Month: May 2024
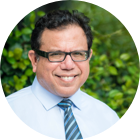
MMS • RSS
Posted on mongodb google news. Visit mongodb google news
With its own MongoDB Atlas development platform at the core, the new MAAP initiative pulls together expertise and technology from cloud hyperscalers, leading GenAI tech providers such as Anthropic and LangChain, and MongoDB consulting/SI partners.
MongoDB has launched a program that provides a complete technology stack, services and other resources to help businesses develop and deploy at scale applications with advanced generative AI capabilities.
The new MongoDB AI Applications Program (MAAP), with the MongoDB Atlas cloud database and development platform at its core, including reference architectures and technology from a who’s who in the AI space including the cloud platform giants, LLM (large language model) tech developers Cohere and Anthropic, and a number of AI development tool companies including Fireworks.ai, LlamaIndex and Credal.ai.
The new program also includes strategic advisory and professional services from MongoDB and from a number of the company’s systems intregrator and consulting partners.
[Related: MongoDB CEO On New AI, LLMs And GenAI Innovation In 2024]
“So many of these enterprise customers are struggling. They’re trying to figure out how to best get an ROI with AI,” said Alan Chhabra, MongoDB executive vice president of worldwide partners, in an interview with CRN.
Many businesses, while recognizing “the transformative potential of GenAI,” are “facing challenges even getting started due to the rapidly evolving landscape, a lack of in-house AI development expertise, and the perceived risk of integrating all these fragmented vendor solutions,” said Greg Maxson, MongoDB senior director and global lead, AI go-to-market and strategic partnerships, during an online press conference prior to today’s announcement.
MongoDB executives on the press call cited the results of an Accenture survey (published in Forbes earlier this year) that found that only 27 percent of C-suite executives said their organizations were ready to scale up generative AI initiatives.
MongoDB is a major player in the AI universe with its popular MongoDB Atlas cloud development platform and MongoDB Atlas Vector Search database for enterprise developers and ISVs. The Atlas Vector Search and Atlas Search Nodes offerings became generally available in December.
The New York-based company also has strategic alliances with Amazon Web Services, Microsoft Azure and Google Cloud – all of whom are major players in the AI and GenAI space with their own AI tools and systems as well as providing the cloud platforms for many AI initiatives. MongoDB’s Atlas Vector Search, for example, its integrated with AWS’ Amazon Bedrock generative AI platform.
Maxson described MAAP as “a one-stop shop of the essential tools and expertise addressing these development challenges” and that “brings together each part of the stack that an enterprise would need in order to build a complete GenAI application.”
Helping customers use Retrieval Augmented Generation (a framework for pulling data into LLMs) is a focus for the new program, Maxson said, as is helping them govern and secure data used by GenAI applications.
In addition to the technical aspects of building GenAI applications, program participants will work with customers upfront to develop comprehensive strategies and roadmaps for building, deploying and scaling generative AI applications. That includes identifying business problems and revenue-generating opportunities where GenAI applications would be applicable.
In addition to MongoDB’s own cloud database platform and MongoDB Professional Services, the program brings together consultants, foundation model developers, cloud infrastructure providers, and generative AI framework and model hosting service providers.
MAAP launch partners include cloud hyperscalers AWS, Google Cloud and Microsoft Azure; foundation model developers Anthropic, Cohere and Nomic; and AI framework and model hosting companies Anyscale, Fireworks.ai, and Together AI. Also participating are LLM data framework provider LlamaIndex, development platform company LangChain, and AI development and security tool company Credal.ai.
“Deploying accurate and reliable AI solutions is a top priority for enterprises today. Through our participation in MongoDB’s AI Applications Program, more businesses will harness the power of Anthropic’s industry-leading Claude 3 model family to develop tailored solutions that drive real business impact,” said Kate Jensen, head of revenue at Anthropic, in a statement. “By integrating Anthropic’s powerful foundation models with MongoDB’s cutting-edge technology and expertise, more enterprises in regulated industries such as financial services and healthcare will confidently deploy customized AI solutions that meet their standards on safety and security.”
Also participating in the MAAP launch are a number of “boutique systems integrators” that MongoDB has previously invested in including PeerIslands, Pureinsights and Gravity 9.
“So you can think of MAAP as a combination of all of the technology components, as well as the professional services, required to get an enterprise up and running with a generative AI app that they can then take and own and run with confidence without needing ongoing continuous support,” Maxson said.
MAAP, slated to be generally available in July, will also have a strong focus on specific vertical industries including automotive, retail and financial services, given the unique AI needs of each, according to Chhabra. He said MongoDB also will take on a more unified support role for GenAI implementations built using the MAAP ecosystem.
Article originally posted on mongodb google news. Visit mongodb google news
MongoDB Launches Ambitious AI Applications Program to Empower Next-Gen Business Solutions
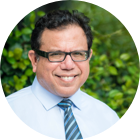
MMS • RSS
Posted on mongodb google news. Visit mongodb google news
In a bold move to democratize the integration of artificial intelligence across various industries, MongoDB has announced the launch of its innovative AI applications program. This initiative is designed to help businesses harness the power of AI by providing them with the tools and frameworks to integrate advanced AI technologies seamlessly into their operations. MongoDB’s President and CEO, Dev Ittycheria, unveiled this program on CNBC’s “Squawk Box,” emphasizing the company’s commitment to leading the AI revolution in database management and business applications.
Bridging the AI Integration Gap
MongoDB’s new program addresses a critical gap in the current tech landscape—the complexity of integrating AI into traditional business processes. Many companies struggle with the rapid pace of AI development and the technical challenges associated with adopting these technologies. MongoDB aims to simplify this process by offering a structured program with reference architectures tailored for specific use cases, built-in integrations, and access to technical expertise.
“We recognize that the influx of new models can be overwhelming for businesses,” Ittycheria explained. “Our AI applications program is crafted to mitigate this by providing clear, actionable guidance and support, helping companies integrate AI with less risk and greater speed.”
Strategic Partnerships and Industry Collaboration
A key component of MongoDB’s strategy is its collaboration with leading AI innovators and tech companies. The program boasts partnerships with major players like Anthropic and other tech firms specializing in AI orchestration and model fine-tuning. This collaborative approach ensures that MongoDB can offer cutting-edge solutions that are versatile and scalable, accommodating the diverse needs of its clients.
These partnerships are pivotal for enhancing MongoDB’s service offerings and keeping the company at the forefront of the AI revolution. MongoDB positions itself as a central hub for AI-driven business transformation by aligning with top-tier AI developers and researchers.
Competitive Landscape and Open Source Implications
During the discussion, Ittycheria also delved into the competitive dynamics of the AI market, particularly highlighting the impact of open-source models like Facebook’s LLaMA 3. These models are setting new standards in performance and accessibility, challenging the viability of closed, proprietary systems. “The rapid depreciation of foundational model assets is reshaping our industry,” Ittycheria remarked. “What was cutting-edge a few months ago quickly becomes outdated as newer, more advanced models emerge.”
This fast-paced evolution raises significant questions about the sustainability of investing in closed AI systems when open-source alternatives accelerate capability and adoption.
Real-World Applications and Customer Impact
Ittycheria shared several compelling use cases demonstrating how AI can revolutionize business operations. One notable example is an automotive company that developed an application capable of diagnosing vehicle issues through sound analysis. This app uses AI to analyze audio recordings of a car in operation, quickly identifying potential problems based on a database of known issue sounds.
Another example involved banks utilizing AI to streamline the costly and complex process of migrating from outdated legacy systems to modern platforms. These applications enhance operational efficiency and significantly improve the customer experience by speeding up service delivery and reducing downtime.
Looking Ahead: The Future of AI in Business
As MongoDB continues to expand its AI program, the focus remains on enabling businesses to not just adapt to but excel in an AI-dominated future. The overarching goal is to transform these advanced technologies from experimental tools into essential components of everyday business processes.
“It’s an exciting time to be at the intersection of AI and enterprise applications,” Ittycheria concluded. “With our AI applications program, we’re not just following trends—we’re creating them, helping businesses unlock the full potential of their data and innovate at scale.”
MongoDB’s initiative represents a significant leap towards making AI a practical and integral part of business operations worldwide. It promises a future where AI and human creativity converge to create unprecedented opportunities for growth and innovation.
Article originally posted on mongodb google news. Visit mongodb google news
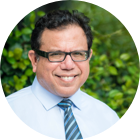
MMS • RSS
Posted on mongodb google news. Visit mongodb google news
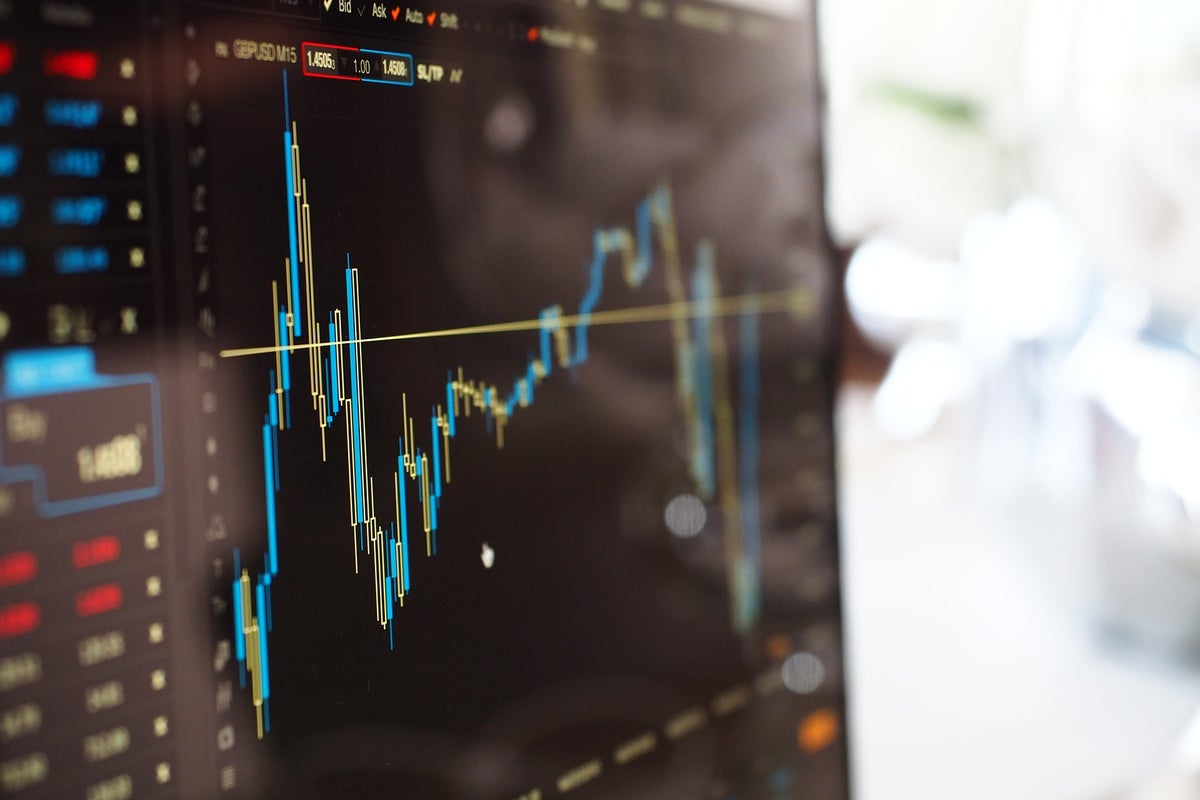
Financial giants have made a conspicuous bearish move on MongoDB. Our analysis of options history for MongoDB MDB revealed 16 unusual trades.
Delving into the details, we found 31% of traders were bullish, while 62% showed bearish tendencies. Out of all the trades we spotted, 12 were puts, with a value of $508,740, and 4 were calls, valued at $152,105.
Expected Price Movements
Taking into account the Volume and Open Interest on these contracts, it appears that whales have been targeting a price range from $320.0 to $430.0 for MongoDB over the last 3 months.
Insights into Volume & Open Interest
In terms of liquidity and interest, the mean open interest for MongoDB options trades today is 277.09 with a total volume of 397.00.
In the following chart, we are able to follow the development of volume and open interest of call and put options for MongoDB’s big money trades within a strike price range of $320.0 to $430.0 over the last 30 days.
MongoDB Option Volume And Open Interest Over Last 30 Days
Significant Options Trades Detected:
Symbol | PUT/CALL | Trade Type | Sentiment | Exp. Date | Ask | Bid | Price | Strike Price | Total Trade Price | Open Interest | Volume |
---|---|---|---|---|---|---|---|---|---|---|---|
MDB | PUT | SWEEP | NEUTRAL | 09/20/24 | $39.35 | $39.2 | $39.2 | $350.00 | $78.6K | 289 | 42 |
MDB | PUT | SWEEP | BEARISH | 05/10/24 | $11.45 | $10.3 | $11.45 | $370.00 | $58.4K | 817 | 51 |
MDB | CALL | SWEEP | BEARISH | 09/20/24 | $34.3 | $32.95 | $32.95 | $430.00 | $56.0K | 20 | 0 |
MDB | PUT | SWEEP | BEARISH | 05/03/24 | $2.0 | $1.85 | $2.0 | $355.00 | $47.6K | 379 | 14 |
MDB | PUT | TRADE | BULLISH | 06/21/24 | $20.25 | $20.0 | $20.0 | $340.00 | $44.0K | 709 | 24 |
About MongoDB
Founded in 2007, MongoDB is a document-oriented database with nearly 33,000 paying customers and well past 1.5 million free users. MongoDB provides both licenses as well as subscriptions as a service for its NoSQL database. MongoDB’s database is compatible with all major programming languages and is capable of being deployed for a variety of use cases.
