Month: December 2023
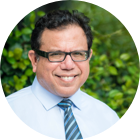
MMS • RSS
Posted on mongodb google news. Visit mongodb google news
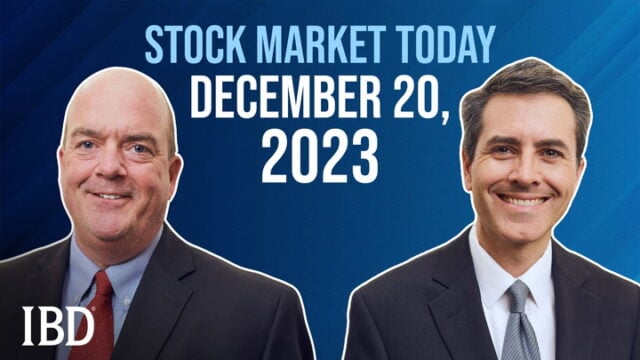
Notice: Information contained herein is not and should not be construed as an offer, solicitation, or recommendation to buy or sell securities. The information has been obtained from sources we believe to be reliable; however no guarantee is made or implied with respect to its accuracy, timeliness, or completeness. Authors may own the stocks they discuss. The information and content are subject to change without notice. *Real-time prices by Nasdaq Last Sale. Realtime quote and/or trade prices are not sourced from all markets.
© 2000- Investor’s Business Daily, LLC All rights reserved
Article originally posted on mongodb google news. Visit mongodb google news
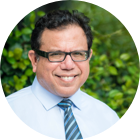
MMS • RSS
Posted on mongodb google news. Visit mongodb google news
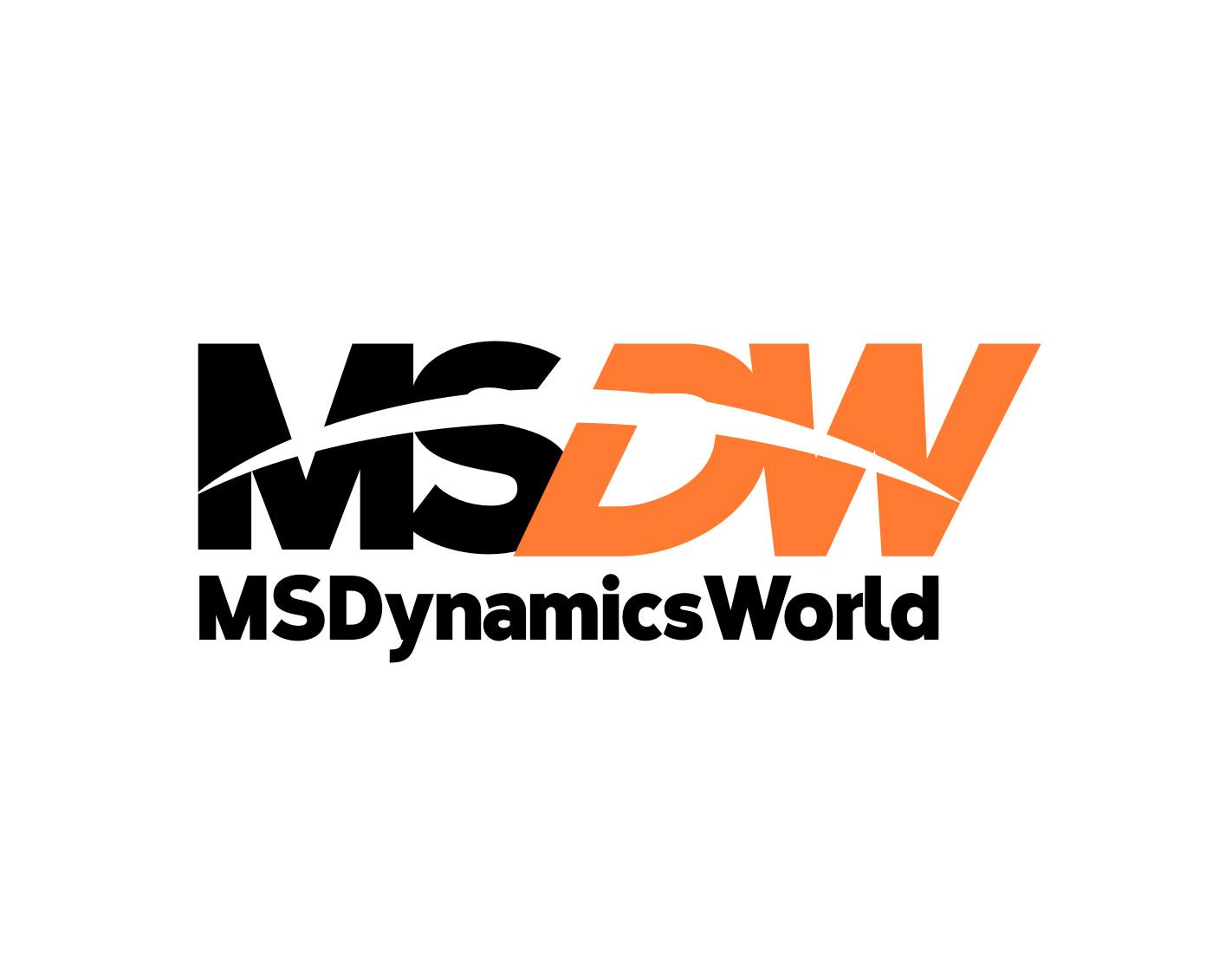
Microsoft CVP Jessica Hawk discussed key benefits of Microsoft’s partnership with MongoDB and their pay-as-you-go self-service database offering. Microsoft is continuing to expand its partnership with capabilities like Semantic Kernel, Microsoft Fabric, and Entity Framework Core. MongoDB Atlas is available over forty Azure regions, including the most recent addition of Doha, Qatar. Hawk wrote:
[W]e know that our customers like using MongoDB to build applications. In year one of our strategic partnership, we collaborated with MongoDB to make it even easier for our joint customers to do more with Microsoft services and MongoDB Atlas on Azure.
Hawk also noted that the two organizations are working together “to further improve GitHub Copilot’s performance using MongoDB schema, among other things.”
Principal product manager Sameer Doultani shared key developments in Azure Cost Management throughout 2023. At the start of the year, Microsoft announced a partnership with the FinOps Foundation and adopted OpenCast cost visibility for AKS in April. New cost analysis features, plus Copilot for Azure appeared on the scene. Additionally, the Azure team added new pricesheet download, alerting, and export options.
Microsoft announced that as a result of customer concerns about the upcoming enforcement of blocking domain fronting on existing Azure Front Door and Azure CDN Standard, Microsoft is postponing blocking domain fronting until …
FREE Membership Required to View Full Content:
Joining MSDynamicsWorld.com gives you free, unlimited access to news, analysis, white papers, case studies, product brochures, and more. You can also receive periodic email newsletters with the latest relevant articles and content updates.
Learn more about us here
Article originally posted on mongodb google news. Visit mongodb google news
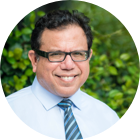
MMS • RSS
Posted on mongodb google news. Visit mongodb google news
When deciding whether to buy, sell, or hold a stock, investors often rely on analyst recommendations. Media reports about rating changes by these brokerage-firm-employed (or sell-side) analysts often influence a stock’s price, but are they really important?
Let’s take a look at what these Wall Street heavyweights have to say about MongoDB (MDB) before we discuss the reliability of brokerage recommendations and how to use them to your advantage.
MongoDB currently has an average brokerage recommendation (ABR) of 1.42, on a scale of 1 to 5 (Strong Buy to Strong Sell), calculated based on the actual recommendations (Buy, Hold, Sell, etc.) made by 26 brokerage firms. An ABR of 1.42 approximates between Strong Buy and Buy.
