanks for listening. We’ll see you next time.
Article originally posted on mongodb google news. Visit mongodb google news
Panagora Asset Management Inc. Has $4.65 Million Holdings in MongoDB, Inc. (NASDAQ:MDB)
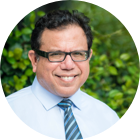
MMS • RSS
Posted on mongodb google news. Visit mongodb google news
Panagora Asset Management Inc. grew its position in MongoDB, Inc. (NASDAQ:MDB – Get Rating) by 53.7% in the 4th quarter, according to the company in its most recent Form 13F filing with the Securities and Exchange Commission (SEC). The firm owned 23,633 shares of the company’s stock after buying an additional 8,258 shares during the quarter. Panagora Asset Management Inc.’s holdings in MongoDB were worth $4,652,000 at the end of the most recent quarter.
Other institutional investors have also made changes to their positions in the company. Seeyond lifted its stake in MongoDB by 308.9% in the 4th quarter. Seeyond now owns 2,723 shares of the company’s stock worth $536,000 after acquiring an additional 2,057 shares in the last quarter. Renaissance Technologies LLC boosted its holdings in shares of MongoDB by 493.2% during the fourth quarter. Renaissance Technologies LLC now owns 918,200 shares of the company’s stock worth $180,738,000 after purchasing an additional 763,400 shares during the period. The Manufacturers Life Insurance Company grew its position in MongoDB by 2.7% during the fourth quarter. The Manufacturers Life Insurance Company now owns 42,038 shares of the company’s stock valued at $8,356,000 after purchasing an additional 1,096 shares in the last quarter. Geode Capital Management LLC increased its stake in MongoDB by 4.5% in the 4th quarter. Geode Capital Management LLC now owns 931,748 shares of the company’s stock valued at $183,193,000 after buying an additional 39,741 shares during the period. Finally, Cipher Capital LP acquired a new position in MongoDB in the 4th quarter valued at approximately $1,372,000. 84.86% of the stock is owned by hedge funds and other institutional investors.
MongoDB Stock Performance
Shares of MongoDB stock opened at $374.51 on Friday. MongoDB, Inc. has a 52-week low of $135.15 and a 52-week high of $398.89. The company has a debt-to-equity ratio of 1.44, a current ratio of 4.19 and a quick ratio of 3.80. The company’s fifty day moving average price is $260.46 and its two-hundred day moving average price is $220.52.
MongoDB (NASDAQ:MDB – Get Rating) last posted its earnings results on Thursday, June 1st. The company reported $0.56 earnings per share for the quarter, beating analysts’ consensus estimates of $0.18 by $0.38. The business had revenue of $368.28 million for the quarter, compared to analysts’ expectations of $347.77 million. MongoDB had a negative net margin of 23.58% and a negative return on equity of 43.25%. The firm’s revenue for the quarter was up 29.0% on a year-over-year basis. During the same quarter in the previous year, the firm posted ($1.15) earnings per share. Analysts anticipate that MongoDB, Inc. will post -2.85 earnings per share for the current year.
Insider Transactions at MongoDB
In related news, CTO Mark Porter sold 1,900 shares of the business’s stock in a transaction on Monday, April 3rd. The stock was sold at an average price of $226.17, for a total value of $429,723.00. Following the sale, the chief technology officer now owns 43,009 shares of the company’s stock, valued at approximately $9,727,345.53. The sale was disclosed in a legal filing with the SEC, which is available through this link. In other news, CAO Thomas Bull sold 605 shares of the firm’s stock in a transaction that occurred on Monday, April 3rd. The shares were sold at an average price of $228.34, for a total transaction of $138,145.70. Following the completion of the sale, the chief accounting officer now owns 17,706 shares of the company’s stock, valued at $4,042,988.04. The transaction was disclosed in a document filed with the SEC, which is accessible through this link. Also, CTO Mark Porter sold 1,900 shares of MongoDB stock in a transaction that occurred on Monday, April 3rd. The shares were sold at an average price of $226.17, for a total transaction of $429,723.00. Following the completion of the transaction, the chief technology officer now owns 43,009 shares of the company’s stock, valued at approximately $9,727,345.53. The disclosure for this sale can be found here. In the last ninety days, insiders sold 106,682 shares of company stock valued at $26,516,196. Corporate insiders own 4.80% of the company’s stock.
Analyst Upgrades and Downgrades
Several equities analysts have recently commented on MDB shares. Citigroup boosted their price target on shares of MongoDB from $363.00 to $430.00 in a report on Friday, June 2nd. The Goldman Sachs Group upped their target price on MongoDB from $280.00 to $420.00 in a research report on Friday, June 2nd. Wedbush reduced their price target on MongoDB from $240.00 to $230.00 in a report on Thursday, March 9th. Barclays boosted their price target on MongoDB from $280.00 to $374.00 in a research note on Friday, June 2nd. Finally, William Blair reaffirmed an “outperform” rating on shares of MongoDB in a research report on Friday, June 2nd. One research analyst has rated the stock with a sell rating, two have given a hold rating and twenty-one have given a buy rating to the company’s stock. Based on data from MarketBeat.com, MongoDB currently has a consensus rating of “Moderate Buy” and an average price target of $328.35.
MongoDB Company Profile
MongoDB, Inc provides general purpose database platform worldwide. The company offers MongoDB Atlas, a hosted multi-cloud database-as-a-service solution; MongoDB Enterprise Advanced, a commercial database server for enterprise customers to run in the cloud, on-premise, or in a hybrid environment; and Community Server, a free-to-download version of its database, which includes the functionality that developers need to get started with MongoDB.
See Also
Receive News & Ratings for MongoDB Daily – Enter your email address below to receive a concise daily summary of the latest news and analysts’ ratings for MongoDB and related companies with MarketBeat.com’s FREE daily email newsletter.