In light of the recent options history for MongoDB, it’s now appropriate to focus on the company itself. We aim to explore its current performance.
Current Position of MongoDB
- Currently trading with a volume of 326,076, the MDB’s price is up by 1.77%, now at $371.64.
- RSI readings suggest the stock is currently may be approaching overbought.
- Anticipated earnings release is in 29 days.
What The Experts Say On MongoDB
A total of 4 professional analysts have given their take on this stock in the last 30 days, setting an average price target of $446.25.
- Maintaining their stance, an analyst from Keybanc continues to hold a Overweight rating for MongoDB, targeting a price of $440.
- In a cautious move, an analyst from Needham downgraded its rating to Buy, setting a price target of $465.
- Reflecting concerns, an analyst from Loop Capital lowers its rating to Buy with a new price target of $415.
- In a cautious move, an analyst from Needham downgraded its rating to Buy, setting a price target of $465.
Options are a riskier asset compared to just trading the stock, but they have higher profit potential. Serious options traders manage this risk by educating themselves daily, scaling in and out of trades, following more than one indicator, and following the markets closely.
If you want to stay updated on the latest options trades for MongoDB, Benzinga Pro gives you real-time options trades alerts.
Market News and Data brought to you by Benzinga APIs
© 2024 Benzinga.com. Benzinga does not provide investment advice. All rights reserved.
Article originally posted on mongodb google news. Visit mongodb google news
MongoDB, Inc. Launches New Program for Enterprises to Build Modern Applications with …
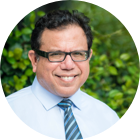
MMS • RSS
Posted on mongodb google news. Visit mongodb google news
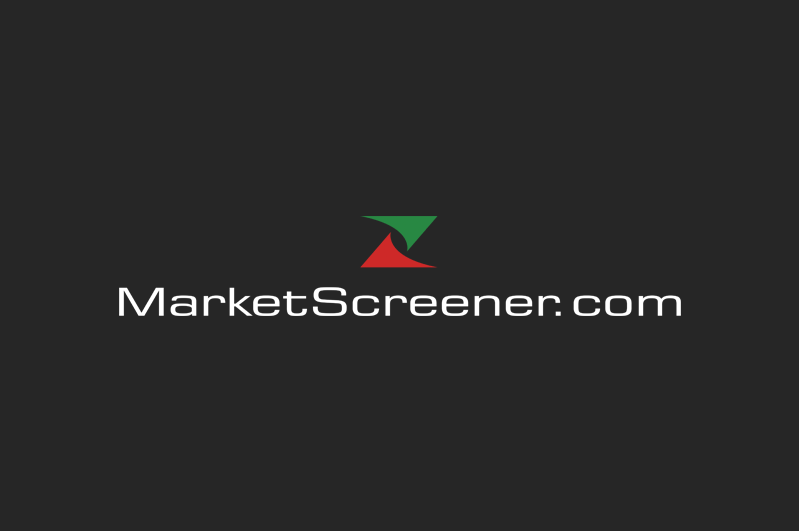
MongoDB, Inc. announced the MongoDB AI Applications Program (MAAP) that is designed to help organizations rapidly build and deploy modern applications enriched with generative AI technology at enterprise scale. Organizations of every size across industries want to immediately take advantage of this technological shift, but are unsure if they have the right data strategy and technology in place that will lead to success in building, deploying, and scaling new classes of applications securely and reliably. Many organizations are stuck with ineffective ways of working with data because of legacy technology that cannot scale, while others attempt to use single-purpose, bolt-on solutions that introduce unnecessary complexity and cost.
As a result, these organizations wind up trading off long-term success– because of outdated technology or add-on solutions–for short-term results with proofs of concept that cannot scale to production, offer enterprise-grade security and reliability, or provide a meaningful return on their investment. MAAP offers customers the technology, full-service engagement, and expert support required to: Develop end-to-end strategies and roadmaps to build, deploy, and scale generative AI applications with hands-on support: Engagement with MAAP begins with highly personalized deep dives. MongoDB Professional Services and consultancy partners then develop strategic roadmaps to rapidly prototype the required architecture, validate that initial results meet customer expectations, and then optimize fully built applications that customers can evolve for use in production.
Customers can continue receiving support from MongoDB Professional Services to develop new generative AI features depending on their needs. Build high-performing generative AI applications that are secure, reliable, and trustworthy: Enterprises must trust that new technology being deployed throughout their organization and in customer-facing applications will behave as expected and will not inadvertently expose sensitive data. MongoDB’s partnerships and integrations have helped company fine-tune AI models to specific use cases, enhancing Spotlight’s performance.
Article originally posted on mongodb google news. Visit mongodb google news
Unveiling MongoDB.Local: Setting the stage for next-gen AI applications – SiliconANGLE
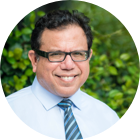
MMS • RSS
Posted on mongodb google news. Visit mongodb google news
At MongoDB Inc.’s flagship developer conference in New York City last year, I spoke with Chief Executive Dev Ittycheria, who offered his take on the burgeoning generative artificial intelligence wave. Ittycheria predicted that as developers began to experiment with new AI tools, including those within MongoDB’s developer data platform, they’d see productivity gains and would be able to build revolutionary new kinds of applications.
This week, MongoDB returns with its annual New York City developer conference, MongoDB.local NYC 2024, which kicks off its world tour of events across 23 cities. I sat down with Ittycheria again recently to hear his vision and predictions on how MongoDB is enabling organizations to maximize their data value from their developer platform for AI-applications.
A year of generative AI experimentation
“Investing in AI became an imperative for every organization this year,” Ittycheria (pictured) told me. “This is a crucible moment for technology, and businesses want to capitalize on generative AI to reimagine how their businesses operate and how they support their customers.”
Despite the enthusiasm, Ittycheria acknowledged that only a small fraction of executives feel fully prepared to meet AI’s demands, and truly transformative AI applications are yet to be fully realized.
According to Ittycheria, the industry’s journey with AI is a marathon, not a sprint, characterized by significant long-term impacts that often overshadow short-term benefits. As organizations continue to test and learn, the goal is to ensure that AI initiatives translate into substantial enterprise outcomes.
Overwhelmed yet opportunistic
“Enterprises are overwhelmed by the rapid pace and breadth of options in the AI space,” Ittycheria explained. He sees a critical need for solutions that simplify the start and management of AI applications, amid the noise of the rapidly evolving AI landscape.
Survey data from ETR indicates how organizations are looking at AI. The data shows that test summarization, support for writing content, customer chat and code generation are the top use cases. However the really game changing use cases haven’t emerged and will likely take longer to demonstrate return.
The research data confirms the growing interest and integration of generative AI and large language models across various business use cases. There is an appetite for the evaluation of AI while most of the action is with developers around code generation and documentation. The business use cases in the areas of customer support and content creation, including marketing and sales copy, have seen increases in interest and implementation.
Specifically, customer support applications show the largest growth, indicating a significant shift toward using AI for enhancing customer interactions. The bottom line is that the market is showing a broader understanding and acceptance of AI technologies in business operations.
According to Ittycheria, organizations are eager to take advantage of the opportunities that can come from generative AI, but they’re overwhelmed. “Right now, enterprises want to unlock value from AI-powered applications, but the space is moving quickly and there’s so much choice that many enterprises don’t know where to start,” he said.
Introducing the MongoDB AI Applications Program
In response to the demand for faster capture of generative AI value, the company is launching the MongoDB AI Applications Program or MAAP, a first-of-its-kind collaboration that brings together industry-leading consultancies and foundation model providers, cloud infrastructure providers, and generative AI frameworks — all in one place.
This initiative provides customers with tailored architectures and expert services to quickly develop and scale AI solutions addressing key business challenges. MAAP aims to be a cornerstone for organizations seeking to leverage AI, with partners such as Anthropic PBC, Amazon Web Services Inc. and Google Cloud signaling strong industry support for MongoDB’s strategic direction.
“Organizations need trusted partners to capitalize on this crucible moment,” Ittycheria told me. “From our flexible document model designed to accommodate different data types critical to building AI apps to our deep partnerships with the leading AI companies and cloud providers, MongoDB offers a unique solution in today’s market.”
MAAP will be available with top-tier consultancies, model providers and cloud service providers to streamline AI adoption. Industry partners include Anthropic, Anyscale, AWS, Cohere, Fireworks.ai, Google Cloud, gravity9, LangChain, LlamaIndex, Nomic, Microsoft Azure, PeerIslands, PureInsights and Together AI.
“This collaboration is a powerful signal that AI leaders recognize that MongoDB is a critical player in enabling organizations to transform with AI,” said Ittycheria.
Capitalizing on the application layer
Companies such as the cloud players, Nvidia Corp. and OpenAI have made significant strides at the infrastructure level with high performance systems and custom chips, MongoDB is focused on harnessing AI at the application layer to enhance developer productivity and customer engagement. This approach is expected to drive rapid adoption and value from organizations’ data across various industries.
According to theCUBE Research analyst Rob Strechay, it is clear that MongoDB is looking up the stack, building an ecosystem of partners that understands the technology and business drivers to realize ROI from AI. “MongoDB is focused on AI as part of the application and by focusing on customer success and not building a foundation model will help MongoDB customers build better data apps that include GenAI and heritage AI,” says Strechay.
Analysts all agree enterprises will reap AI’s value through increases in developer productivity, faster time to market for new applications, and enriched ways for customers to interact with their brands through these applications — all of which will fuel competitive advantage across industries. “It’s the applications built on this infrastructure that will actually make businesses more efficient, enrich customer experiences and transform our lives,” Ittycheria said.
A unified platform for modern application development
At the heart of MongoDB is a globally distributed operational database with a flexible data model that offers developers a natural way to represent diverse data types so they can deploy applications that effortlessly adapt, scale and perform at the level that the most innovative organizations with the most demanding use cases require.
“Fortune 500 giants and startups alike choose MongoDB as the best platform for building scalable, high-performing, and intelligent apps that can efficiently manage diverse data types and adapt to evolving technological requirements, like AI functionality,” said Ittycheria.
The recently launched MAAP claims to bolster customer support through a broad network of partners, purportedly easing the integration of complex technologies into existing systems. MongoDB promotes MAAP as a solution that offers businesses flexibility and a wide range of AI tools to prevent lock-in with any single technology provider.
“We’ve curated an extensive partner ecosystem so that businesses don’t have to worry about complex technology integrations with their tech stack,” noted Ittycheria.
MongoDB for the AI future
Ittycheria’s vision positions MongoDB as a key player in the next surge of technological innovation, aiming to lead the charge in AI development.
As the AI infrastructure market gears up, the AI application developer space is eager to advance new data-driven AI capabilities. With offerings that promise scalability and advanced intelligence, MongoDB is well-positioned to meet the evolving needs of developers and enterprises alike. This strategic pivot not only highlights MongoDB’s robust capabilities but also signifies its potential to significantly influence the trajectory of AI-driven application development.
If Ittycheria’s predictions hold true, MongoDB could well become a cornerstone in shaping the technological landscape of tomorrow.
Photo: MongoDB
Your vote of support is important to us and it helps us keep the content FREE.
One click below supports our mission to provide free, deep, and relevant content.
Join our community on YouTube
Join the community that includes more than 15,000 #CubeAlumni experts, including Amazon.com CEO Andy Jassy, Dell Technologies founder and CEO Michael Dell, Intel CEO Pat Gelsinger, and many more luminaries and experts.
