Month: October 2024
Microsoft Launches Azure Confidential VMs with NVIDIA Tensor Core GPUs for Enhanced Secure Workloads
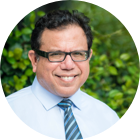
MMS • Steef-Jan Wiggers
Article originally posted on InfoQ. Visit InfoQ
Microsoft has announced the general availability of Azure confidential virtual machines (VMs—NCC H100 v5 SKU) featuring NVIDIA Tensor Core GPUs. These VMs combine hardware-based data protection from 4th-generation AMD EPYC processors with high performance.
The GA release follows the preview of the VMs last year. By enabling confidential computing on GPUs, Azure offers customers increased options and flexibility to run their workloads securely and efficiently in the cloud. These virtual machines are ideally suited for tasks such as inferencing, fine-tuning, and training small to medium-sized models. This includes models like Whisper, Stable Diffusion, its variants (SDXL, SSD), and language models such as Zephyr, Falcon, GPT-2, MPT, Llama2, Wizard, and Xwin.
The NCC H100 v5 VM SKUs offer a hardware-based Trusted Execution Environment (TEE) that improves the security of guest virtual machines (VMs). This environment protects against potential access to VM memory and state by the hypervisor and other host management code, thereby safeguarding against unauthorized operator access. Customers can initiate attestation requests within these VMs to verify that they are running on a properly configured TEE. This verification is essential before releasing keys and launching sensitive applications.
(Source: Tech Community Blog Post)
In a LinkedIn post by Vikas Bhatia, head of product, Azure confidential computing, and Drasko Draskovic, founder & CEO of Abstract Machines commented:
Congrats for this, but attestation is still the weakest point of TEEs in CSP VMs. Current attestation mechanisms from Azure and GCP – if I am not mistaken – demand trust with the cloud provider, which in many ways beats the purpose of Confidential Computing. Currently – looks that baremetal approach is the only viable option, but this again in many ways removes the need for TEEs (except for providing the service of multi-party computation).
Several companies have leveraged the Azure NCC H100 v5 GPU virtual machine for workloads like confidential audio-to-text inference using Whisper models, video analysis for incident prevention, data privacy with confidential computing, and stable diffusion projects with sensitive design data in the automotive sector.
Besides Microsoft, the two other big hyperscalers, AWS and Google, also offer NVIDIA H100 Tensor Core GPUs. For instance, AWS offers H100 GPUs through its EC2 P5 instances, which are optimized for high-performance computing and AI applications.
In a recent whitepaper about the architecture behind NVIDIA’s H100 Tensor Core GPU (based on Hopper architecture), the NVIDIA company authors write:
H100 is NVIDIA’s 9th-generation data center GPU designed to deliver an order-of-magnitude performance leap for large-scale AI and HPC over our prior-generation NVIDIA A100 Tensor Core GPU. H100 carries over the major design focus of A100 to improve strong scaling for AI and HPC workloads, with substantial improvements in architectural efficiency.
Lastly, Azure NCC H100 v5 virtual machines are currently only available in East US2 and West Europe regions.
Article: Adaptive Responses to Resiliently Handle Hard Problems in Software Operations
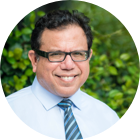
MMS • Laura Maguire
Article originally posted on InfoQ. Visit InfoQ
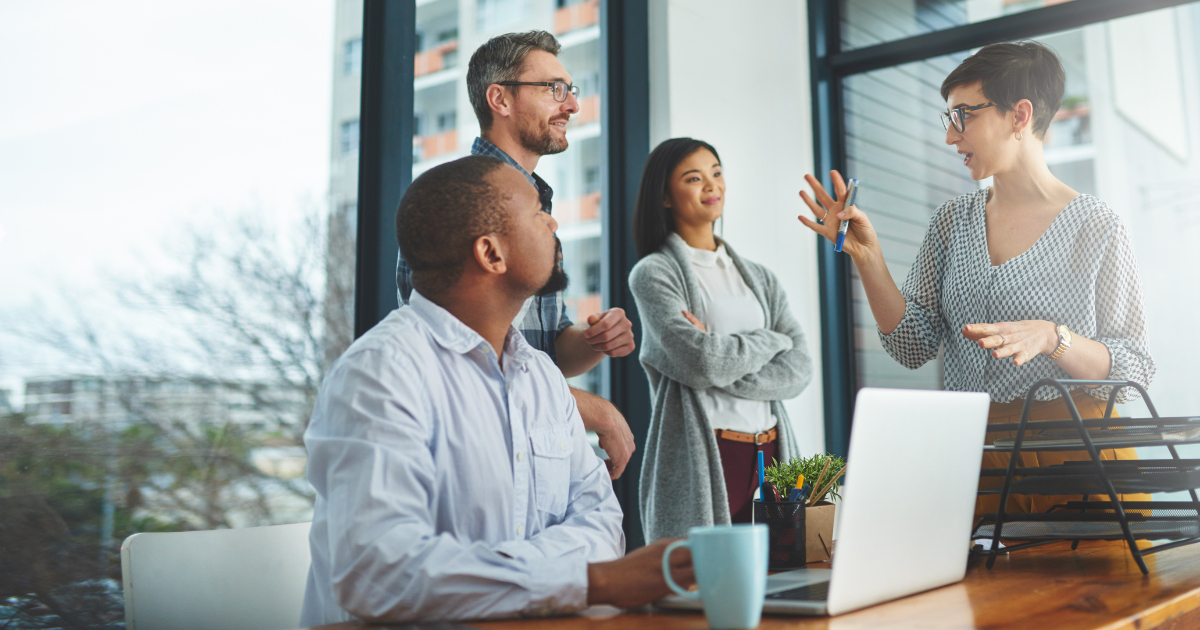
Key Takeaways
- Resilience – adapting to changing conditions in real time – is a hallmark of expert performance.
- Findings from Resilience Engineering studies have revealed generalizable patterns of human cognition when handling complex, changing environments.
- These studies guide how software engineers and their organizations can effectively organize teams and tasks.
- Five characteristics of resilient, adaptive expertise include early recognition of changing conditions, rapidly revising one’s mental model, accurately replanning, reconfiguring available resources, and reviewing to learn from past performance.
- These characteristics can be supported through various design techniques for software interfaces, changing work practices, and conducting training.
As software developers progress in their careers, they develop deep technical systems knowledge and become highly proficient in specific software services, components, or languages. However, as engineers move into more senior positions such as Staff Engineer, Architect, or Sr Tech Lead roles, the scope of how their knowledge is applied changes. At the senior level, knowledge and experience are often applied across the system. This expertise is increasingly called upon for handling novel or unstructured problems or designing innovative solutions to complex problems. This means considering software and team interdependencies, recognizing cascading effects and their implications, and utilizing one’s network to bring appropriate attention and resources to new initiatives or developing situations. In this article, I will discuss several strategies for approaching your role as a senior member of your organization.
Resilience in cognitively demanding software development work
Modern software engineering requires many core capabilities to cope with the complexity of building and running systems at speed and scale and to adapt to continuously changing circumstances. Resilience Engineering offers several concepts that apply to adapting to inevitable pressures, constraints, and surprises.
Resilience has been described in many ways by different fields. It has been used to describe psychological, economic, and societal attributes but comes primarily from ecology. It is used to describe adaptive characteristics of biological and ecological systems, and over the years, our understanding of resilience has changed. In software, perhaps the most impactful description of resilience is from safety researcher David Woods and the Theory of Graceful Extensibility. He defines it as “the ability of a system to extend its capacity to adapt when surprise events challenge its boundaries”.
This means an organization does not just “bounce back” or successfully defend itself from disruptions. Instead, it can respond in such a way that new capabilities emerge. Consider how, as Forbes notes in their article on business transformations, during the pandemic, commercial airlines responded to decreased travel by turning routes into cargo flights or how hotels that had lost travelers began offering daily room rates for employees working from home to stay productive safely.
Similarly, this resilience perspective is helpful for software engineering since “surprises” are a core characteristic of everyday operations in large-scale, continuous deployment environments. A core aspect of system design that allows for more resilient and reliable service delivery comes from designing, planning, and training for surprise handling.
Resilience Engineering techniques for everyday performance improvement
Researchers studying performance in high-demand work – like flying a fighter jet at 1800 mph close to terrain, rapidly shutting down a nuclear power plant after an earthquake, or performing open heart surgery on an infant in distress – have identified important human perceptual, reasoning, and response capabilities that allow someone to respond quickly and appropriately to a given event.
Even with extensive preparations and training, unexpected events can make following a playbook or work process difficult or impossible. Add time pressure and uncertainty about what is happening and how quickly things might fail, and the situation becomes overwhelmingly hard to manage.
People are forced to adapt in these kinds of surprising situations. They must rapidly identify a new way to handle the situation as it deteriorates to prevent the failure’s impacts from spreading. Successful adaptation is often praised as “quick thinking”, and in this article, we’ll explore the basis for quick thinking – or resilient performance – during software incidents.
The theoretical basis for quick thinking is drawn from research into high-consequence work settings. When applied to software operations, it can enhance resilient performance and minimize outages and downtime. Here, these findings are adapted into strategies for action for individual engineers and their organizations. These are:
- Recognizing subtly changing events to provide early assessment and action
- Revising your mental model in real time of how to adjust your actions
- Replanning in real time as conditions change
- Reconfiguring your available technical and human resources
- Reviewing performance for continuous learning
Together, these five capabilities can enable quick and accurate responses to unexpected events and move quickly without breaking things.
Recognizing: The importance of early detection
Early recognition of a problem, changing circumstances, or needing to revise our understanding of a situation is crucial to resilience. Early detection is beneficial in that it allows:
- more possibilities for action because the situation has not progressed very far
- the opportunity to gather more information before needing to act
- the ability to recruit additional resources to help cope with the situation
Early detection is not always possible due to a lack of data or poor representation of the available data. However, engineers can better recognize problems earlier by continually calibrating their understanding of how the system operates under varying conditions and noticing even subtle changes quickly. Here are three practical ways for software engineers to achieve this in day-to-day work:
Calibrating to variance: One approach is to become more familiar with expected vs. unexpected system behavior by regularly monitoring different operating conditions, not just when there is a problem. An active monitoring practice helps calibrate variance, such as when a spike in volume indicates a problem versus when a certain time zone or customer heavily utilizes the service.
Expanding knowledge about changes: Another strategy is to develop a practice of reading incident reports and reviewing what the dashboards looked like at the earliest indication of trouble to get better at noticing what an anomalous event looks like.
Encouraging knowledge transfer: Lastly, another technique for lightweight calibration to help early detection is asking, “What did you notice that caused you to think there was a problem?” whenever a coworker describes a near miss or a time they proactively averted an outage. Their explanations and your interpretations of these vicarious experiences reinforce a more elaborate mental model of nominal and off-nominal behavior.
Revising: The role of mental models in solving hard problems
A mental model is an internal representation of a system’s behavior. All software engineers construct mental models of how the system runs and fails. Mental models typically include information about relationships, interdependencies, and interactivity that allow for inferences. They can also help predict how a system will likely respond to different interventions.
For software engineers, this means mentally sifting through possible solutions, issues, and interactions to determine the most reasonable action. What is reasonable depends on assessing the action against the current and expected future conditions, goals, priorities, and available resources. In other words, to simulate how different choices will impact desired outcomes. A well-calibrated mental model can help engineers effectively simulate and be better prepared to assess the pros/cons of each and what risks may be involved.
But mental models can be – and often are – wrong. As noted in Behind Human Error, mental models are partial, incomplete, and flawed. This is not a criticism of the engineer. Instead, it acknowledges the complex and changing nature of large-scale software systems. No one person will have complete and current knowledge of the system. No one has a perfect understanding of the dependencies and interactions of a modern software system. Most software systems are simply too big, change too much, and too quickly for anyone’s knowledge to be consistently accurate.
Having poorly calibrated knowledge is not the problem. The problem is when you don’t know you have poorly calibrated knowledge. This means engineers must continually focus on model updating. A strategic resilience approach is cultivating a continual awareness of how current or stale your understanding of the situation may be. As a researcher studying how engineers respond to incidents, I constantly look for clues indicating how accurate the responders’ mental models are. In other words, is what they know or believe about a situation or a system correct? This is a signal that model updating is needed. A high-performing team can quickly identify when they’ve got it wrong and rapidly find data to update their understanding of the situation. Some approaches to continual revising include:
Call out the uncertainties and ambiguities: A technique that helps teams notice when their mental models are incorrect or differ is to ask clarifying questions like “What do you mean when you say this query syntax is wrong?” It’s a simple and direct question that my research has shown is not commonly asked. Explicitly asking creates opportunities for others to reveal what they are thinking and allows all involved to make sure they have the same understanding. This is especially crucial as situations are rapidly changing. Teams can develop shorthand ways of ensuring model alignment to avoid disrupting the incident response.
Developing a practice of explicitly stating assumptions and beliefs so that those around can track the line of reasoning and quickly identify an incorrect assumption or faulty belief. This seems so simple, but when you start doing this, you realize how much you “let slide” about inaccurate or faulty mental models in ourselves or others because it seems so small that it doesn’t seem worth revising, or time pressure prevents us from revising. A more junior engineer may be apprehensive about asking clarifying questions about a proposed deployment or hesitate to talk through their understanding of the risks of rolling back a change for fear of being wrong. The more senior engineer may not realize a gap in their mental model or may not want to publicly call out faulty knowledge.
Learn to be okay with being wrong: Software engineers must accept that their mental models will be wrong. Organizations need to normalize the practice of “being wrong”. This shift means that the processes around model updating – like asking seemingly obvious questions – become an accepted and common part of working together. Post-incident learning reviews or pair programming are excellent opportunities to dig into each party’s mental models and assumptions about how the technology works, interacts, and behaves under different conditions.
Replanning: It’s not the plan that counts, it’s the ability to revise
Software engineers responding to a service outage are, for the most part, hard-wired to generate solutions and take action. Researchers Gary Klein, Roberta Calderwood, and Anne Clinton-Cirocco studied expert practitioners in various domains. He showed that anomaly recognition, information synthesis, and taking action were tightly coupled processes in human cognition. The cycle of perception and action is a continuous feedback loop, which means constant replanning based on the changing available information. The replanning gets increasingly tricky as time pressure increases, partly due to the coordination requirements.
For example, replanning in everyday work situations such as in a sprint planning meeting and deciding how to prioritize one feature over another. In this scenario, there is time to consider the implications of changing the work sequencing or priorities. In this situation, it is possible to reach out to any parties affected by the decision and account for their input on how the plan may impact them. It is relatively easy to reorganize the workflow with less disruption for everyone.
Contrast that with a high-severity incident where there may be potential data loss in a critical, widely used internal project management tool. The incident response team thinks the data loss may be limited to only a part of the organization. While there is a slight possibility they could recover this data, it would mean keeping the service down for another day, impacting more users. One team has a critical meeting with an important client and needs the service restored within the next hour. This meant responders had to determine the blast radius of impacted users, the extent of their data loss, and the implications to those teams while the clock was ticking. Time pressure makes any kind of mental or coordinative efforts more challenging, and replanning with limited information can have significant consequences as needed perspectives may be unavailable to weigh in, causing more stress to all involved and forcing unexpected shifts in priorities or undesirable tradeoffs.
