Month: April 2025
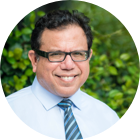
MMS • Janani Narayanan Karthik Ramasamy
Article originally posted on InfoQ. Visit InfoQ
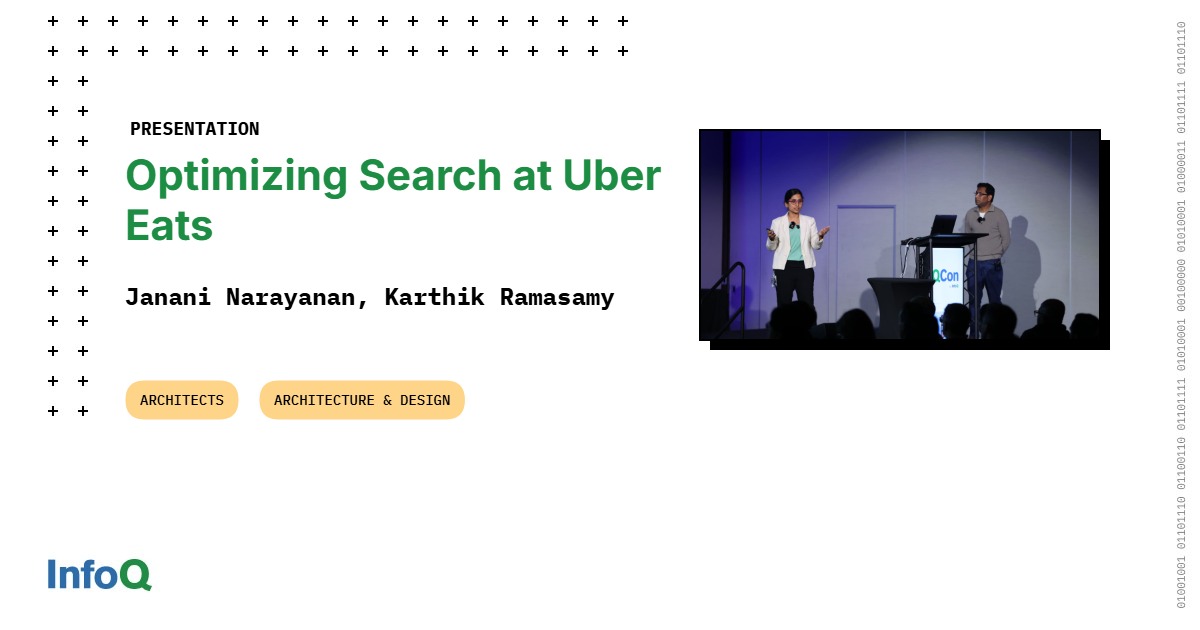
Transcript
Narayanan: Myself and my colleague Karthik here, we are going to be walking you through, as part of this session, on how we solve for scaling the Uber Eats backend and infra architecture, so that we can infinitely scale the number of merchants that are deliverable to any particular eater. I’m Janani Narayanan. I’m a senior staff engineer working on search ranking recommendations for the past few years.
Ramasamy: I’m Karthik Ramasamy. I’m a senior staff engineer. I work in the search platform team. Our team builds the search solutions that powers various search use cases at Uber, including Uber Eats, location search at rides, and other things.
Narayanan: A fun Easter egg here. If you have your Uber Eats app open, you could go there into the search bar and then look up who built this, and you can see the list of all of the engineers who are part of the team.
nX Selection
Which of the following is not among the top 10 searches in Uber Eats? It is unexpected for me when I first saw this. It was actually Mexican. Apparently, not many people care about eating healthy when they order from outside.
The problem that we want to talk about as part of this is, how did we go about expanding selection by nX? Before we go into what does nX mean, I want to spend a little bit of time talking about what selection means. It means different things for different people. If I were to talk to the merchant onboarding team, the operations, they would say that onboarding as many merchants as possible, that is considered as a success metric. That is considered as good selection. If I were to speak to eaters, different kind of eaters will have a different answer to this. Someone could say that I care about getting my food within a particular ETA, so that is good selection. My favorite restaurant, if it is on this platform, then this platform has good selection.
Some other folks can also say that if I get to find new stores, discover more stores, which I wouldn’t have normally found it, or instead of going out of the app and then finding somewhere else, or word of mouth recommendation and then coming back to the app, if the app in itself can curate my preferences based on my order history, based on my search history, you know everything about me, so why don’t you give me something that I haven’t tried before, surprise me. That is considered as good selection. What we are here to talk about is, given all of the real-world aspect of Uber Eats out of the picture, what is a technical challenge that we could solve where restaurants or stores are getting onboarded onto this platform, and the eaters want to have access to more of selection, more of restaurants available to them when they are trying to look it up in any of the discovery surfaces.
To get a sense of how the business is growing, how the market is growing, as we can see, just before the pandemic and through the course of the pandemic, the business has expanded and branched down to multiple different line of businesses. Why this is important is because that is all part of the scale that is inclusive of what we were trying to solve. It is not just about restaurants. Starting from pandemic, we have grocery stores, then retail stores, people who are trying to deliver packages, all of these things are part of the same infra, same ranking and recommendation tech stack, which powers it under the hood. Why this matters is that, up until now, we are talking about it in terms of restaurants and stores, but the index and complexity comes in where in case of a restaurant, if we talk about a single document, it would probably have 20, 30 items, and that’s about it.
If we think about grocery as a line of business, for every single store, there are going to be 100,000 SKUs for each and every store. All of those items also need to be indexed. Onboarding a single grocery store is very different in terms of scale, comparison with onboarding a restaurant. Another example is, before we started working on this, people were able to order from restaurants which are 10 to 15 minutes away from them. Now, you could order from a restaurant which is sitting from San Francisco, you could order it all the way to Berkeley.
Let’s say if you want to order something from Home Depot, and the item that you’re looking for is not here but it is somewhere in Sacramento, you should be able to order it from Uber Eats and then get it delivered to you. That is the breadth of the line of businesses that we wanted to unlock, and also the different challenges in terms of scale that different line of business offers for us. With that in place, in terms of selection, we are specifically focusing on the quantity of selection that is exposed to the eater when they are going to any of these discovery surfaces. The personalization aspect of it, that’s a completely different topic altogether.
Discovery Surfaces (Home Feed, Search, Suggestions, Ads)
What we mean by discovery surfaces, let’s start with terminologies. There are four different discovery surfaces. Which of the surfaces do you guys think most of the orders come from? Home feed. Depending on whether it is e-commerce or online streaming services, the surface area is going to change. For specifically delivery business, it is the home feed. In terms of the tech stack that we are looking at, there are different entry points to this, different use cases that we serve as part of the work that we did. If we take search, for example, there are different kinds of search, restaurant name search, dish search, cuisine search. Home feed has multiple different compartments to this. There is the carousels, which are all thematic based on the user’s order history.
Then we have storefronts, which is a list of stores based on the location. At the top of your home feed, if you look at your Uber Eats app, there would be shortcuts, which will be either cuisine based or promotion based and whatnot. All of these entry points need to work in cohesion. In other words, regardless of whether someone goes through suggestions, where someone is searching for pasta and you are trying to also show pastrami based on the lookahead search. We are looking at McD as a search, and we also want to show Burger King as part of the suggestions that come up. All of these different use cases need to be addressed as part of this. Because if I’m able to find a store or a restaurant through my home feed, but I’m not able to locate it through my suggestions at the same time, that is considered as a poor customer experience. We needed to address all parts of the tech stack in one go, in one XP.
Overall Architecture – Infra Side to Application Layer
Given this, let’s take a look at the overall architecture from the infra side to the application layer. The leftmost thing, that is all the infra layer of it. It is the corpus of all of our stores and indexes, how we index, how do we ingest, all of that goes into that. Then we have the retrieval layer, that is where the application layer and my team and my org starts, where the retrieval layer focuses on optimizing for recall. The more stores that we could fetch, the more stores that we could send it to the next set of rankers so they can figure out what is an appropriate restaurant to show at that time.
The first pass ranker is looking for precision and efficiency. What this means is that as the restaurants or stores are fetched, we want to start looking at, how do we do a lexical match so the user’s query and the document are matched as much as possible in terms of relevance? Then we have the hydration layer, where a lot of the business logic comes into picture in terms of, does it have a promotion, does it have membership benefits involved? Is there any other BOGO, buy one, get one order that we could present and whatnot? ETD information, store images, all of those things come into picture there. Then we have the second pass ranker, which optimizes for the precision. This is where a lot of the business metrics get addressed. We look at conversion rate. We also look at, given the previous order history, all of the other things that I know from different surfaces of interaction from this eater, how do I build the personalization aspect of it so we will be able to give more relevant results to the eater.
Given this overall tech stack, I want to give a sense of scale in terms of the tech stack. Particularly, I would like to draw your attention to two of the line items here. One is the number of stores which are part of the corpus, millions of them.
The other one is the number of matched documents that you use to fetch out of the retrieval layer. When you look at it, the scale sounds like, there’s just only thousands of documents which are matched. What matched means is when I look at the eater’s location, how many stores can deliver to that eater’s location? When we had these tens of thousands of them when we started, we said, if you wanted to make it nX or increase it more, all that we needed to do is fetch more. Let’s just increase our early termination count. Let’s fetch more candidates and then go from there. We did a very basic approach of fetching 200 more stores, two-week XP, and it blew up on our face where we saw that the latency increased, P50 latency increased by 4X. In this example, we could see that the red dot is where the eater is, that is the eater’s location, and as it expands, which is the new red dots that we started adding, that is where the latency started increasing. This is a serious problem. Then we needed to look into where exactly the latency is coming from.
The root cause, as we started diving into it, had multiple aspects to it where we needed to look at different parts of the tech stack to make sure that some design decisions made in, let’s say, ingestion, how does it impact the query layer? How do some of the mechanisms that we have in the query layer, don’t really gel well with our sharding strategy? It was a whole new can of worms that we opened as we started looking into it. First, we started with benchmarking. If there’s a latency increase, especially the retrieval layer, let’s just figure out where exactly it is coming from.
In the search infra layer, we added a bunch of different metrics. Depending on whether we are looking at grocery or eats, there is one step particularly which stood out where we were trying to match a particular document to the query, and then, this document match, put it into your bucket, then move on to the next document. When we iterate over to the next document that matches, that took anywhere between 2.5 milliseconds latency for grocery and 0.5 milliseconds for eats, and that is unexplainable for us. That was unexplainable at that time. It is supposed to take nanoseconds, especially if you have optimized index. Then we started looking into, this is a problem area that we needed to start looking into.
The other area that I want to talk about is how we are ingesting the data, and the pieces will fall in place in the next few slides. For those of you who are following Uber’s engineering blogs, you would now be familiar that Uber does most of its geo-representation using H3. H3 library is what we use to figure out how we tessellate the world and how we make sense out of the different levels that we have in place. Depending on the resolution, the different levels optimize for different behaviors that we want for the eaters and the merchants.
Given this, we represent any merchant and the delivery using the hexagons to say that merchant A can deliver to A, B, C locations by using hexagons in the resolutions. How this gets indexed is if we take this example where we have restaurants A, B, and C, and hexagons are delivery areas which are numbered, the index will be a reverse lookup, where, going by the hexagons, we would say that in this hexagon, two or three different restaurants can deliver to me. Fairly straightforward so far.
From here, what we did is, now that we understand how the index layout looks, this is the second problem that we identified as part of selection expansion. At the time of ingestion, we had this concept of close by and far away, and that is the column that we use to ingest the data. At a store level, the upstream service had the decision to say, I’m going to give you a list of stores and the deliverable hexagons, and I’m going to categorize them as close by and far away. When we did that, if we look at this example, hexagon 7 considers both A and B as far away. If we look at the real data, B is actually much close by, in comparison with A, but the ingestion doesn’t have that information.
Naturally, the query stage also doesn’t have this information. Only the upstream service had this information, which we lost as part of this. This ETD information, without that, we are treating A and B together at the time of rankers, and that was another problem. In terms of search area, even though we say that we’ve only increased by 200 restaurants, going from, let’s say, 5 kilometers to 10 kilometers to so-and-so, would mean that we are increasing the area by square. The search space increases exponentially, even though we say that I’m only trying to deliver from 10 miles to 12 miles or 15 miles, and whatnot. This meant that we are processing a lot number of candidates which will tie in into why going from one document to the other was taking such a long time.
The next thing is the store distribution. If we were to make it as a simple concentric circle around where the eater’s location is and the Lat-Long is, what we could see is, as we start expanding further into more geos, the number of stores or the distribution of stores in the innermost circle versus the outer circle and whatnot is going to be anywhere between 1:9 ratio. We will get more of faraway stores than the close-by stores, and ranking them becomes harder.
Another thing to note is, if we are going to find a restaurant which has much higher conversion rate because that restaurant is more popular and whatnot, but that is in the second circle or the third-most circle, then it is highly likely that in the second pass ranker, that store will get a higher precedence because it has higher conversion rate. In reality, people would want to see more of their close-by stores because a burger is a burger at some point in time. That was one of the problems that we saw as we started fetching more stores where good stores were trumping the close-by stores, and the ranking also needed to account for that.
Search Platform
Ramasamy: Next we’ll go share some insights about the search platform that powers the Uber Eats search. Then we will talk about some optimizations that we did to improve the retrieval limit and also the latency. How much traffic does Uber Eats get per day? It’s tens of millions of requests per day. Uber has an in-house search platform that is built on Apache Lucene. We use a typical Lambda architecture for ingestion. We have batch ingestion through Spark, and then we have real-time ingestion through the streaming path.
One of the notable features that we support in the real-time ingestion is the priority-aware ingestion. The callers can prioritize requests and the system will give precedence to the higher-priority request to ensure a high degree of data freshness for the high-priority request. At Uber, we use geosharding quite heavily. This is because most of our use cases are geospatial in nature. I’ll share some insights on some of the geosharding techniques that we use at Uber. Then, finally, we build custom index layouts and query operators that are tuned for Uber Eats cases that take advantage of the offline document ranking and early termination to speed up the queries.
Here’s the architecture overview of the search platform. There are three key components here. The first one is the batch indexing pipeline. The second component is the streaming or real-time updates path. The third component is the serving stack. We start with the batch ingestion. Usually, these are Spark jobs that takes the data from the source of truth, convert them into search documents, partition them into shards, and then builds Lucene index in Spark. The output of the Spark jobs are Lucene indexes, which then get stored into the object store.
Then updates are then constantly consumed to the streaming path. There is an ingestion service that consumes the updates from the upstream, again converts them into search documents, and then finds the shard the document maps to and then writes to the respective shard. One thing to note here is that we use Kafka as the write-ahead log, which provides several benefits. One of them that we talked earlier is implementing priority-aware ingestion. Because we use Kafka as the write-ahead log, it enables us to implement such features. It also provides us to implement replication and other things using Kafka.
The searcher node, when it comes up, it takes the index from the remote store, and then it also catches up the updates from the streaming path to the write-ahead log, and then it exposes query operators to run the search queries. There is another component here, it’s called the aggregator service. It is actually a stateless service. Its main responsibility is to take the request from upstream, find the shard the request maps to, then send it to the respective searcher node, and execute the queries. Also, aggregate the results and send it back to the caller if there are query fanouts and things like that. That’s the high-level overview of the search platform that powers Uber Eats search.
Sharding Techniques
Next, I will talk about sharding techniques that we use. As we have been talking earlier, that most of our queries are geospatial in nature. We are looking for find me restaurants for given coordinates, or find me grocery stores for given coordinates. We use geosharding to make these queries more efficient. The main advantage of geosharding is that we can locate all the data for a given location in a single shard, so that the queries are executed in a single shard.
At scale, this is quite important, because if you fan out the request to multiple shards, then there is an overhead of overfetching and aggregating the results, which can be avoided by using geosharding. The other benefit is first pass ranking can be executed on the data nodes. The reason being that the data node has the full view of the results for a given query, and then you can push the first pass ranker down to the data node to make it efficient. The two geosharding techniques that we use are latitude sharding and hex sharding. I’ll talk about both of them in detail.
Latitude sharding works this way, where you imagine the world as a slice of latitude bands, and each band maps to a shard. The latitude ranges are computed offline. We use Spark job to compute it. The way we compute is a two-step process. First is we divide the map into several narrow stripes. You can imagine this in order of thousands of stripes. Then we group the adjacent sites to get roughly equal-sized shards. In the first step, we also get the count of documents that maps to each narrow stripe.
Then we group the adjacent stripes such that you get roughly equal-sized shards, the N being the number of shards here. There’s a special thing to note here, like how we handle the documents that falls on the boundary of the shards, that is the green zone that is in this picture. Those are documents that falls on the boundary of two shards. What we do is we index those shards in both of the neighboring shards. That way, the queries can go to a single shard and get all the documents relevant for the given query. The boundary or the buffer degree is calculated based on the search radius. We know that the queries are at the max going to go for a 50-mile or 100-mile radius.
Then we find the latitude degree that maps to that radius, and then that’s the buffer zone. Any document that falls in the buffer zone are indexed in both the shards. With latitude sharding, we get this benefit of cities from different time zones getting co-located in the same shard. In this example, you can see Europe cities and cities from America mixed in the same shard. Why is this important? This is because the traffic in Uber especially follows the sun pattern, where the activities are higher during the day and it slows down during the night. This sharding naturally avoids clustering cities with same busy hours in the same shard.
That helps us a lot in managing the capacity and stuff. We also see some problems or challenges with the latitude sharding. One of them is the bands are too narrow at the center. That’s because the cities are denser in this space, and then you reach a point in some use cases where it’s difficult to divide further, especially considering you have a buffer zone. Over time, the shards become uneven, and some shards, especially towards the center, are larger when compared to the rest of the shards. This creates problems, like your index builds take longer time because you’re bound by the larger shard. Also, those shards experience larger latencies and stuff.
The optimization for this problem is the hex sharding. Hex sharding, we imagine the world as tiles of hexagons. As Janani said, at Uber we use H3 library very extensively. H3 library provides different resolutions of hexagons. The lowest resolution, which means larger hexagons, results in about 100 tiles for the whole world. The highest resolution results in trillions of tiles. Selecting the right resolution is key for using hex sharding. We use some observations and empirical data to decide the hex sizes. At Uber, we generally use for hex sharding, hex size 2 or 3.
