Month: January 2024
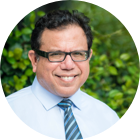
MMS • Anthony Alford
Article originally posted on InfoQ. Visit InfoQ
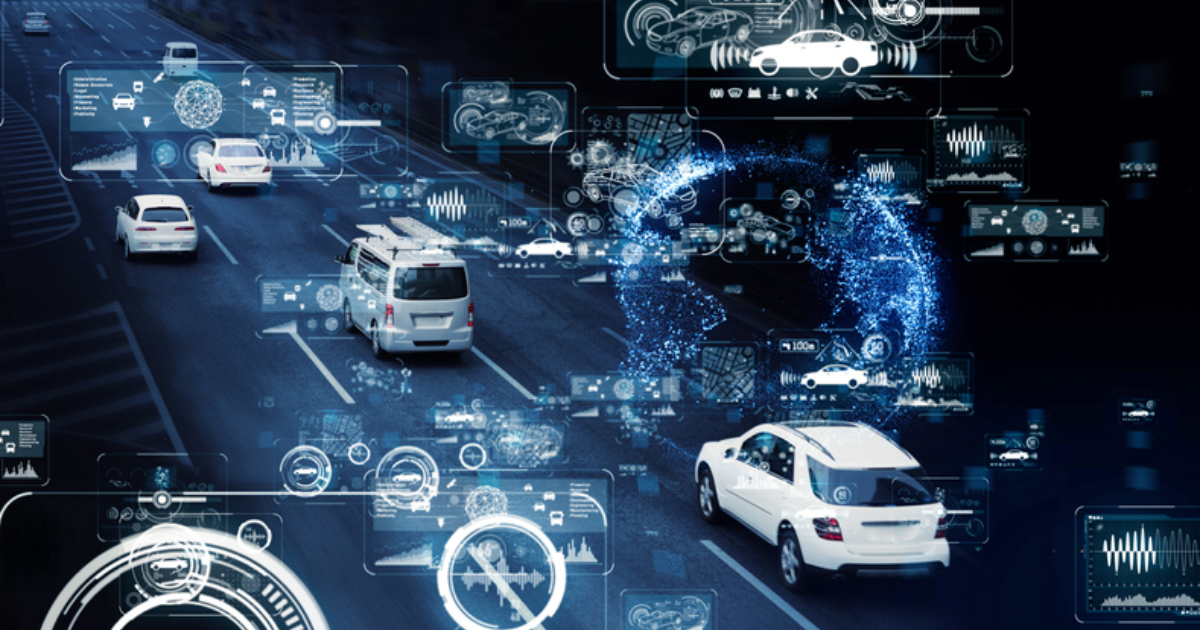
Alphabet’s autonomous taxi company Waymo recently published a report showing its autonomous driver software outperforms human drivers on several benchmarks. The analysis covers over seven million miles of driving with no human behind the wheel, with Waymo cars having an 85% reduction in crashes involving an injury.
Waymo compared their crash data, which the National Highway Traffic Safety Administration (NHTSA) mandates that all automated driving systems (ADS) operators report, to a variety of human-driver benchmarks, including police reports and insurance claims data. These benchmarks were grouped into two main categories: police-reported crashes and any-injury-reported crashes. Because accident rates vary by location, Waymo restricted their comparison to human benchmarks for their two major operating areas: San Francisco, CA, and Phoenix, AZ. Overall, Waymo’s driverless cars sustained a 6.8 times lower any-injury-reported rate and a 2.3 times lower police-reported rate, per million miles traveled. According to Waymo,
Our approach goes beyond safety metrics alone. Good driving behavior matters, too — driving respectfully around other road users in reliable, predictable ways, not causing unnecessary traffic or confusion. We’re working hard to continuously improve our driving behavior across the board. Through these studies, our goal is to provide the latest results on our safety performance to the general public, enhance transparency in the AV industry, and enable the community of researchers, regulators, and academics studying AV safety to advance the field.
Waymo published a separate research paper detailing their work on creating benchmarks for comparing ADS to human drivers, including their attempts to correct biases in the data, such as under-reporting; for example, drivers involved in a “fender-bender” mutually agreeing to forgo reporting the incident. They also recently released the results of a study done by the reinsurance company Swiss Re Group, which compared Waymo’s ADS to a human baseline and found that Waymo “reduced the frequency of property damage claims by 76%” per million miles driven compared to human drivers.
Waymo currently operates their autonomous vehicles in three locations: Phoenix, San Francisco, and Los Angeles. Because Waymo only recently began operating in Los Angeles, they have accumulated only 46k miles, and while they have had no crashes there, the low mileage means that the benchmark comparisons lack statistical significance. Waymo’s San Francisco data in isolation showed the best in comparison to humans: Waymo’s absolute incidents per million miles was lower there than overall, and human drivers were worse than the national average.
AI journalist Timothy B. Lee posted his remarks about the study on X:
I’m worried that the cowboy behavior of certain other companies will give people the impression that AV technology in general is unsafe. When the reality is that the leading AV company, Waymo, has been steadily building a sterling safety record.
In a discussion about the report on Hacker News, one user noted that in many incidents, the Waymo vehicle was hit from behind, meaning that the crash was the other driver’s fault. Another user replied:
Yes, but…there is something else to be said here. One of the things we have evolved to do, without necessarily appreciating it, is to intuit the behavior of other humans through the theory-of-mind. If [autonomous vehicles consistently] act “unexpectedly”, this injects a lot more uncertainty into the system, especially when interacting with other humans.
The raw data for all autonomous vehicle crashes in the United States is available on the NHTSA website.
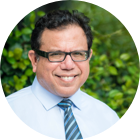
MMS • Abby Bangser Helen Beal Matt Campbell Steef-Jan Wiggers
Article originally posted on InfoQ. Visit InfoQ
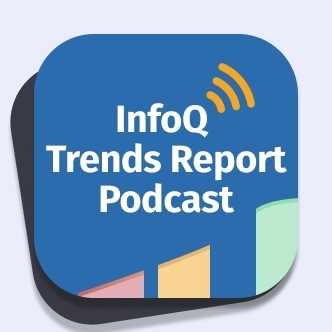
Subscribe on:
Transcript
Introduction
Daniel Bryant: Hello, and welcome to the InfoQ Podcast. I’m your host Daniel Bryant, and today we have members of the editorial staff and friends of InfoQ discussing the current trends within the cloud and DevOps space. We’ll cover topics ranging from AI and low-code, no-code, the changing face of Serverless, the impact of shift left and security and sustainability within the cloud. I’ll start by letting everyone introduce themselves.
Helen Beal: Hi, I’m Helen Beal. I am chief ambassador at DevOps Institute. Now part of the PeopleCert family, also chair of the Value Stream Management Consortium and co-chair of the Value Stream Management Interoperability Technical Committee at Open OASIS and a strategic advisor on Ways of Working. Lovely to be here.
Daniel Bryant: Thank you very much then. Abby, over to you.
Abby Bangser: Hi, yes, I’m Abby Bangser. I’m based in London as a principal engineer at Syntasso working on platform engineering solutions. I’ve also worked with the InfoQ group before, including just recently at QCon London with the debugging production track. Glad to be here.
Daniel Bryant: Fantastic. Matt, over to you.
Matt Campbell: Thank you. Matt Campbell, I’m based in Canada, lead editor for the DevOps queue for InfoQ and then also VP of Cloud Platform for education company called D2L, where we do a lot of platform engineering stuff.
Daniel Bryant: Fantastic. And finally, last one or least Steef-Jan, over to you.
Steef-Jan Wiggers: Yes, Steef-Jan Wiggers. So I’m based in the Netherlands. I’m a lead cloud editor at InfoQ for the last couple of years. My work as an integration Azure architect for i8c in the Netherlands. And Yes, I’m happy to be here.
Is the cloud domain moving from revolution to evolution? And is DevOps dead? [01:25]
Daniel Bryant: Fantastic. Thank you so much for taking time out of your busy days to join us. We’ll launch straight in, try not to be too controversial on the first question, right? But I want to set the high-level piece. Now, two things with the cloud and DevOps space, I’m hearing cloud innovation is slowing down. Our colleague InfoQ Renato did a fantastic piece covering Amazon reinvent and even he said that it’s more evolution rather than revolution. Also, we’ve been seeing here is DevOps dead. We had some interesting conversations around that. So I love to get everyone’s thoughts in terms of what do you see the big picture with cloud and DevOps? How are things moving? Where do you think things are going?
Helen Beal: I think it’s probably true. The innovation has slowed a little bit around clouds. I mean it’s gone really, really fast for a long time and now a lot of people are trying to adopt it. I think we’ve got over things like lift and shift and learned a lot about not doing things like that, but there’s still a lot of work for people to move workloads and re-architect workloads and things like that and adopt the things that have been invented for them.
As for DevOps, definitely not dead, don’t be ridiculous. However, I would say that it has some problems sort of 13 years into its journey. It’s reached some stagnancy, maybe dormancy in some organizations they’re struggling to really get it to the next level. That’s personally one of the reasons I’m particularly interested in Value Stream management is I see that as an option to help people unlock the flow and the value realization parts of it, but definitely not dead. Absolutely in the mainstream, lots of organizations still working on getting it right in their organization. So I’m going to ask Abby to respond and let me know how much of what I’ve said she agrees with.
Abby Bangser: Yes, I think that whole, just to go for the easy one first, I agree on the cloud space. I think there’s been a lot of evolution towards the original goal of cloud computing, which is access to the resources that you need on demand and with easy scalability. And we can see that now moving across more and more types of resources and becoming more ubiquitous, which is looking like evolution even though in those spaces it’s revolutionary. I think that there’s the DevOps conversation’s really interesting ’cause as you say, the again, intention of DevOps, just like the intention of cloud I think is still alive and well.
The intention of giving people access and autonomy in their teams to create business value without blockers is still something that we are all striving for and working hard towards. I think the concept of trying to say it is a developer person and an operations person sitting together on a team was never quite the intention, but was the attempted implementation, which we’re now seeing cracks in after years of trying to do it. And I think that’s showing rise to some of the other changes we’re going to be talking a bit about in platforms, internal platforms, and other things. So absolutely.
Daniel Bryant: Matt, you’re nodding along there a few times.
Matt Campbell: Yes, I would agree. I think one of my favorite topics of late is the sort of cognitive pressure that people are put under. And I think Abby, you were hitting on that and Helen as well, where there’s just in the approach to try to localize the development to try to make sure we could deliver business value faster. We also overloaded teams and even with all the cloud innovation that’s happening, every time something new comes out, you have to figure out how that’s going to fit in your worldview. How I’m going to integrate that into what I’m doing. Does it add value to what I’m doing? And I think we’re seeing everyone has a lot on their plate. The tech landscape changes very quickly. Businesses want to evolve and adapt quickly as well. So I think it’s all somewhat interconnected with the slowdown in that we’re now in a phase where we’re trying to figure out how do we sustainably leverage all of the cool stuff that we’ve invented and created and all these ways of interacting with each other and move it to a place where we can innovate comfortably going forward.
Daniel Bryant: Love it. Steef-Jan, so you’re nodding along there too.
Steef-Jan Wiggers: Well, I did see a massive adoption of the cloud predominantly because of COVID. Yes, I agree that a lot of that gives a burden on development and even I have to deal a lot in the cloud, and this is in some evolution with regards that also concurs with DevOps is where the automated setup environments. So recently some of the cloud providers like Azure have this automated way, not just setting up a dev box but also complete environments. So I see some evolution in that area as well that we start lifting and shifting that type of way so that you have a completely development test, automated development street basically setting that up that we say, “Yes, we try to get the dev and ops sitting together.”
Because I usually still see a little bit of a wall between them and it comes around to identity and access management, that kind of stuff. You still need access to certain resources, or you need to access certain tiers and then you don’t get them because someone on the DevOps side, “Well, I haven’t got the time for it yet.” So some of that stuff is not yet automated. So I still see a little bit of a gap there too, just from personal experience. I still feel that dev and ops, there’s always this little boundary between them, and has to do with predominantly identity and access management.
Daniel Bryant: That’s very interesting, Steef-Jan. I definitely think the whole DevOps, I like everyone sort of riff on that. Joking in a presentation I did a few years ago, said it’s like should really be BizDevQaOps at least, right? There’s a whole bunch of things that should be in there, but you know what developers are like, well engineers, I should say architects, we just like that snappy title. So I think that was a great call out, which I’m sure we’ll dive into many times throughout the podcast.
What is the current impact of AI and LLMs on the domains of cloud and DevOps? [06:19]
I did like their focus on revolution. And Matt, you mentioned about sort of cognitive overload, those kinds of things popularized by Team Topologies, which I think we’ll cover as well. But on that notion, we can’t get away from the impact AI and LLMs in particular had over the last six months and definitely since the last trend report we did. I’d love to get folks thoughts on what value does AI add to someone who’s going to be listening to the podcast either as an engineer, an architect, a technical leader. What do you think the immediate impact is of these kind of large language models and where do you think it’s going?