Of the 26 recommendations that derive the current ABR, 20 are Strong Buy and three are Buy. Strong Buy and Buy respectively account for 76.9% and 11.5% of all recommendations.
Brokerage Recommendation Trends for MDB
Check price target & stock forecast for MongoDB here>>>
While the ABR calls for buying MongoDB, it may not be wise to make an investment decision solely based on this information. Several studies have shown limited to no success of brokerage recommendations in guiding investors to pick stocks with the best price increase potential.
Do you wonder why? As a result of the vested interest of brokerage firms in a stock they cover, their analysts tend to rate it with a strong positive bias. According to our research, brokerage firms assign five “Strong Buy” recommendations for every “Strong Sell” recommendation.
In other words, their interests aren’t always aligned with retail investors, rarely indicating where the price of a stock could actually be heading. Therefore, the best use of this information could be validating your own research or an indicator that has proven to be highly successful in predicting a stock’s price movement.
Zacks Rank, our proprietary stock rating tool with an impressive externally audited track record, categorizes stocks into five groups, ranging from Zacks Rank #1 (Strong Buy) to Zacks Rank #5 (Strong Sell), and is an effective indicator of a stock’s price performance in the near future. Therefore, using the ABR to validate the Zacks Rank could be an efficient way of making a profitable investment decision.
Zacks Rank Should Not Be Confused With ABR
Although both Zacks Rank and ABR are displayed in a range of 1-5, they are different measures altogether.
The ABR is calculated solely based on brokerage recommendations and is typically displayed with decimals (example: 1.28). In contrast, the Zacks Rank is a quantitative model allowing investors to harness the power of earnings estimate revisions. It is displayed in whole numbers — 1 to 5.
Analysts employed by brokerage firms have been and continue to be overly optimistic with their recommendations. Since the ratings issued by these analysts are more favorable than their research would support because of the vested interest of their employers, they mislead investors far more often than they guide.
On the other hand, earnings estimate revisions are at the core of the Zacks Rank. And empirical research shows a strong correlation between trends in earnings estimate revisions and near-term stock price movements.
Furthermore, the different grades of the Zacks Rank are applied proportionately across all stocks for which brokerage analysts provide earnings estimates for the current year. In other words, at all times, this tool maintains a balance among the five ranks it assigns.
There is also a key difference between the ABR and Zacks Rank when it comes to freshness. When you look at the ABR, it may not be up-to-date. Nonetheless, since brokerage analysts constantly revise their earnings estimates to reflect changing business trends, and their actions get reflected in the Zacks Rank quickly enough, it is always timely in predicting future stock prices.
Is MDB a Good Investment?
In terms of earnings estimate revisions for MongoDB, the Zacks Consensus Estimate for the current year has increased 24.4% over the past month to $2.90.
Analysts’ growing optimism over the company’s earnings prospects, as indicated by strong agreement among them in revising EPS estimates higher, could be a legitimate reason for the stock to soar in the near term.
The size of the recent change in the consensus estimate, along with three other factors related to earnings estimates, has resulted in a Zacks Rank #2 (Buy) for MongoDB. You can see the complete list of today’s Zacks Rank #1 (Strong Buy) stocks here >>>>
Therefore, the Buy-equivalent ABR for MongoDB may serve as a useful guide for investors.
Want the latest recommendations from Zacks Investment Research? Today, you can download 7 Best Stocks for the Next 30 Days. Click to get this free report
MongoDB, Inc. (MDB) : Free Stock Analysis Report
To read this article on Zacks.com click here.
Article originally posted on mongodb google news. Visit mongodb google news
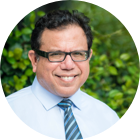
MMS • RSS
Posted on mongodb google news. Visit mongodb google news
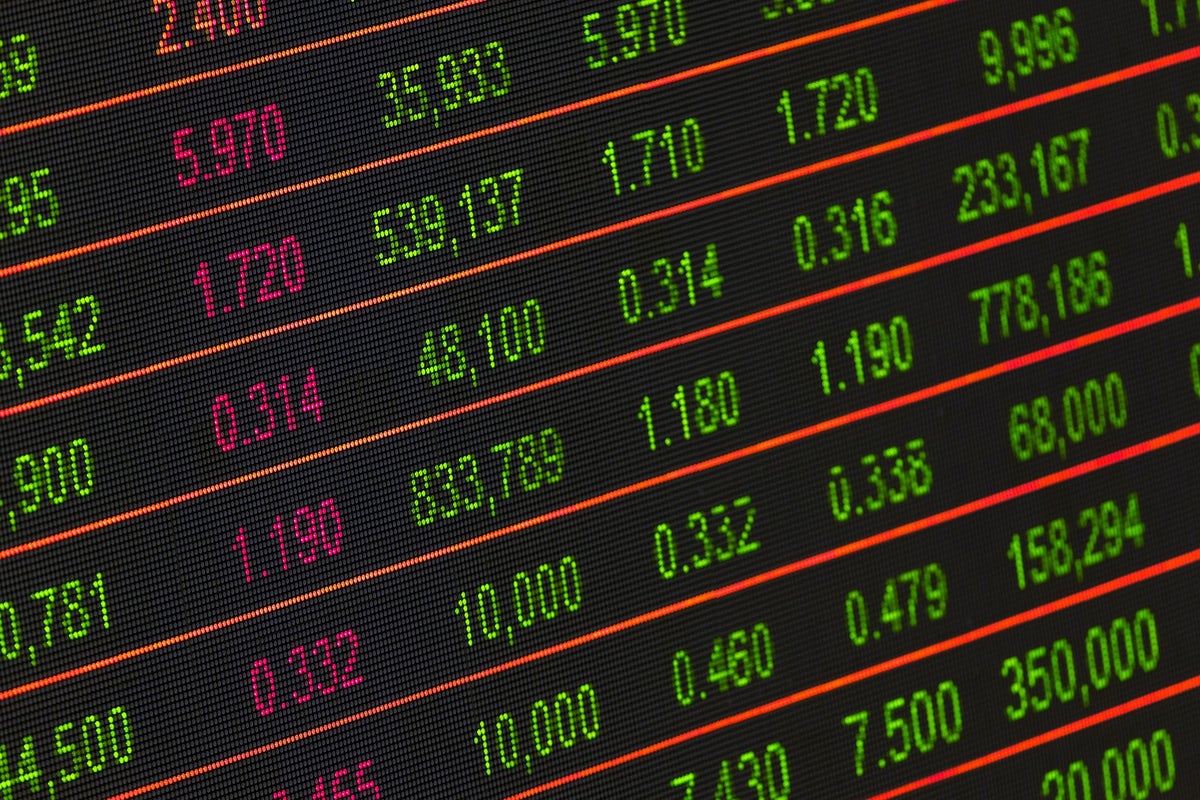
Whales with a lot of money to spend have taken a noticeably bullish stance on MongoDB.
Looking at options history for MongoDB MDB we detected 10 trades.
If we consider the specifics of each trade, it is accurate to state that 60% of the investors opened trades with bullish expectations and 40% with bearish.
From the overall spotted trades, 2 are puts, for a total amount of $195,900 and 8, calls, for a total amount of $469,543.
Expected Price Movements
Taking into account the Volume and Open Interest on these contracts, it appears that whales have been targeting a price range from $200.0 to $460.0 for MongoDB over the last 3 months.
Insights into Volume & Open Interest
Looking at the volume and open interest is a powerful move while trading options. This data can help you track the liquidity and interest for MongoDB’s options for a given strike price. Below, we can observe the evolution of the volume and open interest of calls and puts, respectively, for all of MongoDB’s whale trades within a strike price range from $200.0 to $460.0 in the last 30 days.