Again, we use the same approach of offline jobs to compute the shard boundaries. Basically, we pick a resolution, we compute the number of docs that maps to each resolution, and then group them into N shards, basically N equal shards using bin-packing. We also handle the buffer zones similar to latitude sharding. In hex sharding, you have to imagine the buffer zones also in terms of hexagons. The key here is, choose a resolution that is smaller than the main resolution hex for the buffer zones. Then you index the documents that falls in buffer zone in both the hexes. In this case, the right-side shard shows that the main blue area is the main hexagon and outside are the buffer zone hexagons that gets indexed into it as well to avoid crash out queries. That’s the details on sharding and the architecture of the search platform.
Solution
Next, we will talk about some specific optimizations we did for the Uber Eats use case, taking advantage of the query patterns and other data from the use case to improve the recall and also reduce the latency. The first thing that we did is building a data layout that can take advantage of the query patterns. I will share a couple of data layouts that we used, one for the Eats use case, another for the grocery use case. I’ll also walk through how those layouts helped us to improve the latency. The second technique we’ll talk about is how we use the ETD information that Janani was talking about earlier, how we index that into the search index. Then, how we divide the search space into non-overlapping ranges and then execute them in parallel to improve the latency. Then, finally, we’ll talk about how moving some of the computations that were happening in the query time, such as far away versus nearby computation that Janani was talking earlier, and how that helped to improve the recall and the latency.
Data Layout
I will go through the data layout. This is a data layout that we use for the Eats index. If you look at the Eats query pattern to begin with, you are basically looking for restaurants or items within the restaurants for a given store. We use this insight to also organize the documents in the index.
In this case, we take the city and we co-locate all the restaurants for a given city first. You can see, McDonald’s and Subway, they’re all the restaurants under the city, SF. We then order the items or the menus under those restaurants in the same order. You go with this order, city followed by all the restaurants in that city, and then items for each of the restaurants in the same order as the store. The benefit we get is the faster iteration. A query comes from SF, you can skip over all the documents of other cities that may be in the shard and just move the pointer right to the SF and then find out all the stores. That makes the query faster. The other nice benefit that we get is that if your data is denormalized, in our case, sometimes we denormalize all the store fields into the items as well.
In that case, you have a lot of common attributes for the docs. The item docs for the store will have all similar store level attributes adjacent to each other. This provides better compression ratio. That’s because Lucene uses delta encoding and if you have very sequential doc IDs, then your compression is better. Then, finally, we also order the documents by static rank, which helps us to early terminate the queries once we reach the budget.
Next, I will share a slightly modified version of the index layout that we use for grocery. That’s because of the nature of the grocery data. It’s pretty similar. Again, we first sort by city, then we take the stores, sort by stores, stores are ranked by the offline conversion rate order. Here, the difference is we place the items of the store next to each other. I will share why that is important. This is how the layout looks, city, then store, and the items of the store go to the second store, items of the store, and third store, items of the store.
One benefit, let’s say if you look for a specific posting list with the title as chicken, then you get the store 1, all the items with the title chicken for that store, and store 2, all the items with the title chicken for that store, and store 3. As Janani was saying earlier, grocery scale is very high compared to each. You have hundreds or thousands of items in a single store that can match the given title. When you’re executing a query, you don’t want to be looking for all the items from the same store. You can give a budget for each store, and then once you reach that limit, then you can skip over to the next store. This layout allows us to skip over the stores pretty quickly, but also collecting enough items from a given store. The other benefit that we get, from the business point of view, is it scales us to get diverse results. Your results are not coming from a single store, you also cover all the stores in the search space. That’s the main advantage of this layout.
Next, here’s some interesting numbers that we observed when we moved from one unsorted or unclustered layout to the clustered layouts from location and store. Here’s the latency of a single query that is executed before and after clustering. This query returns about 4K docs. As you can see, the retrieval time before clustering is around 145 milliseconds, and the retrieval time after clustering is 60 milliseconds. It’s about 60% better after clustering the docs based on the query pattern. Down below, the graph shows the doc IDs, time taken to get each hit in the retrieval loop.
As you can see, before sorting, the hits can take anywhere from 10 to 60 microseconds for a single doc. The latency here is in microseconds. After sorting, as you can see, the latency is a lot better, like each hit takes less than 5 microseconds. Here’s the overall improvement in latency that we observed when we rolled out this clustered index layout. You can see more than 50% improvement in P95. We also see equal improvement on P50 latencies as well. The other benefit is index size reduced by 20%.
ETA Indexing
Narayanan: One of the aspects that we talked about as part of ingestion is the metadata that we get from the upstream services was not passed on to the rest of the stack to be able to do meaningful optimizations on top of it. What this means is that if we take restaurant 1 and 2 as part of this example, as we index that restaurant 1 can deliver to hexagon 1, 2, 3, 4, we do not know relative to H1 how far away is H2, how far away is H3, and whatnot. This is an important metadata that we needed to pass it to the rankers so the rankers can penalize the faraway stores in conjunction with the conversion rate that they have available. This information needed to be passed on from the upstream team altogether. We started off with this. Now that we have one more dimension that we needed to index data, we were benchmarking a couple of different approaches of how we could have both the hexagon and the ETD indexed and used in the retrieval layer.
What we finally ended up doing is that for each and every range, after discussions with product and science team and whatnot, we aligned on what ranges make sense in terms of our query pattern and we said, let’s break them down into a few ranges that overall reflects how people are querying its ecosystem. We dissected it by multiple of these time ranges, 0 to 10 minutes, 10 to 20 minutes, 20 to 30 and whatnot. After we dissected it, we also said, from this eater’s location, let’s say hexagon 1, what are the restaurants which are available in range 1, range 2, range 3, and so on. We did that for every single hexagon available. For those of you who are following along and then thinking about, I smell something here, so how about there are other different ways of doing things?
For example, in this case, there is a tradeoff that we make in terms of, where do we want the complexity to be? Should it be in the storage layer or should it be in the CPU? In this case specifically, if we take a particular restaurant A, that restaurant can be in multiple different hex ETD ranges. Restaurant A could be 10 minutes from hexagon 1, 30 minutes from hexagon 2, which means that we store it a couple of times or multiple times in this case. That is a case where at the cost of storage and ingestion level offline optimization, we get the benefit of making the query faster.
Even for this, we tried a couple of different approaches, and we will soon enough have a blog post which talks more about the multiple alternate benchmarks that we did, where we would go in-depth into one of the other approaches we tried. We tried a BKD-tree approach to see, can we do this in log-in operation, and also a couple of other approaches around, I would only maintain the hexagons as part of the KD-tree, but in the retrieval layer, I could make a gRPC call to get the ETD information and then sort it in memory. Will that work? We did a bunch of different things to get there.
Finally, this is how our query layer looks like. Like Karthik mentioned, this is like gRPC layer between delivery and the search platform. We added a new paradigm of these range queries and we started having multiple ranges that we can operate with. This enabled us to leverage the power of parallelization. To visualize this, if a request comes in, let’s say somewhere in the yellow circle, for that request, there will be multiple queries which would be sent from the application layer all the way to the storage layer.
One query would be for the yellow layer, which is the closest bucket, and another query for the light green and dark green and so on. This is how we were able to get this nX in selection expansion at constant latency, regardless of which line of business that we care about. It involved changes in every single part of search and delivery ecosystem, multiple services, multiple engineers to get it to the finish line. After we made all of these changes, we put it into production and we saw that the latency decreased by 50%, which we thought was originally not possible. The cherry on top is we were also able to increase the recall. Before this, we had a different algorithm to query the concentric circle of expanding radius, and in that algorithm, we made a tradeoff between recall and latency. In this case, we were able to get more stores because that is how the rankers are able to see more candidates to make the optimization.
One of the other use cases that we haven’t spoken about so far, but also important enough in terms of customer experience, is the non-deliverable stores. In Uber, at least in the restaurant side, there can be many cases where you would look for a store, but it is not yet available, not yet deliverable, but it is available for pickup. The reason this exists is based on marketplace conditions, where the merchants are located, where we could send couriers, the time of the day and whatnot.
At some time of the day, we won’t be able to deliver to a restaurant, and this deliverability of a particular restaurant is also dynamic. Given this, we still want the eater to know that we do have this restaurant, but for some other reasons, we won’t be able to deliver at this particular point in time. We wanted to support this. Even in this use case, we moved a bunch of complexity from the query layer into the ingestion layer. At the ingestion layer, we did an intersection of how many of these hexagons are deliverable from the store, how many of them are only discoverable. We did that discoverable minus deliverable intersection, stored it in the index, so at the time of query layer, we would quite simply be able to say that, ok, it’s either in the deliverable or in the discoverable, and I could get it from there.
Key Takeaways
Overall, what we wanted to get out of this is, we started from first principles. When the latency shot up, we did a benchmark to understand where it is coming from, and started to narrow it down to the sharding strategy of, I have a San Francisco document and I have a bunch of Japan documents, because Japan has the most concentrated restaurants possible, so given that, if I were to take a San Francisco request and go through a bunch of Japan restaurants, that is obviously going to increase the latency. That is where the millisecond latency in get next doc came in. Index layout is one of the most overlooked pieces of software that we don’t even look at, where we needed to spend the two to three years to understand the query pattern, and then figure out what is it that we needed to do in our index layout so that it can be optimized for the request pattern that we care about.
Then, the sharding strategy needed to be aligned based on what we are trying to get at. We even saw test stores, which were part of the production index, which was adding to this latency. Three times the stores that we had originally were test stores, and we were processing all of those things when we were trying to process a real-time request, so we needed to create a separate cluster for the test stores.
Apart from this, there were a few other expensive operations which used to happen in the query layer. We had some fallbacks available at the query layer. In any distributed system, there is always going to be timeout. There is always going to be some data which is not available from the upstream. When those things happen, we used to have a query layer fallback to say, try to get it from this place, or if you don’t get it from this service, get it from this other service, or get it from a bunch of other places. We moved all of this fallback logic to the ingestion layer, so at the query layer, we just know that I’m going to query and get the data that I need, and all of the corner cases are being handled.
Apart from the parallelization based on ETD, we also had a bunch of other parallelizations in terms of, this is a strong match in terms of query, this is a fuzzy match, and this is either/or match, let’s say Burger and King would mean that I’m going to look for stores which have Burger and also look for stores which have King, and then do a match. We did all of these different things to leverage the non-overlapping subqueries and get the power of parallelization.
Time to Resolution
How much time do you think was expected to be spent to solve this problem? We spent about two to three months to identify where the problem is, because there are multiple different teams, like feed is a separate team, ads is a separate team, suggestions is a separate team, 1000 engineers together. We needed to instrument in multiple different parts to even identify the problem for two to three months. It took us four to six months to get to the first round of XP. Right now, I think this Q1 or so, we are available in the rest of the world too.
Questions and Answers
Participant 1: You did all this moving of stuff to the ingestion side, is there anything you put in there that you don’t need anymore, that you’d want to go back and take out?
Narayanan: This architecture that we have is also something which is evolving. From that perspective, there are some changes that we did in terms of live ingestion. I would give an example. We went with the idea that many use cases need to be live ingested, and then we realized that there are some cases which don’t even need to be ingested at that time, which would also help us in building the indexes faster. The SLAs will become better. One thing that we decided to take out later is when a store moves a location, that location update used to be a live ingestion, which will go through Kafka and then get it into the index.
Operations said, we need to get it in right after the store moves, and it has to be in milliseconds of latency to get it ingested. When we started understanding more of what the use case is, there is a time period involved between when the store decides to move a location, when ops gets to know that, and when tech will start moving it. They usually have made this decision two or three months in advance, and we have time for about a week or two weeks to actually make that transition. We decided that, the priority queue approach that he talked about as part of the ingestion, so we don’t need this as a priority, because this can go as part of the base index build, that is not going to use my compute resources.
Participant 2: You mentioned about the two months to identify the problem, and it takes two weeks to solve it. What kind of observability platform do you use to measure these metrics? Do you use any OpenTelemetry to identify where those queries are slowing down, and what queries are performing?
Narayanan: The expectation when we started the problem was that we will land it in two weeks, not that it took us two weeks to solve the problem.
On OpenTelemetry, we have in-house telemetry services that we have in place. In fact, there is one company branched out of some of the engineers who worked in the team. We use M3. That is our metric system, and that is what we integrated. Jaeger for tracing. When we started instrumenting it, at that time our search infrastructure wasn’t integrated with M3, so that was also something that we needed to do along the way to get it instrumented and then get it out the door. One reason we didn’t do that at that time was because of in-memory usage for, it’s a sidecar agent. Because of that, we didn’t want to have that in-memory usage at the time of production. We spun off a separate cluster, which was very identical in terms of hardware configurations and capacity, and that is where we did all of our benchmarks so that it doesn’t impact production.
Participant 3: You said you use Lucene for indexing. Does that mean that for searching specifically, you have a separate cluster that is specifically used just for searching versus indexing, or is it the same cluster that serves both reads and writes?
Ramasamy: We use the same cluster for search and indexing at the time. If you notice the architecture, we have two components of ingestion. One is the batch ingestion and the real-time ingestion. What we do is we move all of the heavy lifting on the indexing to the batch side, and the live ingestion or real-time ingestion is kept lightweight. Searcher node is utilized mostly for queries. Very little is used for indexing. That’s the current state. We are also working on the next generation system where we are going to fully separate the searcher and the indexer.
Participant 4: I would think that most people are querying for food when they’re at home or at work, and so subsequent queries are going to be pretty common. Do you all do anything in order to reduce the search space, you effectively cache the hex cells on the subsequent calls? For example, if I’m at my house, on the first query, you have to go out and do the work to determine what the boundaries are, but then on the subsequent queries, the geography isn’t changing. The only thing that’s changing is the restaurants. Have you all looked at that type of caching for subsequent queries?
Narayanan: We do have a cache in place, not for the purposes that you’re looking for. We don’t cache some of these requests, because if we look at the session, so throughout the session we do have some information that we maintain in memory, and then we could serve from there. We haven’t done a distributed cache there. Many at times, we want to also be able to dynamically look at store availability, item availability, which changes very often especially during the peak times. People run out of things, like restaurants run out of things. Because of that, we don’t intentionally do caching for that particular purpose.
Also, the delivery radius or the deliverability also expands and shrinks based on search, based on whether there is accidents in that area, rerouting happens and whatnot. There is a lot of those things in place. If there is an incident, someone could go change, rejigger those delivery zones too. We want that real time to reflect, because the last thing someone wants is to be able to add everything into their cart and then see that the store is no longer available. That is the reason we don’t invest heavily in caching at the time of retrieval in that part, but we use it for a different part in the query layer.
See more presentations with transcripts
Podcast: Building Empathy and Accessibility: Fostering Better Engineering Cultures and Developer Experiences
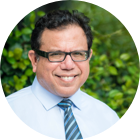
MMS • Erin Doyle
Article originally posted on InfoQ. Visit InfoQ
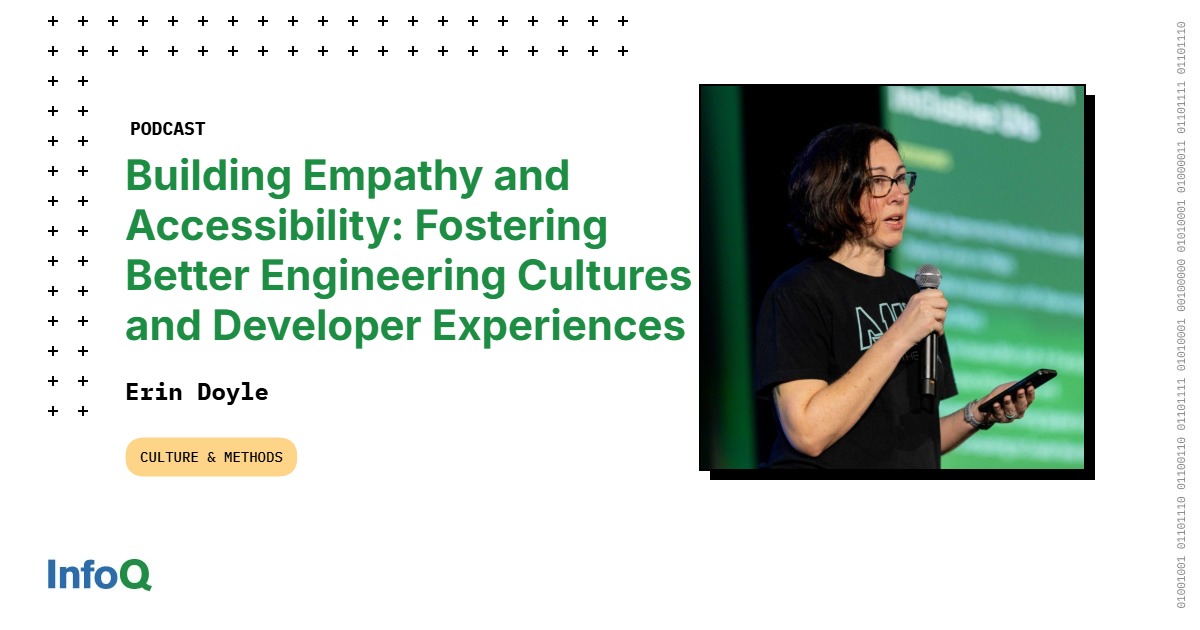
Transcript
Shane Hastie: Good day folks. This is Shane Hastie for the InfoQ Engineering Culture podcast. Today I’m sitting down with Erin Doyle. Erin, welcome. Thanks for taking the time to talk to us.
Erin Doyle: Thank you. Really excited to be here.
Shane Hastie: My normal starting point on these conversations is, who’s Erin?
Introductions [01:06]
Erin Doyle: I would describe myself as a generalist, a jack of all trades. I’ve done a lot over the course of my career. Started out at the Kennedy Space Center doing full stack development for the workers who would work on the space shuttle. After that, I did sort of product support, customer support, solutions engineering, which I wouldn’t say was my favorite job, but I learned a lot about troubleshooting, debugging, jumping into code that I didn’t write, and that was pretty valuable. After that, I did mobile development, which I was brand new to, and it was new to the company, so that was an exciting journey. And then I moved on to a full stack e-commerce team, and that’s when I really dove deep into web accessibility because that was a huge priority for the product, and had to learn all about that. And so then I got into talking and teaching workshops on web accessibility.