In a recent study looking at tradeoff decisions during high-severity incidents, my colleague Courtney Nash and I found that successfully replanning decisions was inevitably “cross-boundary”. A major outage often requires many different roles and levels of the organization to get involved. This meant that an understanding of the differing goals and priorities of each role was essential to being able to quickly replan without sacrificing anyone’s goals. Or, when goals and work need to be changed, the implications of doing so would be clearer to the replanning efforts. These findings and others from the resilience literature provide an important strategy for resilient replanning:
Create opportunities to broaden perspectives: Formal or informal discussions highlighting implicit perceptions and beliefs can influence how and when participants take action during an incident or work planning. They can use this information to revise inaccurate mental models, adjust policies and practices, and help organizations identify better approaches to team structure, escalation patterns, and other supporting workflows. A greater understanding of goals and priorities and how they may shift in response to different demands aids in prioritization during replanning. A crucial part of coping with degraded conditions is to assess achievable goals given the current situation and figure out which ones may need to be sacrificed or relaxed to sustain operations.
Reconfiguring: Adjusting to changing conditions
Surprises seldom occur when it is convenient to deal with them. Instead, organizations scramble to respond with whoever is available and whatever expertise, authority, or budget may be available. Organizations that flexibly and effectively use the given resources can support effective problem-solving and coordination even in challenging conditions. This can be simple things like having a widely accessible communication platform that doesn’t require special permissions, codes, or downloaded apps, allowing anyone who could help to join in the effort seamlessly. It may be more complex – such as an organization that promotes cross-training for adjacent roles.
Or it could be holding company-wide game days to be able to efficiently engineers from multiple teams on a significant outage because they have common ground – they know each other, have some familiarity with different parts of the system than they usually work on, and can rely on their shared experiences to accurately predict who may have appropriate skills to perform complex tasks. Just like you might add, delete, or move resources within your network configuration, a strategy of dynamic reconfiguration of people and software helps resilience by moving expertise and capabilities to where they are needed while minimizing any impacts of degraded performance in other areas. A resilient strategy for reconfiguration in software organizations includes:
Cultivating cross-boundary awareness: Reconfiguring allows an organization to share resources more efficiently when there is accurate knowledge about the current state of the goals, priorities, and work underway of adjacent teams or major initiatives within the organization. Research looking at complex coordination requirements has shown better outcomes for real-time reconfiguring when the parties have a reasonably calibrated shared mental model about the situation and the context for the decision. This enables each participant to bring their knowledge to bear quickly and effectively, to support collaborative cross-checking (essentially vetting new ideas relative to different perspectives) and allows for reciprocity (being able to lend help or relax constraints) across teams or organizations.
Maintaining some degree of slack in the system: Modern organizations are fixated on eliminating waste and running lean. But what is considered inefficient or redundant before an incident is often recognized as critical, or at least necessary, in hindsight. In many incidents I’ve studied, Mean Time To Repair (MTTR) is usually reduced by engineers proactively joining a response even when they are not on-call. This additional capacity, not typically acknowledged or accounted for when assessing the actual requirements for maintaining the system, is nonetheless critical. It is realized due to engineers’ professional courtesy to one another. It is highly stressful to be responsible for a challenging incident or deal with the pressure of a public outage. I’ve seen other engineers jump into the slack to assist even when putting babies to bed or taking vacations. Burnout, turnover, and changing roles are inevitable. Maintaining a slightly larger team than optimally efficient can help make the team more resilient by increasing communication, opportunities to build and maintain common ground, and cross-training for new skills.
Reviewing performance: Continuous learning supports continued performance
There is a difference between how we think work gets done and how work actually gets done. Learning review techniques that focus on what happened, not what was clear after the fact, helps to show how the system behaves under varying conditions and how organizations and teams function in practice. Discussing the contributing factors to the failure, hidden or surprising interdependencies, and other technical details should also include details about organizational pressures, constraints, or practices that helped or hindered the response. This is true even around the “small stuff”, like how an engineer noticed a spike in CPU usage on their day off or why a marketing intern was the only one who knew an engineering team was planning a major update the day before a critical feature launched. When the post-incident review broadens to include both the social and technical aspects of the event, the team can address both software and organizational factors, creating a more resilient future response.
Some strategies for enabling continuous learning to support resilience include:
Practice humility: As mentioned before, inaccurate or incomplete mental models are a fact of life in large-scale distributed software system operations. Listening to and asking clarifying questions helps to create micro-learning opportunities to update faulty mental models (including your own!)
Don’t assume everyone is on the same page: Where possible, always start with the least experienced person’s understanding of a particular problem, interaction, or situation and work up from there, adding technical detail and context as the review progresses. This gives everyone a common basis of understanding and helps highlight any pervasive but faulty assumptions or beliefs.
Make the learnings widely accessible: Importantly, organizations can extend learning by creating readable and accessible artifacts (including documents, recordings, and visualizations) that are easily shared, that allow for publicly asking and answering questions to promote a culture of knowledge sharing, and are available to multiple parties across the organization even non-engineering roles. A narrative approach that “tells the story” of the incident is engaging and helps the reader understand why certain actions or decisions made sense at the time. It’s a subtle but non-trivial framing. It encourages readers to be curious and promotes empathy, not judgment.
Resilience takeaways
Like any other key driver of performance, resilience requires investment. Organizations unwilling or unable to invest in taking a systems approach can reallocate resources to resilient performance in small but repeatable ways by maximizing the types of activities, interactions, and practices that allow for REvision, REcognition, REplanning, REconfiguring, and REviewing. In doing so, we can enable software teams to coordinate and collaborate more effectively under conditions of uncertainty, time pressure, and stress to improve operational outcomes.
Podcast: Generally AI – Season 2 – Episode 4: Coordinate Systems in AI and the Physical World
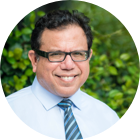
MMS • Anthony Alford Roland Meertens
Article originally posted on InfoQ. Visit InfoQ
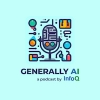
Transcript
Roland Meertens: Anthony, did you ever have to program a turtle robot when you were learning to program?
Anthony Alford: I’ve never programmed a turtle robot, no.
Roland Meertens: Okay, so I had to do this when I was learning Java and in robotics, the concept of a TurtleBot is often that you have some kind of robot you can move across the screen and it has some kind of pen, so it has some trace. So you can start programming, go upward or go forward by one meter, then turn right by 90 degrees, go forward by one meter, turn right by 90 degrees, so that way you trace a pen over a virtual canvas.
Anthony Alford: The Logo language was based on that, right?
Roland Meertens: Yes, indeed. So the history is that the computer scientist Seymour Papert, who created a programming language Logo in 1967, apparently they use this programming language, so these are things I don’t know, to direct like a big robot with a pen in the middle, which would let you make drawings on actual paper.
Anthony Alford: Okay.
Roland Meertens: It’s pretty cool, right?
Anthony Alford: It’s a bit of a plotter, a printer.
Roland Meertens: So apparently in 1967 people learned to program with a physical moving plotter. They immediately start with a robot-
Anthony Alford: That’s pretty cool.
Roland Meertens: Yes, instead of using a virtual canvas. It was round and crawled like a turtle. But the other thing I found is that turtle robots, their first mention is in the 1940s they were invented by Grey Walter and he was using analog circuits as brains, and his more advanced model could already go back to a docking station when the battery became empty.
Anthony Alford: That’s pretty cool. In the ’40s.
Roland Meertens: In the 1940s. Yes. I will put the video in the show notes and I will also put two articles in the show notes. One is the History of Turtle Robots. Someone wrote an article about it for Weekly Robotics and another article on the history of turtle robots as programming paradigms.
Anthony Alford: Very cool.
Roland Meertens: Yes.
Anthony Alford: Slow and steady.
Roland Meertens: Yes, slow and steady and is a great way to get started with programming.
At the Library [02:15]
Roland Meertens: All right, welcome to Generally AI, Season 2, Episode 4, and in this InfoQ Podcast, I, Roland Meertens, will be discussing coordinate systems with Anthony Alford.
Anthony Alford: How’s it going, Roland?
Roland Meertens: Doing well. Do you want to get started with your coordinate system research?
Anthony Alford: Let’s go for it. So I decided to go with an AI theme of coordinates and perhaps you can guess where I’m going. We’ll see.
Roland Meertens: Tell me more.
Anthony Alford: Well, in the olden days, a teacher, for example, in a history class would often ask me and other students to write a paper about some topic. So let’s say the topic is the Great Pyramid of Egypt. Now probably most students don’t know everything about the Great Pyramid and the teacher says anyway, “You have to cite sources”, so you can’t just write anything you want.
Roland Meertens: I always hate this part. Yes, I always say, “I found this on the internet. These people can’t lie”.
Anthony Alford: Well, I’m talking about the days before the internet. But in the 20th century, let’s say, we would go to the library, an actual physical building, and there would be a big drawer full of small cards, the card catalog. These are in alphabetical order, and so we’d scroll through till we get to the Ps and then P-Y, pyramid. Great. Pyramid of Egypt, right?
Roland Meertens: Yes.
Anthony Alford: This card has a number on it. This is the call number for books that are about the Great Pyramid of Egypt. So in the US a lot of libraries use a catalog system called the Dewey Decimal system for nonfiction books. It’s a hierarchic classification system.
Books about history and geography in general, they have a call number in the range from 900 to 999. Within that, books about ancient history are in the range of 930 to 939. Books about Ancient Egypt specifically have call numbers that begin with the number 932. And then depending on what ancient Egyptian topic, there will be further numbers after the decimal point.
Roland Meertens: And maybe a weird question, but were you allowed to go through these cards yourself or did you ask someone else like, where can I find information about Ancient Egypt?
Anthony Alford: Both methods do work. If you’re young and adventurous, perhaps you’ll go to the card catalog and start rifling through. But yes, in fact, a lot of libraries had a person whose job was to answer questions like that: the reference librarian.
Roland Meertens: Yes, because I’m too young, I never saw these cards. My librarians would already have a computer they would use to search.
Anthony Alford: Right. But the point there is that the card catalog is pretty familiar to us in—speaking of search, the card catalog is an index. And it maps those keywords like Great Pyramid of Egypt, it maps those keywords to a call number or to maybe multiple call numbers.
Actually, university libraries, in my experience in the US, they don’t use Dewey Decimal, they use a different classification, but the idea is the same. Anyway, it’s a hierarchy and it assigns a call number to each book.
So to go actually get the physical book, it’s hopefully on a shelf that’s in a cabinet. We call these stacks. That’s the lingo. So the classification hierarchy is itself mapped physically to these stacks. There will be a row of cabinets for the books that are in the 900 to 999 range and maybe one cabinet for the 930 to 939, and then maybe one shelf for 932 and so on. Now that I think of it, this structure is itself somewhat like a pyramid.
Roland Meertens: Perfect example.
Anthony Alford: Hopefully if nobody’s messed with them, the physical order of the books matches the numeric order. So you’re doing an index scan or index search if we’re thinking about it in terms of a database or information retrieval. Because that’s what this is, it’s literally information.
Roland Meertens: Yes. And it is good that it’s indexed by topic because otherwise you don’t know if you’re searching for P for pyramids or G for great pyramids or E for Egyptian great pyramids.
Anthony Alford: Right. If you’re not talking to the reference librarian, you might try all those keyword searches in the index. So now that I’ve got a couple of books, I can use that content in those books to help me produce my essay about the Great Pyramid.
Now that was the bad old days of the 20th century. Here in the 21st century, it’s like you said: you do an internet search or maybe you read Wikipedia. That’s just the first quarter of the 21st century. Now we’re into the second quarter of the 21st century, and we’re in a golden age of AI. We don’t have to even do that. We just go to ChatGPT and copy and paste the assignment from the syllabus web page as a prompt and ChatGPT writes the essay.
Roland Meertens: Quite nice, quite neat.
RAG Time [07:35]
Anthony Alford: Well, in theory. So there’s a couple of problems. First, the teacher said, “Cite your sources”, and you have to do that—in the content where maybe you quote something or a reference you need to put in there. Another thing is ChatGPT is good, but maybe it’s not always a hundred percent historically accurate.
Roland Meertens: Yes, it sometimes makes up things.
Anthony Alford: And it really only knows things that are in its training data, which is large, but maybe there’s some really good books or papers that are not on the internet that might not be in that training data. So I think you know what is the answer.
Roland Meertens: Are we going to retrieve some data before we’re processing it?
Anthony Alford: Yes, it is RAG-time. So the key technology now is retrieval augmented generation, also known as RAG, R-A-G. So the general idea, we know that if we give an LLM some text, like the content of a history book, LLMs are quite good at answering questions or creating summaries of that content that you include with your prompt.
Now ignore the problem of limited context length, which is a problem. The other problem is: how do you know what content to provide it?
Roland Meertens: Yes, you can’t give it the entire stack of books.
Anthony Alford: Exactly. And even if you had the content electronically, and you had picked it out, you want to automate this, right? You don’t want to have to go hunt down the content to give to the LLM.
So finding the right history book, the right content in an electronic database of content, well, we already said it. This is information retrieval. And again, in the old days we’d use natural intelligence: we would use the reference librarian or go look up some keywords in the card catalog.
Roland Meertens: It is too bad that the librarian is not very scalable.
Anthony Alford: Exactly right. We want to automate this and scale it. So let’s take an analogy. The key idea of RAG is: take your LLM prompt and automatically assign it a call number. So now you can go directly from your prompt—your instructions for writing the essay—now we have automatically assigned it a call number, and now you just go get those books automatically and add that with your prompt.
Roland Meertens: Sounds pretty good.
Anthony Alford: Yes, more precisely: we have an encoder that can map an arbitrary blob of text into a point in some vector space with the requirement that points that are near each other in this space represent texts that have similar meanings. So typically we call this an embedding.
So we take an encoder, we apply the encoder to the prompt that turns that into a vector. Then we have all of our books in the universe, we have encoders applied to them, and we get vectors for them. We find the vectors that are close to the vector for our prompt. So easy-peasy, right?
Roland Meertens: Easy-peasy.
Anthony Alford: Right. Well, here’s the problem. So the encoder-
Roland Meertens: Encoding your data.
Anthony Alford: Well, there’s that. Well, I’m just going to assume somebody encoded all the books in the library. That’s a one-time job. The problem is that people usually use BERT as the encoder. Well, the embedding vector that you get is 768 dimensions. And so the question is: what does it mean to be nearby something in a 768-dimensional space?
Roland Meertens: Yes, that depends on what distance function you want to use.
Anthony Alford: That’s exactly right. With call numbers, it was easy because they’re scalars. So the distance function is: subtract.
Roland Meertens: Oh, it’s quite interesting. I never even realized that call numbers could be subtracted.
Anthony Alford: Well, that’s how you do it, right? If you go to find your book 932.35, you probably don’t do a scan. You probably do some kind of bisecting search, or you know you need to go over to the 900s, and then you jump to the middle of the 900s and scan back and forth depending on the number that you’re at.
Roland Meertens: And also for the library, it of course makes sense that they put books which are similar close together.
Anthony Alford: Yes, well, you physically store them in order of their call number.
Roland Meertens: Yes.