MongoDB Call and Put Volume: 30-Day Overview
Significant Options Trades Detected:
Symbol | PUT/CALL | Trade Type | Sentiment | Exp. Date | Strike Price | Total Trade Price | Open Interest | Volume |
---|---|---|---|---|---|---|---|---|
MDB | PUT | SWEEP | BEARISH | 01/19/24 | $380.00 | $148.8K | 333 | 248 |
MDB | CALL | SWEEP | BULLISH | 01/19/24 | $415.00 | $97.9K | 20 | 72 |
MDB | CALL | TRADE | BULLISH | 02/16/24 | $400.00 | $94.2K | 334 | 20 |
MDB | CALL | TRADE | NEUTRAL | 01/19/24 | $200.00 | $63.6K | 107 | 3 |
MDB | PUT | SWEEP | BEARISH | 12/29/23 | $455.00 | $47.1K | 10 | 0 |
About MongoDB
Founded in 2007, MongoDB is a document-oriented database with nearly 33,000 paying customers and well past 1.5 million free users. MongoDB provides both licenses as well as subscriptions as a service for its NoSQL database. MongoDB’s database is compatible with all major programming languages and is capable of being deployed for a variety of use cases.
Current Position of MongoDB
- Trading volume stands at 347,573, with MDB’s price up by 1.09%, positioned at $414.26.
- RSI indicators show the stock to be may be approaching overbought.
- Earnings announcement expected in 77 days.
What The Experts Say On MongoDB
Over the past month, 5 industry analysts have shared their insights on this stock, proposing an average target price of $468.0.
- An analyst from Needham persists with their Buy rating on MongoDB, maintaining a target price of $495.
- An analyst from Piper Sandler persists with their Overweight rating on MongoDB, maintaining a target price of $500.
- An analyst from Mizuho has decided to maintain their Neutral rating on MongoDB, which currently sits at a price target of $420.
- In a cautious move, an analyst from Stifel downgraded its rating to Buy, setting a price target of $450.
- An analyst from RBC Capital has decided to maintain their Outperform rating on MongoDB, which currently sits at a price target of $475.
Options are a riskier asset compared to just trading the stock, but they have higher profit potential. Serious options traders manage this risk by educating themselves daily, scaling in and out of trades, following more than one indicator, and following the markets closely.
If you want to stay updated on the latest options trades for MongoDB, Benzinga Pro gives you real-time options trades alerts.
Article originally posted on mongodb google news. Visit mongodb google news
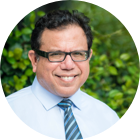
MMS • RSS
Posted on mongodb google news. Visit mongodb google news
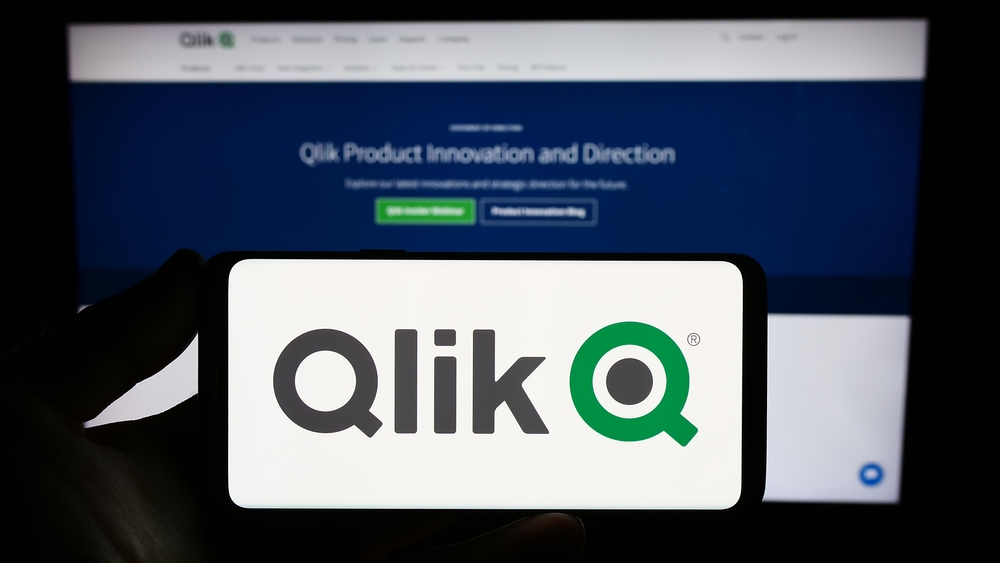
Qlik has acquired Mozaic to simplify data management for large enterprise organizations.
Mozaic features the Data Product Experience platform based on a decentralized architecture. The platform supports organizations in building, securing, deploying and managing data products. It also provides a marketplace for these data products, accessed through different consumption models to enable different use cases.
By integrating Mozaic technology, Qlik hopes to accelerate the implementation and use of data within enterprises. In particular, for cloud platforms that Qlik has partnered with, including Amazon Redshift, Databricks, Google BigQuery, Microsoft Fabric and Snowflake.
Data as a product
Mozaic follows the concept of approaching data as a product. According to Qlik, this strategy aligns with the growing demand for a SaaS-based data product catalog, providing a more efficient and user-friendly experience for managing the data lifecycle. At the same time, it reinforces a solid foundation for AI-driven data management, Qlik states.
Qlik will integrate Mozaic technology into its platform. The technology fits well with Qlik’s existing data quality and governance capabilities. Sharad Kumar, Mozaic’s founder, additionally hints at integrations with Qlik’s future projects.
Financial details of the acquisition were not disclosed. Sharad Kumar will continue his position within Qlik as Regional Head of Data Integration and Quality. In this role, he will continue to work on a Data Product Catalog solution and support users in extracting value from data.
Tip: Cactus ransomware spread through BI platform Qlik Sense
Article originally posted on mongodb google news. Visit mongodb google news
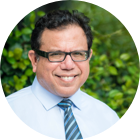
MMS • Artenisa Chatziou
Article originally posted on InfoQ. Visit InfoQ
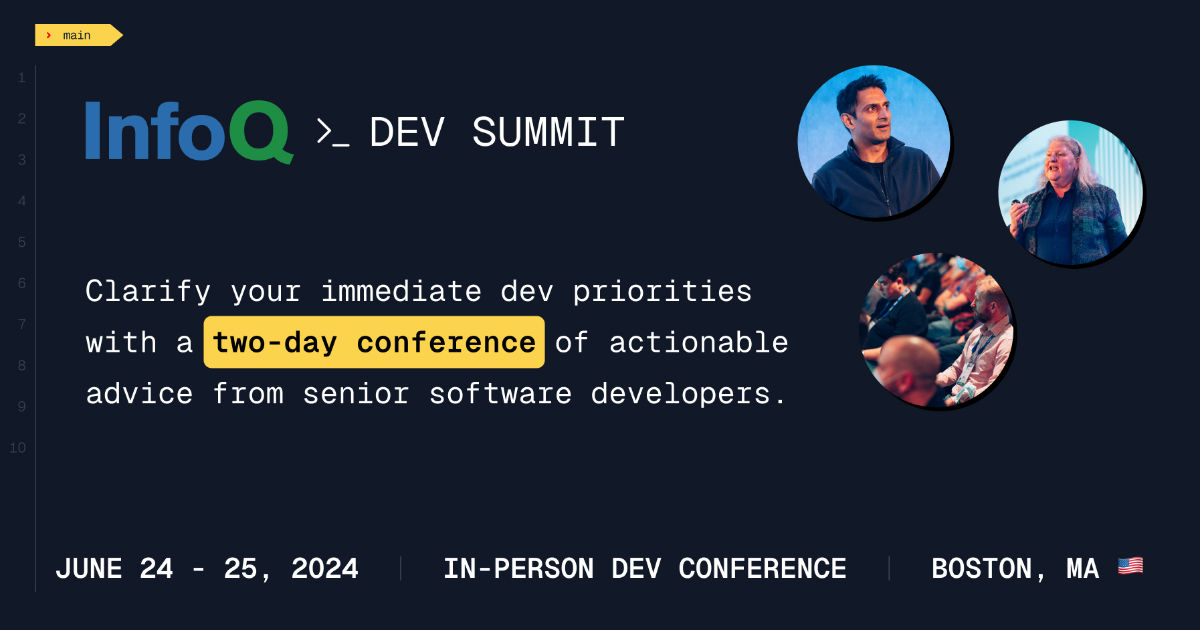
As a senior software developer, understanding and adapting to the latest technologies and techniques is crucial for making informed decisions within your development projects. The need to keep learning, identify shifting trends, and understand how to prioritize software decisions is a constant challenge.