And then after that I did a little consultancy, which was another completely different experience, had to do everything, all the front, all the back, all the DevOps. And then eventually I moved over to a platform team. So, I’d done full stack development forever and then I got into platform development because I love the idea of supporting developers as my customers. I love doing the work to help enable my teammates, fellow developers to work more efficiently, effectively. And so I got to do a lot more of that over on the platform team. I got to wear a lot of hats, SRE, DevEx, DevProd, Ops, everything. So, now my final step in my journey, I just started a new job as a founding engineer at Quotient where we do development productivity software. So, that’s been a dream of mine. And of course as a founding engineer, I get to wear all the hats. The build-up of my career has led to this and I’m really excited about it.
Shane Hastie: Thank you. Let’s dig in first in one of those early passions, accessibility, web accessibility. Why does it matter so much?
Why accessibility matters [03:22]
Erin Doyle: Yes. It’s huge. And primarily it was huge to our customers at that company because we had a white label application for businesses, and these are businesses selling a product, and so, A, there was a lot of legal ramifications of websites that are trying to sell product. If they’re not accessible, people could sue for that. And we did have customers who had been sued for their own websites in the past, so it was a huge priority for them to be safe from legal action. But also if a customer can’t check out, if a customer can’t buy your product, you’re not making as much money.
Unfortunately, the bottom line for the business is maybe not altruistic, but that’s where it started. And once it started, and we could get into that, I could start talking to people about, we need to do this because we care, because accessibility matters to all of us. We should all have an equal experience using the web and mobile. It’s become our world. Everything we do is online. And so if we’re blocking people from being able to do the things they need to be able to do, the things they want to be able to do, that’s just not right. And so to care about our fellow human, we want to make these experiences equal and accessible. So, those are the big reasons why, legal, to make money, and because we care about our fellow humans.
Shane Hastie: What does good accessibility come down to?
Accessibility practices [04:57]
Erin Doyle: I think it’s really understanding your users, your audience and how they’re approaching your product. So, there’s a whole range of limitations. I’m not even going to just call them disabilities because sometimes it’s limitations. Sometimes it’s a temporary limitation that any of us could run into at any point in time. It could be environmental, it could be health related, whatever. It’s that many of us may approach using the web with some sort of limitation, whether it’s manual, meaning, we can’t use our hands in the same ways, maybe we can’t use a mouse, maybe we have dexterity issues, maybe it’s visual. We’re either blind or colorblind or just have impairment in your vision. Maybe it’s auditory, you can’t hear if there’s something that’s playing. So, there’s a lot of ways that people might be limited. We need to understand, based on those limitations, how are they using the web? How are they using computers? How are they using mobile devices? And then actually experiencing that.
I think really we have to try to put ourselves in their shoes as best we can and test out our applications using those same approaches that they might be using. Maybe it’s keyboard only. Maybe you can up the contrast. There’s lots of color contrast tools that maybe people who are colorblind might use. I’ve found issues where suddenly buttons on the page disappeared when the contrast was turned up on the site, and so people wouldn’t even know that those were there. Screen readers, every device has options of screen readers, and so actually using your site with a screen reader and/or keyboard only is quite a different experience. So, really approaching your site with all those and seeing, what is that user experience like and how do we need to modify it?
What are the things that we need to have the screen reader announce to people? Are there things that as the state of the page changes, we need to let them know that this thing is visible now? Or there’s an error, what is it and where is it? So, we need to just approach the user experience differently. And that’s why it really helps if you can get designers involved in this, so it’s not landing at the development stage. It’s sort of like, what is the user experience for someone using a screen reader? What is it for someone using a keyboard, etc. So, then we can develop matching those experiences.
Shane Hastie: There are guidelines. Are they useful?
Making sense of accessibility guidelines and tools [07:56]
Erin Doyle: It can be overwhelming. If you go to the various guidelines that are out there or if you look at the legal, well, and they vary by country. So, what the laws are in the US for accessibility are going to be different from the EU and so forth. So, approaching it from that direction can be really overwhelming. What are all the guidelines? How do I go through a checklist and make sure my site is doing all of these things? There are a lot of tools that help us, that can audit for the low hanging fruit, the things that are just easier to catch, not just statically, but even a runtime. We’ve got tools that you can run into the browser that as the state of the site changes, it can detect those things. But again, those are the really easier things to catch, so that you can at least start there.
I’ve always recommended taking a test-driven approach. Instead of starting with the guidelines and trying to make sure you’re matching them, start with a test-driven approach. Add the tools that are going to make your job a little easier, catch the low hanging fruit and have it tell you, this is inaccessible. And usually they’re really good at telling you why. You can get to more documentation that tells you why is this important? Why is this a problem? Here’s some recommendations on how to fix it. And so you can build up your knowledge set, bit by bit of, okay, these are the things we typically need to look for and these are the ways we typically fix them. And then you can create shared component libraries and things like that. So, you really only need to fix it once and it’s going to roll out to multiple places.
So, there are ways to help you be more efficient and get your arms around all these things to learn and make sure you’re doing. But after that, you really have to, like I said, you have to test the app. You have to find the rough edges of like, oh, this experience is terrible, or maybe technically this is accessible, but it’s really hard to do what I need to do, and then adjust that because we care about the user experience or we should. But you can go so far as auditors, there are many auditors out there that will test your site and will really go through the checklist and make sure you’re fully compliant. There are certifications out there you can get. But what’s tough is it’s a moving target. Every time you add a new feature, every time you make a change, you might inadvertently have just broken something about the accessibility of your site. So, it needs to be baked into the QA process as well to make sure you’re constantly testing and auditing for these things.
Shane Hastie: And as our styles of interaction change, so I’m thinking for many people today, the AI chatbot that’s becoming the core UI. How do we adapt to, as developers, how do we adapt to the new demands?
Adapting to new UI paradigms and accessibility challenges [11:15]
Erin Doyle: Yes. Again, we just have to be constantly testing, constantly learning and looking into those things. That’s a great point, is that this has become sort of a new UI paradigm that we’re running into a lot. And it may be that many of these chatbots, it’s one thing just to figure out, just to learn, how do I even add this AI chatbot? But then digging into making it accessible. So, we can’t just tack on these things and not continue to do our due diligence to make sure the experience is still accessible. Because if this is going to become the main way you interface with these products, you’ve just taken a site that might have been accessible for a lot of people and now added this huge barrier. It goes along with these overlays. I’m sure you’ve seen various websites that have this little icon somewhere at the bottom of the page and with some sort of accessibility icon on it.
And these overlay products really sell themselves as, we’re going to do all this for you, all you have to do is add this little thing to your code and we’re going to magically make it accessible. You don’t have to be an expert on accessibility, you just throw this in. And that’s really false. There are some ways that these overlays actually make things less accessible. Many of them add in their own screen reader, but it’s not as capable as the screen readers that are either built into the OS or that are natively available. So, they really don’t fix or make anything better. They actually hamper the accessibility. So, there’s no shortcuts. Just like everything else in software development, we have to learn our craft. We have to learn languages and frameworks and tools, and this should just really be one of the things that everybody, well, not everybody, front end developers, that just becomes part of your tool set, becomes part of your skill set.
Shane Hastie: Switching topics slightly, you made the point of your very wide experiences brought you to a state where you are comfortable now building products for engineers in that platform enablement, DevEx type space. What’s different when you are building products for engineers?
Building products for engineers [13:58]
Erin Doyle: Yes. First of all, what drew me there was empathy. I, firsthand, understand that experience when you have a workflow that’s painful, when you have friction in your day-to-day work, your feedback loops are slow or there’s just something awkward or annoying or painful or whatever, that you’re constantly being hampered and impeded and just doing the work that you need to do. I know how frustrating that is. I also know how hard it is to be on a product development team where you’re constantly under schedule pressure. We’ve got this feature we’ve got to get out, we’ve got this looming deadline, we don’t have time to go make this thing better or make this easier for us. So, we’re just going to put up with the pain, put up with these things that slow us down. We’re used to it, whatever. Maybe after the next feature launches, we can come back to it and make it better and that maybe in the future never happens.
And so I’ve experienced that over the years and I was always drawn to taking on those tasks of trying to make those things better for my team, so that my team members can stay heads down, focused on getting those features out, and I can try to help enable them to be more efficient. So, I’ve always felt passionate about that. So, when I had a chance to join a platform team, that was sort of my argument of, I know I don’t have a lot of experience in the Ops space or the DevOps space, I’ve got some, but I think I bring a perspective of what it’s like to be a product development engineer and what it’s like to deal with these things that are constantly getting in my way and what it’s like to work with a platform or DevOps team. What it’s like to be on the other side of the wall.
Understanding developer and platform team dynamics [15:50]
And so I was hoping to bring with that, this empathy, this understanding of the developer perspective. And there are all these stereotypes of when you’re on the dev side, you might be thinking, I really need help with this thing, but if I reach out to platform or DevOps, they’re going to think I’m stupid or they don’t have time. They’re so busy with all this stuff that they have to do, they don’t have time to help me with this thing. Or we speak different languages. They might be saying something that’s totally over my head and that might be a little intimidating. So, I have that. I understand what that’s like, and I have felt hampered in the past from collaborating or asking for help from the DevOps team or the platform team. So, jumping to the other side was really fascinating to see the perspective of the platform team working with developers, developers who are constantly being pushed and rushed and forced to cut corners and thus create problems that the platform team has to solve or SREs have to solve.
And so then there’s this stereotype of devs are lazy, devs are always cutting corners, devs don’t care about quality, or they don’t do their due diligence before they do a thing and they don’t want to collaborate with us. When we ask them to work with us to do something like database upgrades for instance, we need to work with them because they need to test their code to make sure it still works with the thing we’ve upgraded, but they don’t have time for this. They don’t make time for these kinds of things because it’s not on their product roadmap. So, I saw both sides of how we see each other over the wall, but having empathy for what it’s like to actually be in the shoes of either side was super powerful for me. And I was able to explain to my teammates, I know we think that maybe the devs are being lazy or maybe they should know more about this thing that we’re having to help them with, but they don’t have time.
Cognitive load and shifting responsibilities in development [18:06]
They’re taking on such a high cognitive load, especially these days as we’ve added so much in the cloud, as we’ve added so much tooling. We’ve got so many products, we’ve got so many options now and we’ve shifted left so much. The DevOps movement shifted a lot left to developers. The, you build it, you run it. Developers have to be responsible for a lot, a lot of infrastructure, a lot of architectural decisions, security, even as we just spoke about web accessibility. We’ve put a lot on their plates. So, in order to continue to meet their deadlines, they are forced to cut corners. They aren’t able to learn how to use every tool and how to really be knowledgeable about everything in the landscape. So, to explain that to my platform team members of like, we’ve got to keep in mind the context here of what they’re dealing with.
And then on the other end, how can we as a platform team change how we are seeing or interpreted by the developers, so that when they do need help, they’ll ask for it. So, they’re comfortable asking for the help they need. When they have to reach into this space of creating new infrastructure for the feature they need or getting the right amount of observability on some new feature, when they need that help, we need to be approachable, we need to be available or else they’re going to do it on their own. Again, going back to the DevOps movement, we have this concept of devs should just be able to do it all on their own in order to move quickly, but that’s asking too much these days. And so I really wanted to promote this idea of, we can meet you where you’re at. The platform team can stretch over the aisle and meet you where you’re at.
And so if you don’t know how to use these tools, if you’re not knowledgeable about the various infrastructure options available these days, let’s collaborate on it. Let’s work on the architectural design together. Bring us in as a partner, so that we can help you do these things to get that feature ready for production. Instead of the developer shouldering it all and not doing as good a job of it because they’re in an area they’re not experienced in. They’re doing their best, but if they don’t have that deep experience, they’re going to make mistakes, they’re going to miss things. And when they miss, it becomes the platform team or the SRE team’s problem later.
We’re going to have to fix that problem that blows up in production way down the road, or we’re going to find that we’re totally missing observability about something and maybe we have errors or we have problems that we don’t even know are happening or we’re not scaling appropriately. There’s so many outcomes based on just that interaction of devs trying to do more than they really are experienced or set up for success to do, and not feeling comfortable asking for help or collaborating with the platform team. So, building psychological safety, where they feel comfortable, they feel safe, they don’t feel judged, being able to reach out and say, “I really need help with this aspect of this feature I’m working on”.
Shane Hastie: Psychological safety, an incredibly important concept that’s become a buzzword. What does it really mean to create a safe environment where people can ask those slightly embarrassing questions or difficult questions?
Fostering psychological safety and collaboration [22:04]
Erin Doyle: Yes. I found that the more I learn about psychological safety, the more I start to see it everywhere, and I start to see that sort of chain reaction or root cause analysis on negative outcomes being the result of a lack of psychological safety. And it can be complex. It can be subtle because we’re humans. We’re humans interacting with other humans, and we’re all approaching those interactions with preconceived notions and whatever our histories are, whatever our psychological issues are. So, it’s complicated. But I’ve really found, and it’s been hard for me, that the more that I can model vulnerability myself, and especially as I’ve become more senior over the years, the more that I can show that there are things I don’t know or there are mistakes that I make, there’s questions I have, the more I hope I’m creating this environment for other people to feel like they can do the same.
As I was earlier on in my career, as I was just starting to become more senior, I felt a ton of pressure to prove myself, to prove that I deserved the role, and that created a lot of bad behavior. I was a perfectionist. I was a workaholic. If there was something I didn’t know, I couldn’t admit it, and I couldn’t ask for help because then people would know that I didn’t know everything and I wasn’t the best. So, it was really hard to take on all that pressure of like, “Oh, jeez, I don’t know this thing, but I don’t want anybody to know that I don’t know”. So, now I’m going to work extra hours. I’m going to work all weekend to learn this thing and try to present myself as if I knew it all along, and that’s really unhealthy.
And as sort of a negative side effect, I created this model, or I set this bar to my teammates that they had to be perfect too. When I never made any mistakes, I always knew everything, I had all the answers, then they felt, especially the more junior people, they thought like, “Oh, this is the standard, and so I too can’t ask questions. I too can’t ask for help because I guess we don’t do that here”. And so I created a really toxic environment around myself without realizing it. And so as I got a little more senior, a little more experienced, and I finally started hearing people talking about this, I think that’s another thing that’s changed a lot over the years, as more people talk about culture, as more people talk about psychology and working together, the more I was hearing things about psychological safety and how we can impact others with our behavior when we’re not cognizant about the model we’re setting for others.
So, it was really hard for me to go from that perfectionist, I have to be perfect, I have to prove myself to what I have to make mistakes. I have to show people that I don’t know everything, if I want them to feel comfortable working with me, if I want us to be able to collaborate. And I did see examples of where maybe I didn’t make the environment comfortable for others, and I knew that they had questions or I knew that they had things they wanted to say that I’d find out later, that they didn’t say to me or they didn’t ask me. And so I realized, oh, I’m not making people feel comfortable approaching me or being open with me with their thoughts or disagreeing with me. That’s a big thing.
If I don’t make the environment comfortable for people to feel like they can offer an alternate view of something, if I make a statement, if I pose a thought, if I do that in a way that’s too assertive, it could cause other people to feel like, “Oh, well, she must know better than I do”. Or “If I offer this contrary viewpoint, I’m going to sound stupid”. Whatever it may be, whatever that fear is that they’re having in their mind, I’m now not going to hear that alternate viewpoint. I might be wrong. I’m wrong all the time. Maybe there’s just some aspect of this that I’m missing or something that I don’t have personal experience with. And so if I’m shutting off that opportunity to hear that, maybe that was a better idea than what I had, maybe they thought of something that I didn’t and I missed. And so we’re so much better off when we can make people feel comfortable saying, “Oh, well what about this?” Or “Have you thought about that?” Without fear of, I don’t know, it being taken personally, it feeling like conflict.
So, somehow we have to create that environment where it’s not personal, it’s just normal. We can have discourse and it’s comfortable and it’s normal, and it’s how we do our work. But again, you have to sort of plant the seeds. You have to lay the foundation, and that comes from senior people modeling that behavior. Really, I noticed it as I was gaining experience when I would see those people that I looked up to, that I thought were really smart, talented, when I saw that they weren’t perfect, that there was things that they didn’t know and they felt comfortable, they weren’t ashamed, they weren’t apologetic, they were just like, “Hey, I’ve got this question”, or, “Oh, I just broke this thing in prod and just so you know, I’m fixing it”. But it’s not apologetic. It’s not scraping. It’s not, “Oh, I’m so sorry I broke prod. I’m so embarrassed”. We don’t have to be embarrassed. We make mistakes.
And so when you’ve got that attitude of like, “Yes. I made this mistake just FYI. I’m working on it”. It really lowers that barrier for us all to be open about like, “Yep. I made this mistake. Here’s what we’re going to do about it”. Or “I could use some help. I missed this thing”, or “I’m not really knowledgeable about this area that I’m working in, maybe someone else is, maybe they can help me”. And so the more we can just make that normal part of just how we work, the more we can allow space for all those things that if we didn’t have, we’re not doing our best work.
Shane Hastie: Being vulnerable, leading by example. It’s hard.
Modeling vulnerability and leading by example [28:36]
Erin Doyle: Yes. It’s really hard. I almost equate it to, I don’t know if you’ve ever jumped off something high up, jumped off into the water or, well, I guess you’d usually be jumping into water, but if you’ve ever taken a leap, I have a fear of heights, so that’s really scary for me. I’m never going to go skydiving or bungee jumping, but those concepts of, I’m going to take a leap and I have to believe that I’m going to be fine. It’s just this scary little thing that I have to get over, but the belief that I’m going to be fine is what pushes me to take that step. I feel that way all the time. I still to this day feel that way all the time. I’ll have a question or a problem or whatever, and I’ll pause and I’ll think, if I ask this, maybe I’ll sound stupid, this could be embarrassing, whatever.
I still have those thoughts, but then I just remind myself of that’s okay, you know that this is okay, and you know that showing this little bit of vulnerability on a regular basis is going to help someone else. So, that’s what kind of helps me take that leap.
Shane Hastie: I’ve learnt a lot of really good insights and good advice in there. If people want to continue the conversation, where can they find you?
Erin Doyle: Yes. The easiest place is probably LinkedIn. I’m on there. And I do have my own website. It’s got a few blog pages on it, unfortunately not as many as I’d like. And it’s also got whatever talks I’ve done or articles I’ve been featured, whatever I’m doing out in the community is listed there. And that’s just erindoyle.dev.
Shane Hastie: Thank you so much for taking the time to talk to us.
Erin Doyle: Thanks. It’s been a lot of fun.
Mentioned:
.
From this page you also have access to our recorded show notes. They all have clickable links that will take you directly to that part of the audio.