Cosine Similarity [12:04]
Anthony Alford: More or less. Anyway, like you said, this distance, the closer they are to zero, like the closer the two call numbers are together, physically the books are closer together.
So anyway, we need a distance function, or the opposite of a distance function, which is a similarity, right? The smaller the distance, the more similar. In the case of these embeddings, people typically use a similarity measure called cosine similarity. Now, if you’ve ever worked with vectors, you probably remember the inner product or sometimes called the dot product.
To explain this without a whiteboard, let’s say we’re in 3D space. So each vector has X, Y, and Z. The dot product of two vectors is you take the X from the first one, multiply by the X from the second one. Then you do that for the two Y components, and then the two Z components, you add those all up. That’s the dot product. And that’s a single number, a scalar.
Roland Meertens: Yes.
Anthony Alford: The geometric interpretation of the dot product is: it’s the length of the first one times the length of the second, and then times the cosine of the angle between them. So you could divide the dot product by the length of the two vectors, and what you’re left with is the cosine of the angle. And if they’re pointing in the same direction, that means the angle is zero and the cosine is 1. If they point in the opposite directions, the cosine is -1. And in between there, if it’s zero, they’re at right angles.
Roland Meertens: Yes, intuitively, you always think that it doesn’t really matter what the magnitude of the interest is, as long as the interests are at least in the same direction, it is probably fine in your library.
Anthony Alford: Yes, and I’m going to explain why. Anyway, the cosine similarity is a number between -1 and +1. And the closer that is to +1, the nearer the two embeddings are for our purposes. And you may wonder why cosine similarity. So again, with 3D space, X, Y, and Z, there’s a distance called the Euclidean distance, which is our normal “The distance between two points is a straight line”, right?
Roland Meertens: Yes.
Anthony Alford: So you basically take the X is the square of the Y is the square of the Zs, add them up and take the square root.
Roland Meertens: As long as we are in a Euclidean space, that’s the case.
Anthony Alford: And in vector terms, that’s just the magnitude of the vector drawn between those two points. Well, if you wonder why you don’t use that, why instead you use cosine similarity, if you look on Wikipedia, it’s something called the curse of dimensionality.
Basically, when you have these really high-dimensional spaces, and if the points are uniformly spread around there, they actually aren’t. The middle of the space and the corners of the space are empty-ish. And most of the points are actually concentrated near the surface of a sphere in the space.
So when all the points are on a sphere, their magnitudes are more or less all the same. And so you don’t care about them. And so the thing that makes them different points is there are different angles. They are at different angles relative to some reference. So that means we don’t care about the magnitude of vectors in the space, we care about the direction, and that’s why cosine similarity.
Roland Meertens: Is there any reason that the magnitude of the vectors tends to be the same?
Anthony Alford: It’s just the way that these sparse high-dimensional spaces…it’s just the math that they work out. And in fact, because the magnitudes are all more or less the same or at least very…you can take a shortcut, you can just use the dot product. You don’t have to get the cosine similarity, you can just do the dot product That’s a nice shortcut because GPUs are very good at calculating dot products.
And so let’s back up, right? We take our prompt, we encode it, we’ve already encoded the content of all the library. We just find the vectors in the library that have the largest dot product with our prompt vector. And in the original RAG paper they did that. It’s called maximum inner product search. So basically you take your queries vector, you do the dot product with the vector of all the documents and take the ones that have the biggest.
What’s the problem now? I bet you know.
Roland Meertens: What is the problem?
Anthony Alford: Well, the problem is you have to—basically every time you have a new prompt, you have to go and calculate the dot product against every other document.
Roland Meertens: If only there was a better way to store your data.
Who Is My Neighbor? [16:50]
Anthony Alford: Well, there’s a better way to search it turns out. The default way is linear complexity. So for a small library, it may be no big deal, but if we’re talking about every book ever written, well, if you compare it with index search in a database, that’s complexity around log(n). So linear is way worse. It’s terrible. So again, it turns out this is a well studied problem and it’s called nearest neighbor search.
Roland Meertens: Approximate nearest neighbors or exact nearest neighbors?
Anthony Alford: Well, one is a subset of the other. So if you go back to the database search, that’s log(n), and you can actually use a tree structure for nearest neighbor search. You can use something called a space partitioning tree and use a branch and bound algorithm. And with this strategy, you’re not guaranteed log(n), but the average complexity is log(n). But this usually is better in a lower dimensional space.
Roland Meertens: Okay, so why is it on average? Do you keep searching or-
Anthony Alford: Well, I think it is just like you’re not guaranteed, but based on the statistics, you can mathematically show that on average you get a log(n) complexity. But remember your favorite algorithm-
Roland Meertens: My favorite algorithm.
Anthony Alford: What was your favorite algorithm?
Roland Meertens: HyperLogLog.
Anthony Alford: Right. So, you already said it, approximate nearest neighbor. When you want to do things at scale, you approximate. So it turns out that a lot of RAG applications use an approximate nearest neighbor search or ANN, which also stands for “artificial neural network”. But just a coincidence.
So there are several algorithms for ANN and they have different trade-offs between speed and quality and memory usage. Now, quality here is some kind of metric like recall. So with information retrieval, you want to get a high recall, which means that of all the relevant results that exist, your query gives you a high percentage of those.
One of the popular algorithms lately for ANN is called hierarchical navigable small world, or HNSW. HNSW is a graph-based approach that’s used in a lot of vector databases. I actually wrote an InfoQ news piece about Spotify’s ANN library, which uses HNSW.
Roland Meertens: Oh, is it Voyager?
Anthony Alford: That’s correct, yes. You must have read it.
Roland Meertens: Oh, I tried it. It’s pretty cool.
Anthony Alford: Oh, okay. Well, you know all about this stuff.
Roland Meertens: Oh, I love vector searching.
Anthony Alford: So I found a nice tutorial about HNSW, which we’ll put in the show notes, and it expressed a very nice definition, concise:
Small world, referring to a unique graph with low average shortest path length, and a high clustering coefficient navigable, referring to the search complexity of the sub graphs which achieve logarithmic scaling, using a decentralized greedy search algorithm and hierarchical, referring to stacked sub graphs of exponentially decaying density.
So all of this to find out: who is my neighbor?
Roland Meertens: Who is your neighbor, and in which space are they your neighbor?
Anthony Alford: Yes. So I think I’ve filled up my context window for today. And for homework, I will let our listeners work out analogies between this topic and library stacks and pyramids.
Roland Meertens: For library stacks, I’m just hearing that they could have multiple boxes with the stacks and you just move from box to box, from room to room.
Anthony Alford: So here’s a very interesting thing. Here in my hometown, there’s a university, North Carolina State University, their engineering library has a robot that will go and get books out of the stacks. It’s basically an XYZ robot, and it’ll move around and get books out of the stacks for you.
Roland Meertens: Oh, nice. That’s pretty cool.
Anthony Alford: Yes, it looks really cool.
Roland Meertens: Always adding an extra dimension, then you can represent way more knowledge.
Anthony Alford: So that’s my fun fact.
Roland Meertens: That’s a pretty good fun fact.
Real World Coordinate Systems [22:02]
Roland Meertens: All right. For my fun fact for today, as the topic is coordinate systems, for software there’s many ways to represent a map in location software. So this can be important for your user data, maybe for helping with people and finding where they are, finding interesting locations close by, and the most popular format here is WGS84.
But what I wanted to dive into is the history of coordinate systems, especially how different countries chose them, and some of the legacy systems which are still in place because the history of coordinate systems, and of course longer than just computers, people wanted to know who owned what land for quite a long time, people wanted to know how to get somewhere for quite a long time.
And, first of all, there’s different ways to project a map. So you want to have a map in 2D, and our Earth is a sphere. In that way, you can project a sphere onto a cylinder, a cone, or just a flat disc on top of the sphere, and you always get some kind of compromise.
So you can choose to keep the angles of the map accurate. That’s, for example, the Mercator projection used by Google Maps. So if you’re going on Google Maps, you’re zooming out, then all the angles are preserved, but the sizes are not very true.
One fun question, by the way, maybe you can help me out with this, Anthony, is that I always ask what is bigger, Greenland or Australia, and by how much?
Anthony Alford: Oh, Australia is quite large. And again, I think the Mercator projection distorts our view of Greenland for those of us who are familiar with it. Australia is much larger, but I couldn’t tell you like by a factor or whatever.
Roland Meertens: Yes, I like to ask this to people because first I ask them what they would estimate and then I show them the Google Maps projection and I ask them if they want to change their guess. And sometimes people change it in the wrong direction, even though they know that Mercator doesn’t preserve size, even though they know that the map is lying. They just can’t get around the fact that Greenland looks really big on the map.
So if you want to fix this, you can use the Mollweide equal-area projection to ensure that all the map areas have the same proportional relationships to areas on the Earth. And the other thing you can do is if you want, for example, to keep the distance constant, there are equidistant projections that have a correct distance from the center of the map.
So this is useful for navigation, for example, if you want to have something centered around the UK that you at least know if I want to go here, it’s equally far as if I want to go here. And here, another fun fact for you is that azimuthal equidistant projection is the one they use for the emblem of the United Nations: this emblem where you see this map from the North Pole, that is an azimuthal equidistant projection where the distance is constant.
Anthony Alford: Okay, nice.
UK Ordnance Survey Maps [25:27]
Roland Meertens: But as I said, I wanted to talk a bit about other systems in the world and which projection they pick and perhaps some of the technical depth and incredibly smart choices they made when doing so.
And, first off, in the UK they have the Ordnance Survey Maps. It’s basically the national mapping agency for Great Britain. And in a previous episode of Generally AI, I already told you about multiple telescopes in the observatory in Greenwich, right?
Anthony Alford: Right. Yes.
Roland Meertens: And I think I also told you that they have multiple telescopes which all have a different prime meridian line, which indicates zero or used to indicate zero. I discovered that the Ordnance Survey meridian was picked in 1801, which is 50 years before this newer prime meridian was released. And nowadays with GPS, the prime meridian moved again. But the Ordnance Survey Maps are basically two prime meridian switches away from what it used to be.
Anthony Alford: I don’t know, but I’m guessing from the name that they would, in the worst case scenario, use these maps to choose targets for artillery. So hopefully they don’t miss.
Roland Meertens: No, actually what I think is probably a good reason to keep the Ordnance Survey Maps the same is that they probably use it to determine whose land belongs to whom.
Anthony Alford: Sure.
Roland Meertens: So you want to be able to keep measuring in the old way as you already determined who owns what land.
Anthony Alford: Makes sense.
Roland Meertens: Otherwise, but we will see this later in this episode, you start publishing error maps like the Netherlands is doing. But it’s interesting that since 1801, when they picked this survey meridian, they were for a long time simply six meters to the east of what people started to call zero for a long time.
I can also imagine that this is still confusing nowadays if people use their own GPS device and compare it to some older document from the 1800s and discover that their place is very much farther away from where they thought it should be. But I’ll post an article to this Ordnance Survey Zero Meridian in show notes.
Netherlands Triangle Coordinate System [27:49]
Roland Meertens: Anyway, moving to a different country, in the Netherlands, the geographic information system, the GIS system, is called Rijksdriehoekscoördinaten. So it’s a “national triangle coordinate system”. And as you can already guess, this mapping is accurate in angles and Wikipedia says it approaches being accurate in the distances, so it’s not accurate in distances.
Anthony Alford: Oh, I see. And so I guess it’s basically you need to orient in the right direction, but the distance is approximate? Is that-
Roland Meertens: Well, the thing is that if you have these coordinates, the angles between your coordinates are the same as the angles in the real world.
Anthony Alford: Cosine distance!
Roland Meertens: Yes. So the coordinates are in kilometers and then meters, right? It’s just that one kilometer in coordinates isn’t a kilometer in the real world. So one kilometer on the map in coordinates isn’t necessarily one kilometer in the real world. So the center of the map is a church in Amersfoort, so basically in the center of the Netherlands. Around there, the scale is 10 centimeters per kilometer too small.
Anthony Alford: Interesting.
Roland Meertens: Yes, I mean, it’s not a big error, it’s just only 10 centimeters.
Anthony Alford: This reminds me again of the last season where the king found out that his land was smaller than the map said it was.
Roland Meertens: Yes. So if you would take the Dutch triangle coordinate system and then determine that you’re going to walk 10 kilometers in the center of the Netherlands, you would have walked one meter too little after walking 10 kilometers.
Anthony Alford: Would you even notice though, right?
Roland Meertens: Indeed, you probably wouldn’t. On the edges, so if you go towards the coast areas into Germany, it’s 18 centimeters per kilometer too large.
Anthony Alford: So you could wind up in Germany and not know it…or would you know it? You might know.
Roland Meertens: You will find out that you’re crossing the border because it says you’re crossing a border.
Anthony Alford: Well, wait, Schengen, you guys are all…you just walk, right?
Roland Meertens: Yes, from where my parents live, you can very easily cycle to Germany. But it’s interesting that because you have such a small country, you can project things in a flat way and-
Anthony Alford: And the country is rather flat as well, I believe.
Roland Meertens: The country is rather flat as well. Yes, indeed. I will get to the height of the Netherlands actually, because that’s also interesting because they use different landmarks than the landmarks used for the triangle coordinate system.
Anthony Alford: Okay.
Roland Meertens: So as I said for the triangle coordinate system, the center of the coordinate system, let me tell you a fun fact about that first. So that’s a church in Amersfoort. And if you look at the coordinates, there’s an X and Y component where X goes from west to east and Y goes from south through north. That’s relatively simple.
But the X coordinates are between zero and 280 kilometers. The Y coordinates in the Netherlands are between 300 and 625. So (0,0) is basically somewhere to the north of Paris. And the nice trick here, which I think is just genius, is that all the coordinates in the Netherlands are positive and the Y coordinates in the Netherlands are always larger than the X coordinates-
Anthony Alford: Interesting.
Roland Meertens: … unlike continental Netherlands. So this removes all the possible confusion around what coordinate. So if I give you two coordinates, I don’t even have to tell you this is X, this is Y.
Anthony Alford: Got it.
Roland Meertens: I can turn them around, I can flip them around. Because as a software engineer, whenever it says coordinates, you get two numbers. I always plot latitude, longitude, trying out combinations to make sure that everything is correct. And here in the Netherlands, if only people would use the national triangle coordinate system, there would be no confusion in your software.
Anthony Alford: Is that a thing that most Netherlanders are aware of?
Roland Meertens: Probably not. I must also say that this coordinate system is not used a lot. Probably mostly for people who are doing navigation challenges or scouting or something.
Although I must say that it is quite nice to take one of those maps because they are divided in a very nice way. It’s very clear how far everything is because with latitude and longitudes, the distance between one latitude or one longitude is different depending on where you are on Earth, right?
Anthony Alford: Yes. But there’s a conversion to nautical miles, but I can’t remember it off the top of my head.
Roland Meertens: That’s a good point. I wanted to say in the Netherlands it’s fixed, but we just learned that it’s 10 centimeters per kilometer too small in the center and 18 centimeters per kilometer too large in the edges.
Anthony Alford: But originally part of the development of the metric system was to take the circumference of the Earth and make fractions of it to be the meter originally. I don’t think it worked out.