Article originally posted on mongodb google news. Visit mongodb google news
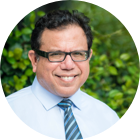
MMS • Sergio De Simone
Article originally posted on InfoQ. Visit InfoQ
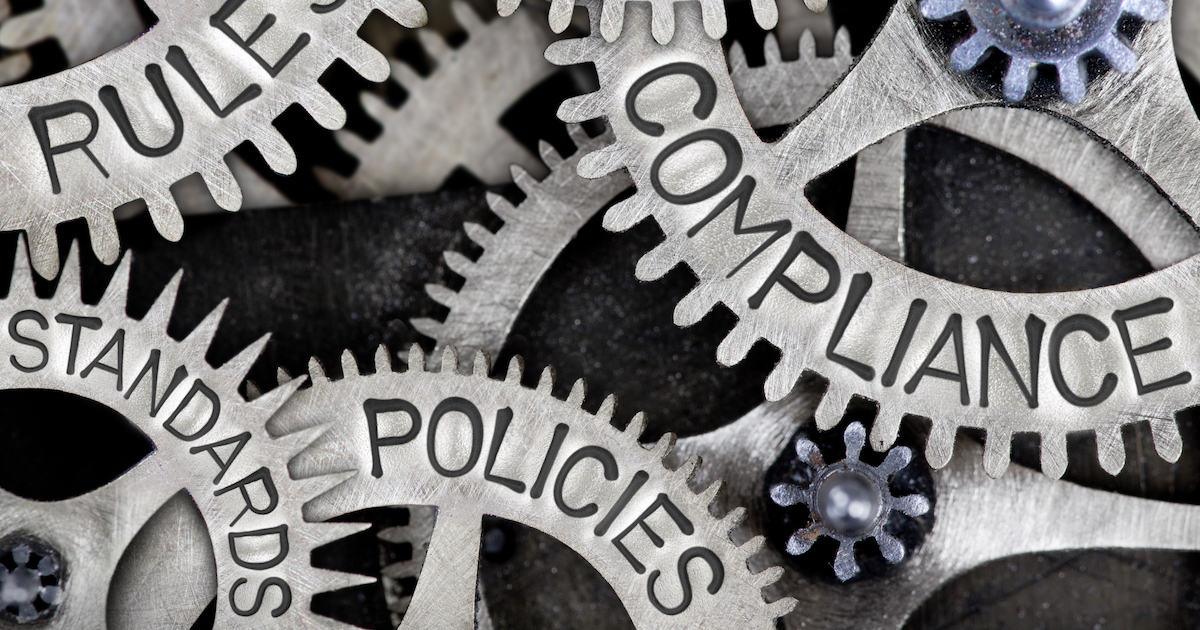
GitHub has launched code scanning support for Swift in beta and announced it will include Swift security advisories in its Advisory Database to extend the capabilities of its Dependabot vulnerability monitor.
GitHub code scanning enables receiving actionable security alerts in pull requests, which are shown as a review on the PR Conversation tab. Swift support extends the set of programming languages that GitHub can scan for weaknesses, which already included C/C++, Java/Kotlin, JS/TS, Python, Ruby, C#, and Go.
Having both Kotlin and Swift support is crucial for CodeQL, the engine that powers GitHub code scanning, due to the growing popularity and adoption of these programming languages. Kotlin and Swift are widely used in mobile app development, particularly for Android and iOS platforms.
Currently, code scanning for Swift covers path injection, unsafe web view fetches, cryptographic misuses, processing of unsanitized data, and more. GitHub says they will increase the number of weaknesses Swift code scanning is able to detect as the beta progresses. For the rest of supported languages, code scanning includes nearly 400 checks and strives to keep false positive rate low and precision high, says GitHub.
Code scanning for Swift uses macOS runners, which are more expensive than Linux and Windows runners. Due to this, GitHub recommends building only the code you want to analyze and targeting only one architecture. Currently, Swift versions from 5.4 to 5.7 can be analyzed on macOS, while Swift 5.7.3 can be also analyzed using Linux.
On the supply chain security front, GitHub also announced they will add curated Swift advisories to the Advisory Database and Swift dependencies analysis to dependency graphs. This will enable Dependabot to send alerts about vulnerable dependencies included in Swift projects.
The GitHub Advisory Database contains known security vulnerabilities and malware (still in beta). Advisories can be curated or not curated. The dependency graph includes all the dependencies of a repository, including direct and indirect dependencies, and is generated automatically for all public repositories. According to GitHub, Swift support will come later in June.
On a related note, GitHub has launched a Bug Bounty program for Kotlin and Swift security researchers to submit new CodeQL queries that can uncover new vulnerabilities in Swift and Kotlin programs.
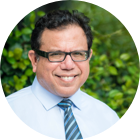
MMS • A N M Bazlur Rahman
Article originally posted on InfoQ. Visit InfoQ
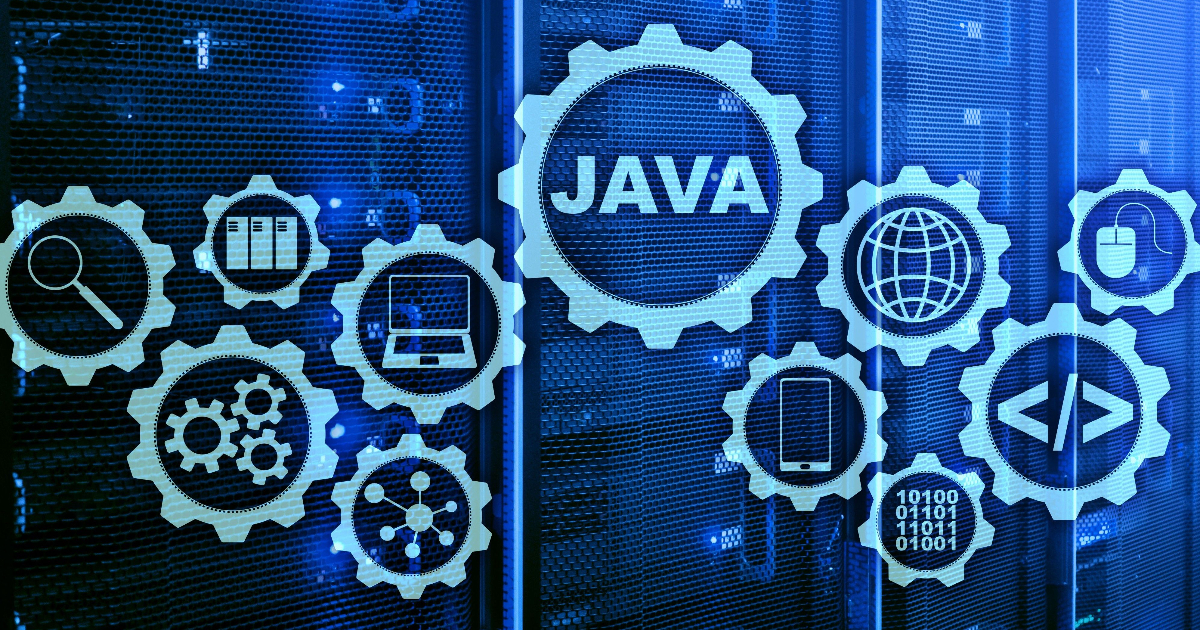
JEP 453, Structured Concurrency (Preview), has been Integrated from the Targeted status for JDK 21. Formerly an incubating API, this initial preview incorporates enhancements in response to feedback from the previous two rounds of incubation: JEP 428, Structured Concurrency (Incubator), delivered in JDK 19; and JEP 437, Structured Concurrency (Second Incubator), delivered in JDK 20. The only significant change in the current proposal is that the StructuredTaskScope::fork(...)
