DataStax is working with the Google Cloud AI/ML Center of Excellence as part of the Built with Google AI program to enable Google Cloud’s generative AI offerings to improve the capabilities of customers using DataStax.
Jun 7th, 2023 10:07am by
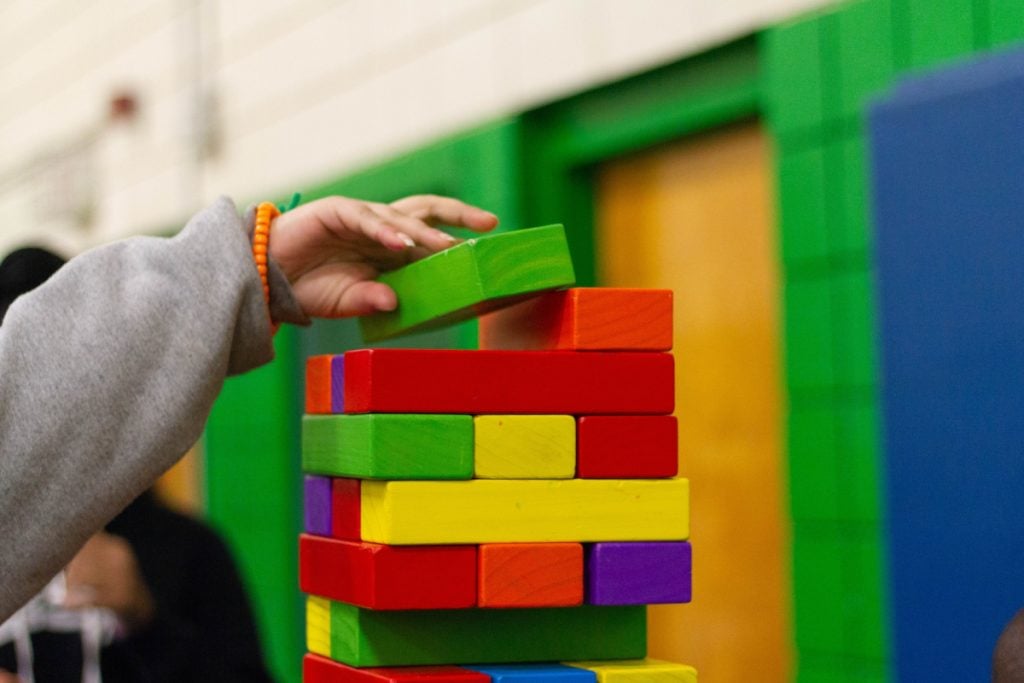
With so much data piling up everywhere, loaded database nodes are becoming a serious challenge for users to search faster and more accurately to find what they are seeking.
DataStax, which makes a real-time database cloud service built upon open source Apache Cassandra, announced today that its Database as a Service (DBaaS), Astra DB, now supports vector search. This is fast becoming an essential capability for enabling databases to provide long-term memory for AI applications using large language models (LLMs) and other AI use cases.
DataStax is working with the Google Cloud AI/ML Center of Excellence as part of the Built with Google AI program to enable Google Cloud’s generative AI offerings to improve the capabilities of customers using DataStax.
Vector search can be difficult to explain to non-mathematics-type people. It uses machine learning to convert unstructured data, such as text and images, into a numeric representation within the database called a vector. This vector representation captures the meaning and context of the data, allowing for more accurate and relevant search results. It also is able to recognize and connect similar vectors in the database within the context of the query in order to produce more accurate results.
Vector search is often used for semantic search, a type of search that looks for items that are related in meaning, rather than just those that contain the same keywords. For example, a vector search engine could be used to find songs that are similar to a user’s favorite song, even if they don’t share any of the same keywords.
‘Vector Search Is Magic’
“Vector search is magic because it understands what you meant vs. what you said (in a query),” DataStax CPO Ed Anuff told The New Stack. “The more complex a piece of content is, turning it into a vector becomes a much more efficient way of finding this similarity without having to try to guess which keywords are (exactly) right.
“Let’s imagine that I have a database of all of the articles you’ve written. The process of turning each one of your articles into a vector is done through an LLM (large language model), and it looks through the entirety of each article. It figures out what are the most important pieces of an article, and the vector that it produces gets to the essence of it in a concise way. For example, even though you might have used the word ‘Cassandra’ many times in an article, it knows the LLM when it transforms into the vector. It knows that your article is about an open-source database – not about the Cassandra constellation or a performance artist named Cassandra,” Anuff said.
Developers create vectors with simple API calls, and they query those vectors on simple API calls. “But they can now put this powerful capability to work. So that’s why vectorization is such a powerful aspect of this,” Anuff said.
Some of the benefits of using vector databases include:
- Scalability: They can scale to handle large amounts of data.
- Flexibility: They can be used to store and manage a variety of data types, including structured, unstructured and semi-structured data.
- Performance: They can provide high performance for queries on large datasets.
Vector search is also used for image search. In this case, the vectors represent the features of an image, such as its color, texture, and shape. This allows for more accurate and relevant image search results, such as finding images that are similar to a user-uploaded image.
DataStax is launching the new vector search tool and other new features via a NoSQL copilot — a Google Cloud Gen AI-powered chatbot that helps DataStax customers develop AI applications on Astra DB. DataStax and Google Cloud are releasing CassIO, an open source plugin to LangChain that enables Google Cloud’s Vertex AI service to combine with Cassandra for caching, vector search, and chat history retrieval.
Designed for Real-Time AI Projects
Coming on the heels of the introduction of vector search into Cassandra, the availability of this new tool in the pay-as-you-go Astra DB service is designed to enable developers to leverage the massively scalable Cassandra database for their LLM, AI assistant, and real-time generative AI projects, Anuff said.
“Vector search is a key part of the new AI stack; every developer building for AI needs to make their data easily queryable by AI agents,” Anuff said. “Astra DB is not only built for global scale and availability, but it supports the most stringent enterprise-level requirements for managing sensitive data including HIPAA, PCI, and PII regulations. It’s an ideal option for both startups and enterprises that manage sensitive user information and want to build impactful generative AI applications.”
Vector search enables developers to search by using “embeddings”; for example, Google Cloud’s API for text embedding, which can represent semantic concepts as vectors to search unstructured datasets, such as text and images. Embeddings are tools that enable search in natural language across a large corpus of data, in different formats, in order to extract the most relevant pieces of data.
New Capabilities in the Tool
In addition, DataStax has partnered with Google Cloud on several new capabilities:
- CassIO: The CassIO open source library enables the addition of Cassandra into popular generative AI SDKs such as LangChain.
- Google Cloud BigQuery Integration: New integration enables Google Cloud users to seamlessly import and export data from Cassandra into BigQuery straight from their Google Cloud Console to create and serve ML features in real time.
- Google Cloud DataFlow Integration: New integration pipes real-time data to and from Cassandra for serving real-time features to ML models, integrating with other analytics systems such as BigQuery, and real-time monitoring of generative AI model performance.
Goldman Sachs Research estimates that the generative AI software market could grow to $150 billion, compared to $685 billion for the global software industry.
Vector search is available today as a non-production use public preview in the serverless Astra DB cloud database. It will initially be available exclusively on Google Cloud, with availability on other public clouds to follow. Developers can get started immediately by signing up for Astra.
Created with Sketch.