THANK YOU
Article originally posted on mongodb google news. Visit mongodb google news
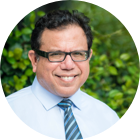
MMS • RSS
Posted on mongodb google news. Visit mongodb google news
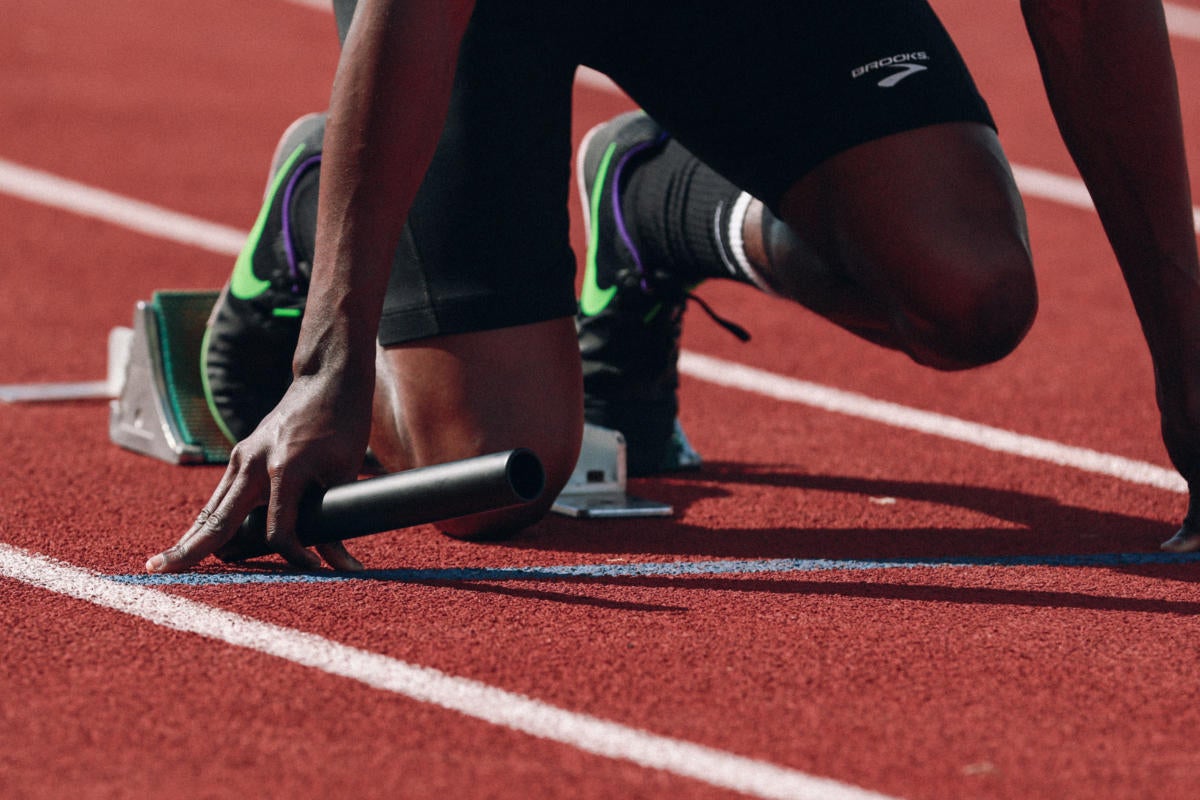
MongoDB has introduced the MongoDB AI Applications Program, or MAAP, to help enterprises jumpstart their development of generative AI applications.
MAAP, according to the company, will offer enterprises strategic advice, professional services, and an integrated end-to-end technology stack from MongoDB and its partners.
These partners include consultancies, foundation model (FM) providers, cloud infrastructure providers, and generative AI framework and model hosting providers including Anthropic, Anyscale, Amazon Web Services (AWS), Cohere, Credal.ai, Fireworks.ai, Google Cloud, gravity9, LangChain, LlamaIndex, Microsoft Azure, Nomic, PeerIslands, Pureinsights, and Together AI.
Typically, an enterprise signing up for the program will see initial engagement from MongoDB Professional Services, which will evaluate the enterprise’s current technology stack and identify business problems to solve.
Once the evaluation is complete, MongoDB Professional Services and partners together will develop strategic roadmaps to prototype the required architecture, validate the initial results against the enterprise’s expectations, and then optimize fully built applications that the enterprise can evolve for use in production, MongoDB said.
Any enterprise that is a part of the program can continue receiving support from MongoDB Professional Services to develop new generative AI features depending on their needs, the company added.
Several enterprises, including ACI Worldwide, Arc XP, and Scalestack, are already part of MAAP, MongoDB said.
MAAP is MongoDB’s way of being more than just a database vendor and an attempt to attract new customers, analysts said.
“Like others in the space, MongoDB is moving beyond serving as a database vendor to supply a broad developer ecosystem that enables rapid innovation. MAAP is a natural extension of this evolution as businesses are navigating how to incorporate the use of generative AI,” said Erik Brown, senior partner of AI and product engineering at digital services firm West Monroe.
Market research firm IDC’s research vice president Arnal Dayaratna believes that MongoDB’s primary, unstated goal behind MAAP is to accelerate the adoption of MongoDB databases, and in particular their vector database technologies.
“MongoDB is launching a generative AI stack that allows customers to choose from a variety of models. But they will likely be steered to or required to use MongoDB database technologies,” Dayaratna said.
The MongoDB AI Applications Program, according to dbInsights’ principal analyst Tony Baer, could also be seen as a reaction to the huge demand from MongoDB’s existing customer enterprises who want to implement generative AI.
Next read this:
Article originally posted on mongodb google news. Visit mongodb google news
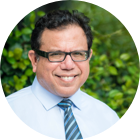
MMS • RSS
Posted on mongodb google news. Visit mongodb google news
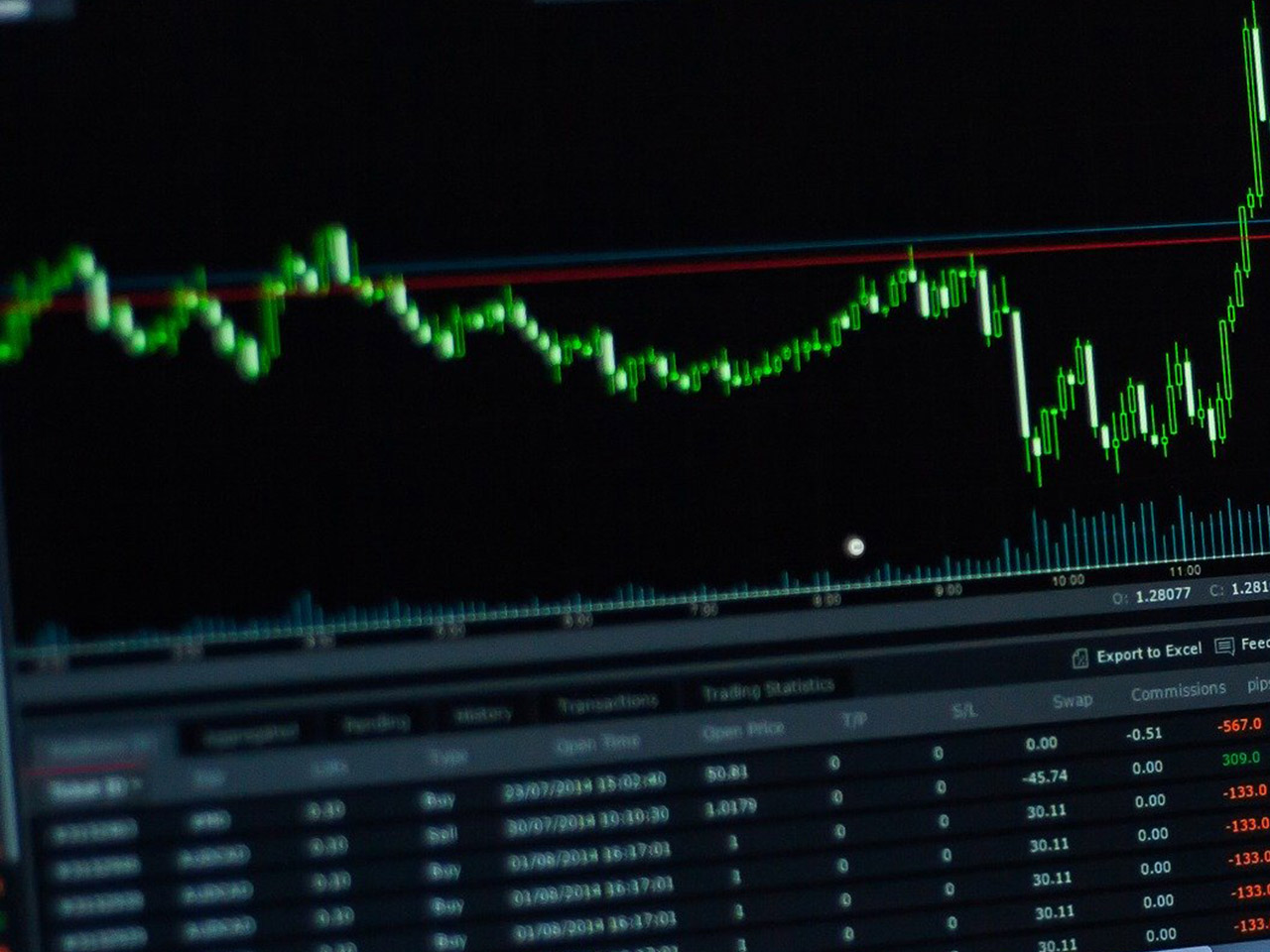
MongoDB, Inc. which can be found using ticker (MDB) have now 30 confirmed analysts covering the stock with the consensus suggesting a rating of ‘buy’. The range between the high target price and low target price is between $700.00 and $272.00 and has a mean share price target at $448.06. (at the time of writing). Given that the stocks previous close was at $371.94 and the analysts are correct then there would likely be a percentage uptick in value of 20.5%. The 50 day MA is $377.87 and the 200 moving average now moves to $384.72. The market cap for the company is 26.60B. The current stock price for Hilton Worldwide Holdings Inc. is currently $365.18 USD
The potential market cap would be $32,039,605,928 based on the market consensus.
The company is not paying dividends at this time.
Other points of data to note are a P/E ratio of -, revenue per share of $23.62 and a -5.35% return on assets.
MongoDB, Inc. is a developer data platform company. Its developer data platform is an integrated set of databases and related services that allow development teams to address the growing variety of modern application requirements. Its core offerings are MongoDB Atlas and MongoDB Enterprise Advanced. MongoDB Atlas is its managed multi-cloud database-as-a-service offering that includes an integrated set of database and related services. MongoDB Atlas provides customers with a managed offering that includes automated provisioning and healing, comprehensive system monitoring, managed backup and restore, default security and other features. MongoDB Enterprise Advanced is its self-managed commercial offering for enterprise customers that can run in the cloud, on-premises or in a hybrid environment. It provides professional services to its customers, including consulting and training. It has over 40,800 customers spanning a range of industries in more than 100 countries around the world.
Article originally posted on mongodb google news. Visit mongodb google news
Podcast: Security Requires Traveling the Unhappy Path – A Conversation with Robert Hurlbut
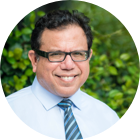
MMS • Robert Hurlbut
Article originally posted on InfoQ. Visit InfoQ
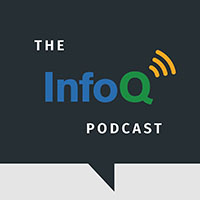
Subscribe on:
Introduction [00:50]
Michael Stiefel: Welcome to the Architects Podcast where we discuss what it means to be an architect and how architects actually do their job. Today’s guest is Robert Hurlbut, who is a Principal Application Security Architect and Threat Modeling Lead at Aquia, a PhD student at Cap TechU and co-host of the Application Security Podcast. It’s great to have you here on the podcast. I’d like to start out by asking you, were you trained as an architect? How did you become an architect? It’s not something you decided one morning, you woke up, got out of bed and said, “Today, I’m going to be an architect.”
How I Became a Security Architect [01:29]
Robert Hurlbut: Interesting story. No, I wasn’t trained directly as an architect. It wasn’t something that when I went to school many, many years ago that I took a track to be an architect. Instead, for many years I was a software developer and my background originally was mathematics and physics. But when I graduated, even while I was in school, I was doing some software development. I had a minor in computer science as well, but as I graduated, I had opportunity to go into the software field to do some application development, and I took it. That’s where I stayed for many years, focused on building applications. So that’s where I started in terms of then learning about software architecture.
I can remember in particular being a part of a couple of engagements. There was a point in time where I started to convert towards being a consultant or contractor really, more than anything else, opportunities to work on lots of different projects, lots of different companies. But being a part of one in particular, I remember someone saying, I’m an architect, and I thought that was a really interesting profession, what they were doing. They did a little bit of coding, but mostly they were designing the system that we were building. They would draw some diagrams and share it with us and they would talk about some decisions that needed to be made.
As I saw what this person was doing, I thought, “Well, that’s a really interesting role to consider in the future. How would I do that myself? How would I get to a place where I could then maybe say I’m an architect or I’m filling that role?” That’s my beginning, introduction to it, not in school, but just through work experiences and seeing others who said they’re architects.
Michael Stiefel: That’s interesting, you took what many people consider to be the traditional path, developer then architect. I would argue that actually there are people who are good at development, there are people who are good at architecture and there are skill sets that are different for different people, and one is not better than the other. Of course, sometimes the architect is also a developer and sometimes the developer does a little architecture. How do you feel about that?
Robert Hurlbut: Yes, great question. Over time I would see different styles, as you mentioned. There would be opportunities to work in an environment or on a team where there is an architect or what you might call a chief architect, that was completely a business role. They did not code at all. They many, many years left that. Now, all they were doing was facilitating, helping other architects on the team who also were just focused on building out system architectures and evaluating some of the work and so forth and interacting with the business. Then, there were others that were more focused on a mixture of the two, developer architects is what we call them, where they definitely did a lot of coding.
But they were also taking some time to draw diagrams on a whiteboard, bring the team together, talk about decisions on the design. For myself, actually, I gravitated towards that role more than anything else, was for many, many years more of a developer architect. I think there are, there are many different flavors and perspectives. I think they’re all needed. There is that someone who can see the big picture and keep us on track. Then, there are others who as a developer architect could already understand the code, already understand the platforms and the various advantages and disadvantages and so forth and can make some recommendations.