Helen Beal: Well, I can start again if you like. I mean yesterday, funny enough, I was doing a similar one of these about observability, but we ended up talking about AI quite a lot and initially in the context of AIOps, which of course isn’t so much about the large language models but more about algorithms and machine learning and absolutely what Matt said about cognitive pressure, cognitive load limits, and humans is a big problem when we are dealing with lots and lots of data coming out of lots and lots of monitoring systems and creating huge amounts of alert noise. So AIOps has a very strong use case there in this instant management, which is where we started having the conversation. But we of course got onto ChatGPT and the generative AI as well. And one of the particularly interesting use cases I found, or I heard from the team yesterday was around, again, instant management, but this time ticketing.
So developers probably remember things like the rubber duck. You’d have a rubber duck on your desk and you’d have to talk to it if you had a problem before you were allowed to bother somebody else with your problem because, generally, you’d find if you had a conversation, you’d come to a solution and they’re doing a similar thing at the insurance company that was on this talk yesterday and they’re actually using it in their ticketing system. So instead of raising a ticket, it’s encouraging you to chat with the AI and then once you get to a point it’s like, “Actually, no, we are going to have to raise a ticket for this thing.” And I think there was a few other ones, of course, the developer kind of Copilot things are getting code snippets and I think there’s just a huge opportunity here for some leapfrogging.
I see it in some of my daily work. So for example, recently, we had a problem where we were writing a new course, and the guy that was writing it was running out of time and we needed some instructor notes writing, and usually in the past that would’ve meant I’d Google the topic, spend 15 minutes digesting the information and then regurgitate something into an instructor note, but I could leapfrog sort of 15 minutes of work by asking ChatGPT the question and then validating the information and shuffling it around into taking the right bits out and the bits I didn’t want out as well. But another slightly off-topic, but interesting ChatGPT story. I was doing some work on a novel that I’m writing this morning and a scene I’m particularly writing, it’s set on a celebrity cooking show and somebody’s about to sabotage the cooking competition.
First of all, I did it in Sudowrite actually, which is a large language program for writers, and it came out with all sorts of suggestions about fiddling with utensils and ingredients. And then I stuck it in Bard and Bard said, I’m a large language model thing and I can’t help you. And then I stuck it in ChatGPT and ChatGPT said basically, I’m morally not allowed to help you with that. You’re trying to sabotage somebody. So I thought that was great though, seeing the ethics going on in the large-scale open tools was fun. Anyway, probably a little off-topic, apologies for that.
Daniel Bryant: Who would’ve thought cooking in the InfoQ Cloud DevOps podcast? Fantastic. Anyone else got any thoughts on that?
Steef-Jan Wiggers: Well, Yes, I do. From more than Microsoft side, I see a huge push through open AI initiatives. Like Helen mentioned, Copilot, so many of the Azure and even Microsoft Cloud products or services now have a Copilot. So you see it in their tooling like Visual Studio, Visual Code, you see it at Microsoft and Dynamics side that they have Copilots, A lot of the services Microsoft, even something recent, the Microsoft Fabric, which is a complete SaaS data lake or lakehouse solution they have is completely infused with AI. So Yes, you definitely see a big push in an investment on the Microsoft side.
Abby Bangser: Yes, I think to your question of what would listeners care about in this space, I think the big thing is just to start getting involved and exploring what is possible and not possible because it’s very easy to poke fun at the prompts that show up on social media of look at how silly this AI is. The robots are definitely not coming for us because it’s true. There are things that you do need to validate. Helen talked about getting information back and then validating it before going off and posting that to a project or to a course. But at the same time having an idea of what can be done for you, even if it’s not yet in the language models today, and where you will be able to add value is I think a really big piece of what we’re trying to figure out right now.
And we’re also just trying to figure out what that evolution will look like. And I think that prompting, there’s that joke about being a prompt engineer instead of being a software engineer. I think it’s a joke today to many people, but actually, that ability to be creative and to think critically about the problem in order to generate useful suggestions that’s effective in a meeting that’s going to be even more effective when you start applying things like a large language model to it. So I think these are all the things that are coming down the pipe at us because of this new technology.
Daniel Bryant: Fantastic. Matt, any thoughts on that one?
Matt Campbell: Well, it sounds like DevOps is going to get replaced by DevPromptOps then.
Daniel Bryant: Yes, love it.
Matt Campbell: Is what you’re saying there. So Yes, I love the idea of leveraging tools like this to make our lives easier. I think that’s always been the dream of technology is that we would have flying cars and be able to not have to work as much. And in some cases, kind of circling back to the first question, a lot of the innovation I think has actually maybe made us work harder in order to achieve some of our outcomes. And I love the idea going back like Helen you were talking about with AIOps and using that for monitoring and especially with distributed systems, as you get observability in there, it’s really hard to try to trace down where something’s actually broken. And having a system be able to do the first stab at that and report back, maybe look over here and remove that something recent at work, somebody wanted to learn how to use Antlere to help process a large amount of data that we had and used ChatGPT to kind of help construct some of the initial scripts as a way to guide their learning in that.
So using it to help prompt you and fill in gaps and give you a jumpstart. I think that as an initial jump-off point is pretty awesome usage of it. I’d love to see more developments in that area.
How do AI-based and ChatGPT-like products impact the low-code and no-code domains? [12:12]
Daniel Bryant: Yes, no, I love all the great sort of comments about the validation, the ethics involved. Sure. And I think we’re all saying the Copilot, the pair programmer, the summarization is super useful. And I was kind of wondering, I was doing a lot of work back in the low-code space a while ago and what do you think the impact of say AI is on low-code? Is it disrupting it, right? The whole low-code, no-code space, or is it going to augment it in that the tools to make things simpler were already there and now they’re perhaps going to get even better. So I’d love to get people’s thoughts on where do you think these low-code, no-code tools are available in the cloud. Where do you think they’re going to go with the impact of AI?
Helen Beal: Funny enough, that came up in the conversation yesterday as well when we were doing takeaways in the same chat from the insurance company that I was talking about when I asked him for his vision of the future, that’s exactly what he wanted. And as a head of DevOps in the technical team, what he actually wanted is his business teams to be able to use AI so that they could drive particularly things like self-healing and remediation around their core business systems. So people are definitely looking for that connection. I haven’t seen anybody having it yet, but something that definitely I’ve seen people have appetite for. Has anybody seen it in action in the real world yet?
Daniel Bryant: So listeners to the podcast, there’s a business opportunity there, right?
Helen Beal: A huge business opportunity. And I found it really interesting because traditionally when I’ve been a consultant, when we’ve had conversations about businesspeople doing stuff with systems, people have started shouting about shadow IT and it being very undesirable to have these businesspeople having their hands in the systems to that extent. So I found it quite interesting that we seem to be turning a corner because AI is actually supporting the businesspeople giving them an amount of knowledge that is probably safe. The small amount of knowledge can be dangerous. It feels like actually AI is lifting us out of that space where we can be trusted. And perhaps in the point that I made in response was it would be great to stop having this division between product management and software engineering. The business as people call it in technology and actually having us collaborate as single value streams would be fabulous.
Abby Bangser: I think it’s really interesting with that point about how dangerous is it to have someone who doesn’t particularly know about code to use these low-code platforms. I think that’s been the biggest debate on their uptake. And I’ve been hearing a lot of increased conversation about ClickOps, which used to be talked about in the sense of clicking around a browser to be able to solve your problem. But today’s products and leaders in that space are talking about how you enable people to click around and do things if that is the right interface for them, but you result in code or something that is version controlled, is declarative in nature, and can be understood and evolved.
And I think that the exciting bit about the LLMs and the AI side of things is that we’re getting better and better code generation. We’re seeing that with Copilot, with Codeium, with lots and lots of other tools. Can we get even better code generation, code generation people can actually use that, don’t want to click around the UI can actually read, can actually evolve, can actually meet standards of our organization’s code. But from that no-code, low-code spot, I think that will be an interesting evolution.
Steef-Jan Wiggers: Yes, I think so too. If you can prompt or talk and then have it out visualized through low-code. I’ve worked a lot with low-code platforms at least in the Microsoft space with logic apps that it can be out generated and then you have your business process right in front of you. You could do clicking it around, that’s correct. But usually, it’s left up to us instead of left up to them. But if you can just have it generated and then your business process right in your face it runs and there’s always kind of a code-behind stuff that is generated for you. So that can definitely work.
The interesting thing though would be in the end of the day, the governance regards to accessing certain part of data. It’s always been the case with low-code. Even if you look at the low-code platforms on the Microsoft side and the cloud side, which is I feel called power automate and that kind of stuff. And then it’s easy for them to use even businesspeople and they call them citizen developers, but in the end of the day it’s also about the data and the governance that they are using at least access to data and then sometimes it gets a bit weary that they get too much power or too much access to certain data.
Matt Campbell: I think that’s the sort of merger between the low-code and platform engineering and that we want to provide a safe platform that complies with our governance needs but also empowers the users of it to be able to get their job done. And I do see even with some of the low-code systems being challenging for end users to use, so getting some of the prompt engineering and ChatGPT stuff into that I think could be helpful in almost telling it what you want, and it kind of helping to build a skeleton for you to flesh out. My guess is we’re going to see a lot more evolution in this area as we look to try to drive more business value quickly and then also see this start to Steef-Jan’s point there, improve in the layer of governance and the platform engineering kind of DevOpsy layer of that so that those platform teams can take care of that part for the users of the low-code without them needing to worry about that or worry about crossing any boundaries you don’t want to be crossing.
How will Platform Engineering evolve? [16:59]
Daniel Bryant: Fantastic summary everyone. And I’ve heard several times sort of implicitly we’ve mentioned about platforms. Now, most of us here have been building platforms for 10, 20 years, but platform engineering has become a thing du jour. People have really locked onto that with good reason like DevOps, many of us are doing DevOps before it became a thing, but giving us a label does help build a community and drive the principles of that space forward. So I’d love to get folks thoughts around platform engineering. Particularly, I was thinking of things like Kubernetes and Service Mesh, all the rage for the last five years. Are they getting pushed down to everyone’s points about hiding some of that complexity, right? Reducing some of that cognitive load. And Abby you pointed out in our sort of a chat before their podcast started around Team Topologies going through a new evolution as well and that everyone, I seem to be chatting to you too is adopting some form of Team Topologies and that platform as a service, not as in paths, but more as in offering a platform as a service is seemingly everywhere at the moment.
Abby Bangser: Yes, I think it’s really interesting to see something like Team Topologies in the early majority and something like self-service platforms in the late majority. When I look at what I see around the industry and around the people that I work with. And I think that one of the big things is what does self-service actually mean in these organizations and what application of Team Topologies is being applied, how much of it is in theory agreed and how much of it’s really driving how you organize your teams and how you organize also their interaction models, not just how you organize their teams but how they interact.
And so I think what I’m seeing is with self-service, a lot of teams are saying they have self-service. I, a couple of years ago was saying I had a self-service platform and the way that people would self-serve from that is they would make a pull request into a repo with some code because they would have to have learned how to use a Terraform modular or how to have extended a YAML values file or something else which was not core to their business value delivery and still required some level of a ticketing system.
It’s different than a ServiceNow or Jira or something else. It is a pull request in GitHub, GitLab and other repository types. But it’s still a ticketing system that relies on a human. And I think today we’re talking a lot more about what is an API, what is something where we can actually separate the concerns of the consumers being the application developers or other members around the organization and the producers being the platform team. And I think that’s where that revolution right now is happening with people because of Team Topologies conversations.
Helen Beal: I’m particularly interested in its application within Value Stream Management as well when we look at the streamline team as being what I would call the kind of core team that’s doing the work that’s going to make the difference to the ultimate customer. And then we’ve got the supporting type teams like the platform teams that help the streamline teams do that work. My particular favorite pattern for the platform team isn’t in the cloud actually it’s in the DevOps space. So it’s historically when we’ve seen adoption of DevOps and specifically CI/CD pipelines, we’ve seen the mushroom up in little development teams all through an enterprise and there becomes a critical mass where people are starting saying, “Well, we’ve got all these different tools we standardize, then there’s absolute uproar because people will get very emotionally attached to their tools quite understandably.” But then you get a whole other group of people in an organization that are desperate for the DevOps tool chains and don’t have the skills and the knowledge.
So actually, having a platform engineering team or a platform team that provide a DevOps tool chain as a service solves a few problems. It makes the whole technology stack much more available to the people that haven’t yet got there. And then kind of enables the conversation around standardization, which is much easier to have if you talk about the kind of architectural configuration of a tool chain rather than the specific flavors of tools within it. And then that central team can talk about what they’ve got skills to support and what might be exceptions that people will need to support themselves if they want to continue using them.
Abby Bangser: I think I just quickly add to that ’cause I think it’s a really good description, how much the change in the perception of the platform engineers on the platform team as a service team to the rest of the organization rather than as the keeper of the complex bottom layer information is what’s really driving all this change instead of being like, “You will use our tools ’cause we told you, you will.” Even if you really attach to the things that you used to use, they are no longer compliant and they’re no longer cost-efficient or sustainable. The engineering teams don’t care about those things. They want to use the things they want to use, and they want to go to production quickly. And today, platform engineering teams are learning about what developer relations looks like and marketing and what does it look like to actually engage with customers and get feedback and have a roadmap that meets their needs, and the business constraints of the platform engineering team is managing and working under.