method returns a [Subtask
] rather than a Future
. This is a preview feature.
Structured Concurrency in JDK 21 is aimed at simplifying concurrent programming by introducing an API for structured concurrency. This approach treats groups of related tasks running in different threads as a single unit of work, thus streamlining error handling and cancellation, improving reliability, and enhancing observability. Let’s see an example:
Response handle() throws ExecutionException, InterruptedException {
try (var scope = new StructuredTaskScope.ShutdownOnFailure()) {
Supplier user = scope.fork(() -> findUser());
Supplier order = scope.fork(() -> fetchOrder());
scope.join() // Join both subtasks
.throwIfFailed(); // ... and propagate errors
// Here, both subtasks have succeeded, so compose their results
return new Response(user.get(), order.get());
}
//...
}
This code creates a new StructuredTaskScope
and uses it to fork two subtasks: one that executes findUser()
and another that executes fetchOrder()
. Once both subtasks have been completed, it creates a new Response
using the results of both subtasks.
Structured concurrency is a preview API, disabled by default. To use the StructuredTaskScope
API, developers must enable preview APIs to compile this code, as shown in the following command:
javac --release 21 --enable-preview Main.java
The same flag is also required to run the program:
java --enable-preview Main
However, one can directly run this using the source code launcher. In that case, the command line would be:
java --source 21 --enable-preview Main.java
The jshell option is also available but requires enabling the preview feature as well:
jshell --enable-preview
In practice, most uses of StructuredTaskScope
will not utilize the StructuredTaskScope
class directly but instead use one of the two subclasses implementing shutdown policies. These subclasses, ShutdownOnFailure
and ShutdownOnSuccess
, support patterns that shut down the scope when the first subtask fails or succeeds, respectively.
Structured concurrency treats groups of related tasks running in different threads as a single unit of work. This approach streamlines error handling and cancellation, improves reliability, and enhances observability. The developers, Ron Pressler, consulting member of the technical staff at Oracle and technical lead for OpenJDK’s Project Loom, and Alan Bateman, an engineer in the Java Platform Group at Oracle, intend to eliminate common risks associated with concurrent programming, like thread leaks and cancellation delays, and to enhance the observability of concurrent code.
The new feature does not aim to replace any of the concurrency constructs in the java.util.concurrent
package, such as ExecutorService
and Future
. It also does not aim to define the definitive structured concurrency API for the Java Platform or a method for sharing streams of data among threads.
Current concurrent programming models, such as the ExecutorService
API, introduce complexity and risks due to their unrestricted patterns of concurrency. These models don’t enforce or track relationships among tasks and subtasks, making the management and observability of concurrent tasks challenging.
The structured concurrency model proposes that task structure should reflect code structure. In single-threaded code, the execution always enforces a hierarchy of tasks and subtasks, with the lifetime of each subtask relative to the others governed by the syntactic block structure of the code.
The new StructuredTaskScope
provides a simpler and safer alternative to ExecutorService
. This API encapsulates a group of related tasks that should be completed together, with failure of any subtask leading to the cancellation of the remaining subtasks.
Further details of the changes, including code examples and a comprehensive discussion of the motivation behind this feature, are available on the OpenJDK website.
This new API is a significant step toward making concurrent programming easier, more reliable, and more observable. It is expected to be particularly beneficial for building maintainable, reliable, and observable server applications. Developers interested in a deep dive into structured concurrency and learning the backstory can listen to the InfoQ Podcast, a YouTube session by Ron Pressler and the Inside Java articles.
French investment firm Seeyond makes bold move to increase stake in innovative database …
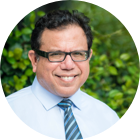
MMS • RSS
Posted on mongodb google news. Visit mongodb google news
Seeyond, a quantitative investment management firm based in France, has raised eyebrows with its recent move to substantially increase its stake in MongoDB, Inc. (NASDAQ:MDB). According to the latest filing with the Securities & Exchange Commission, Seeyond’s total holdings in the company increased by an impressive 308.9% during the fourth quarter of 2020. The firm now owns a total of 2,723 shares of MDB stock, worth approximately $536,000.
So what makes this move so surprising? Well, for starters, MongoDB is a relatively new player in the database industry. Founded in 2007 by Dwight Merriman and Eliot Horowitz, the company didn’t go public until October 2017. Nevertheless, it has already carved out a significant market position thanks to its innovative platform and emphasis on scalability and flexibility.
At its core, MongoDB provides a general-purpose database platform that can be utilized by enterprises of all sizes across a variety of industries. The company’s flagship product, MongoDB Atlas, is a multi-cloud database-as-a-service solution that allows customers to easily migrate databases between cloud providers or use multiple providers simultaneously.