Roland Meertens: I think there’s also a map system where they try to keep the patches the same area, but then you get problems when you want to move from patch to patch. So if you have coordinates or if you have a route which crosses multiple patches, one point on one patch doesn’t necessarily map to the same place on another patch.
Anthony Alford: It’s a tough problem.
Roland Meertens: Yes, and that’s why I like to talk about it. It’s a lot of technical depth and it becomes more difficult once you start doing things with software or self-driving cars or things like that.
In terms of technical depth, the original map of the Netherlands was made between 1896 and 1926. And as you can imagine, we now have way more accurate mapping tools, but I already alluded to the fact that if you already mapped out a place and you say this is your property, you can’t really say, oh, there’s a new coordinate system, let’s go measure everything again and assign this again.
So what they do in the Netherlands, I think on three different occasions they published a correction grid with corrections up to 25 centimeters. So you can take an original coordinate and then apply the correction grids to get the coordinates in what is actually measured.
Anthony Alford: Gotcha. Well, not to derail your talk, but here, again, in North Carolina we have a border with another state, South Carolina, and about 10 years ago they had to adjust it. Basically the border had become ambiguous. It was unclear where it actually was. And so they fixed it and agreed on where the border is. And there were some people who woke up one morning in a different state without having to move.
Roland Meertens: I can tell you one other fun fact about borders in the Netherlands and between Germany and that is that in the Netherlands after World War II, there were some proposals around like, can we maybe have some part of Germany to make up for the Second World War?
So they got a few parts of Germany, but those are super small regions like a village or something. And this wasn’t really working out, taking a long time to move people, make sure everything was working well, build schools, et cetera.
So at some point they gave it back, but then weeks before they were giving back this country, big trucks would already start moving in with loads of goods in them. They would find places in the village to park and hours before this transition happened, big trucks would show up with loads of butter inside. So basically at 12 o’clock at night, the country swaps and these goods never crossed a border, so they didn’t have to pay taxes.
Anthony Alford: Loophole.
Roland Meertens: Yes. So they found a loophole which you could only do one night because some parts changed country overnight.
Anthony Alford: Interesting.
Roland Meertens: One last fun fact here about coordinate systems. You already said the Netherlands is quite flat. Good point. But this grid only tells you XY coordinates and it’s mostly based on locations of church towers to measure angles between. So it’s quite neat. Those are relatively consistent places and you can see between them.
There’s a separate mapping for height above sea level, the new Amsterdam Ordnance Datum, and this is actually used in a lot of Western European countries. And these points are indicated by screws on specific buildings. And I know this because once in high school we had to make an accurate map of a field close to a school and I was tasked to propagate the height from this screw to the rest of the field.
Anthony Alford: Wow.
Roland Meertens: We actually had these systems they use in professional area measuring setups.
Anthony Alford: The surveying tools…a transit.
Roland Meertens: There was something where something was perfectly flat and then we would stand somewhere with a height meter, measure the difference in height, place the measuring device somewhere else, have the person with the height meter stand somewhere else.
We also had to do it twice because the first time we made a mistake, I don’t know anymore what we did, but it’s just teenagers trying to come up with a way to measure a field.
Anthony Alford: Very cool.
Words of Wisdom [37:40]
Roland Meertens: All right. Words of wisdom. Did you learn anything in this podcast or did you learn anything yourself recently?
Anthony Alford: The fact that all the points in a high dimensional space are on a sphere was new to me. Maybe not all, but the fact that they all more or less have similar magnitude. That was an interesting fact that I was not aware of.
Roland Meertens: You would say that that means that there is space in the high dimensional space left over. The place in the middle and the corners could be utilized to store more information.
Anthony Alford: One would think, but then that would mess up the assumption of the cosine distance.
Roland Meertens: Yes, but more space to store. It’s free. It’s free storage.
Anthony Alford: Just add another dimension.
Roland Meertens: Yes, that’s why I always throw all my stuff on the floor in my room. I pay for it, I can store it wherever I want, everywhere in the space.
Anthony Alford: Definitely.
Roland Meertens: One thing from my side in terms of learning things, one recommendation I want to give you is, have you heard of the post office scandal in the UK?
Anthony Alford: No. Tell me.
Roland Meertens: It’s quite interesting. So the post office in the UK adopted a bookkeeping system by Fujitsu called Horizon, and it was basically plagued with bugs. Sometimes the system would duplicate transactions, sometimes it would change some balance when users would press enter at some frozen screen multiple times. So you’re like, oh, it’s frozen…let’s press enter.
Every time something would happen with your balance.
And it was possible to remotely log into the system. So Fujitsu or Horizon could remotely change the balances on these systems without the postmasters knowing. And I learned last week that rather than acknowledging these bugs, these postmasters were sued for the shortfalls in the system because the system would say, you owe us £30,000.
Anthony Alford: Oh, wow.
Roland Meertens: Yes. And so these postmasters were prosecuted, got criminal convictions, and this is still going on and still not fully resolved today.
Anthony Alford: That’s terrible.
Roland Meertens: It is absolutely insane. So I watched this drama series called Mr. Bates versus The Post Office, and I can definitely recommend you to watch this because it tells you a lot about impact your software can have on individuals and to what great length companies are willing to go to hide the impact of bugs or systems like this.
Anthony Alford: Goodness gracious.
Roland Meertens: Yes, it’s insane. We can do a whole episode about the post office scandal I think.
Anthony Alford: That would be depressing.
Roland Meertens: Yes, but I must say it’s very interesting. Every time when you read about this and you think, surely by now they will acknowledge that there can be problems like this, the post office just doubled down, hired more lawyers, created bigger lawsuits, and absolutely ruined the lives of people who were postmasters in the last 20 years actually.
Anthony Alford: Wow.
Roland Meertens: As I said, can recommend this as a thing to watch.
Anthony Alford: Sounds good.
Roland Meertens: Anyways, talking about recommendations. If you enjoyed this podcast, please like it, please tell your friends about it. If you want to learn more things about technology, go to InfoQ.com. Take a look at our other podcasts, take a look at our art course and the conference talks we recorded. Thank you very much for listening and thank you very much, Anthony, for joining me again.
Anthony Alford: Fun time as always.
Roland Meertens: Fun time as always. Thank you very much.
Anthony Alford: So long.
Roland Meertens: Any last fun facts you want to share?
Anthony Alford: Well, I don’t know if we want to put this one on the air, but I was looking at how property is described here in the US in a legal document. So you may know, you may not, that we have a system called Township and Range, and I think it was invented by our President Thomas Jefferson.
After our Revolution, we had all this land that legally speaking was not owned by anyone. So they divided it up into a grid. They laid a grid out over it. So here’s a description of a piece of property:
Township four north, range 12 west. The south half of the north half of the west half of the northeast quarter of the northeast quarter of the north half of the south half of section six.
Roland Meertens: Okay. Yes. So they made a grid and then they went really, really, really, really deep.
Anthony Alford: Subdividing the grid. Yep.
Roland Meertens: Yes, I do like that. When people started mapping this, they were probably like, ah, there’s so much land. It doesn’t really matter how accurate this is. Probably North US, South US is probably enough.
Anthony Alford: Well, what’s interesting, a surveyor was sort of a high status job in the colonial days. George Washington was a surveyor, and Thomas Jefferson amused himself by designing buildings. So these guys took it pretty seriously. That was the age of the Enlightenment and Renaissance men and all that.
Roland Meertens: But if you are not good at mapping, you don’t come home on your ship.
Anthony Alford: Yes, exactly.
Roland Meertens: And if there’s no maps of roads or you don’t know where you are, you don’t reach the village you wanted to get to.
Anthony Alford: Exactly.
Roland Meertens: Yes. Interesting.
Mentioned:
.
From this page you also have access to our recorded show notes. They all have clickable links that will take you directly to that part of the audio.
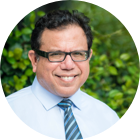
MMS • Milena Nikolic
Article originally posted on InfoQ. Visit InfoQ
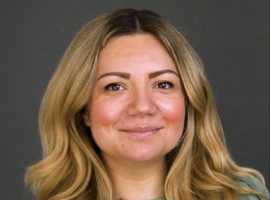
Transcript
Nikolic: I’m going to talk about the lessons we had from scaling Trainline’s architecture. Scaling will mean the usual things around like, how do we handle more traffic? That’s not the only thing we’re going to talk about. I’m also going to talk about the things we have done to make it possible to have more engineers work on our architecture at the same time, so we can speed up the pace of growth and the pace of innovation and all that. I’m also going to talk about scaling the efficiency of the platform itself, so that as the platform grows, it grows in a way that’s efficient, and cost efficient, and all that. You’ll get to hear about business lens of productivity and team impact. Business lens of cost efficiency, and that side of financial business impact, as well as actual traffic growth and being able to handle more business.
Trainline (The Rail Digital Platform)
How many of you know what Trainline is? We are Europe’s number one rail digital platform. We retail rail tickets to users all around the world for rail travel in UK, Spain, France, Italy, really most of Europe. In addition to retailing tickets, we also follow users for the entire rail journey. We are with them on the day helping them with platform information. If there are any disruptions, helping them change their journeys, getting compensation for delays, as well as if there are any planned changes in terms of what they want to do with their ticket.
We provide all of that through our B2C brand, trainline.com, as well as a white label solution to our partners in the rail carrier space, as well as to other parties in the wider travel ecosystem, your GDSs, your TMCs, anyone else in the travel ecosystem who wants to have access and book rail. I’m going to mention a couple of numbers. I’m going to just give you just as a teaser of roughly size of the business, and then some numbers related to the traffic, although we will talk much more about that as we go through the presentation. Then also just the team. I think that gives you an idea of what the company is, and technically what we’re trying to solve.
Just to give you an idea in terms of size of business, we’re a public company. We’re well established, profitable. Last year, we traded about 5 billion net ticket sales. Just gives you an idea of the scale in terms of technical impact as well. It’s very difficult to pick one technical number that represents things, but I have just decided that maybe I show searches, which is, we do around 350 searches per second for journeys and for origin destination pairs. We do that over something like 3.8 million monthly unique routes. If people look for Milan, Rome, or Cambridge to London, or wherever else, that gives you a problem space of search. Then we have about 500 people in tech and product organization out of which majority is tech obviously. If that’s not cool enough, this is cool, isn’t it? I love this innovation.
This just gives you an idea of the problem space in the sense of, we know where each live train in Europe is at any given point in time. A, that’s a lot of data. B, it’s a lot of actions that we need to do. Every time when you see these yellow or red dots, it means a train is delayed or getting canceled or getting changed or something like that. Which means we need to do something with the customers that have tickets for that train, that we sold tickets for that train to notify them in a certain way, and all that. This might be just a graphical representation of some of the scale of the problem and effectively what we’re dealing with.
What’s Difficult About What We Do?
I’ve been at Trainline now, in July it’s going to be three years. I remember as I was joining, I was like, I love trains. Very cool. Just super happy to be doing this. I was like, what do actually 450 engineers actually do? Two of them build the app, two of them build the backend, what does everyone else do? Obviously, I knew there was a bit more, but I was like, I’m not sure this is actually such a hard problem to be requiring that many people. Then, I think over time, or certainly even within first couple of months, it became obvious that certain problems were harder than I thought. I’m going to talk about three of them. There’s certainly not an extensive list of everything that’s hard about it. These are the few where I was like, actually, that’s harder than I thought. The first one is aggregation of supply. We have more than 270 API integrations with individual rail and bus providers.
There is zero standardization in this space. You literally have one-off integration. There is no standardized API model, for any of you that know airline industry, for example, with the GDSs, like Amadeus have done 30 or 40 years ago, which involved standardizing all the airline booking APIs. That didn’t happen in rail. It’s like a lot of very custom integrations. As you can imagine, that comes with problems of high maintenance cost, because everyone is constantly updating their APIs. Non-trivial integration every time when you need to add a new carrier, a new rail company launches. Then I would say, even worse, journey search and planning, combined journeys over the very inconsistent and disintegrated set of APIs, is a problem that needs to be solved, because everyone has different API access patterns but equally different limitations.
For example, we get things like look to book ratios, where we can only search and hit certain API, within the certain limit of proportion compared to how many bookings we do through that company, as well as sometimes pretty strict and very old school rate limits in terms of what we can hit. A lot of complexity comes from that core idea that is ultimately the purpose of Trainline, which is the aggregation of all of the supply. One more thing that’s related to that is that Europe has 100 times more train stations than airports. Just also in terms of scale, there’s a lot here. Getting those journey searches to work, especially combined across multiple APIs and getting them to work fast, that was the problem. Then when I joined, I was like, actually, that is harder than I thought.
The second one, whereas I think this level of aggregation of supply might be somewhat unique to the rail industry aggregation, transactions over a finite inventory are not. I suspect many of you have worked on something similar at a certain stage, like the classic Ticketmaster problem, where you’re not just selling something. In my previous career at Google, I was running part of Google Play Store. We were selling apps, which involve certain complexity, but it’s a digital product, so there is no limited number. Then we can sell as many apps as we want, or as many in-app products as we want. It’s all digital, and you don’t need to check the inventory or anything like that. Right now, we’re selling seats on unique trains, in unique class with a unique fare. It’s quite limited. You can understand that transactionally they’re just much harder to solve, to make it reliable, to make it fast, and all that.
Right now, we handle about 1300 transactions per minute at peak times. That’s up from zero in COVID times. It’s also up from, I think, probably something like 800, close to 3 years when I joined. It has been growing. Some of it as part of rail travel recovery as the economy was recovering post-COVID, and some of it as we were growing, and especially as we were growing in Europe. Does that resonate? Did any of you deal with transactions of a finite inventory and get the complexity of that? I don’t know if everyone else is thinking like, super easy, I don’t know what you’re talking about. There is a layer of complexity here that comes with it.
Then the final thing that also ended up striking me at that early stage that was harder is the speed at which people expect you to fulfill a ticket, to literally give them a barcode they can scan as they walk through the barrier gates. The expectations are high. It has to be within a second. It has to be basically instant. That doesn’t apply. When we buy rail travel, we usually buy it for a couple of months in advance, at best couple of weeks in advance. Usually, that email with an actual ticket arrives couple of hours later. It very rarely arrives instantly. We don’t have that.
Right now, about 60% of all of our tickets bought are bought on the day. Quite a lot of those are actually bought literally as people are walking into Charing Cross station and getting the ticket on Trainline and scanning it straight away. From the point of completing transaction until people having the barcode they can scan on the barrier gates, and that involves interaction with industry level processes, so that the barrier gates can recognize the ticket is valid. Those expectations are pretty high.
3 Lessons on Scaling
That’s some of the taste of some of the things that are hard. Now I’m actually going to go to the juice of the talk, which is going to be three lessons we had on scaling. Then again, as I talk about this, keep in mind, the first one is going to be more around team and productivity, and ultimately, the impact of architecture, both how it enables us and how it slowed us down, as we were scaling team sizes and then changing our teams. The second one is going to be on the cost efficiency and scaling the efficiency of the platform. The third one is going to be on scaling with growth in traffic and achieving higher reliability, or dealing with availability issues and reliability issues. Each one of the lessons, I’m going to start with a story. You need to follow me with the story. Then we’ll eventually get the lesson. Some of these are pretty obvious, some of these are a bit more nuanced. It’s going to take a second to get there.