Matt Campbell: Those are all fantastic points. And Daniel you kind of mentioned like platform engineering is not a new thing as many things in our industry, it’s an old thing that maybe some of us forgot about and then now we’re remembering, and it feels like a new thing. But there are new parts to it I think that maybe we’re bringing up, and Abby you kind of just commented on those where we’re as opposed to treating platform engineering as a thing we build that people must use, we’re trying to find ways to build things that delight our end users and actually drive value and make them want to use it because of the value that it’s adding.
And I think in that regard, Daniel, to your initial question, we are seeing sort of the technologies pushed down a little bit and Helen to your point, that API layer, that interface layer being added to simplify the interaction to help drive better business value and to make it easier for teams to get in there and not have to learn all the intricacies and all the fun stuff that YAML brings to the table as you mentioned, Abby. So Yes, it’s a really exciting time and a really interesting evolution that we’re seeing there. I really love the platform as a product framing that we have this time around.
Daniel Bryant: Yes, love it, Matt. Just to double down on that, when I was at KubeCon, Kubernetes conference, right? I was chatting to folks and the overwhelming anecdotal data that people were pushing Kubernetes down the stack at KubeCon. I find this kind of bizarre, right? My engineers just want to go into some interface and define something or call some API, Abby’s point. And I was like, “Wow, this is KubeCon, right? And it’s really interesting seeing the evolution of trends at a conference where it’s really like about the technology I’ll give you, right? And now it’s pushing more towards the platforms.” I saw a lot of push towards observability, which we’ve covered a few times as well, but not just observability in terms of say SLI service level indicators, but also things around KPIs like key performance indicators. And a big one of that was financial folks are now, particularly in this non-zero interest rate phenomenon we’re going through now folks are really concerned about how much my spending on this platform, no more spinning up some huge database poking around, shutting it down, whatever. You got to justify that cost.
Is FinOps moving to the early majority of adoption? [23:20]
And I think Steef-Jan, you mentioned sort of offline around FinOps going into the early majority. I’d love to get your take on that because I think I’m hearing more about FinOps. I initially learned it from Simon Wardley, fantastic blogs out there, but now I’m seeing it very much go more into the mainstream as you’re pitching Steef-Jan. So I’d love to get your thoughts on that.
Steef-Jan Wiggers: Yes, that’s correct. There’s more companies joining the FinOps Foundation which provides the support and processes around FinOps. I do see a lot of tools popping up that can support your FinOps and give you look into what you’re actually spending, but I feel that it’s also about important about the process and what are you spending versus what value are you getting out of it versus just looking at tools because Yes, the tools give you an idea, “Okay, I’m spending, but it’s the spending what you do just justify it or not.” And that’s what I think is great about FinOps Foundation coming in and more and more companies like Microsoft and I think other companies, cloud companies as well, just adopting it and are part of that journey as well. There’s even ways of getting to know the processes. I think you can even do certifications. In my line of work, I see it more and more the discussion.
We have this running, but are we actually using it? We’re not using it, we just get rid of it or we just turn it down. So even I see that back in our discussion as well, why we have things running? And even on the personal side, I’ve got an MVP subscription which allows me to basically burn money, but then I’m even, I’m starting to get them where ID demos, I’m not using them, you know what, I’m just shutting them down. Although the credits are free, but it doesn’t make sense. So even I’m studying in more aware because FinOps also has to do with sustainability, which I learned during our latest QCon in London from Holly Cummins with this light trade shop making us aware that we have stuff running that costs money. And I think it’s even like 21 billion a year or two ago they were figured out all the cloud costs combined. It’s insane. So it makes us aware that we should only spend when we should spend like a flick of a switch with energy.
Helen Beal: I think it’s related to some of the sustainability and GreenOps things as well. So with the AIOps space, we’ve been having quite a lot of conversations about data and the fact that it seems like you might need to have a lot of data and therefore a lot of storage in order to get the information that you want. But making the differentiation between data at rest and data at motion is quite important in that context. Additionally, the AI steps in, again, obviously that’s part of AIOps, but another way that AI can help us in your classic big data scenarios, increasingly it can identify where you’ve got data that’s not being used where there’s no APIs or anything that is calling. It’s not being used as an asset by any of your applications. So increasingly AI is becoming like a data housekeeper where it can tidy up and save us space. And that’s good from a planetary perspective as well as a personal kind of or a company financial perspective.
Are architects and developers being overloaded with security concerns when building cloud-based applications or adopting DevOps practices? [25:55]
Daniel Bryant: So changing gears at InfoQ, we’ve definitely seen a lot of attention being put on security now with the software supply chains, SBOMs, lots of great technology going on in that space as well. When I go to conferences, I’m hearing anecdotal information from developers that they’re getting a bit overwhelmed with this whole shift left. Some folks are even jumping, it’s more of a dump left as in they’re just dumping all the responsibilities left. Do you know what I mean? Rather than actually thinking about how can developers think more about security? I need the tools, I need the mental models. So I’d love to hear folks’ thoughts on, are you seeing folks in the wild caring more about this? Are they implementing more of the solutions and what’s the impact on developers and architects?
Steef-Jan Wiggers: Yes, I see a lot of them in my line of work. So security becomes more important. You write about the shift left, at least developers are giving the burden of making things more secure when it comes to cloud platforms. They have to deal with the operational side of things too, with identity and access management, but also their, let’s say functions or whatever they’re running like code, accessing what type of system, what type of security model is in place predominantly or most commonly or ALF. And Yes, that’s kind of what you need to do. You need to go to the identity provider to get the token and then access the service or even some of the platform have something like menace identity. So then the cloud platform takes away that and then you have to be aware of that and then it comes back to giving the menace identity access to whether it’s also key vaults and that kind of stuff. So it all migrates into that developer that needs to be aware of all these nitty-gritty details. So it’s definitely can be overwhelming if you’re less say security aware.
Abby Bangser: It’s definitely important. And I’m seeing more of a push towards it by leadership, which is usually the thing that pushes security onto the developers, right? Because developers and not every developer, but many that are there, their first instinct is not to go straight to the SBOMs, right? If they’re being pushed for new features, that’s what they’re focusing on. But when the company has raised that the risk there for security is important enough, they will take on that responsibility and do that work. I think the interesting thing about securities, it’s going through a similar evolution and growth pattern that a lot of technologies go through, which is the first solutions are made by experts and they often need to be used by experts. And eventually, those start to get smoothed to out and become easier to be used by other people in the industry more. And I think that’s maybe the phase that we’re getting into now with the security tooling is people are realizing that some of the early days security tooling isn’t quite user-friendly and the current tooling is trying to evolve into more user-friendly ways of working.
Matt Campbell: Yes, I would agree that there’s definitely a lot of research into SBOM specifically about questionable implementations and value of them and are they actually delivering on what they say they’re going to be delivering. And then I think to your point, Abby, there’s new frameworks coming out like salsa that as just a product developer might be too in-depth for me to actually be able to get into and understand how do I actually do anything with this? And I think the gap that we need to look to fill is circling back to Team Topologies, is treating security as an enablement function and finding ways to have that team build platforms that can help with some of these things, but then also enable through education to make sure the teams understand the important parts of the things that they need to do as they’re working through the code.
I would agree it definitely feels a lot like a dump left at this point with just all these things going on and the big push from leadership, even from the government level to help shore up the supply chain is leading to a lot of… To your point Abby, like expert-driven implementations, which don’t necessarily translate well to ground-level trying to get it into your code.
Is WebAssembly a final realisation of “write once, run anywhere” in the cloud? [29:22]
Daniel Bryant: Now I know we’re mainly focused on high-level techniques and approaches in our trend report, but I did want to dive a little bit deeper for a moment on a couple of cloud technologies. I think these are having an outsized impact and the first one is WebAssembly, Wasm, and the second one is eBPF, which we’re seeing a whole bunch too. Now I’ve just got back from a fantastic event in New York, QCon New York, and there was a really good presentation by Bailey Hayes from Cosmonic on WebAssembly components. I’ve also been reading a whole bunch from Matt Butcher and the Fermyon team about what they’re doing with WebAssembly too. I’m really seeing this promise of the right once run anywhere, which I appreciate is a bit ironic coming from a Java developer. I’ve worked in Java for 20-plus years, and this is one of the platform’s original promises, but I think this is the next evolution of that promise if you like.
Now, both Bailey and Matt and others have been talking about building this case for reusability and interoperability. So the idea with WebAssembly components that Bailey was talking about is you could build libraries effectively in say go and then call them from a rust application and any language that can compile down to Wasm, this kind of promise stands, which I thought was really easy for that true vision of the component model within the cloud. I also thought it was super interesting. You can build your application for multiple platform targets. Now, given the rise of ARM based CPUs across all the cloud hyperscalers and the performance and the price points, this is really quite attractive. I know Jessica Kerr has talked to InfoQ multiple times about the performance increases and some of the cost savings as well that the Honeycomb team saw when they migrated some workloads to the AWS Graviton processes from Intel processors.
So very interesting thinking points there. I’m also seeing Wasm adopted a lot in the format as the cloud platform extension format. For example, I grew up writing Lua code to extend NGINX and OpenResty, and now we’re increasingly seeing Wasm take that space. They’re being used to extend cloud-native proxies like Envoy and the API gateways and service meshes that this technology powers. In regard to eBPF, I’m definitely seeing this more as a platform component developer’s use case. So maybe we as application engineers won’t be using it quite so much. But I’m seeing the implementation of the CNCF’s contain network interface such as cilium, I shouted to my friend Liz Rice and the Isovalent folks doing a lot of great content on this and also seeing a lot in the security space on this. Project Falco or the Falco project and the Sysdig folks are embracing eBPF for security use cases, really nice general-purpose security use cases. And Matt, have you got any thoughts on this too?
Matt Campbell: Yes, no, definitely. We’re seeing a lot of really interesting usages of this technology within observability and then also circling back with security as well because it’s allowing you to get closer to the kernel, closer to what’s actually happening on your systems within your containers. So you’re getting quicker in some ways, more accurate, in some ways safer, less likely to be potentially tampered with in the event of something malicious going on data about how your system’s operating. So that’s been the major push that I’ve seen usage of this. I’ll admit this is not an area that I’m super well versed in, so I’m not going to make any predictions about where it’s going to go, but I think those current usages have definitely been really interesting.
How widely adopted is OpenTelemetry for collecting metrics and event-based observability data? [32:21]
Daniel Bryant: Fantastic, Matt. Fantastic. Should we move on to OpenTelemetry? I saw a couple of folks highlighted OpenTelemetry, and again, we’ve mentioned observability several times in the podcast, and I keep hearing OpenTelemetry all the things because it is great work. I’m lucky enough to work with you, other folks in that space. I’d love to hear folks’ thoughts on where you’re seeing the adoption and what do you think the future is on this space?
Helen Beal: So for me, it’s gone really rapidly in my AIOps world from a, “This is coming soon to, this is what you should be using de facto standard, everyone. It works really well, really, really fast.” Like faster I think than I’ve seen anything else be adopted. Abby’s nodding, she agrees to me.
Abby Bangser: Yes, I just think it’s something that took a long time to get to version one in a lot of languages, but once it got there, it had been being built by so many amazing people around the industry and was cross-vendor to begin with. There was many vendors that were actively putting their engineering time into building this the right way that I think it became quite easy to call it the de facto and it’s so core to your applications, it needs to be that cross-language friendly in order to be used across your platform. So absolutely.
Steef-Jan Wiggers: Yes, I’ve seen it too. Like Helen said, I agree. I see a rapid adoption too because Yes, last year I’ve never heard of it, but now I have, and it’s even Microsoft, even our bracing it and putting it into Azure monitor and stuff. And last QCon London, there was a great presentation on OpenTelemetry. I came about people like Honeycomb IO that have adopted it, so it’s cross-vendor. So there’s a lot of adoption there too. And it’s something similar… I wish happened in the IoT space, getting something standardized. So if it’s around eventing, right? Event driven architect, you’ve got something like cloud events, pretty rapid adoption. You see some services and left and right in cloud and even in Opensource world where they have tooling around event driven architecture with cloud events, and then you have symbol and with OpenTelemetry, right? You got this standardized that they agreed upon and then it becomes vendor-agnostic and that’s super.
So you create telemetry and then you export it and then you can use X amount of tools that all have their own little thing that can do with the telemetry. That’s what I’ve learned from people during QCon. Some of the vendors are supporting it. Yes, we have our own little thing where we do something with memory consumption and execution and that kind of stuff. Yes, it’s pretty interesting. So Yes, even I am excited.
Daniel Bryant: That’s high praise, Steef-Jan. High praise.
Abby Bangser: And I think the vendor-agnostic bit, you can think of it as, “Oh, it’s no vendor lock in. We get away from vendor.” But as you say, what it gives is actually a requirement now that the vendors optimize and provide something that’s more interesting, it’s no longer that they’re competing at getting to the baseline. I can collect your metrics, your events, your traces, and visualize them. Now they have to be doing the next level of interesting thing for them to gain customers and gain market share. That’s the really big power in my mind around something like this. OpenTelemetry or other open standards is the movement of the industry that happens once standards come about.