In addition to Atlas, MongoDB also offers an enterprise-grade commercial server (MongoDB Enterprise Advanced) and a freely-downloadable version aimed at developers (Community Server).
Despite being a relatively young company compared to some of its competitors (such as Oracle or IBM), MongoDB has seen steady growth over the past few years. Its shares currently trade on NASDAQ under the symbol MDB and opened at $374.67 on February 5th.
As for Seeyond’s decision to increase its stake so dramatically – only time will tell whether it was a wise move or not. However, considering MongoDB’s strong market position and focus on innovation within the database space, there may be good reason for investors like Seeyond to take notice and see potential for growth down the line.
Overall though – it’s an interesting move from Seeyond, and one that will certainly be watched with great interest by industry analysts and investors alike.
MongoDB attracts major investors as stock values increase
MongoDB, Inc has experienced significant changes in its holdings and stock values as major investors move to purchase shares. Hedge funds like BI Asset Management Fondsmaeglerselskab A S, Lindbrook Capital LLC, Y.D. More Investments Ltd, CI Investments Inc., and Quent Capital LLC have made significant strides in acquiring more than 84% of the company’s stock. These purchases indicate that MongoDB is a profitable investment for hedge funds and institutional investors.
The news follows announcements made by insiders within the company who sold approximately 106,682 shares amounting to $26,516,196 over the past quarter. CTO Mark Porter sold 1,900 shares on April 3rd at an average cost of $226.17 where he pocketed $429,723 while Director Dwight A Merriman sold 3,000 shares of company’s stock on June 1st among others.
MongoDB provides a general-purpose database platform globally with several products including MongoDB Atlas- a multi-cloud database-as-a-service solution; MongoDB Enterprise Advanced- a commercial database server designed for enterprise customers running in hybrid environments; and Community Server- free-to-download version that includes all features needed to get started with MongoDB.
Despite its business model disruption caused by distributed systems technology competition from Amazon Web Services (AWS), Microsoft Azure Cosmos DB and Google Cloud Spanner offerings, it has emerged lucrative for the New York firm as they reported $368.28 million revenue in Q2
with earnings per share of $0.56 marking a positive surprise on analysts predictions of $347.77 million revenue resulting in earnings per share at $0.18 according to NASDAQ Business Insider News.
The massive interest shown by Institutional investors buying almost half the traded volume with insider selling pressures offsetting demand may have led U.S research firms like Tigress Financial Wedbush William Blair Stifel Nicolaus JMP Securities giving recommendations including ratings cutbacks ultimately resulting in a consensus price target of $328.35.
This disruption leading to MongoDB’s revenue surge is expected to continue, and thus, investors’ interest in its stock may grow as well. With a unique business model that caters to the needs of developers, it looks like MongoDB has become a force to reckon with in the industry.
Article originally posted on mongodb google news. Visit mongodb google news
Presentation: From Zero to A Hundred Billion: Building Scalable Real-time Event Processing At DoorDash
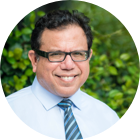
MMS • Allen Wang
Article originally posted on InfoQ. Visit InfoQ
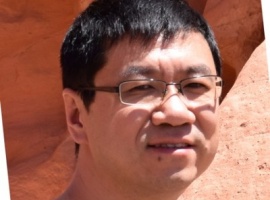
Transcript
Wang: My name is Allen Wang. I’ve been building real time data infrastructure for the past seven years. First at Netflix for the Keystone Data Pipeline and currently at DoorDash, building a real time event processing system called Iguazu. The journey of building those systems give me an opportunity to find some common patterns in successful real time data architectures.
Successful Patterns in Building Realtime Event Processing
In my mind, there are four principles that have passed the test of time. First, decoupling stages. We’ll talk about what those stages are, and why, and how to make that decoupling happen. Second, leveraging stream processing framework. We will cover what stream processing framework can do for you and how you can pick one. Third, creating abstractions. For that, I will list the common abstractions that you can create to facilitate the adoption of your system. Finally, fine-grained failure isolation and scalability. Failures will happen, how do you isolate the failures and avoid bottleneck in scalability? I know those concepts may sound a bit abstract at this point, so I’m going to use a real time event processing system we created at DoorDash as an example, to give you some concrete ideas.
Real Time Events Use Case at DoorDash
First, let’s talk about use cases of real time events. At DoorDash, real time events are an important data source to gain insight into our business, and help us make data driven decisions, just to give you a few examples how real time events are used at DoorDash. First, almost all events need to be reliably transported to our data warehouse, Snowflake, or other online analytical datastores with low latency for business analysis. For example, the dasher assignment team relies on the assignment events to detect any bugs in their algorithm at near real time. The second use case is mobile application health monitoring. Some mobile events will be integrated with our time series metric backend for monitoring and alerting so that the teams can quickly identify issues in the latest mobile application releases. The third use case is sessionization. We will like to group user events into sessions and generate session attributes at real time so that we can better analyze the user behaviors and push real time recommendations.
Historically, DoorDash has a few data pipelines that get data from our legacy monolithic web application and ingest the data into Snowflake. Each pipeline is built differently and can only handle one event. They involve mixed data transports, multiple queuing systems, and multiple third-party data services, which make it really difficult to control the latency, maintain the cost, and identify scalability bottlenecks in operations. We started to rethink this approach and decided to build a new system to replace those legacy pipelines and address the future event processing needs that we anticipated. First, it should support heterogeneous data sources, including microservices, and mobile or web applications, and be able to deliver the events to different destinations. Second, it should provide low latency and reliable data ingest into our data warehouse with a reasonable cost. Third, real time events should be easily accessible for data consumers. We want to empower all teams to create their own processing logic and tap into the streams of real time data. Finally, to improve data quality, we want to have end-to-end schema enforcement and schema evolution.