1. Scaling Team Productivity
When I joined back in July 2021, it was really interesting, I think we had probably about 350 engineers. They were entirely organized in this cluster model. Do people know what cluster model is? It basically means organizing your teams around ownership of the parts of technical stack. We had Android app team, an iOS team, and web team, gateway or like Backend For Frontend team, e-comm, supply, a couple of different supply teams, order processing. All the teams were organized around that part of ownership of the technical surface, which is a way to organize teams. I’ve seen it in other places as well. At that size and scale, 350, and we were growing by another 100 I think over that year, it didn’t work well. The productivity of the team was pretty low. This was very quickly obvious as I started looking, almost any project that you want to do involved at least 5, and often up to 10 different teams.
This was this very old school way of delivery managers managing massive, mega spreadsheets with dependencies and trying to plan, trying to estimate everything up front, and who’s going to hand over to whom when, in which sprint. Obviously, everything will constantly get late, and everything would take three times longer to build. It was pretty slow. I was like, at this scale, this is not the right model for the team and what we need to do here.
In, I think, January 2022, we did a massive reorg of the team where we basically threw the entire structure, and created this structure that’s also fairly standard in industry called platform and verticals. We had platform or horizontals owning technical stack, and verticals having all the people with different skill sets, your Android and iOS and web and .NET engineers and Ruby engineers and everything you would need, shaped around clear ownership of product and business goals. That was the big change we ran around that. Then we had it for about two years. I think that served us well and served a certain purpose that it had. I’ll come into that for a second. It certainly improved alignment to the team to goals, and how much any random engineer would care about delivering something that makes a difference to the business.
It obviously came with other challenges, like models like these always comes with this challenge of tension between platform teams and vertical teams, where vertical teams are trying to land stuff as quickly as they can, as they should. Platform teams are always pushing back and saying, no, you’re hacking that up. No, you need to do it properly. Can you please also refactor this while you do it and get rid of that tech debt, and all that. I think that tension is embedded function of the model, and a good thing to be having. Sometimes it can be frustrating to people on both sides, as you can imagine. Literally just now, a couple of months ago, we have again reorganized in a slightly less drastic way. We have reduced the ownership of the platform just to very core services that are absolutely touched and shared by everyone.
Then the other 50% of the tech surface, went into the verticals which we then renamed diagonals. That’s the model we’re trying now, and that was because we felt we’ll get the best parts of platform and verticals, but we’ll also be able to streamline and remove some of that tension that was happening previously between platform and verticals. I can give a whole talk about this set of organizational changes. That’s not what I’m doing here. I want to talk about the architectural implications of this set of changes and what it means because I think that’s more relevant.
The key question here with all three of the models is effectively to like, who owns each part of the technical surface? Who’s in charge of all the sustain work, mandatory technical upgrades? Who’s in charge in terms of driving the technical strategy and the vision for that part of the code base, moving you to the new patterns in the latest trendy JavaScript framework? No, we’re not doing that. That’s definitely not happening. Who effectively does all that core work both to sustain as well as to drive the technical roadmap? In addition to who effectively builds all the features, and all that? Then the second question here is like, which product or business or tech goal is the team on the hook for? All three of these have different answers to the two questions, that just defines the problem space.
It’s not impossible that if you ask different people at Trainline, they might give you slightly different red-green lights on all of these. I think roughly with my leadership team, we aligned that with clusters, so that size where we were. A is alignment of engineering investment to business goals. P is productivity, how much we’re getting out of the team. Q is the quality, like the quality of the technical work that’s being produced in a way that translates the risk, in terms of like, are we adding technical debt? Are we making platform worse, or are we making it better? That trend.
We felt the clusters were pretty poor for alignment, because no engineers cared about any of the product or business goals. They were just happy to tinker around in their part of the code, because they didn’t own anything end-to-end. Productivity wasn’t great, end-to-end. Everyone was very productive in their little silo, but nothing was shipped fast end-to-end because there were too many teams involved and too many dependencies. Then quality was good, because people worked in a very restrained small part of the overall technology surface. They were very good at it. They cared about making it good. The quality was green.
When we moved to platform and verticals, alignment became super crisp clear. There was a team of engineers on the hook to grow our sales in France. There was a team on the hook to improve monetization, and some that were on the hook to improve customer experience related to refunds or whatever. The goals were very clear, so alignment was perfect. Even on the tech side where you had teams in charge of improving reliability or cost efficiency or all that. Productivity was much better, but the problem with it, again, because of that tension, verticals would say, no, actually, we don’t feel productive because platform is constantly pushing back on us. I think platform had a point to push back on some of those things, but that’s why that one is yellow.
Then quality was actually also pretty good because platform team was policing all the contribution, so they were ensuring that quality. Now with the model we removed, I think we slightly diluted the clarity of the alignment. However, I think we’re really enabling productivity. We were flipping the model previously. I have my favorites among these. I think the most important point is that different things work better at different times for a company. I think, if you do one model for a really long time, sometimes it is good to shift it a little bit, because you get a different balance. This is not necessarily, this is the best and this is the worst, this is the right one, this is the wrong one. I think each organization will have something that works really well, and that is the right thing for it at that time. Again, going back to my previous point.
Let’s talk about what this means for a more technical audience, when you think about it. I think it’s pretty easy to establish that customer and business needs don’t respect architectural boundaries. Is there anyone that would disagree with that statement, or maybe agree? I think it’s entirely fair to say customer business needs don’t respect architecture boundaries. You can design the platform in the most architecturally beautiful way, within months, I promise something will come up where you will be like, “That doesn’t quite fit in into this beautiful logical domain model that I’ve designed. Now we have to think about it.” At the same time, org structure needs to evolve with the business, because business priorities change. What you need to do, that simply needs to change. Along with that, the business strategy and org strategy, technology ownership will move as well.
Something where you thought that a certain set of e-commerce related functions will forever live with that one team, there now might be a different way to look at it. There might be recurring payments and one-off payments. There might be a completely different paradigm shift in terms of, everyone switching to Google Pay and Apple Pay or whatever it is. Technology ownership will move, and will need to move. If you’re not moving it, I think you’re being complacent, or the organization is being complacent and not getting the most out of its technical team.
I think that the way to summarize all of this is Conway’s Law. Who knows what Conway’s Law is? It’s basically this idea that technology ends up getting the shape of the organization that makes it. How you organize your teams, that’s how your technology ends up being built. My insight through this set of changes was that like, Conway’s Law, of course, applies. It’s a fact. The sad thing is that there is no perfect reverse Conway maneuver. It’s really hard to maneuver yourself out of the technical architecture that a certain organizational structure has designed. The actionable advice out of this is, it’s really important to build technology in mind, to build architectures keeping that fact of ownership transfers and external contributions in mind. I think we need to plan for that.
Specifically, I think what that means is enforcing consistency. Every Eng manager or every architect will have their favorite pet tool and pet patterns or ways of path to production, or anything like that. I think the only responsible thing for leaders in the company is just to say, “Sorry, we appreciate you have your weigh-in, how you like doing things, but this is the company way. This is how we do it.” The main reason is that because that person probably won’t be there in a couple of years, but even that team won’t be there, that entire structure will look different. Then if you end up having to patch technology surface in terms of a lot of different patterns, and different sets of tools, and different languages, and different whatnot, that’s the thing that ends up slowing organizations massively.
Because there is no perfect reverse Conway maneuver, the best thing you can do is enforce consistency. Then it’s easier to transfer Lego blocks rather than entire custom-built items, because then you can just reassemble Lego blocks in a different way. That’s the first lesson, consistency is king. As few languages and technologies as possible. Build technology for transferability of ownership and external contributions, even when it comes at the expense of autonomy of individual. I know engineers take that very personally, that autonomy. I did as well when I was writing code.
I think, ultimately, when you look from way higher up in terms of organization, the only thing that makes sense over 3 or 5 or 10 or 15 years. Trainline has been around for 20 years and we still have some stuff in production that was written 15 years ago. I think the best you can do is just like, try to keep things as consistent as possible with few languages, few technologies, and build everything for that kind of transferability.
2. Scaling Costs Sublinearly to Scaling Traffic
We’re going to talk about costs. This is an interesting one, because when I was at Google, we never had to worry about production costs at all. Google had all these endless data centers, and it was all free. I was like a new grad software engineer and I could deploy something that I now know costs probably hundreds of thousands per year, millions, maybe, just in terms of volume. I think Google has potentially changed that philosophy a little bit in the last couple of years. I’ll give you one fact for Trainline. Our AWS bill is about 25% of our overall software engineer compensation bill. It’s non-trivial. It’s a meaningful part of the business. I can easily make a case that, we should spend 10 people worth of time for a year, if we’re going to save 10%, if that ends up being ROI positive for the company. How many of you had to worry about production costs? Most people had to worry about it. That’s good. This will hopefully be useful for you.
When we realized that we’re really growing over the last couple of years, and it felt like cost of platform was growing, not miles faster than the traffic but a bit faster than traffic. I like to run things in an efficient way. It’s my engineering pride to do it in that way. I was like, let’s take a goal. We don’t have to do anything super drastic. CFO is not asking me for drastic cuts. I think just make ourselves disciplined and make sure that we’re running a tight ship. Let’s take on a goal that we’re going to drive down the, at least annual run rate, if not the entire annual bill, 10% down in terms of production cost. Then we looked with my leads, how would that even look? What are the levers we have? We have a massive surface. We’re very microservice oriented.
We have something like 700 services. We have more than 100 databases. I have opinions on whether that’s right or wrong, whether that’s too much. I think having maybe more microservices than engineers is an interesting one. It kind of works. The reason I say that is because when you look at the surface, it’s not immediately obvious where the flab is. You don’t know what’s efficient, what’s not. There is a lot of stuff, a lot of surface, a lot of jobs, a lot of databases, a lot of production platform and data platform, and a lot of different things. We looked a little bit like, at least what levers would we add. We thought about all of this kind of like, ok, we could look and there’s probably some unneeded data we could clean up. We could consolidate on production environments, maybe we don’t need seven non-production environments, maybe we can drop one, and that gives us some savings.
We can review probably some old low-value services that are either deprecated, orphaned. There might be stuff we turn down there. We obviously instantly had this idea of like, are we even right-sized? Are we overprovisioning the platform? We need to review just overall scale. We can look at the data retention policies. Then the tricky one, reviewing architectural choices that we made for services. I think the team has gone very hot for like Cloud Functions for lambdas in the past year. I was like, is that actually efficient? Should we have longer running services or should we shift some of the long running services to Cloud Functions and so on.
This is not necessarily an extensive list. There’s probably more one can do when you think about the overall cloud cost. These are some of the things that I could think about, or that we thought about as a team. Some of them are lower impact in risk. Some of them are higher impact in risk. Now in hindsight, I wish we were a bit more explicit around those things upfront, but we made this list.
Then we decided that we’re just going to delegate this problem down. We have smart engineers. We have smart engineering leaders. We’re going to go to all of our teams that own parts of technology stack, teams in the platform, we’re going to tell them, each one of you is on the hook to drive 10% of the bill down for your area. We had good enough attribution so we would know what’s driving the cost. Can anyone guess what went wrong with that? I thought it was a perfectly valid plan. I was like, it’s too hard to figure out top-down where the flab is. Individual teams know their systems much more, so we’ll just split the bill and we’ll tell them, each one of you brings your part of the bill by 10%, and that should be fine. The problem turned out, of course, that some parts of the platform were already very efficient, and others weren’t. It’s like the reward is disproportionate in these areas.
That 10% for some teams meant they had to really dig in and either spend unnecessarily a lot of engineering time getting the savings, or they started doing risky things, which is actually what ended up being more of a problem for us, specifically, most of them. We were hoping they’ll go review architecture choices, and they’ll switch lambdas to long running servers and a few of these different things. Most of them, they were like, I have a goal to drive this down by 10%, I think I can probably scale a bunch of things down and move from having 7 instances of the server to having 5, and maybe all that. Which in some cases worked, and it was fine.
Equally in the scope of three months, we had something like four outages caused by underprovisioning services. It’s one of those where you get it out and you’re like, it seems like it’s working. Tuesday is the peak morning traffic for us. You do some changes Wednesday or Thursday, and then couple of days later, as we hit the peak, the autoscaling doesn’t work fast enough or something else goes wrong related to being underprovisioned, and platform goes down in the morning peak, and the worst time, when we should be going live.
That’s some of the stories effectively that happened. Ultimately ended up meaning, I think we did projects, which did give us 10% savings, but were possibly not the best value for money because engineers ended up spending more time than they should have really. Then we also had these unintended consequences in terms of outages where people went for what felt like a simple way to save money, but really was actually not what we needed to do at all. The so what of this one is like, cost management is important for long term efficiency of the platform. However, understanding where flab is in a very large technical system with a lot of especially very large fragmented microservice based system, does sadly require those with centralized thinking. I think just delegating that problem down doesn’t work. That was my lesson and I was very sad to learn that.
Equally, predicting which cost reducing efforts are worthy can be tricky, especially for people who don’t have maybe the full big picture but you only know their part of the stack. The lesson here for me was like, don’t blindly push down cost saving goals to individual teams. There are more ways it can go wrong than necessarily right. If I were to do something like this again, I probably would have had a bit more of a centralized task force that can work with individual teams, but evaluate, where is the investment to save actually worth it versus not? That’s basically it. Manage system cost savings efforts centrally. I think fully delegating ends up backfiring.
3. Scaling Large Microservice Based Architecture
The last lesson. The first one was more team productivity impact, and scaling that. The second one was scaling efficiency of the platform. The third one is scaling for growth in traffic and reliability. I am going to briefly try to cover three big bouts of outages we had. In October 2021, I think I barely passed my probation or maybe didn’t even yet, and Trainline goes down for something like four hours, one day, and then it goes down two hours next day. Disaster, like really bad. What we realized happened was that at that stage, world was again recovering from COVID. We were recovering from close to zero traffic back to what was starting to be historical maximums, like day after day and week after week. The entire time through COVID, software development didn’t stop at all. Trainline even didn’t lay off any people.
You had 18 months of 350 engineers writing code that was never tested at scale. What could possibly go wrong when that happens? Imagine batching up 18 months of changes, and then suddenly, it goes to production. It was loose everywhere. It wasn’t one simple cause. The biggest one that we realized, in this particular instance, was that, because it’s very microservice oriented, a lot of new services have been added. All of them were maintaining connections to the database, especially as they were scaling at peak times, they were creating more connections.
Ultimately, the biggest thing that was causing the outage was the contention in terms of database connections. We were running out of pools. This is, especially in the parts of the platform where we still have relational databases. We are on an absolute mission to entirely move to cloud native storage, data actually still have plenty of relational databases. This was the bottleneck where we have them hosted. Even if it’s a mega machine, it’s still hosted on a single machine and cannot keep up with that many connections. That got tweaked, tuned. We survived that period, and we were good for a while.
Then, I don’t know what happens in the fall. Now I have a little bit of PTSD every time it comes to October and November, and I just don’t know what’s going to be next. Somehow completely unrelated, but exactly a year later, we ended up getting to this place where, again, the database was knocking out. I think the previous one was some old Oracles and this one was the SQL Server. It’s not exactly the same thing. It was knocking out, and we didn’t see it coming. We were like, we thought we have super good observability, we keep an eye on everything, and it just starts happening. It’s not like when you do a bad portion, you roll back within couple of minutes, and it’s all safe. This is, again, hour-plus long outages until we’ve switched to a new replica of the database, just not good stuff.
