Month: August 2023
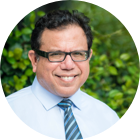
MMS • RSS
Posted on mongodb google news. Visit mongodb google news
MongoDB, a leading provider of database software, is scheduled to release its earnings data after the market closes on Thursday, August 31st, 2023. Analysts are expecting MongoDB to post earnings of $0.45 per share for the quarter. The company has set its second quarter guidance at $0.43-0.46 EPS and its full-year 2024 guidance at $1.42-1.56 EPS.
Investors are closely following MongoDB’s performance, with several institutional investors and hedge funds recently modifying their holdings of MDB. Vanguard Group Inc., for example, raised its stake in MongoDB by 2.1% in the first quarter and now owns 5,970,224 shares of the company’s stock worth $2,648,332,000. State Street Corp also increased its stake by 1.8% during the same period and now owns 1,386,773 shares valued at $323,280,000.
Another notable investor is 1832 Asset Management L.P., which saw an increase in its position in MongoDB by an astonishing rate of 3,283,771% during the fourth quarter. With an ownership of 1,018,000 shares worth $200,383,000 as a result of this growth spurt.
Geode Capital Management LLC also increased its position in shares of MongoDB by 3.8% during the first quarter and currently holds 967,289 shares valued at $225,174,000.
Additionally,Norges Bank acquired a new position in shares of MongoDB during the fourth quarter valued at approximately $147m.Notes from Bloomberg.com show that institutional investors own a significant majority (89.22%) of the company’s stock.
Several brokerages have recently shared their opinions on MDB stock as well.One notable example is Needham & Company LLC which increased their price objective from $250 to $430.Additionally,Capital One Financial began coverage on shares of MongoDB with an “equal weight” rating and a $396.00 price objective. Sanford C. Bernstein also increased their price target on shares of MongoDB from $257 to $424.
Overall, the stock has received positive ratings and an average target price of $378.09 from analysts based on data from Bloomberg.com, indicating a moderate buy consensus.
Investors wanting to learn more about MongoDB’s earnings can register for the company’s earnings conference call using the provided link.
In conclusion, all eyes are on MongoDB as it prepares to release its earnings data. With positive expectations from analysts and strong support from institutional investors, the future looks promising for this leading database software provider.
MongoDB Exceeds Earnings Expectations and Shows Promise for Future Growth
August 24, 2023 – MongoDB (NASDAQ:MDB) recently released its earnings results for the quarter ending June 1st. The company reported earnings per share (EPS) of $0.56, surpassing the consensus estimate of $0.18 by a significant margin of $0.38. Additionally, MongoDB’s revenue for the quarter was $368.28 million, exceeding the consensus estimate of $347.77 million. These positive financial results indicate strong performance and growth for the company.
However, it is worth noting that MongoDB had a negative net margin of 23.58% and a negative return on equity of 43.25%. Despite these challenges, the business’s revenue showed a promising increase of 29.0% on a year-over-year basis compared to the same quarter in the previous year when it posted ($1.15) earnings per share.
As for its stock performance, shares of MongoDB opened at $370.74 on Thursday, reflecting its robust market cap of $26.17 billion. With a price-to-earnings ratio of -79.39 and a beta of 1.13, investors are closely monitoring its movements in the market.
Analysts have expressed their expectations for MongoDB’s future earnings per share with an average estimate of $-3 for both the current fiscal year and the next fiscal year.
The company’s growth potential has attracted several institutional investors and hedge funds who have made recent modifications to their holdings in MDB stock. Vanguard Group Inc., for instance, increased its stake in MongoDB by 2.1% during the first quarter, while State Street Corp raised its stake by 1.8%. Other notable institutional investors include 1832 Asset Management L.P., Geode Capital Management LLC, and Norges Bank.
In terms of insider trading activities, Director Dwight A. Merriman sold 3,000 shares on June 1st at an average price of $285.34, generating a total transaction value of $856,020. Merriman currently holds 1,219,954 shares in the company. Another insider, CRO Cedric Pech, sold 360 shares on July 3rd at an average price of $406.79.
Over the past 90 days, insiders have collectively sold 102,220 shares of MongoDB stock worth $38,763,571. Insiders hold a 4.80% stake in the company.
To summarize, MongoDB’s recent earnings report exceeded expectations and demonstrated growth in revenue. Its stock performance has been notable with a significant market cap. Institutional investors and hedge funds have shown increased interest in the company. However, it is important to monitor the impact of negative net margin and return on equity on future performance. Additionally, it will be interesting to see how insiders’ selling activities influence market sentiment towards MongoDB as we move forward into the next fiscal year.
Reference:
MongoDB (NASDAQ:MDB) – Earnings Release & Outlook
https://www.analystratings.com/industry/MongoDB_Inc_/
Article originally posted on mongodb google news. Visit mongodb google news
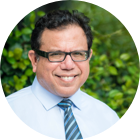
MMS • Felix GV
Article originally posted on InfoQ. Visit InfoQ
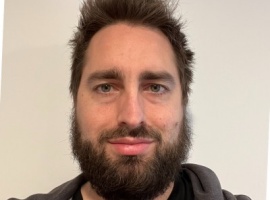
Transcript
GV: My name is Felix. I am a Committer on the Venice Database Project, which was open sourced last year. I’ve been doing data infrastructure since 2011, the majority of which I spent at LinkedIn. You can find some of my thoughts on my blog at philosopherping.com. I’d like to cite one of the nice quotes from Winston Churchill. The nice thing about having the modern data stack, data mesh, real-time analytics, and feature stores is you always know exactly which database to choose for the job. Of course, Winston didn’t say that. As far as I know, no one ever said that. In fact, I hear people saying the opposite. Year after year, they say, the database landscape is getting more confusing. There are just too many options to pick from. Here’s a bunch of data systems, most of which you’re probably familiar with, or at least heard about. What I want to do in this talk is give you a new lens to look through this landscape, and categorize these systems, because within this bunch of systems hiding in plain sight, are several derived data systems. I think it’s useful if we understand the differences between primary data and derived data, and then which systems are optimized for each. Then we can make better choices about our architectures.
Outline
First, I want to lay the groundwork for this discussion by talking about, what is primary data. Then I want to use that to contrast it with derived data, and what is that? Finally, the third section is about four derived data use cases that we have at LinkedIn, including real-time analytics, graph, ML feature storage, and search. For each of those, I’m going to cover the specialized system that we use to serve these use cases at scale at LinkedIn.
What Is Primary Data?
What is primary data? Some related terms, you could say primary data is source of truth data. You could say a primary data system is a system of records. Here’s an attempt at defining primary data. Primary data is that which is created or mutated as a direct result of a user action. Here, I’m putting the emphasis on direct. Hang on to that, it will make sense when we contrast with derived data. What are we looking for in a primary data system? Typically, we’ll want that system to provide durability. That means when you write the data into the primary data system, you expect the data to be persistent. You don’t want it to be only in memory, and then you have a power outage and you lose that, or something like that. You would want it to be on durable medium, like spindles or flash. You might also want the system to guard against machine failures, in which case, you would benefit from the system having replication. Besides machine failures, you might also care about human failures. I deployed a version of my application that corrupts the data, let’s roll back to yesterday’s backup. Backups may be useful to guard against that, or other scenarios, of course.
Finally, perhaps the most important is that, usually, primary data systems benefit from strong consistency. I say usually, and I’m putting some caveats on these things, because none of these are actually hard requirements. You could choose to put your primary data anywhere you’d like. It turns out that in many cases, these are useful characteristics. For strong consistency in particular, the thing is, for user experience reasons, we want to have that. Imagine that the user is editing their account details in your system. If the user submits their edit to the account detail information, and then they reload the page, or maybe they even load it on a different device that they have, they would expect to see the edit that was just acknowledged to be there. You achieve that by having strong consistency.
Some examples of primary data systems include the following. We’ve got the traditional relational databases of the transactional flavor, like OLTP databases, like MySQL, Postgres, and others. You could also store primary data in a NoSQL offering, like Cassandra or Mongo. Of course, nowadays, the cloud is all the rage, so Cosmos DB, DynamoDB, and other similar offerings could certainly host primary data as well. One last thing about primary data is, how does the data escape that system that it’s in? Because sometimes we want to get access to that data elsewhere. That’s critical to our talk here. One way is to ETL a whole snapshot of the database to another location, like your Hadoop grid, or maybe some cloud storage like S3 or Azure Blob Store. Another way is to listen in on the changes as they happen. We call that Change Data Capture. At LinkedIn, we’re pretty big on Change Data Capture, and we built Databus more than a decade ago, then replaced it with our next-gen Change Data Capture system called Brooklin. This one we open sourced a few years ago. It supports data capture out of MySQL, Oracle, and others. There are other options as well, like Debezium is a pretty popular Change Data Capture system as well nowadays.
What Is Derived Data?
Once the primary data escapes the system it’s in, somehow, then it might become derived data. What does it mean? Here are some related terms. Derived data may be processed, or computed, reformatted, massaged, inferred from other data. You can pick and choose any of these terms. Sometimes, many of these things are going to happen at the same time. Then the output of this transformation basically is the derived data, and it is derived from some other data. Therefore, because this data is processed, it is indirect. Earlier, I told you to hang on to the notion that primary data is a result of a direct action of the user, derived data may also be indirectly tied to a user action. That’s an important distinction. It’s not direct. There’s been some processing hub in between. Maybe it’s been loaded into a Hadoop grid or some object storage in order to get processed in batch over there, or maybe we’ve been doing some stream processing on the Change Data Capture. Sometimes we’re doing both of these at the same time, like a Lambda architecture.
There are many different types of derivation of the data. I’m going to go over many examples of how data can be derived for specialized use cases throughout the rest of the talk. I think it’s useful to subcategorize derivation into two flavors, physical and logical. What I mean by that is physical derivation doesn’t change the content of the data, but it changes how it’s organized. For example, you may have the table in your database, which is organized in row-oriented format, which makes it ideal for looking up a single row or a small amount of rows, and retrieving all of that quickly. Then, if you want to do some analytics on this data, that will still work, the row-oriented table will still work. It could be more optimal to reformat the same data into column-oriented format. That would be an example of massaging the data into a different structure. The data is the same, but it’s optimized for another use case.
