Month: April 2025
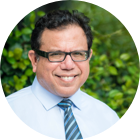
MMS • RSS
Posted on mongodb google news. Visit mongodb google news
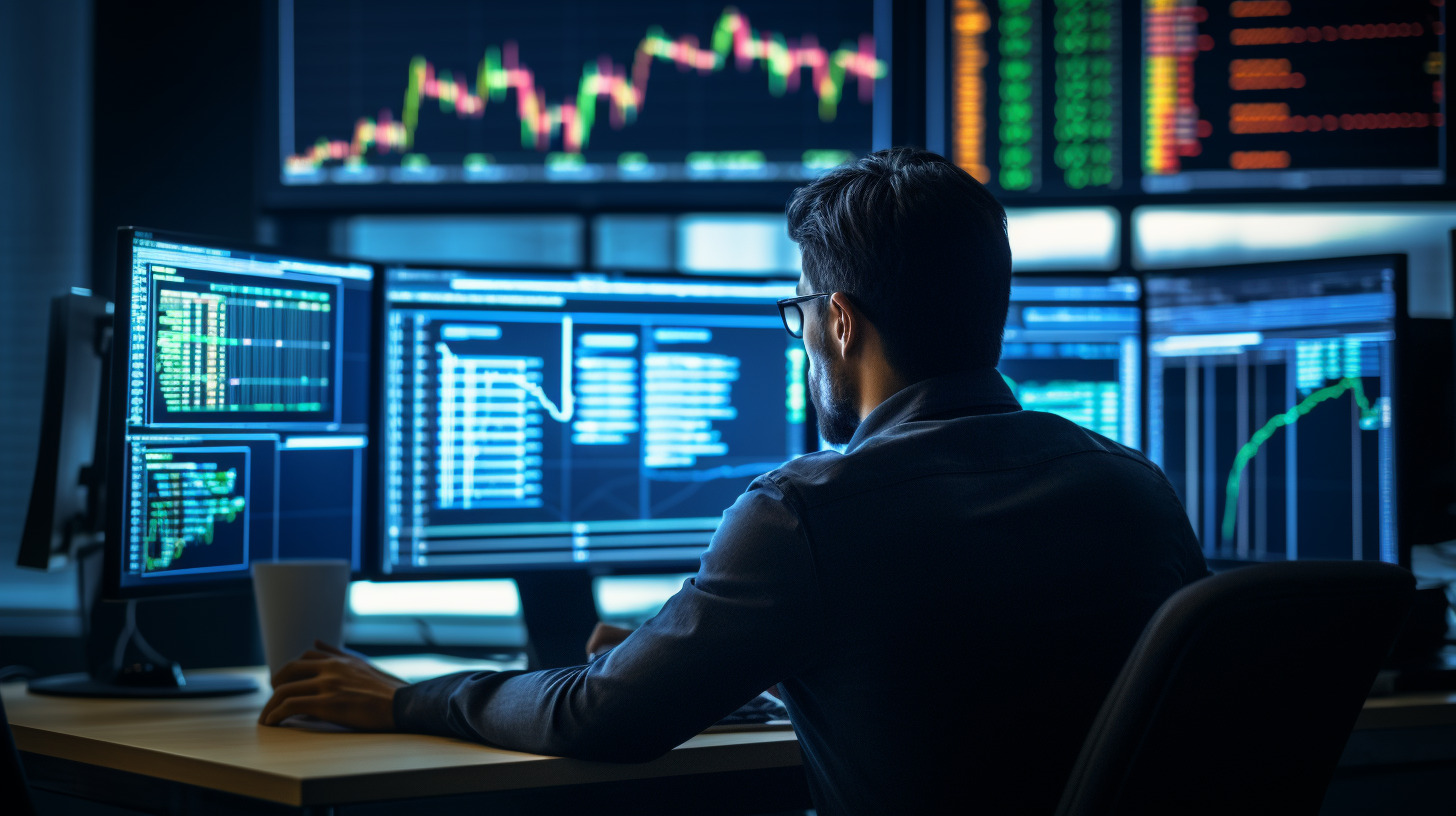
Artificial intelligence is the greatest investment opportunity of our lifetime. The time to invest in groundbreaking AI is now, and this stock is a steal!
My #1 AI stock pick delivered solid gains since the beginning of 2025 while popular AI stocks like NVDA and AVGO lost around 25%.
The numbers speak for themselves: while giants of the AI world bleed, our AI pick delivers, showcasing the power of our research and the immense opportunity waiting to be seized.
The whispers are turning into roars.
Artificial intelligence isn’t science fiction anymore.
It’s the revolution reshaping every industry on the planet.
From driverless cars to medical breakthroughs, AI is on the cusp of a global explosion, and savvy investors stand to reap the rewards.
Here’s why this is the prime moment to jump on the AI bandwagon:
Exponential Growth on the Horizon: Forget linear growth – AI is poised for a hockey stick trajectory.
Imagine every sector, from healthcare to finance, infused with superhuman intelligence.
We’re talking disease prediction, hyper-personalized marketing, and automated logistics that streamline everything.
This isn’t a maybe – it’s an inevitability.
Early investors will be the ones positioned to ride the wave of this technological tsunami.
Ground Floor Opportunity: Remember the early days of the internet?
Those who saw the potential of tech giants back then are sitting pretty today.
AI is at a similar inflection point.
We’re not talking about established players – we’re talking about nimble startups with groundbreaking ideas and the potential to become the next Google or Amazon.
This is your chance to get in before the rockets take off!
Disruption is the New Name of the Game: Let’s face it, complacency breeds stagnation.
AI is the ultimate disruptor, and it’s shaking the foundations of traditional industries.
The companies that embrace AI will thrive, while the dinosaurs clinging to outdated methods will be left in the dust.
As an investor, you want to be on the side of the winners, and AI is the winning ticket.
The Talent Pool is Overflowing: The world’s brightest minds are flocking to AI.
From computer scientists to mathematicians, the next generation of innovators is pouring its energy into this field.
This influx of talent guarantees a constant stream of groundbreaking ideas and rapid advancements.
By investing in AI, you’re essentially backing the future.
The future is powered by artificial intelligence, and the time to invest is NOW.
Don’t be a spectator in this technological revolution.
Dive into the AI gold rush and watch your portfolio soar alongside the brightest minds of our generation.
This isn’t just about making money – it’s about being part of the future.
So, buckle up and get ready for the ride of your investment life!
Act Now and Unlock a Potential 10,000% Return: This AI Stock is a Diamond in the Rough (But Our Help is Key!)
The AI revolution is upon us, and savvy investors stand to make a fortune.
But with so many choices, how do you find the hidden gem – the company poised for explosive growth?
That’s where our expertise comes in.
We’ve got the answer, but there’s a twist…
Imagine an AI company so groundbreaking, so far ahead of the curve, that even if its stock price quadrupled today, it would still be considered ridiculously cheap.
That’s the potential you’re looking at. This isn’t just about a decent return – we’re talking about a 10,000% gain over the next decade!
Our research team has identified a hidden gem – an AI company with cutting-edge technology, massive potential, and a current stock price that screams opportunity.
This company boasts the most advanced technology in the AI sector, putting them leagues ahead of competitors.
It’s like having a race car on a go-kart track.
They have a strong possibility of cornering entire markets, becoming the undisputed leader in their field.
Here’s the catch (it’s a good one): To uncover this sleeping giant, you’ll need our exclusive intel.
We want to make sure none of our valued readers miss out on this groundbreaking opportunity!
That’s why we’re slashing the price of our Premium Readership Newsletter by a whopping 70%.
For a ridiculously low price of just $29.99, you can unlock a year’s worth of in-depth investment research and exclusive insights – that’s less than a single restaurant meal!
Here’s why this is a deal you can’t afford to pass up:
• Access to our Detailed Report on this Game-Changing AI Stock: Our in-depth report dives deep into our #1 AI stock’s groundbreaking technology and massive growth potential.
• 11 New Issues of Our Premium Readership Newsletter: You will also receive 11 new issues and at least one new stock pick per month from our monthly newsletter’s portfolio over the next 12 months. These stocks are handpicked by our research director, Dr. Inan Dogan.
• One free upcoming issue of our 70+ page Quarterly Newsletter: A value of $149
• Bonus Reports: Premium access to members-only fund manager video interviews
• Ad-Free Browsing: Enjoy a year of investment research free from distracting banner and pop-up ads, allowing you to focus on uncovering the next big opportunity.
• 30-Day Money-Back Guarantee: If you’re not absolutely satisfied with our service, we’ll provide a full refund within 30 days, no questions asked.
Space is Limited! Only 1000 spots are available for this exclusive offer. Don’t let this chance slip away – subscribe to our Premium Readership Newsletter today and unlock the potential for a life-changing investment.
Here’s what to do next:
1. Head over to our website and subscribe to our Premium Readership Newsletter for just $29.99.
2. Enjoy a year of ad-free browsing, exclusive access to our in-depth report on the revolutionary AI company, and the upcoming issues of our Premium Readership Newsletter over the next 12 months.
3. Sit back, relax, and know that you’re backed by our ironclad 30-day money-back guarantee.
Don’t miss out on this incredible opportunity! Subscribe now and take control of your AI investment future!
No worries about auto-renewals! Our 30-Day Money-Back Guarantee applies whether you’re joining us for the first time or renewing your subscription a year later!
Article originally posted on mongodb google news. Visit mongodb google news
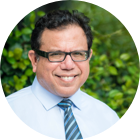
MMS • Craig Risi
Article originally posted on InfoQ. Visit InfoQ
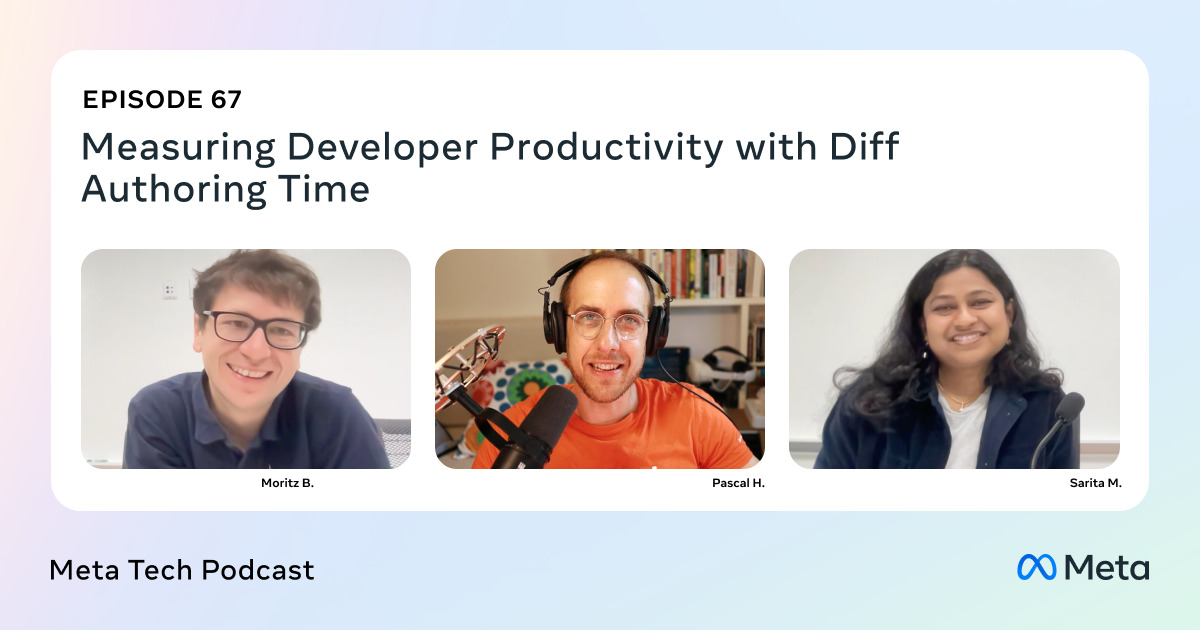
Tracking developer productivity metrics is essential for understanding and improving the efficiency of software development workflows. In fast-paced engineering environments, small inefficiencies can accumulate, impacting overall delivery timelines and code quality. By leveraging precise metrics, organizations can identify bottlenecks, assess the impact of new tools, and make data-driven decisions to enhance developer experience.
Now we can add another new metric to help track the development process better: Diff Authoring Time (DAT). DAT is a new metric developed by engineers at Meta to measure the duration required for developers to submit changes, known as “diffs,” to the codebase, which they shared in a recent Meta Tech Podcast. By tracking the time from the initiation of a code change to its submission, DAT offers insights into the efficiency of the development process and helps identify areas for improvement.
Implementing DAT involves integrating a privacy-aware telemetry system with version control systems, integrated development environments (IDEs), and operating systems. This setup allows for the precise measurement of the time developers spend authoring code changes without compromising privacy. The data collected through DAT enables Meta to conduct rigorous experiments aimed at enhancing developer productivity.
For instance, DAT has been instrumental in evaluating the impact of introducing a type-safe mocking framework in Hack, leading to a 14% improvement in authoring time. Additionally, the development of automatic memoization in the React compiler resulted in a 33% improvement, and efforts to promote code sharing have saved thousands of DAT hours annually, achieving over a 50% improvement.
The significance of DAT lies in its ability to provide a precise yet comprehensive measure of development productivity, facilitating data-driven decisions to enhance engineering efficiency. By aligning internal development workflows with an experiment-driven culture, DAT supports continuous improvement in software engineering practices.
As highlighted in the Meta Tech Podcast, engineers Sarita and Moritz discuss the challenges of measuring productivity, the implementation of DAT, and the new capabilities it unlocks for developers. Their insights underscore the importance of accurate productivity metrics in fostering an environment of continuous improvement within Meta’s engineering teams.
In summary, Diff Authoring Time serves as a tool for Meta to assess and enhance developer productivity, enabling the company to make informed decisions that streamline workflows and improve the overall efficiency of its engineering processes.