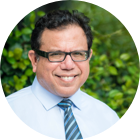
MMS • Rohit Garg Ankit Awasthi
Article originally posted on InfoQ. Visit InfoQ
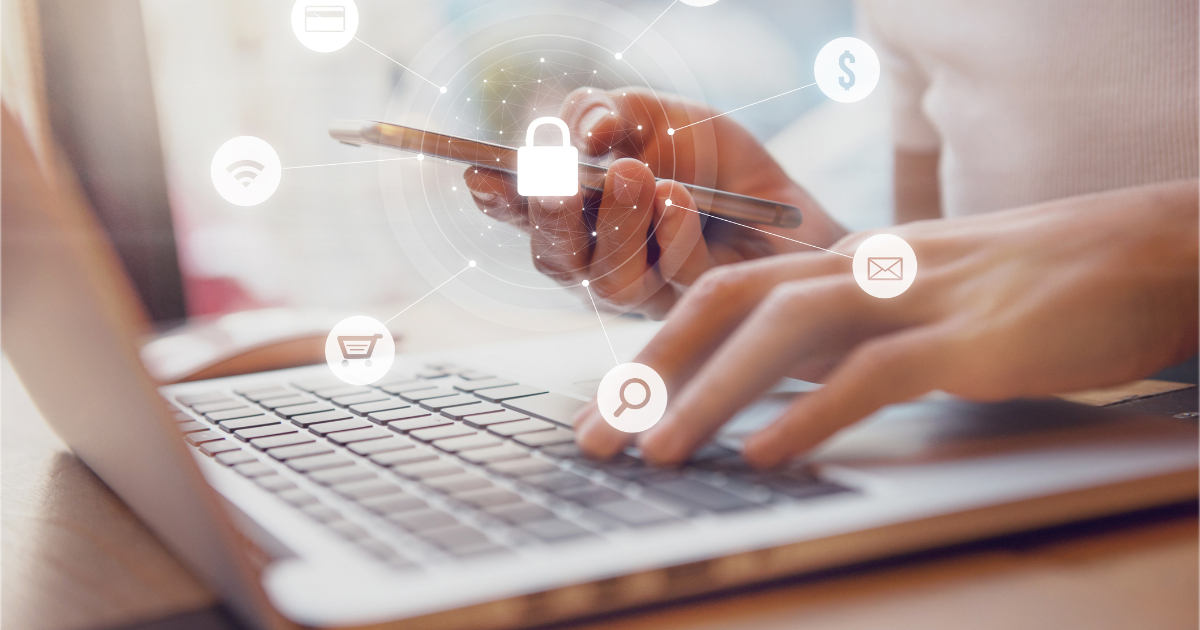
Key Takeaways
- Distributed cloud computing enables efficient data processing across multiple nodes.
- Privacy-enhanced technologies (PETs) ensure secure data analysis with compliance and protection.
- AI-powered tools streamline data processing workflows and identify potential security threats.
- Secure and private cloud computing technologies foster trust among organizations, enabling seamless collaboration.
- The integration of AI, PETs, and distributed cloud computing revolutionizes data processing and analysis.
As the world becomes increasingly digital, the need for secure and private data processing has never been more pressing. Distributed cloud computing offers a promising solution to this challenge by allowing data to be processed in a decentralized manner, reducing reliance on centralized servers and minimizing the risk of data breaches.
In this article, we’ll explore how distributed cloud computing can be combined with Privacy Enhanced Technologies (PETs) and Artificial Intelligence (AI) to create a robust and secure data processing framework.
What is Distributed Cloud Computing?
Distributed cloud computing is a paradigm that enables data processing to be distributed across multiple nodes or devices, rather than relying on a centralized server. This approach allows for greater scalability, flexibility, and fault tolerance, as well as improved security and reduced latency. Here is a more detailed look at three of these architectures: hybrid cloud, multi-cloud, and edge computing.
- Hybrid cloud combines on-premises data centers (private clouds) with public cloud services, allowing data and applications to be shared between them. Hybrid cloud offers greater flexibility and more deployment options. It allows businesses to scale their on-premises infrastructure up to the public cloud to handle any overflow, without giving third-party data centers access to the entirety of their data. Hybrid cloud architecture is ideal for businesses that need to keep certain data private but want to leverage the power of public cloud services for other operations. In a hybrid cloud environment, sensitive data may be stored on-premises, while less critical data is processed in the public cloud.
- Multi-cloud refers to the use of multiple cloud computing services from different providers in a single architecture. This approach avoids vendor lock-in, increases redundancy, and allows businesses to choose the best services from each provider. Companies that want to optimize their cloud environment by selecting specific services from different providers to meet their unique needs can benefit from this tool. However, using multi-cloud can result in data fragmentation, where sensitive information is scattered across different cloud environments, increasing the risk of data breaches and unauthorized access. To mitigate these risks, organizations must implement robust data governance policies, including data classification, access controls, and encryption mechanisms, to protect sensitive data regardless of the cloud provider used.
- Edge computing brings computation and data storage closer to the location where it is needed, to improve response times and save bandwidth. This tool reduces latency, improves performance, and allows for real-time data processing. It is particularly useful for IoT devices and applications that require immediate data processing, such as autonomous vehicles, smart cities, and industrial IoT applications. Edge computing faces a significant security challenge in the form of physical security risks due to remote or public locations of edge devices, which can be mitigated by implementing tamper-evident or tamper-resistant enclosures, and using secure boot mechanisms to prevent unauthorized access, ultimately reducing the risk of physical tampering or theft and ensuring the integrity of edge devices and data.
Distributed cloud computing is enhanced when leveraging PETs, which are designed to protect sensitive information from unauthorized access, while still allowing for secure data processing across distributed systems.
PETs
PETs offer powerful tools for preserving individual privacy while still allowing for data analysis and processing. From homomorphic encryption to secure multi-party computation, these technologies have the potential to transform the way we process data.
To illustrate the practical application of these powerful privacy-preserving tools, let’s examine some notable examples of PETs in action, such as Amazon Clean Rooms, Microsoft Azure Purview, and Meta’s Conversions API Gateway.
Amazon Clean Rooms
Amazon Clean Rooms is a secure environment within AWS that enables multiple parties to collaborate on data projects without compromising data ownership or confidentiality. Amazon provides a virtual “clean room” where data from different sources can be combined, analyzed, and processed without exposing sensitive information. Their framework leverages differential privacy features, which add noise to data queries to prevent the identification of individual data points and maintain privacy even when data is aggregated. Additionally, secure aggregation techniques are employed involving combining data in a way that individual data points cannot be discerned, often through methods like homomorphic encryption or secure multi-party computation (MPC) that allow computations on encrypted data without revealing it.
The core idea behind Amazon Clean Rooms is to create a trusted environment by leveraging AWS Nitro Enclaves, which are a form of Trusted Execution Environment (TEE). Clean rooms provide a secure area within a processor to execute code and process data, protecting sensitive data from unauthorized access. Data providers can share their data with other parties, such as researchers, analysts, or developers, without risking data breaches or non-compliance with regulations.
In a healthcare scenario, Amazon Clean Rooms can facilitate collaboration among different healthcare providers by allowing them to share and analyze anonymized patient data to identify trends in a specific disease without compromising patient privacy. For instance, multiple hospitals could contribute anonymized datasets containing patient demographics, symptoms, treatment outcomes, and other relevant information into a clean room. Using differential privacy, noise is added to the data queries, ensuring that individual patient identities remain protected even as aggregate trends are analyzed.
Secure aggregation techniques, such as homomorphic encryption and secure multi-party computation, enable computations on this encrypted data, allowing researchers to identify patterns or correlations in disease progression or treatment efficacy without accessing raw patient data. This collaborative analysis can lead to valuable insights into disease trends, helping healthcare providers improve treatment strategies and patient outcomes while maintaining strict compliance with privacy regulations.
These improved treatment strategies are achieved through a combination of advanced security features, including:
- Data encryption both in transit and at rest, ensuring that only authorized parties can gain access
- Fine-grained access controls ensure that each party can only use the data for which they are authorized
- Auditing and logging of all activities within the clean room for a clear trail of data access and use
Microsoft Azure Purview
Microsoft Azure Purview is a cloud-native data governance and compliance solution that helps organizations manage and protect their data across multiple sources, including on-premises, cloud, and hybrid environments. It provides a unified platform for data governance, discovery, classification, and compliance, enabling organizations to monitor and report on regulatory requirements such as General Data Protection Regulation (GDPR), Health Insurance Portability and Accountability Act (HIPAA), and the California Consumer Privacy Act (CCPA). With features including automated data discovery and classification, data lineage and visualization, and risk management, Azure Purview improves data governance and compliance, enhances data security and protection, increases transparency and visibility into data usage, and simplifies data management and reporting.
- Data classification. Azure Purview data classification employs a hybrid approach, combining Microsoft Information Protection (MIP) SDK and Azure Machine Learning (AML) to identify sensitive data. It leverages content inspection APIs to extract features from data stores, which are then matched against predefined classification rules or machine learning models (e.g., Support Vector Machines (SVMs) and Random Forests) to assign classification labels (e.g., “Confidential” and “Sensitive”) and corresponding sensitivity levels (low to high). This enables targeted security controls and compliance with regulatory requirements.
- Data lineage. Azure Purview’s data lineage tracks the origin, processing, and movement of data across Azure resources. It constructs a graph from metadata sources like Azure Data Factory and Azure Databricks, illustrating relationships between data assets. This relationship illustration helps users to identify potential privacy risks, ensure compliance, and detect sensitive data misuse by traversing the graph and visualizing data flows.
- Integration with PETs. While Azure Purview itself is not a PET, it can integrate with other tools and technologies that enhance data privacy. For example, it can work alongside encryption tools like Azure Key Vault, access control mechanisms like Azure Active Directory (AAD), and anonymization techniques like k-anonymity and differential privacy. By providing a unified view of data governance and compliance, Azure Purview makes it easier to implement and manage these PETs, ensuring that data privacy is maintained throughout its lifecycle.
Meta’s Conversions API Gateway
Meta’s Conversions API Gateway is a distributed cloud computation framework that focuses on user data privacy and security. It is designed to comply with regulations, helping advertisers and app developers establish trust with their users. By installing it in their managed cloud environments, users maintain control over not just their data but the underlying infrastructure as well.
The platform integrates security and data management by utilizing role-based access control (RBAC) to create a policy workflow. This workflow enables users and advertisers to effectively manage the information they share with third-party entities. By implementing access controls and data retention policies, the platform ensures that sensitive data is safeguarded against unauthorized access, thereby complying with regulatory standards like the General Data Protection Regulation.
Having explored some key examples of PETs, it’s insightful to consider their current level of real-world application. Based on industry research, the following data provides an overview of the adoption rates of various PETs.
Adoption rates of PETs
Technology | Description | Adoption Rate | What is the adoption about? |
Homomorphic Encryption (HE) | Enables computations on encrypted data without decryption | 22% | Companies adopting HE to protect sensitive data in cloud storage and analytics |
Zero-Knowledge Proofs (ZKP) | Verifies authenticity without revealing sensitive information | 18% | Organizations using ZKP for secure authentication and identity verification |
Differential Privacy (DP) | Protects individual data by adding noise to query results | 25% | Data-driven companies adopting DP to ensure anonymized data analysis and insights |
Secure Multi-Party Computation (SMPC) | Enables secure collaboration on private data | 12% | Businesses using SMPC for secure data sharing and collaborative research |
Federated Learning (FL) | Trains AI models on decentralized, private data | 30% | Companies adopting FL to develop AI models while preserving data ownership and control |
Trusted Execution Environments (TEE) | Provides secure, isolated environments for sensitive computations | 20% | Organizations using TEE to protect sensitive data processing and analytics |
Anonymization Techniques (e.g., k-anonymity) | Masks personal data to prevent reidentification | 40% | Companies adopting anonymization techniques to comply with data protection regulations |
Pseudonymization Techniques (e.g., tokenization) | Replaces sensitive data with pseudonyms or tokens | 35% | Businesses using pseudonymization techniques to reduce data breach risks and protect customer data |
Amazon Clean Rooms | Enables secure, collaborative analysis of sensitive data in a controlled environment | 28% | Companies using Amazon Clean Rooms for secure data collaboration and analysis in regulated industries |
Microsoft Azure Purview | Provides unified data governance and compliance management across multiple sources | 32% | Organizations adopting Azure Purview to streamline data governance, compliance, and risk management |
Sources:
The adoption rates illustrate the growing importance of privacy-preserving techniques in distributed environments. Now, let’s explore how AI can be integrated into this landscape to enable more intelligent decision-making, automation, and enhanced security within distributed cloud computing and PET frameworks.
AI in Distributed Cloud Computing
AI has the potential to play a game-changing role in distributed cloud computing and PETs. By enabling intelligent decision-making and automation, AI algorithms can help us optimize data processing workflows, detect anomalies, and predict potential security threats. AI has been instrumental in helping us identify patterns and trends in complex data sets. We’re excited to see how it will continue to evolve in the context of distributed cloud computing. For instance, homomorphic encryption allows computations to be performed on encrypted data without decrypting it first. This means that AI models can process and analyze encrypted data without accessing the underlying sensitive information.
Similarly, AI can be used to implement differential privacy, a technique that adds noise to the data to protect individual records while still allowing for aggregate analysis. In anomaly detection, AI can identify unusual patterns or outliers in data without requiring direct access to individual records, ensuring that sensitive information remains protected.
While AI offers powerful capabilities within distributed cloud environments, the core value proposition of integrating PETs remains in the direct advantages they provide for data collaboration, security, and compliance. Let’s delve deeper into these key benefits, challenges and limitations of PETs in distributed cloud computing.
Benefits of PETs in Distributed Cloud Computing
PETs, like Amazon Clean Rooms, offer numerous benefits for organizations looking to collaborate on data projects while maintaining regulatory compliance. Some of the key advantages include:
- Improved data collaboration. Multiple parties work together on data projects, fostering innovation and driving business growth.
- Enhanced data security. The secure environment ensures that sensitive data is protected from unauthorized access or breaches.
- Regulatory compliance. Organizations can ensure compliance with various regulations and laws governing data sharing and usage.
- Increased data value. By combining data from different sources, organizations can gain new insights and unlock new business opportunities.
The numerous benefits of integrating PETs within distributed cloud environments pave the way for a wide range of practical applications. Let’s explore some key use cases where these combined technologies demonstrate significant value.
Limitations and Challenges
Despite their benefits, implementing PETs can be complex and challenging. Here are some of the key limitations and challenges:
Scalability and performance. PETs often require significant computational resources, which can impact performance and scalability. As data volumes increase, PETs may struggle to maintain efficiency. For example, homomorphic encryption, which allows computations on encrypted data, can be computationally intensive. This can be a major limitation for real-time applications or large datasets.
- Interoperability and standardization. Different PETs may have varying levels of compatibility, making it difficult to integrate them into existing systems. Lack of standardization can hinder widespread adoption and limit the effectiveness of PETs.
- Balancing privacy and utility. PETs often involve trade-offs between privacy and utility; finding the right balance is crucial. Organizations must carefully consider the implications of PETs on business operations and decision-making.
- Data quality and accuracy. PETs rely on high-quality data to function effectively; poor data quality can compromise their accuracy. Ensuring data accuracy and integrity is critical to maintaining trust in PETs.
- Regulatory compliance and governance. PETs must comply with various regulations, such as GDPR and CCPA, which can be time-consuming and costly. Ensuring governance and accountability in PET implementation is essential to maintain trust and credibility.
Use Cases
Distributed cloud computing PET frameworks can be applied to a wide range of use cases, including:
- Marketing analytics. Marketers can use PETs to analyze customer data from different sources, such as social media, website interactions, or purchase history, to gain a deeper understanding of customer behavior and preferences. Businesses can further analyze customer data from different sources, such as demographics, behavior, or preferences, to create targeted marketing campaigns and improve customer engagement. Instead of centralizing the data, they use federated learning to train the model on the decentralized data stored at each hospital.
- Financial analysis. Financial institutions can use AI in distributed cloud computing to analyze financial data from different sources, such as transaction records, credit reports, or market data, to identify trends and opportunities. To preserve customer privacy, the institution uses differential privacy to add noise to the data before feeding it into the AI model.
- Healthcare analytics. Healthcare organizations can use Amazon Clean Rooms and AI to analyze patient data from different sources, such as electronic health records, medical imaging, or claims data, to improve patient outcomes and reduce costs.
- Major video streaming platforms demonstrate practical applications of privacy-enhanced distributed computing. Netflix and Disney+ use edge computing for localized content delivery and regional data compliance. YouTube applies differential privacy for secure viewer analytics and recommendations. Hulu implements federated learning across devices to improve streaming quality without centralizing user data.
Summary
Distributed cloud computing, combined with PETs and AI, offers a robust framework for secure and private data processing. By decentralizing data processing across multiple nodes, this approach reduces reliance on centralized servers, enhancing scalability, flexibility, fault tolerance, and security while minimizing latency and the risk of data breaches. PETs, such as homomorphic encryption and secure multi-party computation, enable secure data analysis without compromising individual privacy, transforming how data is handled.
Looking ahead, future developments may include integrating edge computing to enhance real-time data processing, exploring quantum computing applications for complex problem-solving and cryptography, developing autonomous data management systems that utilize AI and machine learning, creating decentralized data marketplaces that leverage blockchain technology, and incorporating human-centered design principles to prioritize data privacy and security.
“The future of cloud computing is not just about technology; it’s about trust,”
Satya Nadella, CEO of Microsoft.
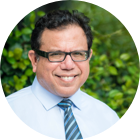
MMS • RSS
Posted on mongodb google news. Visit mongodb google news
Several brokerages have updated their recommendations and price targets on shares of MongoDB (NASDAQ: MDB) in the last few weeks:
- 4/23/2025 – MongoDB had its price target lowered by analysts at Piper Sandler from $280.00 to $200.00. They now have an “overweight” rating on the stock.
- 4/17/2025 – MongoDB was upgraded by analysts at Redburn Atlantic from a “sell” rating to a “neutral” rating. They now have a $170.00 price target on the stock.
- 4/16/2025 – MongoDB had its price target lowered by analysts at Morgan Stanley from $315.00 to $235.00. They now have an “overweight” rating on the stock.
- 4/15/2025 – MongoDB had its price target lowered by analysts at Mizuho from $250.00 to $190.00. They now have a “neutral” rating on the stock.
- 4/11/2025 – MongoDB had its price target lowered by analysts at Stifel Nicolaus from $340.00 to $275.00. They now have a “buy” rating on the stock.