It took a while to analyze what happened. Basically, over that year, we have been adding more of these features that are not purely related to transaction of buying a train ticket, but are actually related to the journey itself, so to traveling. You saw the nice map with trains moving, so we have a lot of features related to that where you can follow your journey. You can get a notification of the platform, the change, all that. All of those additional flows that are related to journey experience, have actually been talking to the orders database, because they need to know what train you’re on. That’s how it was designed.
Most of our observability was centered on all of our transactional flows, not on all of those other flows. What happened over the year was that gradually the traffic mix shift changed, and the load on the orders significantly increased. Because it happened gradually, we didn’t really notice, and especially because it happened and we didn’t think that’s where it’s going to go wrong. That’s how stuff goes wrong. You didn’t expect to go wrong where it does. We had to do some more database related stuff to fix that, and we just survived this. That was that.
Then, just couple of months ago, November this year, we had a fairly insane bout of DDoS attacks from what may or may not be attributed to some nation states related to conflicts that sadly we have in Europe right now. Most of the travel sector is seeing this. We’re not the only ones. Many other companies are seeing that. As that was happening, we had to significantly tighten up our DDoS protections and change a few other things related to that. Then, just as we were feeling good about it, we were like, yes, they’re hitting us so hard and we’re holding up. We’re not buckling at all. We just go down one day, for an hour and a half, whatever. That was the stuff that ended up in press. It happened a couple of times in the scope of a month. As we dug in, initially it looked like we were getting DDoS attacked, but it wasn’t that. There were sloppy retry strategies all around the stack. We didn’t really have a very coordinated retry strategy overall, like who retries what and where.
Even small issues of like network blips or something that goes wrong, could snowball into this fact where we end up DDoSing ourselves. Because something is down, and then, the client retries, and the Backend For Frontend retries, and backend services retry, all that. That ends up creating 10x load that eventually brings the platform down. That was fun. It resolved eventually.
What’s common about all of these? This is the architecture lesson that I got out of it. None of these were caused by a single team, a single change, or a single regression. That’s where it starts to get really difficult. For each one of them, it was a lot of straws, a lot of papercuts that ultimately cause you to bleed to death. That’s how it goes. It’s not like you can point to this one change, and then all your best DevOps processes, and you can very quickly detect it, and roll it back, and all that.
No, things build up over time, just like sloppiness in a certain area multiplied by a large team, everyone chasing their own goals, and a very microservice, kind of spread-out architecture like that. The so what is, predicting a bottleneck in a large microservice based system is really hard. I still don’t know if we quite know how to predict it. You don’t know what you don’t know. Often, you’re looking at the tragedy of commons. It’s not one team gets it wrong.
Everyone just adds a few too many database connections. Everyone just adds a few too many retries. Everyone optimizes slightly wrongly for scaling, and just eventually the whole thing bubbles up. The best lesson I could get out of this, and I think we’re still trying to learn and figure out how we can handle this better is, regularly review longer term traffic mix or load changes. Trainline is pretty good at doing all the release by release, or day by day changes. All of that is automated. You have alerting, monitoring. All of that is pretty solid. You sometimes need someone to sit once a quarter and look like, how did actually mix on couple of critical databases or services change over the past six months, or something like that? What is that telling us? Where is the next bottleneck going to be? I think that’s the one.
That’s something that we’re now trying to put into action. Then, microservice fleet coordination is absolutely critical. Again, it’s having to guide teams and have a really strong architecture function or principal engineering function or however you want to call it, but someone who’s guiding teams, who’s looking at the big picture on top of individual ownership of which teams own which part. Guiding teams on things that could add up to create a lot of mess, so like retry strategies, or scaling policies, or anything that speaks to any scarce resource that’s downstream. That’s that lesson. Observe over longer term, coordinate microservice fleet.
Recap of the 3 Lessons
Consistency is king. I think it’s absolutely critical for productivity in the long run. If you’re in a series A startup or a seed round that’s just trying to churn something out very quickly, and you can throw away that technology, then you won’t care. If you’re genuinely building a business where technology should survive for 5 or 10 or 15 years, then I think just like insisting on consistency, even if engineers won’t love it. Manage system cost saving efforts centrally, because delegation just doesn’t really work. People lose the wider context. Then, observe over longer term and coordinate microservice fleet to avoid many outages.
Questions and Answers
Participant 1: You’ve mentioned building and architecting towards changing the structure and people that intuitively goes against optimizing for knowledge and change management in the near time. How do you balance that?
Nikolic: I think there is a question here of short, medium, long term. I think there is something where people absolutely love, you build it, you own it. I really believe that. As a strategy, you should carry the pager and pay the, being paged in the middle of the night cost of, if you don’t architect or build something properly. However, that really only applies for the first six months of a service, nine, maybe up to a year. After that, again, that person who built it is going to move on anyway. This is a fast-moving industry, talent moves around. People would want a new challenge, all that.
The way we think about it is like, you build it, you own it, but eventually, you need to be ready to hand it over. When we had platform and verticals model, that was the model. Vertical would build a new service. They would be on the hook for it for like six or nine months. The relevant parts of the platform would advise through that period, what do they need? What’s the criteria they need the service to pass for them to adopt it? The idea would be that within 6 to 12 months, they adopt it. There is that transition that forces you to. This is hard. It’s not like, we always get it right.
This is the thing that causes contention in the teams, all that. I think trying to pull teams back out of that mindset, because I’ve seen too many times where you end up with a bus factor of one and there is only one person who knows the service, and no one dares to touch it. That’s not good. Especially if you want to have an agile organization that moves and really always focuses on the most important things, you need to have these Lego blocks that anyone is at least moderately comfortable picking up and saying, yes, I’m not an expert, but I’m comfortable picking this up, and I can take care of it and learn more, and all that.
Participant 2: I have a question about the first lesson that you mentioned, the consistency. If you think about maybe 5 years ago, or maybe 10, when the microservices were out, we were so proud that they can be built with different languages, that you’ve stuff owned by the team, autonomy. Now, after that time, today we’re talking about consistency. Then we say it’s the king. Do you think it’s the future? Do you see any downside to it? How do you see that?
Nikolic: You already need to have different languages for the frontends. In our case, that ends up being three different, like two on Android, and iOS. You have that fragmentation of the skill set that you have in the organization. Then on the backend, we have most of stuff in .NET. Then we have some Ruby stuff that came through acquisition, it was always too big to replatform and it always stayed as a sort of thing. It’s really tricky when you need to put a cross-functional team that needs to deliver something, even if it’s simple, one field being plumbed from the database to the UI. Something that’s meant to be very simple. You still need 10 people to do it, which I find almost laughable. It’s ridiculous.
Because you need your Android pair, an iOS pair, and .NET pair, and Ruby pair. Some of that complexity is hard to get around because of native platforms like Android and iOS having their own languages. That’s why I would really try to bring it down. I know engineers don’t love it, but I draw the line and say, no. I think it’s important to leave a little bit of space for people to innovate and try new things. If the new thing works well and is approved, then there needs to be a path for making it official thing for everything and everyone else. I think just freely letting everyone choose their own thing, very quickly I think it just completely is unmanageable. If the business becomes successful there is no path. What do you do a few years down the line?
See more presentations with transcripts
Java News Roundup: WildFly 34, Stream Gatherers, Oracle CPU, Quarkiverse Release Process
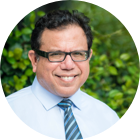
MMS • Michael Redlich
Article originally posted on InfoQ. Visit InfoQ
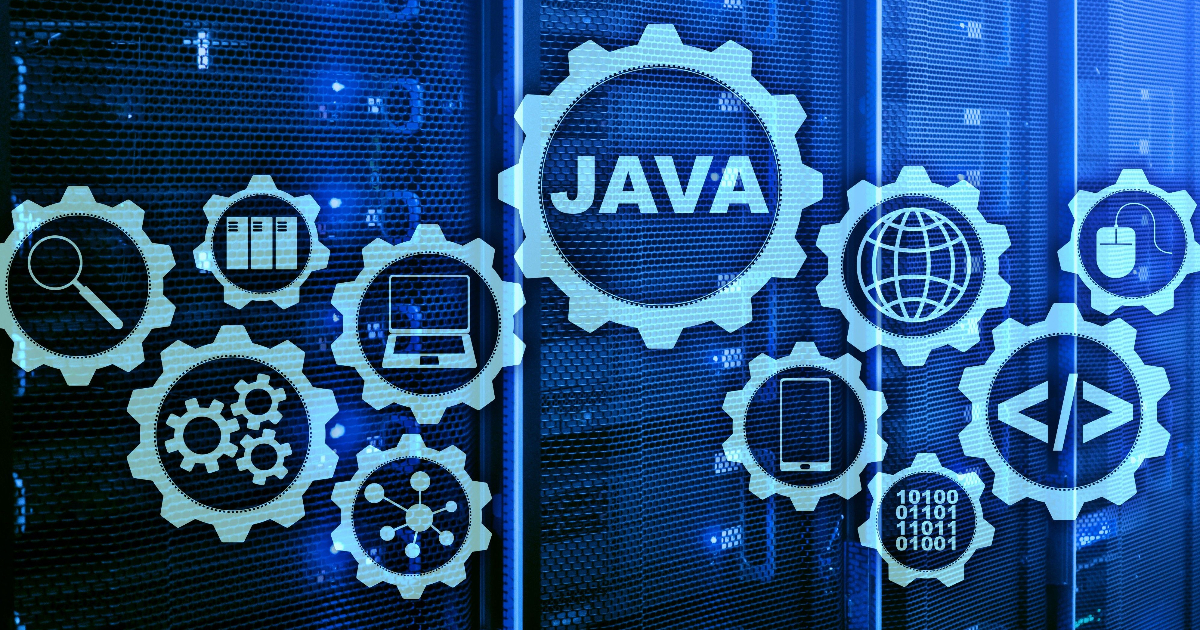
This week’s Java roundup for October 14th, 2024, features news highlighting: the release of WildFly 34; JEP 485, Stream Gatherers, proposed to target for JDK 24; Oracle Critical Patch Update for October 2024; and a potential leak in the SmallRye and Quarkiverse release processes.
OpenJDK
JEP 485, Stream Gatherers, has been promoted from Candidate to Proposed to Target for JDK 24. This JEP proposes to finalize this feature after two rounds of preview, namely: JEP 473: Stream Gatherers (Second Preview), delivered in JDK 23; and JEP 461, Stream Gatherers (Preview), delivered in JDK 22. This feature was designed to enhance the Stream API to support custom intermediate operations that will “allow stream pipelines to transform data in ways that are not easily achievable with the existing built-in intermediate operations.” More details on this JEP may be found in the original design document and this InfoQ news story. The review is expected to conclude on October 23, 2024.
Oracle has released versions 23.0.1, 21.0.5, 17.0.13, 11.0.25, and 8u431 of the JDK as part of the quarterly Critical Patch Update Advisory for October 2024. More details on this release may be found in the release notes for version 23.0.1, version 21.0.5, version 17.0.13, version 11.0.25 and version 8u431.
Version 7.5.0 of the Regression Test Harness for the JDK, jtreg
, has been released and ready for integration in the JDK. The most significant changes include: the restoration of the jtdiff
tool; and support for a LIBRARY.properties
file located in the directory specified in the @library
tag and read when jtreg
compiles classes in that library. There was also a dependency upgrade to JUnit 5.11.0. Further details on this release may be found in the release notes.
JDK 24
Build 20 of the JDK 24 early-access builds was made available this past week featuring updates from Build 19 that include fixes for various issues. Further details on this release may be found in the release notes.
For JDK 24, developers are encouraged to report bugs via the Java Bug Database.
Jakarta EE 11
In his weekly Hashtag Jakarta EE blog, Ivar Grimstad, Jakarta EE developer advocate at the Eclipse Foundation, provided an update on Jakarta EE 11, writing:
GlassFish now passes 84% of the tests in the refactored TCK for Jakarta EE 11. The remaining tests are mainly related to the Application Client Container. The Jakarta EE Platform Project is proposing to deprecate the Application Container in Jakarta EE 12. There are ongoing discussions about how much importance these tests should be given to Jakarta EE 11.
The Jakarta EE 11 Core Profile TCK has been staged, and both Open Liberty and WildFly are passing (or very close to passing) it. So it looks like we will be able to release Jakarta EE 11 Core Profile ahead of Jakarta EE 11 Platform and Jakarta EE 11 Web Profile.
The road to Jakarta EE 11 included four milestone releases with the potential for release candidates as necessary before the GA release in 4Q2024.
BellSoft
Concurrent with Oracle’s Critical Patch Update (CPU) for October 2024, BellSoft has released CPU patches for versions 21.0.4.0.1, 17.0.12.0.1, 11.0.24.0.1, 8u431, 7u441 and 6u441 of Liberica JDK, their downstream distribution of OpenJDK, to address this list of CVEs. In addition, Patch Set Update (PSU) versions 23.0.1, 21.0.5, 17.0.13, 11.0.25 and 8u432, containing CPU and non-critical fixes, have also been released.
With an overall total of 1169 fixes and backports, BellSoft states that they have participated in eliminating 18 issues in all releases.
Spring Framework
The second release candidate of Spring Framework 6.2.0 delivers bug fixes, improvements in documentation, dependency upgrades and many new features such as: a rename of the OverrideMetadata
class to BeanOverrideHandler
to align with the existing naming convention of the other classes, interfaces and annotations defined in the org.springframework.test.context.bean.override
package; and the addition of the messageConverters()
method to the RestClient.Builder
interface to allow setting converters of the RestClient
interface without initializing the default one. This version will be included in the upcoming release of Spring Boot 3.4.0-RC1. More details on this release may be found in the release notes.
Similarly, the release of version 6.1.14 of Spring Framework also provides bug fixes, improvements in documentation, dependency upgrades and new features such as: the removal of support for relative paths in the ResourceHandlerUtils
class that eliminates security issues; and ensure proper exception handling from the isCorsRequest()
method, defined in the CorsUtils
class, upon encountering a malformed Origin
header. This version will be included in the upcoming releases of Spring Boot and 3.3.5 and 3.2.11. More details on this release may be found in the release notes.
The Spring Framework team has also disclosed two Common Vulnerabilities and Exposures (CVEs):
- CVE-2024-38819, a path traversal vulnerability in the Spring Web MVC and Spring WebFlux functional web frameworks in which an attacker can create a malicious HTTP request to obtain any file on the file system that is also accessible to the process on the running Spring application. This CVE is follow up from CVE-2024-38816, Path Traversal Vulnerability in Functional Web Frameworks, that used different malicious input.
- CVE-2024-38820: a vulnerability in which the
toLowerCase()
method, defined in the JavaString
class, had someLocale
class-dependent exceptions that could potentially result in fields not being protected as expected. This is a result of the resolution for CVE-2022-22968 that made patterns of thedisallowedFields
field, defined inDataBinder
class, case insensitive.
These CVEs affect Spring Framework versions 5.3.0 – 5.3.40, 6.0.0 – 6.0.24 and 6.1.0 – 6.1.13.