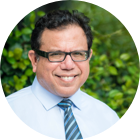
MMS • Anil Rajput Rema Hariharan
Article originally posted on InfoQ. Visit InfoQ
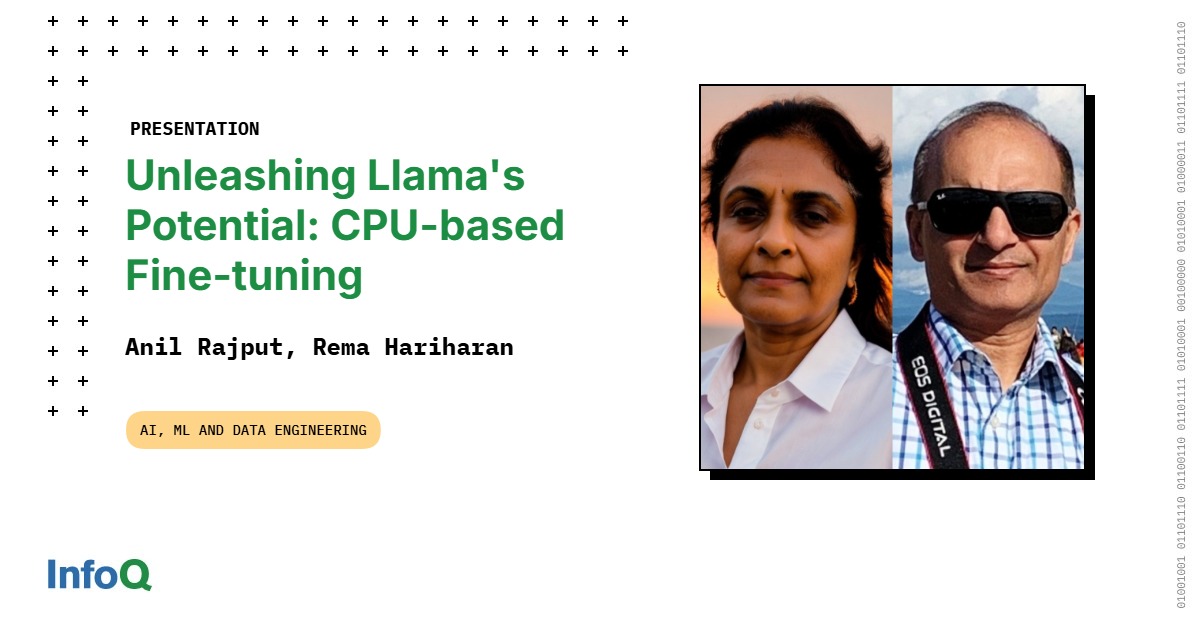
Transcript
Rajput: I come from the hardware background, and we want to optimize. We run benchmarks, and you know the benchmarks are limited use. I wanted to always understand what customers are doing, what are their environments running. One of the things at QCon, I found at the time that the hottest topic in 2018 and 2019 frame, number one is Java. I think 70% to 80% of attendees were Java enterprise and they were solving related problems. The hottest topics were underneath CPUs, most of the deployment. That’s my experience.
Then, suddenly COVID happened. At the time I used to be Intel, and from Intel I changed to AMD. I’m joining back QCon, and that is what has happened since then. CPU has gone tiny and everything is GPU. The whole conference here is no longer Java, on the products, but it’s all about LLMs. I’m also trying to change with that. The interesting topic we bring you is not exactly the same, but the LLMs in particular, Llama running on the CPU. Hold your thing for the GPU part, but we plan to talk about CPUs.
How many folks are aware of the CPU architecture? The reason I wanted to check because many of the optimizations and discussions we want to do here is software-hardware synchronization. Because when we talk about the performance and we hear from many customers that, we were on-prem or we are going into the cloud, and their goals are, I want to save 10%, 20% of TCO, or I want to actually reduce the latency, or I want to do other things. Sure, there are a lot in the architecture of the application, but you can have actually significant performance improvements just from understanding underneath hardware and leveraging it to the best. We want to show you a particular example, how you can actually be aware of the underneath hardware that would be deployed, and design your thing, or architect. It would have both roles, you would have the deployments because some of the decisions are at the deployment time, and others even when you’re writing the code or application.
Hardware Focused Platform Features
We’re not talking about the GPU, and CPU plus GPU interactions, because those analyses become quite different, and how the data is flowing between them. It’s mostly a Llama model or that area being deployed on a CPU platform. Let me share a couple of components, and I’ll talk about each one of them when we take CPUs, cores, simultaneous multi-threading, or in Intel’s case we call Hyper-Threading, the different kind of caches. In caches particularly, I’ll show later a chiplet architecture which is unified. That’s a big change happening in the last three, four years of the deployment.
Of course, the memory when we talk memory, then there are two things, memory capacity and memory bandwidth. Memory latency too, but you don’t have to worry that much about that part. It’s usually memory capacity and bandwidth. Let me start with the CPU side. I wanted to show you one CPU and the two CPUs. The reason it’s good to know about those parts, is because many platforms have the two CPUs, we call them dual socket, and it becomes like two NUMA nodes. Unless you have to cross the application on both the CPUs, that means a cross-socket communication, you want to avoid that. The only time you need that large database, and it needs to have the memory capacity which is needed from both sockets, then it is good.
Otherwise, you want to keep each process and memory local. If it is a 1P platform, you don’t need to worry, but if it is 2P platform, you want to be a little bit aware. I/O side also becomes more interesting that is the I/O device sitting on which CPU, that’s usually much better to keep your process and thing on that socket than the other one. We are not going into the I/O area. If also you work on the I/O disk or network cards, they’re usually on one of the sockets, and how that processing comes are separate talks, actually. Just be aware, is the platform you’re using a 1P or 2P?
Let me go into a little more detail that when you talk about the CPU, typically, you would see a lot of cores, and within a core, you would have L1, L2 caches, and then L3 cache would be underneath. Then of course, you have the memory and I/O or NIC cards. That is a typical design. When we talk about the core, the core you would have SMT thread, L1 cache, L2 cache. This is the only place on the top, the SMT thread which is something you need to think. Do you need to worry about the SMT thread part?
The only part you need to think about it, it just gives you twice the number of threads. When you are designing from the application side, the thread pool size, if it is going to be, let’s say N core system and SMT on, then you want to make sure your thread pool or setting are not hardcoded, they can check how many vCPUs are available. Then you set accordingly. Because I have seen that kind of mistake where people hardcode, we found even in MongoDB or those kinds of things where they hardcode the core at 32 or 16. Suddenly you see when you try to deploy them, a scale-up model, they don’t scale and then you find that the programmer actually hardcoded it.
Now, let me show you, because I talked about the chiplet architecture, what is the difference between a unified L3 and a chiplet architecture. Most of the Intel systems, Intel Xeon, when their GNR is coming next, GNR will be the first chiplet type. Anything before has been unified, you see all the cores sitting within that socket, same L3. On the chiplet side, we try to create a group of cores associated to L3 in each one. That is the chiplet architecture. It has many benefits at the hardware level on the yield, but it has a benefit on the software side. Let me show you what benefit it gives you. I like to show it this way, a little more clarity on the chiplet. One of the benefits you would see that L3 associated to each chiplet, it cannot consume the whole memory bandwidth. On a unified L3 given few cores, if you run a noisy application, it could actually consume the whole memory bandwidth and the rest of the program may not get much bandwidth left, and poor latency suddenly when the noisy neighbor comes in.
One of the benefit of the chiplet architecture is that each chiplet cannot consume the full memory bandwidth. As an example, one platform, if it has 400 Gbps memory bandwidth, one chiplet can’t do more than 40 to 60. Number one, it protects you from the noisy neighbor scenario. Number two, we have the setting in the bus where you have NPS, NUMA Per Socket partitioning. You can set up to four. In this case, it is actually dividing your memory channel into four categories. Most of the cloud are running with NPS1 because they don’t want to manage the schedule of the memory. When you are running in your on-prem, you could actually create four clear NUMA nodes, and it will give you the memory bandwidth.
One of the benefits you would see in this scenario is that, let’s say you wanted to deploy an application on two chiplets, so two L3s, and then you have another application in another NUMA node. What you would see is that they are not colliding on the same memory bandwidth from the channels, and each of the new applications get their own L3. You are able to deploy an application where you have a consistent performance and they’re not consuming each other’s memory or memory bandwidth or the L3 clashing. These are the benefits. The unified L3 does give the benefit where if you need to exchange the data among the L3 or application, that part is faster. Other than that, most of the chiplet architecture benefits outweigh that kind of bandwidth. That’s the reasoning you will see the GNR also going a very similar path of the chiplet. As these caches are increasing, the number of cores are increasing, you cannot have just everything on one unified. It’s just a limitation in the architecture, by the time you have a huge number of cores.
Focus: Software and Synchronization (AI Landscape and LLMs)
Rema will talk with regards to LLMs or the Llamas, what is the role of the SMT, simultaneous multi-threading, what do you need to think about to leverage it best. Same thing on the core that when you have a lot of cores increasing, how do you want to leverage it or what you need to be aware of. Caches play a very important role, and I can tell you from the EDA tools or other areas that you could have 20%, 30% improvement. Because when you’re dividing your problem, just like in the LLM and other space too, if you fit it into the cache versus not fitting, the performance improvements and differences are not 4% or 5%, they are like 20%, 30% as soon as you start fitting in versus moving out. That part for a particular architecture, the more you are high frequency trading or tools or many response time sensitive, throughput time sensitive, you have profiling tools where you can see, is it fitting in or how much is missing? What do I need to adjust? Those kind of specific optimizations from different tools. That part is very important.
Then the memory capacity and the bandwidth part. Because LLMs, as Rema will show you later, when it is compute bound, when it is memory bandwidth bound, and what kind of decision can you do, and actually memory capacity on that extent. I just wanted to give you these details first because Rema will extensively use, this is my analysis and I’m memory bandwidth bound here, and I’m trying to fit in the cache or other area of the chiplet, in her talk. Just wanted to give you a quick idea that even though LLMs, if you look at the bigger picture, it is actually a pretty tiny piece and very complex piece just for the reference. I’m sure you are all aware where the LLM and ChatGPT fits, and another part also that with regard to the timelines, this part is exponentially increasing and changing. We are in exciting times from this change.
Llama
Hariharan: Let’s Llama. Excited to be talking about this Llama who is right now sitting on top of the Andes and everything. Let’s talk about the real thing that we are all interested in. Why Llama in the first place? Why are we not talking about other models? There are so many GPT models that we are all aware of. One of the main reasons to talk about Llama and why we use it for benchmarking and workload analysis is it is a small model. Particularly when we are talking about running it on a CPU, this is one of the smaller models. It comes in multiple sizes, but we still have the small model available.
Relative to other GPT models, this is the smaller one. Not only that, I think we are all aware of this, that Llama was actually trained on publicly available data and it’s fully open source. That’s something that protects us in whatever usage we have. A lot of our customers like to train it for their own select areas. That keeps them more protected because it was trained on publicly available data. There’s nothing to worry about in terms of lawsuits and stuff. This is why I think it’s a small model, open source, and it can be trained.
Let’s look at what our Llama does in action. How does it actually function here? There are two phases of Llama: the prefill phase and the decoding phase. What happens in the prefill phase? Prefill phase, you type something, and whatever you type, basically the model is loaded, and your input data, which is in this case ‘Computer science is’ is what you’re typing, and it is going to predict what’s the next word or next token. The whole model is loaded and the whole model actually works on everything that you have typed. In this case it’s just three words, but you could be sending a whole book there. You could be typing a whole book and putting it as the input data. It processes the entire data you have submitted and then it produces the very first token. Now it need not be one token, it could be a probability distribution over a bunch of tokens. Without loss of generality, let’s just say it’s one token that comes out of it. This portion of the work is extremely compute intensive. We call this the prefill phase. That’s the first phase of it.
The second phase is the decoding phase. What happens is it takes the previous token, and the KV cache that was built and whatever else was created there, and creates the next token, and then the next token, and the next token, and so on, until it reaches the end of sentence. This is the decoding phase. Because you are actually loading the model over and again every time. I said the model is small, but it’s still not small enough to fit into our caches. You really have to pull the model from the memory and load it multiple times, portions of it every time and so on. When you’re doing that there’s a lot of memory bandwidth involved in the second phase. This decoding phase is highly memory bandwidth intensive.
Basically, what happens in the prefill phase is tokenization, embedding, and encoding, and everything. The decode phase, you are actually going to iterate through the whole thing over and again, either deterministically or probabilistically. Like I said, each token produced is actually a probabilistic distribution over a bunch of tokens. You can set configuration parameters where you are actually just going to be greedy and pick the most probable one and move forward. That’s the fast way to do it, but there are ways to do that. Without loss of generality, let’s just say that these are the two stages of the model and we’re taking one token at a time and moving forward.
Now let’s look at the Llama internals. When I say internals, you’re driving a car. You want to look at the inside of the car. You have the engine, you have the transmission, all these things. If you have to make sure that your car is functioning well, you have to make sure you have a good engine, make sure you have a good transmission, and all those things. Let’s look at the internals here. What we see is when Llama is running, there’s going to be a lot of matrix multiplication that happens. Matrix multiplication is one of the key operations that happens here. Dot product is another one. One is cross product, dot product. Scaling and softmax computation. Weighted sum. Last but not the least, passing through multiple layers and aggregation of everything. These are the primitives that go into the Llama internals.
In order to get the best performance, what we really need to do is to optimize these primitives. Not just optimize these primitives, that happens through all the BLAS and MKL libraries that have been written. Also, optimize it for a given hardware platform. Now, the optimization, some of it is common optimization. Other part is specific to a particular hardware. That’s about something we have to be aware of, what is the latest thing that optimizes these? What are the latest libraries that optimize these for a particular hardware that you are running it on?
Next, let’s talk about metrics. Metrics is clearly something that comes from the user. A user decides what the metrics are, what is important to them. Let’s look at it. I’m showing you pretty much the same diagram that we showed before, but slightly differently placed. What happens in any LLM, not just Llama, you give the input. You are giving the input, and then if you have typed in ChatGPT, which all of us do, we’re waiting for some time, especially if the input is long. It goes blink, blink, blink. I don’t know whether my words are lost in the ether space or what happened. That is the time that the initial prefill phase is working on it. Then at the end of it comes the first token. Something is happening there, and I’m happy.
Then after that, all the tokens follow. Sometimes you can see when the output is large, as you are trying to read, there’s more that’s coming. It doesn’t all appear in one shot. All these tokens are coming slowly. Token is not exactly a word, but tokens are usually converted to words. I won’t go into the details of that at this point. What are the metrics here? The time it took from the time I gave my input to when I started seeing something appearing on my screen. That’s the time to first token. I’m iterating it just for completeness of the talk. Then all these tokens appear all the way to the end. The total latency is something that’s very important. I care whether I got my entire response or not. Inverse of that is the throughput. One divided by latency is the throughput, basically. Throughput is something that’s super important for all of us. We are ready to wait a little bit longer if we are really asking it to write an essay. If I’m just asking a yes or no question, you better be quick. I’m going to pretty much say the same things.
