Month: May 2024
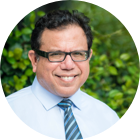
MMS • Steef-Jan Wiggers
Article originally posted on InfoQ. Visit InfoQ
At the annual Build conference, Microsoft announced the public preview of Azure Compute Fleet, a new service that streamlines the provisioning and management of Azure compute capacity across different virtual machine (VM) types, availability zones, and pricing models to achieve desired scale, performance, and cost.
Compute Fleet enables users to access Azure’s quick compute capacity in a specific region by launching a combination of Standard and Spot VMs at the best price and highest availability. Different pricing models, including Reserved Instances, Savings Plan, Spot, and Pay-As-You-Go (PYG) options, can help users achieve better price-performance ratios.
Microsoft’s Azure principal product manager, Rajeesh Ramachandran, wrote:
Tell us what you need, capacity and instance-wise, for Standard and Spot VM, and Compute Fleet will create both Standard and Spot VMs from a customized SKU list tailored to your workload requirements with the capability to maintain the Spot VM target capacity in the fleet.
Overview of Azure Compute Fleet (Source: Tech Community blog post)
Users can deploy up to 10,000 VMs with a single API, using Spot and Standard VM types together. Aidin Finn, an Azure MVP, tweeted:
Be handy for those times when I need 10k VMs with a single command.
Deploying Compute Fleet with a predefined fleet allocation strategy allows users to optimize their mix of VMs for capacity and cost efficiency or a balance of both. This will help alleviate concerns about the complexity of determining optimal VM pricing, available capacity, managing Spot evictions (instances that are closed down because another customer reserved them), and SKU availability.
Both AWS and Google Cloud Platform (GCP) provide services like Azure Compute Fleet for managing large deployments of virtual machines and instances across different environments. AWS offers AWS Systems Manager Fleet Manager and Amazon EC2 Fleet. The former provides a centralized interface to manage servers across AWS and on-premises environments. At the same time, the latter allows users to launch and manage a collection of EC2 instances across multiple instance types, availability zones, and pricing models through a single API call.
On the other hand, GCP offers Google Cloud Deployment Manager to automate the creation and management of Google Cloud resources.
Currently, Azure Compute Fleet is available in the following regions: West US, West US2, East US, and East US2. The documentation pages provide more details on Azure Compute Fleet.
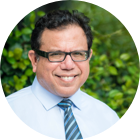
MMS • Steef-Jan Wiggers
Article originally posted on InfoQ. Visit InfoQ
At the annual Build conference, Microsoft announced the public preview of Azure Compute Fleet, a new service that streamlines the provisioning and management of Azure compute capacity across different virtual machine (VM) types, availability zones, and pricing models to achieve desired scale, performance, and cost.
Compute Fleet enables users to access Azure’s quick compute capacity in a specific region by launching a combination of Standard and Spot VMs at the best price and highest availability. Different pricing models, including Reserved Instances, Savings Plan, Spot, and Pay-As-You-Go (PYG) options, can help users achieve better price-performance ratios.
Microsoft’s Azure principal product manager, Rajeesh Ramachandran, wrote:
Tell us what you need, capacity and instance-wise, for Standard and Spot VM, and Compute Fleet will create both Standard and Spot VMs from a customized SKU list tailored to your workload requirements with the capability to maintain the Spot VM target capacity in the fleet.
Overview of Azure Compute Fleet (Source: Tech Community blog post)
Users can deploy up to 10,000 VMs with a single API, using Spot and Standard VM types together. Aidin Finn, an Azure MVP, tweeted:
Be handy for those times when I need 10k VMs with a single command.
Deploying Compute Fleet with a predefined fleet allocation strategy allows users to optimize their mix of VMs for capacity and cost efficiency or a balance of both. This will help alleviate concerns about the complexity of determining optimal VM pricing, available capacity, managing Spot evictions (instances that are closed down because another customer reserved them), and SKU availability.
Both AWS and Google Cloud Platform (GCP) provide services like Azure Compute Fleet for managing large deployments of virtual machines and instances across different environments. AWS offers AWS Systems Manager Fleet Manager and Amazon EC2 Fleet. The former provides a centralized interface to manage servers across AWS and on-premises environments. At the same time, the latter allows users to launch and manage a collection of EC2 instances across multiple instance types, availability zones, and pricing models through a single API call.
On the other hand, GCP offers Google Cloud Deployment Manager to automate the creation and management of Google Cloud resources.
Currently, Azure Compute Fleet is available in the following regions: West US, West US2, East US, and East US2. The documentation pages provide more details on Azure Compute Fleet.
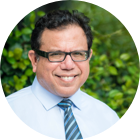
MMS • Bruno Couriol
Article originally posted on InfoQ. Visit InfoQ
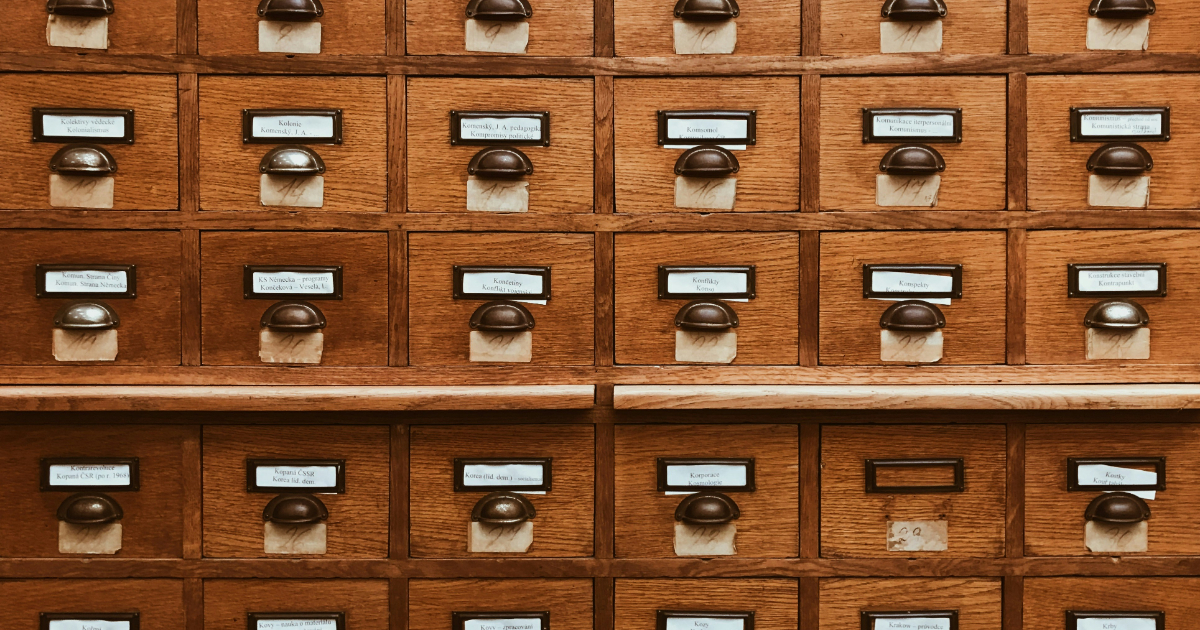
With the recently released PGlite, a Wasm build of Postgres that is packaged into a TypeScript client library, developers can run Postgres queries in the browser with no extra dependencies. PGlite is used for reactive, real-time, local-first apps.
Developers could already run SQLite in the browser with WebAssembly. However, running PostgreSQL in the browser previously required spanning a virtual machine and there was no way to persist data. With PGlite, developers can run PostgreSQL queries in a JavaScript environment and persist data to storage in the file system (Node/Bun) or indexedDB (browser).
PGlite was created to support use cases associated with reactive, real-time, local-first applications. ElectricSQL, which self-describes as a local-first software platform for modern apps with instant reactivity, real-time multi-user collaboration, and conflict-free offline support, uses PGlite to sync on demand with a server. They explain:
Local-first is a new development paradigm where your app code talks directly to an embedded local database and data syncs in the background via active-active database replication. […] ElectricSQL gives you instant local-first for your Postgres. Think of it like “Hasura for local-first”.
PGlite includes parameterized SQL queries, an interactive transaction API, pl/pgsql support, web worker execution, and more. PGlite is 2.6MB gzipped. PGlite can be used in memory in the browser as follows:
const db = new PGlite()
await db.query("select 'Hello world' as message;")
Developers can also persist the database to indexedDB:
const db = new PGlite("idb://my-pgdata");
One Reddit developer emphasized testing as an additional use case for PGlite.
Code testing is a big one for me. I’m currently using in-memory SQLite for tests and I’m often running into differences between SQLite and Postgres (default values, JSON handling, etc). This could allow me to use the real thing without running a full Docker instance.
Another developer said:
No one using Postgres in the cloud is going to use this as an alternative, but there are at least two use cases where this could be very useful:
You want your app to be local first (snappy, great offline support, etc) but sync data to a server. This is the ElectricSQL use case.
You want a serious data store in-browser. SQLite via Wasm already fits this use case, but it’s nice to have options.
PGlite is open-source software under the Apache 2.0 license. It is being developed at ElectricSQL in collaboration with Neon. PGlite is still in the early stages and plans to support pgvector in future releases.