But also, because they understand the platform, can make some recommendations on system design, on the tools that are being used and so forth. I think all of those are needed, and I don’t see one better than another.
Understanding the Business Is the Foundation for Architecture [06:04]
Michael Stiefel: But thinking about what you just said, it seems to me that there are two things that all those kinds of architects need. One is they do have to understand the business to some extent or another, so that they have to be able to talk technology to the business people and business to the technology. Because one of the things that I’ve discovered over the years is that what looks easy on the outside can be hard actually to implement. Then, conversely, what looks hard to implement can be easy. Someone has to explain to the business people, this is a great idea, but it’s going to take a lot of work even though… It’s easy to write English language statements, but computers don’t understand English language statements, so that can be very difficult to do.
The other thing is that there are certain things that can’t be written to a use case. Security, which is your area of expertise we’ll get to, you can’t write a use case that says, make the system secure. Or, scalability, or responsiveness, or all these kinds of what we like to call the ilities, can’t be written into a use case and therefore have to be the responsibility if not to implement, but at least to be responsible for it. Because in my experience, if no one’s responsible for it or if everybody’s responsible for it, it never gets done.
Robert Hurlbut: Correct, yes.
Michael Stiefel: Do you have any opinion about that?
Robert Hurlbut: Absolutely. I agree a hundred percent that for myself and many architects, business is actually one of the most important things to realize, is that we’re working with a business. We need to understand the business goals. We need to understand the business priorities. That does impact influence, help us navigate what we’re doing, where we’re going. It helps us ask the questions, why are we doing what we’re doing and as well as how, but why? Why here? Why now? Why is that a goal? Why is that and how does that reflect, or will be reflected into the work that I’m doing, and our team is doing and so forth? Absolutely, you got to understand the business, and that means also communication.
Another aspect of an architect is that communication with your teams, but also with the business. Being able to translate those requirements, being able to translate those goals into, as you mentioned, the use case. If I build a use case, that doesn’t mean anything if I can’t understand it, if I can’t translate it into something that a business person understands, as well as development team understands, that can turn into a reality. That’s an important aspect as well for an architect.
Michael Stiefel: Around those lines, before we get into security directly, because that’s clearly one of those things that you can’t write a use case for. Some people argue that we don’t have to think about architecture, that sometimes architecture magically emerges out of the development process. As a security architect and a threat modeler, do you have an opinion about that? Is there certain circumstances where that’s true and others where it’s not?
Securing the Network Is Not Securing the Application [09:14]
Robert Hurlbut: Yes, I do. I do. In fact, if I can give a little bit of background, I did switch or start to switch more and more towards security architecture about 20 years ago when I really just learned about application security. I already understood that network security was a thing. We already talked about firewalls, we talked about ways to protect the network.
Michael Stiefel: Incidentally, if I might just interject for a second, that’s a good example of something you can’t write in a use case. Firewalls don’t appear in use cases. Networks don’t necessarily appear in use cases.
Robert Hurlbut: Very true, very true. But I also noticed, and others did as well in the industry, we didn’t really talk a lot about the software itself and the security requirements around the software. It was more or less thought that the network and any firewall rules and things like that you might put in place, that’s going to take care of us, that’s going to protect us from the outside. But of course, what we do is we open ports, web pages, at least way back when we’re mostly on port 80, which is completely wide open, with data being set and so forth. But in terms of emergent architectures just from development, and do we need architects or not, and I know you have a very famous talk, we don’t need no stinking architects or something like that, if I remember correctly.
Michael Stiefel: Yes, yes.
Software Security Must Be Actively Pursued [10:38]
Robert Hurlbut: But especially within security, the idea that I will somehow fall into being secure, that as I develop and I develop and I develop, I will just somehow or another be secure. That goes back to that whole idea of relying on the network, relying on the perimeter for you so that you don’t have to think about these things. But reality is on the software side, there are decisions that you make. There are opportunities if you do not think ahead of how you’re developing your software that can lead you or leave you open with vulnerabilities, leave you open to potential attacks and compromise. As a result, you do have to step back and think about the design and think about being intentional with how you’re putting things together. That’s where architecture comes in.
Michael Stiefel: Actually, I would divide those things into two categories. There’s one category that says avoid a SQL injection attack. Those are sort of, tactical, developers can do them. How do you make sure that you hash passwords and all that stuff that is tactical security. Very often when you see people who talk about security, especially to developers, it’s this tactical security, but you are actually speaking at a much higher level of architectural security, system security, which does not fit into a tactical approach. But you actually need someone looking at the whole picture and deciding that, “Well, yes, this may make sense if you just consider the technology. But if you actually look at the way the real world works, that isn’t going to fly.”
This might be a good segue into what you see as these important issues in security. What is it that people who are developers need to understand? What are these things that architects need to understand, and what do you see as the major problems in security? I guess I put three big topics together in one, so you can answer them in any order that you want.
Robert Hurlbut: Okay, sure. Well, from a developer’s perspective, you’re right. There’s a lot of tactical recommendations to help a system be secure and to write what we call secure code. Meaning that you’re still thinking, developers thinking ahead about… Let’s just take SQL injection. How do I deal with that? Typically, as recommended, we use parameterized queries. That means if we take input from a user, we’re not just taking it as is, as a string and then sending it directly to the database or concatenating strings and sending that as a command to a database. That opens you up to a SQL injection attack. Instead, we recommend, as a tactical measure, to use parameterized queries, which is instead of concatenating strings and just taking whatever the user input is, instead to use parameterized queries.
That just simply means that we take those statements as essentially, if it’s a string, we recognize it as a string parameter. Then, it gets sent to the database, not as a command but just simply data. It doesn’t get executed, and that’s the key thing. We don’t want data to be executed as commands, instead of we want them as simply what they are, they’re parameters into a statement to the database to retrieve data and so forth. We give that kind of guidance. Developers have to think ahead when they’re writing out their code. This is the safe way to write code or the secure way to write code. That comes into play. For architects, there are some other things to think about. Larger things, if you will, in terms of approaches and common issues.
Authentication, authorization, that’s a particular set of things for identity and access management that can get completely wrong. Some will say, “Well, I’ve authenticated, and to me that’s the same as authorization.” I’ve identified who you are, but have I really determined what you can do? Authorization. Well, maybe not, and you can get that confused. You can get them out of order and so on. Those are some things in terms of a design approach, and thinking about how you build out your architecture to accommodate cryptography is another one. Really a big one. Certainly don’t roll your own. That’s what we always recommend. It takes years and years.
Michael Stiefel: Don’t build your own car.
Robert Hurlbut: Don’t build your own car. It takes years to get really good algorithms, I’m sorry, encryption algorithms and so forth to be able to, or hashing algorithms, to be able to do what we need to do for cryptography. You evaluate, what are the best ones out there? There’s lots and lots of testing.
Michael Stiefel: There’s decades of experience and testing.
Robert Hurlbut: Experience and testing, and it changes over years. One time, SHA-1, then SHA-2. Then, of course, MD5 is a long time ago for hashing. You definitely don’t want to do that, but you need to also keep up-to-date. Those are what architects need to do as well. Keep up-to-date with some of those kinds of advancements in terms of how are we making sure that we’re using the best algorithms and the best techniques to be able to store data and prevent it from being captured by others who shouldn’t have access. Those are some things that architects need to think about and continually evaluate and help them with their designs. There was a third one you mentioned. I forgot-
Michael Stiefel: Well, before we get to the third one, authorization to me is a particularly interesting example because you have to authorize and know how to authorize in different parts of the application. Because I could be doing something and trying to, depending on my credentials, I may or may not be allowed to run this report or see certain columns in a report. When you think about, I once had to tackle that issue, you may be able to run a report but can’t see certain columns out of the database, that could get quite sophisticated to do. There are other examples of this. These are things that developers may or may not know how to do or to deal with the security infrastructure, and how to do this.
This is a good example, I think, and you can tell me whether I’m right or wrong, of where there’s a play between the security architect and the application developer that the application developer may not be aware of.
Threat Modeling [17:20]
Robert Hurlbut: Yes, agree. Agree. That’s been my focus and why I have focused on over many years looking more and more at threat modeling. Threat model is a way of looking at a system, analyzing, typically a representation of a system, which means it could be description of the system, of what you’re doing. It could be a diagram that helps you understand what’s going on in the system. But essentially, trying to evaluate any particular security or privacy issues within that system that have some impact or could potentially have some impact on that application. Of course, it can impact your performance, other things as well, but especially the security, the integrity of that system.
Michael Stiefel: That’s an interesting point you just raised about how it impacts the performance, which again, shows why you need this, this is something that comes in the purview of an architect. Because somebody who writes something that’s performant just from a code point of view and doesn’t take… To encrypt or decrypt takes time. For example, if lots of packets are coming in and you have to encrypt or decrypt each one of them, either because they’re signed or they’re encrypted, that’s a performance impact and that has to be taken into consideration when you’re writing your algorithm or making your promises of what your uptime is or whatever it is. Those are examples of cross-cutting concerns that are just not a development issue.
Resiliency [18:45]
Robert Hurlbut: Yes, absolutely. Another common issue is denial of service attacks. How am I building my system to be resilient towards those types of attacks? Attacks could be, a denial of service attack could be external, but also could be internal. External, we like to think, well, I have a bunch of bots and maybe it’s a distributed denial of service attack where you have bots everywhere on machines that are trying to call services, trying to upload large files that you don’t expect put in. I’ve seen 200 character passwords into a form and just do that repeatedly for trying to log in and take your authentication system down if you can.
I’ve seen those kinds of things and that’s what we think of. But the other kind is internal, where services I rely on, databases, other APIs, external APIs that I rely on. Other things that I’m trying to-
Michael Stiefel: Credit card agencies.
Robert Hurlbut: Credit card agencies, exactly. Yes, how do I build that in for resiliency? Airlines are notorious for, and different companies that sell books and other kind of resources, are notorious for this where they figured out, do I just sit there and wait for the billing to happen? No, you take the order and then you go do the billing later, and then you verify that the credit card worked, and then you come back, and those kinds of things.
Michael Stiefel: Also, the business enters in there because it depends on, well, is this a million dollar order or order for five cents? The five cents, you might decide in the business case, let them have the order, 99% of the time people are honest. If it turns out to be dishonest, I’ve only lost five cents. On the other hand, if it’s a 10 million order coming from a company you’ve never heard of before, then that’s a different story. Again, these things are not cut and dried, and this requires interaction between the developer, the architect, the business. These are not simple things.
Security As a Business Requirement [20:47]
Robert Hurlbut: Absolutely. Yes, the business was the third. The business understanding that security is a business issue, we’re seeing that more and more these days. There was a time where security was thought to be a nice add-on or sort of, we’ll get to it when we get to it, but we’re in a hurry now to get this shipped out. In version two, maybe we’ll think about more security type of thing. You can’t do that anymore. It’s a first world citizen for most organizations now, it has to be. There are customers who rely on the security of organizations. How is my data stored? Are you protecting that data? What about financial transactions, are you protecting those? If you lose a lot of money, do I lose a lot of money as a customer? Those kinds of things.
We already talk about the business goals of performance and some resiliency, but it also relates to security as well. Security is a first world citizen as well in all the other things that we’re thinking about now, and it has to be for many organizations that it can’t be a byproduct. It can’t be an afterthought. That’s another aspect as well to think about.
Michael Stiefel: You were talking a little bit before about threat modeling, and that also raises the question now, what do you see as the key issues in security that developers need to be sensitive to, architects need to know, things that companies neglect or don’t think about? If you want to encompass that in threat modeling or whatever you see to be the appropriate way to raise that issue, just go ahead.
Looking at the Big Picture [22:34]
Robert Hurlbut: Well, yes, and I appreciate the callback to threat modeling. Where I was going with that earlier is that that’s a good way to communicate the concerns, security concerns to teams. Developers understand a bit about the bigger picture when they see a threat model of a system. They’re understanding that this is not just a particular place and code, but on the bigger picture, how do these various components interact, how these various systems interact, and what does that mean in terms of transferring data, saving data, moving data, authenticating against the data that’s being sent and so forth for the data that’s being sent. That’s where a threat model can help.
For architects, threat models, again, help them determine and understand requirements. A developer would like to see requirements, would like to see, here’s what I need to build. A threat model can help an architect think about those security requirements and turn those into something that is actionable for developers. Then, on the business side, the threat models also help, ideally the threat models align with and understand those business goals and priorities. For example, if we say what’s most important is protecting anywhere that we have open ports to the outside world. We need to consider our threat model there. Who are our customers and how are we authenticating those? How are we authorizing those calls that they make into our APIs, into any endpoint that we’re exposing, and what data are they sending?
What data are we sending out to them and so on. So that in terms of the business side, a threat model can help with that, in terms of aligning those goals, aligning those priorities, and being able to focus on, where do we spend our time, where do we spend our money and so on to be able to accomplish some of those goals?