Basically, throughput is a real performance. Throughput actually marks how the system is being used. TTFT is something that’s super important. It is a result of that compute intensive phase. The TTFT is something that can be manipulated quite a bit by giving specialized hardware many times. For example, AMX is used as well. That definitely helps reducing the TTFT. GPUs also reduce TTFT. Throughput, on the other hand, is mostly controlled by the memory bandwidth, because the model is loaded over and again. The larger your output gets as it is pumping through more tokens, the throughput is controlled by the memory bandwidth.
Deployment Models – How Are Llamas Deployed?
Now let’s talk about the deployment models. I just thought it is important to show that our CPUs fit in whether you’re using GPUs or just CPUs. Smaller models particularly, can be run very well on street CPUs. Even when you’re running on GPUs, a CPU is involved. A CPU is connected to the GPUs. That typically suits larger models and allows for mixed precision and better parallelism and all that. Talking about GPUs, you can see deployments like these as well. They basically have a network of GPUs. What is important in this case is how these GPUs are connected. GPUs have to be connected through an NVLink or an InfiniBand. They need fast connections between all these GPUs and the CPU and GPU as well.
Typically, CPU connects to a GPU through the PCIe. It can get even more complex. You can have not just a network of GPUs, but even the inputs can be fed in a different way. You can have audio input, video input, and they’re processed differently, fed into the model, and then there are layers that are being handled by different sets of GPUs. You can make this as complex as you want to. We’re not going to get into all these complexities. Life is difficult even if you take a simple case. Let’s stick to it.
Llama Parameters
Let’s get into the details. First, let’s get familiar with some of the jargon that we use. They’re not exactly jargon, we’re all familiar with it, but let’s get them on the board here. The main three parameters that we’ll talk about are input tokens, output tokens, batch size. Those are three things that you will hear whenever you look at any benchmark publication or workload details corresponding to not just Llama, but in general, all LLMs. Input tokens, clearly that’s what you’re typing on the screen. That’s the input you’re providing. A paragraph, a quick set of prompts, whatever. Output tokens is what it produces. Tokens, again, are not the words that you see, but tokens are related to the words that you see. What is a batch size? Batch size can go all the way from one to whatever that you want. What does it look like here? Basically, if you give just one thing at a time, you give one prompt, wait for the response, then give the next prompt. You can say, tell me a story. Llama tells you a story.
Then, tell me a scary story. It tells you another one, and so on. You can give multiple prompts at the same time, like him, he’s showing an example of batch size equal to 4 there. All of them will be processed together. What can also happen is that some prompts are done earlier than the others. The parallelism doesn’t stay the same throughout. They are also working on things like dynamic batch sizes and so on. We’ll not get into all those details right now here. We’ll keep it simple. We’ll assume that a batch size of 4 is given, and then batch output of 4 is produced, and then next 4, and so on, if I say batch size equal to 4. What is a Llama instance? That’s the first thing I put there. Llama instance is your Llama program that you’re running. You can run multiple instances of these on the same system. You don’t have to run just one. You can run multiple instances. We will talk about how things scale and so on. Each instance is an instantiation of the Llama program.
Selecting the Right Software Frameworks
Let’s talk about selecting the right software frameworks. First, everything started with PyTorch. That’s the base framework that we started with. It has good support. It’s got a good community support, but except it doesn’t have anything special for any particular hardware or anything like that. It’s not optimized. It’s a baseline you can consider. Then came TPP that was created by Intel. TPP was done by Intel. A lot of what you see in TPP is optimized more for Intel. Given that it is optimized for Intel, it actually works pretty well on AMD as well. We also get a good gain going from the baseline to going to using TPP.
Then came IPEX. IPEX actually incorporates TPP right within. IPEX was built on top of TPP. That, again, was done by Intel. Also, benefits Intel a little bit more than it benefits AMD. Last but not the least is our favorite thing, which is ZenDNN. How many of you have used ZenDNN? It was recently released. The thing about ZenDNN is it builds on top of what is already there, obviously. It was recently released. It gives a good boost to the performance when you run it on AMD hardware, particularly. Let’s look at some numbers since I said this is better than this and so on.
As you can see, if I mark the baseline as equal to 1, so what I’m plotting here is various software optimizations: baseline, TPP, IPEX, and then finally Zen. You can see going from baseline to TPP is more than a factor of 2. These are all performance based on our hardware only. There are no competitive benchmarking numbers or anything presented in this talk. Going from baseline to IPEX, you get nearly a 3x. It’s a lot more than that. Then with Zen, you get even more of a boost above where IPEX is. One thing I have to say that in Zen, the advantage that you get will keep increasing as the batch size increases. It is actually optimized to benefit from higher core counts that we have, number one.
Secondly, the large L3 caches that we have as well. There’s a lot of code refactoring that went into it, and there’s a lot of optimization that has gone into it, but the benefits actually increase. As you can see with two batch sizes that I’ve shown, 1 and 16, it shows a 10% advantage over IPEX when you start, and then it goes to a little higher, and then I know I’ve not added those graphs, but the benefit actually does increase as the batch size increases.
Hardware Features and How They Affect Performance Metrics
Let’s come to the core of this talk. Here you have various hardware features, and the question is, how are they going to affect my performance metrics? How do we optimize things in order to use all these things optimally? Let’s first talk about cores. In this graph, what I’m showing here is how Llama scales. I’m using only one instance of Llama, size 16, size 32, 64, and 128. You can see I’ve gone eight times in size from the leftmost to the rightmost. That’s a factor of 8 involved, but the amount of gain that I’ve got is less than 50%. The software does not scale. There are multiple reasons to it. We can get into that.
Basically, the performance that you get with size 16 seems to be mostly good enough. Maybe I should run multiple of 16s rather than run a large instantiation of the same thing. What I plotted earlier, what I showed in the previous graph was basically throughput. The throughput doesn’t scale a whole lot, and that’s the main thing that as somebody who’s trying to use the system and getting the most out of it, I’m interested in throughput. The user is also interested in the TTFT. The TTFT does benefit, not a whole lot, but there is some benefit when you make the instance larger. Not a whole lot. As you can see, going from 16 to 128, it dropped by about 20% or something. Not a whole lot. That parallelism and that CPU capacity that you’re throwing in does benefit in terms of performance.
Moral of the story, additional cores do offer only incremental value. TTFT also benefits. The reason these two have to be taken together is there could be possibly a requirement on the TTFT when you’re working with a customer to say, I want my first token to appear within so many milliseconds or seconds, whatever it may be. You have to bear that in mind when you’re trying to say, can I make the instance really small and have a whole lot of them? There is another thing to it as well, which is, what happens here is when you have too many instances, each instance is going to consume memory. I’ll come to that later. You may not have that much memory to deal with it.
Next, let’s talk about SMT, the symmetric multi-threading. You have a core and then you have a sibling core. On each core, there are two of them that are operating. Are you going to get benefit from using the SMT core? Let’s take a look. The blue lines here show you what’s a performance improvement. I’m only plotting the improvement. I’m not giving you raw numbers, nothing. When you’re running a single instance, so this is a single instance of size 16 that I’m running. What have I done there? There’s nothing else running on the system. Remember my CPU has 128 cores. These are all run on our Turing. We have 128 cores, but I’m using only 16 of them. That means the background is very quiet. Nobody else is using the memory bandwidth. Memory bandwidth wise, we are not constrained at all.
The only constraint that’s coming here is from the core itself. It’s CPU bound. What happens there, you are getting a good boost by using the SMT thread. With and without SMT thread, if you actually run it twice, you can see that you get a good boost. The orange line on the other hand is the kind of boost that you will see when you’re actually running everything. You’re running all the 16, 16, 16, you’re running all of them together. What happens then, you’re actually going to be constrained by the memory bandwidth. Your memory bandwidth becomes a constraint. Really, there is no advantage or disadvantage. As you can see, the percentage is in single digits. The statistical variation is what you’re seeing there, nothing else.
Moral of the story again, SMT does not hurt, even in the fully loaded case, but it is going to give you a lot of benefit if the background is going to be quiet. Particularly that’s important because, let’s say you’re running on a cloud, on AWS or one of these things, you don’t assume everybody is running a Llama. You take an instance of size 16 and you’re running there. Maybe most likely that everybody else is quiet or doing very little thing. You will get that benefit, so use your SMT there.
This is the most important thing. You will see a big difference here, memory bandwidth. What is the role of memory bandwidth? What I did was, on a Turing system, we have a bandwidth of 6,000 megatransfers per second. I clocked it down to 4,800, 20% reduction in the bandwidth. When I did that, the question was, how much are we going to affect the overall performance? Remember I told you that the prefill phase is affected mostly by the CPU and the decoding phase is affected by the memory bandwidth. When you do that, that’s a substantial difference. Here is the opposite role. There are two things that I plotted here.
The first thing is a single instance, the dark brown one. When I just run a single instance, that means it doesn’t matter my memory bandwidth, whether it’s 6,000 or 4,800, I have plenty, for a single instance that’s running. When I run all the instances, that is when you can see the memory bandwidth really hitting you hard and it gets affected very badly. Basically, moral of the story here is that, use as much bandwidth as you can get. If the cloud is going to constrain you for the amount of bandwidth that you’re going to use, it’s worth paying for that if you have to pay for extra bandwidth. I know it can be manipulated how much bandwidth each instance gets.
Next let’s talk about role of caches here. I don’t have a graph here, but I can talk through this. Caching is important. Remember we are constrained on memory bandwidth. If we can get the data from caches, it’s better. However, you cannot fit the whole model into cache. What really happens is your model gets loaded over and again. Just by nature of this particular workload, it’s a use and throw model. That means you’re going to load the weights, use it to compute something, and that’s it. You’re not going to reuse it. The only way you can reuse it is if you use a higher batch size. If you’re going to process 64 of them together, so all 64 will be using the same type of weights in order to do the computation.
Otherwise, if you’re just using a batch size equal to 1, it is a use and throw model. You can actually see a very large L3 miss rate. Using a higher batch size is crucial in order to increase the caches. Earlier I talked about what happens as you scale a particular instance. I’m going to talk about what happens when you change the number of instances, how you use more instances, how does your performance scale. As you can see, now the two bars that you see there, the blue one, that’s what I’m using as a basis, is running just a single 16-core instance. The orange ones are running 16 of the 16-core instances. I’m running 16 of them in parallel.
If everything was ideal and everything you would have had, the height of the orange bar would have been 16. It would have been 16 times performant, but no, there are other constraints that come into play. Your memory bandwidth is a big constraint. You don’t get 16x, but you get nearly 10x, 12x performance overall compared to the single instance where there is no memory bandwidth constraint and you’re running all of them together. This is for different situations. Chat is where you have short input, short output. Essay is where short input, long output. Summary is very long and short. Translation is both long. In all the cases, you’re getting at least a 10x improvement against the baseline that we’re looking at. Basically, running parallel instances is the way to go, pretty much.
I talked about batches a lot. I said use higher batch sizes. What is our return? Throughput-wise, look at the return that we are getting. As the batch size increases, going from 1 to 128, I got more than 128x of performance. The reason is I’m getting much higher L3 hit rates. I’m getting things from the cache instead of going to the memory. I’ve reduced my latency. I’ve made my CPU much more performant here. I’m using my cores a lot more. I’m getting more than 128x return when I’m using 128 batch size. You don’t get anything for free. The place where it hurts is the TTFT. Your TTFT actually goes up also. That’s not a good thing. That makes sense.
If I’m working on 20 projects at the same time, everybody is going to be complaining. All my customers are going to be complaining that I’m not giving them the solution, but yes, I’m working day and night. That’s what matters to us as users of computers. We want to use our machines day and night and get the maximum throughput. After one month, I think everybody will get the answer when I’m working on 20 projects, but next day, probably not. That’s what we are seeing. That comes at a cost of TTFT. The TTFT does grow as well as you are running more in parallel.
These are various things that I already said. Do not use larger instances, if you can afford it. Use more instances. In order to harvest performance, use larger batch sizes. Also, the whole thing is going to be a balancing act for the most part between TTFT and overall memory needs. I said memory needs now, and I want to get into it immediately now after this. TTFT is a requirement that’s going to be placed on you by a customer. Memory needs is something that is going to grow as the number of parallel instances increases and as the batch sizes increases as well. Some formulae out there: fundamentally, I want to say this, the memory need comes from three different factors. Number one, the model itself. The larger the model, the more you’re going to load. If you have an 8 billion model, so each one is going to take 2 bytes. The next thing that is going to add to the memory is activations.
Last but not the least, the KV cache as well. The KV cache keeps growing as you’re building it as well, as you’re processing more. The total requirement comes from all three of them together. Basically, if you’re having multiple instances, in this case, let’s say I computed my need for memory as 41 gigabytes, so 41 times 32, if I start 32 instances in parallel. I said 32 instances, because typically on AMD systems, we like to keep an instance on one thing called the CCD or the Core Complex Die, which has 8 cores. We have 32 of them, so 8 times 32 is our whole system. That comes to 1.3 terabytes, which is really close to the total amount of memory I have on the system, 1.5 terabytes. This is when, when you have too many instances, you start seeing swapping. This calculation is something that we urge you to do in order to get a good idea of how to get the maximum out of the system. You don’t want swapping. Swapping is not a good thing.
I know I did a back-of-the-envelope calculation there, and did that. That calculation, most of the times is slightly under. It depends on which framework you are using. With ZenDNN, it’s pretty close by, as you can see, in most cases. In the case of IPEX, it was using even more memory. I have seen this go the other way as well, not for Llama, for some of the other use cases that we have run, where ZenDNN will take more memory and so on. I’m not making a statement on this here at all. The point is you have to be aware of that this is only a back-of-the-envelope calculation, but you have to look at what your framework is using.
If you’re using ZenDNN or IPEX, whichever it is, just see how much total memory that your instance is going to use. This is again one more thing that I want to say, free floating versus dedicated. Please pin your instances. This is probably the worst case I have shown, happened at least in one case. Doesn’t always happen, but when you pin it, each one is going to run on a different set of cores, and you’re going to get the returns proportionately. When you don’t pin, there is no telling. Pretty much all of them will run on the same bunch of cores, or they will be context switching back and forth. Either way, you pay the penalty. This is the worst case that I’ve shown. It’s not going to be as bad as this, but it can be as bad as this.