Introducing Iguazu
Two years ago, we started the journey of creating from scratch a real time event processing system named Iguazu. The important design decisions we made is to shift the strategy from heavily relying on third party data services to leveraging open source frameworks that can be customized and better integrates with the DoorDash infrastructure. Fast forward to today, we scaled Iguazu from processing just a few billion events to hundreds of billions of events per day with four nines of delivery rate. Compared to the legacy pipelines, the end-to-end latency to Snowflake is reduced from a day to just a few minutes. This is the architecture overview of Iguazu. The data ingestion for microservices and mobile clients are enabled through our Iguazu Kafka proxy before it lands into Kafka. We use Apache Kafka for Pub/Sub and decoupling the producers from consumers. Once the data lands into Kafka, we use stream processing applications built on top of Apache Flink for data transformation. That is achieved through Flink’s data stream APIs and Flink SQL. After the stream process is done, the data is sent to different destinations, including S3 for data warehouse, and data lake integrations, Redis for real time features, and Chronosphere for operational metrics. In the following slides, I’ll discuss the details of each of those major components.
Simplify and Optimize Event Producing
Let’s first talk about producing events. The main focus is to make event producing as easy as possible and optimized for the workload of analytical data. To give more context, let me first explain the requirements for processing analytical data. First, analytical data usually comes with high volume and growth rate. As an example, in the process of making restaurant order on DoorDash, tens of thousands of analytical events are published. As we strive to improve user experience and delivery quality, more user actions are tracked at fine granularity, and these lead to the higher growth rate of analytical data volume compared with order volume. Therefore, compared with transactional data, analytical data requires higher scalability and cost efficiency. On the other hand, because analytical data are almost always processed and analyzed in aggregated fashion, minor data loss will not affect the quality of analysis. For example, if you collect 1 million results in an A/B test, and randomly drop 100 events from the results, most likely it will not affect the conclusion of the experiment. Typically, we have found a data loss of less than 0.1% will be acceptable for analytical events.
Leveraging Kafka REST Proxy
Let’s look at the measures that we have taken to ensure the efficiency and the scalability in analytical event publishing. As I mentioned in the overview of the Iguazu architecture, we choose Kafka as the central Pub/Sub system. One challenge we face is how we can enable average DoorDash service to easily produce events through Kafka. Even though Kafka has been out there for quite some time, there are still teams struggling with the producer internals, tuning the producer properties, and making the Kafka connection. The solution we created is to leverage the Confluent Kafka REST proxy. The proxy provides a central place where we can enhance and optimize event producing functionalities. It provides the abstractions over Kafka with HTTP interface, eliminating the need to configure Kafka connections from all services and making event publishing much easier.
The Kafka REST proxy provides all the basic features that we need out of the box. One critical feature is that it supports event batching. You probably know that batching is a key factor in improving efficiencies in data processing. Why would it specifically help improving efficiency in event publishing to Kafka? What we have found out is that the Kafka brokers workload is highly dependent on the rate of the producing requests. The higher the rate of producing requests, the higher the CPU utilization on the program, and more workers will likely be needed. How can you produce to your Kafka with high data volume better than low rate of produce requests? As a formula in the slide suggests, you need to increase the number of events per request, which essentially means increasing the batch size. Batching comes in with tradeoff of higher latency in event publishing. However, processing of analytical events are typically done in an asynchronous fashion and does not require sub-second latency in getting back the final results. Small increase in the event publishing latency is an acceptable tradeoff. With Kafka REST proxy, you can batch events in each request from client side and rely on the Kafka client leader proxy to further batch them before producing to the broker. The result is that you will get the nice effect of event batching across client instances and applications. Let’s say you have an application that publishes events at a very low rate, which would lead to inefficient batching. The proxy will be able to mix these low volume events with other high-volume events in one batch. It makes event publishing very efficient and greatly reduce the workload for the Kafka brokers.
Proxy Enhancements
The Kafka REST proxy provides all the basic features we need out of the box, but to further improve its performance and scalability, we added our own feature like multi-cluster producing, and asynchronous request processing. Multi-cluster producing means the same proxy can produce to multiple Kafka clusters. Each topic will be mapped to a cluster, and this ensures that we can scale beyond one Kafka cluster. It also enables us to migrate topics from one Kafka cluster to another, which helps to balance the workload and improve the cost efficiency of Kafka clusters. Asynchronous request processing means the proxy will respond to the produce request as soon as it pulls the Kafka records into the Kafka clients producer buffer without waiting for the broker’s acknowledgment. This has a few advantages. First, it significantly improves the performance of the proxy and helps reduce the back pressure on the proxy’s clients. Second, asynchronous request processing means the proxies spend less time block waiting for the broker’s response and more time for the proxy to process requests, which lead to better batching and throughput. Finally, we understand that this asynchronous mode means clients may not get the actual clear response from the broker and may lead to data loss. To mitigate this issue, we added automated retries on the proxy side on behalf of the client. The number of retries is configurable and each subsequent retry will be done on a randomly picked partition to maximize the chance of success. The result is minimal data loss of less than 0.001%, which is well in range of acceptable data loss level for analytical events.
Event Processing with Flink
Now that I have covered events producing, let’s focus on what we have done to facilitate event consuming. One important objective for Iguazu is to create a platform for easy data processing. Apache Flink’s layered API architecture fits perfectly with this objective. We choose Apache Flink, also because of its low latency processing, native support of processing based on event time, and fault tolerance and built-in integrations with a wide range of sources and sinks, including Kafka, Redis, Elasticsearch, and S3. Ultimately, we need to understand what stream processing framework can do for you. We’ll demonstrate that by looking at a simple Kafka consumer. This is a typical Kafka consumer. First, it gets records from Kafka in a loop. Then it will update a local state using the records, and it just retrieves and produce a result from the state. Finally, it will push the result to a downstream process, perhaps over the network.