A logical derivation is where the data itself changes. The input is different from the output. It could be a little different or very different. An example of a logical derivation, where the data is just a little different is maybe you’re putting the data in lowercase. Or maybe you’re stripping out the plural suffix to the word when you find it. These are like tokenization techniques that you may find in a search use case, for example. The data looks pretty similar, but it’s a little bit transformed. That makes it more useful for this type of use case. It could also have a logical derivation that makes it completely unrecognizable. For example, in machine learning, if you have a training job that takes as input some text that’s been generated by users, and then maybe also some user actions about whether they liked a certain thing, or whether they shared it, and so on, all of these input signals. Then, the output of the ML training job is just a bunch of floating-point values. Those may be the weights of a neural net, or maybe some scores about a prediction of the likelihood that the user will click on a given article, if it was presented to them. It had some relation to the input, but it’s completely unrecognizable from our perspective. That would be an example of a logical derivation that changes the data a lot.
We covered in the last section, what are the qualities that a primary data system may benefit from. Let’s do the same for derived data. Given that derived data is processed, it’s great if the derived data system has first class support for ingesting these processed outputs, so ingesting batch data, ingesting stream data. There wouldn’t be much of a point in having the derived data system if it didn’t have some specialized capability. If the derived data system does exactly the same stuff that your primary data system does, then you might as well just have your primary data system. Either these are capabilities that are not found at all in the primary data system, or maybe they’re there but not as efficiently implemented in the primary data system. That brings me to the last point that oftentimes a derived data system is going to offer some superior performance in some niche application.
The thing which we typically do not find in a derived data system is strong consistency. That’s in opposition to the primary data systems. If you think about it, again, from the user standpoint, the user experience, typically, it does not matter for a derived data system to have strong consistency. Because the data in it is not appearing there as a direct result of a user action. It’s happening after some background process or asynchronous process has done something with the data. By the time the data comes out of the stream processor that generated it, it doesn’t matter too much at that point if the last mile, like the interaction between the stream processor and the derived data system, whether that is strongly consistent or not. It doesn’t really matter. Because the whole thing that happened before that was already asynchronous anyway. Therefore, from the user’s perspective, the whole thing is asynchronous, regardless of whether the last mile is strongly consistent or not. Therefore, if you don’t have strong consistency, then you don’t have read after write semantics either. It’s important when using those systems to be cognizant of that and to make sure we don’t misuse the systems by assuming they provide something that they do not.
Here are a few examples of open source derived data systems. There are search indices like Solr and Elasticsearch. Real-time analytics systems like Pinot, Druid, ClickHouse, and some ML feature storage systems like Venice. I want to linger for a little moment on these two search indices, Solr and Elasticsearch. I want to linger here to highlight some of the subtlety about consistency, because these systems, Solr and Elasticsearch, both offer a REST endpoint for writing documents into the index. You have a REST endpoint, you post to it, and then it gets acknowledged as a 200 OK, something like that. You could easily assume that because the REST endpoint acknowledged your request, that’s it, your data is there. It’s persistent and readable. If you do a query against the search index, you will find the document that you just put in there. That would be only halfway true. When the POST comes back successfully, your data is durable, but it is not yet readable. That’s an important distinction. Why is it not durable? Because these search indices have a periodic process that will go in and do what they call a refresh or a commit. Depending on the system, the terminology is different, but it’s the same idea. Then, after this refresh of the index happens, then the data that was persisted after the last refresh, then that data becomes readable also.
You could force the system to give you strong consistency. You could say, I’ll post my document, and then I’ll force a refresh. That would make it look like the search index has strong consistency. It would truly have strong consistency at this point. What I want to get at is that this is not really a production grade way to use this system. If you were doing this trick, where you post your document and then force a refresh, you could theoretically use Solr or Elasticsearch as your system of record, this could be your source of truth. When you look at the best practices for these systems, you see that this is not quite desirable. When you look at the best practices, they tell you, actually, don’t even bother sending one document at a time, because that’s awfully inefficient for this system. Instead, accumulate some batch of documents. Then once you reach some sweet spot, like maybe 5000 documents, or it depends on the size of each document, of course, then at that point only, send me the batch. That is the more efficient way of using this system. If you send me too much documents to index, the system may kick it back. It may say, I’m overloaded, you got to back off, and try again later.
You see that these best practices don’t align very well with this being a system of record. In fact, the production grade way to run this system is to put a buffer in front. Imagine that you have Kafka as your buffer, you write documents, one at a time into Kafka. Then you have some connecting application like Kafka Connect, that will accumulate those documents into batches of the right size, and then post those batches to the search index at the rate the search index prefers. If the search index kicks it back, then the connecting application will take care of the backoff, retries, and all of the logic to make this reliable and scalable and efficient. The interesting thing here is, although Solr and Elasticsearch, which are quite old, maybe more than a decade old, although those systems were built with a REST endpoint that makes it look like you’re going to get strong consistency out of this thing, in practice, it’s not really standalone. You ought to use something else as part of the mix. If you look at more recent derived data systems, like Venice and Pinot, you see that those systems were built from the ground up to use a buffer. Essentially, their write API is a buffer. You give a buffer to those systems, or they have a buffer built in even. You see the evolution of these derived data systems, like we cut to the chase. In the newer systems, instead of dumping the complexity on the user to stand up all this infrastructure around it, the system is just built in to work in the production grade way.
Derived Data Use Cases at LinkedIn – Real-Time Analytics
Speaking of production grade, I want to now make this even more concrete by talking about four categories of derived data use cases that we have at LinkedIn, because each of those has between hundreds and thousands of unique use cases. Let’s get started with real-time analytics. For that we use Pinot, which ingests a bunch of data from Hadoop and Kafka. At LinkedIn, we’re old school, so we still use Hadoop. We probably have one of the biggest Hadoop deployments on the planet at this point. In your mind’s eye, whenever you see this slide, you can substitute Hadoop for S3 or Azure Blob Store, whatever favorite thing you have for storing batch data. Then, similarly, instead of Kafka, you could substitute like Kinesis, or Pulsar, or whatever, as the streaming input. An example of use case served by Pinot is, who viewed my profile. Here you can see the count of unique viewers per week in the past 90 days, and you have various toggles at the top to filter the data. Pinot is great for that. You can ingest events data, like profile view events, for example, and index it inside Pinot. That works great because Pinot is perfect for immutable data, meaning that not that the whole dataset is immutable, but rather, each record in the dataset is immutable. You can append a new record, but you cannot change old records. It’s also great for time-series data. If you have a metric that comes out once per minute, and then maybe there’s a bunch of different metrics that have different dimensions attached to them, and each of them comes out once a minute, this would be also a great fit. Once you have that data inside Pinot, you can do expressive SQL queries on top of that, like filtering, aggregation, GROUP BY, ORDER BY. This is like what I just showed in the example use case.
In terms of types of derivation that are supported by Pinot, one of them is to transcode the data from a bunch of formats. The data coming in from the stream into Pinot could be in Avro format. That’s what we use at LinkedIn, or it could be in protobuf, or Thrift format, or others. The data that comes from the batch source could be in ORC, Parquet. Pinot will transcode all of that into its native representation of each of the data types. Then, it’s going to take those bits of data and encode them using column-oriented techniques. For example, dictionary encoding is, if you have a column of your table that has a somewhat low cardinality, then instead of encoding each of the values itself, you can instead assign an ID for each of the values and encode the IDs, which if the IDs are smaller than the value, then you have a space saving. Then, you can also combine that with run-length encoding, which means that instead of recording every single value of the column, you encode, essentially, how many times each of the values have it. For example, I had a value 10, 100 times, and then I had value 12, 150 times, and so on. This makes the data even smaller, especially if the column is sorted, but even if it’s not sorted, you can still leverage run-length encoding. Depending on the shape of the data, it could yield some pretty big space savings.
Why does that matter? In the case of Pinot, for many of the use cases, we want to have the whole dataset in RAM in order to support very fast queries. How big the data is, directly affects cost, because RAM is costly. Furthermore, the less data there is to scan the faster our scans of the data can go. Minimizing space improves both storage cost and query performance. Besides these physical derivation techniques, Pinot also supports a variety of logical derivations. You can, for example, project some of the columns of the data and throw away the rest. You can also filter out some of the rows of the data. If you have some complex types in the data, as you could find in Avro or protobuf, like some struct or nested record type of situation in the data, then you can flatten that out, which makes it easier to query in SQL. Pinot is open source. If you want to find information about it, there’s a bunch of great information on the web. The official documentation is really good, you can find it at docs.pinot.apache.org. There’s also a bunch of videos, interviews, podcasts, and so on about Pinot. I’m sure you’ll find information if you’re looking for it.
Use Case – Graph
Our next system is our graph database, which is called LIquid. It also ingests data from Hadoop and Kafka, in our case. I want to quickly clarify, though, graph databases are not inherently derived data systems. There are graph databases, which are intended to be primary data systems. For example, Neo4j from my understanding is designed to be a primary data system, it provides strong consistency and so on. In our case, what we wanted to do with this graph data is to join data coming out of a bunch of other databases. We have a bunch of source of truth databases for each of our datasets, and we want to join all of those into the economic graph, and then run queries on that economic graph. For us, it made sense to build LIquid as a derived data system. One of the usages is finding out the degrees of separation between the logged in member and any other member of the site. Next to each name, you see, first, second, third. This is the degree of separation between you and another member. You see that sprinkled across the whole site, like next to each name, but it also affects things you don’t see. There are visibility rules, for example, that are based on the degree of separation. This affects a bunch of things throughout the whole site.
Another use case served by LIquid is mutual connections. If you look at someone’s profile, you can see the mutual connections, which are essentially my first-degree connections who also happen to be first-degree connections of the other person. That is achieved by a graph traversal query in LIquid. Another query you can do with LIquid is your connections that work at a company. This means, essentially, give me all of my first-degree connections who work at a given company. There’s a bunch more. These graph traversal queries can be used to express even more complex things. For example, I could say, give me all of my second-degree connections that have gone to one of the same schools that I went to, and where the time that we went there overlaps. Essentially, we have a chance of having been classmates. You could do these things with joins in SQL. In some cases, that works great. If the query is a bit complex, if it has self joins, or recursive joins, you can do these things in SQL, but sometimes it starts getting pretty hairy. A graph-oriented language is another tool in the toolkit, and sometimes it makes it much easier to express some of these queries. In some cases, also makes it much more performant as well.