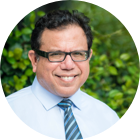
MMS • Sergio De Simone
Article originally posted on InfoQ. Visit InfoQ
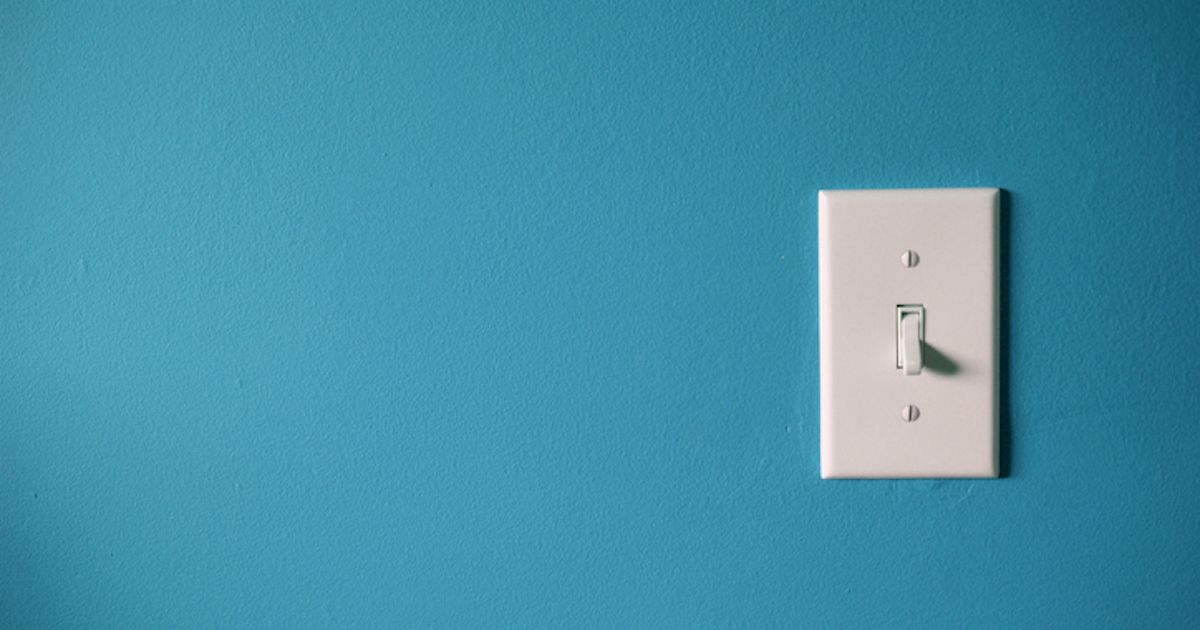
To ensure their Spanner database keeps working reliably, Google engineers use chaos testing to inject faults into production-like instances and stress the system’s ability to behave in a correct way in the face of unexpected failures.
As Google engineer James Corbett explains, Spanner builds on solid foundations provided by machines, disks, and networking hardware with a low rate of failure. This is not enough, though, to guarantee it works correctly under any circumstances, including bad memory or disks corrupting data, network failures, software errors, and so on.
According to Corbett, using fault-tolerant techniques is key to masking failures and achieving high reliability, including checksums to detect data corruption, data replication, using the Paxos algorithm for consensus, and others. But to make sure all of them work as expected, you need to exercise those techniques and make sure they are effective. This is where chaos testing comes into play, consisting of deliberately injecting faults into production-like instances at a much higher rate than they would arise in a production environment.
We run over a thousand system tests per week to validate that Spanner’s design and implementation actually mask faults and provide a highly reliable service. Each test creates a production-like instance of Spanner comprising hundreds of processes running on the same computing platform and using the same dependent systems (e.g., file system, lock service) as production Spanner.
The faults that are injected belong to several categories, including server crashes, file faults, RPC faults, memory/quota faults, and Cloud faults.
Server crashes may be produced by sending a SIGABRT signal at any time to trigger the recovery logic, which entails aborting all distributed transactions coordinated by the crashed server, forcing all clients accessing that server to fail over to a distinct one, and using a disk-based log of all operations to avoid losing any memory-only data.
File faults are injected by intercepting all file system calls and randomly changing their outcome, for example by returning an error code, corrupting the content on a read or write, or never returning to trigger a timeout.
Another area where a similar approach is followed is interprocess communication with RPC. In this case, RPC calls are intercepted to inject delays, return error codes, and simulate network partitions, remote system crashes, or bandwidth throttling.
Coming to memory faults, the Spanner team focuses on two specific behaviors: simulating a pushback state, whereby a server becomes overloaded and clients start redirecting their requests to less busy replicas, and leaking enough memory so the process is killed. Similarly, they simulate “quota exceeded” errors, whether they come from disk space, memory, or flash storage per user.
Injecting Cloud faults aims to test abnormal situations related to the Spanner API Front End Servers. In this case, a Spanner API Front End Server is crashed to force client sessions to migrate to other Spanner API Front End Servers and make sure this does not entail any impact for clients besides some additional latency.
Finally, Google engineers also simulate an entire region becoming unreachable due to several possible causes including file system or network outages, thus forcing Spanner to serve data from a quorum of other regions according to the Paxos algorithm.
Thanks to this approach based on a fault-tolerant design coupled with continuous chaos testing, Google efficiently validates Spanner’s reliability, concludes Corbett.
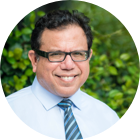
MMS • Anthony Alford
Article originally posted on InfoQ. Visit InfoQ
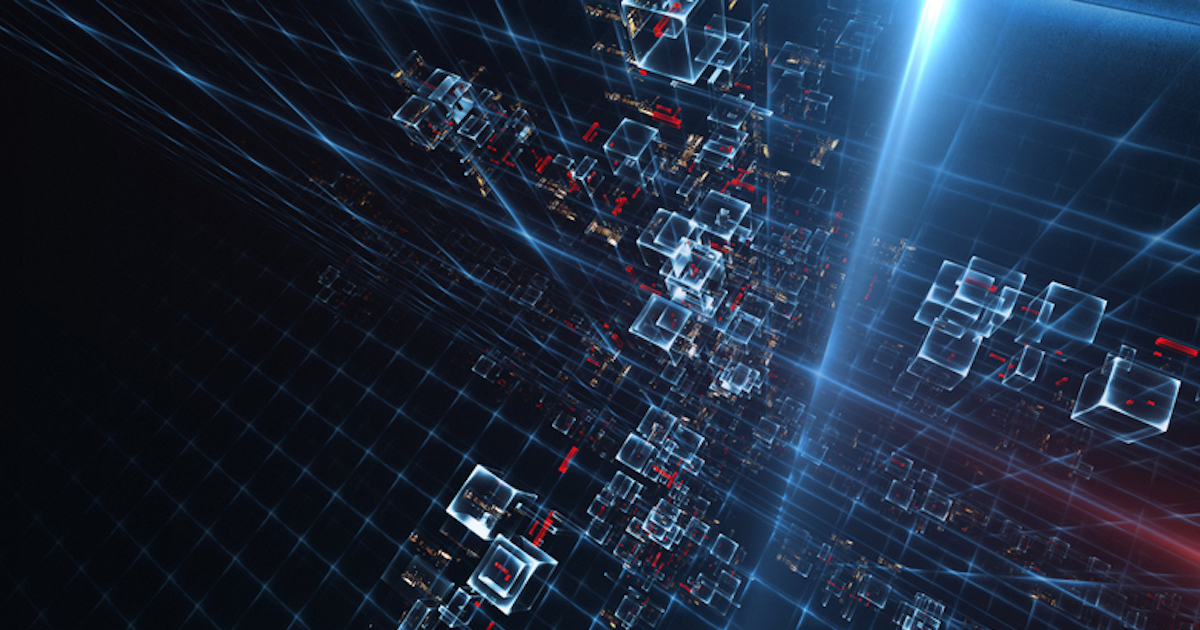
OpenAI recently announced the latest version of their GPT AI foundation model, GPT-4o. GPT-4o is faster than the previous version of GPT-4 and has improved capabilities in handling speech, vision, and multilingual tasks, outperforming all models except Google’s Gemini on several benchmarks.
The “o” in GPT-4o stands for “omni,” reflecting the model’s multi-modal capabilities. While previous versions of ChatGPT supported voice input and output, this used a pipeline of models: a distinct speech-to-text to provide input to GPT-4, followed by a text-to-speech model to convert GPT-4’s text output to voice. The new model was trained end-to-end to handle audio, vision, and text, which reduces latency and gives GPT-4o access to more information from the input as well as control over the output. OpenAI evaluated the model on a range of benchmarks, including common LLM benchmarks as well as their own AI safety standards. The company has also performed “extensive external red teaming” on the model to discover potential risks in its new modalities. According to OpenAI:
We recognize that GPT-4o’s audio modalities present a variety of novel risks. Today we are publicly releasing text and image inputs and text outputs. Over the upcoming weeks and months, we’ll be working on the technical infrastructure, usability via post-training, and safety necessary to release the other modalities. For example, at launch, audio outputs will be limited to a selection of preset voices and will abide by our existing safety policies. We will share further details addressing the full range of GPT-4o’s modalities in the forthcoming system card.
OpenAI gave a demo of the GPT-4o and its capabilites in their recent Spring Update livestream hosted by CTO Mira Murati. Murati announced that the new model will be rolled out to free users, along with access to features such as custom GPTs and the GPT Store which were formerly available only to paid users. She also announced that GPT-4o would be available via the OpenAI API and claimed the model was 2x faster than GPT-4 Turbo, with 5x higher rate limits.
In a Hacker News discussion about the release, one user noted:
The most impressive part is that the voice uses the right feelings and tonal language during the presentation. I’m not sure how much of that was that they had tested this over and over, but it is really hard to get that right so if they didn’t fake it in some way I’d say that is revolutionary.
OpenAI CEO Sam Altman echoed this sentiment in a blog post:
The new voice (and video) mode is the best computer interface I’ve ever used. It feels like AI from the movies; and it’s still a bit surprising to me that it’s real. Getting to human-level response times and expressiveness turns out to be a big change. The original ChatGPT showed a hint of what was possible with language interfaces; this new thing feels viscerally different. It is fast, smart, fun, natural, and helpful.