Michael Stiefel: This is actually quite interesting. One of the things that occurs to me when I listen to this, and you did a little bit of this when you described threat modeling, but there are people who are going to listen to this podcast who are developers. One of the biggest problems developers have or complaints is that this is not concrete enough. In other words, to me, one of the great skills in being a developer is being very literal minded. A computer has to be told the simplest things to do. In other words, if you build a table, if you don’t screw in all the screws exactly right, the table is pretty secure, it’s okay. But if you don’t metaphorically tighten all the screws properly in the software application, you can have severe problems.
The problem I see that a lot of developers have when they listen, not so much the tactical security recommendations, but the strategic security implications, is that they’re not concrete enough. I don’t understand this. It seems to me, one of the roles of the architect and specifically the security architect, is to make this as concrete and as clear as possible to the developers so they’re not left with something they feel, “Oh, this is just sort of mushy stuff.”
Following the Unhappy Path [25:53]
Robert Hurlbut: Right, right. No, that’s a great point. Well, one of the things that I’ve done, and I can remember doing this as a developer for many years, is to use unit tests and test driven development to think about how does my code react to tests, testing for functionality. But one thing that we don’t always do as developers, I mean not every developer, but let’s say initially they don’t, is to think about the error cases.
Michael Stiefel: The unhappy path.
Robert Hurlbut: The unhappy path, thank you. The happy and the unhappy path. Yes, so the unhappy path. That’s a lot of what we’re doing in security, right? We are thinking about the unhappy path.
Michael Stiefel: Well, developers tend to be optimistic sort, I can do this, I can do that. The focus always is in the happy path, and you won’t be surprised, and I no longer am surprised, but people don’t even check error codes or don’t even put catch handlers and have fallback points in their application to build. It’s like they just expect the exception to be handled by the great exception handler in the sky without having any idea of what state the application is when they catch the exception.
Robert Hurlbut: Right, and so for concrete guidance for developers is to include those unhappy paths. What are the potential error conditions that you need to test for and validate that the code is able to handle those correctly? What do you expect if these happen? What’s the error that I should see? Whether it be stored in the log file or returned back to the user on a screen. What should we see? Those kinds of things, not just the happy path, but the unhappy path. That’s, again, where thinking through those security issues, those what could go wrong, that’s a question that we ask quite often in threat modeling, what could go wrong?
Not a common question for everybody when they’re thinking about the happy path, but when you think about the unhappy path, that really helps pinpoint what could go wrong here.
Application Recovery and Stability [28:05]
Michael Stiefel: Well, especially if you’re, for example, in the middle of a transaction and some of it has to be rolled back and some of it doesn’t. You also have to think about what the equilibrium or stable points are in the application. In other words, when you’ve handled the error, what’s the state of the data? What’s the state of the application? Can you go back? Let me pick a concrete example that’s sort of… Let’s say you are enrolling a new member and you have membership data, you have bank information, because let’s say they’re going to take some service that you charge for and you need all this information, but let’s say there’s an error in the application data.
Let’s say they didn’t put in, they left out some field, so do you throw everything away even though they got the account information for charges right, but they forgot some piece of information, you’re going to throw it all away and they can enter them again? Of course, all the database gurus will tell you, don’t have nulls in the database, but here’s an example of from the user perspective or even the security perspective, because you have sensitive information that you may be gathering. Do you just throw it all away, make the user do it again, or do you save what you can and then somehow have an application state that says, this person can’t go on until they enter this data?
Robert Hurlbut: Right, which are good decisions to make.
Michael Stiefel: Yes, and they’re different for everybody. They depend on the business, but part of the unhappy path is thinking about how unhappy are you.
Robert Hurlbut: Right. How unhappy would we be if this happened?
Michael Stiefel: Right.
Robert Hurlbut: Yes.
Michael Stiefel: And how do you recover?
Robert Hurlbut: And how do you recover? Those are some things, in terms of concrete for developer, concrete action items, those are some things that could be really helpful in detailing for developers, here’s what to look for, here’s how to find those unhappy paths, react to them, deal with them, come back later, as you mentioned. Maybe if you have some information missing, how do I validate that I have information missing, that I need to maybe go and request that again from the user and so forth. Those are kinds of things. Again, it also reflect the business as well.
Conscious Decision Making [30:25]
Michael Stiefel: Absolutely. In fact, the developer can’t make those decisions themselves. They need business constraints to make those decisions. What I like to tell people is, if you don’t give that guidance to a developer, well, when the developer writes the if-then clause, for example, at 2:00 AM in the morning, they’re going to make that decision de facto whether you like it or not.
Robert Hurlbut: Absolutely. What we like to say is, when we’re talking about threat modeling and when I do training or just talking about in general with a team, is we always say, we’re all threat modeling. Maybe it’s new to everyone, but the reality is we’re always threat modeling. We’re always making decisions in our head. We’re thinking about, “Okay, here’s what I’m going to do. But what if that doesn’t work?” We do that all the time. We go to cross the street, we look around both ways before we stepped off the curb.
Michael Stiefel: If we don’t look both ways, that’s a decision too.
Robert Hurlbut: That’s a decision too, absolutely. We’re making decisions all the time, and certainly developers are making decisions as they write code, and so we’re already doing that. But the idea is that if we present that as a technique, but in some cases, like I said, I don’t want to just simply make that decision for a developer, I need to know that’s what I need to put in because that’s based on our business rules, based on our requirements and so forth. That’s what is going to really help.
Michael Stiefel: People have to understand that not to decide is to decide.
Robert Hurlbut: Is a decision. Absolutely.
Michael Stiefel: What you’re essentially saying, it’s better to make these decisions consciously, and if necessarily painfully, so you’re aware of them. What I always like to say to people is, you don’t want to wake up one morning and find out that your business is on the front page of the Wall Street Journal because you had some security breach.
Robert Hurlbut: Absolutely.
Michael Stiefel: That’s what we used to call a career limiting move.
Evaluating Risk [32:25]
Robert Hurlbut: Well, and that’s the value of security analysis, threat modeling, another word for it, secure architecture review, is doing that work so that you are aware. Because like you said, the worst thing is not being aware. If you’re aware, at least at that point, you can make some decisions regarding risk. We haven’t talked about that yet.
Michael Stiefel: Go ahead. Why don’t you talk a little bit about risk? Because it is important.
Robert Hurlbut: Sure, sure. Absolutely important. We’ve talked a little bit about vulnerabilities indirectly. SQL injection is a vulnerability, usually based on a code error. Just doing something either intentionally or unintentionally, and it results in a vulnerability, which is basically a way for an attacker or a threat actor to be able to compromise the system using that particular vulnerability. The threat is, the result of that threat or that vulnerability rather being exploited, so the SQL injection example as a vulnerability, the threat would be somebody could use that to retrieve data, change data in the database. Maybe inherently it has more authority than the typical user should have. As a result, they can maybe drop the database.
I’ve seen that before in the middle of a demo, not so great. They could do that, so all kinds of potential threats. The risk comes into play when you become aware of those threats and then you evaluate those threats. What’s the likelihood of that threat happening? Some threats are really difficult to do where you can say, “Well, if we had those vulnerabilities, maybe we can get access to the database, but maybe we have limited access to that database. Maybe the user that’s calling the database is not an admin user, which we hope, but just a regular user. It only has access to that one database, that one table, that one whatever, and so the threat is limited.
But maybe that database is, for whatever reason, using an admin account to make the calls. Well, now you’ve got all the privileges and so your likelihood, and the other is the impact of that threat. First is the likelihood of that threat, and the second is the impact. If that threat was realized, if somebody was able to get to our database and be able to exfiltrate all the data in my database, what’s the result? What could happen? Is that bad? Does that put us in the Wall Street Journal the next day, because everybody finds out we had a big data breach? That’s where your risk comes in. That’s what we usually say, is that combination of the likelihood and the ease of exploitation of that threat as well as the impact of that threat, if both are relatively high, we got a high risk.
If both are relatively low, we call it a low risk. Then, you evaluate in between, low to high, what are they? To get an idea of, if we don’t fix this, if we don’t, as we identify these threats, if we don’t fix them, if we don’t mitigate them, are we willing to accept that risk and the results of it losing some money, losing some reputation, or having a reputation impacted, or maybe transfer the risk? We just simply give a message. One common one I see is going into a coffee shop and it says, “We have open Wi-Fi here.” Why do they tell you that? There’s no password on the Wi-Fi? Well, you said, well, that’s ease of use, right? But the reality is, that’s transferring the risk to you.
You’re responsible if you connect to something that’s very sensitive, and so that’s just transferring the risk to you. Then, other coffee shops will give you a password and provide it and so forth, and try to minimize the risk. The other, of course, is once you understand those risks, then you mitigate, and so then it minimizes the risk. But risk comes into play, just understanding what the threats are, understanding how likely these threats could be exploited and the impact of those threats. Then, that helps you then determine your next actions once you understand some of those risks.
Look at System Vulnerabilities, Not Scenarios [36:27]
Michael Stiefel: Something that you said raises something that I have thought about often in terms of threat modeling. Thinking about what the outside world could do versus what are the weaknesses of your system, it seems to me that if you try to think, and again, it depends on obviously your experience in your business, of what kind of outside actors and what could they do and what could be the scenarios? This is a huge number of them. But on the other hand, if you think of, as you were talking about before, about how vulnerable is our database? In some case, you don’t care whether it’s the Chinese government or some mobster trying to get in or some teenage hacker. You don’t think of those scenarios.
You think of in terms of, what are the weaknesses of the database? What are the weaknesses of our authorization system? Focus on the internal weaknesses in your threat model as opposed to postulating what might happen. Because after all, it’s the risks that you don’t think of that really will get you. It’s like the Secretary of Defense Rumsfeld used to say, there are the known knowns and there are the known unknowns, which a lot of what threat modeling is all about, but the unknown unknowns is what really is the problem. To me, one of the ways of getting at those unknown unknowns is to think of what the weaknesses of your system are as opposed to trying to think about what someone on the outside might do.
Robert Hurlbut: Absolutely. Another thing to consider in that regard is sometimes we’ll spend, like you just said, a lot of time on beefing up our authentication protocols and process and so forth, which we should, don’t get me wrong, that’s important. But for services that say, all I have to do is, or for anybody to do to get into the system is they click on this link, they add themselves as a new user of the system, and now they have a login, guess what? Anybody can do that. Not just your customers, but also your attackers. Now, they have the same privileges as a regular user who’s doing what you hope is a good thing, and the attacker who has very malicious intent. Now, it’s beyond your authentication. They’re all treated the same.
With that in mind, you don’t know who the attacker is, if it’s this regular user or if it’s an attacker. But then like you said, you have to think about, how do I protect the system? What are the issues, security issues within my system that is not considering necessarily which user is what? We just need to make sure that we’re protecting the system and understanding those security issues and resolving them for that very reason that you mentioned. We may or may never know, is this an insider, an outsider that’s now become like an insider and going through the system? We don’t know. But what we can know and to help us with the unknowns is, like you said, to focus on those issues.
Do Not Forget the End User Has a Job To Do [39:37]
Michael Stiefel: One last thing that I’d like to bring up before we get to the questions that I like to ask my guests is that sometimes people have to think about the advice they give to their end users as well as any development. One of my favorite pet peeves is the advice that people, I’m not talking about banks or financial institutions, because you have to understand the difference between internal and external attacks. But when people tell me I should change my password every 30 days, well, to me that’s ridiculous. Because the only time that that will help you is if your password happens to be stolen in the small interval between when you change your password and they attack. But you have the other 29 days, 23 hours and 55 minutes of vulnerability.
That makes no sense to me. Now, again, in the bank, from an internal perspective, changing your password may be important because you want to make sure people who leave the bank… I’m trying to make this context specific, but sometimes people have this very blasé attitude towards security because they don’t think of the convenience of the user and making it difficult for them. Instead of making people memorize gobbledygook passwords as opposed to long passphrases, which accomplish the same, you have to also think of not only the internals of the system, but users who will try to circumvent the security because they want to get their job done.
Robert Hurlbut: Right, right. No, good points. It’s an interesting challenge for users and end users to help them understand some security basics, but also allow them to get their job done. Ultimately, that’s another business goal. We want them to be able to do their work or do what they’re doing because we want them to be successful. It reflects on us and so forth. But in terms of guidance in general, I know NIST, for example, which is a government agency that typically makes recommendations for security requirements and so forth a few years ago said, “Yes, no need to keep changing passwords all the time.” It doesn’t really serve a good purpose like you just mentioned. It’s better to have a better password, phrases, and so forth.
Or, for end users, we can recommend password managers and there are some good tools out there for that to help them if they need to have a different password. The most common issue, of course, is everybody reusing the same password. If those get pulled out of a data breach and then used by attackers to continue to try to log into all kinds of systems, because they figure, “Well, that person probably used it elsewhere, we’ll try it everywhere.” We’re adding numbers, let’s just keep adding a number every time when we have to be forced to change the password, and that doesn’t make it secure.
Michael Stiefel: Right, but people often do that because they’re told to change their password every 15 days.
Robert Hurlbut: Right, instead of helped with other tools like password managers and so forth, and passphrases and things like that.
Michael Stiefel: Well, that actually raises an interesting question, that’s going to get me a little far afield, but how much of these security attacks are automated and how much is somebody, a human looking at the data? Forget about AI now, let’s not go there, using artificial intelligence for attacks, because I’m sure they’re already thinking about that, if they haven’t already done it.