- 4/1/2025 – MongoDB was upgraded by analysts at Daiwa America to a “strong-buy” rating.
- 4/1/2025 – MongoDB is now covered by analysts at Daiwa Capital Markets. They set an “outperform” rating and a $202.00 price target on the stock.
- 4/1/2025 – MongoDB had its price target lowered by analysts at Citigroup Inc. from $430.00 to $330.00. They now have a “buy” rating on the stock.
- 3/31/2025 – MongoDB had its price target lowered by analysts at Truist Financial Co. from $300.00 to $275.00. They now have a “buy” rating on the stock.
- 3/7/2025 – MongoDB had its price target lowered by analysts at Macquarie from $300.00 to $215.00. They now have a “neutral” rating on the stock.
- 3/6/2025 – MongoDB had its “buy” rating reaffirmed by analysts at Citigroup Inc..
- 3/6/2025 – MongoDB had its price target lowered by analysts at Barclays PLC from $330.00 to $280.00. They now have an “overweight” rating on the stock.
- 3/6/2025 – MongoDB had its price target lowered by analysts at Piper Sandler from $425.00 to $280.00. They now have an “overweight” rating on the stock.
- 3/6/2025 – MongoDB had its price target lowered by analysts at Morgan Stanley from $350.00 to $315.00. They now have an “overweight” rating on the stock.
- 3/6/2025 – MongoDB had its price target lowered by analysts at Oppenheimer Holdings Inc. from $400.00 to $330.00. They now have an “outperform” rating on the stock.
- 3/6/2025 – MongoDB had its price target lowered by analysts at Royal Bank of Canada from $400.00 to $320.00. They now have an “outperform” rating on the stock.
- 3/6/2025 – MongoDB had its price target lowered by analysts at Wedbush from $360.00 to $300.00. They now have an “outperform” rating on the stock.
- 3/6/2025 – MongoDB had its price target lowered by analysts at Robert W. Baird from $390.00 to $300.00. They now have an “outperform” rating on the stock.
- 3/6/2025 – MongoDB was downgraded by analysts at Wells Fargo & Company from an “overweight” rating to an “equal weight” rating. They now have a $225.00 price target on the stock, down previously from $365.00.
- 3/6/2025 – MongoDB had its price target lowered by analysts at The Goldman Sachs Group, Inc. from $390.00 to $335.00. They now have a “buy” rating on the stock.
- 3/6/2025 – MongoDB had its price target lowered by analysts at Stifel Nicolaus from $425.00 to $340.00. They now have a “buy” rating on the stock.
- 3/6/2025 – MongoDB had its price target lowered by analysts at Truist Financial Co. from $400.00 to $300.00. They now have a “buy” rating on the stock.
- 3/6/2025 – MongoDB had its price target lowered by analysts at Bank of America Co. from $420.00 to $286.00. They now have a “buy” rating on the stock.
- 3/6/2025 – MongoDB had its price target lowered by analysts at Canaccord Genuity Group Inc. from $385.00 to $320.00. They now have a “buy” rating on the stock.
- 3/6/2025 – MongoDB had its price target lowered by analysts at Needham & Company LLC from $415.00 to $270.00. They now have a “buy” rating on the stock.
- 3/5/2025 – MongoDB had its “sector perform” rating reaffirmed by analysts at Scotiabank. They now have a $240.00 price target on the stock, down previously from $275.00.
- 3/5/2025 – MongoDB is now covered by analysts at Cantor Fitzgerald. They set an “overweight” rating and a $344.00 price target on the stock.
- 3/5/2025 – MongoDB was downgraded by analysts at KeyCorp from a “strong-buy” rating to a “hold” rating.
- 3/4/2025 – MongoDB was given a new $350.00 price target on by analysts at UBS Group AG.
- 3/4/2025 – MongoDB had its “buy” rating reaffirmed by analysts at Rosenblatt Securities. They now have a $350.00 price target on the stock.
- 3/3/2025 – MongoDB was upgraded by analysts at Monness Crespi & Hardt from a “sell” rating to a “neutral” rating.
- 3/3/2025 – MongoDB had its price target lowered by analysts at Loop Capital from $400.00 to $350.00. They now have a “buy” rating on the stock.
MongoDB Stock Up 6.5 %
NASDAQ MDB opened at $173.21 on Friday. MongoDB, Inc. has a 12-month low of $140.78 and a 12-month high of $387.19. The firm’s 50 day moving average is $197.65 and its 200-day moving average is $249.73. The company has a market capitalization of $14.06 billion, a PE ratio of -63.22 and a beta of 1.49.
MongoDB (NASDAQ:MDB – Get Free Report) last posted its quarterly earnings results on Wednesday, March 5th. The company reported $0.19 earnings per share for the quarter, missing analysts’ consensus estimates of $0.64 by ($0.45). MongoDB had a negative net margin of 10.46% and a negative return on equity of 12.22%. The company had revenue of $548.40 million during the quarter, compared to the consensus estimate of $519.65 million. During the same quarter in the prior year, the business earned $0.86 earnings per share. As a group, sell-side analysts expect that MongoDB, Inc. will post -1.78 EPS for the current year.
Insider Activity
In other MongoDB news, CAO Thomas Bull sold 301 shares of the stock in a transaction dated Wednesday, April 2nd. The shares were sold at an average price of $173.25, for a total value of $52,148.25. Following the transaction, the chief accounting officer now directly owns 14,598 shares of the company’s stock, valued at $2,529,103.50. This represents a 2.02 % decrease in their position. The transaction was disclosed in a legal filing with the Securities & Exchange Commission, which is available through this hyperlink. Also, CEO Dev Ittycheria sold 8,335 shares of the firm’s stock in a transaction that occurred on Tuesday, January 28th. The stock was sold at an average price of $279.99, for a total transaction of $2,333,716.65. Following the sale, the chief executive officer now owns 217,294 shares of the company’s stock, valued at $60,840,147.06. This trade represents a 3.69 % decrease in their ownership of the stock. The disclosure for this sale can be found here. In the last ninety days, insiders have sold 47,680 shares of company stock valued at $10,819,027. 3.60% of the stock is owned by company insiders.
Institutional Inflows and Outflows
A number of institutional investors and hedge funds have recently added to or reduced their stakes in MDB. Vanguard Group Inc. boosted its stake in shares of MongoDB by 0.3% in the fourth quarter. Vanguard Group Inc. now owns 7,328,745 shares of the company’s stock valued at $1,706,205,000 after buying an additional 23,942 shares during the period. Franklin Resources Inc. boosted its stake in shares of MongoDB by 9.7% in the 4th quarter. Franklin Resources Inc. now owns 2,054,888 shares of the company’s stock valued at $478,398,000 after purchasing an additional 181,962 shares in the last quarter. Geode Capital Management LLC boosted its position in MongoDB by 1.8% in the fourth quarter. Geode Capital Management LLC now owns 1,252,142 shares of the company’s stock valued at $290,987,000 after buying an additional 22,106 shares in the last quarter. First Trust Advisors LP boosted its holdings in shares of MongoDB by 12.6% during the 4th quarter. First Trust Advisors LP now owns 854,906 shares of the company’s stock valued at $199,031,000 after acquiring an additional 95,893 shares in the last quarter. Finally, Norges Bank bought a new stake in MongoDB during the 4th quarter worth $189,584,000. 89.29% of the stock is owned by institutional investors and hedge funds.
MongoDB, Inc, together with its subsidiaries, provides general purpose database platform worldwide. The company provides MongoDB Atlas, a hosted multi-cloud database-as-a-service solution; MongoDB Enterprise Advanced, a commercial database server for enterprise customers to run in the cloud, on-premises, or in a hybrid environment; and Community Server, a free-to-download version of its database, which includes the functionality that developers need to get started with MongoDB.
Featured Articles
Receive News & Ratings for MongoDB Inc Daily – Enter your email address below to receive a concise daily summary of the latest news and analysts’ ratings for MongoDB Inc and related companies with MarketBeat.com’s FREE daily email newsletter.
Article originally posted on mongodb google news. Visit mongodb google news
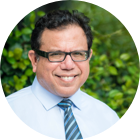
MMS • RSS
Posted on mongodb google news. Visit mongodb google news
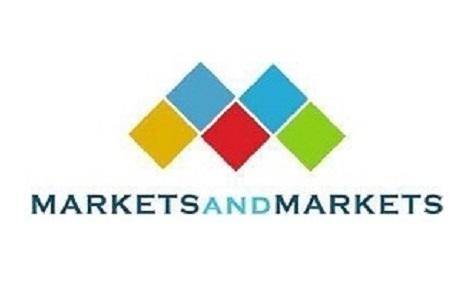
Microsoft (US), Elastic (US), Alibaba Cloud (China), MongoDB (US), Redis (US), SingleStore (US), Zilliz (US), Pinecone (US), Google (US), AWS (US), Milvus (US), Weaviate (Netherlands), and Qdrant (Berlin) Datastax (US), KX (US), GSI Technology (US), Clari
Vector Database Market by Offering (Solutions and Services), Technology (NLP, Computer Vision, and Recommendation Systems), Vertical (Media & Entertainment, IT & ITeS, Healthcare & Life Sciences) and Region – Global Forecast to 2028.
The global vector database market [https://www.marketsandmarkets.com/Market-Reports/vector-database-market-112683895.html?utm_campaign=vectordatabasemarket&utm_source=abnewswire.com&utm_medium=paidpr] is projected to expand from USD 1.5 billion in 2023 to USD 4.3 billion by 2028, reflecting a compound annual growth rate (CAGR) of 23.3%. This growth is being fueled by the rapid advancement of artificial intelligence (AI) and machine learning (ML), a rising demand for real-time data processing, and the increasing adoption of cloud computing technologies.
Download PDF Brochure@ https://www.marketsandmarkets.com/pdfdownloadNew.asp?id=112683895 [https://www.marketsandmarkets.com/pdfdownloadNew.asp?id=112683895&utm_campaign=vectordatabasemarket&utm_source=abnewswire.com&utm_medium=paidpr]
The vector database market is expanding, and vendors are adopting a strategic focus to attract customers. Vector databases are a powerful new technology well-suited for many applications. As the demand for machine learning and AI applications grows, vector databases will likely become even more popular. Vector databases are essential for many machine learning and AI applications, such as natural language processing, image recognition, and fraud detection; this is because vector databases can efficiently store and query large amounts of high-dimensional data, which is the type of data used in machine learning and AI. These services are increasing the demand for the vector database market.
The NLP segment holds the largest market size during the forecast period.
In the Natural Language Processing (NLP) context, the vector database market is a rapidly evolving sector driven by various factors. Vector database is instrumental in NLP applications for efficient storage, retrieval, and querying of high-dimensional vector representations of textual data. In NLP, a vector database is used for tasks like document retrieval, semantic search, sentiment analysis, and chatbots. They help store and search through large text corpora efficiently. Companies like Elasticsearch, Milvus, and Microsoft have been actively serving NLP applications. Many organizations also develop custom solutions using vector databases. The proliferation of text data on the internet and within organizations drives the need for an efficient vector database for text indexing and retrieval. Storing and searching for text embeddings enables content tagging, which is vital for content classification and organization in NLP applications.
The growth of the vector database market in NLP is due to the increasing importance of efficient text data management and retrieval. As NLP plays a significant role in various industries, including healthcare, finance, e-commerce, and content generation, the demand for advanced vector database solutions will persist and evolve. This trend will likely drive further innovations in vector databases, making them increasingly efficient and tailored to NLP-specific needs. NLP-driven applications aim to understand the context and meaning behind text data. Traditional databases may struggle to capture complex semantic relationships between words, phrases, and documents. Vector databases excel in storing and retrieving high-dimensional vector representations of text, which capture semantic relationships; this enables semantic search capabilities, allowing users to find information based on the meaning and context rather than relying solely on keywords.
Semantic search involves finding documents or pieces of text that are semantically similar to a given query. It goes beyond keyword matching and considers the meaning of words and phrases. NLP techniques enable understanding the semantic meaning of words, phrases, and documents. It goes beyond traditional keyword-based search and considers the context and relationships between terms.
Healthcare and Life Sciences vertical to record the highest CAGR during the forecast period.
The healthcare industry vertical is seeing a rise in using vector databases as a valuable tool. It offers medical professionals assistance in various areas, such as diagnosing diseases and creating new drugs. Vector database algorithms learn from vast sets of medical images and patient records, allowing them to detect patterns and anomalies that may go unnoticed by humans; this leads to more accurate and faster diagnoses and personalized treatments for patients. Vector database is used in healthcare, particularly in medical imaging. Generating high-resolution images of organs or tissues aids doctors in detecting early-stage diseases. Additionally, vector databases can assist in identifying new drug candidates for drug discovery by generating virtual molecules and predicting their properties. Furthermore, it can analyze patients’ medical history and predict the efficacy of different treatments, enabling the development of personalized treatment plans.
Our analysis shows North America holds the largest market size during the forecast period.
As per our estimations, North America will hold the most significant market size in the global vector database market in 2023, and this trend will continue. There are several reasons for this, including numerous businesses with advanced IT infrastructure and abundant technical skills. Due to these factors, North America has the highest adoption rate of the vector database. The presence of a growing tech-savvy population, increased internet penetration, and advances in AI have resulted in an enormous usage of vector database solutions. Most of the customers in North America have been leveraging vector databases for application-based activities that include, but are not limited to, text generation, code generation, image generation, and audio/video generation. The rising popularity and higher reach of vector databases are further empowering SMEs and startups in the region to harness vector database technology as a cost-effective and technologically advanced tool for building and promoting business, growing consumer base, and reaching out to a broader audience without a substantial investment into sales and marketing channels. Several global companies providing vector databases are in the US, including Microsoft, Google, Elastic, and Redis. Additionally, enterprises’ increased acceptance of vector database technologies to market their products modernly has been the key factor driving the growth of the vector database market in North America.
Request Sample Pages@ https://www.marketsandmarkets.com/requestsampleNew.asp?id=112683895 [https://www.marketsandmarkets.com/requestsampleNew.asp?id=112683895&utm_campaign=vectordatabasemarket&utm_source=abnewswire.com&utm_medium=paidpr]
Unique Features in the Vector Database Market
Vector databases are specifically designed to handle high-dimensional data, such as feature vectors generated by AI and machine learning models. Unlike traditional databases that manage structured rows and columns, vector databases enable fast similarity search and efficient handling of complex, unstructured data formats like images, audio, text embeddings, and video.
One of the standout features of vector databases is their ability to perform real-time similarity searches using Approximate Nearest Neighbor (ANN) algorithms. This allows applications such as recommendation engines, semantic search, fraud detection, and image recognition to deliver instant and highly accurate results.
Modern vector databases are built for scalability, supporting billions of vectors across distributed environments. With support for parallel computing and hardware acceleration (such as GPU-based processing), these databases maintain low latency and high throughput even as data volume grows.
Vector databases are often designed to work directly within AI/ML ecosystems. They support native integration with model inference engines, data preprocessing tools, and popular ML frameworks like TensorFlow, PyTorch, and Hugging Face, allowing for streamlined development and deployment workflows.
Major Highlights of the Vector Database Market
As artificial intelligence and machine learning continue to proliferate across industries, the need to store, manage, and search high-dimensional vector data has become essential. Vector databases serve as a foundational layer in AI/ML infrastructures, powering functions like recommendation systems, natural language processing, and image recognition.
Use cases requiring real-time, context-aware search capabilities-such as chatbots, intelligent virtual assistants, and fraud detection systems-are on the rise. Vector databases uniquely enable these applications by supporting similarity-based searches that go beyond keyword matching, offering deeper and more intuitive results.
While initially centered around tech giants and research labs, vector databases are now gaining traction in a wide range of industries including healthcare, e-commerce, finance, and media. Organizations are leveraging vector data to enhance personalization, automate decision-making, and extract insights from unstructured content.
The market is witnessing a rise in cloud-native vector databases and open-source solutions, making them more accessible and scalable. Vendors are offering managed services and seamless integration with popular cloud platforms, enabling faster deployment and lower operational overhead.
Inquire Before Buying@ https://www.marketsandmarkets.com/Enquiry_Before_BuyingNew.asp?id=112683895 [https://www.marketsandmarkets.com/Enquiry_Before_BuyingNew.asp?id=112683895&utm_campaign=vectordatabasemarket&utm_source=abnewswire.com&utm_medium=paidpr]
Top Companies in the Vector Database Market
The prominent players across all service types profiled in the vector database market’s study include Microsoft (US), Elastic (US), Alibaba Cloud (China), MongoDB (US), Redis (US), SingleStore (US), Zilliz (US), Pinecone (US), Google (US), AWS (US), Milvus (US), Weaviate (Netherlands), and Qdrant (Berlin) Datastax (US), KX (US), GSI Technology (US), Clarifai (US), Kinetica (US), Rockset (US), Activeloop (US), OpenSearch (US), Vespa (Norway), Marqo AI (Australia), and Clickhouse (US).
Microsoft is a prominent global information technology leader, providing software and diverse licensing suites. The company develops and maintains software, services, devices, and solutions. Its product offerings include Operating Systems (OS), cross-device productivity applications, server applications, business solution applications, desktop and server management tools, software development tools, and video games. The company also designs, manufactures, and sells devices like PCs, tablets, gaming and entertainment consoles, other intelligent devices, and related accessories. It offers a range of services, which include solution support, consulting services, and cloud-based solutions. The company also offers online advertising. Microsoft is a global leader in building analytics platforms and provides production services for the AI-infused intelligent cloud. It generates revenue by licensing and supporting a range of software products. Microsoft caters to various verticals, including finance and insurance, manufacturing and retail, media and entertainment, public sector, healthcare, and IT and telecommunications. It has a geographical presence in more than 190 countries across North America, Asia Pacific, Latin America, the Middle East, and Europe. In November 2020, the company pledged a USD 50 million investment in the ‘AI for Earth’ project to accelerate innovation. As large-scale models become potent platforms, the company continues to bring rich AI capabilities directly into the data stack. In the past year, OpenAI achieved advanced training models such as GPT-3-the world’s largest and most advanced language model-on Azure AI supercomputer. Microsoft exclusively licensed GPT-3, allowing it to leverage its technical innovations to deliver cutting-edge AI solutions for its customers and create new solutions that harness the power of advanced natural language generation.