On the first look, the code is really simple and does the job. However, questions will arise over time. First, note that the code does not commit Kafka offset, and this will likely lead to failures to provide any delivery guarantee when failures occur. Where should the offset commit be added to the code to provide the desired delivery guarantee be it at-least once, at-most once, or exactly-once. Second, the local state object is stored in memory and it will be lost when the consumer crashes. It should be persisted along with the Kafka offset so that the state can be accurately restored upon failure recovery. How can we persist and restore the state when necessary? Finally, the parallelism of Kafka consumer is limited by the number of partitions of the topic being consumed. What if the bottleneck of the application is in processing of the records or pushing the results to downstream, and we need higher parallelism than the number of partitions. Apparently, it takes more than a simple Kafka consumer to create a scalable and fault tolerant application. This is where stream processing framework like Flink shines. As shown in this code example, Flink helps you to achieve delivery guarantees, automatically persists and restores application state through checkpointing. It provides a flexible way to assign compute resources to different data operators. These are just a few examples that stream processing framework can offer.
One of the most important features from Flink is layered APIs. At the bottom, process function allows engineers to create highly customized code and have precise control on handling events, state, and time. The next level up, Flink offers data stream APIs with built-in high-level functions to support different aggregations and windowing, so that engineers can create a stream processing solution with just a few lines of code. On the top we have SQL and table APIs, which offer casual data users the opportunity to write Flink applications in a declarative way, using SQL instead of code. To help people at DoorDash leverage Flink, we have created a stream processing platform. Our platform provides a base frame Docker image with all the necessary configurations that are well integrated with the rest of the DoorDash infrastructure. Flink’s high availability setup and Flink internal metrics will be available out of the box. For better failure isolation and the ability to scale independently, each Flink job is deployed in a standalone mode as a separate Kubernetes service. We support two abstractions in our platform, data stream APIs for engineers and a Flink SQL for casual data users.
You may be wondering why Flink SQL is an important abstraction we want to leverage. Here’s a concrete example. Real time features are an important component in machine learning, for model training and prediction. For example, to predict an ETA of a DoorDash delivery order requires up to date store order count for each restaurant, which we call a real time feature. Traditionally, creating a real time feature requires coding history and parsing the application and transforms and arrays the events into real time features. Creating Flink applications requires a big learning curve for machine learning engineers and becomes a bottleneck when tens or hundreds of features need to be created. The application created often have a lot of boilerplate code that are replicated across multiple applications. Engineers also take the shortcut to bundle the calculation of multiple features in one application, which lacks failure isolation or the ability to allocate more resources to a specific feature calculation.
Riviera
To meet those challenges, we decided to create a SQL based DSL framework called Riviera, where all the necessary processing logic and wiring are captured in a YAML file. The YAML file creates a lot of high-level abstractions, for example, connecting to a Kafka source, and producing to certain things. To create a real time feature, engineers only need to create one YAML file. Riviera achieved great results. The time needed to develop a new feature is reduced from weeks to hours. The feature engineering code base is reduced by 70% after migrating to Riviera. Here’s a Rivera DSL example, to show how Flink SQL is used to calculate store order count for each restaurant, which is an important real time feature used in production for model prediction.
First, you need to specify the source sink and a schema used in the application. In this case, the source is a Kafka topic and a sink is a Redis cluster. The process logic is expressed as a SQL query. You can see that we used the built-in COUNT function to aggregate the order count. We also used hot window. The hot window indicates that you want to process the data that are received in the last 20 minutes and refresh the results every 20 seconds.
Event Format and Schema: Protocol between Producers and Consumers
In the above two sections, we covered event producing and consuming in Iguazu. However, without a unified event format, it’s still difficult for producers and consumers to understand each other. Here, we will discuss the event format, and schemas, which serve as a protocol between producers and consumers. From the very beginning, we defined a unified event format. The unified event format includes an envelope and payload. The payload contains the schema encoded event properties. The envelope contains context of the event, for example, event creation time, metadata, including encoding method and the references to the schema. It also includes a non-schematized JSON blob called custom attributes. This JSON section in the envelope gives users a choice, where they can store certain data and evolve them freely without going through the formal schema evolution. This flexibility proves to be useful at an early stage of event creation, where frequent adjustments of schema definition are expected. We created serialization libraries for both event producers and the consumers to interact with this standard event format. In Kafka, the event envelope is stored as a Kafka record header, and the schema encoded payload is stored as a record value. Our serialization library takes the responsibility of converting back and forth between the event API and the properly encoded Kafka record so that the applications can focus on their main logic.
We have leveraged Confluent schema registry for generic data processing. First, let me briefly introduce schema registry. As we know, schema is a contract between producers and consumers on data or events that they both interact with. To make sure producers and consumers agree on the schema, one simple way is to present the schema as a POJO class, which is available for both producers and consumers. However, there’s no guarantee that the producers and the consumers will have the same version of the POJO. To ensure that a change of the schema is propagated from the producer to the consumer, they must coordinate on when the new POJO class will be made available for each party. Schema registry helps to avoid this manual coordination between producers and consumers on schema changes. It is a standalone REST service to store schemas and serve the schema lookup requests from both producers and consumers using schema IDs. Where schema changes are registered with schema registry, it will enforce compatibility rules and reject incompatible schema changes.
To leverage the schema registry for generic data processing, schema ID is embedded in the event payload so that the downstream consumers can look it up from schema registry without relying on the object classes on the runtime class path. Both protobuf and Avro schemas are supported in our serialization library and the schema registry. We support protobuf schema, because almost all of our microservices are based on gRPC and protobuf supported by a central protobuf Git repository. To enforce this single source of truth, avoid duplicate schema definition and to ensure the smooth adoption of Iguazu, we decided to use the protobuf as the primary schema type. On the other hand, the Avro schema is still better supported than protobuf in most of the data frameworks. Where necessary, our serialization library takes the responsibility of seamlessly converting the protobuf message to Avro format and vice versa.
One decision we have to make is when we should allow schema update to happen. There are two choices, at build time or producers runtime. It is usually tempting to let producers freely update the schema at the time of event publishing. There are some risks associated with that. It may lead to data loss because any incompatible schema change will fail the schema registry update and cause runtime failures in event publishing. It will also lead to spikes of schema registry update requests causing potential scalability issues for the schema registry. Instead, it will be ideal to register and update the schema at build time to catch incompatible schema changes early in the development cycle, and reduce the update aka call volume to the schema registry.