Robert Hurlbut: Of course.
Michael Stiefel: Because big data analysis seem just right. You harvest a whole bunch of usernames, passwords, security credentials, past phrases, and you just machine learning. I’m sure governments have started to think about automated attacks on each other based on big data. But anyway, leave that aside. Something that you and I would have to worry about is if our password is stolen, let’s say, which I’m sure because… or important data that has been stolen, because we know the credit card company, not credit card companies, but the credit scorers websites have been attacked. The information’s out there. I’m sure my Social Security number is public knowledge on the dark somewhere because it’s been stolen.
Do we assume that someone, unless you are a public figure and somebody wants to go after you, I presume most of these retries of these shared passwords are automatic. It’s not someone’s looking at Robert or Michael and saying, “Well, this is the pattern and let me figure this out.” It’s just automated.
Robert Hurlbut: It’s automated. Yes. Lots and lots of bots that are doing this over and over again. One of the recommendations is for organizations to look at common password lists that may have the top 25,000 or 100,000 or more list of passwords. Whenever a user, end user sets up their login, they would put in their password and then compare it to that list and say, “Sorry, but you can’t use that because it’s part of our common compromised password list. Try again.”
Michael Stiefel: I presume they’re comparing them on a hash and encrypted basis?
Robert Hurlbut: Of course, right. Of course, of course. But the point is that can help with the end user, it helps the end user to not just put password one, or 12345, or something like that.
Michael Stiefel: Or, you can’t use one of your last 10 passwords that you’ve… Yes. Is there anything else that you think is important to bring up that we haven’t talked about that you can think of?
Robert Hurlbut: No. I think I’ve stressed this before, but just to highlight it again, is the importance of spending that time taking a look at the system, not so much as an attacker, but more as, I have these things I need to defend. I need to determine or understand where are there security issues within my system. It helps you then determine your risk. What can I fix today? What am I probably not going to fix because the risk is low? Because it’s better to know than not know. I always recommend take some time, take a step back, look at your system, think about the threat model of some of the things that you’re doing, the various business decisions that you’re making, various activities, and so forth.
At least you’ll have that, because if you don’t, it’s worse. It’s better just to take a moment and review and understand, and that will pay dividends in the long run.
The Architect’s Questionnaire [46:17]
Michael Stiefel: Now, I get to the part of the podcast where I like to ask the questions, I’ll ask all the architects, but it gives me insight into why people become architects, what they like about architects. It’s not that I’m going to build any big data model of all the responses I get, but nonetheless, I think it’s important for people to understand what makes an architect. What’s your favorite part of being an architect?
Robert Hurlbut: For me, it is seeing that mixture of business, technology, of… Well, we like to sometimes go back to people, tools, and technology, or process. It’s that combination of working with people, working with the business, working with the technology, and putting those together to build interesting designs, secure designs, of course, that’s my main focus, but designs that really help us with the business goals, helps people to be able to do the work they’re doing. That’s really, in terms of being an architect, seeing all those things come together.
Michael Stiefel: What is your least favorite part of being an architect?
Robert Hurlbut: This one is a tough one. As a security architect, I probably would say more so than an application architect years ago, as a security architect is the challenge of getting folks to recognize the importance, again, of security. Even though I say everybody’s talking about it, there are still holdouts, if you will, that say, security, we don’t need that. We’ll do it later. It’ll come out in the wash eventually. The reality is, especially we talked about authorization, you cannot bolt that on. That’s probably my least, is just still getting pushback occasionally on the need for security.
Michael Stiefel: Interesting. Is there anything creatively, spiritually, or emotionally about architecture or being an architect?
Robert Hurlbut: Well, actually, it goes back to my first one, but just the seeing something being built that was designed, seeing something that I’ve been a part of, or I’ve worked with thousands and thousands of teams, especially as a security architect, helping lots and lots of teams think about threat models. Seeing teams incorporate some of those techniques and ideas and taking a system that did well, but doing even better and improving in maybe even small ways, but there’s improvement and more secure or better resiliency, better performance, and so on. Seeing those I think definitely is very fulfilling. There’s some creative aspects to it too, but it’s just that satisfying to see that.
Michael Stiefel: What turns you off about architecture or being an architect?
Robert Hurlbut: Not as much as it used to be, but sometimes long hours. Sometimes it can be in terms of, especially as you’re designing and thinking through some really tough problems, a lot of things we’ve already solved, which is great. You can go back and review what are some patterns that are already out there to help us solve some problems, and that really helps. But then, there are some that say, well, this seems new, or this seems a little different. Taking the time and working through that can be a little challenging. It’s not that I don’t like it, but it certainly can be challenging. It can be time-consuming to work through some new things, especially if you’re under a time crunch, which most of us are. Especially in many development teams and projects. That can be an interesting part of not being the most fun part.
Michael Stiefel: Not that you would prescribe them when they’re not appropriate, but do you have any favorite technologies that you just love to work with?
Robert Hurlbut: Well, of course, as a developer, I always liked, and developer/architect, I liked IDEs on the Microsoft side. I’ve done C, C++, C#, .NET. I’ve also done some Java, so the various IDEs that came out. Any of those that help me build out some of the things that I’m developing but may also incorporate some, can I figure out what my components I’m building? Can I diagram them? Can I take a look at it? Does that tool help me with that? Some IDEs do, but apart from that, I think drawing tools have been really, certainly in the last number of years, good drawing tools. I don’t have any particular that I can mention exactly, but just good drawing tools that help you visualize a system that you’re building or thinking about.
Being able to draw those out, whether it’s UML or data flow diagrams or some kind of flow diagram. Those are the things I really love, is helping to put ideas to a diagram that I can then use that to communicate with other people.
Michael Stiefel: What about architecture do you love?
Robert Hurlbut: I think the thing I love most about it is it’s a foundation. When I was a developer, we’re building things up from just a few lines of code, and then it becomes something else, it becomes something else. Now, I have many, many classes, many lines of code and all that sort of thing. But ultimately, it sits on a foundation, a design of some sort, and that’s what I love. It’s just seeing that foundation that all of these things rely on and the importance of it. That’s what I think I enjoyed the most and love about it, is just it provides that good foundation and framework for all the things that we’re talking about and doing and making decisions about and so forth.
Michael Stiefel: Conversely, what about architecture do you hate?
Robert Hurlbut: Hate is such a strong word.
Michael Stiefel: Well, that’s why I picked it.
Robert Hurlbut: I know. I think the thing I hate the most is brittle architecture, because you could also, just like anything else, when you’re building a foundation for a house, depending on if you put a good foundation in, it helps for a solid house. If you put a bad foundation or no foundation or all you’re relying on is emergent foundation or architecture, if you have not put any thought to your architecture, you still have an architecture, but it’s brittle. That’s the thing I hate, is it will just happen, it will just happen. That to me is a mistake and that could be detrimental, especially as you move along and rely on some aspects of your design, of your architecture or emergent architecture.
Then, find out you have failures that are not being handled correctly and that’s causing all kinds of issues later on, and it’s harder to go back and fix that retroactively, very, very difficult.
Michael Stiefel: What profession, other than being an architect, would you like to attempt?
Robert Hurlbut: Well, as you mentioned at the very beginning, I am right now working on a PhD. It’s in space cybersecurity. Actually, that’s the next area of interest in terms of space infrastructure and architecture of systems and space systems and so forth. I’m looking at potential academia, looking at how I can help in other industries. I think still some aspects of architecture, but to be determined where that will go and where that will take me. But that’s, I think, the next thing that I’m looking at that may or may not be related directly to architecture.
Michael Stiefel: Do you ever see yourself as not being an architect anymore?
Robert Hurlbut: I don’t really think so. I think I will always have some aspect of… It’s hard to, once you’re in this a number of years, it’s hard to see the system as not as an architect and understanding how things work and foundations and so forth. Yes, difficult for me to see that outside of what I’m doing now, that I won’t be doing that for years and years to come.
Michael Stiefel: When a project is done, what do you like to hear from the clients or from your team?
Robert Hurlbut: That this helped us make a difference. That the work that we’ve done building out a good foundation, good architecture, secure architecture, resilient architecture, that this has made a difference. That’s what I like to hear. That’s what I like to see, and for teams to have that, hopefully, that feeling that we feel pretty good about what we built, really good about what we built and would like to do more of it.
Mentioned:
.
From this page you also have access to our recorded show notes. They all have clickable links that will take you directly to that part of the audio.
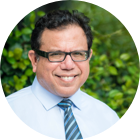
MMS • Shreya Rajpal
Article originally posted on InfoQ. Visit InfoQ
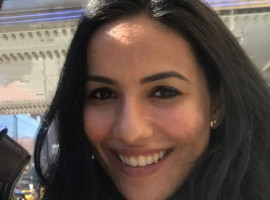
Transcript
Rajpal: I’m going to be talking about guardrails for enterprise applications and breaking down the problem of reliability, safety, consistency for large language models. My name is Shreya. I am currently the CEO and co-founder of Guardrails AI. I’ve spent about a decade in machine learning. I started out doing research and publishing papers of top-end use in classical AI, deep learning. I then spent a number of years in self-driving cars working in everything in machine learning and self-driving cars, including sensors, perception, decision making. I really enjoyed that job, but then left to join an MLOps company based here in the Bay Area as their first hire, and led the ML infra team while I was there. I started Guardrails AI, which is a company I now lead.
AI Evolution
This is why we care about this, in the last year or so we’ve seen a Cambrian explosion of applications in AI. There are a lot of really interesting applications that we see, Auto-GPT and completely fully agentic workflows are something that we’ve seen. In addition to that, we’ve also seen very specific applications in high value use cases, so mental illness, medicine, law, sales. This is one of my favorite tweets that captures some of the early sentiment around what LLMs and AI will do for us, which is like, software engineering as we know it is dead, AI can code better than us. We can see interest in AI over time, really expanding. If you look at the timeline here, somewhere around this point is the ChatGPT release, and immediately interest in AI skyrocketed. Suddenly, everybody who was not related to software, like my mom was talking about artificial intelligence, and for the first time got what work I did. It’s really nice to see everybody’s imaginations sparked with what we’ll be able to build with AI systems.
The reality is a little bit different. As you work with AI applications, you come across many issues with them that lead to, at the end of the day, reduced value from what you’re able to get out of using AI. I think this was a case that got a lot of attention, because this was a very real-world implication of somebody overly relying on AI systems when they aren’t at a place where they’re very reliable. A lawyer used ChatGPT in court, and cited fake cases, and is commonly now known as the ChatGPT lawyer. I think the quality of answers on Stack Overflow, for example, has gone down, a bunch of other use cases. I’ve quoted some figures from an article which was published by Sequoia Capital, about the state of the world in generative AI. If you look at path to 100 million users, ChatGPT is one of the fastest climbing applications that got to 100 million users. Then if you actually look at like one month retention, or daily active users of those applications, generative AI applications tend to have much fewer retention. I haven’t included the DAU graph here, but go check it out at this link, https://www.sequoiacap.com/article/generative-ai-act-two/. Much fewer compared to traditional applications that have also seen that meteoric rise. This is a very interesting trend. It points to the case that right now out of generative AI, people aren’t getting the value that you really need to be able to get from other software systems that you used to.
Why is this the case? A common symptom that we see with people is like, my application completely worked in prototyping, it never failed. The moment I handed it off to someone else, it started erroring out, or my API stopped working. I think the root cause of the symptom is that machine learning is fundamentally non-deterministic. This is not a bug but a feature. If machine learning was fully deterministic, it wouldn’t have the creativity that all of us have come to rely on for ChatGPT, other generative AI use cases. It wouldn’t adapt so well or it wouldn’t be as flexible as it is for a lot of applications. A lot of the goal here is like, how do we work with something that is still really useful but works differently than traditional software that we’ve been used to. I’ve outlined that problem here, which is that any software API, when you build with it, that software API is going to be deterministic. Maybe that service might be down sometimes, but any query that you send it, or any response that you send it is going to give you the same output response, time after time. As a result of this property, we’re able to build really complex software systems that chain together multiple APIs, and all depend on each other.
Machine learning is different. Even if availability or uptime might be high for machine learning models, fundamentally, you’re going to end up getting different responses from a machine learning model every single time you use it. For example, let’s say you are building a generative AI application. You read all of the best guides on how to do prompt engineering, you end up constructing the best prompt for yourself. You’re like, ok, I have this problem solved, now this prompt works. Every time you run that prompt in production, even without changing anything, you’re going to end up seeing different responses. It doesn’t mean that the ChatGPT or OpenAPI API is down, it just means that the model fundamentally responds to the same input differently. As a result, a lot of the applications that people are building using generative AI still are marrying traditional software systems. You chain together multiple APIs, you try to get the LLM to reason and that output is sent to another LLM, chaining is a very common abstraction that has gotten a lot of popularity. This fundamentally breaks down and doesn’t work the moment you factor into account how common it is for AI model APIs to break down or just generate outputs that are not in line with what you expect them to be or what you tested them out to be.