Recognizing this need, InfoQ is delighted to announce a new two-day conference, InfoQ Dev Summit Boston 2024, taking place June 24-25, 2024. This event is designed to help senior developers navigate their immediate development challenges, focusing exclusively on the technical aspects that matter right now.
Why Attend InfoQ Dev Summit Boston 2024?
Practitioner-Curated Topics
One of the standout features of the InfoQ Dev Summit is its agenda. This is curated by senior developers with the goal of focusing the content on the critical technical challenges that are encountered by today’s software development teams.
Actionable Technical Talks
The summit features over 20 sessions of technical talks from senior software developers spread across two days. These sessions are designed to offer practical advice, providing attendees with actionable insights that can be immediately applied to their current development decisions and project prioritization.
Content You Can Trust
InfoQ stands committed to offering genuine learning experiences, free from hidden marketing or sales pitches. Attendees can expect real-world talks from senior software developers that will provide relatable and valuable advice.
‘We Care’ Experience
The InfoQ Dev Summit is not just about technical learning; it’s also about fostering an inclusive and safe environment for all participants. The summit adheres to a strict code of conduct, ensuring a respectful space where everyone’s contributions are valued.
Ready to level up?
Early bird savings and team discounts are available until February 13th. For teams of three or more attending from the same company, email info@devsummit.infoq.com, mentioning the group size to receive a personalized discount.
The InfoQ Dev Summit creates a unique opportunity for senior software developers to learn transformative insights that will directly impact their immediate development roadmap and decision-making processes.
The InfoQ Team looks forward to connecting with you in Boston, June 24-25, 2024, for the InfoQ Dev Summit.
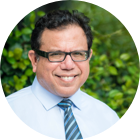
MMS • RSS
Posted on mongodb google news. Visit mongodb google news
Foundations Investment Advisors LLC acquired a new position in shares of MongoDB, Inc. (NASDAQ:MDB – Free Report) in the 3rd quarter, according to its most recent filing with the Securities & Exchange Commission. The fund acquired 587 shares of the company’s stock, valued at approximately $203,000.
Several other institutional investors have also recently made changes to their positions in the business. Price T Rowe Associates Inc. MD raised its holdings in shares of MongoDB by 13.4% during the first quarter. Price T Rowe Associates Inc. MD now owns 7,593,996 shares of the company’s stock worth $1,770,313,000 after purchasing an additional 897,911 shares during the last quarter. Vanguard Group Inc. increased its holdings in MongoDB by 2.1% in the first quarter. Vanguard Group Inc. now owns 5,970,224 shares of the company’s stock valued at $2,648,332,000 after buying an additional 121,201 shares in the last quarter. Jennison Associates LLC increased its holdings in MongoDB by 101,056.3% in the second quarter. Jennison Associates LLC now owns 1,988,733 shares of the company’s stock valued at $817,350,000 after buying an additional 1,986,767 shares in the last quarter. State Street Corp increased its holdings in MongoDB by 1.8% in the first quarter. State Street Corp now owns 1,386,773 shares of the company’s stock valued at $323,280,000 after buying an additional 24,595 shares in the last quarter. Finally, 1832 Asset Management L.P. increased its holdings in MongoDB by 3,283,771.0% in the fourth quarter. 1832 Asset Management L.P. now owns 1,018,000 shares of the company’s stock valued at $200,383,000 after buying an additional 1,017,969 shares in the last quarter. Hedge funds and other institutional investors own 88.89% of the company’s stock.
Insider Activity
In other news, CAO Thomas Bull sold 518 shares of the business’s stock in a transaction that occurred on Monday, October 2nd. The shares were sold at an average price of $342.41, for a total value of $177,368.38. Following the sale, the chief accounting officer now directly owns 16,672 shares of the company’s stock, valued at approximately $5,708,659.52. The transaction was disclosed in a legal filing with the Securities & Exchange Commission, which is available through this hyperlink. In related news, CFO Michael Lawrence Gordon sold 7,394 shares of the company’s stock in a transaction that occurred on Monday, October 2nd. The shares were sold at an average price of $345.20, for a total transaction of $2,552,408.80. Following the sale, the chief financial officer now directly owns 99,469 shares of the company’s stock, valued at approximately $34,336,698.80. The sale was disclosed in a legal filing with the SEC, which is available through this link. Also, CAO Thomas Bull sold 518 shares of the company’s stock in a transaction that occurred on Monday, October 2nd. The stock was sold at an average price of $342.41, for a total transaction of $177,368.38. Following the sale, the chief accounting officer now directly owns 16,672 shares in the company, valued at $5,708,659.52. The disclosure for this sale can be found here. In the last three months, insiders have sold 298,337 shares of company stock valued at $106,126,741. Company insiders own 4.80% of the company’s stock.
Analyst Upgrades and Downgrades
A number of research analysts have weighed in on MDB shares. Bank of America started coverage on shares of MongoDB in a report on Thursday, October 12th. They issued a “buy” rating and a $450.00 price objective for the company. Sanford C. Bernstein lifted their price objective on shares of MongoDB from $424.00 to $471.00 in a report on Sunday, September 3rd. Barclays lifted their price objective on shares of MongoDB from $470.00 to $478.00 and gave the company an “overweight” rating in a report on Wednesday, December 6th. Oppenheimer boosted their target price on shares of MongoDB from $430.00 to $480.00 and gave the stock an “outperform” rating in a research note on Friday, September 1st. Finally, Mizuho boosted their target price on shares of MongoDB from $330.00 to $420.00 and gave the stock a “neutral” rating in a research note on Wednesday, December 6th. One analyst has rated the stock with a sell rating, two have given a hold rating and twenty-two have given a buy rating to the company. According to data from MarketBeat, the stock currently has a consensus rating of “Moderate Buy” and an average target price of $432.44.
Check Out Our Latest Report on MongoDB
MongoDB Stock Performance
NASDAQ:MDB opened at $409.78 on Wednesday. The company has a debt-to-equity ratio of 1.18, a current ratio of 4.74 and a quick ratio of 4.74. The firm has a market capitalization of $29.58 billion, a price-to-earnings ratio of -155.22 and a beta of 1.19. The company’s 50-day moving average is $380.03 and its two-hundred day moving average is $379.13. MongoDB, Inc. has a 1-year low of $164.59 and a 1-year high of $442.84.
MongoDB (NASDAQ:MDB – Get Free Report) last posted its earnings results on Tuesday, December 5th. The company reported $0.96 earnings per share for the quarter, beating the consensus estimate of $0.51 by $0.45. The company had revenue of $432.94 million for the quarter, compared to analyst estimates of $406.33 million. MongoDB had a negative net margin of 11.70% and a negative return on equity of 20.64%. MongoDB’s revenue was up 29.8% on a year-over-year basis. During the same period in the previous year, the business posted ($1.23) EPS. Analysts forecast that MongoDB, Inc. will post -1.64 EPS for the current year.
About MongoDB
MongoDB, Inc provides general purpose database platform worldwide. The company offers MongoDB Atlas, a hosted multi-cloud database-as-a-service solution; MongoDB Enterprise Advanced, a commercial database server for enterprise customers to run in the cloud, on-premise, or in a hybrid environment; and Community Server, a free-to-download version of its database, which includes the functionality that developers need to get started with MongoDB.