The first release candidate of Spring Data 2024.1.0 delivers expanded support for Spring Data Value Expressions where property-placeholders may be leveraged in repository query methods annotated with @Query
. There were also updates to sub-projects such as: Spring Data Commons 3.4.0-RC1, Spring Data MongoDB 4.4.0-RC1, Spring Data Elasticsearch 5.4.0-RC1 and Spring Data Neo4j 7.4.0-RC1. More details on this release may be found in the release notes.
Similarly, the release of Spring Data 2024.0.5 and 2023.1.11 ship with bug fixes and respective dependency upgrades to sub-projects such as: Spring Data Commons 3.3.5 and 3.2.11; Spring Data MongoDB 4.3.5 and 4.2.11; Spring Data Elasticsearch 5.3.5 and 5.2.11; and Spring Data Neo4j 7.3.5 and 7.2.11. These versions will be included in the upcoming releases of Spring Boot and 3.3.5 and 3.2.11.
WildFly
The release of WildFly 34 primarily focuses on WildFly Preview, a technical preview variant of the WildFly server. New features include: support for Jakarta Data 1.0, MicroProfile Rest Client 4.0 and MicroProfile Telemetry 2.0; a new Bill of Materials for WildFly Preview; and four new system properties (backlog
, connection-high-water
, connection-low-water
and no-request-timeout
) for configuration in the HTTP management interface. More details on this release may be found in the release notes. InfoQ will follow up with a more detailed news story.
Quarkus
The Quarkus team has disclosed that they recently discovered a potential leak in their Quarkiverse and SmallRye release processes and reported that there was no damage.
Clement Escoffier, Distinguished Engineer at Red Hat, summarized the issue, writing:
We’ve uncovered a security flaw in the release process for Quarkiverse and SmallRye that could have allowed malicious actors to impersonate projects and publish compromised artifacts.
We’ve implemented a new, more secure release pipeline to address this. If you’re a maintainer, you’ve received a pull request to migrate to the new process. Quarkus itself is not affected by this issue, only SmallRye and Quarkiverse.
As a result, they have implemented a more secure release process and wanted to share the details with the Java community. InfoQ will follow up with a more detailed news story.
Micrometer
The first release candidate of Micrometer Metrics 1.14.0 provides bug fixes, improvements in documentation, dependency upgrades and new features such as: expose an instance of the TestObservationRegistry
class via the assertThat()
method from the AssertJ Assertions
class; expand metrics to include virtual threads data; and improved performance with the initialization of the Tags
class from already sorted array of unique tags. More details on this release may be found in the release notes.
Similarly, versions 1.13.6 and 1.12.11 of Micrometer Metrics also feature bug fixes, improvements in documentation and a new feature that improves the memory usage of the StepBucketHistogram
class by eliminating an internal field of the buckets that can be acquired from an instance of the FixedBoundaryHistogram
class when needed. Further details on these releases may be found in the release notes for version 1.13.6 and version 1.12.11.
The first release candidate of Micrometer Tracing 1.4.0 ships with dependency upgrades and new features: support for list values in tags in the Span
and SpanCustomizer
interfaces; and make the OtelSpan
class public instead of private to eliminate use of reflection to act upon the underlying OpenTelemetry Span
interface. More details on this release may be found in the release notes.
Similarly, version 1.3.5 and 1.2.11 of Micrometer Tracing 1.4.0 simply provide dependency upgrades. Further details on these releases may be found in the release notes for version 1.3.5 and version 1.2.11.
Project Reactor
The first release candidate of Project Reactor 2024.0.0 provides dependency upgrades to reactor-core 3.7.0-RC1
, reactor-netty 1.2.0-RC1
, reactor-pool 1.1.0-RC1
, reactor-addons 3.6.0-RC1
, reactor-kotlin-extensions 1.3.0-RC1
and reactor-kafka 1.4.0-RC1
. Based on the Spring Calendar, it is anticipated that the GA version of Project 2024.0.0 will be released in November 2024. Further details on this release may be found in the changelog.
Next, Project Reactor 2023.0.11, the eleventh maintenance release, provides dependency upgrades to reactor-core 3.6.11
and reactor-netty 1.1.23
. There was also a realignment to version 2023.0.11 with the reactor-pool 1.0.8
, reactor-addons 3.5.2
, reactor-kotlin-extensions 1.2.3
and reactor-kafka 1.3.23
artifacts that remain unchanged. More details on this release may be found in the changelog.
Piranha Cloud
The release of Piranha 24.10.0 delivers bug fixes and notable changes such as: ensure that an instance of the Eclipse Jersey InjecteeSkippingAnalyzer
class is installed when needed; and use of the Java PrintStream
class or the isWriterAcquired()
method, defined in the in the DefaultWebApplicationResponse
class, in the DefaultServletRequestDispatcher
class as a response to a top-level exception. Further details on this release may be found in their documentation and issue tracker.
Apache Software Foundation
The third milestone release of Apache TomEE 10.0.0 provides bugs fixes, dependency upgrades and new features such as: an improved import of data sources and entity managers that obsoletes the use of the ImportSql
class; and a new RequestNotActiveException
class, that replaces throwing a NullPointerException
, when an instance of a Jakarta Servlet HttpServletRequest
is invoked on a thread with no active servlet request. More details on this release may be found in the release notes.
JobRunr
The release of JobRunr 7.3.1 provides new features such as: an instance of the JobDetails
class is now cacheable when injecting an interface instead of an implementation; and an enhanced JobRunr Dashboard that includes tips for diagnosing severe JobRunr exceptions for improved clarity of notifications. Further details on this release may be found in the release notes.
Keycloak
Keycloak 26.0.1 has been released with bug fixes and enhancements: a clarification of the behavior of multiple versions of Keycloak Operator installed in the same cluster operator; and improved error logging during a transaction commit. More details on this release may be found in the release notes.
JDKUpdater
Version 14.0.59+79 of JDKUpdater, a new utility that provides developers the ability to keep track of updates related to builds of OpenJDK and GraalVM. Introduced in mid-March by Gerrit Grunwald, principal engineer at Azul, this release resolves an issue with the calculation of the next update and the next release date of the JDK. More details on this release may be found in the release notes.
Gradle
The first release candidate of Gradle 8.11.0 delivers new features such as: improved performance in the configuration cache with an opt-in parallel loading and storing of cache entries; the C++ and Swift plugins now compatible with the configuration cache; and improved error and warning reporting in which Java compilation errors are now displayed at the end of the build output. More details on this release may be found in the release notes.
OpenAI Releases Swarm, an Experimental Open-Source Framework for Multi-Agent Orchestration
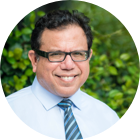
MMS • Sergio De Simone
Article originally posted on InfoQ. Visit InfoQ
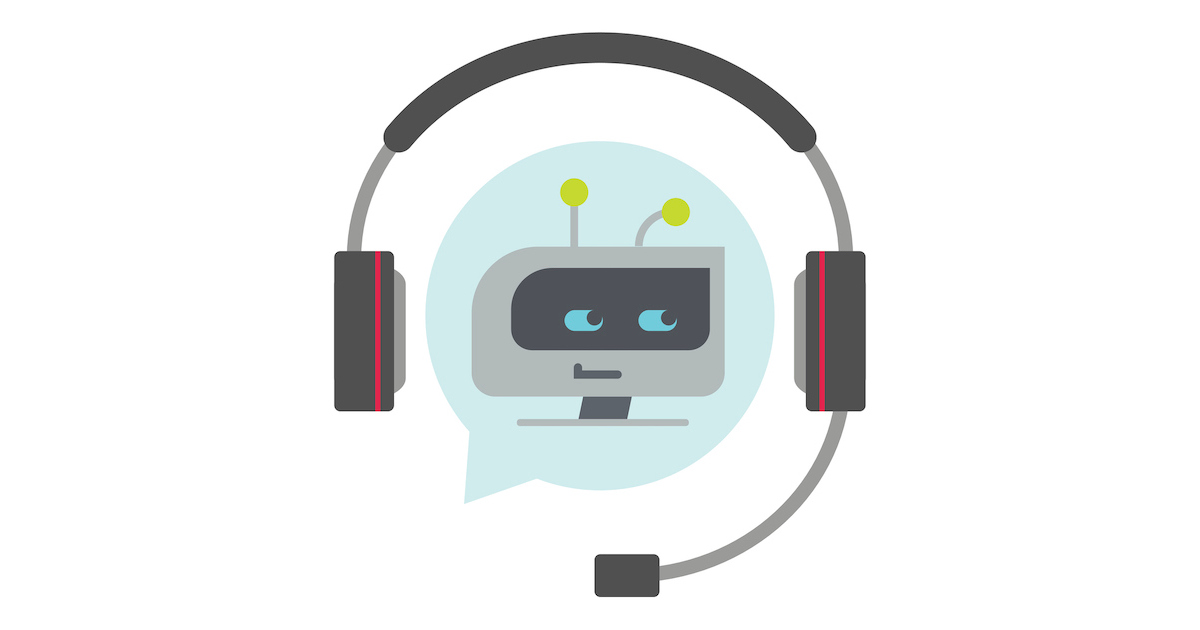
Recently released as an experimental tool, Swarm aims to allow developers to investigate how they can have multiple agents coordinate with one another to execute tasks using routines and handoffs.
Multi-agent systems are an approach to building more complex AI systems where a task is broken into subtasks. Each task is then assigned to a specialized agent that is able to choose the most appropriate strategy to solve it. For example, you could build a shopper agent with two sub-agents, one managing refunds and the other managing sales, with a third agent, a triage agent, determining which sub-agent should handle a new request.
Swarm explores patterns that are lightweight, scalable, and highly customizable by design. Approaches similar to Swarm are best suited for situations dealing with a large number of independent capabilities and instructions that are difficult to encode into a single prompt.
As mentioned, Swarm is based on the concepts of routines and handoffs. In this context, a routine is a set of steps and tools to execute them, while a handoff represents the action of an agent handing off a conversation to another agent. This implies loading the corresponding routine and provide it with all the context accumulated during the previous conversation. For example, the following snippet shows how you could define a sale and a refund agent:
def execute_refund(item_name):
return "success"
refund_agent = Agent(
name="Refund Agent",
instructions="You are a refund agent. Help the user with refunds.",
tools=[execute_refund],
)
def place_order(item_name):
return "success"
sales_assistant = Agent(
name="Sales Assistant",
instructions="You are a sales assistant. Sell the user a product.",
tools=[place_order],
)
To manage handoffs, you can define a triage agent like in the following snippet which includes two functions, transfer_to_sales_agent
, transfer_to_refund_agent
that return their corresponding agent. You also need to add a transfer_to_triage_agent
tool to our refund_agent
and sales_assistant
definitions.
triage_agent = Agent(
name="Triage Agent",
instructions=(
"Gather information to direct the customer to the right department."
),
tools=[transfer_to_sales_agent, transfer_to_refund_agent],
)
...
refund_agent = Agent(
...
tools=[execute_refund, transfer_to_triage_agent],
)
...
sales_assistant = Agent(
...
tools=[place_order, transfer_to_triage_agent],
)
The pattern described above, where you use a triage agent, is just one way to manage handoffs and Swarm supports the use of distinct solutions.
Examples of alternative frameworks to create multi-agent systems are Microsoft’s AutoGen, CrewAI, and AgentKit. Each of them takes a different stance about how to orchestrate agents and which aspects are essential to it.
Multi-agent systems aim to enable the creation of more complex systems by working around some limitations of LLMs, like single-turn responses, lack of long-term memory, and reasoning depth.
It is important to understand, though, that decomposing a complex agent into a multi-agent system is not necessarily an easy task. As Hacker News commenter ValentinA23 points out, the process “is very time consuming though, as it requires experimenting to determine how best to divide one task into subtasks, including writing code to parse and sanitize each task output and plug it back into the rest of the agent graph though, as it requires experimenting to determine how best to divide one task into subtasks, including writing code to parse and sanitize each task output and plug it back into the rest of the agent graph”.
Another Hacker News commenter, LASR, raises a concern that the distinct agents will diverge in time:
The problem with agents is divergence. Very quickly, an ensemble of agents will start doing their own things and it’s impossible to get something that consistently gets to your desired state.
Finally, Hacker News user dimitri-vs mentions that the fast evolution of current LLMs, e.g., GPT o1 and Sonnet 3.5, makes it so that “it is much easier to swap in a single API call and modify one or two prompts than to rework a convoluted agentic approach. Especially when it’s very clear that the same prompts can’t be reused reliably between different models”.
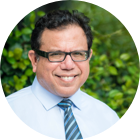
MMS • RSS
Posted on mongodb google news. Visit mongodb google news
Wrapping up Q2 earnings, we look at the numbers and key takeaways for the data storage stocks, including MongoDB (NASDAQ:MDB) and its peers.
Data is the lifeblood of the internet and software in general, and the amount of data created is accelerating. As a result, the importance of storing the data in scalable and efficient formats continues to rise, especially as its diversity and associated use cases expand from analyzing simple, structured datasets to high-scale processing of unstructured data such as images, audio, and video.
The 5 data storage stocks we track reported a strong Q2. As a group, revenues beat analysts’ consensus estimates by 2.5% while next quarter’s revenue guidance was 1.3% above.
Inflation progressed towards the Fed’s 2% goal recently, leading the Fed to reduce its policy rate by 50bps (half a percent or 0.5%) in September 2024. This is the first cut in four years. While CPI (inflation) readings have been supportive lately, employment measures have bordered on worrisome. The markets will be debating whether this rate cut’s timing (and more potential ones in 2024 and 2025) is ideal for supporting the economy or a bit too late for a macro that has already cooled too much.
Luckily, data storage stocks have performed well with share prices up 11% on average since the latest earnings results.
MongoDB (NASDAQ:MDB)
Started in 2007 by the team behind Google’s ad platform, DoubleClick, MongoDB offers database-as-a-service that helps companies store large volumes of semi-structured data.
MongoDB reported revenues of $478.1 million, up 12.8% year on year. This print exceeded analysts’ expectations by 3%. Overall, it was a very strong quarter for the company with an impressive beat of analysts’ billings estimates and full-year revenue guidance exceeding analysts’ expectations.
“MongoDB delivered healthy second quarter results, highlighted by strong new workload acquisition and better-than-expected Atlas consumption trends. Our continued success in winning new workloads demonstrates the critical role MongoDB’s platform plays in modern application development,” said Dev Ittycheria, President and Chief Executive Officer of MongoDB.
MongoDB delivered the slowest revenue growth of the whole group. The company added 52 enterprise customers paying more than $100,000 annually to reach a total of 2,189. Interestingly, the stock is up 12.7% since reporting and currently trades at $277.
Is now the time to buy MongoDB? Access our full analysis of the earnings results here, it’s free.
Best Q2: Commvault Systems (NASDAQ:CVLT)
Originally formed in 1988 as part of Bell Labs, Commvault (NASDAQ: CVLT) provides enterprise software used for data backup and recovery, cloud and infrastructure management, retention, and compliance.
Commvault Systems reported revenues of $224.7 million, up 13.4% year on year, outperforming analysts’ expectations by 4.2%. The business had an exceptional quarter with an impressive beat of analysts’ billings estimates and full-year revenue guidance exceeding analysts’ expectations.