Getting ‘Correct’ Outputs Always is Hard
Summarizing the problem, is that getting correct outputs always is hard. This is a fundamental assumption that we take for granted with traditional software systems. Some common issues of incorrectness that you end up seeing in applications are hallucinations, falsehoods, lack of correct structure. For example, a lot of people use ML APIs to generate JSON payloads that then you can use to query a tool or something. Those JSON payloads are often structured in weird ways. This is all on the output side. Even on the input side, you might be susceptible to prompt injection attacks, which is essentially a framework where someone can cause you to reveal your prompt, which might be your secret sauce, something that you don’t actually want to reveal. By, in a very oversimplified way, like asking the LLM nicely, but often requires a lot of hacking around the limits of what the LLM response will do and won’t do. You basically end up seeing the developer’s prompt which you can use maliciously. To further complicate the problem, the only tool that is available to any developer that is working with a generative AI system is the prompt. This is a very novel world where you can’t really code your way out of these problems, you often have to once again, write a bunch of English and figure out how different models respond to the same English prompt.
All of this combines together into a situation where the use of LLMs is limited, wherever correctness is critical. For example, one of the most common, most successful applications of LLMs that we see is GitHub Copilot. I use it in my workflows every day. Most of the folks at my company use it. If you look at like, what makes it tick, it’s that the LLM output is generated, and if it’s wrong, you just brush it off, move on. Only if it’s correct do you accept that output, and then actually incorporate it. This has limited value. This makes it so that you can’t really use LLMs in healthcare applications, or in banking, or corporate or enterprise applications where there’s a substantial cost to the LLM being incorrect. This is a problem that I personally find very fascinating. Not just because it’s like, you have this fascinating technology on your hands, how do you make it work with how we build software today? Also, because this is a pattern that we see a lot in self-driving cars, for example, where ML models are again stochastic at the end of the day, but they need to work in a real-world scenario where there’s a substantial cost to that ML model being wrong.
Guardrails AI (Safety Firewall Around LLMs)
The problem here that I’m focused on, and Guardrails AI the open source is focused on, is, how do we add correctness guarantees to LLMs? This is a screenshot of the open source. This is the entire problem that the open source focuses on. I’m going to dig deeper into how architecture, we situate ourselves into a part of the stack that makes it so that we’re able to assess these problems. Guardrails AI acts as a safety firewall around your LLMs. This is a high-level system overview. If you look at how traditional AI applications are built, you look at, there’s some AI application. Within that AI application, you have a component, which is your LLM logic. That LLM logic, basically, there’s an input that is a prompt. That prompt gets sent to your LLM API. That LLM API returns an output, and that output flows back into your application. How Guardrails AI is different is that we essentially advocate for using a verification suite, in addition to any LLM API that you use. This sits something like a sidecar in addition to your LLM API, and is essentially acting as a safety firewall around your LLM, and making sure that all of the functional risks and guarantees that you care about are all accounted for. Now, instead of sending your output back directly to your application logic, you would first pass that output to your verification suite. This verification suite would have a bunch of independently implemented guardrails. These guardrails all look for different risk areas, different safety concerns within your system. Let’s say one of these safety concerns could be making sure that there is no personally identifying information in your output. If you don’t want to send any PII that is accidentally generated by the LLM to your user, this can be something that filters that. Making sure that there is no profanity in your system, especially if this is a customer facing application.
Let’s say you’re also building a commercial application. For example, you are McDonald’s, and you want to build a chatbot for taking orders via chat, even if a customer asks you about Burger King, you never actually want to be talking about Burger King. It also extends to non-English output. For example, let’s say you’re generating code with an LLM, there’s a lot of code generation applications that large language models are really good at. You might want to make sure that any code that is generated is executable and correct for your development environment. Finally, let’s say you’re using LLMs to summarize an email, or summarize really long internal design documents, so you can make sure that the summary is actually grounded in the reality of what that document is, and is correct, and is not hallucinating any outputs. Now your workflow looks like, instead of passing that output directly to your end user, the verification suite independently passes and fails. You can set policies on how much you care about each single guardrail. Maybe mentioning competitors is not as bad for you, but profanity is really bad. That is one of those things that you can configure and make sure that is handled appropriately. Only if you pass validation do you send this out into your application logic. If you fail validation, Guardrails uses a very unique and novel capability off large language models, which is their ability to self-heal. Essentially, if you provide them enough context about why they’re wrong, more often than not, LLMs have the ability to correct themselves and incorporate your feedback. In this case, Guardrails will automatically construct a new prompt for you that talks to the LLM in a way so that it’s more likely to correct itself. This whole loop carries over again until you pass verification or until your policies give out and you are out of budget.
Why Not Use Prompt Engineering or a Better Model?
Why not use prompt engineering or a better model? First of all, an alternative to using this safety firewall is directly trying to control the LLM output with a prompt. As we discussed before, LLMs are sarcastic. The same input, even if you have the best prompt available to you does not guarantee the same output. Finally, prompts don’t offer any guarantees, because LLMs don’t always follow instructions. Anecdotally, a lot of people that I talk to and work with, they go to these extremes of like, ok, something really bad will happen, like a man will die if you don’t give your output in this certain fashion, which is not a very sustainable way of building good, reliable software. I think the alternative is controlling the LLM output with a better model directly. The first is if you want to fine-tune a model, and this allows you to really build a better foundation for your application. The issue still remains that it’s expensive and time consuming to train and fine-tune a model on any custom data. My entire background in machine learning, I’ve spent on training or fine-tuning models or building infrastructure to train and fine-tune models. A lot of the explosion that we’re seeing with applications is because of how easy it is to now not have to do that, not have to build a dataset that works for your problem, not have to manage the training infrastructure, but instead just call an API and get the right output. Doing this, to some degree, limits the usability of generative AI applications. Alternatively, if you’re using commercial APIs, so for example, you rely on OpenAI GPT-4, like newer GPT releases, I think the issue there ends up being that you as a developer don’t have any control over model version updates. As someone who works with these models every day, one of the things that happens is that even without official model version releases, under the hood, the models are updated constantly. Once again, if you have a prompt that you think will work really well for one specific model, and you’re just trying to use it for the next better model, often the model will change out from under you, and those same prompts will stop working.
What Guardrails AI Does
What Guardrails AI does, in this framework of building more reliable generative AI applications. It’s a fully open source library that, first of all, offers a framework for creating these custom validations that we talked about in the previous slide. The second is that it offers the orchestration of prompting and verification and re-prompting or any other failure handling policies that you’ve implemented. It is a library and a catalog of many commonly used validators that you might need across your use cases. We’re going to dig into a few of them later. Finally, it’s a specification language for communicating requirements to LLMs, so that you’re priming the LLM to be more correct than it ordinarily would, by taking some of the prompt engineering pain away from you. How does each guardrail actually work under the hood? We talked about PII removal. We talked about executable code. Guardrails by themselves really are any executable program that you can use for making sure that your output works for you. One common thing that we see here is like grounding via any external system. For example, if you want to make sure that your code is executable, or if you generate a SQL query for some natural language question, making sure that that SQL works for you by hooking it up to your database or a sandbox of your database, and making sure that there’s no syntax errors, the output that you get is actually output that works for you. You can use rules-based heuristics for making sure that your LLM output is correct for you. For example, let’s say you’re using your LLM to perform data extraction from a PDF. You read the PDF and you generate unstructured data. You can make sure that any unstructured data you generate follows rules that you expect from that system. Interest rates must always be followed by a percentage sign. Dollar values must always be between x and y ranges. You can use traditional machine learning methods. For example, you can use embedding classifiers, decision trees, K-Nearest Neighbors classifiers, to make sure that any output that you get is correct for some constraints. Let’s say you have a previous treasure trove of data that you know is correct. You can use more traditional ML methods to make sure that the new data that you extract is similar to the data that you know is correct. You can use some high precision deep learning classifiers. This essentially entails making sure that you’re not using GPT scale models, but you’re still using a model that you can reliably host and serve. That can act as a watchdog to make sure that your output is correct and doesn’t violate any concerns. Finally, you can use the LLM as a self-reflection tool. Making sure that you ask the LLM to examine the response that you got, examine the correctness criteria that you specified, and make sure that the response respects that correctness criteria.
I also talked about handling invalid outputs, and I talked about re-asking a little bit. Guardrails, the open source framework, offers a bunch of policies that you can configure on how you want to handle your validators failing on your verification suite. For example, let’s say this is the response that you end up getting, let’s say you’re extracting a JSON with two values, one is transaction fee, and one is the value of the transaction fee, which is 5, but you have some guardrail, which is a very easy rule-based guardrail here, which essentially always inline checks that the value is less than 2.5. You can handle this using a bunch of different policies that you configure. We talked about re-asking where there’s a new prompt that Guardrails construct for you. For example, you’re an assistant, correct your previous response, which is incorrect because of XYZ reasons. Then, finally, send that response over to the LLM, and end up getting the new response, which in this case is transaction fee and 1.5. You can programmatically fix any output. This isn’t always possible, especially for a lot of the more complex, more sophisticated guardrails that we have. In this case, for simple rules-based guardrails, you can often just programmatically fix this to, for in this case, like the maximum value of your threshold. You can filter any incorrect or offending values. In this case, you don’t throw away the entire LLM response, you essentially make sure that you’re only getting rid of the incorrect value of 5. This also works for string outputs. Let’s say you have a large paragraph, and some sentences within that paragraph are hallucinated, or incorrect, or contain profanity, or whatever. In that case, instead of throwing away the entire paragraph, you can basically just remove those few sentences that are incorrect, make sure the fluency of your overall passage isn’t affected, and generate a new response. You can respond with a canned policy, or a statement about why your response is incorrect. For example, “Sorry, no appropriate response possible.” This is more appropriate for using when you essentially make sure that your cost of getting some requirement wrong, is so substantial that not answering the customer’s question is actually a better compromise, in this case. This is one of my favorite ones, which is noop. You pass the incorrect output as is to your customer. This is when this isn’t a very sensitive requirement for you. At the same time, every single guardrail that fails is logged for you. In this case, what you end up getting is very rich metadata about every single response that is served for you in runtime, and everything that’s right or wrong about that response. You can use this to build better systems, train a better model. We talked about how hard it is to create a dataset for model training. You can use that for creating your model training dataset. Finally, you can also raise an exception, in which case you can handle that in your code or programmatically.
Example: Internal Chatbot with ‘Correct’ Responses
I want to now walk through some applications of, let’s say, you’re building an internal chatbot and you care about getting correct responses from that chatbot every time you use it. Let’s say you have a mobile application and you want a chatbot that is able to look at the help center articles of your application, and that anybody can come in ask an English language question, and then you answer that question. This is often referred to as the Hello World of generative AI applications which is a chat your data app. Then the correctness criteria that you care about, first of all, don’t hallucinate. Second is, don’t use any foul language. This is table stakes, like don’t swear at your customer, basically. Finally, don’t mention any competitors. If somebody asks you like, who is the best burger joint in town, don’t say Burger King when you’re McDonald’s. In this case, we basically talk about implementing three different guardrails to make sure that this problem works for us. Essentially, we solve this problem by implementing provenance guardrails, which contain checking for embedding similarity with any code that you have. This essentially ensures that any output that is generated is similar to your source content. Making sure that any output that you have is classified to be correct using some NLI or natural language inference model. Finally, using LLM self-reflection.
How Do You Prevent Hallucinations?
We listed out this criteria of, how do you prevent hallucinations? This is a pretty complex problem, is a very active area of research at this time. Often the question is like, ok, I can’t really control my model, how do I actually in practice correct hallucinations? Add guardrails. We have this thing that we like to call provenance guardrails, where provenance guardrails, essentially, make sure that every single LLM utterance that is generated has some provenance in the source of truth that it came from. These guardrails are active and available for you to use today in the open source. Why this ends up being a problem is that these models are often so powerful and trained on the entire internet that even when you give them, “Ok, these are my help center articles. Make sure that you don’t respond with anything that isn’t in the help center articles.” The model will often quote things from there, but then use its general knowledge, or quote something that it has memorized from the internet. In spite of creating retrieval augmented generation applications that try to constrain the outputs of the model, you still end up getting hallucinations in practice. Provenance guardrails act as a way to do inline hallucination detection and mitigation while you’re running and building an application.
Example: Getting Responses with ‘Correct’ Responses
Let’s see what this looks like in practice as you’re building something. By now we’re familiar with our diagram that we went over earlier. I think the only difference is that this time around, our verification suite consists of provenance, profanity, and peer institution. Making sure that every utterance has some source and isn’t hallucinated. Making sure that there’s absolutely no profanity in your output. Making sure that there’s no reference to peer institutions. As you can imagine, all of these are complex problems by themselves. It’s almost as hard to solve for these safety issues as it is to build an application. All of these are guardrails that are available for you in the open source today, because we’ve done the hard work of doing the machine learning and solving for these ML problems in the open source. Let’s say you have the system and a user comes in with a question of, how do I change my password? If you’re familiar with building AI applications, what this turns into is a prompt, where you’re force going to prime the LLM by saying something like, you’re a knowledgeable customer service representative. Somebody has a question of like, how do I change my password? Make sure you answer this question to the best of your knowledge. Then you give it a list of articles that it is ok to answer from. You’re going to be like, in my help center articles, these are the articles that might be relevant. As an LLM, reason over these articles and make sure that the output that you get answers the customer’s question to the best of your ability.