Along with GPT-4o, OpenAI released a new MacOs desktop app for ChatGPT. This app supports voice mode for conversing with the model, with the ability to screenshots to the discussion. OpenAI has also launched a simplified “look and feel” for the ChatGPT web interface.
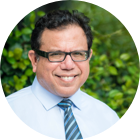
MMS • A N M Bazlur Rahman
Article originally posted on InfoQ. Visit InfoQ
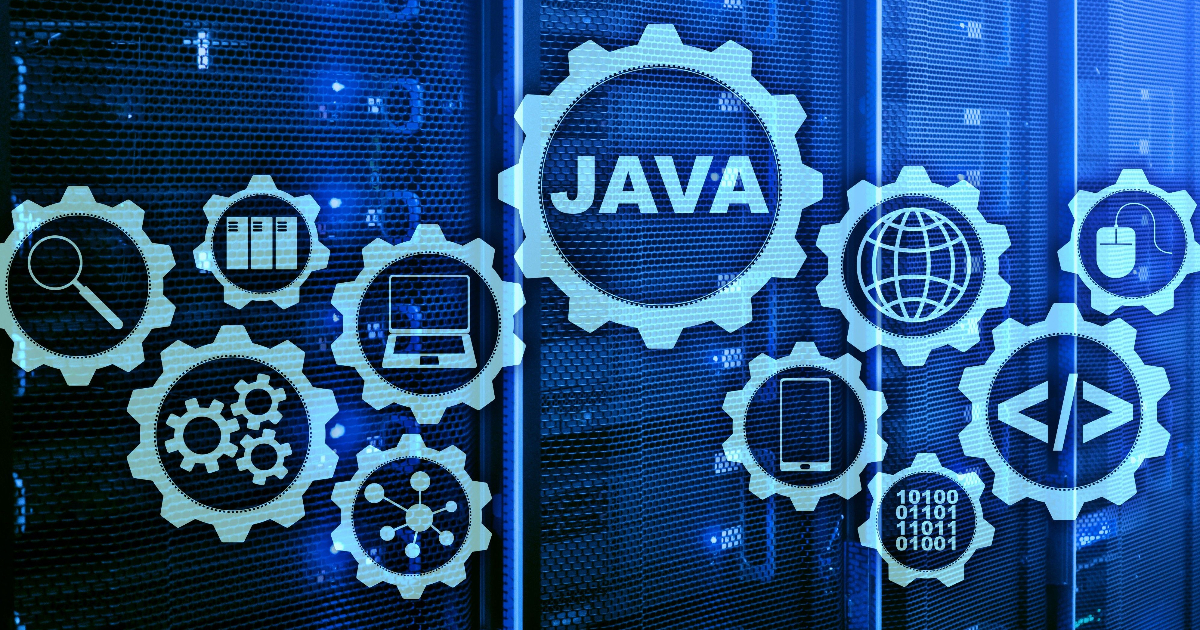
JEP 467, Markdown Documentation Comments, has been promoted from Proposed to Target to Targeted for JDK 23. This feature proposes to enable JavaDoc documentation comments to be written in Markdown rather than a mix of HTML and JavaDoc @
tags. This will allow for documentation comments that are easier to write and read in source form.
This update’s primary goal is to simplify the process of writing and reading documentation comments in Java source code. By allowing Markdown, which is known for its simplicity and readability, developers can avoid the complexities associated with HTML and JavaDoc tags. Existing documentation comments will remain unaffected, ensuring backward compatibility.
Furthermore, the update extends the Compiler Tree API, allowing other tools that analyze documentation comments to handle Markdown content effectively.
It’s important to note that this update does not include automated conversion of existing documentation comments to Markdown syntax. Developers must manually update their documentation to take advantage of the new feature.
Java documentation comments traditionally use HTML and JavaDoc tags, a practical choice in 1995 but has since become less convenient. HTML is verbose and challenging to write by hand, especially for developers who may not be familiar with it. Inline JavaDoc tags, such as {@link}
and {@code}
, are cumbersome and often require referencing documentation for proper usage.
Markdown, in contrast, is a lightweight markup language that is easy to read and write. It supports simple document structures like paragraphs, lists, styled text, and links, making it a suitable replacement for HTML in documentation comments. Additionally, Markdown allows the inclusion of HTML for constructs that it does not directly support, providing flexibility while reducing complexity.
Consider the following JavaDoc comment for java.lang.Object.hashCode
written in the traditional format:
/**
* Returns a hash code value for the object. This method is
* supported for the benefit of hash tables such as those provided by
* {@link java.util.HashMap}.
*
* The general contract of {@code hashCode} is:
*
* - Whenever it is invoked on the same object more than once during
* an execution of a Java application, the {@code hashCode} method
* must consistently return the same integer, provided no information
* used in {@code equals} comparisons on the object is modified.
* This integer need not remain consistent from one execution of an
* application to another execution of the same application.
*
- If two objects are equal according to the {@link
* #equals(Object) equals} method, then calling the {@code
* hashCode} method on each of the two objects must produce the
* same integer result.
*
- It is not required that if two objects are unequal
* according to the {@link #equals(Object) equals} method, then
* calling the {@code hashCode} method on each of the two objects
* must produce distinct integer results. However, the programmer
* should be aware that producing distinct integer results for
* unequal objects may improve the performance of hash tables.
*
*
* @implSpec
* As far as is reasonably practical, the {@code hashCode} method defined
* by class {@code Object} returns distinct integers for distinct objects.
*
* @return a hash code value for this object.
* @see java.lang.Object#equals(java.lang.Object)
* @see java.lang.System#identityHashCode
*/
This comment can be written in Markdown as follows:
/// Returns a hash code value for the object. This method is
/// supported for the benefit of hash tables such as those provided by
/// [java.util.HashMap].
///
/// The general contract of `hashCode` is:
///
/// - Whenever it is invoked on the same object more than once during
/// an execution of a Java application, the `hashCode` method
/// must consistently return the same integer, provided no information
/// used in `equals` comparisons on the object is modified.
/// This integer need not remain consistent from one execution of an
/// application to another execution of the same application.
/// - If two objects are equal according to the
/// [equals][#equals(Object)] method, then calling the
/// `hashCode` method on each of the two objects must produce the
/// same integer result.
/// - It is _not_ required that if two objects are unequal
/// according to the [equals][#equals(Object)] method, then
/// calling the `hashCode` method on each of the two objects
/// must produce distinct integer results. However, the programmer
/// should be aware that producing distinct integer results for
/// unequal objects may improve the performance of hash tables.
///
/// @implSpec
/// As far as is reasonably practical, the `hashCode` method defined
/// by class `Object` returns distinct integers for distinct objects.
///
/// @return a hash code value for this object.
/// @see java.lang.Object#equals(java.lang.Object)
/// @see java.lang.System#identityHashCode
Markdown documentation comments are indicated using ///
at the beginning of each line instead of the traditional /** ... */
syntax. This helps to avoid conflicts with embedded /* ... */
comments in the examples, which is increasingly common in documentation comments.
The Markdown parser used in this implementation is the CommonMark variant, with enhancements to support linking to program elements and simple GFM (GitHub Flavored Markdown) pipe tables. JavaDoc tags can still be used within Markdown documentation comments, ensuring that existing JavaDoc features are retained.
The parsed documentation comments are represented by com.sun.source.doctree
package in the Compiler Tree API. To handle uninterpreted text, a new type of tree node, RawTextTree
, is introduced, with a new tree-node kind, DocTree.Kind.MARKDOWN
, indicating Markdown content. The implementation leverages the commonmark-java library to transform Markdown to HTML.
The introduction of Markdown support for JavaDoc comments marks a significant improvement in Java’s documentation capabilities, making it more accessible and easier to maintain. This change is expected to enhance developer productivity and improve the overall readability of Java documentation.
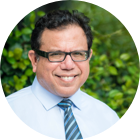
MMS • Steef-Jan Wiggers
Article originally posted on InfoQ. Visit InfoQ
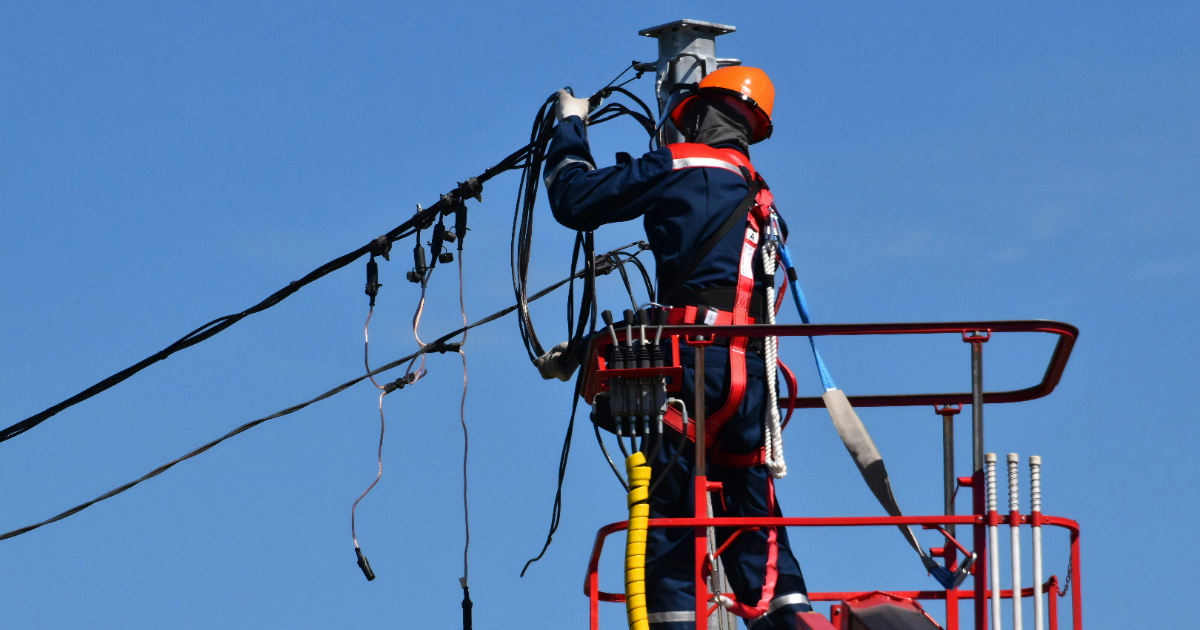
An Australian superannuation fund manager, UniSuper, using Google Cloud for an Infrastructure-as-a-Service (IaaS) contract, found it had no disaster recovery (DR) recourse when its entire infrastructure subscription was deleted.