One challenge we faced is how we can centrally automate the schema update at build time. The solution we created is to leverage the central repository that manages all of our protobuf schemas and integrate the schema registry update as part of its CI/CD process. When a protobuf definition is updated in the pull request, the CI process will validate the change with the schema registry and it will fail if the change is incompatible. After the CI passes, and the pull request is merged, the CD process will actually register or update the schema registry and publish the compiled protobuf JAR files. The CI/CD process not only eliminates the overhead of manual schema registration, but also guarantees the early detection of incompatible schema changes and the consistency between the [inaudible 00:26:34] protobuf class families and the schemas in the schema registry. Ultimately, this automation avoids schema update at runtime and the possible data loss due to incompatible schema changes.
Data Warehouse and Data Lake Integration
So far, we have talked about event producing, consuming, and event format. In this slide, I’ll give some details on our data warehouse integration. Data Warehouse integration is one of the key goals of Iguazu. Snowflake is our main data warehouse, and we expect events to be delivered to Snowflake with strong consistency and low latency. The data warehouse integration is implemented as a two-step process. In the first step, data is consumed by a Flink application from a Kafka topic and uploaded to S3 in the parquet format. This step leverages S3 to decouple the stream processing from Snowflake so that Snowflake failures will not impact stream processing. It will also provide a backfill mechanism from S3 given Kafka’s limited retention. Finally, having the parquet files on S3 enables date lake integrations as the parquet data can be easily converted to any desired table format. The implementation of uploading data to S3 is done through Flink’s StreamingFileSink. When completing an upload as part of Flink’s checkpoint, StreamingFileSink guarantees strong consistency and exactly once delivery. StreamingFileSink also allows customized bucketing on S3 which means you can flexibly partition the data using any fields. This optimization greatly facilitates the downstream consumers.
At the second step, data is copied from S3 to Snowflake tables via Snowpipe. Snowpipe is a Snowflake service to load data from external storage at near real time. Triggered by SQS messages, Snowpipe copies data from S3 as soon as they become available. Snowpipe also allows simple data transformation during the copy process. Given that Snowpipe is declarative and easy to use, it’s a good alternative compared to doing data transformation in stream processing. One important thing to note is that each event has its own stream processing application for S3 offload and its own Snowpipe. As a result, we can scale pipelines for each event individually and isolate the failures.
Working Towards a Self-Serve Platform
So far, we covered end-to-end data flows from clients to data warehouse, and here we want to discuss the operational aspect of Iguazu and see how we are making it a self-serve to reduce operational burdens. As you recall, to achieve failure isolation, each event in Iguazu has its own pipeline from Flink job to Snowpipe. This requires a lot more setup work and makes the operation a challenge. This diagram shows the complicated steps required to onboard a new event, including creation of the Kafka topic, schema registration, creation of a Flink stream processing job, and creation of the Snowflake objects. We really want to automate all these manual steps to improve operational efficiency. One challenge we face is how we can accomplish it under the infrastructure as code principle. At DoorDash, most of our operations, from creating Kafka topic, to setting up service configurations involve some pull request to different Terraform repositories and requires code review. How can we automate all these? To solve the issue, we first worked with our infrastructure team to set up the right pull approval process, and then automate the pull request using GitHub automation. Essentially, a GitHub app is created, where we can programmatically create and merge pull requests. We also leverage the Cadence workflow engine, and implemented the process as a reliable workflow. This pull automation reduced the event onboarding time from days to minutes. We get the best of both worlds, where we achieve automations, but also get versioning, all the necessary code reviews, and consistent state between our code and the infrastructure.
To get us one step closer to self-serve, we created high level UIs for a user to onboard the event. This screenshot shows a schema exploration UI, where users can search for a schema, using brackets, pick the right schema subject and version, and then onboard the event from there. This screenshot shows the Snowpipe integration UI where users can review and create Snowflake table schemas and Snowpipe schemas. Most importantly, we created a service called Minions that does the orchestration, and have it integrated with Slack so that we get notifications on each step it carries out. On this screenshot, you can see about 17 actions it has taken in order to onboard an event, including all the pull requests, launching the Flink job, and creating Snowflake objects.
Summary
Now that we covered the architecture and designs of all the major components in Iguazu, I’d like to once again review the four principles that I emphasized. The first principle is to decouple different stages. You want to use a Pub/Sub or messaging system to decouple data producers from consumers. This not only relieves the producers from back pressure, but also increases the data durability for downstream consumers. There are a couple of considerations you can use when choosing the right Pub/Sub or messaging system. First, it should be simple to use. I tend to choose the one that’s built with a single purpose and do it well. Secondly, it should have high durability and throughput guarantees. It should be highly scalable, even when there’s a lot of consumers and high data fanout. Similarly, stream processing should be decoupled from any downstream processes, including the data warehouse, using a cost-effective cloud storage or data lake. This guarantees that in case there’s any failures in the data warehouse, you don’t have to stop the stream processing job and you can always use the cheaper storage for backfill.
As a second principle, it pays to invest on the right stream processing framework. There are some considerations you can use when choosing the right stream processing framework. First, it should support multiple abstractions so that you can create different solutions according to the type of the audience. It should have integrations with sources and sinks you need, and support a variety of data formats like Avro, JSON, protobuf, or parquet, so that you can choose the right data format in the right stage of stream processing. The third principle is to create abstractions. In my opinion, abstraction is an important factor to facilitate the adoption of any complicated system. When it comes to the real time data infrastructure, there are a few common abstractions worth considering. For example, leveraging Kafka REST proxy, creating event API and serialization library, providing a DSL framework like Flink SQL, and providing high level UIs with orchestrations. Finally, you need to have fine-grained failure isolation and scalability. For that purpose, you should aim to create independent stream processing jobs or pipelines for each event. This avoids resource contention, isolates failures, and makes it easy to scale each pipeline independently. You also get the added benefits of being able to provide different SLAs for different events and easily attribute your costs at event level. Because you are creating a lot of independent services and pipelines to achieve failure isolation, it is crucial to create orchestration service to automate things and reduce the operational overhead.