Daniel Bryant: One more technology thing I’d love to quickly look at is Serverless. Last year we said Serverless as a baseline and we see Serverless popping up all over the place. Steef-Jan, I was reading one of your news items, I think it was recently about Serverless for migrations, things like that. I don’t hear the word Serverless that much anymore, but is it because it’s becoming just part of the normal choices? We push the technology away, but we are embracing the actual core products that use it. So Yes. What’s thoughts on adoption levels of Serverless?
Steef-Jan Wiggers: Well, I think Serverless becomes just managed. We have a managed service for you. And then usually a lot of these services have something like outer scale in it and you have units that you need to pay for. So there’s the micro billing stuff. So it’s what you consume and it’s all migrated into what we call a managed service. Sometimes you see popup, Yes, we have a tier in our product that now is called Serverless something, Serverless database or Serverless, any type of resources when it’s AWS, Google or Microsoft, a lot of their services now also have some Serverless component in that too, which means the outer scale, which means the micro billing, which means all the infrastructures abstracted away, which you don’t see. So making it easier for the end user basically. And sometimes dropping even a name Serverless.
Daniel Bryant: Nicely said.
Matt Campbell: Yes, that move from sort of Serverless as an architectural choice of say building on Lambdas versus building on virtual machines to Steef-Jan’s point versus moving to managed services. So I think that’s the transition we’re maybe seeing here. I think Serverless became that kind of proverbial hammer and then everything became a nail where we’ve seen a few cases recently, some very overblown in the news of companies moving from Serverless back to monolithic applications. But the move towards managed services really does fit the mold with the platform engineering approach. Trying to reduce cognitive overload, shifting who is working on what to other people. For most of our businesses, our customers are not buying us because we built the best Serverless architecture. They’re buying us because we built the best product. So having somebody who’s an expert in running those managed services, run them for you is just good business sense in a lot of ways. So I think that might be the shift that we’re seeing here.
Abby Bangser: Absolutely, and I think one of the things we might be seeing is the impact of the value Serverless actually coming out in other ways at this stage. And again, moving on from the architectural decision and saying, “What does Serverless give us?” It gives us scaling to zero. It gives us the ability to cost per request. And so we talked about FinOps already. Your ability to understand where to cut costs has to be dependent on what is your cost of customer acquisition? What is your cost of customer support and of a request. That’s where Serverless really shined and now people know that’s possible and they’re asking for that. Now, does it have to be done through a service architecture? Absolutely not. But it’s something that now the organization is asking from their engineering team and that’s coming out in other architectures as well.
How is the focus on sustainability and green computing impacting cloud and DevOps?
[37:58]
Daniel Bryant: So we’ve talked about the changes of pricing several times there, and I’d like to sort of wrap this together in terms of Steef-Jan, you’ve touched on it earlier on, of pricing relating to the impact and sustainability, things like that being sort of charged maybe on how much you are consuming or polluting, arguably these kind of things, right? I’d love to get people’s thoughts on the adoption level of thinking about prices in architectures and choosing managed services as Matt said there, and where you think that’s going in the future.
Helen Beal: I’m interested to hear the others’ view on how much of this falls into the SRE camp and into the SLXs because I can’t be bothered to say them all, but it feels to me like this is the natural kind of node of the technology team to figure this stuff out. What do other people think?
Steef-Jan Wiggers: Yes, I agree. It’s my day-to-day. If you start thinking about architecture and then thinking about the services, how you want to componentize, how you want to create the architecture, and then it comes boils down to, okay, what type of isolation do, or do we not need security in the end? Then you’re coming into the price ranges of what type of products. If it’s completely what I’ve recently learned, like a term called air gap. So the environment needs to be air gap. That means, okay, then we end up in the highest skew of almost all our services, networking, firewalls, everything.
And that comes with a quieter cost. You’re talking about TCOs that come into over 10, 20, maybe 30,000 a month. And do you really need that? Is that really a requirement? If you’re a big enterprise presumably, but if you’re a small, medium, small business, I’m not so sure. And there’s other ways to secure things. And even some of the cloud vendors also providing some of the services are touching the middle ground. So not high-end or low-end with the enterprise feature, but just in the middle they could be secured just enough.
What are our predictions for the future of the cloud and DevOps spaces?
Daniel Bryant: I’d like to take a moment now for some final thoughts from each of you and it can be a reflection on where we’ve come from. Maybe looking forward to the future, something you are excited about, maybe something for the listeners you’d like them to take away. So should we go around the room? Shall I start? Matt, I’ll start with you if that’s all right.
Matt Campbell: Yes, of course. I, excellent conversation. Some really, really cool things that are covered off here. For me, the thing that I’m most excited to see and is feeling like I’m harping on the same thing, but anything that we can do in the industry to help reduce how much things we need to think about while we’re working so that teams can focus in on their area and have the biggest impact, I think that’s going to start to drive more innovation. We start to get happier people. People are excited to come to work, they’re focused on a thing. They’re not trying to juggle 10 different things and now figure out what is an SBOM, why are they throwing more acronyms at me?
So those kind of innovations, mergers of AIOps and ChatGPT and to platform engineering and even the sustainability and FinOps stuff like trying to find ways to reduce costs and reduce the amount of things that we’re working with, I think are all good shifts in that direction. So I guess if there’s a kind of a call to action, it’s try to build things that move us in that direction, I think that’s a healthy way for our industry to go.
Daniel Bryant: Love it. Abby, do you want to go next?
Abby Bangser: Sure. I think anytime we have these kinds of conversations about trends, it’s hard not to talk about the fact that a different word for that might be hype cycles. And I think there’s a lot of things that can feel like hype cycles, but the power behind what creates that hype is that there is a nugget of opportunity there. And I think what ends up happening is it’s easy to dismiss the nuance and the opportunity to the fact that there is marketing jargon and hype around it that maybe is overselling something or is particularly overselling the application of it. Not just what it can do, but also how widely applicable it can be.
And I think what’s been really interesting about the trends on this call that I’ve really enjoyed is that a lot of them are not new. We talked about from the first get-go how we’ve been building platforms for decades across the team here. And I think at the same time we are doing new things, we are learning from our experiences, and we are actively seeing evolution to create more value for our users, for our industry. And I think that’s really exciting right now.
Daniel Bryant: Fantastic stuff. Helen, do you want to go next?
Helen Beal: I’m going to be quite quick and just say that I think I’m going to go and have a chat with Bard about ClickOps ’cause that sounds very interesting.
Daniel Bryant: Fantastic. Steef-Jan, over to you.
Steef-Jan Wiggers: Yes, really happy about all the developments that happen in the cloud, the sustainability, the FinOps, but also seeing Opensource being adopted everywhere. I really enthusiastic about the OpenTelemetry. I’m interested seeing that cloud events. I see that adoption overall of what’s happens in that Opensource helps us also standardize and maybe even make our lives a little bit better than have to juggle around with all kinds of standards and things you need to do. I hope that the AI-infused services, like the Copilots and stuff and ChatGPT can make us more productive and try to help us do our jobs better. So I’m excited about that too. So Yes, I said that’s just a lot of things going on. Even enjoy talking to the other guys. We even learned a lot of things today, so that’s also cool.
Daniel Bryant: Amazing. I’ll say thank you so much all of you for taking time out to join me today. We’ll wrap it up there. Thanks a lot.
.
From this page you also have access to our recorded show notes. They all have clickable links that will take you directly to that part of the audio.
Presentation: How to Build a Reliable Kafka Data Processing Pipeline, Focusing on Contention, Uptime and Latency
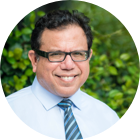
MMS • Lily Mara
Article originally posted on InfoQ. Visit InfoQ
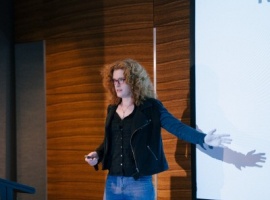
Transcript
Mara: My name is Lily Mara. I’m an engineering manager at a company called OneSignal in San Francisco. We write customer messaging software that helps millions of app developers connect with billions of users. We send around 13 billion push notifications every single day. I have been writing Rust since around 2016, and writing it professionally at OneSignal since 2019. I’m one of the authors of the book, “Refactoring to Rust.” That’s available now in early access at manning.com.
Once Upon a Time
I’m going to talk a little bit about how we took one of our highest throughput endpoints and made it stop bringing the website down every day. We’re going to be talking about how we took a synchronous endpoint that was leaving a ton of load on the database at our HTTP edge layer, and moved that into an asynchronous workload that we could much more control the internal impact of. Since this is a retrospective, we’re going to be jumping back in time a little bit by a couple of years. At this time, we were sending around 8 billion push notifications every day. We had a team of around 10 engineers on what was then called the backend team at OneSignal. We had maybe 25 engineers, total. When you’re operating at that sort of, we considered it largest scale with that small team, you have to make a lot of simplifying assumptions about your problem space. This is going to come into play later on as we get into the architecture of the system. It’s important to remember that a lot of the tradeoffs that we made, we made because we had specific performance goals that we were trying to serve. We had other things that we didn’t quite care so much about.
The problem that we’re trying to solve is backing the API that allows our customers to update the data that’s associated with their subscriptions. At OneSignal at the time, our data model was centered on subscriptions, which we defined as a single installation of an application on a single device. One human person might have many OneSignal subscriptions associated with them for different applications and different devices. Each subscription can have a number of key value properties associated with them. Customers might send us HTTP requests like this one that allow them to update those properties. For example, you can see, in this instance, someone is sending us an HTTP PUT request, so that they can update the First Name and Last Name fields to Jon Smith. This request also gives us the subscription ID, which correlates with the row ID in the database, as well as the app ID, which is more or less a customer dataset ID. You can see in this instance we have one human person and she has a mobile device. She has a web browser that’s on a laptop or desktop. The mobile device has two subscriptions that are associated with it, it has an SMS subscription and it has a mobile push subscription for a mobile app. The web browser also has a web push subscription that are associated with it. There are multiple subscriptions across multiple devices, but they all correspond with the same human person. At this time, we didn’t really have a data model that allowed for the unification of these subscription records to one human person, like we do now. You can see each of these subscriptions has associated with it in the database, an account type property. This might be something that a customer uses to do substitution at the time they deliver a notification. They might do some templating that allows them to send a slightly different message body to people with different account types. They can also just do segmentation that allowed, send this message only to people who have VIP account levels. Send this message to people who have user account types. This system is fairly flexible. Our customers do like it. Somebody has to maintain the infrastructure that allows you to store arbitrary amounts of data.
The original format of this was an HTTP API that performed synchronous Postgres writes. Our customer makes an HTTP request, either from their own servers or from our SDKs on their users’ devices. We block the HTTP request in our web servers, and the Postgres write completes, and we send a 200 back to the device. This works for a time. It certainly works at 100 users. It probably works at 10,000 users. Maybe it even works at several million users. Once we started getting into the billion range, we have billions of users, we’re sending billions of notifications a day, and a notification send was, generally speaking, fairly well correlated with these property update requests. Because after a notification sends, maybe a user takes some action, and a customer wants to update the subscriptions properties as a result of this. Eventually, we grow to such a scale that we get hammered by traffic incessantly, generally speaking, at the hour and half hour marks, because people love to schedule their messages to go out on the hour, on the half hour. Nobody is scheduling their push notifications to be delivered at 2:12 in the morning. People are scheduling their messages to be delivered at 9:00 in the morning, because it’s just easier. It makes more sense as a marketer, and it comes more naturally. Everybody is doing that, meaning at that hour mark, we are getting tons of traffic at this endpoint. It would frequently lead to issues with Postgres, as it really couldn’t keep up with the volume of writes that we’re sending it. There’s a huge enormous bottleneck here. Very frequently, this would just cause our entire HTTP layer to go down, because all of our Postgres connections would just be completely saturated, running these synchronous HTTP writes.
The Queuing Layer
What did we do? We took this synchronous system, and we made it asynchronous by introducing a layer of queuing. What is a queue? Queues are a very fundamental data structure that are talked about in computer science classes. They work in a very similar way to the queue you would wait in at the café. Items are enqueued at one side. They sit on the queue for a period of time. Then, in the end, items are dequeued from the other side, and they’re handed off to some processor that does something with them. We can control the rate at which messages are dequeued. We can slow down our processor, or we could try to speed up our processor, so that messages sit in the queue for as little time as possible. Or we could slow our processor down a little bit, so that we don’t overwhelm the processor in the end, because remember we’re running Postgres writes here. If there’s a huge spike in the number of requests that come in, really all that happens is more messages sit in the queue, and our Postgres servers don’t get overloaded because they’re processing a more or less constant rate of messages per second, or at least a rate of messages that has some known upper bound on it. We are introducing an additional metric here. In our previous world, really the only metrics that we had were maybe the number of requests that we’re processing at one time, and the CPU memory limits on our web workers and on our Postgres workers. Now we have this new thing that we need to measure. It’s going to turn out to be really important for us to be measuring this. It’s the number of messages that are sitting in that queue waiting to be processed. This is the metric that we call the lag of our queue. We have four messages right now that are sitting in this particular queue. We have a red, blue, orange, and green message that are sitting in the queue waiting to be processed. We have a purple message that has been removed from the queue so it’s no longer waiting. We would say that we have four messages in lag for this queue that’s represented on this slide.