UniSuper had previously migrated its VMware-based hardware infrastructure from two data centers to Google Cloud, using the Google Cloud VMware Engine. As part of its private cloud contract, UniSuper had its services and data duplicated across two Google Cloud regions. However, this regional separation was effectively virtual because both regions lost copies due to an internal Google error. There was no external disaster recovery facility in place.
This led to an outage that affected over 620,000 UniSuper fund members, leaving them unable to access their superannuation accounts for over a week.
Although UniSuper had backup systems in place, the deletion affected both geographies, causing a complete loss of data. Fortunately, UniSuper had additional backups with another provider, which helped minimize data loss and speed up restoration.
In a joint statement by Google and UniSuper:
This is an isolated, ‘one-of-a-kind occurrence’ that has never before occurred with any of Google Cloud’s clients globally. This should not have happened. Google Cloud has identified the events that led to this disruption and taken measures to ensure this does not happen again.
Yet, not everyone is convinced that it’s a one-of-a-kind issue; Daniel Compton, a software developer focusing on Clojure and large-scale systems, dedicated a blog post on the occurrence and concluded:
Given how little detail was communicated, it is difficult to make a conclusive statement about what happened, though I personally suspect UniSuper operator error was a large factor. Hopefully, APRA, Australia’s superannuation regulator, will investigate further and release a public report with more details.
And a respondent on a Hacker thread stated:
I really would like to hear the actual story here since it is basically impossible. It actually was “Google let customer data be completely lost in ~hours/days.” This is compounded by the bizarre announcements – UniSuper putting up TK quotes on their website, which Google doesn’t publish and also doesn’t dispute.
Furthermore, given the additional backups, a respondent on the same Hacker News commented:
Interestingly, the Australian financial services regulator (APRA) requires companies to have a multi-cloud plan for each of their applications. For example, a ‘company critical’ application needs to be capable of migrating to a secondary cloud service within four weeks.
I’m not sure how common this regulation is across industries in Australia or whether it’s common in other countries as well.
And lastly, a respondent on a Reddit thread wrote:
Customer support just isn’t in Google’s DNA. While this could have happened on any provider, this happens far more often on Google.
This story is a classic reminder of the rule of 1: 1 is 0, and 2 is 1. Thank goodness they could recover from a different provider.
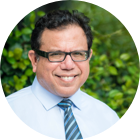
MMS • Glenn Engstrand
Article originally posted on InfoQ. Visit InfoQ
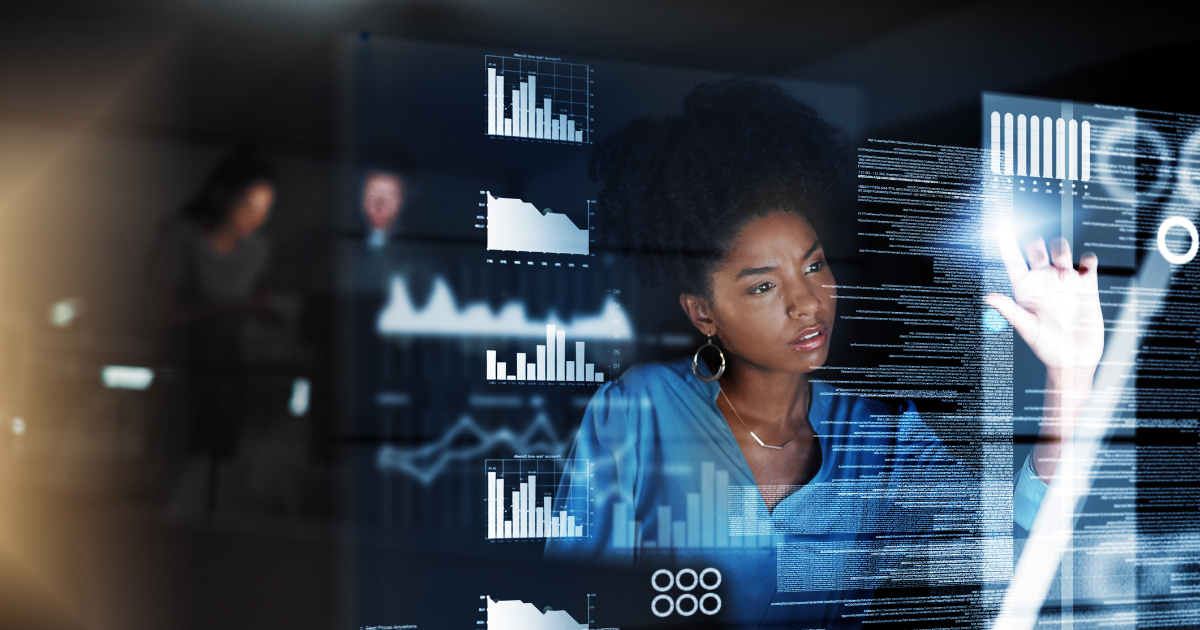
Key Takeaways
- To help understand if current LLM tools can help programmers become more productive, an experiment was conducted using improved code coverage of unit tests as an objective measure.
- No-cost LLMs were chosen to participate in this experiment; ChatGPT, CodeWhisperer, codellama:34b, codellama:70b, and Gemini. These are all free offerings which is why Github Copilot is not on this list.
- An experiment was designed to test each of the above selected LLM’s ability to generate unit tests for an already coded, non trivial web service. Each of the above mentioned LLMs were tasked with the same problem and prompting. Then the output was combined with the existing open source project which was then compiled and unit tests run. A record was kept of all the corrections needed to get the build to pass again.
- None of the LLMs could perform the task successfully without human supervision and intervention but many were able to accelerate the unit test coding process to some degree.
It hasn’t even been two years since OpenAI announced ChatGPT which is the first mainstream Large Language Model from a generative pre-trained transformer to be made available to the public in a way that is very easy to use.
This release triggered lots of excitement and activity from Wall Street to the White House. Just about every fortune 500 company and tech start up is trying to figure out how to capitalize on LLMs. The developer ecosystem is ablaze with supporting tools and infrastructure such as Lang Chain that accelerates the integration of existing applications with LLMs.
At a recent tech conference in Denver, Eric Evans (creator of Domain-Driven Design) “encouraged everyone to start learning about LLMs and conducting experiments now, and sharing the results and learnings from those experiments with the community.”
This article documents my personal contribution to that effort. This research is not based on any requirements or direction from any of my employers past or present.
The Experiment
I decided to come up with an experiment that I could perform on each of the more prevalent LLMs that are available in order to make comparisons between them and to explore the boundaries of what LLMs are capable of at least for the near term. I am not interested in or even worried about how LLMs will replace coders. You still need experienced developers when using LLMs because you need to be particularly vigilant about reviewing the suggestions. I am more interested in how LLMs can help coders become more productive by automating the more time consuming and menial yet still very important parts of writing code. I am referring to unit tests, of course.
Many will claim that unit tests are not a good place for LLMs because, in theory, with Test Driven Development you write the tests first and then the code afterwards. I have had experience in a fair number of companies where the unit tests were almost an afterthought and the amount of code being covered by the tests was directly proportionate to the amount of time remaining in the sprint. If coders could write more unit tests faster with LLMs, then there would be more code coverage and higher quality. I can already hear the TDD die-hards up in arms. Sorry but that is just the cold hard truth of it. Besides, how are you going to mock the external dependencies in the tests without first knowing the internal details of the implementations?
I have an open source repository where I implement identical microservices in various programming languages and tech stacks. Each implementation includes access to MySql fronted by Redis. I put each microservice on the same load test where I collect and analyze the performance data in order to make comparisons. I took a service class from the Java on Spring Boot implementation and scooped out all but three of the public, routable methods. Then I took the unit test code and removed all but one of the unit tests. I left the imports, setup and the annotation-based dependency injection in place. In the prompting, I ask for the other two unit tests to be generated. The entire prompt is 250 lines (about 1300 words) in length. Why did I use the Java on Spring Boot stack? There is a lot of code readily available online, without licensing restrictions, which was used as some of the training data for the LLMs. Spring Boot’s approach is heavily annotation-based which requires deeper understanding than simply studying the code itself.
I have approached this problem like it was a scientific experiment but it is not a very good one. An experiment is valuable only if it is reproducible but all of these technologies under evaluation are constantly evolving and the owning organizations spend a lot of money innovating on these products, and hopefully improving them. It is highly unlikely that you would arrive at the same results were you to conduct the same experiment today. Nevertheless, I parked a copy of the prompt that I used here just in case you wished to try.