Summary
Recommendations for optimization: you know that the initial part of the run is core bound, and the second part is memory bound, so use more memory bandwidth if you can get it. Parallelism helps. Use the best software that you can use for your hardware. For Zen, definitely we recommend zentorch. These things will evolve with time as well, but this is where you have to do your due diligence and homework and identify what is the best software that fits your case. Pin instances as much as possible.
Questions and Answers
Participant 1: How are you capturing some of these metrics? What specific metrics and what tools are you using for the metric calculation of observability?
Hariharan: We know how many tokens we are sending. Typically, when we run Llama, we know our input, output tokens. The output tokens is the total number of tokens that are produced by the model, and we know how long it took to run. That’s what we use to compute the throughput. Again, for TTFT, what we do is, for any particular input token size, we set the output token equal to 1, and run it and estimate what the TTFT is going to be. Typically, we know that that is something that the user is actually simply waiting for.
Participant 1: How about CPU and other physical metrics, especially on cloud providers? Are you using hardware counters? How are you measuring swapping?
Hariharan: I have not run it on the cloud yet. Running it on bare metal, we have our regular tools to measure the utilization and also the counters and everything. We have our own software, and general-purpose software as well.
See more presentations with transcripts
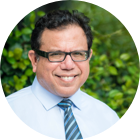
MMS • Vlad Khononov
Article originally posted on InfoQ. Visit InfoQ
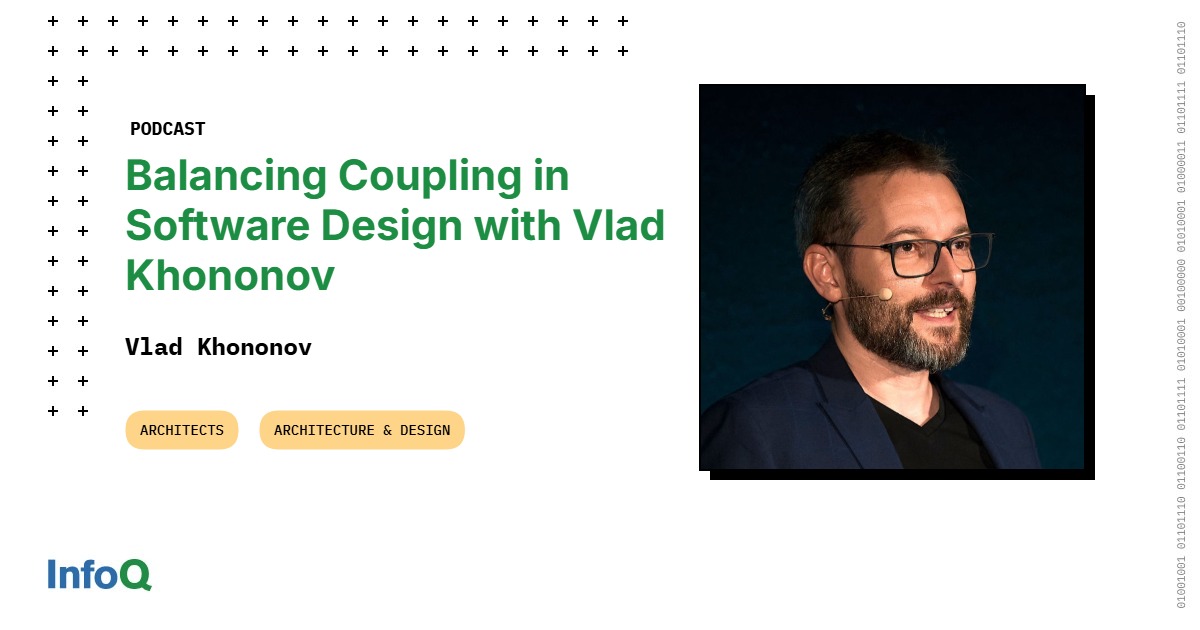
Transcript
Thomas Betts: Hello and welcome to another episode of the InfoQ Podcast. Today I’m joined by Vlad Khononov. Vlad is a software engineer with extensive industry experience working for companies large and small in roles ranging from webmaster to chief architect. His core areas of expertise include software architecture, distributed systems, and domain-driven design. He’s a consultant, trainer, speaker, and the author of Learning Domain-Driven Design. But today we’re going to be talking about the ideas in Vlad’s latest book, Balancing Coupling in Software Design. Vlad, welcome to the InfoQ Podcast.
Vlad Khononov: Hey Thomas. Thank you so much for having me.
Balance coupling is the goal, not no coupling [01:07]
Thomas Betts: So the title of your book, Balancing Coupling, and I think a lot of architects and engineers are familiar with the idea of wanting low coupling, we want to have our systems loosely coupled. But as your book points out, that’s really an oversimplification that we don’t want to have no coupling, we need to have a balanced coupling. So can you explain why that’s an oversimplified idea to say, we just want loose coupling everywhere?
Vlad Khononov: Yes. So by the way, a loose coupling is okay. What I’m really afraid of is people saying, let’s decouple things. Let’s have completely independent components in our system, which is problematic because if you ask yourself, what is a system? What makes a system? Then the answer is a system is a set of components working together to achieve some overarching goal. Now, in order to achieve that goal, it’s not enough to have those components, they have to work together. Those interactions is what makes the value of that whole system greater than the sum of its components, sum of its parts. And those interactions is what we usually call coupling. If you look that word up in a dictionary, coupled means connected.
So to make the system work, we need coupling. Now, of course, too much of a good thing is going to be bad. We need water, any living organism that we know of on this planet needs water to survive. However, if you’re going to drink too much water, well guess what’s going to happen? Nothing good is going to happen. Same with coupling. We cannot eliminate it because just as in the case of water, you’re not going to survive, a system is not going to survive. So we need to find that “just right” amount of coupling that will make the system alive. It will allow it to achieve that overarching goal.
Thomas Betts: I like the idea of if we add too much water, maybe that’s how we get to the big ball of mud, that everything is completely connected. And we can’t see where there should be good separations between those couples, you can’t see the modules that should be there that make the system understandable. And I know that’s part of it is, we want to get to small enough modules that we can understand and work with and evolve over time without having to handle the entire big ball of mud, if you will.
If the outcome can only be discovered by action and observation, it indicates a complex system [03:35]
Thomas Betts: So that coupling itself, that’s not the problem. The problem really is the complexity. And I think people sometimes correlate the two that if I have a highly coupled system that everything’s talking to each other that’s causing the complexity. Can you distinguish where coupling and complexity are not always the same thing, one isn’t always the bad?
Vlad Khononov: Yes. That’s a great point. And the thing is, when we are designing the system, we need to find that “just right” amount of coupling to make it work. And if you go overboard, as you said, we’ll end up with that monster that we usually call “big ball of mud”. And that pretty much describes what we are afraid of, complexity. I guess anyone with a few years of experience in software engineering has that experience of working on a big ball of mud project that maybe it works, but nobody has the courage to modify it because you don’t know what’s going to happen following that change. Whether it’s going to break now or it’s going to break a week later after it was deployed to production. And what is going to break? And that relationship between an action and its outcome is my preferred way of describing complexity.
If you’re working on a system and you want to do something, and you know exactly what’s going to happen, that’s not complexity. If you can ask someone, and some other external expert knows what’s going to happen, that’s not complexity either. However, if the only way to find out the outcome of the thing you want to do is to do it and then observe what happens, then you’re dealing with a system that is complex, and that means that the design of that system makes those interactions much harder than we as people can fathom. We have our cognitive limits, our cognitive abilities, if you look at studies, they’re not looking good by the way. And it means that the design of that system exceeds our cognitive abilities, it’s hard for us to understand what’s going on there. Of course, it has something to do with coupling. However, it’s not because of coupling, but because of misdesigned coupling.
Thomas Betts: Yes. And then I think your book talks about the idea of sharing too much knowledge, that coupling is where knowledge is being transferred. And so the idea of cognitive load being exceeded, the knowledge that I have to have in order to troubleshoot this bug is, I have to understand everything. Well, I can’t understand everything and remember it all, so I’m just going to try and recreate it. And in order for me to try and recreate it, I have to have the full integration stack, right? I have to have everything running, be able to debug all the way through. And the flip side of that is somebody wants to be able to have that experience because they’re used to having the big monolith, the big ball of mud. They’re like, “I don’t understand it, so I’m going to just see what happens”.
Once they’re working in microservices, then they get to, “Well, I can’t actually step through the code once I send the request to the other call, how do I know what happens?” How do you help get people into that mindset of you’re making it better, but it’s a different shift of the paradigm that you can’t just run everything, but the benefit is you don’t have to know about it once it goes past that boundary.
Three dimensions of coupling [07:23]
Vlad Khononov: Yes. And that’s the thing about coupling, we are way too used to oversimplify it. As, Hey, coupling is bad. Let’s eliminate all the coupling, that’s how we get modular software systems. However, if you look what happens when you connect any two components, when you couple any two components in a system. What happens beneath the surface? Then you’ll see that coupling is not that simple, it’s not uni-dimension. Actually, it manifests itself in three dimensions. As you mentioned, first of all, we have that knowledge sharing. You have two components working together. How are they going to work together? How are they going to communicate with each other? How are they going to understand each other? They need to exchange to share that knowledge.
Then we have the dimension of distance. If you have two objects in the same file, then the distance between the source code of the two objects is short. However, if those two objects belong to different microservices, then you have different code bases, different projects, different repositories, maybe even different teams. Suddenly the distance grows much bigger. Why is that important? Well, the longer the distance that is traveled by the knowledge, the sooner it’ll cause that cognitive overload. And we’ll say, “Hey, that’s complexity. We need to decouple things”. So distance is a very important factor when designing coupling.
And the third dimension is a dimension of time, of volatility because oh, why do we care? We want to be able to change the system. We wanted to change its components, their behavior. Maybe we will modify existing functionalities, maybe we’ll add new ones. For that, we want to make sure that the coupling is just right. However, if that is not going to happen, maybe because the component is a part of a legacy system, or maybe the business is not interested in investing any effort in that specific area, then the effect of coupling is going to be much lower. So we better prioritize our efforts on other parts with higher volatilities.
Distance and knowledge sharing are intertwined [09:49]
Thomas Betts: So I want to talk about that distance part first. I think that’s a new way of thinking of the problem because I think we can relate to, I’m going to separate this into microservices and that’ll solve my problem. And if you go back to the combination of how much knowledge is being shared, and how far away it is. Well, if I have all the code in my monolith, then the distance between the code is pretty low, right? I can change all the code all at once, but that also leads to a lot of complexity because I might not be able to easily see what code I need to change because there’s too much of it.
Now, if I take it into the microservices approach, I can say, I only need to change this. There’s only so much code to look at, I can understand it. But if I say, if I make a change here, I also need to make a change in this upstream or downstream service, that they have to know that I’m making a change. Then you’re saying that, that’s where the knowledge comes in, the knowledge being shared is tightly coupled. Is that a good explanation of what you’re trying to say?
Vlad Khononov: Yes, yes. That’s where complexity gets complex. Essentially, we have two types of complexities when working on any system. First, let’s say that you’re working on one of its components, and it is a small big ball of mud, let’s call it a small ball of mud. Then we could say that the local complexity of that component is high. We don’t understand how it works, and if you want to change something, we don’t know what’s going to happen. Now, there is another type of complexity and that’s global complexity, and this one is about the interactions on a higher level of abstraction. Say we have our component and other components of that system, and they’re integrated in a way that makes it hard to predict how changing one of the components is going to be, whether it’s going to require simultaneous changes in other components. So that’s global complexity.
The difference between the two as you mentioned is distance. And way back when the microservices hype just started, people wanted to decouple things by increasing the distance because previously we had all the knowledge concentrated in a monolith, let’s call it the old-school monolith. Everything in one physical boundary. Now, back then decoupling involved extracting functionalities into microservices, so we increased the distance. However, way too many projects focused just on that, on increasing the distance. They were not focused enough on, “Hey, what is that knowledge that is going to travel that increased distance?” And that’s how many companies ended up transforming their old-school monoliths into new shiny distributed monoliths. So they kind of traded local complexity into global complexity.
Coupling is only a problem if a component is volatile [13:04]
Thomas Betts: And that only becomes a problem when that third element, that third dimension of volatility rears its head. Because as long as those two things don’t change, the fact that they share knowledge over a long distance shouldn’t matter. But if one of those has to make a change and it has to affect the other one, now you’ve got the distributed ball of mud problem, that everything in two different services has to change. You actually made the problem worse by going to microservices. So that’s where all three factors have to be considered, correct?
Vlad Khononov: Yes, exactly. And that’s funny because all those companies that tried doing that, of course, they didn’t decompose their whole systems on the very first day of that microservice endeavor. No, they start with a small proof of concept, and that proof concept is successful. So they said, “Hey, let’s go on. Let’s proceed and apply the same decomposition logic everywhere else”. Now, the difference is that POC is usually down on something that is not business critical, its volatility is low. So you are kind of safe introducing complexity there. So the mistake was taking those less business critical components, extracting them, and thinking that they will achieve the same result with other components of the system. And of course, there once you step into that distributed big ball of mud area, well, suddenly microservices became evil and people started praising monoliths.
Thomas Betts: Right. We didn’t understand what we were doing, we didn’t understand why we were trying to accomplish it. We thought the problem was “everything’s too close, we’ll solve it by just moving it apart”. But if you don’t factor in, how is the knowledge changing? How is the volatility affected? Because yes, that first one might work, it doesn’t matter if they’re close together in one monolith or separate. If there’s no volatility, if things aren’t changing, it doesn’t matter where it lives.
But once you get to, this is something that we’re going to be making changes to really quickly. Because that was the other thing that people said, if we go to microservices, we can make changes really quickly, and then they maybe make even more changes faster, but they run into all these issues that separate teams in separate modules and separate microservices are trying to change things all at once, and then they lead back to, we have to still have all this communication, or we have this major integration step that just you weren’t ready for it because you did the thing wrong. When you make them move to microservices, you have to consider all three factors. What is changing? And if I know it’s going to change, what do I do differently then? Because obviously we still want to break those things up, but how do I say this is going to be a volatile module, it’s going to have core business, it’s going to be evolving. What’s the solution then? Because I want to be able to change it.