Commvault Systems achieved the biggest analyst estimates beat among its peers. The market seems happy with the results as the stock is up 18.6% since reporting. It currently trades at $146.23.
Is now the time to buy Commvault Systems? Access our full analysis of the earnings results here, it’s free.
Weakest Q2: Snowflake (NYSE:SNOW)
Founded in 2013 by three French engineers who spent decades working for Oracle, Snowflake (NYSE:SNOW) provides a data warehouse-as-a-service in the cloud that allows companies to store large amounts of data and analyze it in real time.
Snowflake reported revenues of $868.8 million, up 28.9% year on year, exceeding analysts’ expectations by 2.1%. Still, it was a slower quarter as it posted a miss of analysts’ billings estimates.
As expected, the stock is down 11.6% since the results and currently trades at $119.45.
Read our full analysis of Snowflake’s results here.
Couchbase (NASDAQ:BASE)
Formed in 2011 with the merger of Membase and CouchOne, Couchbase (NASDAQ:BASE) is a database-as-a-service platform that allows enterprises to store large volumes of semi-structured data.
Couchbase reported revenues of $51.59 million, up 19.6% year on year. This number was in line with analysts’ expectations. Zooming out, it was a mixed quarter as it also produced full-year revenue guidance exceeding analysts’ expectations but a miss of analysts’ billings estimates.
Couchbase had the weakest performance against analyst estimates and weakest full-year guidance update among its peers. The stock is down 14.4% since reporting and currently trades at $16.25.
Read our full, actionable report on Couchbase here, it’s free.
DigitalOcean (NYSE:DOCN)
Started by brothers Ben and Moisey Uretsky, DigitalOcean (NYSE: DOCN) provides a simple, low-cost platform that allows developers and small and medium-sized businesses to host applications and data in the cloud.
DigitalOcean reported revenues of $192.5 million, up 13.3% year on year. This number surpassed analysts’ expectations by 2%. Overall, it was a very strong quarter as it also recorded full-year revenue guidance exceeding analysts’ expectations and a solid beat of analysts’ ARR (annual recurring revenue) estimates.
DigitalOcean achieved the highest full-year guidance raise among its peers. The stock is up 49.7% since reporting and currently trades at $43.55.
Read our full, actionable report on DigitalOcean here, it’s free.
Join Paid Stock Investor Research
Help us make StockStory more helpful to investors like yourself. Join our paid user research session and receive a $50 Amazon gift card for your opinions. Sign up here.
Article originally posted on mongodb google news. Visit mongodb google news
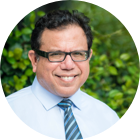
MMS • RSS
Posted on mongodb google news. Visit mongodb google news
The latest research study released by HTF MI on Global Cloud Database and DBaaS Market with 143+ pages of analysis on business Strategy taken up by key and emerging industry players and delivers know-how of the current market development, landscape, sales, drivers, opportunities, market viewpoint and status. Cloud Database and DBaaS market study is a perfect mix of qualitative and quantitative Market data collected and validated majorly through primary data and secondary sources.
Key Players in This Report Include:
Microsoft Azure, AWS (Amazon), Google Cloud, Oracle, IBM, Alibaba Cloud, MongoDB, SAP, Teradata, Redis Labs, Couchbase, DataStax, Snowflake, ArangoDB, MariaDB, InfluxDB, SingleStore, Neo4j, Citus Data, YugabyteDB
Download Sample Report PDF (Including Full TOC, Table & Figures) 👉 https://www.htfmarketreport.com/sample-report/3355026-2020-2025-global-cloud-database-and-dbaas-market-report-production-and-consumption-professional-analysis?utm_source=Ganesh_OpenPR&utm_id=Ganesh
According to HTF Market Intelligence, the Global Cloud Database and DBaaS market is expected to grow from 14 billion USD in 2023 to 50 billion USD by 2032, with a CAGR of 21% from 2024 to 2032. The Cloud Database and DBaaS market is segmented by Types (SQL, NoSQL, Hybrid, Distributed, Multi-cloud), Application (Banking, Retail, Healthcare, Manufacturing, Government) and by Geography (North America, LATAM, West Europe, Central & Eastern Europe, Northern Europe, Southern Europe, East Asia, Southeast Asia, South Asia, Central Asia, Oceania, MEA).
Definition:
Cloud Database is a service that allows users to store and manage data in a cloud environment, providing scalable and flexible database solutions without the need for on-premises infrastructure.
Dominating Region:
• North America, Europe, Asia
Fastest-Growing Region:
• Cloud Database and DBaaS
Have a query? Market an enquiry before purchase 👉 https://www.htfmarketreport.com/enquiry-before-buy/3355026-2020-2025-global-cloud-database-and-dbaas-market-report-production-and-consumption-professional-analysis?utm_source=Ganesh_OpenPR&utm_id=Ganesh
The titled segments and sub-section of the market are illuminated below:
In-depth analysis of Cloud Database and DBaaS segments by Types: SQL, NoSQL, Hybrid, Distributed, Multi-cloud
Detailed analysis of Cloud Database and DBaaS segments by Applications: Banking, Retail, Healthcare, Manufacturing, Government
Geographically, the detailed analysis of consumption, revenue, market share, and growth rate of the following regions:
• The Middle East and Africa (South Africa, Saudi Arabia, UAE, Israel, Egypt, etc.)
• North America (United States, Mexico & Canada)
• South America (Brazil, Venezuela, Argentina, Ecuador, Peru, Colombia, etc.)
• Europe (Turkey, Spain, Turkey, Netherlands Denmark, Belgium, Switzerland, Germany, Russia UK, Italy, France, etc.)
• Asia-Pacific (Taiwan, Hong Kong, Singapore, Vietnam, China, Malaysia, Japan, Philippines, Korea, Thailand, India, Indonesia, and Australia).
Buy Now Latest Edition of Cloud Database and DBaaS Market Report 👉 https://www.htfmarketreport.com/buy-now?format=1&report=3355026
Cloud Database and DBaaS Research Objectives:
– Focuses on the key manufacturers, to define, pronounce and examine the value, sales volume, market share, market competition landscape, SWOT analysis, and development plans in the next few years.
– To share comprehensive information about the key factors influencing the growth of the market (opportunities, drivers, growth potential, industry-specific challenges and risks).
– To analyze the with respect to individual future prospects, growth trends and their involvement to the total market.
– To analyze reasonable developments such as agreements, expansions new product launches, and acquisitions in the market.
– To deliberately profile the key players and systematically examine their growth strategies.
FIVE FORCES & PESTLE ANALYSIS:
In order to better understand market conditions five forces analysis is conducted that includes the Bargaining power of buyers, Bargaining power of suppliers, Threat of new entrants, Threat of substitutes, and Threat of rivalry.
• Political (Political policy and stability as well as trade, fiscal, and taxation policies)
• Economical (Interest rates, employment or unemployment rates, raw material costs, and foreign exchange rates)
• Social (Changing family demographics, education levels, cultural trends, attitude changes, and changes in lifestyles)
• Technological (Changes in digital or mobile technology, automation, research, and development)
• Legal (Employment legislation, consumer law, health, and safety, international as well as trade regulation and restrictions)
• Environmental (Climate, recycling procedures, carbon footprint, waste disposal, and sustainability)
Get 10-25% Discount on Immediate purchase 👉 https://www.htfmarketreport.com/request-discount/3355026-2020-2025-global-cloud-database-and-dbaas-market-report-production-and-consumption-professional-analysis?utm_source=Ganesh_OpenPR&utm_id=Ganesh
Points Covered in Table of Content of Global Cloud Database and DBaaS Market:
Chapter 01 – Cloud Database and DBaaS Executive Summary
Chapter 02 – Cloud Database and DBaaS Overview
Chapter 03 – Key Success Factors
Chapter 04 – Global Cloud Database and DBaaS – Pricing Analysis
Chapter 05 – Global Cloud Database and DBaaS Background or History
Chapter 06 – Global Cloud Database and DBaaS Segmentation (e.g. Type, Application)
Chapter 07 – Key and Emerging Countries Analysis Worldwide Cloud Database and DBaaS Market
Chapter 08 – Global Cloud Database and DBaaS Structure & worth Analysis
Chapter 09 – Global Cloud Database and DBaaS Competitive Analysis & Challenges
Chapter 10 – Assumptions and Acronyms
Chapter 11 – Cloud Database and DBaaS Research Methodology
Thanks for reading this article; you can also get individual chapter-wise sections or region-wise report versions like North America, LATAM, Europe, Japan, Australia or Southeast Asia.
Contact Us:
Nidhi Bhavsar (PR & Marketing Manager)
HTF Market Intelligence Consulting Private Limited
Phone: +15075562445
sales@htfmarketreport.com
About Author:
HTF Market Intelligence Consulting is uniquely positioned to empower and inspire with research and consulting services to empower businesses with growth strategies, by offering services with extraordinary depth and breadth of thought leadership, research, tools, events, and experience that assist in decision-making.
This release was published on openPR.
Article originally posted on mongodb google news. Visit mongodb google news
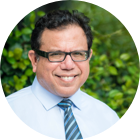
MMS • RSS
Posted on mongodb google news. Visit mongodb google news
MongoDB Queryable Encryption allows customers to securely encrypt sensitive application data and store it in an encrypted format within the MongoDB database. It also enables direct equality and range queries on the encrypted data without the need for cryptographic expertise. Adding range query support expands data retrieval options, allowing for more powerful search capabilities.
You can configure Queryable Encryption using the following methods:
- Automatic encryption: Allows encrypted read and write operations to be performed seamlessly, without requiring explicit encryption and decryption commands for individual fields.
- Explicit encryption: Offers the ability to perform encrypted read and write operations through the encryption library of your MongoDB driver, where you must define the encryption logic throughout your application.
“We’ve heard from customers that they need state-of-the-art security to protect their sensitive data, but not all of them have the specialized expertise to implement it. With MongoDB Queryable Encryption, we’re delivering just that—Queryable Encryption makes it easier for organizations to protect their data without compromising performance or compliance. The addition of range query support to MongoDB Queryable Encryption provides even more flexibility and powerful search capabilities, while ensuring encrypted data remains safe through its entire lifecycle. Queryable Encryption enables developers to perform expressive queries on fully encrypted data, helping customers across industries effectively manage sensitive information while retaining business-critical querying capabilities,” Kenn White, Security Principal, MongoDB, told Help Net Security.
Organizations across all industries and sizes can leverage the benefits of Queryable Encryption, including:
- Data protection: Ensures data remains encrypted throughout its lifecycle, minimizing the risk of exposure or breaches of sensitive information.
- Regulatory compliance: Provides the tools needed to meet data protection requirements, such as GDPR, CCPA, and HIPAA, through encryption at every stage.
- Streamlined operations: Simplifies the encryption process, eliminating the need for custom solutions, specialized cryptography expertise, or third-party tools.
- Separation of duties: Enables stricter access controls by preventing MongoDB and even customers’ database administrators from accessing sensitive data.
Article originally posted on mongodb google news. Visit mongodb google news
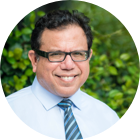
MMS • RSS
Posted on mongodb google news. Visit mongodb google news
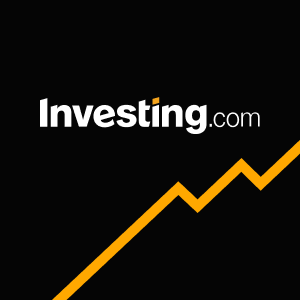
NEW YORK – MongoDB , Inc. (NASDAQ:MDB), a leader in prepackaged software services, announced today the redemption of all its outstanding 0.25% Convertible Senior Notes due in 2026. The total principal amount of the notes to be redeemed is $1,149,972,000. This action is in accordance with the terms set forth in the Indenture agreement dated January 14, 2020, with U.S. Bank Trust Company, National Association, as Trustee.
The company has scheduled the redemption for December 16, 2024, at which point holders of the notes will receive 100% of the principal amount plus accrued and unpaid interest from July 15, 2024, up to but not including the redemption date. Interest on the notes will cease to accrue following the redemption date.
Noteholders have the option to convert their notes into shares of MongoDB’s Common Stock at any time before the conversion deadline at 5:00 p.m. (New York City time) on December 13, 2024. The conversion rate has been set at 4.9260 shares of Common Stock per $1,000 principal amount of the notes. This rate includes an additional 0.1911 shares per $1,000 principal as a result of the redemption call. The conversion rate is subject to adjustment in certain circumstances as described in the Indenture.
MongoDB has elected to settle conversions with shares of its Common Stock, along with cash in lieu of any fractional shares, following the issuance of the redemption notice up to the conversion deadline.
This financial maneuver is part of MongoDB’s strategic financial management and is detailed in the company’s latest filing with the Securities and Exchange Commission. The information is based on a press release statement issued by MongoDB, Inc. on Wednesday. Investors and note holders are advised to review the terms of the redemption and conversion options as they make their financial decisions regarding the company’s notes.
In other recent news, MongoDB, a leading database platform, has been the subject of numerous analyst reviews following robust second-quarter earnings. The company reported a 13% year-over-year revenue increase, amounting to $478 million, primarily driven by the success of its Atlas (NYSE:ATCO) and Enterprise Advanced offerings. This impressive performance led to an addition of over 1,500 new customers, bringing MongoDB’s total customer base to over 50,700.
Analysts from DA Davidson, Piper Sandler, and KeyBanc Capital Markets have respectively raised their price targets for MongoDB to $340, $335, and $330, while maintaining their positive ratings. Oppenheimer has also increased its price target to $350, maintaining an Outperform rating. These adjustments reflect the company’s strong performance and the belief in its continued growth.
Looking forward, MongoDB’s management anticipates third-quarter revenue to range between $493 million and $497 million. The full fiscal year 2025 revenue is projected to be between $1.92 billion and $1.93 billion, based on the company’s recent performance and analyst expectations. These recent developments underscore the confidence in MongoDB’s potential and its capacity to maintain a positive growth trajectory.
MongoDB’s decision to redeem its convertible notes aligns with its strong financial position, as highlighted by recent InvestingPro data. The company’s market capitalization stands at $20.55 billion, reflecting its significant presence in the prepackaged software services sector. An InvestingPro Tip reveals that MongoDB holds more cash than debt on its balance sheet, which likely supports its ability to redeem these notes.
Despite the substantial redemption amount of over $1.1 billion, MongoDB’s financial health appears robust. The company’s revenue for the last twelve months as of Q2 2025 reached $1.82 billion, with a notable revenue growth of 22.37% over the same period. This growth trajectory is further supported by another InvestingPro Tip indicating that net income is expected to grow this year.
For investors considering the conversion option, it’s worth noting that MongoDB’s stock has shown resilience with a 10.22% price return over the past three months. However, the company’s high Price / Book multiple of 15.09 suggests that the stock is trading at a premium relative to its book value.
These insights are just a snapshot of the comprehensive analysis available on InvestingPro, which offers 11 additional tips for MongoDB, providing a more complete picture for investors evaluating the company’s financial strategies and market position.
This article was generated with the support of AI and reviewed by an editor. For more information see our T&C.
Article originally posted on mongodb google news. Visit mongodb google news