OpenAI’s ChatGPT
It was the early days of ChatGPT, at the end of 2022, that brought transformer-based Large Language Models and OpenAI into the media spotlight. Of course I had to include ChatGPT in this experiment. There were a lot of rough patches in those early days: a small context window, low quality output, prompt forgetting, confident hallucinations. It’s in a much better place now. Like all of the other technologies evaluated here, I used the free version. There are concerns around the revealing of proprietary information via prompt leaking when using these commercial LLMs. That is why I based the experiment on open source. There is nothing proprietary to leak. Prompt leaking is unavoidable because your prompts are used to fine-tune the LLM which, over time, improves the quality of its answers in the future.
How did ChatGPT perform? It did okay. The explanation of the results was concise and accurate. The output was useful but it did have bugs, especially in the dependency injection and mocking areas. The test coverage was just okay. The unit test code had assertions for individual properties, not found, and not null. Even though there were bugs, I still considered the output to be useful because I felt that it would have taken me more time to type the code myself than to fix the bugs from the generated code.
Amazon CodeWhisperer
The next technology that I evaluated in this way was Amazon CodeWhisperer. This is a plugin for Visual Studio Code. The experience is basically a better statement completion. You start typing and it finishes the line. Sometimes it completes a block of code. You then choose to either accept or reject the proposed change. Most of the time, I accepted the change then made whatever corrections were required to fix any bugs in the generated code. I felt the most productive with CodeWhisperer.
I believe that CodeWhisperer is similar in approach to Github Copilot which I did not evaluate because that costs money whereas CodeWhisperer was free. When it comes to the internals of CodeWhisperer, Amazon keeps their cards close to the vest. It’s probably more than just an LLM but it does have that LLM feel to it. I suspect that CodeWhisperer was phoning home all the time because the IDE would freeze up often. You can disable CodeWhisperer which would result in the IDE becoming responsive again.
Code Llama
Ollama is an open source platform that allows you to build and run LLMs. It makes available a fair number of open source models that have already been pre-trained, including Meta’s Code Llama model. There is a lot of interest in these open source LLMs. Noted venture capitalist Bill Gurley identified Llama 2 (included in Ollama’s library of models) as OpenAI’s biggest threat at a summit in UCLA last year. Remember that prompt leaking issue that I mentioned earlier? Because you are hosting Ollama on VMs that are directly under your control, there is little possibility of prompt leaking.
Although it is not required, you are definitely going to want to run this on a system with a reasonably powered GPU. I don’t have a GPU on my personal laptop, so I provisioned a machine learning linux with CUDA system on an a2-highgpu-1g VM with a nvidia-tesla-a100 (312 TFLOPS) from the Google Cloud Platform to run the tests. More specifically, I used the codellama:34b model. From the meta blog that introduced this model, “Code Llama is a code-specialized version of Llama 2 that was created by further training Llama 2 on its code-specific datasets, sampling more data from that same dataset for longer. Essentially, Code Llama features enhanced coding capabilities, built on top of Llama 2.” I ran the same test with codellama:70b on a NVIDIA A100 40GB and did see a slight improvement in the code coverage of the resulting generated code. Provisioning that instance cost $3.67 per hour at the time of this writing.
The results were a little bit worse than ChatGPT but not too bad. There were compile errors, missing packages and imports, and mocking and dependency injection bugs. With the 34b model, the only code coverage was to assert not null. With the 70b model, that was replaced with an assertion that what was returned from the service call matched what got injected in the mock of the underlying DAO call. There is no explanation or online references included in the results with Code Llama. The generated output did include code comments that did not add much value.
Google Gemini
The last LLM that I performed this experiment on was Gemini which is Google’s rebranding of Bard. Like Code Llama, the generated output neglected to include the package statement or the imports which were available in the input. That was easy to fix. I am not sure if that was a mistake or simply a different interpretation of what I was asking for. Like all of the chat-based technologies, the output also had similar bugs with the dependency injection and mocking code. The code coverage was a little bit better as there were also tests for both cache hit and cache miss. The explanation was slightly better than ChatGPT and it cited the source that it used the most, which was not the open source repo where all of the code in the experiment came from. This was useful the same way that ChatGPT was useful. It took less time to fix the bugs than to code the two methods from scratch.
Conclusion
It can be quite challenging to obtain accurate and reliable numeric measurements for something as subjective as software quality. The following table attempts to summarize these findings in a numeric and therefore comparative way. The Myers Diff Algorithm is used to measure the number of lines of code added and deleted (a modified line is counted as both an add and a delete) needed to fix the bugs in the generated unit test code (because you are definitely going to have to fix the generated code). The Jacoco Code Coverage is the percentage of instructions (think Java byte code) covered by the unit tests divided by the total number of instructions (both covered and missed).
LLM-Based Generation of Unit Tests Results Summary By the Numbers
Generative AI Offering | Explanatory Analysis | Myers Diff Algorithm | Jacoco Code Coverage |
ChatGPT | yes | 8 | 29.12% |
CodeWhisperer | no | 26 | 27.81% |
codellama:34b | no | 117 | 23.42% |
Gemini | yes | 69 | 31.23% |
From these experiments, it became quite obvious that there was no Artificial General Intelligence present in the generation of any of this unit test code. The lack of any professional level comprehension of annotation based dependency injection and mocking made it clear to me that there was nothing deeply intelligent behind the figurative curtain. Quite simply, an LLM encodes a large number of documents tokenizing the input and capturing the context of that input based on its structure in the form of a transformer based neural network with a large number of weights known as a model.
When asked a question (such as a coding assignment), the model generates a response by predicting the most probable continuation or completion of the input. It considers the context provided by the input and generates a response that is coherent, relevant, and contextually appropriate but not necessarily correct. In that way, you can think of LLMs as a kind of complex, contextual form of search (albeit way more sophisticated than an Inverse Document Frequency or PageRank weighted stemmed term based search over skip lists that you find in web based search engines or Lucene circa 2020).
What I did find in the LLMs included in this experiment was a really good code search capability that turned out to be useful to an experienced developer. What was a disappointment for me with ChatGPT, Ollama, and Gemini was that I have been conditioned to expect human intelligence on the other side of a chat window. However, I have no such conditioning with statement completion. CodeWhisperer didn’t disappoint not because the AI was better but because the user experience did a better job at managing my expectations.
What’s Next?
There are also a few corporate concerns that may need to be addressed before adoption of unit test code completion style generative AI can exit the experimental phase.
I have already discussed prompt leaking. That should be a big issue for corporations because a lot of the corporation’s code will need to get included in the prompting and most corporations typically view their code as proprietary. If your prompting doesn’t go back to a model instance that is shared with other corporations, then you don’t have to worry about prompt leaking with other corporations. One option is to run the LLM locally (such as Ollama) which requires that every developer works on a machine with a sufficiently powered GPU. Another option is to subscribe to a single tenant, non-shared version of ChatGPT or Gemini. Yet another option is to turn off prompt driven fine tuning of the model entirely. The third option is currently available but not the second option.
The other concern is around cost. I suspect that generative AI pricing today is focused on increasing market share and does not yet cover all the costs. In order to transition from growth to profitability, those prices are going to have to increase. That NVIDIA A100 40GB I mentioned in the Code Llama section above costs around $10,000-ish today. There is also the question of power consumption. There is ongoing innovation in this area but typically, GPUs consume about three times as much power as CPUs. The single tenant approach is more secure but also more expensive because the vendor cannot benefit from economies of scale outside of building the foundation model. The improvement in productivity was only marginal. Cost is going to factor into the equation of long-term usage.
As I mentioned earlier, this space is moving fast. I did most of these experiments in early 2024. Since then, Amazon Code Whisperer got its own enterprise upsell. There are some early versions of both ChatGPT and Gemini fueled plugins for both IntelliJ and VS Code. Meta has released Llama 3 and, yes, it’s already available in Ollama. Where will we be this time next year? In terms of product placement, strategic positioning, government regulation, employee disruption, vendor winners and losers, who can say? Will there be AGI? Nope.
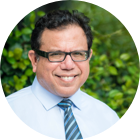
MMS • Bruno Couriol
Article originally posted on InfoQ. Visit InfoQ
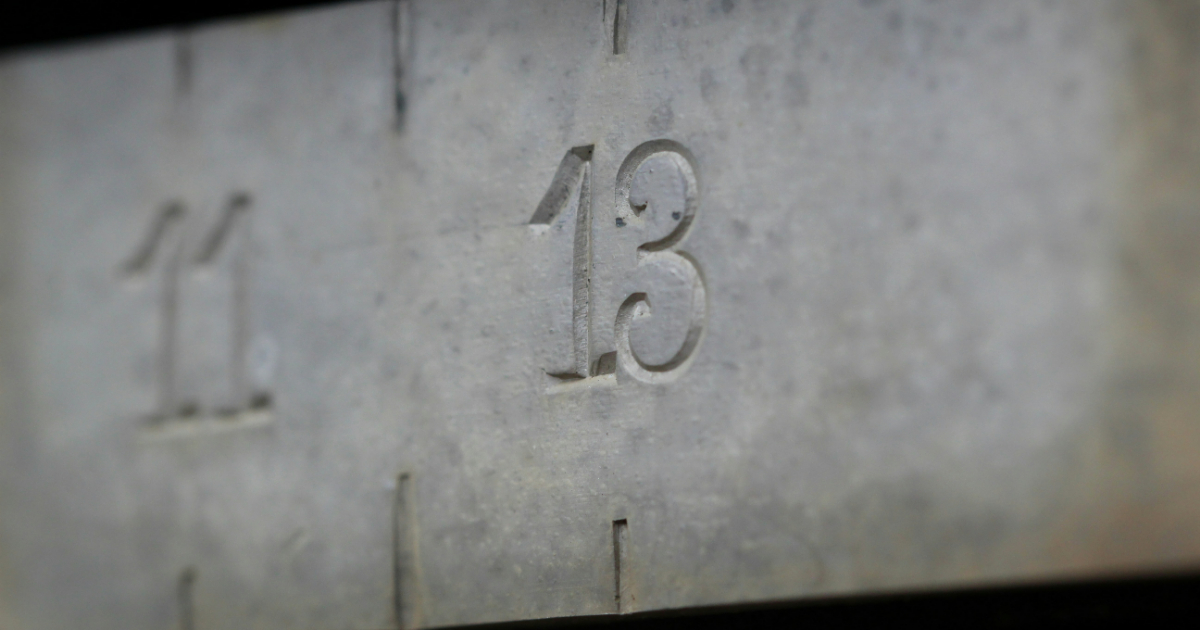
The Airbnb Tech Blog recently detailed how Airbnb enhances accessibility for users with vision difficulties. Through careful implementation of text resizing guidelines, Airbnb maintains web content, functionality, and a good user experience even as the text font size is doubled.