Alibaba Group operates as an online and mobile commerce company. Alibaba Cloud is a cloud computing arm and a BU of the Alibaba Group. Alibaba Cloud, founded in 2009, has headquarters in Hangzhou, China. It is a publicly held company and operates as a subsidiary of Alibaba Group. It offers cloud computing services, such as database, elastic computing, storage and Content Delivery Network (CDN), large-scale computing, security, and management and application services. Alibaba Cloud provides a comprehensive suite of cloud computing services to power international customers’ online businesses and Alibaba Group’s eCommerce ecosystem. Alibaba Cloud’s global operations are registered and headquartered in Singapore. The company has international teams stationed in Dubai, Frankfurt, Hong Kong, London, New York, Paris, San Mateo, Seoul, Singapore, Sydney, and Tokyo. As of 2019, Alibaba Cloud has 55 availability zones across 19 regions worldwide. AnalyticDB for PostgreSQL provides vector analysis to help implement approximate search and study of unstructured data. AnalyticDB for PostgreSQL vector databases is a DBMS that integrates the in-house FastANN vector engine. AnalyticDB for PostgreSQL vector databases also provides end-to-end database capabilities such as ease of use, transaction processing, high availability, and high scalability.
Elastic, based in the US, is renowned for its Elastic Stack, which includes Elasticsearch, a highly scalable search and analytics engine designed for storing, searching, and analyzing structured and unstructured data in real-time. While Elasticsearch is not a traditional vector database per se, its capabilities in handling large volumes of data with near-instantaneous search and analysis make it relevant in contexts requiring fast retrieval and analysis of vectors or similar data structures. Elastic’s solutions are widely used across industries for logging, security information and event management (SIEM), application performance monitoring (APM), and more, emphasizing flexibility and scalability in data management and analytics.
Weaviate, based in the Netherlands, specializes in providing a scalable and flexible vector database designed specifically for handling large-scale, complex data sets. It leverages a schema-first approach to organize data into structured vector representations, enabling efficient querying and retrieval of complex relationships and patterns within the data. Weaviate’s database is optimized for handling high-dimensional vectors and supports advanced search capabilities, making it suitable for applications requiring real-time analysis, natural language processing (NLP), recommendation systems, and other AI-driven use cases. Their platform emphasizes the integration of machine learning models and IoT devices, facilitating the creation of intelligent, data-driven applications across various domains.
MongoDB, headquartered in the US, is a prominent player in the vector database market. MongoDB offers a robust document-oriented database that supports JSON-like documents with dynamic schemas, making it highly flexible for handling complex data structures and unstructured data. In the vector database market, MongoDB provides features that cater to real-time analytics, high-speed transactions, and scalability across distributed systems. Its capabilities in managing large volumes of data efficiently and its ability to integrate with various programming languages and frameworks position MongoDB as a versatile choice for organizations seeking scalable and performant database solutions in the vector database market.
Media Contact
Company Name: MarketsandMarkets Trademark Research Private Ltd.
Contact Person: Mr. Rohan Salgarkar
Email:Send Email [https://www.abnewswire.com/email_contact_us.php?pr=vector-database-market-worth-43-bn-by-2028-key-companies-include-microsoft-elastic-mongodb-redis-singlestore]
Phone: 18886006441
Address:1615 South Congress Ave. Suite 103, Delray Beach, FL 33445
City: Florida
State: Florida
Country: United States
Website: https://www.marketsandmarkets.com/Market-Reports/vector-database-market-112683895.html
Legal Disclaimer: Information contained on this page is provided by an independent third-party content provider. ABNewswire makes no warranties or responsibility or liability for the accuracy, content, images, videos, licenses, completeness, legality, or reliability of the information contained in this article. If you are affiliated with this article or have any complaints or copyright issues related to this article and would like it to be removed, please contact retract@swscontact.com
This release was published on openPR.
Article originally posted on mongodb google news. Visit mongodb google news
Presentation: Lessons & Best Practices from Leading the Serverless First Journey at CapitalOne
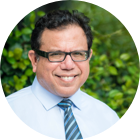
MMS • George Mao
Article originally posted on InfoQ. Visit InfoQ
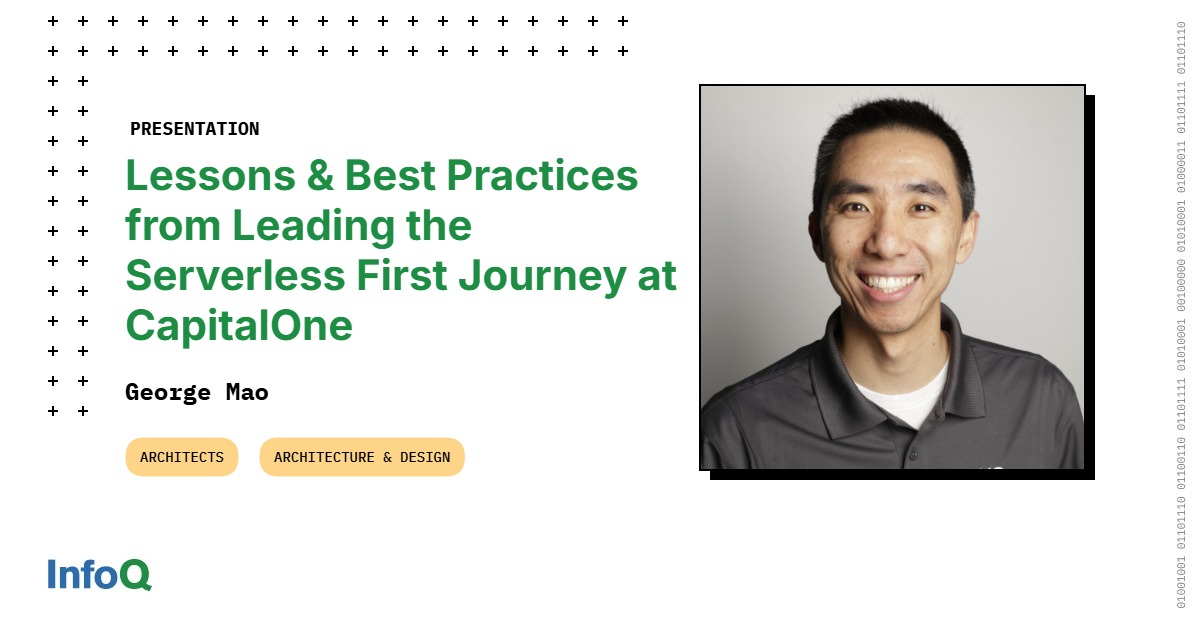
Transcript
Mao: My name is George. I am currently a Senior Distinguished Engineer at Capital One. I lead a lot of our AWS serverless technology implementations. I’m responsible for helping our teams implement best practices and everything we do on AWS. Before I joined Capital One, I was the tech leader at AWS for serverless computing, so I spent a lot of time, basically since the beginning of 2015, where serverless was first created at Amazon.
Capital One is one of the largest banks in the United States. We’re generally somewhere at 10 or 11 in terms of ranking. We’re not that big internationally. We do have a pretty good presence in the UK, but that’s about it. What’s unique about us is we’re mostly structured like a tech organization, so we have about 9,000 software engineers. In 2020, we completed our all-in migration into AWS. As far as I know, I think we’re one of the only major banks in the world that has ever done an all-in like this. Now what we’re trying to do is modernize our entire tech stack running in the cloud. What that means is becoming more cloud-native, taking advantage of all of the AWS managed services, and then becoming more efficient in the cloud.
Outline
This is what we’re going to talk about. I’ll cover why we decided to make this journey. In chapter 2, we’ll talk about some of the lessons that we’ve learned, and I’ll share with you so that you might not run into some of the trouble that we ran into. Then we’ll go through a bunch of best practices that you can take home and implement in your organizations.
Chapter 1: Why Did Capital One Adopt a Serverless-First Approach?
Why did Capital One adopt a serverless-first approach? Many of you are in the financial industry, in banking, or in related industries. Capital One has a ton of regulations and a ton of things that we have to follow to meet our auditing and compliance needs. A lot of that stuff stems from vulnerability assessments, to addressing problems and all kinds of issues that we find that has to be addressed immediately. An example is like, every 60 to 90 days, we have to rehydrate an EC2 instance, regardless of what we’re doing with that instance. By our measurements, on an average team of 5 engineers, that team spends 20% of our time simply working on EC2, delivering things that don’t really add value, but we have to do because of the industry that we’re in. This is basically the gold standard of a traditional architecture that Amazon tells us to implement.
For highly available, you would deploy EC2 instances across multiple availability zones, two at least, at Capital One we do at least three. Then you would just create autoscaling groups so that they can spin up and down as they need. The goal here is to allow Amazon to handle the scaling of your instances based on metrics or failure. Then you have load balancers and NAT gateways ahead of them so that they can front your traffic and then spread load across your clusters. When you have an environment like this, think about the things that you have to maintain. This is just a small list. We have to maintain the EC2 infrastructure, the networking behind it, all the IP addresses, the VPC subnets, the AMIs that go on to the instances, updates, patches, scaling policies, everything that is in that picture, some engineer has to touch. What you’ll notice is none of this stuff adds any value to your customers. All of this is basic needs that you have to deliver to make your applications work in a traditional architecture.
Pre-serverless, our responsibility looked like this. We would deploy stuff to the cloud, and then we’d deploy infrastructure to AWS, and what that really means is EC2 compute. We’d choose operating systems that go on the EC2 instances. Then, generally, we containerize our applications. I think that’s becoming the standard these days. Then we run app servers on these containers. This is a tried-and-true method that most enterprises run today. Then we deploy our business apps that run on top of them. When you go to capitalone.com, all of the stuff that the customers see go top-down through this stack. Everything below business apps is what we call run-the-engine tasks, so things that are necessary behind the scenes to even begin deploying applications on top. If you talk to AWS, they’ll use a term called undifferentiated heavy lifting.
If anybody has spoken to AWS people, they like to say that a lot. It’s basically things that your developers hate doing. I don’t know how to do any of this stuff. I know how to write app code. I’m not a EC2 engineer. When you move into serverless, your architectures generally are event-based, and they really become one of three types. Synchronous, an example would be, you create a REST API. Requests come through API Gateway, and then API Gateway drives requests to your Lambda functions. An example would be, maybe you have an order submitted on your website, and that’s an event, but that’s a synchronous event because it needs to return an order ID to your customer who is waiting for that confirmation. If you can do asynchronous workloads, that’s even better, because then you can decouple the work that’s happening at the frontend with what’s happening at the backend. Has anybody purchased something from amazon.com before? I have a package arriving every other day or something at my garage.
All the orders are asynchronous. You click order, your credit card isn’t charged immediately, they have an order processing system. They can take hundreds and millions of orders without even having a system up on the backend that’s processing them. It’s decoupled and asynchronous. That’s actually the best way to write serverless applications. The last piece is poll-based. One of the best and unknown features of AWS is they have something called a poller system. A poller system is their fleet of workers that will poll certain event sources on your behalf and deliver records from those event sources to your Lambda functions. You don’t have to do any of that work. Examples are DynamoDB, Kinesis, SQS, anything that’s in those data sources, AWS will poll and deliver to you. That removes all of the scaling and the need that you have to do in order to process those events.
If you look at serverless architectures, generally, all of that stuff at the bottom is just handled by AWS. We don’t have to do any of that stuff. We just decide, do we want to run Lambda, which is Functions as a Service, or Fargate, which is Containers as a Service. Then, we just write our business logic right on top of that. Our engineers are basically only working with that top box. The first thing they do is write application code. They don’t have to worry about patching and operating systems and all that stuff. Engineers love this. Our developers really like this type of development. That means there’s no more burden on our developers. All of that time spent doing all those EC2 activities are just entirely gone. We all know that human costs are generally the most expensive piece of any application team. That’s why we moved into serverless. Today, we are trying to be serverless first, everywhere, where possible. That’s our goal. We’re still pushing forward into that space.
Chapter 2: Lessons Learned, and the Launch of Our Serverless Center of Excellence
We’ve learned a lot of lessons, and I’ll share some with you, so that if you’re doing this exercise, you won’t run into some of the challenges that we learned. There is going to be a learning curve. A beginner serverless developer generally will write Lambda functions in the console. Who’s done this before? You can write app code directly in the console. It’s really cool because you can save it and execute that function immediately. The bad news is there’s no CI/CD, and this goes right out to production if it’s there, and you can change it at any time without any version control. You also can’t debug or trace a Lambda function in the console.
For those who have worked on Lambda, there is no way to debug or trace. What do you do? Basically, you write print statements everywhere. Don’t copy this code and put it into production, but all it’s doing is writing print statements so that I can see the value of these variables that I have. Back when Lambda was first released, this was the only way to test functions. Everybody did this because there was no other way to test functions. Today, there’s a tool called SAM. It’s the Serverless Application Model. It comes in two pieces. One is the CLI, which you install locally on your machine. What that will do is you’ll basically install an image of the Lambda container on your machine as a Docker image. This will allow you to run your Lambda functions locally exactly as it would be in the AWS environment. That means you’ll see log generation. You’ll see exactly the same thing you would see if you ran it live in AWS.
Second, you can use SAM to perform your CI/CD deployment. It’ll do deploys. It’ll do code synchronization. It’ll do everything that you can do to push it through your development stack. If anybody has used CloudFormation, it’s pretty verbose. You can have a 50-page template for your application. That’s not great. What Amazon has done is they’ve created a shorthand syntax for serverless components that make it a lot more concise. Here’s an example. I’m writing two Lambda functions. First one is called FooFunction. Second one is called BarFunction. They’re both Node.js based 16, both memory size 128. Entry points are defined by the handler property. Just with five lines of code for each function, this will deploy into AWS without a massive CloudFormation template. The backend AWS is translating this to a real CFT, CloudFormation. You don’t have to worry about any of that translation. We use this everywhere. I encourage all of our engineers to move to this method because you can test applications really easily.
The next thing that was new for us is that the unit of scale for Lambda is concurrency. That’s a brand-new concept to almost everybody touching serverless. The traditional unit of scale is TPS, RPS, transactions per second, requests per second. That drives how wide you need to scale your EC2 cluster. With Lambda, it’s a little bit different. Concurrency is the number of in-flight requests that your Lambda functions are processing at any given second. Lambda only bills us when we run them. If you’re not running anything, there’s no cost. That’s really cool. What that means is when you’re not running anything, there are no environments available to run your functions. The very first time you have to run your function, it goes through something called a cold start.
A cold start is all of the work Amazon has to do to bring your code into memory, initialize the runtime, and then execute your code. That pink box right there is all of the overhead that’s going to happen before your function can begin executing. Once your function’s warm, the second time it’s invoked, it doesn’t have to go through that method. It’s going to be warm, and that’s what Amazon people will talk to you about as warm starts. The second invoke is going to be really fast. This will drive your concurrency across your entire fleet of Lambda functions. You could have 1,000 concurrent functions that you need to scale to 2,000. All of those new containers are going to go through this cold start. Keep that in mind. That’s usually the first thing Lambda engineers run into. I talk about this formula all the time with our engineers. This is the formula that Amazon uses to measure concurrency, and it’s average requests per second, TPS, driven to Lambda, multiplied by the average duration in seconds.
If you look at these three examples here, we’re all driving 100 TPS, RPS. These Lambda functions run at about half a second, so 500 milliseconds. That means your concurrency needs are going to be 50. It actually drives down your concurrency needs because you’re running for under a second. If you double your duration to 1 full second, your concurrency now is going to be 100. If you double that again, same TPS, but now you’re running for 2 seconds, your concurrency needs are 200. You’re going to need 200 warm containers serving all of this traffic, and you have to be able to scale into that. This is a concept that you’ll likely have to work through as you walk into your serverless journey.
The next thing here is, before we ran into serverless, or started working on serverless, our infrastructure costs were generally managed by our infrastructure team, and our developers were not really concerned with cost. With Lambda, everybody is responsible for cost. At re:Invent 2023, one of the 10 top tenets that Amazon gave us was, everybody is responsible for cost, and that’s 100% true when you move into serverless. Lambda has two pricing components.
First is number of invocations per month, and it’s tiny, it’s 20 cents per million. We don’t even look at this. This first component of the formula, we just ignore, because it’s basically dollars. The second is compute. If compute is measured in gigabyte seconds, and that sounds complicated, but gigabyte seconds is the combination of memory allocated to your function multiplied by the duration that function runs for. Memory allocated in megabytes times the milliseconds that that function runs for. The bottom line there is just focus on the compute cost. The number of invocations is relevant. You can run 1 million invokes for free on every account forever. If you’re under that, you could run Lambda for very cheap. Going along the same thing that we learned is every Lambda function operates and generates a report structure in CloudWatch logs every single time it’s invoked. There’s always going to be a start, always going to be an end, and always going to be a report line. The report line is the most important line that you should be aware of.
What you’re going to see there is, at the bottom in the report line, they’ll give you all of the metrics that you need to understand how your function executed. One of the most important ones is duration. This function ran for, it’s a little bit small, but 7.3 milliseconds. It was billed for 8 milliseconds. Anybody know why? Lambda rounds us up to the nearest 1 millisecond. It’s the most granular service that AWS, or I think any cloud provider offers. Everybody else is either at 1 second or 100 milliseconds. This really represents pay-for-use. It’s the best service that we can find that’s pay-for-use. I configured this function at 256 megs, max memory used is 91 megs. Remember, Amazon bills us at memory configured, not used. This is a piece of confusion that my engineers run into a lot. It doesn’t matter if you use 1 out of a gig, Amazon’s going to bill you for a gig of memory. We’ll get into that. Sometimes there’s a great reason for why you might overprovision memory.
Capital One, we operate thousands of accounts. We have over 1000 accounts. We have tens of thousands of Lambda functions spread out across those accounts, which means we have to be able to handle compliance. We have to be able to control these functions. We have to have standards so we can do these things. Metrics and logs, we have to understand how long to save them for and be able to maintain these functions.
In order to do that, we learned that we needed to create a center of excellence because what we were doing before was, we were making isolated decisions across single lines of businesses that would affect other lines of businesses. That creates tech debt and it creates decisions that have to be unrolled. We created a center of excellence and now we use it to basically talk to all of our representatives in each line of business that we can make a correct decision. I’ll talk through some examples that we’ve worked on.
Some of the sample things that our center of excellence leads is everything from Lambda defaults. What should a Lambda default be? What are the programming languages that we even allow? What are their naming conventions or the default memory settings that we’re going to choose? AWS regularly deprecates runtimes because Java 8 is deprecated. They don’t want to support Java 8. We also talk about how we want to deprecate our runtimes because if we wait too long and Amazon’s deprecated theirs, we’re not going to be able to deploy on these deprecated runtimes anymore. The center of excellence also handles something really important, which is training and enablement. We host a serverless tech summit twice a year. We have internal certifications on serverless. We have continuous enablement, and to educate our engineers on a regular basis.