Read More
Want to see what other hedge funds are holding MDB? Visit HoldingsChannel.com to get the latest 13F filings and insider trades for MongoDB, Inc. (NASDAQ:MDB – Free Report).
Receive News & Ratings for MongoDB Daily – Enter your email address below to receive a concise daily summary of the latest news and analysts’ ratings for MongoDB and related companies with MarketBeat.com’s FREE daily email newsletter.
Article originally posted on mongodb google news. Visit mongodb google news
AWS Announces Amazon Braket Direct: Reserve Dedicated Capacity on Different Quantum Devices
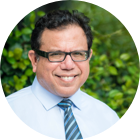
MMS • Steef-Jan Wiggers
Article originally posted on InfoQ. Visit InfoQ
AWS recently announced the availability of Amazon Braket Direct, a new Amazon Braket program allowing quantum researchers to dive deeper into quantum computing.
Amazon Braket is a fully-managed quantum computing service from Amazon Web Services (AWS). With Braket Direct program, users get dedicated, private access to the full capacity of various quantum processing units (QPUs). Moreover, they do not, according to the company, have to wait for resources and can connect directly with quantum computing specialists to receive expert guidance from quantum hardware providers such as IonQ, Oxford Quantum Circuits, QuEra, Rigetti, or Amazon Quantum Solutions Lab for their workloads. In addition, users get early access to features and devices with limited availability to conduct their research.
Channy Yun, a principal developer advocate at AWS, writes:
You can now use Braket Direct to reserve the entire dedicated machine for a period of time on IonQ Aria, QuEra Aquila, and Rigetti Aspen-M-3 devices for running your most complex, long-running, time-sensitive workloads, or conducting live events such as training workshops and hackathons, where you pay only for what you reserve.
Amazon Braket Direct (Source: AWS News Blog)
Braket Direct offers not only on-demand access to quantum computers, yet also the option for dedicated device access through reservations. These reservations provide exclusive usage of a chosen quantum device, allowing users to schedule sessions at their convenience, with the flexibility to cancel up to 48 hours in advance at no extra cost.
Furthermore, users can engage with quantum computing experts directly within the Braket management console for additional guidance on workloads, selecting from options such as free office hours with Braket experts, professional services offered by quantum hardware providers, or assistance from the Amazon QSL.
A respondent on a Reddit thread commented on “house” cloud services like DWave, Rigetti, and IBM versus the AWS offering:
A current benefit of the in-house clouds is a richer API and generally more control over the quantum computer. Rigetti gives a whole host of tools for, say, efficient variational algorithm execution and direct reservation of the machine. The SDK is also extremely comprehensive in its capabilities, including compilation.
A benefit of an offering like AWS is ubiquity, familiarity with their permissions and billing systems, common API for multiple machines (even if the API isn’t as rich), and ease of integration with other Amazon products, of which they have many.
Braket Direct is currently generally available in all AWS regions where Amazon Braket is available, and its pricing details are available on the pricing page.
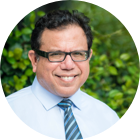
MMS • Susanne Braun
Article originally posted on InfoQ. Visit InfoQ
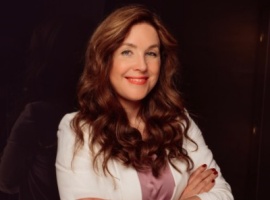
Transcript
Braun: I would like to directly start with a quote from Pat Helland, who said in 2009, there is an interesting connection between so-called application-based eventual consistency, and certain quality attributes. If you look at the quality attributes that he mentions, and also at the ones that are closely related, you will realize these are all quality attributes that we commonly try to achieve in modern software architecture design. Let it be cloud native, let it be reactive systems, let it be offline-first, you name it. Therefore, I believe it’s really important to not only understand the main concept of eventual consistency, but also its pitfalls. What I quite often observe is that the discussion around eventual consistency is a bit one-sided. Because if you look at the product context, when it was heavily promoted by people such as Eric Brewer, you will see that there’s actually more to it. Eric Brewer proposed BASE as a counter concept to ACID transaction guarantees, and he said, we will not only have to forfeit the consistency of ACID, but also the isolation of ACID. This is quite significant.
ACID vs. BASE
Let me very briefly contrast ACID versus BASE again. The important part around ACID consistency is that it was defined in a time when we used to design our systems with a single database that was very central, that was a single source of truth. Here, ACID consistency was all about that single source of truth always being in a consistent state, so that in particular, also, all invariants are met at all times. Eventual consistency, on the other hand, as promoted by BASE, the first thing is, eventual consistency is only applicable in the context of a distributed system. Second, it also only makes sense if we replicate data in the system, for example, in order to increase availability or something like that. What eventual consistency then says is that, actually, most of the time, the replicated data is not in a consistent or identical state. Eventual consistency guarantees that, over time, the different copies converge state-wise towards each other. This is very much about convergence actually. Isolation, this is, of course, all about concurrency control, a tough topic, as you probably agree.
Consistency vs. Isolation
Let me just summarize when we talk about consistency in distributed systems, it’s very often and very much about masking actually the distributed nature of the system and rather make it again appear as one system. When it comes to isolation, here, this is all about trying to mask the effects of concurrent execution and providing the illusion to the application developer that the programs he is writing are currently the only user of the system so that he does not have to care about the different pitfalls around concurrency. Let me also bring the official definition of eventual consistency. The term was not invented by Eric Brewer. Actually, it is much older and was already in the ’90s coined by Douglas Terry, who is a very famous distributed systems researcher, and also has basically worked for most of the big hyperscalers. He originally said, a system providing eventual consistency guarantees that replicas would eventually converge to a mutually consistent state. Meaning that as soon as any update activity has ceased, then we will eventually reach identical content in a different system. This definition is a bit abstract. It’s not very hard to grasp and to really understand the implications for your system. I think he agreed, because a few years later he provided a more pragmatic definition of eventual consistency. He then said, ok, a system provides eventual consistency if, first of all, each update operation is eventually received by each replica. Second, what we need for the convergence guarantee is that any operations that are not commutative, meaning that they are order dependent, they of course need to be executed in the same order at each replica.
The third thing that we need to achieve convergence is of course determinism, meaning that each update operation needs to produce the same result deterministically at each replica. Remember, whenever you have to deal with eventual consistency, for example, because you are using a database management system that only provides eventual consistency, the only thing that you get is this convergence guarantee. This has quite some implications for our applications. Because you will have to handle data that is potentially outdated. If you update outdated data, you have update conflicts. If you’re not able to properly resolve these update conflicts, in retrospect, you can have quite severe concurrency anomalies such as lost updates, for example. Of course, events and operations might come out of order and you also have to deal with that. You probably agree that this is potentially a huge source of human error.
The next thing is, you do not get any isolation guarantees. I’m not even talking about serializable. It’s also, you don’t get basic stuff, such as Repeatable Read, for example. I believe Repeatable Read is something we’re constantly relying on when we write transaction programs. For example, very often, we first read some data out of the database, we evaluate some conditions on that data we have just loaded. Then, depending on whether or not our condition evaluates successfully, we do an update of the data or not. When doing so we, of course, assume that the data we have read in the beginning does not change in the course of our transaction because of other concurrent users. If you would have to care about that, then everything becomes much more complicated. This means that we have to deal with much more concurrency control things. You all know how it is. First of all, it’s hard to test. If there are any issues, mostly they first emerge in production, so the first time when there’s real heavy load on the system. Then, of course, it’s very hard to reproduce, and even harder to debug. This is also quite a huge source of human error.
When it comes to eventual consistency, it’s on one hand, very interesting and an important topic. On the other hand, it’s also quite challenging. You would assume there’s a lot of research going on to mitigate some of the toughest challenges, and this is also true. There’s a dozen of different variants of weak consistency available. Eventual consistency is just one variant, which is probably the most popular one. However, I worked at Fraunhofer as a researcher for several years, and even me, at the time when I was a researcher, it was hard to keep up with all the different variants and things going on there. What is even worse is that these are very often just very minor improvements and nothing like the big step. What I think is rather valuable in this context is also something that Pat Helland said in this regard. He said also in 2009 that he believes that it is time for us to move past the examination of eventual consistency only in terms of updates and storage systems, but the real action comes, the interesting things come when we start to examine eventual consistency in the context of concrete domain and operational semantics.