The Web Content Accessibility Guidelines are a set of standards and recommendations to make web content more accessible for individuals with disabilities. Mild visual disabilities are a fairly common occurrence. The CDC estimates the prevalence of the issue to be 3 out of 5 in America. Furthermore, as many Reddit users mention, not only users with a visual disability may reach out to text resizing capabilities of their browsers (or website, when available):
Anyone with a visual disability is likely to have a “large font size” option set in their browser. [lightmatter501]
And yes, people scale the font size with keyboard or mouse buttons. Because of accessibility mostly. [throwtheamiibosaway]
For some pages, I view the browser at 110% or 125% zoom since my monitor is 3440×1440 and sometimes the font is too small to read. I guess that still counts as accessibility. [Reddit user]
I just do it if I want to lean back in my chair for a while, or if I’m showing someone something, or if I’m sharing my screen on Zoom. [superluminary]
I change the minimum font size because of only one particular website I like to visit. But I am young and still have good eyes. [lontrachen]
The WCAG 1.4.4 Resize Text (Level AA) guideline requires that except for captions and images of text, text can be resized without assistive technology up to 200 percent without loss of content or functionality. Airbnb’s blog post details how they use font scaling as a complement to zoom scaling. Font scaling ties to the ability to increase or decrease text font size without necessarily affecting non-text elements of the page. Using browser zooming capabilities on the other hand scales all web content proportionally, which may lead to a suboptimal experience for some users.
The core idea consists of using em
and rem
CSS units instead of px
units. px
units are fixed and do not vary with the user-preferred font size. rem
units on the other hand are relative to the font size of the root element. The root element defaults to 16px
in many browsers, so 1rem
is often equal to 16px
. Setting font sizes with rem
units is a good idea because it is designed to adapt to the user’s browser preferences. em
is also a relative unit of measurement that unlike rem
is relative to the font size of the parent element or the font-size of the nearest parent with a defined font size.
The blog article explains:
The choice between
em
andrem
units often comes down to the level of control and predictability required for font scaling. Whileem
units can be used, they can lead to cascading font size changes that may be difficult to manage, especially in complex layouts. In contrast,rem
units provide a more consistent and predictable approach to font scaling, as they are always relative to the root element’s font size. […]In the case of Airbnb, the team decided to prioritize the use of rem units specifically for font scaling, rather than scaling all elements proportionally.
The blog article additionally goes into detail about spreading the corresponding design choices across the entire codebase (which uses two different CSS-in-JS systems), ensuring designers and developers adopt the new approach, and solving cross-platform issues (e.g., Safari on mobile). Airbnb deemed the experience successful:
Choosing font scaling as the product accessibility strategy brought about a range of significant benefits that notably enhanced our platform’s overall user experience. Making that change using automation to convert to rem units made this transition easier. When looking at our overall issues count after these changes were site-wide, more than 80% of our existing Resize Text issues were resolved. Moreover, we are seeing fewer new issues since then.
Developers are invited to refer to the full article and review the detailed technical explanations and demos that are provided.
Podcast: Deepthi Sigireddi on Distributed Database Architecture in the Cloud Native Era
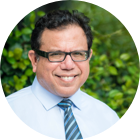
MMS • Deepthi Sigireddi
Article originally posted on InfoQ. Visit InfoQ
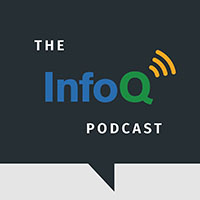
Subscribe on:
Transcript
Srini Penchikala: Hi everyone. My name is Srini Penchikala. I am the Lead Editor for AI/ML and the Data Engineering community at InfoQ and also a podcast host. In today’s podcast, I will be speaking with Deepthi Sigareddi, technical lead for Vitess, a Cloud Native Computing Foundation graduated open-source project. We will discuss the topic of distributed databases architecture, especially in the cloud native era where it’s critical for the database solutions to provide capabilities like performance, availability, scalability and resilience. Hi Deepthi, thank you for joining me in this podcast. First question, can you introduce yourself and tell our listeners about your career and what areas have you been focusing on recently?
Introductions [01:12]
Deepthi Sigireddi: Hi Srini. Thank you for inviting me to speak with you today. I am currently the technical lead for Vitess, open-source project and also the Vitess engineering lead at PlanetScale, which is a database as a service company. That database as a service is actually built on Vitess. My career started a long time ago and almost from the beginning I have worked on databases, but to start with as an application developer, so there is a database back-end, Oracle, DB2, Informix, and you are writing things that will run against, that data is stored in the tables and applications will fetch the data, do something with them.
But what happened very early on starting in the year 2000 is that I was working on doing supply chain planning solutions for the retail industry. And the retail industry has massive amounts of data. So we had to actually start thinking about how do you run anything on these massive amounts of data? Because the hardware that was available to us at that time, it was actually not possible to load everything into memory and process it and then write out the results. So we had to come up with parallelizable, essentially parallel computing solutions to work with these monolithic databases where people kept their data.
I did that for about 10 years and then I moved on to working on cloud security at a startup that was eventually acquired by IBM, and this was mobile device management, so mobile security. And even there you have a lot of data and it was being stored in Oracle and we were actually doing what is now called custom sharding because we would store different users data in different schemas in the same Oracle database so it was essentially a multi-tenant system. Fast-forward a few more years, and then I joined PlanetScale and started working on Vitess, which is a massively scalable distributed database system built around MySQL. MySQL is the foundation, but MySQL is monolithic and cannot actually scale beyond the limits of one server. And what Vitess does is that it actually solves many of those limitations for the largest users of MySQL.
Srini Penchikala: Yes. MySQL is a popular open-source database and it’s been around for a long time so Vitess is built on top of that. So as you said, Vitess is a distributed database. Can you discuss the high-level architecture of how the database works, how it brings this distributed data management capabilities to MySQL?
Deepthi Sigireddi: So let me give a little bit of history of Vitess first, which will set the context for all the things that Vitess is capable of doing. Vitess was created at YouTube in 2010, and the reason it was created is because YouTube, at that time, was having a lot of difficulty dealing with the amount of traffic they were receiving and all of the video metadata was stored in MySQL. The site would basically go down every day because it was just exploding and MySQL was not able to keep up. By that time, YouTube was already part of Google, but they were running their own infrastructure and some of the engineers decided that this was not a situation that you could live with. It was simply not tenable to continue the way they were going, and they decided that they had to solve this problem in some fundamental way, and that’s how Vitess was born and it grew in phases.
So they basically, to start with, identified what was the biggest pain point, like MySQL runs out of connections, stuff like that so let’s do some connection pooling. Let’s put a management layer in front of MySQL so that we can reduce the load on MySQL type of things, right? But eventually, it has grown to where we are. So with Vitess, you can take your data and shard it vertically, meaning you take your set of tables and put a subset on a different MySQL server and Vitess can make it transparently look as if there is only one database behind the scenes, even though there are multiple MySQL servers and different tables live on different servers.
Or you can do horizontal sharding where a single table is actually distributed across multiple servers but still looks like a single table to the application. So that’s a little bit of history and features. In terms of architecture, what Vitess does is that as part of the Vitess system, every MySQL that is being managed has a sidecar process, which we call VTTablet, which manages that MySQL.
And then the process that applications interact with is called VTGate. It’s a gateway that accepts requests for data and that can be MySQL protocol or it can be gRPC calls and it will decide how to route those queries to the backing VTTablets, which will then send the queries to the underlying MySQL and then return the results to VTgate, which will aggregate them if necessary and then send them back to the client application. So at a very high level, you have the client talks to VTGate. VTGAte figures out how to parse, plan, execute that query, sends it down to VTTablets, which will send it down to MySQL, and then the whole path travels back in reverse with the results.
Cloud Native Databases [07:05]
Srini Penchikala: Yes, I’m interested in getting into specifics of a distributed database. But before we do that, I would like to ask a couple of high-level questions. So what should the database developers consider when looking for a cloud-native database if they have a database need and they would like to leverage a cloud database? What should they look for in what kind of high-level architecture and design considerations?
Deepthi Sigireddi: At this point in time, almost every commercially available database has a cloud offering and they are capable of running in the cloud. That was not always the case. So really, the things that you want to look for when you are looking for a cloud database are usability because it’s important. You have very little control over how the database is being run behind the scenes so you have to be able to see what the configuration is, whether it works for you and be able to tune it to some extent. There’ll be some things that any service provider will not allow you to tune, but it needs to be usable for your application needs.