Apache Kafka
A queue, like I said earlier, is just an abstract data structure. In the real world, we don’t use abstract data structures, we use concrete implementations of data structures. In our case, we use Apache Kafka. Apache Kafka is a very nice high performance message log system. It has a lot of neat features that you don’t get with just the basic abstract queue. It has a ton of use cases beyond what we’re going to discuss in this talk. We’re going to talk about how we use it to back our Postgres writes. How does Kafka work? How are these things structured? With a basic queue that you might import from your programming languages’ standard library, you might have a list of messages that sits in memory. You might remove things from that list of messages as they’re being processed as you dequeue them. That’s not what we get in Apache Kafka. We have a number of data structures that are represented on this slide, so let’s talk about them one at a time. The first thing that’s on here is the producer. This is the thing that enqueues messages to Apache Kafka. In our case, this was our HTTP server that’s taking messages from the HTTP API, and it’s adding them to our Kafka queue by calling the Send method on Kafka. It’s adding them to Kafka in something called a topic. This is a logical grouping of messages, where messages have the same purpose. Each message has an auto-incrementing numerical ID called an offset, that starts at 0 and it gets bigger over time.
The thing that pulls messages off of Kafka, that dequeues messages is called a consumer. In this case, we have a single consumer, it’s called A, and it has pulled the first message off of the log of the queue. The thing that’s interesting here is, you’ll notice that that message is still on the queue. It hasn’t been removed, it’s still on the topic. The thing that’s different about Kafka from the typical queue implementation is that the messages don’t actually get deleted from Kafka’s data structures, they stay there, and the thing that changes is just the pointer inside the topic to which message has been read. This is going to give us a little bit of flexibility in terms of how this is implemented. It’s important to note that, really, what we’re changing is we’re just moving this pointer around in there. You notice that our consumer A has read message 0. It processes message 0. Then once it’s done, it sends Kafka a message called a commit. That advances this pointer, so it moves the pointer from 0 to 1. The next time we ask Kafka for a message, it’s going to give us the next message in the log back.
One of the nice flexible things that you can do with Kafka because it has this moving pointer thing is you can actually have multiple consumers, which read from the same exact topic, and every consumer is actually going to be able to see every single message that’s on the topic. This is beneficial if you wanted to have a single Kafka topic that has just a ton of data on it that might be useful in broad contexts, and maybe one application reads 90% of the data, and another application reads 30% of the data, obviously, those add up to more than 100%. There could be overlaps. Because each consumer is going to see every message that’s on there, you get a lot of flexibility in how you want to utilize that data. In this instance, we can see that there are two consumers that are both reading from the same topic, and they’re at different points in the topic. As your user base grows, you probably want to be able to process more than one message at a time. Both of these consumers are just looking at a single message, they’re looking at different messages. They are both looking at single messages and they are both going to want to process every message that’s on that queue.
Kafka Partition
What do we do about this? It turns out that Kafka has a feature that’s built in for scaling things horizontally, that is called a partition. I know that I presented each topic as an independent queue of messages, previously. It turns out that within a topic, there are numbered partitions. These are the actual logs of messages that have independent numbering schemes for offsets. They can be consumed independently by either single instances of a consumer or, indeed, multiple instances of a consumer. In this instance, on here we have a topic that has two partitions. We could have two instances of the consumer that were running in two different Kubernetes pods, or on two physical servers that were separated and had dedicated resources, or they could simply be running in two threads of the same consumer instance.
We talked previously about lag. Notice that these two partitions, they have independent lag from each other, because they are two different queues with two different positions in the queue. Partition 1 actually doesn’t have any lag, because it’s red up to the end of the queue. Partition 0 has 3 messages in lag, because there are still message 2, 3, and 4 that are sitting on the queue and waiting to be processed by the consumer. You might say that the topic as a whole has three messages in lag, because it’s just those three messages from partition 0. You could subdivide that down into the two partitions. Indeed, in most cases, if you have a resource that’s sitting back there behind just one of the partitions, you will very often find that one of your partitions, or one grouping of your partitions starts to lag. It’s, generally speaking, not smeared across the whole range.
We have our multiple partitions, but when we have a message that we want to add to our Kafka topic, what do we do with it? Which of those partitions does it go to? There’s a couple of different ways that we can distribute messages to our different partitions inside the Kafka topic. The first one is fairly intuitive.
If we don’t tell Kafka which partition we want a message to go to, we just get round-robin partitioning. In this instance, there are three topics. Each time we add a message to our Kafka topic, Kafka is just going to pick one of those partitions, and just assign the message to it. This might be a perfectly acceptable partitioning strategy for many use cases. In our case, this was not really going to work for us. Because, remember, we were doing Postgres writes. Each of these messages was a subscription update that had a customer dataset ID and a row ID on it. We have a cluster of Postgres servers that are partitioned by customer dataset ID. We don’t have one Postgres server per customer, but we do have a Postgres server that is responsible for a range of customer dataset IDs. This meant at the time that we were adding our message to Kafka, we actually knew at that time which Postgres server the message was going to be written to eventually. At the time, we generated our message and wrote it into Kafka, we would actually assign it explicitly to a partition that was tied to that particular Postgres server. Now, again, this, as is shown on here, gives us some amount of separation between the Postgres servers. You may notice that within each Postgres server, there’s really not any concurrency, there’s a single partition that’s responsible for each Postgres server. We are not going to have the ability to do concurrent writes on our Postgres server, we’re going to be fairly slow. The way that we would get around this is by having multiple Kafka partitions that would be responsible for each Postgres server. In this case, we have four partitions, and two Postgres servers. Each Postgres server has two Kafka partitions that are associated with it. We can process those two partitions concurrently, meaning we can perform two concurrent writes to our Postgres servers.
Issues
This strategy will get you fairly far, but we did run into some issues with it. This system isn’t super flexible. What happens in a world where we want to change that concurrency number? There’s a lot of reasons why we might want to do this. Maybe we’re able to get some hardware updates, and we have better CPUs that are available for our Postgres servers, and so we want to increase the concurrency of our writes. Or maybe we decide that we actually want to prioritize reads, and we’re ok with slightly higher message latency, so we want to reduce the concurrency of our Postgres writes. In this instance, we would have to do what’s called repartitioning our Kafka topic. This entails some rather interesting gymnastics. If we had a topic that had four partitions, and we wanted to go to a topic that had six partitions, so we wanted to be able to change our write concurrency from 2 to 3, we would have to create a new empty topic that had 6 partitions, and we would have to rewrite all of the data from the old topic to the new topic. We would have to manage doing that at the same time as we were shifting new writes onto the new topic. If you want to maintain message ordering while doing something like this, it’s really quite a juggling act. This is something that we wanted to engineer our way out of having to do every time that we wanted to change our Postgres concurrency level.
What do we do instead? How do we get around this kind of issue with the strategy? The solution that we developed here was to concurrently process Kafka messages in memory within each Kafka partition. This is something that we called subpartition processing. This is not something that, generally speaking, folks like Confluent consultants will recommend that you do, but we have found it to be very useful and extremely effective for meeting our particular needs. What does this look like? Inside of our consumer instance, as mentioned, we have a number of partitions that are sitting there in memory and processing concurrently. Within each one of those partitions, Kafka has a number of messages that it knows about. Inside of our consumer instance, inside the partition level, we have a number of workers, these are more or less threads that are sitting there, and they’re able to process messages concurrently within the partition. Instead of asking Kafka for the next message, we would ask it for the next four messages. We would process those concurrently, send our writes to Postgres concurrently. If it were that easy, this would be an extremely boring and short talk. Once you start doing this, things start to get a lot more complicated. We’ll run into a lot of issues along the way. The first of which, that becomes extremely obvious when you try and achieve this, is, it becomes a lot more difficult to manage commits. Let’s talk about why that is.
If you’re processing messages concurrently that are linearly ordered in Kafka, eventually you’re going to get to a point where you process messages out of order. Let’s imagine that we have message 0, 1, 2, and 3 that are all sitting there in memory, they’re being processed concurrently, and we just so happen to finish processing message 0, and message 3 at the same time, while 1 and 2 are still processing. What do we do in that situation? If we follow these standard Kafka rules, what we would do is we would send Kafka a commit for message 0, telling Kafka, I finished the processing on message with offset 0, and you can mark it as done. If I ask you for more messages in the future, you don’t have to give me message 0 because it’s done, it’s over. Once you’ve done this, remember we’ve also finished processing message 3 concurrently, so we would also send Kafka a commit for the message with offset 3. Kafka says, “Ok, that’s great. Message 3 is finished, so I will never show you message 3, or any of the messages before message 3 again.” Because it turns out that Kafka does not store the completion of every single message, it only stores the last message, or it only stores the greatest message that has been committed, because it assumes that you read the queue from the beginning to the end, and you don’t skip any messages, like we’re doing. This is a problem. This isn’t going to evict message 1 and 2 from the memory of our worker, but it is going to prevent us from replaying those messages. If we get into a situation like, say, our consumer instance restarts, or crashes, or something like that, while message 1 and 2 are still being processed. If the consumer instance comes back up and it asks Kafka for the messages again, it’s never going to get message 1 and 2 replayed, because Kafka thinks that they’ve been committed, they’ve finished processing so it doesn’t need to show them again. For our purposes, we consider this to be an unacceptable data integrity solution. We wanted to make sure that every update was being processed by the database.
What is the solution here? How do we get out of this problem? What do we do with this message 3 given that we can’t send it to Kafka? The solution that we came up with was to create a data structure in the memory of our Kafka Consumer, which we called a commit buffer. Instead of sending messages to Kafka when they’re committed, we write them into this in memory commit buffer. It has one slot for all of the messages that are in memory. It just has: is completed, is not completed. Right now, it has two completed messages in it, 0 and 3. You can see it has empty slots there for 1 and 2. We have the messages written to the buffer. Then what we’re going to do is we’re going to start at the beginning, and scan for a contiguous block of completed messages. If we do that, we can see that there’s a single completed message at the beginning, message 0. We can take that completed message, and we can send it as a commit to Kafka. Then after that, we need to do nothing for a little bit. Because if we look at the beginning, there’s not a single committed message that’s at the beginning of that commit buffer, so it’s not safe for us to send Kafka any more commits at this point. We need to sit around, and we need to wait for messages 1 and 2 to be completed by our Kafka Consumer, at which point, we can scan again from the beginning for the longest block of contiguous messages. We find that 1, 2 and 3 are completed, and we can send Kafka a commit, or the message with offset 3, and it will know that 3 and everything before it have been completed, and I don’t ever need to replay them.
Model Concession
There is a concession of this model. It’s a pretty important one that you definitely need to design around. This model uses something called at-least-once delivery. There’s a couple of different models of Kafka Consumers and generally asynchronous processors like this. This means that messages are going to be replayed. It means you will definitely get to a point where a Kafka Consumer reads the same exact message more than one time. It will happen. You need to design your system to account for this possibility. There’s a lot of different ways you can do this. You might have a Redis server that sits there and tracks an idempotency key at the time that your message is completed. We actually took the Kafka offset from the message and we wrote it into Postgres, and we use that as a comparison at the time we were doing our write to make sure we didn’t update any rows more than once, if we’ve already applied that update. This is something that you have to design into your system, or you will get inconsistent data. Imagine you’re ticking a counter and you apply the same counter tick more than once, you’re going to get bad data, so you’ve got to design around it. There are other models of delivery. There’s at-most-once delivery, which is where you know you’re not going to see something twice, they might not show up once. There’s also exactly-once delivery, which is a very large bucket that you pour money into, and at the end, it doesn’t work.
Review
Let’s review all the pieces that we’ve talked about here. Some of this is standard Kafka, and some of it is specific to what we were trying to do at OneSignal. We have a Kafka topic that contains a number of partitions, which are independent queues of messages. Each message has an auto-incrementing numerical offset, that’s sort of like its ID. We have producers that enqueue messages into Kafka, and we have consumers which dequeue messages out of Kafka. We can control the concurrency of our consumers via Kafka partitioning, the number of partitions we create, and this subpartitioning thing that is very specific to what we’re doing at OneSignal. The really nice thing about this subpartitioning scheme is since our workers are really just threads in the memory of our consumer, we can control the number of those extremely easily. Right now they’re essentially numbers in a configuration file of our Kafka Consumer. We can change those by redeploying the Kafka Consumer. In theory, we could design a way to change those while a process was running without having any downtime whatsoever. We don’t do that, because we don’t feel that that level of liveliness is necessary. We can be very flexible up to the point that we think is useful.
Postgres Writes
It’s also very important to remember that these Kafka Consumers are performing Postgres writes. Let’s talk about these Postgres writes. Let’s talk about what they’re actually doing, because the fact that we’re doing Postgres writes is actually quite important and quite relevant, and it’s going to have a lot of impact on how we manage concurrency in this system. We’re doing Postgres writes. Postgres has a bunch of rows in it. All these Kafka updates are fundamentally saying, take this Postgres row and update some property on it. Let’s imagine for a moment that we receive two Kafka updates at the same time, or around the same time, and we process those two concurrently. They’re both trying to update the same exact row in Postgres, one of them is setting a property called a to 10, and another one is setting the property a to 20. Imagine we got the one setting a to 10 first, and the one setting a to 20 second. I don’t know why a customer might send us two conflicting property updates in quick succession like this. It’s really not my concern. The thing that is my concern is trying to make sure that we replay our customer’s data, and we apply our customer’s data in exactly the order that they sent it to us. If we’re processing these two things concurrently, we might just so happen to process the message setting a to 20 first, meaning in the database we get 20. Then, at a later time, we finish the message setting a to 10, meaning we get 10 in the database. That might be confusing from a customer’s perspective, because they sent us set it to 10, set it to 20, so it would seem like the property should be set to 20. This is not an acceptable state of the world for us. We need to design around this problem.