DDD Layered Architecture
This is not straightforward what he means. Let me very quickly show you the typical three-layered architecture as promoted, for example, by domain driven design. This could be a three-layered architecture of a microservice, for example. Typically, in the top layer, we have the application layer that takes care of controlling overall application flow. We have application services there. Here, the application service methods are usually protected by transaction boundaries. Usually, when you start such an application service method, at the same time, also a database transaction is started in the infrastructure layer. When it comes to concrete domain logic, this is usually delegated to the domain layer. The application service will, for example, use a repository to query and aggregate. Aggregate again is a domain driven design concept where you cluster domain objects that have similar update behaviors or domain objects that usually need to be updated together. You load these domain objects via the repositories, and then the actual domain logic is implemented in methods of these aggregates. An aggregate method will probably be invoked, and in the course of that method, the state of the aggregate also might change. These changes, of course, need then, again, to be propagated into the infrastructure layer and translated into, for example, SQL statements. What Pat Helland was referring to is that when we deal with eventual consistency, we usually try to handle it completely in the infrastructure layer. What he believes is that it could be very beneficial if he would try to also consider it in the domain layer itself, because this is the part where the actual domain logic lives. This is the idea he was talking about.
Recap of Concurrency Control in Relational DBs
This is also not straightforward, why this should be beneficial by any means. In order to understand that, I need to very quickly recap how concurrency control is usually implemented within relational database management systems. The database management system usually tracks, on a very low-level, basic read and write operations on data items. Then it defines so-called conflict relationships between these read and write operations. For example, when two transactions write the same data item, then this is a conflict relationship, both operations are conflicting. The same is true for reading and writing the same database item by two different transactions. The only thing that is basically compatible are pure read operations. The database monitors these operations and uses the conflict relationships to build a so-called conflict graph. You all know probably that if this conflict graph does not have any cycle, this means that the concurrent execution of the transactions has actually been equivalent to a serializable or has been equivalent to a serial execution, which is commonly referred to as conflict serializability. If we know the execution of concurrent transactions is equivalent to a serial execution, we all know that by no means there can have been any concurrency anomalies because it was equivalent to serial execution. That’s the idea.
Let me give you an example. Here we have two transactions that run concurrently. We have a green transaction and a blue transaction. Here we can see that the green transaction reads a data item a, and this data item a is also later written by the blue transaction. This means that if we assume a serial execution, the green transaction must have run before the blue transaction. This is why we have this dependency in the conflict graph, green transaction must happen before the blue transaction. We can do this the same way with all other operations to determine the conflict relationships. The thing here is that also the blue transaction reads a data item b that is afterwards written by the green transaction. Again, as the blue transaction does not see the changes made by the green transaction later, the blue transaction must have run before the green transaction. We get another dependency in our conflict graph. This is, of course, a contradiction. The green transaction cannot run before the blue, and at the same time, the blue transaction run before the green one. Here we would have a circle. We would know that this is not equivalent to serial execution. We don’t have conflict serializability, and so, in this case, the database needs to assume that there might have been the worst case of a concurrency anomaly. A relational database management system would prevent this concurrent schedule either by means of locks or by rolling back one of the two transactions.
Business Semantics – Banking
The interesting part is now if we look at what has actually happened in the domain layer, what happened on a semantic level. Let’s do that. We will see that these transactions have been two transactions from the banking domain. Both transactions withdraw some amount of money from account a and deposits some other amount of money to account b. The thing is, if we look at this in more detail, you will realize that at a semantic level, nothing bad has happened. No concurrency anomaly or something whatsoever. This is mainly due to the fact that operations in the domain layer, withdraw and deposit, these operations are commutative. They commute, it doesn’t matter whether we first execute both withdraw operations and then both deposit operations, or if we execute them in an interleaved manner, as these operations are commutative, and all operations have been executed atomically, we don’t have any issues there. This concurrent execution of transactions could have actually been run and there would not have happened anything bad. However, the database management system does not know anything about the semantics and the domain semantics, so it has to assume the worst case. This problem happens in practice quite often. A lot of concurrent executions could actually run in parallel without any issues, but the database management system always has to assume the worst case because it’s not aware of the domain semantics and the domain logic.
Multilevel Transactions
This problem was known for quite a long time. Researchers already tried to solve that in the ’90s with so-called multilevel transactions. The idea here was to exploit the semantics of operations in higher levels of our architecture. The ultimate goal was to increase concurrency, of course. What they do is that they basically decompose transactions first into operations, operations again into suboperations, until we end up with low-level operations in the infrastructure layer. Typically, we only have three layers, which pretty well matches to our three-layered architecture. Usually, we have transactions at the top. We have domain operations or business operations in the middle layer. We have low-level read and write operations in the infrastructure layer. Then at each level, also a conflict relationship is defined. In the infrastructure layer, we keep the existing conflict relationships, as I just explained before, whereas in the domain layer, we consider domain operations that are not commutative to be conflicting. Then, we just have to build a conflict graph at each level. If every graph is acyclic, then what we get is something called multilevel serializability. The interesting thing is that, in practice, multilevel serializability is nearly as good in terms of guarantees as standard serializability.
Multilevel Transactions Example
Let’s look at an example. We have, again, our banking transactions. The approach is actually quite similar. We first start to analyze whether the domain operations are conflicting. We basically apply the same approach as before. We just treat domain operations as if the domain operations would be transactions. We analyze, the conflict relationship is quite similar, but this time only between domain operations and not transactions. What we get then is the conflict graph as depicted on the upper right part of the slide. We have two dependencies, but we don’t have a cycle. Then, if we go one level upper or one level above, we would like to understand the conflicts between our transactions. We will see that we actually don’t have domain operations there that are conflicting because all domain operations commute. We don’t have any operation at all that is conflicting with another one, meaning that our conflict graph is basically empty. This also means that it doesn’t have any cycles. The conflict graphs are acyclic at each level. If we had multilevel transactions, this concurrent execution of transactions could have run, and we would have a much higher concurrency as with standard serializability.
Domain Operation Design
This is, of course, a good thing. We can apply this principle not only locally to one microservice. Of course, we can also increase concurrency or concurrent execution of domain operations or update operations that run at different replicas or nodes of a system. That’s why it’s of course interesting to think about how we can optimize the design of our domain operations, so that these operations can run concurrently and conflict-free at different nodes of a system with eventual consistency. Let’s look into that in more detail. I would like to again start with a statement from Pat Helland. He also said in 2009 that we should start to design our domain operations to be ACID 2.0 compliant, meaning that these operations should be associative, commutative, and idempotent. What we get then are truly distributed operations that can be executed at any node of the system in any order, and it still yields, in the end, the same consistent state. We also don’t have to do complex conflict resolution and things like that.
An interesting application of this ACID 2.0 principle are so-called conflict-free replicated data types. These are special data types with built-in conflict resolution, because these types, they not only have normal update method or operations, but they also ship with a commutative merge operation, that is at the same time designed to be a least upper bound of two conflicting versions. I know this sounds very complex. CRDTs are grounded in algebraic theories of monotonic semilattices and things like that. What I can do is I can provide you an easy-to-understand example so that you can better understand how the mechanics of CRDTs are, let’s put it like that. A very good example is actually how Amazon resolves conflicts on their shopping cart service. Assume that we have two conflicting versions of the same shopping cart, maybe this is because of a network partition. The user has been directed because of that to another replica, so now at the end, we have two versions, and we need to reconcile this in some way. What Amazon actually does, it just takes the two shopping carts, and it builds the union of these two shopping carts. As you might realize now, this union operation is an operation that is associative, commutative, and idempotent. Further, the union of two sets is also always the least upper bound of these two sets, just to give you a bit of background on the mathematics stuff.