Compatibility, whatever it is that you’re doing needs to be supported by the database you’re looking at because there are many, many cloud databases. There’s Amazon RDS, Google Cloud SQL, Mongo has a cloud offering. So you have SQL databases, NoSQL databases, Oracle has cloud database offerings so there are many options so that compatibility is very important. And then uptime. You have no control over how well the database is staying up, and many times the database is critical to operations so you have to look at the historical uptime and the SLA that the cloud provider is providing you.
What you should not need to worry about are things like, will my data be safe? Will my database be available? Will I lose my data? Can I get my data out? All those are baseline things that the cloud providers need to make sure happen and you shouldn’t have to worry about them, but you need to check those boxes when you’re choosing an option.
Srini Penchikala: And kind of more of a follow-up question to that. I know not every application requires a massively parallel distributed database. So what are the things that database developers and application developers should look for when they could use a database that’s not a cloud-native database?
Deepthi Sigireddi: That’s a good question. I don’t know that, at this point of software system evolution, there are very many situations where you can’t use a cloud database. Usually, it is because you have highly sensitive data that legally you are not allowed to store in a third party. Because otherwise, in terms of encryption, in terms of network security, things have come a long way, and in general, it is safe to do things in the cloud. So the only thing I can really think of is legal reasons that you would not use something in the cloud.
Srini Penchikala: Yes. Also, I kind of misphrased my question. So I think cloud databases are always good choices for pretty much most of the applications, but the distributed database may not be needed for some of the apps so you can use a standalone database in the cloud.
Deepthi Sigireddi: Correct. Not everyone needs an infinitely scalable database – that is actually perfectly right. And that is why when you are starting off, you want to choose your database based on usability, based on compatibility with whatever applications and frameworks you’re already using, whatever tooling you’re already using, and just choose something that you can rely on that will stay up. So that goes back to my previous explanation of how people should make these decisions.
Sharding and Replication [11:06]
Srini Penchikala: So assuming that a distributed database is the right choice, one of the architectural highlights of Vitess is sharding, how it manages the database across different shards. So can you tell us how data sharding or partitioning works as well as data replication works in Vitess database?
Deepthi Sigireddi: Okay. Sharding was designed to provide scalability, right? So we’ll talk about that first, and then replication is being used to provide high availability, so we’ll talk about that next. In Vitess, sharding is customizable, meaning you choose which column in your table you want to use as the sharding key. It is not hardcoded, it is not fixed, and you can also choose the function based on which you are sharding. So we have several functions available and we also have a public interface using which people can build custom sharding functions as well. Essentially, what it means is that whenever you are inserting a row into a sharded table, VTGate will compute which shard it needs to go into and it will write it into that shard. And then whenever you are trying to read a row, VTGate will compute which shard that row lives in and fetch it from that shard.
Sometimes there are queries where you cannot compute specifically one shard. For example, if you say, “Get me all the items in my store, which cost more than a million dollars.” For a query like that, you don’t know which shard the data is living in. In fact, it can live in multiple shards. So what VTGate will do is called a scatter query. It will actually send that query to all the shards and gather the results and bring them back. So this is basically how sharding works from the user point of view. Behind the scenes, we have a lot of tooling that helps people to move from their currently unsharded databases. So people get into terabytes of data and then everything starts slowing down and managing things becomes very difficult. At that point, they decide, “Okay, we need to shard and either we need to do vertical sharding, which is separate tables into multiple instances or horizontal sharding”. We’ll focus on horizontal sharding.
So we have the tools using which you can define what your sharding scheme is, how you want to shard each table based on which column. You choose the tables that you want to keep together and first you may do a vertical sharding to move them onto their own servers, and then you do a horizontal sharding to split them up across servers, and the tooling will basically copy all the data onto the new shards and it’ll keep it up to date with your current database to which you can keep writing.
Your system is up and running, you can copy everything in the background, and then when you’re ready to switch to your sharded configuration and you issue that command, we will stop accepting all writes for a very small period of time. This should take less than 30 seconds, maybe 10 or 15 seconds. We’ll stop accepting all writes on the original database or databases and switch everything over to the new shards and then we’ll open it up again for writes so that the new data will flow to the new shards. And in this process, you can optionally also set up what we call a reverse replication so that the old databases are kept in sync with new data and changes that are happening on your new shards just in case you need to roll back.
So we have the facility to do a cut over and then reverse it if something goes wrong because things can always go wrong. So we have a lot of tooling around how you do the sharding, how you make it safe, how you do the cut overs, rollbacks, all of those things.
Srini Penchikala: Okay, that’s for the sharding. Is replication… How does the replication work?
Deepthi Sigireddi: So in our modern software world, application services, they have to stay up all the time. Downtime is not acceptable, right? Downtime is tracked for every popular service and beyond a certain point, users will just leave. If your service is not up, they will go somewhere else. They have options. Given that Vitess is backing many… Originally it backed YouTube. YouTube had to be up 24 by 7, people all over the world are using it. And then over time, other places like Slack, GitHub, Square Cash, you want to make a money transaction. You don’t want the database to be down, right? You don’t want the service to be down. So the service needs the database to be up at all times because you have this stack where you have the user, who’s interacting with the web app from a browser, and then you have all these other components and your uptime is only as high as the weakest of those components.
It’s like the weakest link concept, right? So everything has to be highly available. And the way, traditionally, everybody has achieved high availability with MySQL is through replication, because MySQL comes with replication as a feature so you can have a primary which is actually being written to, and then you can have replicas which are followers, and they’re just getting all the changes from the primary and applying the changes to their local database, and it’s like a always available copy of the data.
So in Vitess also, we use replication to provide high availability, and we have tooling around how to guarantee very high availability. So let’s say you want to do planned maintenance and you have a primary and you have one or more replicas. In the old days, if you have one MySQL server, you have to take it down for maintenance and then it’s unavailable. What we can do is we can actually transition the leadership from the current primary to a replica that is following, and we’ll basically say, “Okay, let’s find the one that is most caught up. If they are lagging, let’s get them to catch up and then switch over so that you have a new primary. And during that period, whatever request we are getting from applications, we’ll hold them up to some limit.” Right? Let’s say a thousand requests.
We can buffer, we can do buffering so that requests don’t error out and it’ll take five or 10 seconds for this transition to happen and after that, we can start serving requests again. So we use replication for high availability and around that replication, we have built features to do planned maintenance, but we also handle unplanned failures. So let’s say the primary went down for some reason. It’s out of memory, there’s a disk error, there’s a network issue, something or the other. The primary MySQL is not reachable.
In Vitess, we have a monitoring component called VTOrc, which stands for Vitess Orchestrator, and there’s a history behind that name as well. VTOrc monitors the cluster and it will say, if the primary is unreachable, I’ll elect a new one and I’ll elect whichever one is most ahead so that we don’t lose data. It will monitor replication on the replicas and make sure that they are always replicating correctly from the cluster primary and it can monitor for some other error conditions as well and fix them. So that’s how Vitess handles high availability by having all of this tooling to handle both planned maintenance and unplanned failures.
Srini Penchikala: So let’s quickly follow up on that, Deepthi, is like you mentioned, if one of the nodes in the cluster is not available or needs to be down for maintenance and the second node or replica node will take over the leadership. So again, the data needs to be properly sharded, partitioned and replicated, right? And then the primary comes back up so now we want to go back to the previous primary node.
So how much of this process is automated using the tools you mentioned, the VT Orchestrator, and how much of this is manual and also what is the maximum delay in switching between the nodes?
Deepthi Sigireddi: For planned maintenance, because it has to be initiated by someone, it’s not automated by Vitess. But Vitess users automate them in some fashion because typically what will happen is that they’re doing a rolling upgrade of the cluster, whether it’s a Vitess version upgrade or it’s some underlying hardware upgrade, you basically want to apply it to all the nodes, so it has to be triggered from outside. So they will call a Vitess command, because we have a command line interface. They will call a Vitess command. You can call an RPC also, but most people use the command line, which will trigger that failover.
Now, the other part of it, the unplanned failures. As long as VTOrc is running, it does the monitoring and the failover so no human intervention is needed actually for that, and that’s the whole idea of it. In terms of timing, we’ve seen things happen as quickly as five to 10 seconds, and especially during the planned failures because we buffer the requests, applications don’t even see it, or even if they see it is seen as a slight response delay versus errors. When you have an unplanned failure, then yes, applications are going to see errors, but typically, it is under 30 seconds because we can detect the failure in less than 15 seconds and then repair it immediately.
Srini Penchikala: For most of the use cases, I think that is tolerable, right? So that…
Deepthi Sigireddi: I think 30 seconds when you have an unplanned failure, like a node failure in a cloud or something like that, or an out of memory error, is actually really good because what used to happen is that somebody would have to be paged and they would have to go and do something manually, which would always take at least 30 minutes.
Consistency, Availability, and Distributed Transactions [21:31]
Srini Penchikala: Absolutely, and it’s planned. So usually the users are aware of the change and they’re expecting some outage. We cannot have a database discussion without getting into the transactions. So I know they’ve been the topic of the talk for a long time. Some people love them, some people hate them depending on how we use them. So distributed databases come with a distributed transaction kind of thing. So how does Vitess balance the conflicting capabilities of data consistency and data availability? So if you want one, you can have the other good old cap theorem. So how do the transactions work in the Vitess database?