Distance affects where code lives as well as the lifecycle to maintain related components [16:22]
Vlad Khononov: Yes. That dimension of space distance is very tricky, and what makes it even trickier is that it has, let’s call it sub dimensions. So first we have that physical distance between source codes. The greater that distance gets, the harder it is going to be to modify the two components simultaneously. So that’s one thing. We have another force that works in the opposing direction, and that’s lifecycle coupling. The closer things are, the more related their life cycles. So they will be developed, tested, deployed together. If you have components implemented in the same physical boundary, for example.
As you go toward the other end, then you are reducing those lifecycle dependencies. And then we have social technical factors, those two components are implemented by the same team, or do we have to coordinate the change with multiple teams? And suddenly the distance can grow even larger, and the lifecycle coupling will be reduced even further. So distance is super important, but as you mentioned, what makes it all, let’s call it painful, is that knowledge that is going to travel that distance.
Thomas Betts: Right. So if I know that this thing is going to be changing, in some ways those changes affect the knowledge that is being shared, right? If I’m adding new features and functionality, that means there’s more knowledge in this module. And if I have to communicate those changes, that’s the challenge. So is the trade-off of, I’m going to have more volatility in this module, I have to reduce the knowledge that’s being shared, reduce that integration strength of how tightly those two things are coupled. Is that a matter of defining good API batteries, for example?
Vlad Khononov: Yes. So we have to manage that knowledge that we are sharing across the boundaries, we have to make it explicit. Now, the thing about knowledge is, as you said, the more knowledge we’re sharing, the more cascading changes will follow because the more knowledge we share, the harder chances that the piece of that shared knowledge will change, and then we’ll have to communicate that change to the other component, to the coupled component.
Four levels for measuring coupling [19:10]
Vlad Khononov: Now, how do we evaluate knowledge? What units should be used to measure knowledge? That’s a tricky question. It’s tricky, and I’m not sure we have an answer for that. However, what we do have is a methodology from the ’70s called structure design. And in it there was a model for measuring or for evaluating interdependencies between components of a system called module coupling. That model had six levels, they were focused around the needs of systems that were written in those days. But essentially these levels describe different types of knowledge that can be exchanged across boundaries of components.
In my model, in the balanced coupling model, I adapted module coupling and changed its name to integration strength. I had to change its name because the levels of the model are completely different because again, they have to be accessible to people working on modern systems. I reduced the levels to four basic types of knowledge to make it easier to remember them. And if you need finer-grained details, then you can use a different model from a different era called connascence to measure the degrees of those types of knowledge.
Intrusive coupling [20:47]
Vlad Khononov: So the basic four types of knowledge are from highest to lowest. First of all is intrusive coupling. Say you have a component with a public interface that should be used for integration, however, you say, “Okay, that’s fine. I have a better way. I will go to your database directly, pick whatever I need, maybe modify it”. In other words, intrusive coupling is all about using private interfaces for integration.
Once you introduce that dependency on private interfaces, you basically have a dependency on implementation details. So any change can potentially break the integration. So with intrusive coupling, you have to assume that all knowledge is shared.
Thomas Betts: Right. That’s the classic, if you have a microservice, you own your own database. And no one else is allowed to go there, they have to go through this boundary. And I like that you’re calling back to, these are papers written 50 years ago. And no one was talking about microservices there, no one was talking about having several databases, but it’s still the same idea; if I can structure this so that in order for this to go through, it has to go through this module. That’s why C++ evolved to have object-oriented design to say, “I have this class and it has behavior, and here’s public and private data”. And that’s what you’re talking about, if you can just get all the way through, there’s no point in having that public versus private interface.
Vlad Khononov: Yes. Yes. It’s funny, if you look at one of the books from that period, one that I particularly like is called Composite/Structure Design by Glenford Myers. And if you ignore the publishing date, it sounds like he is talking about the problems we’re facing today. It’s crazy. It’s crazy.
Thomas Betts: What’s the next level after that intrusive coupling?
Functional coupling [22:45]
Vlad Khononov: Yes. So after intrusive coupling, we have functional coupling. And here we’re sharing the knowledge of functional requirements. We’re shifting from how the component is implemented, to what that component implements, what is that business functionality? Again, that’s quite a high amount of knowledge that is shared by this type because if you share that kind of knowledge, then probably any change in the business requirements is going to affect both of the coupled components, so they will change together.
Model coupling [23:22]
Vlad Khononov: Next, we have model coupling, which means we have two components that are using the same model of the business domain. Now, DDD people will get it right away. But the idea is when we are developing a software system, we cannot encode all the knowledge about its business domain, it’s not possible. If you are building a medical system, you’re not going to become a doctor, right? Instead, what we are doing is we’re building a model of that business domain that focuses only on the areas that are relevant for that actual system. Now, once you have two components based on the same model, then if you have an insight into that business domain and you want to improve your model, then guess what? Both of them will have to change simultaneously. So that’s model coupling.
Contract coupling [24:17]
And the lowest level is contract coupling. Here we have an integration contract, you can think about it as a model of a model that encapsulates all other types of knowledge. It doesn’t let any knowledge of the implementation model outside of the boundary, that means you can evolve it without affecting the integration contract. You’re not letting any knowledge of functional requirements across the boundaries, and of course, you want to protect your implementation details.
Examples of the four types of coupling [24:51]
Thomas Betts: Right. So just to echo that back. If you’re talking about, you said DDD people will get this right away. If I have a new invoice coming in that I want to pay, maybe I have an expense management system where somebody says, “Here’s a new thing to pay, I’m going to submit it to the expense management system”, and it has to go through an approval process to say, yes, it’s approved. Then all the way at the end we have our accounts payable person who’s going to log in and say, “Oh, I need to go pay this invoice, I have to pay the vendor”, right? There’s an invoice that flows all the way through the system, but if you say, “I need to know how is it going to get paid at the end, all the accounting details upfront”, it’s tightly coupled.
If you think about it from who’s doing the work, you might have the invoice request that starts in expense management, and then the paid invoice. And those ideas of, I have one model, but the words sound the same, but ubiquitous language says in this domain, that’s what this means. And I work on accounting systems, so the invoice, whether you’re in accounts payable or accounts receivable, we both have invoices, but they’re exactly the opposite. Am I going to pay someone or is someone going to pay me? And so ubiquitous language helps us reduce the cognitive load because I know in this space, I’m only talking about this part of the workflow because it’s satisfying this person, this role, they’re doing their job.
And so that’s going to the levels of coupling you’re talking about. The contract coupling says, I’m going to hand off from here, to the next, to the next, and I don’t have to know what’s going to happen a week from now with this because once it exceeds my boundary, I’m done with it. And the intrusive coupling is, they’re all editing the same database record and everybody knows about all the details. And somewhere above that is, I have to know that there’s this next workflow of pay the invoice versus submit the invoice, and everybody knows about those things. Is that a good example of how to see those different layers in there?
Vlad Khononov: Yes, absolutely. Absolutely. There are so many creative ways to introduce intrusive coupling. There are such interesting death-defying stunts we can pull. For example, maybe you’re introducing, not a dependency, but you rely on some undocumented behavior, that’s intrusive coupling. Maybe you’re working in, let’s say an object-oriented code base and a component that you are interacting with returns you an array or a list of objects, and then you can go ahead and modify it. And because it’s reference type, it’s going to affect the internals of that component. So that’s another creative example of intrusive coupling. By the way, a reader of the book sent it to me. And I was like, “Oh, why haven’t I thought about it when I was writing the book? It’s such a great example”.
Modularity is the opposite of complexity [28:01]
Thomas Betts: Yes. Well, I think what you’re describing is, that’s the difference between the local and the global complexity, right? We think about these as microservices, I’m going to separate big modules out. But the same problems occur within our code base because even if you’re working in a monolith, you can structure… This is where the book talked about modular monoliths. You can set up your code, so even if it’s stored in one repository, you can make it easier to understand. And that gets to, this class doesn’t have to know about the 900 other classes that are in the project, I only know about the 10 that are close to me.
Vlad Khononov: Yes. Exactly. And by the way, it brings us back to the topic of complexity, or rather the opposite of complexity. So if complexity is, if we’re going to define it as the relationship between an action and its outcome, then modularity is the opposite. It’s a very strong relationship between an action and its outcome. So if we want to design a modular system, we want to be able to know what we have to change, that’s one thing. And the second thing is, once we make the change, what’s going to happen? That I would say is the idea of modularity.
Modular monoliths can reduce complexity [29:19]
Vlad Khononov: Now, how can we do it? How can we achieve what you described? Let’s say that you have a monolith that can be a big ball of mud, but it also can be a modular monolith. If the thing is, the core ideas are the same. You can increase the distance, you don’t have to step across its physical boundary. You can introduce distance in the form of modules within that monolith. You can put related things together because let’s say you have one boundary with lots of unrelated things. And how can we define unrelated things? Things that are not sharing knowledge between them.
So if they’re located close to each other, then it will increase the cognitive load to find what we have to change there, right? So we can reduce the cognitive load by grouping related things, those components that have to share a knowledge in logical groups, logical modules. And that’s how we can achieve modular monoliths, which is by the way, in my opinion, the first step towards decomposing a system into microservices because it’s way easier to fix a mistake once you are in the same physical boundary.
Thomas Betts: Right. You’re keeping the distance a little bit closer, you’re separating it logically into separate namespaces, different directory structures, but you’re not making a network call, right?
Vlad Khononov: Exactly.
Thomas Betts: That’s definitely increasing the distance. You’re not necessarily handing over to another team. You might be, but maybe it is still the same team just saying, “Hey, I want to be able to think about this problem right now, and I don’t want to have to think about these other problems”, and so let me just split the code. But that causes you as an architect designing this to say, “What makes sense? What do I move around? Where am I having the problem understanding it because there’s too much going on, there’s too much local complexity? And let’s look for that and figure out how do I increase the distance a little bit so that the knowledge that’s being shared stays within the things that are close”. And you start looking for, have I introduced distance while not reducing the knowledge, right? That’s what you’re trying to do, is have the knowledge transfer go down that integration strength when you’re adding distance, right?
If shared knowledge is appropriately high, then balance it with distance [31:45]
Vlad Khononov: Yes. Yes, absolutely. We always want to reduce integration strength; we want to always minimize the knowledge. But if you’re familiar with the business domain, you kind of know that, hey, here, I need to use the same model of the business domain, here we have closely related business functionalities. So it doesn’t matter if you want to reduce it to the minimum, you can’t. You have to remain on that level of, let’s say for example, functional coupling. Once you observe that level of knowledge being shared, then you have to take it into consideration, and balance it with another dimension, which is distance. Don’t spread those things apart because otherwise that’s going to be cognitive load, and as a result, complexity.
Thomas Betts: Right. And again, this is where the volatility comes into place. So if I’m focused on, let’s go from our big ball of mud to having a more organized modular monolith. Then I can look at, oh, where are we seeing lots of changes? Where’s the business evolving a lot and where is it not? And so I can now focus on, if we’re going to pull one service out, because let’s say we actually have scaling needs, we need to make sure that this part of the system can grow up to 10 times the size, but the rest of it, we don’t need to scale up as big. Those types of things you can look at, well, what’s volatile? And then if you pull it out of that monolith, you say, “I’m adding the distance, have I reduced the knowledge to a safer coupling level?” I haven’t kept that high integration strength, that you still know about my private methods and how to call my database even though I pulled you out because you haven’t actually done anything to solve the volatility problem, right?
Evaluating volatility requires understanding the business domain [33:35]
Vlad Khononov: And volatility, initially it sounds like something simple, the simplest dimension of the three. Oh my god, it’s not. It’s tricky because to truly predict the rate of changes in a component, it’s not enough to look at maybe your experience, or at the source code because there are things we can differentiate between, essential volatility and accidental volatility or accidental in-volatility. Accidental volatility can be because of, or design of the system, things are changing just because that’s the way the system is designed. And accidental in-volatility can happen. Let’s say that you have an area of the system that the business wants to optimize, but it is designed in such a way that people are afraid to touch it. And the business is afraid to touch it, to modify it as well as a result. So to truly, truly evaluate volatility, you have to understand the business domain. You have to analyze the business strategy, what differentiates that system from its competitors. Again, DDD people are thinking about core subdomains right now.
Thomas Betts: Yes.
Vlad Khononov: And once you identify those areas based on their strategic value to the company, then you can really start thinking about the volatility levels desired by the business.
Thomas Betts: You mentioned things happen internal and external, so the business might have, we want to pursue this new business venture, or this was an MVP, and the MVP has taken off, we want to make sure it’s a product we can sell to more people, but we need to make changes to it. So there are business drivers that can change the code, but there’s also internal things. Like I just need to make sure my code is on the latest version of whatever so that it’s not sitting there getting obsolete, and hasn’t gotten security patches or whatever. So some of those, the system’s just going to evolve over time because you need to keep, even the legacy code, you need to keep up to date to some standards. And then there’s the, no, we want to make big changes because the business is asking us to, right? So the architect has to factor in all of those things, as well as I think you mentioned the socio-technical aspects, right? Who is going to do the work? All of this comes into play, it’s not always just one simple solution. You can’t just go to loose coupling, right?
Balancing the three dimensions of coupling [36:13]
Vlad Khononov: Yes. It’s complicated. I’m not going to say that it’s complex, but it’s complicated. But the good news is that once you truly understand the dynamics of system design, it doesn’t really matter what level of abstraction you’re working on. The underlying rules are going to be the same, whether it’s methods within an object or microservices in a distributed system, the underlying ideas are the same. If you have a large amount of knowledge being shared, balance it by minimizing the distance. If you’re not sharing much knowledge, you can increase the distance. So it’s one of the two, either knowledge is high and the distance is low, or vice distance is high but knowledge is low. Or, or things are not going to change, which is volatility is low, which can balance those two altogether.
Thomas Betts: Right. So if you just looked at strength and distance, how much knowledge is being shared over too long? That looks bad. But if it’s never going to change, you don’t care. If it does change, then it’s not balanced. On the flip side, if it’s going to change a lot, then you need to think about the relationship between the integration strength and the distance. So if there’s not much knowledge being shared over a long distance, that’s okay, or if there’s a lot of knowledge shared over a small distance, that’s okay. So you can have one but not both, if things are changing. But if things aren’t changing, you don’t care.