If you look at it like this, it’s quite simple. It’s also very powerful. It guarantees convergence without complicated conflict resolution. It also has some drawbacks. Following this approach, it means that if the user has deleted items from the shopping cart such as, for example, the soap, could be the case that he has deleted it, then that deleted item might reappear. This is a drawback that Amazon accepts, because, for Amazon, it is so important that this shopping cart service is highly available. If it’s not available for only just a few seconds or minutes, Amazon loses really a very big amount of money. For Amazon, this is a better compromise. They accept that from time to time, they might have to manually resolve these things, or that the user returns items that he has ordered.
Let me also give you a bit of a better understanding of what is a distributed operation all about. If you look at the image, I think it’s very apparent that if we have multiple replicas executing domain operations concurrently, then there needs to be a point when these replicas synchronize and exchange update operations. Then of course, these operations from the other replica, or remote operations are executed, this of course means that the operations are executed in different orders at different replicas. When you have a truly distributed operation, this doesn’t matter. You get the same result at each replica, independent of the execution order. Very often, this is not complicated to realize. In a lot of cases, it’s actually no big deal. There are also cases where it can be quite challenging.
I just want to give you one example when it’s not straightforward, so that you have a better feeling about it and know what I mean. This is an example with collaborative text editing. Assume we have two replicas, a green one and a red one. Both replicas execute operations concurrently without direct synchronization. Of course, at some point, as already mentioned, they will exchange update operations. The green operation at some point will also be executed on the red replica, but it will run on a different state than on a green replica because we have concurrent operations run before. Let me go into the details here. We assume we have a very simple text here, it’s just a very brief hello world text with some typos of course. Let’s assume that at the green replica, the typo in the second word was fixed. Here, an r has been inserted at position 6 of the text. On the second replica, the red one, the first word has been corrected. An e and an l has been inserted. If a node just simply re-executes the insert of the r on position 6, this is of course not what we are intending to get as a result. This is just to give you an example. This is not easy to fix, however, there exists already quite a bunch of libraries that implement CRDTs exactly aimed at collaborative text editing. That’s it regarding domain operation design. Of course, we will not be able to design all our domain operations to be ACID 2.0 compliant.
Domain Data Design
The second thing that we can also do is we can also try to optimize the design of our domain objects. Also here, I would like to start with a quote from Pat Helland who said in 2016, immutability changes everything. Correspondingly, immutability is also an important classification criterion in a taxonomy that I have developed as part of my PhD at Fraunhofer. What I basically say is that when it comes to data, or aggregates, or domain objects, whatever, I basically say, there’s trivial ones, and there’s non-trivial ones. Of course, the trivial ones are rather easy to deal with under eventual consistency, whereas the non-trivial ones are quite challenging in relation to eventual consistency. Let me introduce the taxonomy. The first thing you’ll need to ask is, is my data actually immutable? If this is the case, you have immutable aggregates in your domain model, which is a good thing, because that also means you cannot have updates, which means you cannot have update conflicts. Typical examples for immutable aggregates are domain events. Also, think of all the machine learning use cases, a lot of time-series data such as machine sensor data, market data, and so on, is actually immutable.
The next frame you need to ask yourself is, my data might not be immutable, but maybe it is the case that I can just derive or calculate this data on demand at any time out of other data. That of course means you also don’t need to update these aggregates, you just calculate them whenever you need them. Here, let me give you also some examples. Of course, any kind of aggregated data comes to mind such as data warehouse reports, KPIs, and things like that. Here, again, the machine learning use case, think about your recommendations in your online store, or the timeline in your social media application, these are all generated data. The last thing that you can ask is, my data might not be immutable, I also cannot derive it, but it might be the case that there is just a single updater that from a domain perspective is allowed to make updates to that object or aggregate. Then you have a dedicated aggregate. Of course, then it’s also very improbable you have conflicts, because that would mean that the same user working with different devices and doing things, this is also something that is rather not possible. Here, examples are any kind of crowd data. For example, your reviews in online stores, your social media posts, but also dedicated master data such as user profiles, account settings, and so on. All other data is rather non-trivial in relation to eventual consistency.
Still, we also divide these non-trivial aggregates into three different subclasses, depending on how challenging they are. In order to classify that, we use update frequency during peak times. Correspondingly, the probability for simultaneous updates during peak times and again corresponding the probability for concurrency anomalies. On the lowest level, we have so-called reference aggregates. This is data that is rather long-lived, that is rarely updated, but that’s often also very business critical, and referenced a lot from other data. Examples here are master data such as CRM data, resources, products, things like that. Then we have activity aggregates. These aggregates capture the state of activities with multiple actors. Think about classical business processes, workflows, and things like that. Here, of course, depending on the state of the activity, we might have peak times with more requests. Correspondingly, here we have a certain probability that anomalies occurred. Here, you should probably think about a feasible strategy for conflict resolution. The most challenging ones are the collaboration result aggregates. These capture the result of collaborative knowledge work, such as, for example, multiple people offering a text. It can also be a whiteboard diagram, or a CAP model. Of course, here, the probability for conflict is very high. Correspondingly, you should have a strategy that can automatically resolve conflicts, because, otherwise, this might have a very negative impact on your user experience.
This is one thing that you might ask, which data do I have in my domain model? Another thing that you can ask is, how severe is it actually, that my data is corrupted because of conflicts and concurrency anomalies. This is something I think we need to discuss with the domain experts. It’s not something we can decide on our own. Also, here, given the Amazon example, I think it’s perfectly valid to accept a certain degree of corruption, if on the other hand availability is crucial for your business. The third thing I would like to ask is, how often do these different classes actually occur in practice? How many instances of them do we have? I believe, if we look at traditional information systems, such as ERP systems, CRM systems, and so on, I believe we have a comparatively high degree of non-trivial aggregates. Maybe this is also an explanation why when we designed these systems, we commonly preferred to use relational database management systems, which here provide the highest guarantees in terms of consistency and isolation.
If on the other hand we look at social media, we have the perfect opposite. The largest share of data is actually trivial. This might also be the reason why social media very often pioneered eventual consistency concepts, and might also be the reason why eventual consistency is chosen very often here. The last thing is to look at the systems that we are building right now or in the future. I believe these systems are heavily data intensive systems. I also believe that in these data intensive systems, we have all kinds of aggregate classes. For me, this is a very strong indicator that we can no longer continue with general decisions with regards to consistency, but we have to much more come to the point where we make much more situational decisions and decisions based on the context, the concrete domain semantics.
Best Practices
What I would like to share now are some best practices with you. Of course, the first thing I would recommend is to use trivial aggregates whenever feasible. Of course, it’s perfectly clear we won’t have a lot of examples where the whole domain model can be built out of trivial aggregates. On the other hand, I think we can more often apply them, than we usually might believe at first sight. A typical example here is stock management. You can model your domain model for stock management as given on the left-hand side with the antipattern. You have here a stock item with a counter that counts the current number of items in stock. This of course also means, if you have a lot of incoming and outgoing goods, a lot of movement here, you will have a lot of concurrency and probably you will then have conflicts on this counter attribute that are very hard to resolve. On the left-hand side, this is an activity aggregate that is, of course, not trivial, but you could design your domain model differently and only build it with trivial aggregates. One thing you could do is you could keep a history of all goods receipts and all order confirmations, these are of course immutable. Then, based on these goods receipts and order confirmations, you could in theory at any time calculate the current number of items that are in stock. That would be then a derived aggregate.