Deepthi Sigireddi: So I think consistency and availability applies even if you are not using transactions. So I’ll talk about my view of how things happen in Vitess regarding consistency and availability first, and then we’ll talk about transactions. So if people are always reading from the primary instance of a shard, then there is actually no consistency problem in Vitess because that’s always the most up-to-date view of the data. That is the authoritative view of the data. When we get into consistency issues is that in order to scale read workloads, people will run many replicas and they will actually start reading data from the replicas. So then we get into consistency issues because replicas may be caught up to different extents. It may just be one or two seconds. So you have A, which is the primary, you have B and C, and maybe B is just one second behind A and C is two seconds behind B.
And if you fetch some data from B and some from C, you may end up with an inconsistent view of the data, right? The way, historically, Vitess has dealt with it is if consistency is important, you always read from the primary. If it’s not important, you read from the replicas. Now, people have used tricks like if in one user session they’re actually writing anything, then they always read from the primary. If it’s a read-only session, then they read from replicas or they will write something in a transaction and before they read something, they’ll set a timer like 60 seconds. So for the next 60 seconds, just read from the primary so that you get the up-to-date data. After that, you can go back to reading from replicas so people use these kinds of tricks.
What we would really like to do, and this gets a little bit into the roadmap, is to provide a feature in Vitess where you can specify that I want the read-after-write type of consistency, and Vitess can guarantee it whether you are going to a primary or to a replica. That’s a feature that we don’t have yet. Because you always have to have something more to do, no software is a hundred percent done. It’s never perfect so this is one of the things we would like to do. Now, coming to transactions. In a distributed system, there is always a possibility of something ending up being a distributed transaction.
Ideally, most write transactions go to one shard, in which case there’s actually no distributed transaction problem because MySQL itself will provide the transactional guarantees. But maybe in one transaction you’re updating two rows and they live in different shards like it’s a bank transaction and you have a sender and you have a recipient and you want to subtract $10 from the sender’s balance and add $10 to the recipient’s balance. And either both need to happen or neither of them should happen so that you actually have the consistency of the data.
You don’t want money to disappear or get created from thin air. So Square Case actually solved this problem and they came up with a very creative solution without actually having the true distributed transaction support in Vitess. And the way they did it was that they write this in a ledger and they use that to reconcile things after the fact so that even if something failed, they’re always able to repair it. The other thing we do in Vitess, which gets us out of some failure modes, is that when there is a distributed transaction, and suppose it involves four shards, right? We don’t execute all of the writes in parallel.
So we say, “Okay, let’s write to shard one. If it fails, we roll it back. Let’s write to shard two. If that fails, we can roll back both two and one.” So we have open transactions to all four shards and we’ll do one at a time and if at any point there is a failure, we roll back all of them. Once we have done the write when we have to commit the transaction, that is when we issue all the four commits in. Once MySQL has accepted a write, the probability of it rejecting a commit is actually very low, and it will only happen if you have a network problem and you’re not able to reach the server or if the server goes down. So we’ve reduced the universe of possible failures when you’re doing this type of a best effort distributed transaction. So that’s what we call it in Vitess. We are doing best effort distributed transactions. These are not truly atomic distributed transactions. But truly atomic distributed transactions is another thing we have on the roadmap that we would like to get to in the next one or two years.
Database Schema Management Best Practices [27:20]
Srini Penchikala: Switching gears a little bit, so schema management and data contracts are getting a lot of attention lately for a good reason. One of the interesting features is the VTAdmin that’s used for schema management APIs. Can you discuss about this a little bit and also share what you’re seeing as best practices on creating, managing and versioning schemas for the data models?
Deepthi Sigireddi: Okay, so VTAdmin is a part of Vitess that was built a couple of years ago. Previously, we had a very simple web UI, which you would use to see the state of the cluster and get some… It was mostly read-only. You could take some actions on the Vitess components and so on. So VTAdmin is like the next generation new generation administration component for Vitess and it has an API and a UI and all the APIs are well-structured. The problem with the previous thing we had was that we had HTTP APIs. We did not have gRPC APIs, and the response is not well-structured so no one could rely on a contract for the response. This time around, the API responses are well-structured. They are protobuf responses and you can build things relying on them. So we have a structured gRPC API and a VTAdmin API, and the UI actually talks to the API and gets the data and then renders it on the browser.
There are many things we show in the VTAdmin UI, and one of the things is schemas. And right now you can view the schemas, but you can’t really manage them from the UI. Even though we have the APIs behind the scenes, we haven’t built out the UI flows for doing the schema management so that’s something that will also be in the future. It’s in the roadmap. In terms of best practices for managing schemas and schema versions, I know there are lots of tools out there which people use to manage schema versions. Every framework comes with its own internal table, which versions the schemas and so on. In my personal opinion, store your schema and git. Store it in a version control system. And schema changes are actually a very fraught topic for the MySQL community because for at least 10 years or maybe even more, there have been multiple iterations of online schema change tools built for MySQL.
Because historically, MySQL performed very poorly when you tried to do a schema change on a large table, which is under load, right? So there would be locks taken, which would block access to the table completely. You couldn’t write anything to it, things would become unusable. MySQL itself, the MySQL team at Oracle, has been improving this and they have actually made a certain category of schema changes instant so that things don’t get locked or blocked or whatever. So they’ve been improving it, but of course, the community needed this many years before anything was done in Oracle’s product so there have been many iterations of these schema change tools.
Our stance is that schema changes should be non-blocking. They should happen in the background, and ideally, you need to be able to revert them without losing any data. So those are the principles on which Vitess’s online schema change system is built. So Vitess has an online schema change system and you can say that, “Hey, this is the schema change I want to do, start it, just cut it over when it’s done, or wait for me to tell you and I’ll initiate a cut over.” So all those things are possible with Vitess online schema changes and the biggest thing is they’re non-blocking. Your system will keep running.
Obviously, there’ll be a little additional load because you have to copy everything in the background while the system is still running, and you have to allow for that, but you shouldn’t be running the system at a hundred percent CPU and memory all the time anyway, so you should have room for these kinds of things. So that’s basically what we do in Vitess for non-blocking schema changes.
Vitess Database Project Roadmap [31:43]
Srini Penchikala: What are some of the new features coming up on the roadmap of Vitess project and interesting features that you can share with our listeners?
Deepthi Sigireddi: So compatibility and performance are things that we continuously work on so MySQL compatibility. MySQL keeps adding syntax and features, and it’s not like even historically at any point we supported a hundred percent of MySQL’s syntax. It has been an ongoing effort. So there is work happening on that pretty much continuously. A couple of things were added very recently. One is Common Table Expressions and the other one is window functions. The support for them is pretty basic, but we’ll be working on expanding the support for that so that we can cover all of the forms in which those things can be used. Performance is another area. So one of the ways in which Vitess removes stress from the backing MySQL instances is by using connection pooling.
Everybody doesn’t get a dedicated connection because MySQL connections are very heavy. They get a lightweight session to VTGate, which multiplexes everything over a single gRPC connection to a VTTablet, and the VTTablet maintains a pool of connections to MySQL. So we recently shipped a new connection pooling implementation, which makes the performance of the connection pool much better in terms of wait times or even memory utilization because we would tend to cycle through the whole pool and then go back. Whereas, if your queries per second is not very high, you may not even need to use the whole pool. You may not even need to keep a hundred or 150 open connections to MySQL so it’s just a much more efficient connection pooling, and we have ongoing work to improve our benchmarks.
We benchmark query performance on Vitess every night, and we publish those results on a dedicated website called benchmark.vitess.io, and we have an ongoing effort, basically continuous improvement of the benchmarks. Beyond that, in terms of functionality, we recently shipped point-in-time recovery, like the second iteration of point-in-time recovery in Vitess. There had been a previous iteration four years ago, and we’ll be making improvements to that and to online DDL based on user feedback.
Online Resources [34:20]
Srini Penchikala: Okay, thank you, Deepthi. Do you have any recommendations on what online resources our listeners can check out to learn more about distributed database in general or Vitess database in particular?
Deepthi Sigireddi: Vitess is part of the Cloud Native Computing Foundation. Google donated Vitess to CNCF in 2018, and Vitess graduated from CNCF in 2019. We have a website, vitess.io, where we have documentation, we have examples, we have quick start guides to downloading and running Vitess on your laptop. You can run the examples on a laptop. You can run it within Kubernetes. Vitess has always run within Kubernetes, so all of those are available on our website. And we also have links to videos because myself and some of our other maintainers and also community members, people who are using Vitess in their own companies, they go and talk about what they are doing.
So we have links to those videos on our website, but you can also just go to YouTube and search for Vitess and there are plenty of talks, and some of those talks are actually very good in terms of providing an introduction. A much more detailed introduction than what I just did to Vitess features and architecture, and they have some nice diagrams which are easier to consume sometimes than words in terms of how the architecture works.
Srini Penchikala: Before we wrap up today’s discussion, do you have any additional comments or any remarks?
Deepthi Sigireddi: I just want to say that working on Vitess and Open Source has been a really positive experience for me, and I encourage people to get involved in something that’s bigger than just whatever company or team you are working in, because it opens you up to new interactions with people, new experiences. It just enriches life as a software developer.
Srini Penchikala: Sounds good. Thank you, Deepthi. Thank you very much for joining this podcast. It’s been great to discuss one of the very important topics in cloud hosted database solutions, the distributed databases topic. And also, it’s great to talk to a practitioner like you who brings a lot of practical knowledge and experience to these discussions. To our listeners, thank you for listening to this podcast. If you would like to learn more about data engineering topics, check out the AI/ML and Data Engineering community web pages on infoq.com website. I encourage you to listen to the recent podcasts and check out the articles and news items the InfoQ team has been posting on the website. Thank you, Deepthi. Thanks for your time.
Deepthi Sigireddi: Thank you, Srini. This was great.
.
From this page you also have access to our recorded show notes. They all have clickable links that will take you directly to that part of the audio.