What kinds of derivation are supported in LIquid? We transcode the data from Avro and other formats, as is the case with Pinot, because LIquid has its own representation of the data. We convert the data to triples. That means subject, predicate, object. An example of a triple is member ID connected to member ID. Another example might be member ID works at company ID, or member ID studied at school ID. There can be a variety of things that are represented. Essentially, this is the whole data model. You can represent everything via triples. It’s a very simple, but also very powerful abstraction for the data. This allows us to join together a bunch of disparate datasets that exist throughout our ecosystem. In terms of logical derivation, as data gets ingested into LIquid, you can filter out if there are some records you don’t want in there. You can also do other kinds of massaging to the data to make it more amenable to being queried in the graph.
Use Case – ML Feature Storage
For ML features, we store them primarily in Venice. Again, Venice ingests data from Hadoop and Kafka. Venice was open sourced last year. It is a project I work on. One of the use cases that we serve with Venice is people you may know. Actually, people you may know is served by both LIquid and Venice. The flow of data in this use case goes roughly like this. First of all, we query LIquid to find out what are all the second-degree connections that the logged in user might know. That’s a huge set, way too many to present. There is some early filtering happening right there inside LIquid based on some heuristics that are part of the economic graph. What comes out of that is a set of 5000 candidates. These are people you potentially might know. Five thousand is still a lot, so we want to dwindle that down further. At that point, we turn around and query Venice. For each of the 5000 members, we’re going to query a bunch of ML features that relate to them, and we’re also going to push down some ML feature computation inside Venice. There are embeddings, which are arrays of floats representing the member. We do things like dot product or cosine similarity, these types of vector math inside Venice, and return just a result of that computation. These features that are either fetched directly or computed all come out of Venice, and then are fed into a machine learning model that will do some inference. For each of the members that are part of the candidate set, we will infer a score, which is how likely is it that you might know this person. Then we’ll rank that and return the top K. That’s why you see very few recommendations but hopefully highly relevant ones.
The feed is another example where we’re going to take the posts that have been created by your network. That’s going to be a huge set, again, so we cannot present all of that. We want to do some relevance scoring, for which we retrieve a bunch of ML features out of Venice, and then pass that into an ML inference model, and score each of the posts, rank that, and return the top K most relevant ones. Both of these, people you may know and the feed, are what we call recommender use cases. Venice is great for loading lots of data quickly and frequently. The output of ML training jobs is very high volume. The ML engineers want to refresh that data frequently, maybe once a day or several times a day, the whole dataset. Or, alternatively, they may want to stream in the changes as soon as they happen. Once the data is in Venice, the queries that are supported on the data are fairly simple comparatively to the other systems we looked at. There is NoSQL, or graph traversal, or anything like that, it’s essentially primary key lookups. There is a little more than that, like you can project some fields out of the document, or you can push down some vector math, like I said earlier, to be executed inside the servers, colocated with the data for higher performance. Essentially, these are the types of capabilities that are useful for ML inference workloads.
In terms of the types of derivation supported in Venice, it’s basically all physical. We reorganize the data in certain ways, but we don’t really change the logical content of the data. I mentioned earlier that ML training is a type of logical derivation, but it’s not happening directly inside Venice. It’s happening in some processing framework upstream of Venice, before it lands in Venice. In terms of what happens inside Venice itself, we transcode data from other formats. If the data is already in Avro, then we keep it in Avro, inside Venice. Then we sometimes compress the data if it’s amenable to that, like if there’s a lot of repetition in the data, we have found that using Zstandard compression is extremely effective. What we do there is, because a lot of our data comes from batch pushes, we have all the data there in a Hadoop grid, which is a great place to inspect the data and train a dictionary on it. Then we train a dictionary that is optimized for that specific dataset and push that alongside the data. That allows us to leverage the repetitiveness that exists across records in the dataset. In the most extreme cases, we’ve seen datasets get compressed 40x, a huge compression ratio. In most cases, it’s not that good. Still, it gives us a lot of savings on this system, which is primarily storage bound, lots of data stored in it, and lots of data getting written to it.
Then we put the data in our storage engine, which is RocksDB. We use both of RocksDB’s formats, block based, which is optimized for when the dataset is larger than RAM and you have an SSD for the rest, for the cold data. Then we have the plain table format, which is optimized for the datasets that are all in RAM. Depending on the use case, we choose one or the other. Then, besides that, we join datasets from various sources, like similar idea as in LIquid. We also have a type of derivation that we call collection merging. What that means is, in your Venice table schema, you can define that some column is actually a collection type, like a map or a set. Then, you can add elements into that, one at a time, and Venice will do the merging for you. You can also remove elements in the same way without needing to know what the rest of the collection is. If you want to find out more about Venice, we’ve got our documentation up at venicedb.org. We’ve got all our community resources there, like our Slack instance, our blog posts, and stuff like that, if you want to learn more.
Use Case – Search
The last system I want to cover is for our search use cases. That system is called Galene. Again, it ingests data from batch and streams. An example is the search box you see at the top. If you type Ashish, in there, you’ll see a bunch of people named Ashish, and a few companies also named Ashish, in there. If you typed the right keyword, you could also find schools or pages and so on. This is what we call a federated search. It queries many search verticals, and then coalesces all of that into a single view. Galene is great for retrieving documents that match some predicate that you’re interested in. Then scoring the relevance of each of those documents, which is achieved via arbitrary code and ML models that run directly inside the searchers. Then, ranking those documents by their relevance score, and returning the top K most relevant ones. This is essentially what Galene does. The way that it achieves that is it has two kinds of data structures, which are essentially the way that it physically derives the data coming into it. Actually, there are two flavors of forward index, one for the data that is present in the documents, like maybe the documents are tagged with the author of the document, the date it was modified. These are characteristics that may be useful as part of the search or as part of the ranking. These are stored in a forward index, which looks like the left-hand table here. For doc ID, here is some associated value, like the content or maybe some metadata about the document, like the author, or timestamp, and other things. There’s another flavor of forward index, which is called Da Vinci, which is used for ML features. Da Vinci is actually a subcomponent of Venice, the previous system I talked about. Venice is actually a component inside Galene, and the Da Vinci part is open source. You can reuse that in your own applications if you’d like.
The inverted index is implemented as Lucene, which is the same technology underpinning Solr and Elasticsearch, at least from my understanding. In addition to Lucene, we’ve implemented our own proprietary special sauce on top of that to make it more performant. The inverted index looks roughly like the table on the right-hand side. For each of the tokens that are present in the corpus, for each of these tokens, we store a list of the document IDs that are known to contain these tokens. What do I mean by token? Tokenization is a type of logical derivation that helps make the data easier to search. The simplest form of tokenization could be viewed as a physical derivation. It’s just like split the text by spaces, and each of the words are going to be a token. That can work, but it’s a little simplistic and it doesn’t work super great, because that those tokens are not clean enough. In order to make the tokens more useful for searching, we’re going to do additional things, like we’re going to strip out punctuation, we’ll put them all in lowercase. We might substitute words for some root word, like let’s say, if the language has a notion of gender, of course, all the languages have plural and singular, these are all variants of the same root word. We may index on the root word instead. Then, also, similarly, transform at query time, whatever the user provides into the root word, and then it matches inside the inverted index. You could also do synonyms. All of these allow the tokens to make it more likely that you get a hit when you search.
Here’s a high-level architecture of what the Galene system looks like. This is a simplified architecture. Essentially, you got a request coming from the application hitting a broker node, that will then essentially split the request across a bunch of shards, which are searchers. Each of these searchers has the three data structures I talked about, the inverted index for doing the document retrieval, then the forward index that contains other metadata about the documents that we may want to use for scoring, and ML features that we also use for scoring that are stored in Da Vinci. The reason we have Da Vinci in there is it supports a higher rate of refresh of the data, which our ML engineers prefer. We’ve got all of this inside the searcher, and then the searcher returns the top K most relevant documents that it found, to the broker. The broker coalesces all of those results, does another round of top K, and then returns that to the application.
Conclusion – To Derive or Not to Derive?
First of all, small scale is easier. If your data is of a small scale, maybe you can do everything I just talked about in a single system. You could do everything in Postgres, for example. Why bother having more moving parts, if one will do the job? Even if you’re at a large scale, you could still take advantage of this principle, by trying out an experiment at smaller scale. Let’s say you don’t yet have a dedicated solution for search, and you want to add a search functionality to your business, but you feel like you don’t have the business justification for it. Maybe you can build a very quick and dirty prototype of search on top of your relational database, expose that to just 1% or a 10th of a percent of your users, gather some usage data on that. Maybe that solution is sufficiently scalable for that fraction of your users, and it gives you some useful input about whether that’s a good product market fit. It can help you justify, now that this is demonstrably useful, let’s build a large-scale version of this with a specialized system.
Another way to say what I just said is, use what works until it doesn’t. Make your stack as simple as possible, but no simpler. That’s important, because sometimes having fewer moving parts is what makes your stack simpler, but sometimes fewer moving parts is actually what makes it more complicated. At some point, you reach a level of workload where doing everything all in one system is a liability. If you have a transactional workload that gets negatively impacted by some analytical workload that happens in the same box, that may not be a good thing. In that case, you may think about splitting it into separate systems. Maybe you still choose the same tech on both sides here, maybe Postgres is your transactional, and Postgres is your analytical, and they’re just connected by some replication in between. Maybe that works great, at least for a while. Then, since you have that separation anyway, you could also start feeding that replication stream into a more specialized solution as well. Keep your options open. Most importantly, avoid sunk cost fallacy. Just because your architecture worked five years ago, doesn’t mean it’s still the best today. Your workload constantly grows and changes, so you have to adapt to that and not be beholden to the past design choice. That’s difficult, but try to stay agile and adapt as much as you can.
See more presentations with transcripts
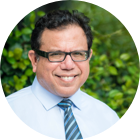
MMS • RSS
Posted on mongodb google news. Visit mongodb google news
US Bancorp DE raised its holdings in MongoDB, Inc. (NASDAQ:MDB – Free Report) by 24.0% during the first quarter, according to the company in its most recent Form 13F filing with the Securities & Exchange Commission. The fund owned 2,740 shares of the company’s stock after acquiring an additional 530 shares during the period. US Bancorp DE’s holdings in MongoDB were worth $639,000 at the end of the most recent quarter.