Vlad Khononov: Yes. And of course, things are not changing today, maybe something is going to change on the business side tomorrow. And as an architect you have to be aware of that change and its implications on the design. The classical example here is, I am integrating a legacy system, nobody is going to change it, and I can just go ahead and grab whatever I need from its database, that’s fine. Another classic example is, again, DDD influence, some functionality that is not business critical, but you have to implement it, which is usually in DDD lexicon is called a supporting subdomain. Usually they’re going to be much less water than core subdomains. However, business strategy might change, and suddenly that core subdomain will evolve into a core one. Suddenly there is that big strategy change that should be reflected in the design of the system. So it’s three dimensions working together, and whether it will end up with modularity or complexity depends on how you’re balancing those forces.
Thomas Betts: Right. And I think you got to the last point I wanted to get to is, we can design this for today based on what we know, but six months, six years from now, those things might shift not because of things we can predict right now. And if you try and design for that future state, you’re always going to make some mistakes, but you want to set yourself up for success. So do the small things first. Like if it is reorganize your code so it’s a little easier to understand, that seems like a benefit, but don’t jump to, I have to have all microservices.
And I liked how you talked about how this can be applied at the system level, or the component level, or the code level. I think you described this as the fractal approach of, no matter how much you keep looking at it, the same problem exists at all these different layers of the system. So that coupling and balance is something you have to look at, at different parts of your system either inside a microservice at the entire system level, and what are you trying to solve for at different times, right?
Vlad Khononov: Yes. And that’s by the way, why I’m saying that if you pick up a book from the ’70s, like that book I mentioned, Composite/Structured Design, it looks way too familiar. The problems that they’re facing, the problems they’re describing, the solutions they’re applying are also going to be quite familiar once you step over those terms that are used there because those terms are based on languages like FORTRAN and COBOL. Yes, you need some time, some cognitive effort to understand what they mean. But yes, the underlying ideas are the same, it’s just a different level of abstraction that was popular back then. Not popular, that’s all they had back then.
Wrapping up [40:57]
Thomas Betts: So if you’ll want to follow up with you or want to learn more about your balanced coupling model, any recommendations of where they can go next?
Vlad Khononov: Yes. So on social media aspect, I am the most active on LinkedIn at the moment. I have accounts on other social networks like Bluesky, Twitter, et cetera. Right now LinkedIn is my preferred network. At the moment I’m working on a website called Coupling.dev, so if you’re listening to this, I hope that it is already live and you can go there and learn some stuff about coupling.
Thomas Betts: Well, Vlad Khononov, I want to thank you again for being on the InfoQ Podcast.
Vlad Khononov: Thank you so much, Thomas. It’s an honor and a pleasure being here.
Thomas Betts: And listeners, we hope you’ll join us again soon for a future episode.
Mentioned:
.
From this page you also have access to our recorded show notes. They all have clickable links that will take you directly to that part of the audio.
Announcing QCon AI: Focusing on Practical, Scalable AI Implementation for Engineering Teams
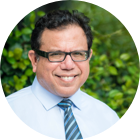
MMS • Artenisa Chatziou
Article originally posted on InfoQ. Visit InfoQ
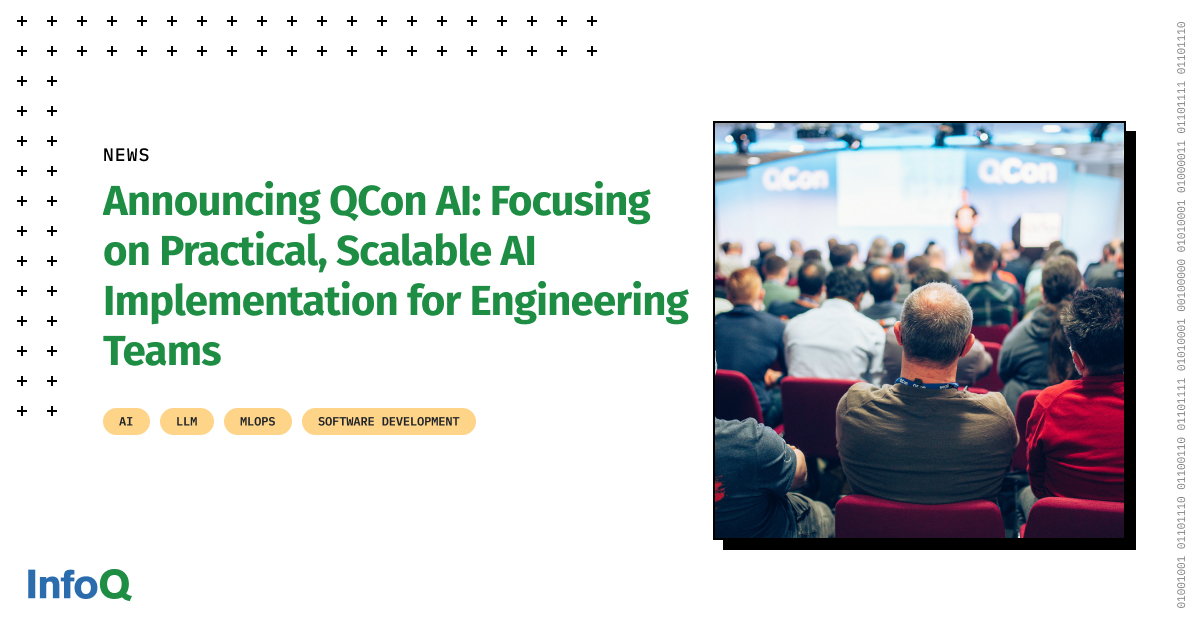
QCon conferences have been a trusted forum for senior software practitioners to share real-world knowledge and navigate technology shifts for nearly two decades. Today, as artificial intelligence adoption transitions from experimental phases to running within critical enterprise systems, InfoQ and QCon are introducing QCon AI, a new conference dedicated to the practical challenges of building, deploying, and scaling AI reliably. The inaugural event will take place in New York City on December 16-17, 2025.
This conference is designed specifically for senior software developers, architects, and engineering leaders – the practitioners tasked with making AI work securely, reliably, and effectively within complex enterprise environments. Recognizing that the landscape is filled with hype, QCon AI focuses squarely on what’s working now. The program, curated by senior engineers actively running AI systems at scale, prioritizes actionable patterns and blueprints over theoretical possibilities.
QCon AI builds directly on the QCon legacy of facilitating peer-to-peer learning without hidden product pitches. As QCon AI 2025 Conference Chair, Wes Reisz, Technical Principal @ Equal Experts, ex-VMWare, ex-ThoughtWorks,16-time QCon Chair, and InfoQ Podcast Co-host emphasizes.
“Forget the AI hype. QCon AI is focused on helping you build and scale AI reliably. The teams speaking at QCon AI share how they are delivering and scaling AI – warts and all”.
Attendees can expect deep dives into the practicalities of integrating AI into the software development lifecycle, architecting resilient and observable production AI systems, managing MLOps, optimizing costs, ensuring responsible governance, and proving business value. We believe it’s crucial to share hard-won lessons – including failures – from those who have navigated these challenges.
A key theme for QCon AI is the collaborative nature of successful AI implementation. Scaling AI isn’t a solo task; it requires alignment across multiple departments, including development, MLOps, platform, infrastructure, and data teams. QCon AI is structured to benefit teams attending together. Shared learning experiences can accelerate the adoption of effective patterns, foster better cross-functional understanding, reduce integration risks, and help align team members, from Staff+ engineers and architects to ML engineers and engineering leaders, on strategy and execution.
By bringing together experienced practitioners to share what truly works in enterprise AI, QCon AI aims to equip engineering teams with the confidence and knowledge needed to move from prototype to production successfully.
More information about QCon AI, including program details as they become available, can be found on the conference website.
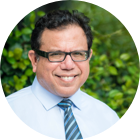
MMS • RSS
Posted on mongodb google news. Visit mongodb google news
LPL Financial LLC lowered its position in MongoDB, Inc. (NASDAQ:MDB – Free Report) by 7.0% in the fourth quarter, according to the company in its most recent disclosure with the SEC. The firm owned 36,670 shares of the company’s stock after selling 2,771 shares during the period. LPL Financial LLC’s holdings in MongoDB were worth $8,537,000 at the end of the most recent quarter.
A number of other institutional investors and hedge funds have also made changes to their positions in the business. Hilltop National Bank grew its stake in shares of MongoDB by 47.2% in the fourth quarter. Hilltop National Bank now owns 131 shares of the company’s stock valued at $30,000 after buying an additional 42 shares in the last quarter. NCP Inc. acquired a new position in MongoDB during the fourth quarter worth approximately $35,000. Continuum Advisory LLC increased its stake in MongoDB by 621.1% in the third quarter. Continuum Advisory LLC now owns 137 shares of the company’s stock valued at $40,000 after purchasing an additional 118 shares during the period. Versant Capital Management Inc boosted its stake in shares of MongoDB by 1,100.0% during the fourth quarter. Versant Capital Management Inc now owns 180 shares of the company’s stock worth $42,000 after buying an additional 165 shares during the period. Finally, Wilmington Savings Fund Society FSB acquired a new position in MongoDB in the 3rd quarter valued at about $44,000. 89.29% of the stock is currently owned by hedge funds and other institutional investors.
Wall Street Analysts Forecast Growth
MDB has been the subject of several research analyst reports. Citigroup cut their price objective on MongoDB from $430.00 to $330.00 and set a “buy” rating for the company in a research report on Tuesday, April 1st. Macquarie decreased their price target on shares of MongoDB from $300.00 to $215.00 and set a “neutral” rating on the stock in a report on Friday, March 7th. Rosenblatt Securities restated a “buy” rating and issued a $350.00 price target on shares of MongoDB in a report on Tuesday, March 4th. Royal Bank of Canada dropped their price objective on MongoDB from $400.00 to $320.00 and set an “outperform” rating on the stock in a report on Thursday, March 6th. Finally, Morgan Stanley dropped their target price on shares of MongoDB from $350.00 to $315.00 and set an “overweight” rating on the stock in a report on Thursday, March 6th. Seven analysts have rated the stock with a hold rating, twenty-four have given a buy rating and one has issued a strong buy rating to the stock. According to MarketBeat.com, the company currently has an average rating of “Moderate Buy” and a consensus target price of $312.84.
Check Out Our Latest Research Report on MongoDB
MongoDB Stock Performance
MongoDB stock traded down $7.01 during midday trading on Monday, reaching $147.38. 5,169,359 shares of the company’s stock were exchanged, compared to its average volume of 1,771,877. The firm has a market cap of $11.97 billion, a price-to-earnings ratio of -53.79 and a beta of 1.49. MongoDB, Inc. has a 52 week low of $140.96 and a 52 week high of $387.19. The stock’s 50-day simple moving average is $236.68 and its 200 day simple moving average is $261.78.
MongoDB (NASDAQ:MDB – Get Free Report) last released its quarterly earnings results on Wednesday, March 5th. The company reported $0.19 earnings per share for the quarter, missing the consensus estimate of $0.64 by ($0.45). The firm had revenue of $548.40 million for the quarter, compared to analyst estimates of $519.65 million. MongoDB had a negative return on equity of 12.22% and a negative net margin of 10.46%. During the same period in the previous year, the business posted $0.86 earnings per share. Research analysts forecast that MongoDB, Inc. will post -1.78 earnings per share for the current year.
Insiders Place Their Bets
In related news, CAO Thomas Bull sold 301 shares of the firm’s stock in a transaction on Wednesday, April 2nd. The stock was sold at an average price of $173.25, for a total transaction of $52,148.25. Following the completion of the transaction, the chief accounting officer now directly owns 14,598 shares of the company’s stock, valued at $2,529,103.50. This trade represents a 2.02 % decrease in their position. The sale was disclosed in a document filed with the Securities & Exchange Commission, which is available at this hyperlink. Also, CFO Srdjan Tanjga sold 525 shares of the stock in a transaction on Wednesday, April 2nd. The stock was sold at an average price of $173.26, for a total transaction of $90,961.50. Following the sale, the chief financial officer now owns 6,406 shares of the company’s stock, valued at approximately $1,109,903.56. This trade represents a 7.57 % decrease in their ownership of the stock. The disclosure for this sale can be found here. Insiders have sold 58,060 shares of company stock worth $13,461,875 over the last ninety days. Corporate insiders own 3.60% of the company’s stock.
About MongoDB
MongoDB, Inc, together with its subsidiaries, provides general purpose database platform worldwide. The company provides MongoDB Atlas, a hosted multi-cloud database-as-a-service solution; MongoDB Enterprise Advanced, a commercial database server for enterprise customers to run in the cloud, on-premises, or in a hybrid environment; and Community Server, a free-to-download version of its database, which includes the functionality that developers need to get started with MongoDB.
See Also
Before you consider MongoDB, you’ll want to hear this.
MarketBeat keeps track of Wall Street’s top-rated and best performing research analysts and the stocks they recommend to their clients on a daily basis. MarketBeat has identified the five stocks that top analysts are quietly whispering to their clients to buy now before the broader market catches on… and MongoDB wasn’t on the list.
While MongoDB currently has a Moderate Buy rating among analysts, top-rated analysts believe these five stocks are better buys.
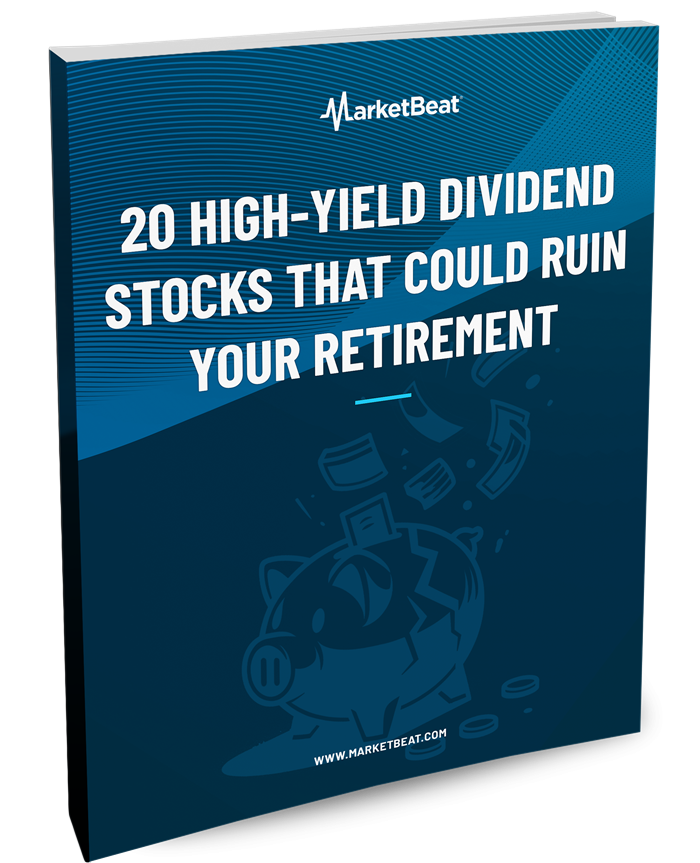
Almost everyone loves strong dividend-paying stocks, but high yields can signal danger. Discover 20 high-yield dividend stocks paying an unsustainably large percentage of their earnings. Enter your email to get this report and avoid a high-yield dividend trap.