What are we trying to do? We need to maximize concurrency. We want to make sure that we are saturating our Postgres connections, and getting as much out of those as we possibly can. We need to minimize contention. If there’s a single row that’s getting a bunch of updates sent to it, we can never process those updates concurrently, because they might complete out of order, and that might lead to an inconsistent state of the customer’s data. We don’t want to do that. What do we do? We created some more queues, because it’s queues all the way down. We love queues. Recall that we had a number of processors for messages in memory, those subpartition processors. Instead of having them just grab messages randomly off the main partition queue, what we did is we took the subscription ID, which is the row ID, and we hash that. We merge load it by the number of workers that we had in memory, and then we assigned it to a queue that was tied one to one to those processors. You can see, in this case, we have the real queue that represents every message that’s in the Kafka partition.
We have a blue queue that represents some portion of the messages that happens to hash, and the red queue that happens to hash to the same queue. Because these hashes are based on the subscription ID, which is the row ID, we know that updates, which are bound for the same row, will never complete concurrently, because they will be in the same queue which has only a single processor associated with it. However, since these row IDs we assume are assigned basically fairly, these queues are going to have a bunch of messages in them, and we’ll be able to process lots of things concurrently.
Unifying View of the Kafka Consumer
There’s been a lot of data structures that we’ve talked about, let’s try to put them all together into one grand unifying view of what our Kafka Consumers look like. At the far end, we have a producer that enqueues messages into our Kafka topic, and it tells Kafka, please send this to this particular partition, because it’s going to wind up in this Postgres database. That Kafka topic has its messages dequeued by a consumer, which is consuming multiple partitions of messages concurrently. Within those partitions, we take the row ID, we hash it, and we use that to assign a message to a particular subpartition queue, which is tied to a particular subpartition processor. Once messages are finished being processed by those subpartition processors, we add them to a commit buffer, and eventually commit them to Kafka. These processors are all sending a bunch of writes to some Postgres servers.
This got us pretty far, but at a certain point, we started running into some more issues, because there’s always more issues than you think there’s going to be. It’s always so simple in theory. It wasn’t this simple, I thought it’s so beautiful. We ran into some more issues. We’re adding additional layers of in memory queuing, so we’re asking Kafka to give us lots of messages. When there’s not a lot of Kafka lag, this works just fine, because you can ask Kafka for all the messages, and there’s not very many of them. At a certain point, you’re going to lag your Kafka queue, because you’re going to have some operational issues that cause your messages to stop processing. There’s going to be a bunch of messages that build up and lag. This means that your consumers are going to ask Kafka for too many messages, and you’re going to overload the memory of your consumer processes, which is going to make them fall over, which means they’re not going to process messages, which means the lag is going to get bigger, which means your problem is not going to go away on its own. How did we solve this one? It was fairly simple. We added a cap on the number of messages that each consumer instance was allowed to hold in memory. Once we got to a certain number of messages in memory, it just stopped asking Kafka for messages. It would check back at a later time to see if some messages had cleared out, and some memory space had opened up. Once we did this, to our shock and awe, everything was fine for an amount of time. At the end of that amount of time, things were no longer fine. This not fineness had the following characteristics. We started getting paged intermittently on high amounts of lag on our Kafka topic. There was a very clear demarcation line between the fine and the not fine metrics.
Everything was just ticking along at a very low baseline level, and then all of a sudden, out of nowhere, line goes up in a bad way. We delved into the stats on our consumer instances with some expectations that were very soundly busted. We expected that our consumer instances would have had their CPU jump way up, because there’s a bunch more messages to process. I’m working really hard to process those messages. We expected CPU to go way up, and we expected the number of idle connections to go way down. In reality, we saw the exact opposite happen, we saw the CPU usage go way down and the number of idle connections jump way up, almost to the maximum. This confused us to no end. We didn’t really have a lot more observability options in this. At this time, really, we were just dealing with metrics. We had some unstructured logs that were sitting on the boxes, like the boxes, not in the Kubernetes config store. At this time, I insisted on getting us to a point where we had centralized logging. It was something that I wanted when I first joined the company. It was not prioritized at that time, but I used this as a jumping off point to be like, “No, we need centralized logging. This is the point.” We added some centralized logging, so all of our workers started sending data to a central location. It wasn’t a lot of data. It was relatively simple. We sent in the app ID, which was the customer dataset ID. We sent in the subscription ID, which is the row ID, the SQL statement that was being executed, and the consumer instance. This allowed us to do some stuff like group our logs by which customer dataset ID was sending us the most updates. What we found was surprising. We found that almost all of the updates that were coming through were coming from a single customer, which we’re going to call clothes.ly. It was something on the order of, if clothes.ly was doing maybe 500 updates per second, our next largest customer was doing 30. Every other customer on that same pod was doing maybe a combined rate of 100. It was really dominating the logs. Since we had it, we also grouped by the subscription ID, the row ID. We actually found that there was a single row ID that was dominating our logs. A single row ID was getting all the updates.
What was happening here? It’s important to remember that it wasn’t just the case that we were getting a lot of updates for a single row. It was also the case that we stopped doing everything else. Remember, number of idle connections jumped way up, CPU jumped way down. There was really nothing going on except the processing of these single updates, the single subscription updates. What was happening? We wanted to see what was in those updates. It was a bunch of updates to single fields at a time. Basically, every single one of those updates was completely incompatible. There were constant updates happening to the location field, so it was jumping from San Francisco, to New York, to Tokyo, back to San Francisco, to Boise. It was just all over the place. It made really no sense to us. We looked in Postgres and we started just refreshing this particular subscription. Location is constantly changing, key-value pairs are constantly changing, but there were a couple fields that were staying very consistent. The device type was always email. That was never changing. The identifier, which is what we use to message a particular subscription, it might be an email, if it’s an email subscription, or it might be a push token, or an iOS or an Android device. It just depends on the subscription type. The identifier was never changing. It was always set to admin@clothes.ly.
This raised alarm bells for us. Let’s talk about why. OneSignal started as a push notification company. We started branching out from that a couple years ago, we started moving into omnichannel messaging that let our customers reach their user base across multiple platforms. The first way that we did that was by adding a method to our SDKs called setEmail. What this does, as implied, if you have a push SDK that has a subscription in it, and you call the setEmail method, it will create a new subscription that has that email address as its delivery method. It will set the parent of that push record to the new email record. It will store that email record in the SDK. Anytime you update a property from that SDK, it will duplicate the update to that email record. We use this parent property to check and see how many records were linked to this admin@clothes.ly record. Clothes.ly had around 5 million users total, and about 4.8 million of those users had the same exact parent record. Almost every single one of those users had had setEmail admin@clothes.ly called in its SDK. Every time one of those 4.8 million users had any of its properties updated, we had an identical update mirrored to this one central record. Our Kafka queue was subtly weighted with too many updates for this one subscription record.
Why is this a problem? Why is this such a big deal, if there’s like a little bit more, there’s like a just thumb on the scale? Why is this so bad? Remember that our subpartition queues, these are not totally independent queues. These are views onto a single real queue, the Kafka partition. In a normal situation, where there’s a basically fair distribution of messages, we have three queues here. When the green processor finishes processing its message, it can grab the next green message off queue 2, and the next one, and the messages are spaced out about the same. There’s always going to be more messages to run. If we put a thumb on the scale, and we weight this a little bit unfairly, when the green queue finishes processing this message, that’s the only message that’s in memory right now. Remember there’s a limit on how many messages that we can pull into memory. There’s a real Kafka partition that’s representing this queue of messages here, so we can’t advance the queue to look for more green messages until we process some of these red messages. What’s going to happen is the green queue, and then later on the blue queue, queue 1, those are going to process their messages, and there’s not going to be any work for them to do. Unfortunately, because of the way that Postgres works, because we’re sending lots of updates to one row, Postgres is actually going to get slower because Postgres is not super great at having one row get hammered with updates. This is a worst-case situation for us. In reality, it was slightly worse than I’m discussing here, because I’m zoomed in to the partition level, but because our limit of messages was at the consumer level, at the application level, it was actually affecting every single partition that was assigned to a single consumer instance. It was smeared across a number of partitions, not just the one partition that had clothes.ly’s app on it.
What did we do in this situation? The first thing that we did that very quickly resolved this particular problem was we skipped updates that were going to the clothes.ly admin record. We fixed the limiting, so it was zoomed in to the partition level, so you wouldn’t be able to blow up the whole consumer level stats by having one partition go haywire like this. We also put limits in place that stopped customers from linking so many records together using our SDKs, because that was a fairly obvious misuse case. Customer saw setEmail, and they assumed that they were supposed to set it to their email of the account owner.
Lessons Learned
What did we learn during this talk? What did we learn as a result of this very inventive customer? We learned how we can take intensive API write workloads, and we can shift them from the API layer down into asynchronous workers to reduce the operational burden of those writes. We learned how we could do subpartition queuing to increase the currency of our Kafka consumers in a configurable and flexible way. We also learned some of the struggles that you might face while you’re trying to do subpartition queuing. We learned how centralized observability was valuable to us in tracking down this particular issue. We also learned that no matter how creative your engineering and design and product teams may be, your customers are almost certainly more creative.
See more presentations with transcripts
Java News Roundup: GlassFish 8.0-M1, 2023 Highlights from Spring, BellSoft and WildFly
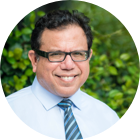
MMS • Michael Redlich
Article originally posted on InfoQ. Visit InfoQ
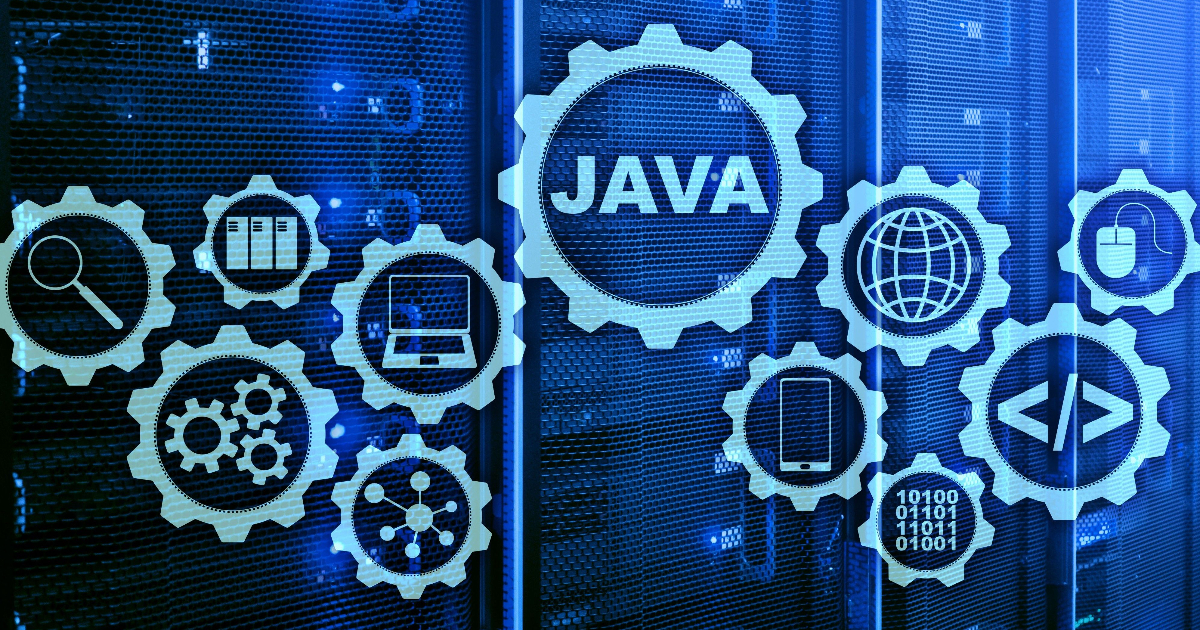
It was very quiet for the week of December 25th, 2023, but InfoQ found a few news items of interest that include: Eclipse GlassFish 8.0.0-M1, Apache Camel 3.22.0, Gradle 8.6-RC1, an updated draft specification for JEP 455, and retrospectives into the 2023 highlights from Spring, BellSoft and WildFly.
OpenJDK
Aggelos Biboudis, principal member of technical staff at Oracle, has published an updated draft specification for JEP 455, Primitive types in Patterns, instanceof, and switch (Preview). This JEP, under the auspices of Project Amber and currently in Candidate status, proposes to enhance pattern matching by allowing primitive type patterns in all pattern contexts, and extend instanceof
and switch
to work with all primitive types.
JDK 23
There was no activity in the JDK 23 early-access builds this past week. Build 3 remains the latest update. Further details on this release may be found in the release notes.