Other hedge funds and other institutional investors have also recently bought and sold shares of the company. GAM Holding AG acquired a new stake in shares of MongoDB during the first quarter valued at about $5,394,000. Clearstead Advisors LLC acquired a new stake in shares of MongoDB during the first quarter valued at about $36,000. Y Intercept Hong Kong Ltd acquired a new stake in shares of MongoDB during the first quarter valued at about $2,591,000. Banco Bilbao Vizcaya Argentaria S.A. boosted its stake in shares of MongoDB by 24.1% during the first quarter. Banco Bilbao Vizcaya Argentaria S.A. now owns 1,369 shares of the company’s stock valued at $312,000 after purchasing an additional 266 shares in the last quarter. Finally, Brighton Jones LLC acquired a new stake in shares of MongoDB during the first quarter valued at about $1,270,000. Institutional investors own 89.22% of the company’s stock.
Insiders Place Their Bets
In other news, Director Dwight A. Merriman sold 6,000 shares of the firm’s stock in a transaction on Friday, August 4th. The stock was sold at an average price of $415.06, for a total value of $2,490,360.00. Following the completion of the transaction, the director now directly owns 1,207,159 shares of the company’s stock, valued at $501,043,414.54. The transaction was disclosed in a filing with the Securities & Exchange Commission, which is available through this hyperlink. In related news, Director Dwight A. Merriman sold 6,000 shares of MongoDB stock in a transaction on Friday, August 4th. The stock was sold at an average price of $415.06, for a total transaction of $2,490,360.00. Following the completion of the sale, the director now owns 1,207,159 shares of the company’s stock, valued at $501,043,414.54. The transaction was disclosed in a filing with the Securities & Exchange Commission, which is available through this link. Also, Director Dwight A. Merriman sold 1,000 shares of MongoDB stock in a transaction on Tuesday, July 18th. The shares were sold at an average price of $420.00, for a total value of $420,000.00. Following the sale, the director now directly owns 1,213,159 shares of the company’s stock, valued at approximately $509,526,780. The disclosure for this sale can be found here. Insiders sold 102,220 shares of company stock valued at $38,763,571 over the last ninety days. 4.80% of the stock is currently owned by insiders.
Analyst Upgrades and Downgrades
MDB has been the subject of several analyst reports. Guggenheim lowered shares of MongoDB from a “neutral” rating to a “sell” rating and raised their price target for the stock from $205.00 to $210.00 in a report on Thursday, May 25th. They noted that the move was a valuation call. KeyCorp upped their target price on MongoDB from $372.00 to $462.00 and gave the company an “overweight” rating in a research report on Friday, July 21st. Citigroup increased their price target on MongoDB from $363.00 to $430.00 in a report on Friday, June 2nd. Morgan Stanley increased their price target on MongoDB from $270.00 to $440.00 in a report on Friday, June 23rd. Finally, Mizuho increased their price target on MongoDB from $220.00 to $240.00 in a report on Friday, June 23rd. One equities research analyst has rated the stock with a sell rating, three have issued a hold rating and twenty have assigned a buy rating to the stock. Based on data from MarketBeat.com, the company has an average rating of “Moderate Buy” and a consensus target price of $378.09.
Read Our Latest Analysis on MongoDB
MongoDB Stock Performance
NASDAQ:MDB opened at $360.75 on Friday. The company has a debt-to-equity ratio of 1.44, a current ratio of 4.19 and a quick ratio of 4.19. The business has a fifty day simple moving average of $391.10 and a two-hundred day simple moving average of $297.69. MongoDB, Inc. has a 1 year low of $135.15 and a 1 year high of $439.00. The company has a market capitalization of $25.46 billion, a P/E ratio of -77.25 and a beta of 1.13.
MongoDB (NASDAQ:MDB – Get Free Report) last released its earnings results on Thursday, June 1st. The company reported $0.56 earnings per share (EPS) for the quarter, topping analysts’ consensus estimates of $0.18 by $0.38. MongoDB had a negative return on equity of 43.25% and a negative net margin of 23.58%. The business had revenue of $368.28 million during the quarter, compared to the consensus estimate of $347.77 million. During the same period last year, the business posted ($1.15) earnings per share. The business’s revenue for the quarter was up 29.0% on a year-over-year basis. On average, analysts expect that MongoDB, Inc. will post -2.8 earnings per share for the current year.
MongoDB Company Profile
MongoDB, Inc provides general purpose database platform worldwide. The company offers MongoDB Atlas, a hosted multi-cloud database-as-a-service solution; MongoDB Enterprise Advanced, a commercial database server for enterprise customers to run in the cloud, on-premise, or in a hybrid environment; and Community Server, a free-to-download version of its database, which includes the functionality that developers need to get started with MongoDB.
See Also
Want to see what other hedge funds are holding MDB? Visit HoldingsChannel.com to get the latest 13F filings and insider trades for MongoDB, Inc. (NASDAQ:MDB – Free Report).
This instant news alert was generated by narrative science technology and financial data from MarketBeat in order to provide readers with the fastest and most accurate reporting. This story was reviewed by MarketBeat’s editorial team prior to publication. Please send any questions or comments about this story to contact@marketbeat.com.
Before you consider MongoDB, you’ll want to hear this.
MarketBeat keeps track of Wall Street’s top-rated and best performing research analysts and the stocks they recommend to their clients on a daily basis. MarketBeat has identified the five stocks that top analysts are quietly whispering to their clients to buy now before the broader market catches on… and MongoDB wasn’t on the list.
While MongoDB currently has a “Moderate Buy” rating among analysts, top-rated analysts believe these five stocks are better buys.
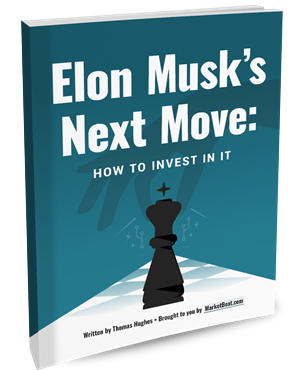
Wondering when you’ll finally be able to invest in SpaceX, StarLink or The Boring Company? Click the link below to learn when Elon Musk will let these companies finally IPO.
Article originally posted on mongodb google news. Visit mongodb google news
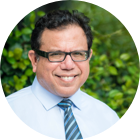
MMS • RSS
Posted on mongodb google news. Visit mongodb google news
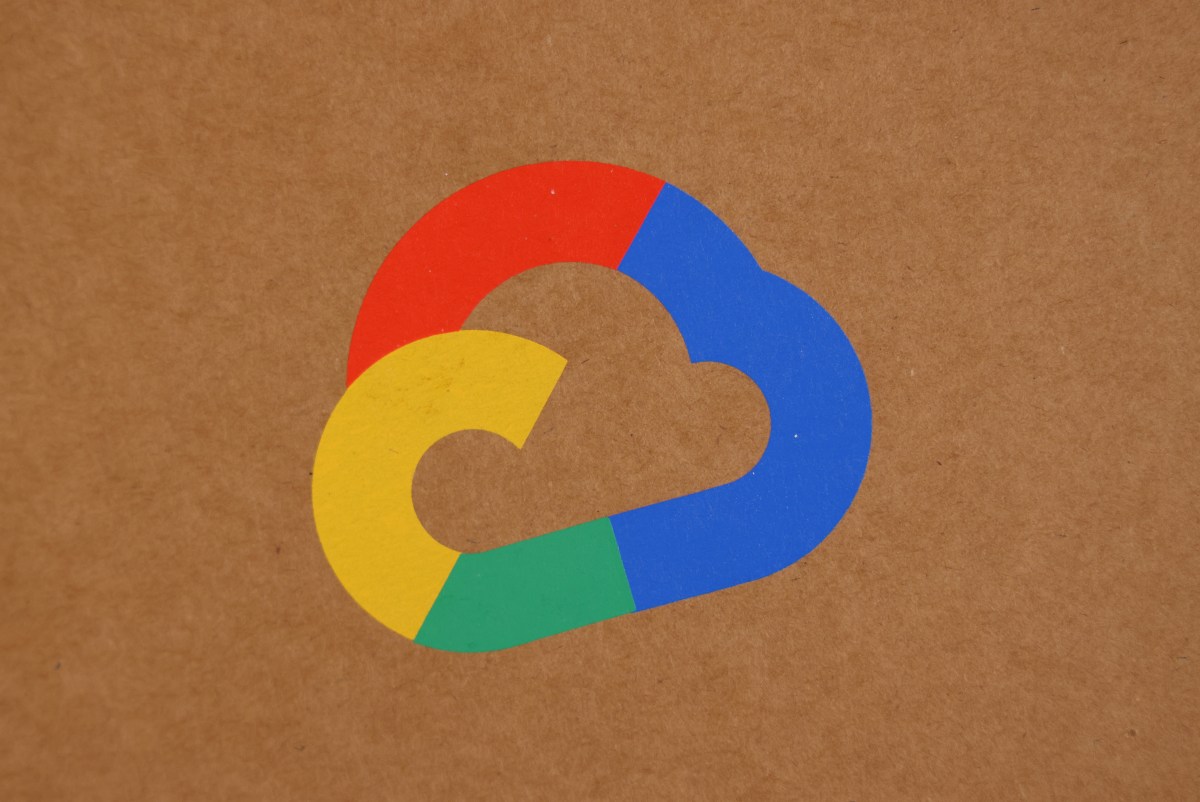
Google Cloud’s annual Next event is happening in San Francisco next week (and we’ll be on the ground to cover all of the announcements), but ahead of the event, Google Cloud today put a spotlight on its partner ecosystem. It’s no secret that in its early days, Google’s cloud efforts were somewhat hindered by its inexperience in working with large enterprises and the consultancies, professional service firms and partners they rely on. But when Thomas Kurian took on the CEO role in 2019, he set Google Cloud on a path to build out that expertise and an open partner ecosystem, which is now starting to pay off.
In a blog post today, Google puts its focus on its AI and data services partners like Confluent, DataRobot, MongoDB, Redis, Datastax, Elastic and Neo4j. Given the current hype around generative AI, that’s perhaps no surprise, but this is also the story of Google Cloud learning how to sell to enterprises and embracing more traditional ways of selling to them.
“We have support from the top all the way to the bottom — top-down from Thomas [Kurian] — on us creating the most open ecosystem we can,” Stephen Orban, the VP for Migrations, Independent Software Vendors (ISVs), and Marketplace at Google Cloud, told me. “We build our products and services on open standards and embrace that from a product development perspective, but we’re also making sure we have the most open ecosystem […] where we have ISVs and partners who both complement and extend our platform that we lean into quite heavily — and we view it as a strategic lever for our growth.” When the ISVs grow, Google Cloud grows, after all.