Article originally posted on mongodb google news. Visit mongodb google news
Java News Roundup: Jakarta EE 11 Web Profile, GlassFish, TornadoVM, Micronaut, JHipster, Applet API
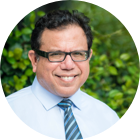
MMS • Michael Redlich
Article originally posted on InfoQ. Visit InfoQ
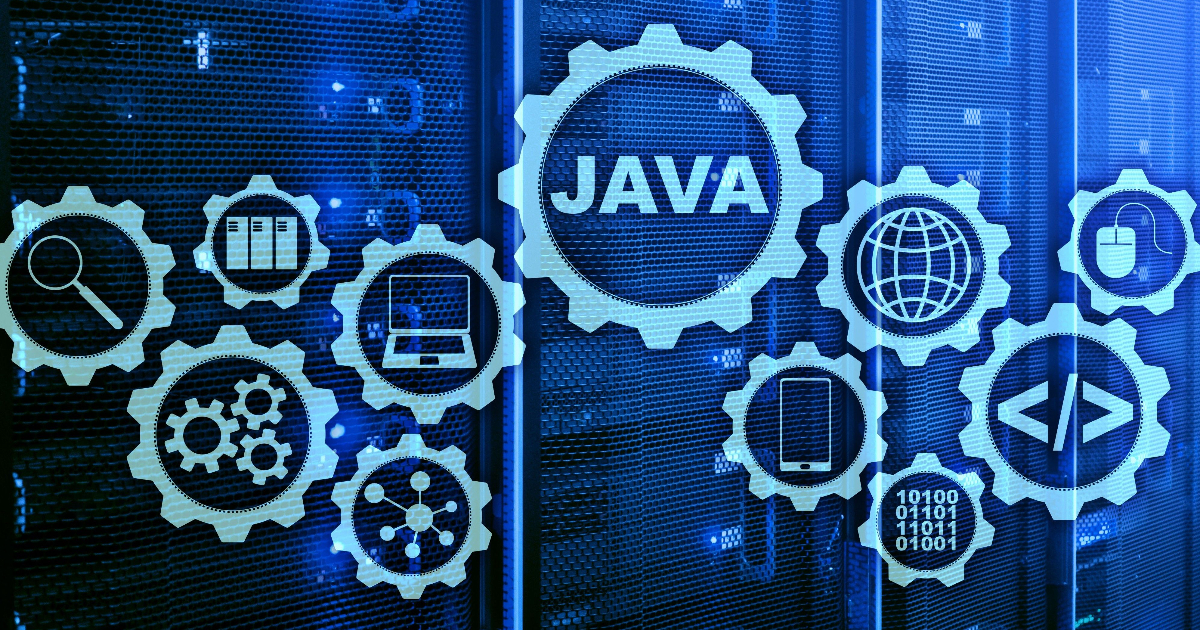
This week’s Java roundup for March 31st, 2025 features news highlighting: the formal release of the Jakarta EE 11 Web Profile; the eleventh milestone release of GlassFish 8.0.0; point releases TornadoVM 1.1.0, Micronaut 4.8.0 and JHipster 8.10.0; and a new JEP candidate to remove the Applet API.
OpenJDK
JEP 504, Remove the Applet API, was elevated from its JEP Draft 8345525 to Candidate status. This JEP proposes to remove the Applet API, deprecated in JDK 17, due it’s continued obsolescence since applets are no longer supported in web browsers.
JDK 25
Build 17 of the JDK 25 early-access builds was made available this past week featuring updates from Build 16 that include fixes for various issues. More details on this release may be found in the release notes.
For JDK 25, developers are encouraged to report bugs via the Java Bug Database.
GlassFish
The eleventh milestone release of GlassFish 8.0.0 delivers bug fixes, dependency upgrades and improved specification compatibility for various new features of Jakarta EE 11. This relese passes the final Jakarta EE 11 Web Profile TCK. Further details on this release may be found in the release notes.
Jakarta EE 11
In his weekly Hashtag Jakarta EE blog, Ivar Grimstad, Jakarta EE Developer Advocate at the Eclipse Foundation, provided an update on Jakarta EE 11, writing:
Jakarta EE 11 Web Profile is released! It’s a little later than planned, but we’re finally there, and Jakarta EE 11 Web Profile joins Jakarta EE 11 Core Profile among the released specifications. It has been a tremendous effort to refactor the TCK.
Eclipse GlassFish was used as the ratifying compatible implementation of Jakarta EE 11 Web Profile. I would expect other implementations, such as Open Liberty, WildFly, Payara, and more to follow suit over the next weeks and months. Check out the expanding list of compatible products of Jakarta EE 11.
The road to Jakarta EE 11 included four milestone releases, the release of the Core Profile in December 2024, the release of Web Profile in April 2025, and a fifth milestone and first release candidate of the Platform before its anticipated release in 2Q 2025.
TornadoVM
The release of TornadoVM 1.1.0 provides bug fixes and improvements such as: support for mixed precision FP16
to FP32
computations for matrix operations; and a new method, mapOnDeviceMemoryRegion()
, defined in the TornadoExecutionPlan
class that introduces a new Mapping On Device Memory Regions feature that offers device buffer mapping for different buffers. More details on this release may be found in the release notes.
Micronaut
The Micronaut Foundation has released version 4.8.0 of the Micronaut Framework featuring Micronaut Core 4.8.9 that include: improvements to the Micronaut SourceGen module that now powers bytecode generation of internal metadata and expressions; and the ability to activate dependency injection tracing so that developers can better understand what Micronaut is doing at startup and when a particular bean is created. There were also updates to many of Micronuat’s modules. Further details on this release may be found in the release notes.
Quarkus
Quarkus 3.21.1, the first maintenance release, ships with bug fixes, dependency upgrades and improvements such as: allow execution model annotations (@Blocking, @NonBlocking, etc.) on methods annotated with SmallRye GraphQL @Resolver
due to the resolver throwing an error; and a resolution to a Java UnsupportedOperationException
when using the TlsConfigUtils
class to configure TLS options in a Quarkus project using the Application-Layer Protocol Negotiation (ALPN) extension. More details on this release may be found in the release notes.
JHipster
The release of JHipster 8.10.0 provides notable changes such as: a workaround to a ClassCastException
using Spring Boot and Hazelcast upon logging in to a JHipster application; numerous dependency upgrades, most notably Spring 3.4.4; and many internal improvements to the code base. Further details on this release may be found in the release notes.
The release of JHipster Lite 1.31.0 ships with a dependency upgrades to Vite 6.2.4 that resolves two CVEs affecting previous versions of Vite 6.2.4 and 6.2.3, namely: CVE-2025-31125, a vulnerability, resolved in version 6.2.4, in which Vite exposes content of non-allowed files using URL expressions ?inline&import
or ?raw?import
, to the development server; and CVE-2025-30208, a vulnerability, resolved in version 6.2.3, where the restrictions imposed by the Vite /@fs/
filesystem variable can be bypassed by adding expressions, ?raw??
or ?import&raw??
, to the URL and returns file content if it exists. More details on this release may be found in the release notes.
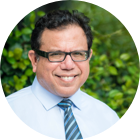
MMS • RSS
Posted on mongodb google news. Visit mongodb google news
MongoDB, Inc. (NASDAQ:MDB – Get Free Report) insider Cedric Pech sold 1,690 shares of MongoDB stock in a transaction dated Wednesday, April 2nd. The shares were sold at an average price of $173.26, for a total transaction of $292,809.40. Following the transaction, the insider now directly owns 57,634 shares of the company’s stock, valued at approximately $9,985,666.84. This trade represents a 2.85 % decrease in their ownership of the stock. The sale was disclosed in a document filed with the Securities & Exchange Commission, which is available through this hyperlink.
MongoDB Stock Down 4.5 %
NASDAQ:MDB traded down $7.01 during trading hours on Monday, hitting $147.38. 5,169,359 shares of the stock traded hands, compared to its average volume of 1,771,869. The stock has a 50 day moving average price of $236.68 and a 200 day moving average price of $261.78. The company has a market cap of $11.97 billion, a price-to-earnings ratio of -53.79 and a beta of 1.49. MongoDB, Inc. has a 1 year low of $140.96 and a 1 year high of $387.19.
MongoDB (NASDAQ:MDB – Get Free Report) last issued its earnings results on Wednesday, March 5th. The company reported $0.19 earnings per share for the quarter, missing the consensus estimate of $0.64 by ($0.45). The firm had revenue of $548.40 million during the quarter, compared to the consensus estimate of $519.65 million. MongoDB had a negative return on equity of 12.22% and a negative net margin of 10.46%. During the same period in the prior year, the company posted $0.86 EPS. Equities analysts forecast that MongoDB, Inc. will post -1.78 earnings per share for the current fiscal year.
Institutional Trading of MongoDB
Institutional investors and hedge funds have recently added to or reduced their stakes in the business. Vanguard Group Inc. grew its holdings in MongoDB by 0.3% during the 4th quarter. Vanguard Group Inc. now owns 7,328,745 shares of the company’s stock valued at $1,706,205,000 after buying an additional 23,942 shares in the last quarter. Franklin Resources Inc. boosted its position in shares of MongoDB by 9.7% during the fourth quarter. Franklin Resources Inc. now owns 2,054,888 shares of the company’s stock worth $478,398,000 after acquiring an additional 181,962 shares during the last quarter. Geode Capital Management LLC grew its stake in MongoDB by 1.8% during the fourth quarter. Geode Capital Management LLC now owns 1,252,142 shares of the company’s stock valued at $290,987,000 after acquiring an additional 22,106 shares in the last quarter. First Trust Advisors LP increased its holdings in MongoDB by 12.6% in the 4th quarter. First Trust Advisors LP now owns 854,906 shares of the company’s stock valued at $199,031,000 after acquiring an additional 95,893 shares during the last quarter. Finally, Norges Bank purchased a new stake in MongoDB in the 4th quarter worth $189,584,000. Institutional investors own 89.29% of the company’s stock.
Wall Street Analyst Weigh In
A number of research analysts have issued reports on MDB shares. KeyCorp cut MongoDB from a “strong-buy” rating to a “hold” rating in a research note on Wednesday, March 5th. Mizuho lifted their price target on MongoDB from $275.00 to $320.00 and gave the stock a “neutral” rating in a research report on Tuesday, December 10th. Loop Capital cut their price objective on shares of MongoDB from $400.00 to $350.00 and set a “buy” rating on the stock in a research report on Monday, March 3rd. Stifel Nicolaus decreased their target price on shares of MongoDB from $425.00 to $340.00 and set a “buy” rating for the company in a report on Thursday, March 6th. Finally, Tigress Financial raised their price target on shares of MongoDB from $400.00 to $430.00 and gave the company a “buy” rating in a research report on Wednesday, December 18th. Seven equities research analysts have rated the stock with a hold rating, twenty-four have issued a buy rating and one has issued a strong buy rating to the stock. Based on data from MarketBeat.com, MongoDB presently has an average rating of “Moderate Buy” and an average price target of $312.84.
Check Out Our Latest Analysis on MongoDB
About MongoDB
MongoDB, Inc, together with its subsidiaries, provides general purpose database platform worldwide. The company provides MongoDB Atlas, a hosted multi-cloud database-as-a-service solution; MongoDB Enterprise Advanced, a commercial database server for enterprise customers to run in the cloud, on-premises, or in a hybrid environment; and Community Server, a free-to-download version of its database, which includes the functionality that developers need to get started with MongoDB.
See Also
Before you consider MongoDB, you’ll want to hear this.
MarketBeat keeps track of Wall Street’s top-rated and best performing research analysts and the stocks they recommend to their clients on a daily basis. MarketBeat has identified the five stocks that top analysts are quietly whispering to their clients to buy now before the broader market catches on… and MongoDB wasn’t on the list.
While MongoDB currently has a Moderate Buy rating among analysts, top-rated analysts believe these five stocks are better buys.
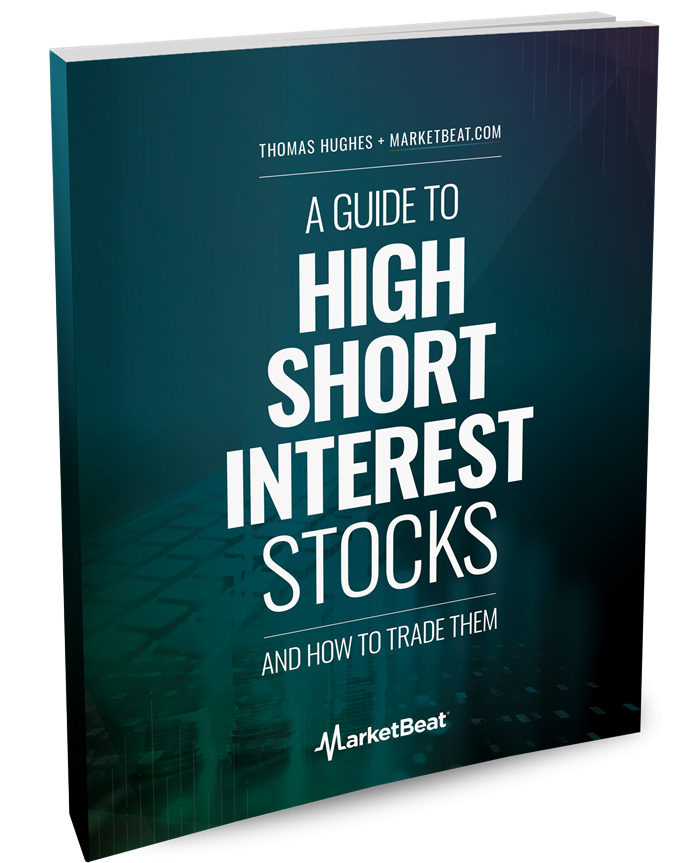
MarketBeat’s analysts have just released their top five short plays for April 2025. Learn which stocks have the most short interest and how to trade them. Enter your email address to see which companies made the list.