Let’s say, the initial output that you end up getting looks something like this, where you have some raw LLM output that says, log into your account, go to user settings by clicking on the top left corner, and click, Change Password. This is a toy example. Essentially, settings aren’t at the top left corner of your application. This is somewhat obfuscated, but this is an actual real example of someone that I was working with that was using Guardrails for their use case where the chatbot would hallucinate, where certain components of your application should be, even though it has no ability to visually look at your application. These types of hallucinations often end up being pretty common, and end up misleading your customer or don’t end up giving useful responses to your customers. When this output passes through your verification systems, yes, there’s no profanity in it. There’s also no reference to any peer institution or to a competitor of yours. Your provenance guardrail would fail because that utterance does not have any bearing in the source of truth or in the articles that you provided your LLM. In this case, because we said re-asking as our error correction policy for provenance, a re-ask prompt is automatically generated where a simplified version of that re-ask prompt looks something like this. You talk about specifically what was wrong in your response, what the error looked like, as well as the newer instructions. In this toy example, we end up getting the correct response, which is basically, click on the menu to go to settings. This time around your verification passes and you’re able to route that output out to your LLM.
Complex Workflows with Guardrails
This is what happens on a one-shot workflow where you have a single LLM call, and you want to safeguard that LLM call with guardrails. Now, we come back to our original motivation of, I want to build really complex systems where I want to use my ML modeling APIs, but I want to make sure that my ML modeling APIs are reliable and secure and safeguarded. What this ends up looking like is that, instead of a single LLM API call, you now have two LLM API calls, each of them have specific prompts that work for them. Typically, the output of one LLM API is fed as the input to the prompt of the other API. In practice, this leads to the issue of like compounding errors as well, as you can imagine, because you essentially don’t have water tightness on any of these APIs. As you move down the chain, you end up getting more incorrect responses. The guardrails way of the world in working with these systems essentially, is making sure that you have verification logic that surrounds every single LLM API that you’re making, so that the first output first passes through your verification suite, and only clears that suite once you know that that output is functionally correct. If we fail, we obviously go through our re-prompting strategy. If it passes validation, only then you go on to the next stage, where you then again go through this verification logic, and make sure that you send your response back to the AI application only on passing the verification logic. If we zoom out from this framework, overall, this reduces the degree of like compounding error, and on the entire workflow level, you end up having applications that are much more robust to the inconsistencies with working with LLMs.
Example: Guardrails for Customer Experience Chatbot
I’ve included some example of what types of guardrails you typically end up needing as you’re building a customer experience chatbot. We talked about like provenance and hallucinations. Typically, any unviolatable constraints that you have, like making sure that you can trust the output, making sure that your output is compliant. Or that there’s brand safety and you aren’t violating any constraints of like not using a language that wouldn’t be supported by your brand. Making sure that you’re not leaking any sensitive information. In general, making sure that your output has reliability, as well as data privacy. In practice, what this might end up looking like is that you only use vetted sources for building trust. You can encode guardrails for specific laws. For example, if you’re a healthcare company, making sure that you’re never giving any medical advice, because as an AI system, you’re not allowed to give medical advice. Never using any angry or sarcastic language for your AI system. As well as making sure that you’re able to control the input and output of any information that is sent into the LLM or retrieved from the LLM, and there’s no leakage of any sensitive data. Finally, only responding within category boundaries. Let’s say you’re building a customer support chatbot, if somebody is just like chatting with you about something that doesn’t pertain to the boundaries of that system, making sure that you’re guarding against like proper use of any application that you build.
Examples of Validations
More examples of validations that we care about. We talked about hallucination, of course. Never giving any financial or healthcare advice is another common criteria. As you can probably expect, this is a pretty complex machine learning problem of, in the first case even detecting what financial advice is or what medical advice is. Never asking any private questions. Never asking the customer for, give me your social security number or any private or sensitive information. Not mentioning competitors. Only making sure that each sentence is from a verified source and accurate. No profanity. Guarding against prompt injection, as well as never exposing the prompt or the source code of your application.
Summary
Guardrails AI is a fully open source framework. You can use it for creating any custom validator, as well as looking through the catalog of validators to plug into your AI applications, and making them safe and performant and reliable. You can use it for orchestrating the validation and verification of your AI systems. You can use it as a specification language to make sure that you’re communicating your requirements to the LLM correctly. To learn more, you can check out the GitHub repo at github.com/ShreyaR/guardrails. Our website is guardrailsai.com.
Questions and Answers
Participant 1: I liked the name Guardrails, you somehow arranged the Wild Wild West into a path that you can actually control. When you create the validators and now your input is going to go to the validator, how do you route it to the appropriate one? You have some logic, let’s say, I have three validators, I’m going to send to the first one, then I’m going to send to the second one, and I’m going to send to the third one, or how do you ascertain which one goes first?
Rajpal: There’s two ways of doing it today. I think the first one is that all validators are executed parallelly. You send your output, or your input to every single validator at the same time. I think in addition to that, we also have a specification language that allows you to stagger the execution of those validators. Some validators are typically more expensive. I talked about hallucination excessively. We have multiple different ways of targeting hallucination. Typically, there’s this tradeoff between the fastest and cheapest methods to run will also not be the most accurate methods. You can stagger them in a way so that you run the fast and cheap one first, and only if it fails, do you go down to the more expensive method. We have this specification language that allows you to pipeline them in different ways.
Participant 1: Is that why you actually have MapReduce them, where your mapper is doing things in parallel, and then the reduce takes the end?
Rajpal: The MapReduce example, I included, is another common example of building AI applications. A common issue that folks who are building with these systems have to wrangle is their context limit. With GPT-4, for example, or with most common GPT APIs, you can only send 4000 tokens, and let’s say you have a massive book. In that case you have to break that book down into 4000 tokens each, do your map stage over each book, and then do a final reduce stage using the LLM. That was a system that uses that MapReduce framework with Guardrails included at the mapping stage as well as at the reducing stage.
Participant 2: Thank you, again, for that thought. I was going to ask a question about context windows [inaudible 00:39:55] because of course, every time that you have to re-prompt [inaudible 00:40:00] the context window. So my question I’m going to focus on is about cost, actually. So you mentioned that there’s a guardrail without a procedure sometimes you re-ask the question [inaudible 00:40:14]. What has been your [inaudible 00:40:19] re-prompting to make it more accurate?
Rajpal: I think there’s two points I would highlight with respect to cost. All of the re-prompting is configurable. If you’re working with a guardrail that you don’t care about as much, or there’s some cost to getting it wrong, but you can use it using other methods. You don’t always have to re-prompt, you can just filter out the incorrect values or use a programmatic fix when available. Or just be like, ok, maybe I can’t answer this query. Typically, what I’ve seen people do is only configure re-prompting as their policy when it’s a guardrail, where, if this guardrail is wrong, or if this check fails, then my output just straight up isn’t useful to me. For example, I really like the idea of healthcare advice or financial advice as one of those things where some of the folks, for example, that we work with, they’re like, the cost of accidentally including healthcare advice in my output is so severe to me as an organization, when I might as well not respond, in which case I’m not giving value to my customer, or I might take that extra hit of re-prompting. Those are typically those subsets of guardrails on which people configure re-prompting. This is not as much on the software level, but more on the scope of problem level, a lot of the places where people end up using these systems are where the alternatives are typically way more expensive. Often, you typically have to wait in line for an hour to get an answer to your question, in which case companies are often ok with taking on that extra cost of re-prompting when necessary.
Participant 3: About your verification suite, how do you handle false positives?
Rajpal: A lot of the guardrails that we have are ML based as well, in which case they come with their own irreducible error or their own possibility to basically be wrong sometimes. I think how I like to look at this problem is, on some level, what we’re doing is ensembling, which is basically this technique of making different machine learning models work together, where each model has different strengths and weaknesses. My favorite analogy to use for this is that you’re stacking together different sieves, where each sieve has holes in different locations. You end up getting systems where the stack of sieves is way more watertight than each sieve individually would be. For example, for provenance or for our anti-hallucination guardrails, we have a bunch of internal testing, which allows us to estimate what the efficacy of the system is. The idea is that you mix and match a few of these together and ensemble the guardrails, that with the LLM ends up getting you to, overall, a much more robust system. That said, you’re working with machine learning, you’re never going to have zero error. The idea is to drive down the error as much as possible, using the tools that are available to us.
Participant 3: Once you start getting false positives, will that translate somehow into a new guardrail. I don’t know. [inaudible 00:44:06] dynamic guardrail or something like that where [00:44:11].
Rajpal: I’ll ground my answer in the example of provenance. In the example of provenance, I talked about embedding the similarity versus an NLI classifier. With embedding the similarity, which is the fast, cheap, and not as accurate method of detecting hallucination, what we end up finding is that it had a lot of false positives. Then, that allows us to figure out like, we have some estimation of the uncertainty of that system. What we end up doing basically is like K-Nearest Neighbors classification, and then what we end up seeing is like, based on our KNN classification, if the distance is greater than some threshold, then we probably don’t have a very good estimate for this point, in which case, you then route it. The failure policy for this guardrail is to send it to the more performant but then much more expensive guardrail, which is more accurate. That failover and failing onto different systems is part of it.
Participant 4: If along with having guardrails with different style, have you considered maybe fine-tuning the model with an updated cost function that incorporates the data.
Rajpal: Our approach to that system is basically to make it very easy for you as a developer to log the outcomes of those guardrails. Where, you’re running this live production system, you’re serving thousands of requests every day, so you end up getting over a month of running this, like a decent dataset with features about what works for each request or not. You can directly use that dataset, which we make it very easy to export, like for your fine-tuning. It’s a very interesting point where you can use it basically in your loss function of training a model and basically add it to your loss and basically get a much more representative loss. I haven’t seen anybody do that today. I don’t see a reason why not because the guardrails, like I said, are independently implemented, like modules basically. Yes, theoretically, you could.
See more presentations with transcripts
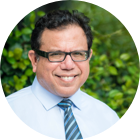
MMS • Steef-Jan Wiggers
Article originally posted on InfoQ. Visit InfoQ
Microsoft recently announced the general availability (GA) of Virtual Network flow logs, a new capability of the Network Watcher service in Azure.
Azure Network Watcher provides network monitoring and troubleshooting capabilities to increase observability and actionable insights. It offers out-of-the-box health metrics, topology visualization, connectivity monitoring, traffic monitoring, and a diagnostics suite. The virtual network flow logs capability was in public preview last year and is now generally available. It allows users to gather data about the IP traffic passing through their virtual networks. This data can be used to monitor and optimize usage, troubleshoot connectivity issues, ensure compliance, and analyze network security.
Virtual network flow logs are designed with user-friendliness and flexibility in mind. They’re a breeze to deploy for specific networks, subnets, or interfaces, and they capture layer-4 IP traffic data without impacting performance. The captured traffic is stored in a convenient JSON format for analysis, and you even have the option to enrich logs with metadata using Traffic Analytics, providing valuable insights into user behavior and security threats.
John Savill, a principal technical architect at Microsoft, summarizes in a YouTube episode on the Virtual Network flow logs:
At the Virtual Network level, I can enable flow logs. It no longer skips things when no NSG is applied. It’s really designed for that large-scale usage. It supports telling me hey, is it encrypted. It supports security admin rules and will log whether it is closest to a particular nick in the order of the application. I can hook in optionally into Traffic Analytics as well for that built-in visualization and use kQL (Kusto Query Language) against it to get that extra insight.
Traffic Analysis Example (Source: Microsoft Tech Community blog post)
Virtual Network flow logs can be accessed directly from storage accounts or integrated with out-of-box visualization such as Power BI. Finally, logs can be integrated with 3rd party applications for network and security analysis, like Cisco XDR, Darktrace, IBM QRadar, and Splunk.
Furthermore, Virtual Network flow logs have a wide range of practical applications. They can monitor traffic behavior, identify unknown or unwanted traffic, and track traffic levels. They also provide insights into application behavior through IP and port filtering. Additionally, they enable the analysis of cross-region traffic with GeoIP data and capacity forecasting. They play a crucial role in ensuring compliance with enterprise access rules. They can aid network forensics and security analysis by analyzing flows from compromised IPs and exporting logs to Intrusion Detection System (IDS) or Security Information and Event Management (SIEM) tools for further investigation.
Users can enable Virtual network flow logs on one or more virtual networks using Azure Portal, PowerShell, or AzCLI, and Network Security Groups (NSGs) are not required to be attached to those virtual networks.
AWS and Google Cloud (GPC) offer capabilities like virtual network flow logs, such as Virtual Private Cloud (VPC) flow logs in AWS. These logs capture IP traffic information within a VPC and allow publishing to Amazon CloudWatch Logs, S3, or Data Firehose for various tasks like security diagnostics and traffic monitoring. Similarly, GCP provides VPC Flow Logs for VM instances and Google Kubernetes Engine nodes, offering network monitoring, security analysis, and expense optimization functionalities accessible through Cloud Logging with options for log exportation.
Lastly, virtual network flow logs will be billed based on the number of logs generated. The Network Watcher pricing page, which specifically addresses the collection of network flow logs, provides more details.