One muscle Google Cloud had to build over the years is co-selling with its partners. In its early days, Google hoped that its technology would essentially sell itself, but these days, the Google Cloud marketplace is stocked with solutions from hundreds of vendors, many of which have close relationships with Google (and the other hyperclouds) to market and sell their products together.
Alan Chhabra, MongoDB’s executive VP for worldwide partners and international sales, noted that he thinks this change is in part due to Kurian’s experience at Oracle. “I was very excited because I knew of Thomas’s history and experience and accomplishments at Oracle. He was an engineering-first CEO but really understood go-to-market. I consider him very similar to MongoDB CEO Dev Ittycheria. They know how to cross engineering with sales better than most CEOs in the world and I felt like Google needed that at the time — because it is a balance.”
He explained that MongoDB quickly established a close business and engineering partnership with Google after Kurian took over. “We had a phone call and in that call, Thomas explained that he wanted to be the cloud that embraced open source,” Chhabra said.
It’s worth noting that at the time, many open-source businesses, including MongoDB, were wrestling with how to react to the likes of AWS releasing their own services based on existing open source projects. It was at Kurian’s first Cloud Next in 2019 that the company announced that it would deeply integrate products from open-source companies like Confluent, DataStax, Elastic, InfluxData, MongoDB, Neo4j and Redis Labs — a list that looks quite similar to the partners the company is highlighting today. While Google didn’t spell it out at the time, that announcement was very much meant to draw a line between how Google Cloud and AWS were going to work with open-source companies.
That approach is now paying off for Google and its partners. “I remember that moment because they were there for the open-source community,” Chhabra said. “They were there for database and data companies like MongoDB and we remember that and loyalty is important to us. Since then, we’ve had phenomenal growth between the two companies, especially in verticals like retail. And we’ve got a commitment with Google and they’ve committed items to us.”
Data is obviously the lifeblood of AI and even though Microsoft, thanks in large parts to its OpenAI partnership, is often seen as a leader, many enterprises specifically use Google Cloud because of its AI services like Vertex AI.
DataRobot CEO Debanjan Saha, who previously worked at AWS and Google, echoed what I heard from other partners as well: “if you look at Google, for a long time, they were a consumer company. The cloud is probably the first enterprise business for them. And there’s a little bit of learning that Google is going through. I think they made a huge amount of progress in the last three or four years, especially after Thomas Kurian took charge of the cloud business. But still, relatively speaking, Microsoft, for example, has been doing that longer than Google has, but I think Google is learning very fast and the gap is closing.”
He noted areas where Google could still improve, including the processes for co-marketing, co-selling, and marketplace execution. “I know that they’re working really hard on those,” Saha added.
Google’s Orban noted that the company often looks for partners who complement its services. “That’s all about recruiting the ISV, making sure they’re well-architected on Google Cloud with their solution. Most ISVs, I would say, lean towards the SaaS model now. They’re building their SaaS in such a way that it is optimized for going to market with us. It’s about building the joint go-to-market together.”
These days, those discussions also often become about generative AI and how Google can help enterprises leverage it. “GenAI has overnight become a CEO- and boardroom-level conversation, where cloud migrations sometimes are traditionally a CIO-level agenda,” Orban said. “So overnight, we’ve seen the demand from customers from very high up in the organization. And we’re in a very fortunate position where a lot of customers have come to trust and recognize Google as a brand for artificial intelligence.”
He noted that while Google has its own foundational models, it still partners with the likes of Anthropic and Cohere. “Our strategy is quite explicit: to build the most open and innovative AI ecosystem on Earth,” he said. “If our customers want to work with a particular partner, we want to be there to support them and make sure that they have a great experience on Google Cloud.”
Article originally posted on mongodb google news. Visit mongodb google news
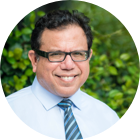
MMS • RSS
Posted on mongodb google news. Visit mongodb google news
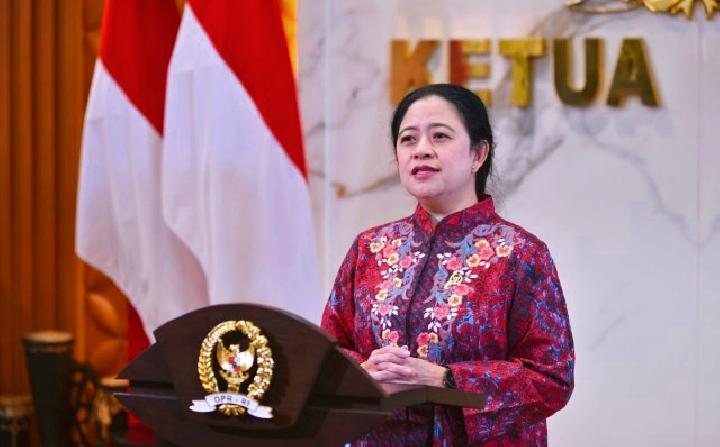
INFO NASIONAL – Ketua DPR RI Puan Maharani menyoroti nasib korban Pemutusan Hubungan Kerja (PHK) yang tak kunjung mendapat pekerjaan baru. Ia pun menekankan pentingnya kolaborasi Pemerintah dengan pelaku industri untuk membantu mengatasi permasalah pengangguran di Indonesia.
“Pemerintah dapat memberikan bantuan kepada pekerja yang terkena PHK, seperti pelatihan keterampilan dan bantuan mencari kerja. Perusahaan dapat menciptakan lapangan kerja dengan membuka investasi dan mengembangkan teknologi baru,” kata Puan dalam keterangan tertulisnya, Rabu, 23 Agustus 2023.
“Upaya seperti ini bukan hanya memberikan harapan baru bagi ribuan pekerja yang kehilangan pekerjaannya, tetapi juga memberikan dampak positif terhadap pemulihan ekonomi nasional,” tutur Politisi Fraksi PDI-Perjuangan ini.
Puan pun mendukung upaya Pemerintah melalui Kementerian Ketenagakerjaan (Kemenaker) yang melakukan pendekatan kepada para pengusaha di Indonesia untuk turut memperhatikan para pekerja yang terkena PHK. Terutama perusahaan-perusahaan yang tengah bertumbuh secara positif.
“Komitmen Negara untuk menjamin hak warganya mendapat pekerjaan dan penghidupan layak harus didukung dari peran para pelaku industri. Pendekatan yang baik ini kami harapkan dapat menghasilkan kolaborasi untuk mengurangi pengangguran di Tanah Air,” kata Puan.
Menurut data Kemenaker, jumlah korban PHK di Indonesia pada tahun 2022 sebanyak 25.114 orang. Sedangkan pada tahun sebelumnya mencapai 127.085 orang. Puan mendorong Pemerintah untuk mengintensifkan upaya menurunkan angka korban PHK pada tahun 2023 ini.
“Meskipun ada penurunan jumlah beberapa tahun terakhir, tapi penyerapan tenaga kerja korban PHK masih kurang maksimal. Upaya Pemerintah membentuk tenaga kerja yang dibutuhkan pelaku usaha juga dapat menekan jumlah pengangguran,” ucapnya.
Lebih lanjut, Puan menyoroti peningkatan pertumbuhan ekonomi Indonesia yang berpotensi dapat menciptakan lapangan kerja baru. Hal ini lantaran perusahaan yang tumbuh sehat akan memerlukan produksi lebih banyak barang maupun jasa untuk memenuhi permintaan yang meningkat.
Scroll Untuk Melanjutkan
“Peluang ini harus dimanfaatkan Pemerintah agar pertumbuhan ekonomi dapat menciptakan lapangan kerja baru, khususnya untuk korban PHK. Pekerja yang Menjadi korban PHK akan memiliki lebih banyak kesempatan untuk mendapatkan pekerjaan baru di perusahaan yang sedang berkembang,” kata Puan.
Di sisi lain, mantan Menko PMK itu mengapresiasi upaya Pemerintah untuk mewujudkan lingkungan kerja yang aman dari segala bentuk kekerasan seksual. Puan pun mendukung ketegasan Pemerintah yang mendorong para pelaku industri mengimplementasikan Kepmenaker Nomor 88 Tahun 2023 tentang Pedoman Pencegahan dan Penanganan Kekerasan Seksual di Tempat Kerja.
“Untuk menekan jumlah kekerasan seksual di tempat kerja harus ada komitmen dari pelaku usaha. Pemerintah juga harus rajin melakukan sosialisasi terkait aturan tersebut, yang dibarengi dengan upaya dari manajemen perusahaan memberi edukasi kepada karyawannya soal pencegahan tindakan kekerasan seksual,” tuturnya.
Puan berharap pimpinan perusahaan menciptakan lingkungan kerja yang mendukung kesetaraan gender. Termasuk juga mendukung pekerja perempuan yang kerap mengalami diskriminasi di tempat kerja.
“Jika ada oknum karyawan yang melakukan tindakan pelecehan terhadap perempuan, perusahaan harus menjadi garda terdepan dalam menentukan sikap dan pemberian sanksi tegas. Sehingga, akan menjadi pelajaran berharga bagi seluruh karyawan,” kata Puan.
Puan juga mengajak seluruh pemangku kepentingan, baik Pemerintah, perusahaan, serikat pekerja, dan masyarakat, untuk bekerja sama dalam mewujudkan lingkungan kerja yang aman dan berkelanjutan bagi perempuan.(*)
Article originally posted on mongodb google news. Visit mongodb google news
Pictet Asset Management SA Increases Stake in MongoDB, Inc. while Insiders Sell Shares
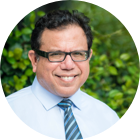
MMS • RSS
Posted on mongodb google news. Visit mongodb google news
Pictet Asset Management SA, a prominent asset management firm, has recently increased its stake in MongoDB, Inc. by 2.6% during the first quarter of this year. According to their Form 13F filing with the Securities and Exchange Commission (SEC), Pictet Asset Management SA now owns 23,681 shares of MongoDB, worth approximately $5,521,000. This increase in holdings reflects the confidence that Pictet Asset Management SA has in the company’s performance.
In other news related to MongoDB, Chief Revenue Officer Cedric Pech recently sold 360 shares of the company’s stock on July 3rd at an average price of $406.79 per share. This transaction amounted to a total value of $146,444.40. Following this sale, Pech now directly holds 37,156 shares of MongoDB stock valued at approximately $15,114,689.24. This transaction was legally disclosed through a filing with the SEC.