Article originally posted on mongodb google news. Visit mongodb google news
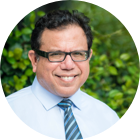
MMS • RSS
Posted on mongodb google news. Visit mongodb google news
MongoDB, Inc. (NASDAQ:MDB – Get Free Report) CAO Thomas Bull sold 301 shares of the firm’s stock in a transaction that occurred on Wednesday, April 2nd. The stock was sold at an average price of $173.25, for a total transaction of $52,148.25. Following the completion of the transaction, the chief accounting officer now owns 14,598 shares in the company, valued at $2,529,103.50. This represents a 2.02 % decrease in their position. The sale was disclosed in a legal filing with the SEC, which is accessible through this hyperlink.
MongoDB Stock Down 4.5 %
MongoDB stock traded down $7.01 during trading hours on Monday, hitting $147.38. The stock had a trading volume of 5,169,359 shares, compared to its average volume of 1,771,869. The stock’s 50-day moving average price is $236.68 and its 200-day moving average price is $261.78. MongoDB, Inc. has a 12-month low of $140.96 and a 12-month high of $387.19. The stock has a market capitalization of $11.97 billion, a P/E ratio of -53.79 and a beta of 1.49.
MongoDB (NASDAQ:MDB – Get Free Report) last announced its quarterly earnings data on Wednesday, March 5th. The company reported $0.19 earnings per share for the quarter, missing analysts’ consensus estimates of $0.64 by ($0.45). The firm had revenue of $548.40 million during the quarter, compared to analysts’ expectations of $519.65 million. MongoDB had a negative return on equity of 12.22% and a negative net margin of 10.46%. During the same quarter in the previous year, the firm earned $0.86 earnings per share. On average, research analysts expect that MongoDB, Inc. will post -1.78 EPS for the current fiscal year.
Institutional Investors Weigh In On MongoDB
Institutional investors have recently modified their holdings of the business. Strategic Investment Solutions Inc. IL purchased a new position in MongoDB in the 4th quarter worth about $29,000. Hilltop National Bank grew its stake in shares of MongoDB by 47.2% in the fourth quarter. Hilltop National Bank now owns 131 shares of the company’s stock worth $30,000 after acquiring an additional 42 shares during the period. NCP Inc. purchased a new position in shares of MongoDB during the fourth quarter valued at approximately $35,000. Continuum Advisory LLC lifted its position in MongoDB by 621.1% during the third quarter. Continuum Advisory LLC now owns 137 shares of the company’s stock valued at $40,000 after purchasing an additional 118 shares during the period. Finally, Versant Capital Management Inc boosted its holdings in MongoDB by 1,100.0% in the fourth quarter. Versant Capital Management Inc now owns 180 shares of the company’s stock worth $42,000 after purchasing an additional 165 shares during the last quarter. 89.29% of the stock is owned by hedge funds and other institutional investors.
Analyst Ratings Changes
MDB has been the topic of a number of recent analyst reports. Macquarie cut their price target on shares of MongoDB from $300.00 to $215.00 and set a “neutral” rating on the stock in a report on Friday, March 7th. Loop Capital cut their target price on MongoDB from $400.00 to $350.00 and set a “buy” rating on the stock in a research note on Monday, March 3rd. China Renaissance initiated coverage on MongoDB in a report on Tuesday, January 21st. They set a “buy” rating and a $351.00 price target on the stock. Royal Bank of Canada cut their price objective on MongoDB from $400.00 to $320.00 and set an “outperform” rating on the stock in a research report on Thursday, March 6th. Finally, Rosenblatt Securities reissued a “buy” rating and set a $350.00 target price on shares of MongoDB in a research note on Tuesday, March 4th. Seven research analysts have rated the stock with a hold rating, twenty-four have given a buy rating and one has issued a strong buy rating to the company. According to data from MarketBeat.com, MongoDB presently has an average rating of “Moderate Buy” and a consensus target price of $312.84.
Get Our Latest Analysis on MongoDB
MongoDB Company Profile
MongoDB, Inc, together with its subsidiaries, provides general purpose database platform worldwide. The company provides MongoDB Atlas, a hosted multi-cloud database-as-a-service solution; MongoDB Enterprise Advanced, a commercial database server for enterprise customers to run in the cloud, on-premises, or in a hybrid environment; and Community Server, a free-to-download version of its database, which includes the functionality that developers need to get started with MongoDB.
Further Reading
Before you consider MongoDB, you’ll want to hear this.
MarketBeat keeps track of Wall Street’s top-rated and best performing research analysts and the stocks they recommend to their clients on a daily basis. MarketBeat has identified the five stocks that top analysts are quietly whispering to their clients to buy now before the broader market catches on… and MongoDB wasn’t on the list.
While MongoDB currently has a Moderate Buy rating among analysts, top-rated analysts believe these five stocks are better buys.
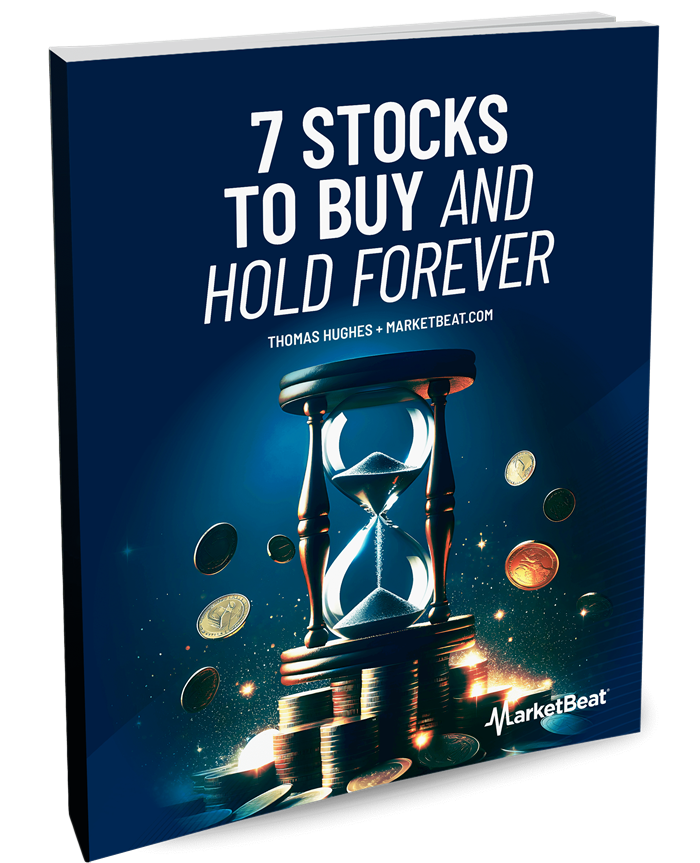
Enter your email address and we’ll send you MarketBeat’s list of seven stocks and why their long-term outlooks are very promising.
Article originally posted on mongodb google news. Visit mongodb google news
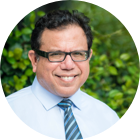
MMS • RSS
Posted on mongodb google news. Visit mongodb google news
MongoDB, Inc. (NASDAQ:MDB – Get Free Report) CFO Srdjan Tanjga sold 525 shares of the company’s stock in a transaction dated Wednesday, April 2nd. The shares were sold at an average price of $173.26, for a total value of $90,961.50. Following the completion of the sale, the chief financial officer now owns 6,406 shares of the company’s stock, valued at $1,109,903.56. This represents a 7.57 % decrease in their position. The transaction was disclosed in a legal filing with the SEC, which can be accessed through this hyperlink.
MongoDB Stock Down 4.5 %
Shares of NASDAQ MDB traded down $7.01 during mid-day trading on Monday, reaching $147.38. 5,169,359 shares of the company were exchanged, compared to its average volume of 1,771,869. The stock has a market capitalization of $11.97 billion, a P/E ratio of -53.79 and a beta of 1.49. The company’s 50-day simple moving average is $236.68 and its 200-day simple moving average is $261.78. MongoDB, Inc. has a twelve month low of $140.96 and a twelve month high of $387.19.
MongoDB (NASDAQ:MDB – Get Free Report) last issued its quarterly earnings data on Wednesday, March 5th. The company reported $0.19 earnings per share (EPS) for the quarter, missing analysts’ consensus estimates of $0.64 by ($0.45). The firm had revenue of $548.40 million for the quarter, compared to the consensus estimate of $519.65 million. MongoDB had a negative return on equity of 12.22% and a negative net margin of 10.46%. During the same quarter in the prior year, the firm earned $0.86 EPS. As a group, equities research analysts expect that MongoDB, Inc. will post -1.78 EPS for the current year.
Institutional Inflows and Outflows
Large investors have recently added to or reduced their stakes in the company. OneDigital Investment Advisors LLC boosted its position in shares of MongoDB by 3.9% in the fourth quarter. OneDigital Investment Advisors LLC now owns 1,044 shares of the company’s stock valued at $243,000 after acquiring an additional 39 shares during the period. Hilltop National Bank raised its stake in MongoDB by 47.2% in the 4th quarter. Hilltop National Bank now owns 131 shares of the company’s stock worth $30,000 after purchasing an additional 42 shares in the last quarter. Avestar Capital LLC boosted its holdings in MongoDB by 2.0% in the 4th quarter. Avestar Capital LLC now owns 2,165 shares of the company’s stock valued at $504,000 after purchasing an additional 42 shares during the period. Aigen Investment Management LP grew its position in shares of MongoDB by 1.4% during the 4th quarter. Aigen Investment Management LP now owns 3,921 shares of the company’s stock worth $913,000 after purchasing an additional 55 shares in the last quarter. Finally, Perigon Wealth Management LLC increased its holdings in shares of MongoDB by 2.7% during the fourth quarter. Perigon Wealth Management LLC now owns 2,528 shares of the company’s stock worth $627,000 after purchasing an additional 66 shares during the period. Institutional investors own 89.29% of the company’s stock.
Analysts Set New Price Targets
Several research analysts recently weighed in on the stock. Tigress Financial lifted their price objective on shares of MongoDB from $400.00 to $430.00 and gave the stock a “buy” rating in a research note on Wednesday, December 18th. Stifel Nicolaus decreased their price target on shares of MongoDB from $425.00 to $340.00 and set a “buy” rating for the company in a report on Thursday, March 6th. Daiwa America upgraded shares of MongoDB to a “strong-buy” rating in a research report on Tuesday, April 1st. DA Davidson lifted their target price on MongoDB from $340.00 to $405.00 and gave the stock a “buy” rating in a report on Tuesday, December 10th. Finally, Needham & Company LLC lowered their price target on MongoDB from $415.00 to $270.00 and set a “buy” rating for the company in a report on Thursday, March 6th. Seven analysts have rated the stock with a hold rating, twenty-four have given a buy rating and one has given a strong buy rating to the company. Based on data from MarketBeat, the stock currently has an average rating of “Moderate Buy” and an average price target of $312.84.
Read Our Latest Stock Analysis on MDB
About MongoDB
MongoDB, Inc, together with its subsidiaries, provides general purpose database platform worldwide. The company provides MongoDB Atlas, a hosted multi-cloud database-as-a-service solution; MongoDB Enterprise Advanced, a commercial database server for enterprise customers to run in the cloud, on-premises, or in a hybrid environment; and Community Server, a free-to-download version of its database, which includes the functionality that developers need to get started with MongoDB.
Further Reading
Before you consider MongoDB, you’ll want to hear this.
MarketBeat keeps track of Wall Street’s top-rated and best performing research analysts and the stocks they recommend to their clients on a daily basis. MarketBeat has identified the five stocks that top analysts are quietly whispering to their clients to buy now before the broader market catches on… and MongoDB wasn’t on the list.
While MongoDB currently has a Moderate Buy rating among analysts, top-rated analysts believe these five stocks are better buys.
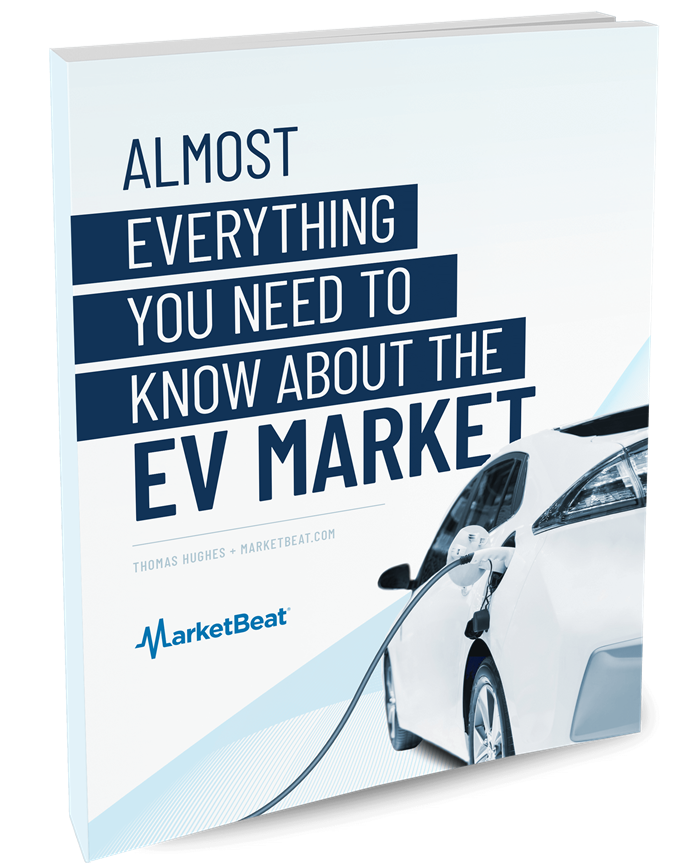
Looking to profit from the electric vehicle mega-trend? Enter your email address and we’ll send you our list of which EV stocks show the most long-term potential.
Article originally posted on mongodb google news. Visit mongodb google news