JDK 22
Similarly, there was also no activity in the JDK 22 early-access builds. The latest update remains at Build 29. More details on this build may be found in the release notes.
For JDK 23 and JDK 22, developers are encouraged to report bugs via the Java Bug Database.
Eclipse GlassFish
The first milestone release of Eclipse GlassFish 8.0.0 delivers support for Jakarta EE 11-M1 with full implementations of the Jakarta Security 4.0.0-M1 and Jakarta Faces 4.1.0-M1 specifications, and a partial implementation of the Jakarta Servlet 6.1.0-M1 specification. JDK 17 is the required minimal version at this time, but may be updated to JDK 21 in the next milestone release. There is support for JDK 21, and the final version of GlassFish 8 is targeted to certify on JDK 21 for Jakarta EE 11. More details on this release may be found in the release notes.
GraalVM
Oracle has announced that Oracle GraalVM is now available as a Paketo buildpack. In collaboration with the Paketo team, Oracle GraalVM has been integrated into the Oracle buildpack. This allows developers to add both the Native Image and Oracle buildpacks to a buildpack configuration file for executing the application.
Apache Software Foundation
The release of Apache Camel 3.22.0 ships with bug fixes, dependency upgrade and new features/improvements such as: support for start and end dates in the Camel Quartz component; the ability to use the old Micrometer meter names or follow the new Micrometer naming conventions; and provide a tracing strategy to trace each processor for Camel OpenTelemetry as part of the migration process from Camel OpenTracing. More details on this release may be found in the release notes.
Gradle
The first release candidate of Gradle 8.6 provides: support for custom encryption keys in the configuration cache via the GRADLE_ENCRYPTION_KEY
environment variable; improvements in error and warning reporting; improvements in the Build Init Plugin to support various types of projects; and enhanced build authoring for plugin authors and build engineers to develop custom build logic. More details on this release may be found in the release notes.
Spring Framework
Josh Long, Spring developer advocate at Broadcom, published This Year in Spring – 2023, a retrospective into the 2023 highlights. These include: support for Artificial Intelligence with the introduction of the Spring AI project; continued GraalVM native image support in Spring Boot 3.0+; support for virtual threads and Project Loom; support for Coordinated Restore at Checkpoint (CRaC) with the release of Spring Boot 3.2; support for Docker-driven development where Spring Boot can derive connectivity information from either a local Docker Compose description file or Testcontainers; and the release of Spring Modulith 1.0 that provides production-readiness, IDE support and improved testability.
Long also published the latest edition of his A Bootiful Podcast with Joris Kuipers, CTO at Trifork and former senior consultant at VMware. Recorded live in October 2023 from the SpringOne tour in Amsterdam, Long spoke to Kuipers about topics such as his career, the Spring ecosystem and GraalVM. They also answered questions via chat from attendees.
BellSoft
Alex Belokrylov, CEO at BellSoft, provided a retrospective into BellSoft’s 2023 highlights, noting:
This year was filled with overcoming challenges, seizing opportunities, taking part in fruitful engagements, and participating in unforgettable events.
Technical highlights included: the introduction of Alpaquita Containers; launch of the Performance Edition line with the release of Liberica JDK 11 Performance Edition; introduction of Liberica JDK with CRaC; and ongoing commitment to OpenJDK and GraalVM that includes four quarterly releases with security patches and critical fixes.
Highlights of BellSoft’s engagement with the Java community included: 30 presentations at 28 global events, such as JNation and Devoxx, by Dmitry Chuyko, performance architect at Bellsoft; and participation in the 25th anniversary celebration of the Java Community Process in New York City in September 2023.
WildFly
Brian Stansberry, senior principal software engineer at Red Hat, provided an end-of-year summary on Wildfly and the contributions by the Java community. Highlights included: three major version releases of WildFly 28, 29 and 30; new extensions for the MicroProfile Telemetry and MicroProfile Long-Running Actions specifications; implementations of most of the MicroProfile 6.0 specification, with updates to MicroProfile 6.1 in the upcoming release of WildFly 31; support for JDK 21; more than 2000 issues and enhancements resolved in the WildFly main code; and a license change of the WildFly code base to Apache License 2.0.
There was also a significant amount of work on improving documentation and tooling related to getting started with WildFly. Stansberry also announced that WildFly 31 will be released in January 2024.
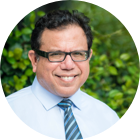
MMS • RSS
Posted on nosqlgooglealerts. Visit nosqlgooglealerts
The report gives an abstract and quantitative examination of the Global Nosql .The examination relies upon the division of the Nosql which focuses on monetary and non-money related factors impacting the Nosql improvement. The report joins a genuine scene which concludes the market position in the focal parts, including new help offered, thing dispatches, business associations, combinations and acquisitions in the past five years.
Companies operating in the Nosql
Microsoft SQL Server, MySQL, MongoDB, PostgreSQL, Oracle Database, MongoLab, MarkLogic, Couchbase, CloudDB, DynamoDB, Basho Technologies, Aerospike, IBM, Neo, Hypertable, Cisco, Objectivity
The report highlights of emerging examples, with principal drivers, risks, and likely entryways In the Nosql. The crucial creators across the world in the worldwide Nosql are organized in the report. Considering such things introduced in the Nosql, the around the world Nosql is ordered Into different segments. The part overpowered the Nosql and held the greatest piece of around the world Nosql in the year 2020, and continues to govern the market in 2021 are positive in the report.
We Have Recent Updates of Nosql in Sample Copy@ https://www.mraccuracyreports.com/report-sample/346435
Considering use, the around the world Nosql is ordered into different application sections. The application section that is depended upon to drive the slice of the pie of the Nosql in the next few years are highlighted and thought about in the report. The indispensable components of advancement in this application segment are explained in the report. The areas that addressed the greatest pay part of around the world Nosql in 2022 are considered in the report. Additionally are depended upon to continue with the edge over its opponents in the regarded time span are considered in the report. The grounded establishment and innumerable Vessel Monitoring System Software associations in these regions are organized in the report.
By the product type, the market is primarily split into:
Key-Value Store, Document Databases, Column Based Stores, Graph Database.
By the end-users/application, this report covers the following segments:
Data Storage, Metadata Store, Cache Memory, Distributed Data Depository, e-Commerce, Mobile Apps, Web Applications, Data Analytics, Social Networking
Elements of the Report:
• New game plans and commitments that market players can imagine are in like manner discussed in the report.
• The possible entryways for business trailblazers and effect of the Coronavirus pandemic are associated with the around the world Nosql.
• New things and organizations that are thriving in this speedy progressing around the world Nosql’s monetary environment are discussed in the report.
• The report discusses the how certain advancement things, market frameworks, or game plans could assist with showcasing players.
• The pay open entryways and the growing new game plans are discussed in the report.
• The unquestionable characteristics of each part and market open entryways are explained in the report.
• The powers during the pandemic are relied upon to accelerate the hypothesis pace in the around the world Nosql are point by point in the report.
• The report gives proposition on the way forward in the around the world Nosql.
Table of Contents
1.1 Study Scope
1.2 Key Market Segments
1.3 Players Covered: Ranking by Vessel Monitoring System Software Revenue
1.4 Market Analysis by Type
1.4.1 Nosql Size Growth Rate by Type: 2020 VS 2028
1.5 Market by Application
1.5.1 Nosql Share by Application: 2020 VS 2028
1.6 Study Objectives
1.7 Years Considered
1.8 Continue…
Inquiry for Buying Report @ https://www.mraccuracyreports.com/checkout/346435
This report tends to a couple of key requests:
• What is the by and large expected advancement of around the world Nosql after Coronavirus vaccination or treatment is found?
• What are the new essential methodologies that can be executed post-pandemic to remain merciless, agile, client driven, and helpful in the around the world Nosql?
• Which unequivocal regions are depended upon to drive improvement in the around the world Nosql?
• What are key government approaches and interventions did by driving around the world Nosql countries to help with advancing gathering or improvement of Vessel Monitoring System Software.
If you have any special requirements, please contact our sales professional (sales@mraccuracyreports.com), No additional cost will be required to pay for limited additional research. we are going to make sure you get the report that works for your desires
Thank you for taking the time to read our article…!!
ABOUT US:
Mr Accuracy Reports is an ESOMAR-certified business consulting & market research firm, a member of the Greater New York Chamber of Commerce and is headquartered in Canada. A recipient of Clutch Leaders Award 2022 on account high client score (4.9/5), we have been collaborating with global enterprises in their business transformation journey and helping them deliver on their business ambitions. 90% of the largest Forbes 1000 enterprises are our clients. We serve global clients across all leading & niche market segments across all major industries.
Mr Accuracy Reports is a global front-runner in the research industry, offering customers contextual and data-driven research services. Customers are supported in creating business plans and attaining long-term success in their respective marketplaces by the organization. The industry provides consulting services, Mr Accuracy Reports research studies, and customized research reports.
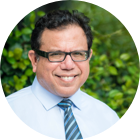
MMS • RSS
Posted on mongodb google news. Visit mongodb google news
Oak Thistle LLC acquired a new stake in shares of MongoDB, Inc. (NASDAQ:MDB – Free Report) in the third quarter, according to its most recent filing with the Securities and Exchange Commission. The fund acquired 748 shares of the company’s stock, valued at approximately $259,000.
Other hedge funds also recently made changes to their positions in the company. Atika Capital Management LLC raised its holdings in MongoDB by 5.3% during the 2nd quarter. Atika Capital Management LLC now owns 31,500 shares of the company’s stock valued at $12,946,000 after buying an additional 1,575 shares during the period. Raymond James & Associates increased its stake in MongoDB by 227.2% in the second quarter. Raymond James & Associates now owns 39,361 shares of the company’s stock worth $16,177,000 after purchasing an additional 27,331 shares during the period. Mirae Asset Global Investments Co. Ltd. purchased a new stake in MongoDB in the second quarter worth $6,704,000. American Trust purchased a new position in MongoDB during the 2nd quarter valued at about $349,000. Finally, Empower Advisory Group LLC bought a new stake in shares of MongoDB in the 1st quarter valued at about $7,302,000. 88.89% of the stock is currently owned by institutional investors.
MongoDB Trading Down 2.0 %
MDB opened at $408.85 on Monday. The stock has a market capitalization of $29.51 billion, a PE ratio of -154.87 and a beta of 1.19. The company has a debt-to-equity ratio of 1.18, a quick ratio of 4.74 and a current ratio of 4.74. MongoDB, Inc. has a one year low of $164.59 and a one year high of $442.84. The business’s 50 day moving average price is $388.24 and its 200 day moving average price is $380.91.
MongoDB (NASDAQ:MDB – Get Free Report) last announced its earnings results on Tuesday, December 5th. The company reported $0.96 earnings per share (EPS) for the quarter, beating analysts’ consensus estimates of $0.51 by $0.45. The company had revenue of $432.94 million for the quarter, compared to analyst estimates of $406.33 million. MongoDB had a negative return on equity of 20.64% and a negative net margin of 11.70%. The firm’s quarterly revenue was up 29.8% compared to the same quarter last year. During the same quarter in the previous year, the company earned ($1.23) earnings per share. As a group, equities research analysts expect that MongoDB, Inc. will post -1.64 EPS for the current year.
Insider Transactions at MongoDB
In other news, CEO Dev Ittycheria sold 100,500 shares of the company’s stock in a transaction on Tuesday, November 7th. The stock was sold at an average price of $375.00, for a total transaction of $37,687,500.00. Following the sale, the chief executive officer now owns 214,177 shares of the company’s stock, valued at $80,316,375. The transaction was disclosed in a filing with the Securities & Exchange Commission, which can be accessed through this hyperlink. In related news, CEO Dev Ittycheria sold 100,500 shares of the stock in a transaction on Tuesday, November 7th. The stock was sold at an average price of $375.00, for a total value of $37,687,500.00. Following the transaction, the chief executive officer now owns 214,177 shares of the company’s stock, valued at $80,316,375. The transaction was disclosed in a filing with the Securities & Exchange Commission, which is accessible through this link. Also, CFO Michael Lawrence Gordon sold 21,496 shares of the stock in a transaction on Monday, November 20th. The shares were sold at an average price of $410.32, for a total value of $8,820,238.72. Following the completion of the transaction, the chief financial officer now directly owns 89,027 shares in the company, valued at approximately $36,529,558.64. The disclosure for this sale can be found here. Over the last 90 days, insiders have sold 149,882 shares of company stock valued at $57,313,539. 4.80% of the stock is currently owned by company insiders.
Wall Street Analysts Forecast Growth
Several research analysts recently commented on the stock. Royal Bank of Canada boosted their price objective on shares of MongoDB from $445.00 to $475.00 and gave the stock an “outperform” rating in a research note on Wednesday, December 6th. Argus boosted their target price on shares of MongoDB from $435.00 to $484.00 and gave the stock a “buy” rating in a research report on Tuesday, September 5th. Scotiabank assumed coverage on shares of MongoDB in a research report on Tuesday, October 10th. They set a “sector perform” rating and a $335.00 target price on the stock. Canaccord Genuity Group boosted their target price on shares of MongoDB from $410.00 to $450.00 and gave the stock a “buy” rating in a research report on Tuesday, September 5th. Finally, Capital One Financial raised shares of MongoDB from an “equal weight” rating to an “overweight” rating and set a $427.00 price objective on the stock in a report on Wednesday, November 8th. One equities research analyst has rated the stock with a sell rating, two have assigned a hold rating and twenty-two have issued a buy rating to the stock. According to MarketBeat.com, the company has an average rating of “Moderate Buy” and an average price target of $432.44.