Beyond the Architecture
Here are some final thoughts that go beyond the architecture. First, I find it important to build products using a platform mindset. Ad hoc solutions are not only inefficient, but also difficult to scale and operate. Secondly, picking the right framework and creating the right building blocks is crucial to ensure success. Once you have researched and find the sweet spots of those frameworks, you can easily create new products by combining a few building blocks. This dramatically reduces the time to building your products, and the effort to maintain the platform. I would like to use a Kafka REST proxy as an example. We use it initially for event publishing from internal microservices. When the time comes for us to develop a solution for mobile events, we found the proxy is also a good fit because it supports batching and JSON payload, which are important for mobile events. With a little investment, we made it work as a batch service, which save us a lot of development time.
Questions and Answers
Anand: Would you use a REST proxy if loss is not an option?
Wang: I mentioned that we added the extra capability to do asynchronous request processing. That is designed purely for analytical data, because it would introduce maybe very minor data loss, because we are not giving the actual broker acknowledgments back to the client. You can also use the REST proxy in a different way, where it would give precisely what the broker acknowledgment is. Basically, you’re adding a date hop in the middle, but client would know definitely whether this producing request is successful or not, or it can timeout, sometimes it can timeout. Let’s say, you send something to the proxy and the proxy crashed, and then you don’t hear anything back from the proxy, but on the client side, you will know it timed out, then you will try to do some kind of retry.
Anand: I’m assuming REST requests coming to the REST proxy, you accumulate it in some buffer, but you’re responding back to the REST clients if they’re mobile or whatever, that you’ve received the data, but it’s uncommitted. Therefore, you can do larger writes and get a higher throughput sense.
Wang: Yes, of course, that also had to be changed, if you are really looking for lossless data publishing. It’s not desirable to have that behavior.
Anand: Also curious about alternatives to Flink. One person is using Akka. They’re moving off Akka towards Flink, and they were wondering what your alternative is.
Wang: I haven’t used Akka before. Flink is the primary one that I use. I think Flink probably provides more data processing capability than Akka including all the abstractions it provides, the SQL support, and the fault tolerance built on top of Flink’s internal state. I would definitely recommend using these kinds of frameworks that provide these capabilities.
Anand: Also curious about alternatives to Flink. We used to use Airflow plus Beam on data flow to build a similar framework. Did your team evaluate Beam? Why choose Flink? We usually hear about Beam and Flink, because Beam is a tier above.
Wang: I think Beam does not add a lot of advantages in streaming.
Anand: Beam is just an API layer, and it can be used with Spark streaming or with Flink.
Wang: Unless you want to have the capability of being able to migrate your workload to a completely different stream processing engine, Beam is one of those choices you can use. I also know that some of the built-in functionalities on streaming parts and edges are not transferable to another. I think most likely they’re not. You are using certain built-in functionalities of those stream processing frameworks, which is not exposed with Beam. It’s actually hard to migrate to a different one. I think Beam is a very good concept, but in reality, I feel it’s actually more efficient to stick to the native stream processing framework?
Anand: It’s a single dialect if the two engines below have the same functionality, but the two engines are diverging and adding their own, so having a single dialect is tough.
How do you handle retries in the event processing platform? Are there any persistence to the problematic events with an eventual reprocessing?
Wang: The retries, I’m sure what is that context? In both the event publishing stage, and event processing stage or consuming stage, you can do retries. Let’s say in publishing stage, of course, when you try to publish something, the broker gives you a negative response, you can always retry.
Anand: What they’re saying is, how do you handle retries in the event processing platform? The next one is, if you have a bad message, do you persist these away, like in a dead letter queue, with an eventual reprocessing of it once it’s patched?
Wang: In the context of data processing or consuming the data, if you push something to the downstream and that failed, or you’re processing something that failed, you can still do a retry, but I think dead letter queue is probably the ultimate answer. That you can push the data back to a different Kafka topic, for example, and have the application consumed again from that dead letter queue. You can add a lot of controls on like, how many times you want to retry, and how long you want to keep retrying. I think dead letter queue is essential if you really want to minimize your data loss.
Anand: Apache Kafka is a popular choice, especially for real time event stream processing, I’m curious of presenter’s opinion on simpler event services such as Amazon SNS. How does one decide if you truly need something as powerful as Kafka, or perhaps a combination of SNS and SQS for added message durability? Could they be enough for a use case?
Wang: I think SNS as a queue has helped different kinds of use cases, they are more like point-to-point messaging system. Kafka on the other side, it’s really helped emphasize on streaming. For example, in Kafka, you always consume a batch of messages and your offset commit is on the base level batch, not individual messages. Kafka has another great advantage where you can have different consumers consume from the same queue or same topic and it will not affect each other. Data fanout is a very good use case of Kafka. Having data fanout is a little bit difficult to deal with, with SNS and SQS.
Anand: I’ve used SNS, SQS together. SNS is topic based, but if a consumer joins late, they don’t get historical data, so then you basically have like multiple consumer groups as SQS topics. Then each consumer group has to link to that SQS topic. What it didn’t work for, for me, is Kafka, when the producer wants a durable, it doesn’t matter how many consumer groups you add later, different apps. With SNS, SQS, you end up adding the SQS topic, or the SQS queue for another consumer group, but there’s no historical data. I think that’s the difference. Kafka supports that, SNS, SQS doesn’t actually solve that problem.
Are there any plans in exploring Pulsar to replace Kafka? What are your thoughts on Pulsar if you already explored it?
Wang: We actually explored Pulsar in my previous job at Netflix. At that time, Pulsar was not super mature, it still had some stability issues. In the end, I think Kafka is still simple to use. I think Pulsar has tried to do a lot of things. It’s tried to accomplish both, supporting stream processing and also supporting this point-to-point messaging. It tried to accommodate both. It becomes a bit more complicated or complex architecture, than Kafka. For example, I think Pulsar relies on another service or open source framework called BookKeeper, Apache BookKeeper. There’s multiple layers of services in between. We think that deployment installation operation could be a headache, so we choose Kafka, we want to stick with Kafka for its simplicity.
Anand: You have the option of doing go time versus directly hooked in. I think that option is only at publish time, like producer time.
See more presentations with transcripts