Another thing I would like to highlight is that, if you look at domain driven design and the aggregate pattern, domain driven design recommends you to cluster domain objects with a similar update behavior and put them under the same aggregate boundary. That same update behavior is very fuzzy. Here, you can see, this is a domain model from smart farming. This is about operations to be executed on fields such as, for example, a harvesting operation. Here we have fields where the operation is to be executed. We have assignment of staff and machinery. We also have documentation records. This is actually a German thing. We have a lot of rules here. One thing is that the operator of a machine needs to document, for example, how much chemistry has been put on the field. These documentation records, if you look at our taxonomy, this is actually a dedicated object, because this documentation record can only be created by the operator on the machine and only that operator would be allowed to update it, because we do not want to allow manipulation afterwards. As this is a dedicated aggregate, it’s much less that we have conflicts on these domain objects. It makes a lot of sense to separate these different domain objects and have one aggregate for the dedicated data and keep the operation data in a separate aggregate as well.
We also believe that this can be generalized. This should be a general rule. It makes perfect sense to when you realize you have domain objects that are actually part of different classes of our taxonomy, then these domain objects should also be separated and put into separate aggregates with separate aggregate boundaries. Here the example, a field is, of course, master data. It’s rarely updated. It is therefore a reference aggregate and should be kept separately. With that, we can of course also increase the share of trivial aggregates within our domain model. We know that a certain part of the domain model is secure, and we don’t need to care about it. Here, we have one non-trivial aggregate left, which is the operation aggregate and, of course, we need to look at that in more detail and find out whether or not we need some appropriate conflict management.
The last thing I would like to share with you is, in case you really need strong transactional guarantees, please consider a pattern called primary copy replication. It’s very commonly also applied in the context of microservice architectures. The idea is to have here dedicated microservice for certain aggregates or entities, for example, the order service that is the only service with the permission to update order data. All other microservices might, of course, replicate orders, but they are not allowed to update them. In case they need to update they need to delegate to the order service.
Resources
We have this documented in a guide that is freely available on GitHub, https://github.com/EventuallyConsistentDDD/design-guidelines/blob/main/ECD3-Domain-Objects-Design-Guide-v1.2.pdf. If you like what I just presented, please have a look. You can find there a much more comprehensive documentation with code examples, and text further explaining the ideas behind it. I also would like to mention that we not only developed these best practices and guidelines based on our experience in collaborations with industry clients, but we also did a number of empirical evaluations. We evaluated these methods in collaboration with industry partners, collected data systematically in the course of an actual research study. The result of the study you can find in this paper, https://arxiv.org/pdf/2108.03758.pdf. It’s freely available. It’s also a good read. We got the best paper award at one of the top software engineering conferences for that. At the moment, I’m no longer with Fraunhofer as I submitted my PhD last year. I joined SAP Signavio in Berlin.
See more presentations with transcripts
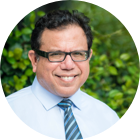
MMS • Johan Janssen
Article originally posted on InfoQ. Visit InfoQ
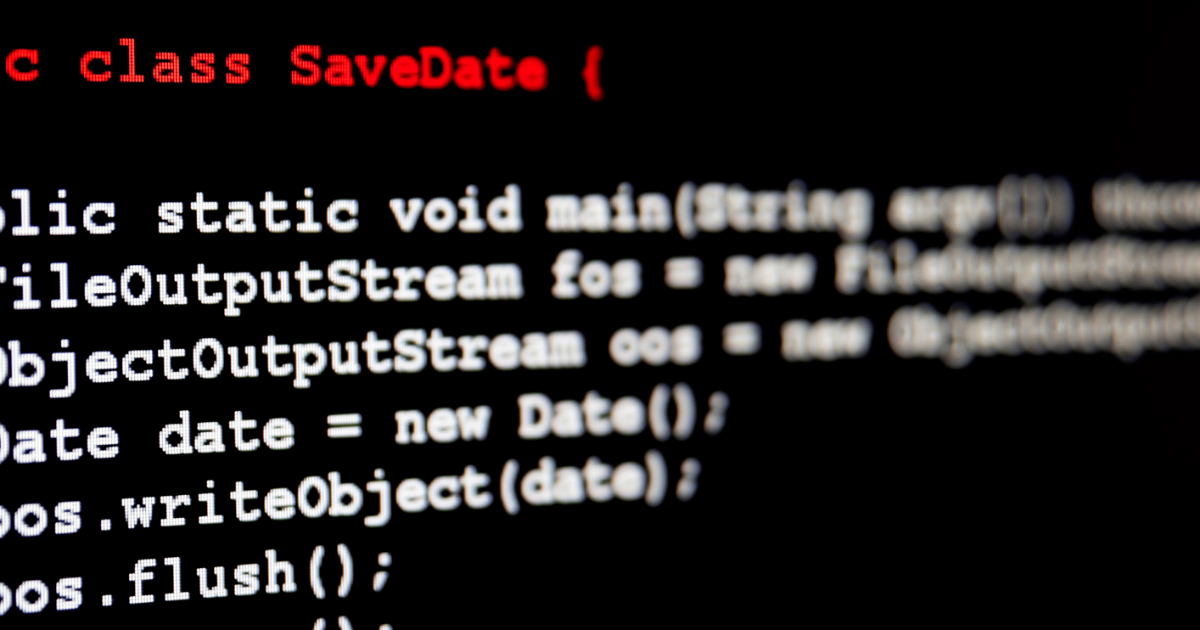
BellSoft has released versions 17 and 21 Liberica JDK, their downstream distribution of OpenJDK, with Coordinated Restore at Checkpoint (CRaC). This feature allows developers to create a snapshot of a running application at any point in time (checkpoint). This snapshot is then used to start the application in milliseconds by restoring the state of the application.
CRaC is based on the Linux feature Checkpoint and Restore in Userspace (CRIU), which means that builds are only available for the x86_64 and AArch64 CPU architectures running the Linux operating system. CRIU offers checkpoint and restore functionality and is used by various solutions such as Docker and Podman.
CRaC stores the state of a running application, including the Java heap, JIT-compiled code, native memory and settings. Developers should ensure that no sensitive data like passwords are present in the stored state. During initialization, a seed is generated via the Java Random
class, which means that the numbers after a snapshot restore are predictable. A new seed should be created in the afterRestore()
method in order to achieve randomness after a restore. Using the Java SecureRandom
class is an even better solution to clean the seed and lock the random operations before the snapshot and subsequently remove the lock in the afterRestore()
method.
The coordinated checkpoint and restore makes sure the application is aware of the fact it’s being paused and restarted. It makes sure the network connections and open file descriptors are closed to make the process more reliable. The process also allows the application to cancel checkpoints when it’s not yet ready because user data is being saved, for example.
The following command can be used to start MyApplication
and specify the checkpoint-data directory, which will eventually contain the JVM data, whenever a snapshot is created:
$ java -XX:CRaCCheckpointTo=checkpoint-data MyApplication
Now, the jcmd command may be used to create a snapshot:
$ jcmd MyApplication JDK.checkpoint
Afterwards, the application can be started by restoring the state of the snapshot in the checkpoint-data directory:
$ java -XX:CRaCRestoreFrom=checkpoint-data
Other solutions that allow faster startup of applications, such as Ahead of Time (AOT) compilation, used by GraalVM and Application Class Data Sharing (AppCDS) used by Quarkus, for example, also offer fast startups. However, those solutions don’t support further optimizations with the JIT compiler during runtime.
Bellsoft advises CRaC be mainly used for applications with the following characteristics: short running, low CPU limits, replicated and frequently restarted.
CRaC, originally developed by Azul, has become one of the OpenJDK projects. Azul has also included CRaC in Zulu, their own downstream distribution of OpenJDK. CRaC is becoming more mainstream and supported by tools such as Spring Boot, Quarkus, Micronaut and AWS Lambda SnapStart.
More information about using CRaC with a regular Java application and a Spring Boot application can be found in the How to use CRaC with Java applications blog, written by Dmitry Chuyko, Performance Architect at Bellsoft.