Another significant sale was made by Director Dwight A. Merriman on August 4th when he sold 6,000 shares of MongoDB stock at an average price of $415.06 per share, totaling $2,490,360.00 in value. Following this transaction, Merriman now directly owns 1,207,159 shares in the company with a valuation of $501,043,414.54.
It is important to note that over the past ninety days alone, insiders have collectively sold 102,220 shares of company stock with an approximate value of $38,763,571. Such insider activity indicates possible shifts in sentiment or outlook among those closely involved with the company’s operations.
MongoDB is a well-known organization within the database technology sector and offers innovative solutions for enterprises seeking to manage large volumes of data efficiently and effectively. The company’s success is evident through its growing interest from investors such as Pictet Asset Management SA.
Investors and industry analysts should closely monitor these developments and insider activities as they may provide valuable insights into the future performance and prospects of MongoDB. For investors, it is essential to consider multiple factors before making any investment decisions, including macroeconomic conditions, market trends, and company-specific fundamentals. Additionally, conducting thorough research and analysis will allow for a more informed decision-making process.
In conclusion, Pictet Asset Management SA’s increased stake in MongoDB indicates their confidence in the company’s potential. The recent insider sales by individuals such as Cedric Pech and Dwight A. Merriman may also offer important insights into the direction of the company. As always, it is crucial for investors to conduct their due diligence and carefully evaluate all available information before making any investment decisions.
Investor Holdings and Analyst Sentiment Propel MongoDB’s Growth in the Technology Sector
MongoDB (NASDAQ: MDB), a leading technology company specializing in database management, has seen significant changes in its investor holdings according to recent reports. Several large investors have made modifications to their stake in the company, with Raymond James & Associates increasing its stake by 32.0% during the first quarter. PNC Financial Services Group Inc. also grew its holdings by 19.1% during the same period.
Raymond James & Associates now owns 4,922 shares of MongoDB’s stock worth $2,183,000 after purchasing an additional 1,192 shares in the last quarter. Similarly, PNC Financial Services Group Inc. now holds 1,282 shares valued at $569,000 after acquiring an additional 206 shares.
MetLife Investment Management LLC recently entered into a new position with MongoDB during the first quarter, investing approximately $1,823,000. Panagora Asset Management Inc. also increased its holdings by 9.8%, owning 1,977 shares valued at $877,000 after acquiring an additional 176 shares during the period.
In addition to these changes among individual investors, Vontobel Holding Ltd., a prominent institutional investor, grew its holdings in MongoDB by an impressive 100.3%. They now own 2,873 shares worth $1,236,000 after acquiring an additional 1,439 shares.
These modifications contribute to the overall landscape of ownership within MongoDB as hedge funds and other institutional investors now own a significant majority (89.22%) of the company’s stock.
Analyst reports have closely followed these investor activities and their impact on MongoDB’s market value and prospects for growth. Piper Sandler recently upped their price objective on the stock from $270.00 to $400.00 in a report published on June 2nd.
Truist Financial also revised their price objective upwards from $365.00 to $420.00 in a report issued on June 23rd. JMP Securities gave an optimistic outlook, upping the price objective from $400.00 to $425.00, and rating the stock as “outperform” in a report published on July 24th.
Meanwhile, Tigress Financial significantly increased their price objective from $365.00 to $490.00 in a report released on June 28th. KeyCorp’s research analysts also provided a positive view, raising their projections from $372.00 to $462.00 and granting the stock an “overweight” rating in their report on July 21st.
With regards to overall analyst sentiment, Bloomberg.com reports that the average rating for MongoDB stands at “Moderate Buy,” accompanied by an average target price of $378.09.
As of August 24, 2023, shares of NASDAQ:MDB opened at $370.74, with a 50-day moving average price of $391.60 and a two-hundred day moving average price of $297.24.
MongoDB boasts strong financials with a current ratio and quick ratio both standing at 4.19. The company has demonstrated resilience in its debt management with a debt-to-equity ratio of 1.44.
Over the past year, MongoDB’s stock has experienced considerable volatility, ranging from its low point at $135.15 to its peak at $439 per share.
The company currently has a market capitalization of approximately $26.17 billion and operates with a forward price-to-earnings (P/E) ratio of -79.39 while maintaining a beta of 1.13.
In its latest earnings results for the quarter ending June 1st, MongoDB reported earnings per share (EPS) of $0.56—surpassing analysts’ consensus estimate by an impressive margin of $0.38.
Furthermore, MongoDB achieved significant revenue growth during this period, with revenue amounting to $368.28 million, an increase of 29.0% compared to the same period the previous year.
Overall, market analysts forecast that MongoDB will post -2.8 EPS for the current fiscal year—an indication of positive expectations for the company’s financial performance moving forward.
As MongoDB continues to attract investors and analysts’ attention, its unique approach in database management technology positions it favorably within the market. The company’s ability to adapt and innovate has proven critical in driving growth and capturing investor confidence, making it an intriguing prospect for those seeking long-term investment opportunities within the technology sector.
Article originally posted on mongodb google news. Visit mongodb google news
MongoDB Inc. Sees Surge in Options Trading and Growing Interest from Institutional Investors
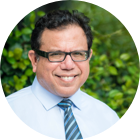
MMS • RSS
Posted on mongodb google news. Visit mongodb google news
MongoDB, Inc. (NASDAQ:MDB), a leading technology company, experienced an unusual surge in options trading on Wednesday, indicating heightened investor interest. A staggering 36,130 call options were purchased on the company, marking a significant increase of 2,077% compared to the typical volume of 1,660 call options.
This surge in trading activity reflects a growing sentiment among stock traders regarding MongoDB’s potential for future growth and profitability. It suggests that investors are increasingly optimistic about the company’s prospects and are willing to take positions accordingly.
In addition to the increased options trading, several hedge funds and institutional investors have made changes to their positions in MongoDB recently. Jennison Associates LLC notably boosted its position in the company by a tremendous 101,056.3% during the second quarter. The firm now holds 1,988,733 shares of MongoDB’s stock valued at approximately $817,350,000.
Furthermore, other institutional investors like 1832 Asset Management L.P., Price T Rowe Associates Inc., Renaissance Technologies LLC, and Norges Bank have also significantly increased their stakes in MongoDB over recent quarters. Collectively, hedge funds and institutional investors now own approximately 89.22% of the company’s outstanding shares.
It is worth noting that Director Dwight A. Merriman sold a considerable amount of MongoDB stock recently. On August 4th alone, he sold 6,000 shares at an average price of $415.06 per share. Following this transaction, he retains ownership of 1,207,159 shares valued at $501,043,414.54 as disclosed in filings with the Securities & Exchange Commission.
Looking into MDB stock performance on Thursday morning following these developments , it opened at $370.74 – indicating steady performance relative to previous closing prices . Over the past year MDB shows immense market volatility with a fifty-two week low of $135.15 and a fifty-two week high of $439.00.
From an earnings perspective, MongoDB reported strong results in its latest financial quarter, which ended on June 1st. The company’s earnings per share (EPS) stood at $0.56 for the quarter, surpassing analysts’ consensus estimates by a significant margin of $0.38. MongoDB’s positive performance was further reinforced by a 29% increase in revenue to $368.28 million during the same period.
While the company’s return on equity and net margin have been negative recently, its overall financial performance remains promising due to substantial revenue growth. Analysts are cautiously optimistic about MongoDB’s future prospects and forecast a projected EPS of -2.8 for the current fiscal year.
In conclusion, MongoDB Inc.’s recent surge in options trading and increased interest from institutional investors reflect growing confidence in the company’s potential for future success and profitability. Despite some negative financial indicators, MongoDB’s strong quarterly results indicate its resilience and ability to generate substantial revenue growth. As always, investors should exercise caution and conduct thorough research before making any investment decisions based on market trends or news developments surrounding individual companies.
MongoDB Exceeds Analyst Expectations with Strong Q2 Earnings
August 24, 2023 – MongoDB, a leading technology company, recently announced its earnings results for the quarter ending June 1st. The report revealed that the company exceeded analysts’ expectations with earnings per share (EPS) of $0.56, surpassing the consensus estimate of $0.18 by an impressive $0.38.
Despite a negative return on equity of 43.25% and a negative net margin of 23.58%, MongoDB reported revenue of $368.28 million for the quarter, which exceeded the consensus estimate of $347.77 million. This represents a significant increase of 29.0% in revenue compared to the same quarter in the previous year.
Analysts predict that MongoDB will post -2.8 earnings per share for the current fiscal year, indicative of a challenging period for the company.
In response to these results, various equities analysts have shared their opinions on MDB shares. William Blair reaffirmed an “outperform” rating on shares of MongoDB, expressing confidence in the company’s future performance. VNET Group also reissued a “maintains” rating on shares of MongoDB, further supporting positive sentiment towards the company.
Capital One Financial recently initiated coverage on MongoDB and assigned an “equal weight” rating along with a price objective of $396.00 for the company. Needham & Company LLC also lifted their price objective on MongoDB from $250.00 to $430.00, highlighting their optimism regarding its prospects.
Overall, twenty analysts have given MongoDB a buy rating, while three assign it as a hold rating and one analyst has rated it as a sell stock.Outlook
As reported by Bloomberg, MongoDB currently holds a consensus rating of “Moderate Buy” among analysts, with an average price target of $378.09.
Though these findings point to robustness in parts within MDB’s business model and successful strides towards profitability in certain areas such as revenue growth, MongoDB does face challenges in terms of negative return on equity and net margin. It remains to be seen how the company will address these concerns moving forward.
Investors and stakeholders are advised to keep a close eye on MongoDB’s progress as it navigates through this complex landscape. With ongoing developments within the technology sector, it is crucial for companies like MongoDB to adapt and innovate in order to maintain a competitive edge.
Please note that the information provided in this article is based on recent reports and analyst ratings and may be subject to change as more data becomes available. Investors are encouraged to conduct their research before making any investment decisions based on this information.
About MDB
MongoDB, traded on NASDAQ under the ticker MDB, is a leading technology company known for its innovative database platform. The company offers flexible and scalable solutions that empower organizations to develop applications with unparalleled speed and agility. With its robust cloud offerings, MongoDB continues to shape the future of data management in a rapidly evolving technological landscape.