Get Our Latest Analysis on MongoDB
MongoDB Profile
MongoDB, Inc provides general purpose database platform worldwide. The company offers MongoDB Atlas, a hosted multi-cloud database-as-a-service solution; MongoDB Enterprise Advanced, a commercial database server for enterprise customers to run in the cloud, on-premise, or in a hybrid environment; and Community Server, a free-to-download version of its database, which includes the functionality that developers need to get started with MongoDB.
Further Reading
Want to see what other hedge funds are holding MDB? Visit HoldingsChannel.com to get the latest 13F filings and insider trades for MongoDB, Inc. (NASDAQ:MDB – Free Report).
Receive News & Ratings for MongoDB Daily – Enter your email address below to receive a concise daily summary of the latest news and analysts’ ratings for MongoDB and related companies with MarketBeat.com’s FREE daily email newsletter.
Article originally posted on mongodb google news. Visit mongodb google news
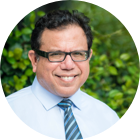
MMS • RSS
Posted on mongodb google news. Visit mongodb google news
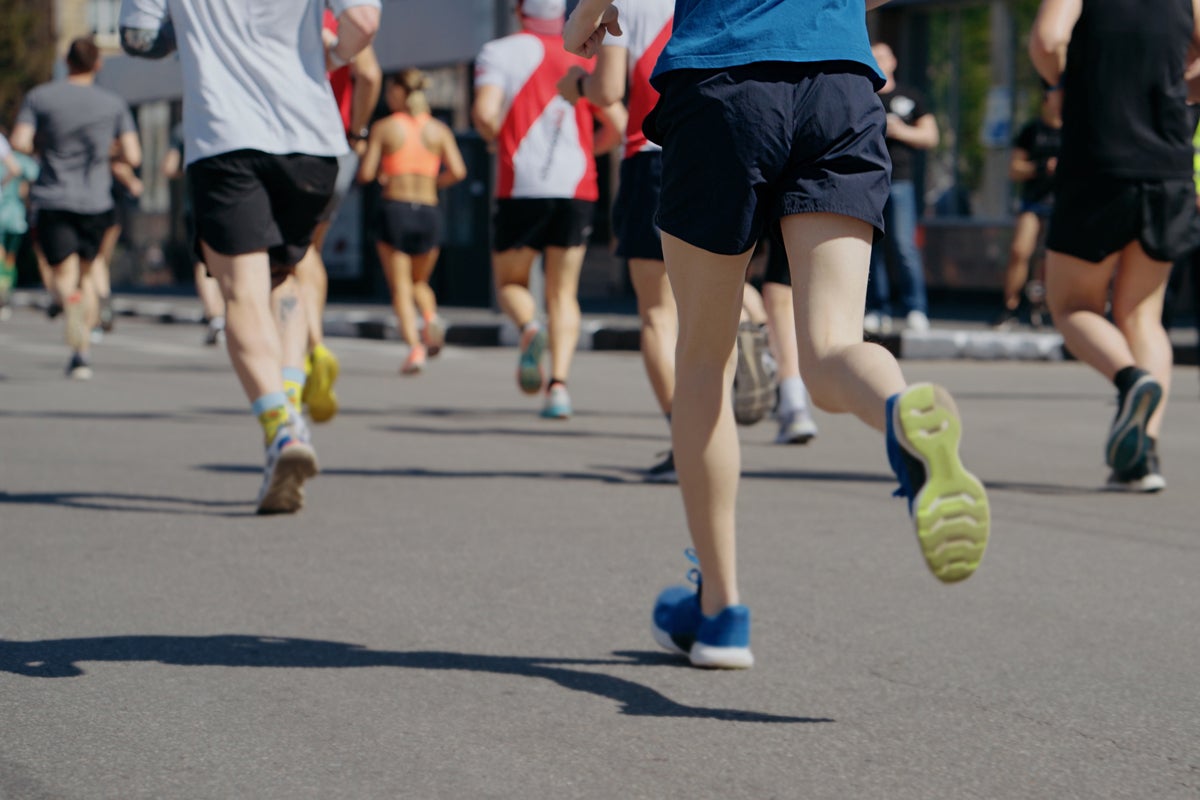
Open source pioneer Bruce Perens gets one thing right and most things wrong in a recent interview on the future of open source. He’s absolutely correct that “our [open source] licenses aren’t working anymore,” even if he’s wrong as to why. (He says “businesses have found all of the loopholes.”)
No, the problem is that open source has never been more important, yet less relevant to the biggest technology trends of our time: cloud computing and artificial intelligence. In 2024, we need open source to catch up with these technologies.
Clouds gathering over open source
It’s fashionable in some quarters to blame companies like MongoDB (disclosure: I work for MongoDB), Neo4j, Elastic, HashiCorp, etc., for allegedly polluting open source with licenses like the Business Source License, Commons Clause, and Server Side Public License (SSPL). But the problem isn’t so much these companies as the fact that they tried to distribute cloud services under open source licenses that simply don’t work for the cloud.
Don’t believe me? Ask Stefano Maffulli, executive director of the Open Source Initiative (OSI), which shepherds the Open Source Definition (OSD). In an interview, Maffulli told me, “Open source kind of missed the evolution of the way software is distributed and executed.” All open source licenses were conceived in a pre-cloud era and assume an outdated method for distributing software. With the Affero General Public License (AGPL), the OSI embraced a hack that wasn’t cloud native. As such, Maffulli continues, “We didn’t really pay attention to what was going on and that led to a lot of tension in the cloud business.”
Some of that tension played out while I was working at AWS. My current employer, MongoDB, tried to get the SSPL approved as an official open source license by the OSI. Eventually, the company withdrew from the process, which was unfortunate. If you like the GPL, you should like the SSPL, as it’s basically a cloudified GPL. Unlike the Business Source License and more recent licenses, the SSPL doesn’t discriminate against certain kinds of use of the software (i.e., there is no restriction on running the software in production for commercial or competitive purposes). It simply says that if you distribute the software as a service, you need to make available all other software used to run it, because what good is freedom to inspect, modify, and run software if the essential software infrastructure to power it is completely closed? (You can see the differences between the AGPL and SSPL clearly delineated here.)
In 2024, the OSI needs to get serious about updating its open source definition to be relevant for the cloud. It doesn’t need to be the SSPL, but it does need to reflect the fact that most software isn’t distributed in the same way the OSD’s “open source” contemplates. We’re still using horse-and-buggy definitions of open source to try to capture electric cars and rocket ships of our modern reality.
Making open source meaningless in the AI era
As much as cloud has outpaced open source, AI has rendered it utterly meaningless. I’ve discussed this at length (see here and here), but it comes down to a fundamental question: What is the “code” that open source would hope to preserve?
In a conversation with Aryn CEO Mehul Shah, we hashed through this problem of “code.” Quoting that article at length:
The first is to think of curated training data like the source code of software programs. If we start there, then training (gradient descent) is like compilation of source code, and the deep neural network architecture of transformer models or [large language models] is like the virtual hardware or physical hardware that the compiled program runs on. In this reading, the weights are the compiled program.
This seems reasonable but immediately raises key questions. First, that curated data is often owned by someone else. Second, although the licenses are on the weights today, this may not work well because those weights are just floating-point numbers. Is this any different from saying you’re licensing code, which is just a bunch of 1s and 0s? Should the license be on the architecture? Probably not, as the same architecture with different weights can give you a completely different AI. Should the license then be on the weights and architecture? Perhaps, but it’s possible to modify the behavior of the program without access to the source code through fine-tuning and instruction tuning. Then there’s the reality that developers often distribute deltas or differences from the original weights. Are the deltas subject to the same license as the original model? Can they have completely different licenses?
We can’t, in short, simply say a large language model is open source, because we can’t even yet decide what, exactly, should be open. This is similar to the problem the SSPL was trying to resolve, but it’s even more complicated. “There is no settled definition of what open source AI is,” argues Mike Linksvayer, head of developer policy at GitHub. We’re nowhere near resolving that quandary.
Fortunately, this time around, the OSI isn’t asleep at the OSD wheel and is actively working through what the OSD should be for AI. However, Maffulli stresses, “It’s an extremely complex scenario.” My New Year’s wish for our industry is that the OSI takes responsibility for upgrading the OSD for both cloud and AI. We’ve spent the last few years castigating companies for not abiding by open source principles that the OSI failed to make relevant for the biggest trends in software. This year, that needs to stop.
Next read this:
Article originally posted on mongodb google news. Visit mongodb google news
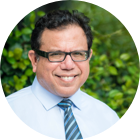
MMS • Patrick Zhang
Article originally posted on InfoQ. Visit InfoQ
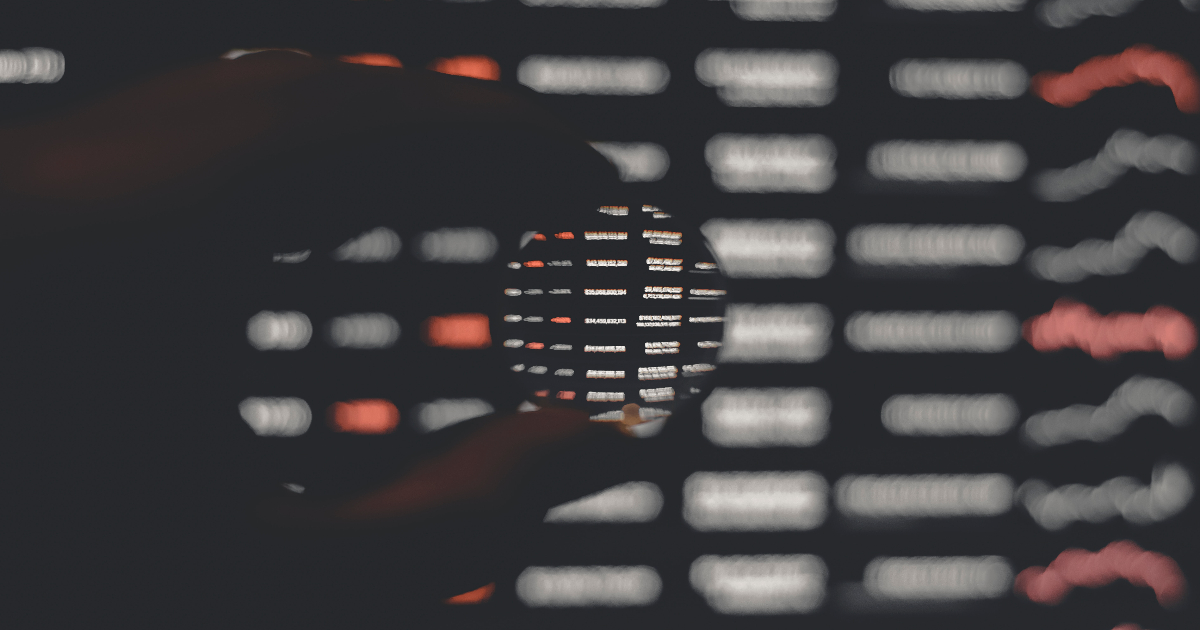
Uber has developed a new tool named CheckEnv to address the complexity of its microservices architecture, where numerous loosely coupled services interact through remote procedure calls (RPCs). This tool is designed to swiftly detect and address RPC calls crossing between different environments, such as production and staging, which could lead to undesirable outcomes like data inconsistencies or unexpected behaviors.
CheckEnv utilizes dependency graphs, which represent service-to-service calls, providing insights into communication patterns and dependencies. This visualization helps in pinpointing cross-environment RPC calls. The system employs advanced graph analysis techniques to automate the detection process, integrating these capabilities into Uber’s monitoring and alerting systems for prompt resolution of such issues.
The tool incorporates both real-time and aggregated dependency graphs. The real-time graph is updated continually, capturing essential metrics and identifying potential issues in service dependencies. The aggregated graph, on the other hand, provides a historical perspective of service interactions, aiding in the analysis of system performance over time.
CheckEnv operates on two graph data storage systems, Grail and Local Graph, within Uber. These platforms aggregate and store call graph data, with CheckEnv providing APIs to access and retrieve information like service dependencies and paths leading to production dependencies. This setup enhances the ability to identify anomalies, troubleshoot issues, and optimize the microservices architecture.
An example of CheckEnv’s application is in Uber’s synthetic load testing platform, Ballast. Here, it detects potential cross-environment calls during load tests, ensuring a secure and reliable testing environment by alerting users to any potential issues before they escalate.
Looking ahead, Uber plans to expand the capabilities of CheckEnv and its underlying data ingestion pipeline, MazeX, to construct a more powerful graph. This expansion aims to enhance the system’s ability to analyze communication patterns between services, optimizing data flow and improving service efficiency. This graph-based approach is expected to address various challenges within the microservice architecture, like real-time fault detection and workflow management.