Here’s an example of a development standard. You can create an alias that points to a Lambda function, and that alias is just like a pointer. You can use that to invoke your function. We mandate that every development team uses a standard alias called LIVE_TRAFFIC. That is the only entry point for my function. What this does is it allows me to jump across any development team and understand where this function is executed from and what all the permissions are. I work across every dev team that exists at Capital One, and this helps me a lot. Many other people could be transitioning from one team to another and they can easily onboard really quickly. Another thing that we standardize is we require versioned rollouts for all Lambda functions so that we can roll back if there’s a problem. We require encryption on our environment variables. We don’t want to have sensitive data exposed in environment variables.
Other thing is, if you’re working at AWS, you can tag nearly every resource out there. It’s just a key-value pair to give you some metadata. Basically, we have a set of standardized tags that will help us understand who owns this application, who to contact if there’s a problem, and who gets paged, essentially. Some other things here, IAM, we have some standardized rules on IAM and what you can and can’t do. Mostly is with no wildcards anywhere in your IAM policies.
Then, we have open-sourced an auditing tool called Cloud Custodian. It’s just cloudcustodian.io, but we actually use this to audit all of these rules that we’re putting in place. If anybody deploys anything that doesn’t meet these standards, it immediately gets caught. Also, I highly encourage you to use multi-account strategies. What we do is we deploy an account per application group. Then, we give that application group multiple accounts representing each development tier, so dev all the way through pod. What that allows you to do is separate blast radius, but also give you separate limits, AWS limits on every account.
Chapter 3: Best Practices for All – Development Best Practices
We’re going to talk about best practices that I’ve learned throughout 10 years of working with serverless. We’ll start with development best practices. Here’s a piece of sample code. Basically, the concept here is, don’t load code until you need it, so lazy load when you can. If you look at the top here, the very first line, that is a static load of the AWS SDK, just the DynamoDB client, and it’s just some SDKs allowing me to list tables in my account. It’s going to do that on every single invocation of this function, but if you look at the handler method, down below, there are two code paths. The first code path actually will use this SDK. It’s going to do this interaction with Dynamo. The second code path isn’t going to do anything with Dynamo.
However, on every invoke of this function, any cold start is going to load in this SDK. 50% of my invocations, in this case, are going to go through a longer cold start because I’m pulling in bigger libraries and more things than I need. What you can do, a really good strategy is lazy load. In the same example, if you define the same variables up ahead in the global scope, but you don’t initialize them, down in the handler method, you can initialize those SDKs only when you need them, so on the first code path, right there, that first if statement. What you need to do is you need to check if those variables are initialized already.
If they’re already initialized, don’t do it again. This is going to avoid extra initialization, and 50% of the time, it’s going to go to the second code path. You need to look at the profile and anatomy of your function and see what code path your applications are following. If you have anything that has separate paths like this, I highly encourage you to lazy load what you can, not just the SDK, but anything else that you might be using as dependencies.
Next concept is, use the right AWS SDK. If you look at Java, the version 1 of the Java SDK was created before Lambda was even in existence. What that meant was the SDK team had no idea that they needed to optimize for Lambda. That SDK is 30-plus megs. If you were to use the version 1 Java SDK, you’re going to have 30 megs of dependencies. Use all of the latest SDKs for Java. You want to use version 2. It allows you to modularize and only pull in the pieces that you need. Same thing with Node. For those who are using Python, you’re lucky. They do upgrade in place on Boto3, so you don’t have to do anything. We continue to use Boto3. Next thing here is, try to upgrade to the latest runtimes for Lambda. Because what Amazon does is they will upgrade the images they use behind the scenes. What you’ll notice is the latest runtimes for Lambda, so Node 20, Java 21, and Python 3.12 and beyond, use what Amazon calls Amazon Linux 2023. That image is only 40 megs. Everything else before uses AL2, Amazon Linux 2, which is 120 megs.
Behind the scenes, it’s just a lot more efficient. You’re going to cold start better, perform a lot better. Then, I know you guys have Java 8 running around everywhere. We did. We still do. If you can get out of it, simply moving from Java 8 to Java 17 gives you a 15% to 20% performance boost. That’s a free upgrade if you can get there. Next is just import what you need. Don’t import extra things like documentation and sample code and extra libraries, because in AWS, when you’re running these, they’re not going to be useful. You’re not going to be able to read them.
An example here, this is a Node package.json. I accidentally imported mocha, which is my test suite, and esbuild. None of those things are going to be useful when I’m running my Lambda function. All they’re going to do is add to the package size. Lambda actually has a package size limit. You can only deploy a 50-meg zip or 250 megs uncompressed. If you have too many libraries, you’re going to run into this limit and you’re not going to be able to deploy.
One of Gregor Hohpe’s main concepts is always to use AWS configuration and integration instead of writing your own code where possible. Think about this piece of architecture where if your Lambda function needs to write a record to Dynamo, and then there’s some other resource waiting to process that record, we could do it like this, where the Lambda function first writes to Dynamo, it waits for the committed response, and then it publishes a notification to SNS or SQS telling the downstream service that, ok, we’re done and we’re ready to process.
Then that downstream service may live on Lambda or EC2, wherever, and then it goes and queries the Dynamo table and processes the work. This is a fully functional app, it’ll work, but we can do better. What I would do is take advantage of out-of-the-box AWS features. You can write to Dynamo, and then within Dynamo, there’s a feature called DynamoDB Streams, and it’s basically a stream of changes that have happened on that table. You can set up Lambda to listen to that stream, so you don’t even have to poll the stream. All you’re really doing in this example is two Lambda functions: one is writing, one is receiving events. You’re not even polling. These will be cheaper, faster, easy to scale. In general, think about your application architectures and try to move towards this type of architecture. Use Lambda to transform data, not to move data. That’s the key principle that we have.
Then, the last development tip I have here is establish and reuse. Objects that are going to be used more than once should only be loaded once, globally. Every Lambda function has an entry point, and it’s called the handler method, right there in the middle. Everything outside of that is global scope. During a cold start, everything above that will be executed. During a warm start, entry point begins right at the handler method. All of the global scope stuff is held in memory and ready to go. A lot of times, we have to pull secrets in order to hit some downstream system. Secrets don’t change that often. What you can do is load it once during global scope and reuse it every time you get warm invokes down below. Just make sure you’re checking to see if that warm secret is available, not expired, and ok to use. You can use the same concept for pretty much anything that can be reused across Lambda invocations.
Build and Deploy Tricks
Next part is some tips on how to build and deploy your Lambda functions. We talked a little bit about this. Make sure you’re deploying small packages, as small as possible. Minify, optimize, and remove everything that you don’t need. Here’s a Node-based Lambda function. It’s written in SAM, which we talked about earlier. The name is called first function. It’s pretty basic. It’s just a Node.js function, memory size 256, and it’s using something called arm64 as the application, the CPU architecture. We’ll talk a little bit about that. This is a strategy for how I can build a really optimized function. I’m using esbuild.
For those who are doing Node stuff, esbuild is a very common build process. Once I use esbuild, it will create and generate a single minified file for deployment, and it will combine all dependencies and all source code into a single file. It’s not going to be human-readable, which doesn’t really matter, because you can’t debug in production anyways. I’m formatting as an es module, and then I’m just outputting into the esbuild. When I do an esbuild, this function is 3.3 megs in size. It’s got the AWS SDK in it, and it’s tiny. If I don’t do esbuild, it’s a 24-meg package, a standard zip. This is zipped and compressed with the AWS SDK. I have almost no source code in this, 24 megs. The largest I can get to is 50, so I’m already almost halfway there just because I included the AWS SDK. If we look at performance, this is a screenshot of a service called AWS X-Ray. X-Ray gives me a trace of the entire lifecycle of my function’s invocation. You can see it topped it down.
The first line is initialization, and that’s the cold start time my function took to really become ready to run. This is my esbuild function, and it took 735 milliseconds to cold start. The actual runtime was 1.2 seconds, so 1.2 minus 735 milliseconds is the actual invocation of my function. If we look at my standard zip file build for that function, it was at over 1,000 milliseconds, so 300 milliseconds slower. That’s basically 40% faster because I used esbuild, simply by changing the method of build for my application. This type of optimization exists for pretty much every language out there, but Node is my default programming language, so this is the example that I have. Next thing is, remove stuff that you don’t need or turn off things that you don’t want.
In Java, Java has a two-tier compilation process. By default, it’s going to go through both tiers. Tier one is standard compilation, tier two is optimization. Lambda functions are not generally living that long for tier two to have any good effect. You can just turn it off. There’s an environment variable called JAVA_TOOL_OPTIONS. You can set this and it’ll turn it off. I think 90% of the time, you’ll see cold start performance improvements when you do this.
Optimize Everything
Optimize, so memory allocation controls CPU allocation. What that means is, there’s a direct proportional relationship between memory and CPU. If you notice, you can’t specify CPU on your lambda function, only memory. If you have a 256-meg function, if you were to drop that to 128, that cuts your CPU allocation in half. Same thing, 512. If you were to double that to a gig, you get double the CPU power. Think about this. If you double your memory for your functions, can you run twice as fast in all scenarios? Is that fact or fiction? The answer is, it depends. Depends on your code and depends on if you’ve multithreaded your code to take advantage of the number of vCPUs Amazon gives you. It’s all dependent on the use case. The principle here is, you must test your application.
The best way to do that, that I found, is using Lambda Power Tuner. It’s an open-source tool. It’s going to generate a graph by creating multiple versions of your Lambda function at many different memory settings, and it’ll show you exactly which one is the best. Red line here represents performance. Basically, invocation time, lower the better. Blue line represents cost. Also, lower the better, but we’ll walk through this.
At 256 megs, we can see the cost is ok, pretty low, but performance is really bad, upwards of 10,000 milliseconds. If we move this function to 512, you can see cost actually drops a little bit, but performance increases drastically, time drops by a factor of two. If we continue increasing to 1 gig, we see more performance improvements, almost at no cost. Go to 1.5 gigs, we start seeing some increase in the invocation cost, and then past that, we’re basically wasting money. Every single Lambda function is going to perform differently based on your use case, based on your memory, based on your runtime. Make sure you’re running this code against your functions as you go through your QA and performance tests.
Billing basics. Lambda pricing, remember this, the formula is always memory configured times the duration it runs for. If you look at this chart here, it’s very interesting. We have three lambda functions, all running 1 million invokes per month, 128 megs for the first one, if it runs for 1,000 milliseconds, it’s going to cost you $2.28. That same function bumped up to 256 megs, if it were to run twice as fast, it’s going to cost you the exact same amount. However, if you bump it to 512, so you 4x the memory, you don’t improve performance, so back in that chart that we saw, it’s going to get a 4x increase in cost. Anytime you’re thinking about performance and cost tradeoff, it’s a direct proportional relationship on both sides of this formula. We talked a little bit about ARM. ARM is that little chip that’s in all of our mobile phones. It’s faster. It’s more cost efficient. It’s more power efficient. It’s going to be cheaper, 20% generally from AWS. Try to move to ARM if you can. It’s free to move, it doesn’t cost us anything.
Then, logs cost money. Logs are pretty expensive. They’re 50 cents to ingest, 3 cents to store. You get charged every month, forever. I’ve seen applications where logging costs more than the Lambda compute itself. When somebody from finance finds that, that’s generally not a fun conversation. Reduce logging when you can. Think about swapping to infrequent access, which reduces cost by 50%. There’s a tradeoff, you won’t be able to do live subscription features on those logs. You can set a retention policy as well. You can age out these logs as you need to based on your data retention policy. I like to use this as a guide here between levels of environments. That way, you don’t have logs around too long.
Observe Everything
The last area we’re going to talk about is observability. If you’re in AWS, there are tons of metrics out there and it really gets confusing. One of the most important ones at the account level is a metric called ClaimedAccountConcurrency. This is really just the sum of all possible Lambda configurations that are actively using concurrency in your account. By default, AWS will only give you 1,000 concurrent Lambda functions as a cap. It’s a soft cap. You can ask for more. Your goal here is to create an alarm off of this metric so that your SRE team can be warned when you’re approaching that hard cap, or the soft cap, which you can lift if you start approaching that.
Next thing here is, at a function level, we talked about Lambda operating a poller and then delivering those records to your functions on your behalf. There’s no metric that AWS gives us for that. I don’t know why there isn’t, but they don’t give us a metric. If SQS is delivering 5, 10, 20, 100 messages per second to your function, there’s no way for you to tell how many you’re getting. Make sure you create a metric on your own. What I would do is use Lambda Powertools for that. It’s a free SDK, open source. Here’s an example in Node on how to do that. It’s really easy. You can use something called the EMF format, which is the embedded metric format. It looks just like that. That’s the EMF format. It writes a JSON log into CloudWatch logs, which gets auto-ingested by AWS, and creates that metric for you.
That’s basically the cheapest way to create metrics. It’s much cheaper than doing PutMetricData calls. Those are really expensive calls. Try to avoid that API call at all costs. It’s really cool because it’s all asynchronous. There’s no impact on your Lambda performance.
Then these are the top things that we’ve put together that have caused us a lot of pain. Just be careful about setting maximum configurations for your Lambda functions. Usually, that results in high bills. You want to set lower configs. You want your functions to error and timeout, rather than allowing them to expand to the largest possible setting. Number two, don’t PutMetricData. That’s really expensive. Number three is, there’s a mode called provisioned concurrency. You can actually tell AWS to warm your functions up when you need them and keep them warm. The downside is, if you do that, it’s going to cost you money if you don’t use that concurrency. Be careful about setting that too high and be careful about setting the provisioned concurrency equal to anything that’s close to your account concurrency because that will cause other functions to brown out. Then, just think through the rest here.
The very last one I’ll talk a little bit about, which is, don’t use the wrong CPU architecture. Back when we talked about moving to ARM, not every workload performs better on ARM. If you think about your mobile phones, we can watch videos, we can send messages, and those cost no power. If you go on your computer, desktop at home, and you watch some YouTube video, it consumes a gigantic amount of power because it’s running on an x86 architecture. Your use case will have a heavy impact on the right CPU architecture. Use the right libraries compiled for the right CPU architecture. A lot of us are doing, like compression is a good example, or image manipulation. All of those libraries have compilation libraries for ARM and x86, and make sure you’re using the right one for the right place.
Questions and Answers
Participant 1: What’s the incentive for Amazon to provide decent performance? If the metric is time times memory, then why wouldn’t they just give all the serverless all the cheap rubbish CPUs that don’t perform very well?
Mao: If you think about how Lambda functions work, they’re not magic. Behind the scenes, when you want to invoke a Lambda function, that function has to be placed on an EC2 instance somewhere. What Amazon wants to do is optimize the placement of that container in their EC2 fleet so that they can optimize the usage of a single EC2 instance. If you think about an EC2 instance, it may have 3 gigs of memory. If I have a 1 gig function that I run for a long amount of time, and you’re not doing anything else, I might get placed on that 3-gig instance, and the rest of that instance is empty. That’s extremely wasteful for AWS. They don’t want to do that. What they actually want to do is they want to pack that instance as much as possible so that they can have high utilization and then pass on the EC2 savings to the rest of AWS. They’re incentivized for us to improve performance.
The worst-case scenario for them is I create a Lambda function and I run it once and never again, because they have to allocate that environment, and based on your memory setting, they have to decide what to do. There’s a gigantic data science team behind the scenes at Amazon that’s handling all of this. I don’t know the details anymore, but that’s what they’re paid to do.
Participant 2: Can you talk more about how Capital One does automated testing with so many Lambdas? You mentioned you use, I think it was called SAM. Do you use that in your CI pipelines as well for testing?
Mao: Every release that goes out there, basically every merge or commit into main ends up running our entire test suite and we use SAM to do most of that stuff. SAM is integrated right into our pipeline, so it executes all of our unit tests and acceptance tests right in the pipeline. We customize all of it to work for SAM, but at the beginning, none of this existed, because EC2 doesn’t have any of this. We had to upgrade our entire pipeline suite to handle all of that.
Participant 3: Lambda functions now can support containers and it has way higher resources, you can have bigger container images. My question is about performance, especially cold starts. Have you tested using containers for Lambda functions and did it have any implication on the performance and especially cold starts?
Mao: Remember I said Lambda functions are packaged as zip files, 50-meg zip, 250 uncompressed. There’s a secondary packaging mechanism called just containers. You can package your function as a Docker image, that allows you to get to 10 gig functions if you need to have a lot of dependencies. I don’t recommend defaulting to that because there are a lot of caveats once you go there. You lose a lot of features with Lambda.
For example, you can’t do Lambda layers. Behind the scenes, it’s a packaging format. It’s not an execution format. What AWS is doing is they’re taking that container and they’re extracting the contents of it, loading it into the Lambda environment and running that, just like your zip is run. You’re not really getting any of the benefits of a container and you’re going to end up with container vulnerabilities. I recommend just using it if you have a large use case where you can’t fit under 50 or 250 megabytes. Generally, I see that when you’re doing like large AI, ML models that can’t fit in the 50-meg package or you just have a lot of libraries that all get put together, so like if you’re talking to a relational database, Oracle, you might be talking to Snowflake, and just a ton of libraries you need that can’t fit. I recommend just stay with zip if you can. If you can’t, then look at containers.
Participant 4: Following up on the testing question from earlier. Lambda function tends to be almost like an analogy of a Unix tool, a small unit of work. It might talk to a Dynamo SNS, SQS. One of the challenges I’ve at least encountered is that it’s hard to mock all of that out. As far as I know, SAM doesn’t mock the whole AWS ecosystem. There are tools that can try to do that, like LocalStack. How do you do local development at Capital One given so many integrations with other services?
Mao: I get this question from our engineers all the time. SAM only mocks three services, I think. It’s Lambda itself. It’s the API Gateway, which is the REST endpoint. It can integrate with Step Functions local and DynamoDB local. Everything else, if you’re doing SQS or SNS, it cannot simulate locally. AWS is not interested in investing more effort in adding more simulation. LocalStack is an option. If you use LocalStack, you can stand up, basically, mocks of all of these services. What you’re going to have to do on the SAM side is configure the endpoints so they’re talking to local endpoints, all of it. What I usually recommend our team do is, SAM actually has an ability to generate payload events for almost every AWS service. You can do sam local generate, and then there’s a SQS and then the event type.