Article originally posted on mongodb google news. Visit mongodb google news
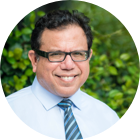
MMS • RSS
Posted on mongodb google news. Visit mongodb google news
Pictet Asset Management SA lifted its stake in shares of MongoDB, Inc. (NASDAQ:MDB – Free Report) by 2.6% in the 1st quarter, according to its most recent Form 13F filing with the Securities and Exchange Commission (SEC). The fund owned 23,681 shares of the company’s stock after purchasing an additional 598 shares during the quarter. Pictet Asset Management SA’s holdings in MongoDB were worth $5,521,000 at the end of the most recent quarter.
Several other large investors have also modified their holdings of MDB. Raymond James & Associates raised its stake in shares of MongoDB by 32.0% during the first quarter. Raymond James & Associates now owns 4,922 shares of the company’s stock worth $2,183,000 after purchasing an additional 1,192 shares during the last quarter. PNC Financial Services Group Inc. grew its holdings in MongoDB by 19.1% during the first quarter. PNC Financial Services Group Inc. now owns 1,282 shares of the company’s stock worth $569,000 after acquiring an additional 206 shares during the period. MetLife Investment Management LLC acquired a new position in MongoDB during the first quarter worth $1,823,000. Panagora Asset Management Inc. grew its holdings in MongoDB by 9.8% during the first quarter. Panagora Asset Management Inc. now owns 1,977 shares of the company’s stock worth $877,000 after acquiring an additional 176 shares during the period. Finally, Vontobel Holding Ltd. grew its holdings in MongoDB by 100.3% during the first quarter. Vontobel Holding Ltd. now owns 2,873 shares of the company’s stock worth $1,236,000 after acquiring an additional 1,439 shares during the period. Hedge funds and other institutional investors own 89.22% of the company’s stock.
Wall Street Analysts Forecast Growth
MDB has been the topic of a number of recent analyst reports. Piper Sandler upped their price objective on MongoDB from $270.00 to $400.00 in a report on Friday, June 2nd. Truist Financial upped their price objective on MongoDB from $365.00 to $420.00 in a report on Friday, June 23rd. JMP Securities upped their price objective on MongoDB from $400.00 to $425.00 and gave the stock an “outperform” rating in a report on Monday, July 24th. Tigress Financial upped their price objective on MongoDB from $365.00 to $490.00 in a report on Wednesday, June 28th. Finally, KeyCorp upped their price objective on MongoDB from $372.00 to $462.00 and gave the stock an “overweight” rating in a report on Friday, July 21st. One research analyst has rated the stock with a sell rating, three have assigned a hold rating and twenty have issued a buy rating to the stock. According to MarketBeat.com, the stock presently has an average rating of “Moderate Buy” and an average target price of $378.09.
Read Our Latest Stock Analysis on MongoDB
MongoDB Stock Up 2.4 %
Shares of NASDAQ:MDB opened at $370.74 on Thursday. The stock’s 50-day moving average price is $391.60 and its two-hundred day moving average price is $297.24. The company has a current ratio of 4.19, a quick ratio of 4.19 and a debt-to-equity ratio of 1.44. MongoDB, Inc. has a 12-month low of $135.15 and a 12-month high of $439.00. The firm has a market cap of $26.17 billion, a price-to-earnings ratio of -79.39 and a beta of 1.13.
MongoDB (NASDAQ:MDB – Get Free Report) last issued its quarterly earnings results on Thursday, June 1st. The company reported $0.56 earnings per share (EPS) for the quarter, topping analysts’ consensus estimates of $0.18 by $0.38. MongoDB had a negative return on equity of 43.25% and a negative net margin of 23.58%. The firm had revenue of $368.28 million for the quarter, compared to analyst estimates of $347.77 million. During the same period last year, the company earned ($1.15) EPS. The firm’s quarterly revenue was up 29.0% on a year-over-year basis. On average, research analysts forecast that MongoDB, Inc. will post -2.8 EPS for the current year.
Insiders Place Their Bets
In other news, CRO Cedric Pech sold 360 shares of MongoDB stock in a transaction dated Monday, July 3rd. The stock was sold at an average price of $406.79, for a total value of $146,444.40. Following the transaction, the executive now directly owns 37,156 shares of the company’s stock, valued at approximately $15,114,689.24. The transaction was disclosed in a filing with the Securities & Exchange Commission, which is accessible through the SEC website. In other news, CRO Cedric Pech sold 360 shares of MongoDB stock in a transaction dated Monday, July 3rd. The stock was sold at an average price of $406.79, for a total value of $146,444.40. Following the transaction, the executive now directly owns 37,156 shares of the company’s stock, valued at approximately $15,114,689.24. The transaction was disclosed in a filing with the Securities & Exchange Commission, which is accessible through the SEC website. Also, Director Dwight A. Merriman sold 6,000 shares of MongoDB stock in a transaction dated Friday, August 4th. The shares were sold at an average price of $415.06, for a total value of $2,490,360.00. Following the completion of the transaction, the director now directly owns 1,207,159 shares in the company, valued at $501,043,414.54. The disclosure for this sale can be found here. In the last ninety days, insiders have sold 102,220 shares of company stock valued at $38,763,571. Insiders own 4.80% of the company’s stock.
MongoDB Profile
MongoDB, Inc provides general purpose database platform worldwide. The company offers MongoDB Atlas, a hosted multi-cloud database-as-a-service solution; MongoDB Enterprise Advanced, a commercial database server for enterprise customers to run in the cloud, on-premise, or in a hybrid environment; and Community Server, a free-to-download version of its database, which includes the functionality that developers need to get started with MongoDB.
Recommended Stories
Receive News & Ratings for MongoDB Daily – Enter your email address below to receive a concise daily summary of the latest news and analysts’ ratings for MongoDB and related companies with MarketBeat.com’s FREE daily email newsletter.
Article originally posted on mongodb google news. Visit mongodb google news
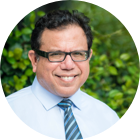
MMS • RSS
Posted on mongodb google news. Visit mongodb google news
MongoDB, Inc. (NASDAQ:MDB – Get Free Report) was the target of unusually large options trading on Wednesday. Stock traders purchased 36,130 call options on the company. This is an increase of 2,077% compared to the typical volume of 1,660 call options.
Insiders Place Their Bets
In other MongoDB news, Director Dwight A. Merriman sold 6,000 shares of the firm’s stock in a transaction on Friday, August 4th. The stock was sold at an average price of $415.06, for a total value of $2,490,360.00. Following the completion of the sale, the director now directly owns 1,207,159 shares of the company’s stock, valued at $501,043,414.54. The transaction was disclosed in a document filed with the Securities & Exchange Commission, which is accessible through this link. In other news, Director Dwight A. Merriman sold 6,000 shares of the firm’s stock in a transaction on Friday, August 4th. The stock was sold at an average price of $415.06, for a total value of $2,490,360.00. Following the completion of the transaction, the director now directly owns 1,207,159 shares of the company’s stock, valued at $501,043,414.54. The transaction was disclosed in a filing with the Securities & Exchange Commission, which is available through this hyperlink. Also, Director Dwight A. Merriman sold 1,000 shares of the firm’s stock in a transaction on Tuesday, July 18th. The stock was sold at an average price of $420.00, for a total value of $420,000.00. Following the completion of the transaction, the director now directly owns 1,213,159 shares of the company’s stock, valued at $509,526,780. The disclosure for this sale can be found here. Insiders sold 102,220 shares of company stock valued at $38,763,571 over the last ninety days. 4.80% of the stock is currently owned by insiders.
Institutional Inflows and Outflows
A number of hedge funds and other institutional investors have recently made changes to their positions in the company. Jennison Associates LLC boosted its position in shares of MongoDB by 101,056.3% in the 2nd quarter. Jennison Associates LLC now owns 1,988,733 shares of the company’s stock worth $817,350,000 after purchasing an additional 1,986,767 shares in the last quarter. 1832 Asset Management L.P. boosted its position in shares of MongoDB by 3,283,771.0% in the 4th quarter. 1832 Asset Management L.P. now owns 1,018,000 shares of the company’s stock worth $200,383,000 after purchasing an additional 1,017,969 shares in the last quarter. Price T Rowe Associates Inc. MD boosted its position in shares of MongoDB by 13.4% in the 1st quarter. Price T Rowe Associates Inc. MD now owns 7,593,996 shares of the company’s stock worth $1,770,313,000 after purchasing an additional 897,911 shares in the last quarter. Renaissance Technologies LLC boosted its position in shares of MongoDB by 493.2% in the 4th quarter. Renaissance Technologies LLC now owns 918,200 shares of the company’s stock worth $180,738,000 after purchasing an additional 763,400 shares in the last quarter. Finally, Norges Bank bought a new position in MongoDB in the 4th quarter valued at approximately $147,735,000. Hedge funds and other institutional investors own 89.22% of the company’s stock.
Analyst Upgrades and Downgrades
A number of equities analysts have recently commented on MDB shares. William Blair reaffirmed an “outperform” rating on shares of MongoDB in a report on Friday, June 2nd. VNET Group reissued a “maintains” rating on shares of MongoDB in a research note on Monday, June 26th. Capital One Financial assumed coverage on MongoDB in a research note on Monday, June 26th. They set an “equal weight” rating and a $396.00 price objective for the company. Needham & Company LLC lifted their price objective on MongoDB from $250.00 to $430.00 in a research note on Friday, June 2nd. Finally, 22nd Century Group reissued a “maintains” rating on shares of MongoDB in a research note on Monday, June 26th. One equities research analyst has rated the stock with a sell rating, three have assigned a hold rating and twenty have given a buy rating to the company. According to MarketBeat, the company currently has a consensus rating of “Moderate Buy” and a consensus price target of $378.09.
Get Our Latest Research Report on MDB
MongoDB Stock Up 2.4 %
MDB stock opened at $370.74 on Thursday. MongoDB has a fifty-two week low of $135.15 and a fifty-two week high of $439.00. The business’s 50 day moving average price is $391.60 and its two-hundred day moving average price is $297.24. The company has a debt-to-equity ratio of 1.44, a quick ratio of 4.19 and a current ratio of 4.19. The company has a market capitalization of $26.17 billion, a price-to-earnings ratio of -79.39 and a beta of 1.13.
MongoDB (NASDAQ:MDB – Get Free Report) last posted its earnings results on Thursday, June 1st. The company reported $0.56 earnings per share for the quarter, topping analysts’ consensus estimates of $0.18 by $0.38. MongoDB had a negative return on equity of 43.25% and a negative net margin of 23.58%. The firm had revenue of $368.28 million for the quarter, compared to the consensus estimate of $347.77 million. During the same quarter in the prior year, the business earned ($1.15) EPS. The business’s revenue for the quarter was up 29.0% on a year-over-year basis. As a group, analysts forecast that MongoDB will post -2.8 earnings per share for the current fiscal year.
About MongoDB
MongoDB, Inc provides general purpose database platform worldwide. The company offers MongoDB Atlas, a hosted multi-cloud database-as-a-service solution; MongoDB Enterprise Advanced, a commercial database server for enterprise customers to run in the cloud, on-premise, or in a hybrid environment; and Community Server, a free-to-download version of its database, which includes the functionality that developers need to get started with MongoDB.
See Also
This instant news alert was generated by narrative science technology and financial data from MarketBeat in order to provide readers with the fastest and most accurate reporting. This story was reviewed by MarketBeat’s editorial team prior to publication. Please send any questions or comments about this story to contact@marketbeat.com.
Before you consider MongoDB, you’ll want to hear this.
MarketBeat keeps track of Wall Street’s top-rated and best performing research analysts and the stocks they recommend to their clients on a daily basis. MarketBeat has identified the five stocks that top analysts are quietly whispering to their clients to buy now before the broader market catches on… and MongoDB wasn’t on the list.
While MongoDB currently has a “Moderate Buy” rating among analysts, top-rated analysts believe these five stocks are better buys.
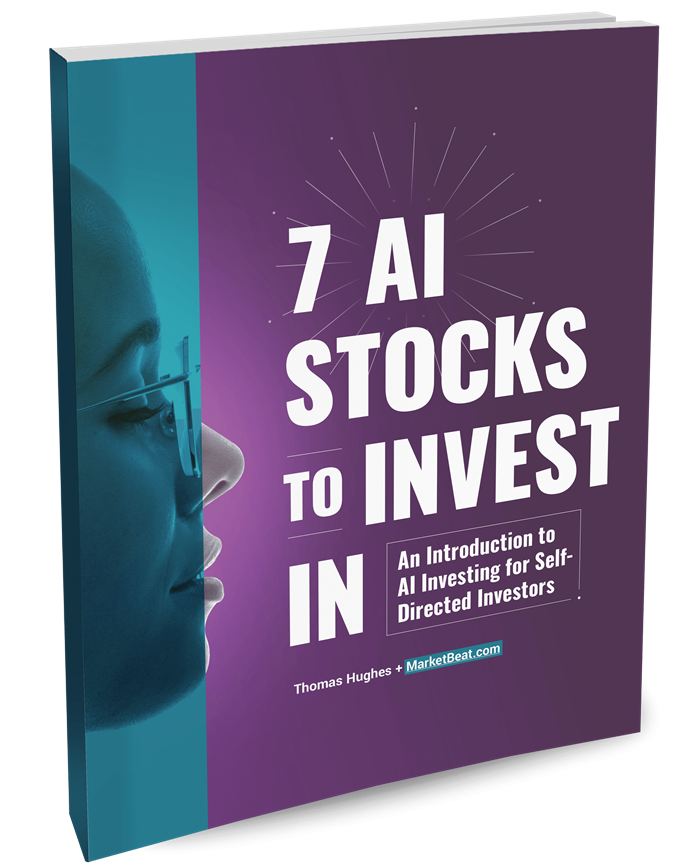
As the AI market heats up, investors who have a vision for artificial intelligence have the potential to see real returns. Learn about the industry as a whole as well as seven companies that are getting work done with the power of AI.
Article originally posted on mongodb google news. Visit mongodb google news
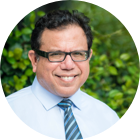
MMS • RSS
Posted on mongodb google news. Visit mongodb google news
MongoDB (NASDAQ:MDB – Get Free Report) is scheduled to release its earnings data after the market closes on Thursday, August 31st. Analysts expect MongoDB to post earnings of $0.45 per share for the quarter. MongoDB has set its Q2 guidance at $0.43-0.46 EPS and its FY24 guidance at $1.42-1.56 EPS.Persons that wish to register for the company’s earnings conference call can do so using this link.
MongoDB (NASDAQ:MDB – Get Free Report) last issued its earnings results on Thursday, June 1st. The company reported $0.56 earnings per share (EPS) for the quarter, topping the consensus estimate of $0.18 by $0.38. The firm had revenue of $368.28 million for the quarter, compared to the consensus estimate of $347.77 million. MongoDB had a negative net margin of 23.58% and a negative return on equity of 43.25%. The business’s revenue was up 29.0% on a year-over-year basis. During the same quarter in the prior year, the firm posted ($1.15) earnings per share. On average, analysts expect MongoDB to post $-3 EPS for the current fiscal year and $-3 EPS for the next fiscal year.
MongoDB Stock Up 2.4 %
Shares of MongoDB stock opened at $370.74 on Thursday. The company has a market cap of $26.17 billion, a price-to-earnings ratio of -79.39 and a beta of 1.13. The company’s 50 day moving average is $391.60 and its 200 day moving average is $297.24. MongoDB has a twelve month low of $135.15 and a twelve month high of $439.00. The company has a quick ratio of 4.19, a current ratio of 4.19 and a debt-to-equity ratio of 1.44.
Analysts Set New Price Targets
A number of brokerages recently weighed in on MDB. Needham & Company LLC increased their price objective on shares of MongoDB from $250.00 to $430.00 in a research report on Friday, June 2nd. Capital One Financial began coverage on shares of MongoDB in a research report on Monday, June 26th. They set an “equal weight” rating and a $396.00 price objective on the stock. Sanford C. Bernstein increased their price objective on shares of MongoDB from $257.00 to $424.00 in a research report on Monday, June 5th. 58.com reiterated a “maintains” rating on shares of MongoDB in a research report on Monday, June 26th. Finally, JMP Securities increased their price target on shares of MongoDB from $400.00 to $425.00 and gave the stock an “outperform” rating in a research report on Monday, July 24th. One investment analyst has rated the stock with a sell rating, three have issued a hold rating and twenty have given a buy rating to the company. Based on data from MarketBeat.com, the stock has an average rating of “Moderate Buy” and an average target price of $378.09.
Get Our Latest Analysis on MDB
Insider Buying and Selling at MongoDB
In other MongoDB news, Director Dwight A. Merriman sold 3,000 shares of the business’s stock in a transaction that occurred on Thursday, June 1st. The stock was sold at an average price of $285.34, for a total transaction of $856,020.00. Following the completion of the transaction, the director now owns 1,219,954 shares in the company, valued at $348,101,674.36. The sale was disclosed in a document filed with the Securities & Exchange Commission, which can be accessed through this link. In other news, CRO Cedric Pech sold 360 shares of MongoDB stock in a transaction that occurred on Monday, July 3rd. The shares were sold at an average price of $406.79, for a total transaction of $146,444.40. Following the completion of the sale, the executive now owns 37,156 shares of the company’s stock, valued at $15,114,689.24. The transaction was disclosed in a legal filing with the Securities & Exchange Commission, which is accessible through this hyperlink. Also, Director Dwight A. Merriman sold 3,000 shares of MongoDB stock in a transaction that occurred on Thursday, June 1st. The shares were sold at an average price of $285.34, for a total transaction of $856,020.00. Following the sale, the director now directly owns 1,219,954 shares of the company’s stock, valued at approximately $348,101,674.36. The disclosure for this sale can be found here. Insiders sold 102,220 shares of company stock worth $38,763,571 over the last 90 days. Company insiders own 4.80% of the company’s stock.
Institutional Trading of MongoDB
Several institutional investors and hedge funds have recently modified their holdings of MDB. Vanguard Group Inc. raised its stake in MongoDB by 2.1% in the first quarter. Vanguard Group Inc. now owns 5,970,224 shares of the company’s stock worth $2,648,332,000 after buying an additional 121,201 shares in the last quarter. State Street Corp raised its stake in MongoDB by 1.8% in the first quarter. State Street Corp now owns 1,386,773 shares of the company’s stock worth $323,280,000 after buying an additional 24,595 shares in the last quarter. 1832 Asset Management L.P. increased its position in shares of MongoDB by 3,283,771.0% during the fourth quarter. 1832 Asset Management L.P. now owns 1,018,000 shares of the company’s stock worth $200,383,000 after purchasing an additional 1,017,969 shares in the last quarter. Geode Capital Management LLC increased its position in shares of MongoDB by 3.8% during the first quarter. Geode Capital Management LLC now owns 967,289 shares of the company’s stock worth $225,174,000 after purchasing an additional 35,541 shares in the last quarter. Finally, Norges Bank acquired a new position in shares of MongoDB during the fourth quarter worth $147,735,000. Institutional investors own 89.22% of the company’s stock.
About MongoDB
MongoDB, Inc provides general purpose database platform worldwide. The company offers MongoDB Atlas, a hosted multi-cloud database-as-a-service solution; MongoDB Enterprise Advanced, a commercial database server for enterprise customers to run in the cloud, on-premise, or in a hybrid environment; and Community Server, a free-to-download version of its database, which includes the functionality that developers need to get started with MongoDB.
Featured Stories
This instant news alert was generated by narrative science technology and financial data from MarketBeat in order to provide readers with the fastest and most accurate reporting. This story was reviewed by MarketBeat’s editorial team prior to publication. Please send any questions or comments about this story to contact@marketbeat.com.
Before you consider MongoDB, you’ll want to hear this.
MarketBeat keeps track of Wall Street’s top-rated and best performing research analysts and the stocks they recommend to their clients on a daily basis. MarketBeat has identified the five stocks that top analysts are quietly whispering to their clients to buy now before the broader market catches on… and MongoDB wasn’t on the list.
While MongoDB currently has a “Moderate Buy” rating among analysts, top-rated analysts believe these five stocks are better buys.
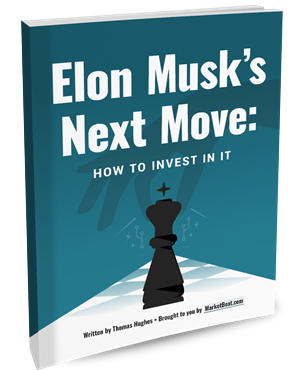
Wondering when you’ll finally be able to invest in SpaceX, StarLink or The Boring Company? Click the link below to learn when Elon Musk will let these companies finally IPO.
Article originally posted on mongodb google news. Visit mongodb google news