Then you can invoke your function using that payload that it generates. Then you can simulate what it would look like if you were to get a real event from one of those sources. That’s usually the best place to start. LocalStack is good as well. We actually just test integrating into development, so like your local SAM might talk to a development SQS resource. That’s really the best way to test.
Ellis: You’ve done a lot already. What’s on your to-do list? What’s the most interesting thing that you think you’re going to get to in the next year?
Mao: Right now, we’ve been focused on compute, like moving our compute away from EC2. I think the next is data. Our data platforms, we do a lot of ETL. I think everybody does a lot of ETL. We use a lot of EMR. We’d like to move away from that. EMR is one of the most expensive services that you can put into production at AWS. You pay for EC2, you pay for the EMR service, and then you pay for your own staff to manage that whole thing. We want to move to more managed services in general, so like Glue, and other things that don’t require management of EC2. I think data transformation or data modernization is definitely big.
See more presentations with transcripts
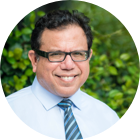
MMS • Craig Risi
Article originally posted on InfoQ. Visit InfoQ
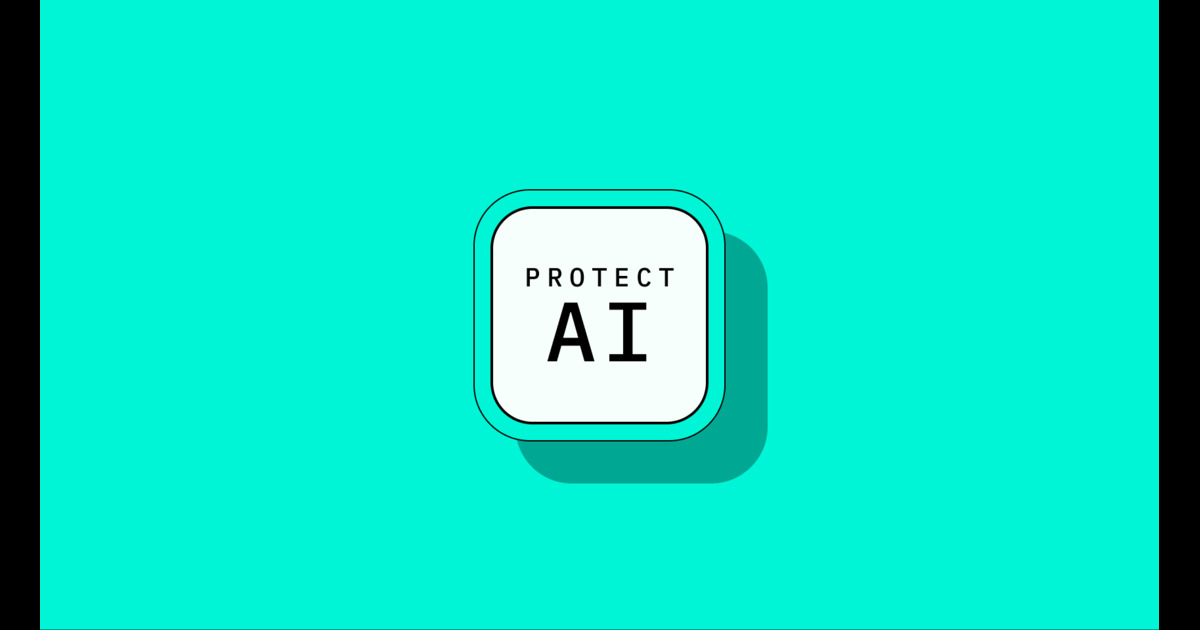
Edera has announced the general availability of Edera Protect 1.0, a Kubernetes security solution designed to enhance container isolation and address longstanding security challenges in cloud-native environments. Unlike traditional container security tools that focus on post-deployment detection, Edera Protect introduces a “zone”-based architecture, providing strong isolation between containers by default. This approach aims to eliminate entire classes of threats, such as container escapes and credential theft, by re-architecting the standard container runtime.
Edera Protect integrates with existing Kubernetes infrastructure, allowing organizations to enhance their security posture without disrupting developer workflows. In the general availability release of Edera Protect 1.0, several technical enhancements have been introduced to support secure, scalable container isolation in Kubernetes environments. One of the most significant changes is improved scalability: the system now supports over 250 secure zones per node on hardware with 64 GB of RAM. This advancement enables denser multi-tenant workloads, a common requirement in enterprise Kubernetes clusters.
A key improvement in resource management comes with the introduction of memory ballooning. This feature allows zones to dynamically adjust their memory allocation based on real-time demand, helping reduce resource overprovisioning while maintaining strong isolation boundaries. To address performance concerns around container startup times, warm zones were introduced. This capability should reduce the time it takes to spin up containers, bringing performance levels closer to what teams expect from native Docker environments.
The release also broadens platform compatibility. Amazon Linux 2023 is now supported, and integration with the Cilium Container Network Interface (CNI) allows users to combine Edera’s security architecture with existing advanced networking and observability tools. These integrations aim to support a wider range of infrastructure setups without requiring major changes to existing environments.
The 1.0 release includes Prometheus metrics and health endpoints, making it easier for teams to monitor zone health, resource usage, and system behavior. Additionally, a Terraform module has been introduced for Amazon EKS, simplifying the process of deploying Edera Protect into AWS-based Kubernetes clusters.
The release of Edera Protect 1.0 represents a step towards addressing the inherent tension between platform velocity and security in Kubernetes environments. By providing strong isolation at the architectural level, Edera aims to reduce the reliance on complex, layered security tools and enable organizations to run secure, multi-tenant workloads more efficiently.
Looking ahead, Edera has said they plan to expand the capabilities of Protect by introducing support for defining security boundaries at the Kubernetes namespace layer and deeper integration with cloud provider security features. This continued development underscores Edera’s commitment to enhancing container security and supporting the evolving needs of cloud-native organizations.
Inflection Points in Engineering Productivity for Improving Productivity and Operational Excellence
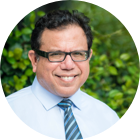
MMS • Ben Linders
Article originally posted on InfoQ. Visit InfoQ
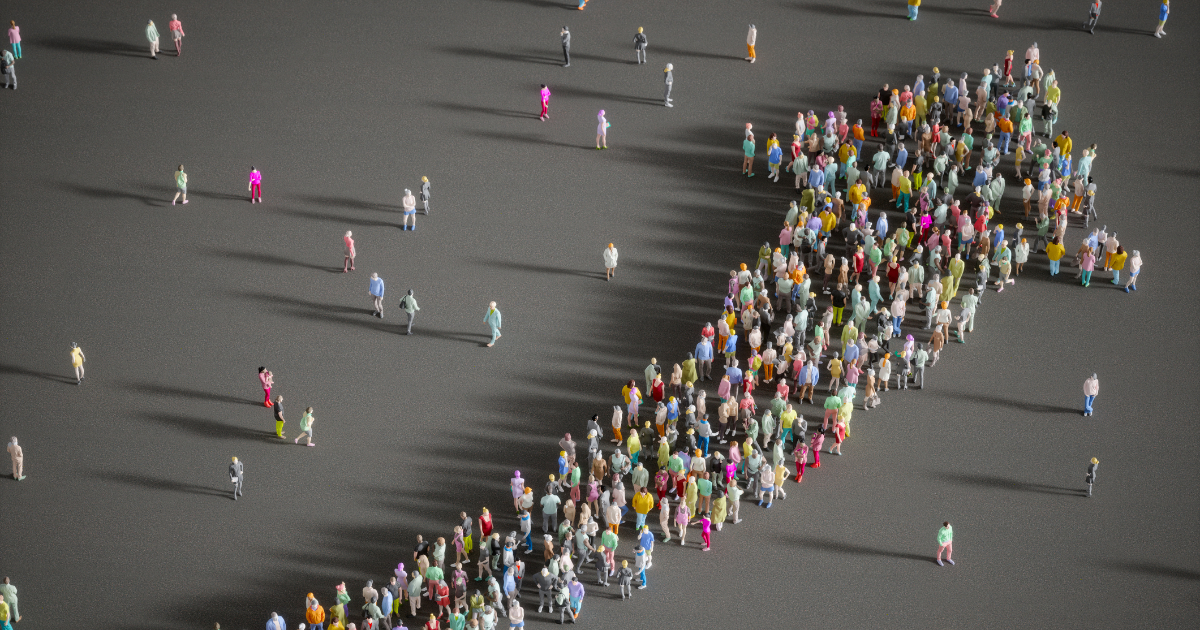
As a company grows, investing in custom developer tools may become necessary. Initially, standard tools suffice, but as the company scales in engineers, maturity, and complexity, industry tools may no longer meet needs, Carlos Arguelles said at QCon San Francisco. Inflection points, such as a crisis, hyper-growth, or reaching a new market, often trigger these investments, providing opportunities for improving productivity and operational excellence.
Carlos Arguelles gave a talk about Amazon’s inflection points in engineering productivity. When a company first starts, it doesn’t make sense for it to create its own developer tools, as there are plenty of excellent ones available in the industry, he said. But as a company grows (in number of engineers, in maturity, in customer adoption, in domains), investing in its own developer tools starts making sense:
An inflection point is when that investment in engineering productivity that didn’t make sense before now suddenly does. This could be because the industry tools do not scale, or because the internal tools can be optimized to integrate better with the rest of the ecosystem, as Arguelles explained.
A little papercut where each developer is wasting a couple of minutes per day in toil can add to hundreds of millions of dollars of lost productivity in a company like Amazon or Google.
The more obvious inflection point that made investments in engineering productivity become feasible is the number of engineers. Maybe it didn’t make sense for your company to have its own CI/CD proprietary tooling when there were 3000 engineers, but it does when there are 10,000 engineers, because the savings in developer productivity with a toolchain optimized for the ecosystem have a significant return on investment, Arguelles said.
He mentioned that the opposite is true as well. When your company is in hypergrowth it may make sense to have duplicate tools (each organization creating its bespoke tool so that it can independently move fast), but when the company stops growing (which is what happened in 2023 with all the big tech layoffs), it makes sense to consolidate tooling and defragment the world.
Arguelles gave some more examples of inflection points, like reaching a certain level of maturity where you need to raise the bar in terms of engineering or operational excellence can provide an inflection point or entering an entirely different and new market. Sometimes the inflection point is a crisis or even a single operational issue that could have been prevented with the right tooling:
For example, Amazon invested significantly in a number of load, stress, and chaos testing tools after the Prime Day incident of 2018 (where the Amazon Store was unavailable for hours during the busiest shopping day of the year). We had been talking about doing that for years, but that incident helped us sharpen our focus and build a solid case for funding those investments.
Inflections can also happen when an organization experiences hyper-growth:
I saw Amazon double in size every year, from 3000 engineers when I started in 2009, to 60k-70k in 2022. What this meant in practice is that we needed to be thinking about skating to where the puck was going to be, not where it currently was.
Scaling needs and security needs often meant sooner or later we needed to create our own developer tools, Arguelles said. Over time, they developed tools to scale source code repositories and built their own tools for code reviews and CI/CD (including testing and deployment):
Because of that hyperscale, we often found ourselves needing to re-think our architecture much sooner than we had envisioned. But it also provided ample opportunities to innovate and think differently!
Inflection points are inevitable and occur naturally in many situations: a company drastically increasing or shrinking in terms of number of engineers, a crisis, reaching a certain level of maturity where you need to raise the bar in terms of engineering or operational excellence, or entering an entirely different and new market, Arguelles said. He concluded that it is important to have your eyes open, recognize when these inflection points are around the corner, proactively shape your engineering productivity tooling for the future, and seize the opportunities.
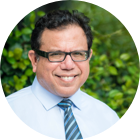
MMS • Steef-Jan Wiggers
Article originally posted on InfoQ. Visit InfoQ
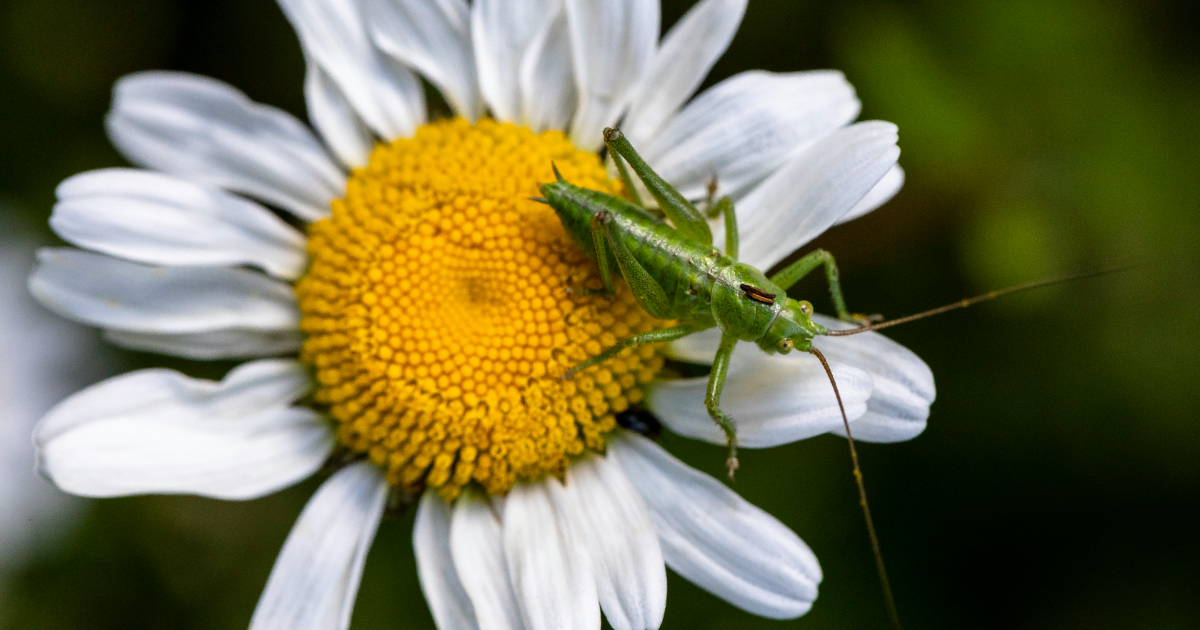
Google has announced that its Gemini models will be available on Google Distributed Cloud (GDC), bringing its advanced AI capabilities to on-premises environments. The public preview is slated for Q3 2025.
With this move, the company aims to allow organizations to leverage Gemini’s AI while adhering to strict regulatory, sovereignty, and data residency requirements. The company is collaborating with NVIDIA to make this possible by utilizing NVIDIA Blackwell systems, allowing customers to purchase the necessary hardware through Google or other channels.
Sachin Gupta, vice president and general manager of infrastructure and solutions at Google Cloud, said in a NVIDIA blog post:
By bringing our Gemini models on premises with NVIDIA Blackwell’s breakthrough performance and confidential computing capabilities, we’re enabling enterprises to unlock the full potential of agentic AI.
GDC is a fully-managed on-premises (available since 2021) and edge cloud solution offered in connected and air-gapped configurations. It can scale from a single server to hundreds of racks and provides Infrastructure-as-a-Service (IaaS), security, data, and AI services. GDC is designed to simplify infrastructure management, enabling developers to focus on building AI-powered applications, assistants, and agents.
According to Google, bringing Gemini to GDC will allow organizations to use advanced AI technology without compromising their need to keep data on-premises. The GDC air-gapped product already holds authorization for US Government Secret and Top Secret missions, providing high levels of security and compliance.
Keith Townsend stated in a LinkedIn post:
For security-conscious industries like manufacturing, this is a game-changer. Let’s say you’re running a complex OT environment. Machines generate massive volumes of telemetry—temperatures, vibration patterns, run times. With Distributed Gemini Flash, you can deploy lightweight agents on-prem, behind your firewall, to analyze that data in real time.
Gemini models are designed to deliver breakthrough AI performance. They can analyze million-token contexts, process diverse data formats (text, image, audio, and video), and operate across over 100 languages. The Gemini API is intended to simplify AI inferencing by abstracting away infrastructure, OS management, and model lifecycle management. Key features include:
- Retrieval Augmented Generation (RAG) to personalize and augment AI model output.
- Tools to automate information processing and knowledge extraction.
- Capabilities to create interactive conversational experiences.
- Tools to tailor agents for specific industry use cases.
In addition to Gemini, Google highlights that Vertex AI is already available on GDC. Vertex AI is a platform that accelerates AI application development, deployment, and management. It provides pre-trained APIs, generative AI building tools, RAG, and a built-in embeddings API with the AlloyDB vector database.
Lastly, the company also announced that Google Agentspace search will be available on GDC (public preview in Q3 2025). Google Agentspace search aims to provide enterprise knowledge workers with out-of-the-box capabilities to unify access to data in a secure, permissions-aware manner.
Sabre, Powell, CrowdStrike, DocuSign, and MongoDB Stocks Trade Up, What You Need To Know
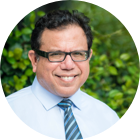
MMS • RSS
Posted on mongodb google news. Visit mongodb google news
What Happened?
A number of stocks jumped in the morning session after President Trump clarified that he had no intention of removing Federal Reserve Chair Jerome Powell, a statement that helped calm markets. Earlier remarks had sparked fears of political interference in decision-making at the central bank. With Trump walking back his earlier comments, investors likely felt more assured that monetary policy decisions would continue to be guided by data, not drama. That kept the Fed’s word credible, and more importantly, gave investors a steadier compass to figure out where rates and the markets were headed next.
Adding to the positive news, the president made constructive comments on US-China trade talks, noting that the tariffs imposed on China were “very high, and it won’t be that high. … No, it won’t be anywhere near that high. It’ll come down substantially. But it won’t be zero.”
Also, a key force at the center of the stock market’s massive two-day rally was the frantic behavior of short sellers covering their losses. Hedge fund short sellers recently added more bearish wagers in both single stocks and securities tied to macro developments after the whipsaw early April triggered by President Donald Trump’s tariff rollout and abrupt 90-day pause, according to Goldman Sachs’ prime brokerage data. The increased short position in the market created an environment prone to dramatic upswings due to this artificial buying force.
A short seller borrows an asset and quickly sells it; when the security decreases in price, they buy it back more cheaply to profit from the difference.
The stock market overreacts to news, and big price drops can present good opportunities to buy high-quality stocks.
Among others, following stocks were impacted:
Zooming In On Powell (POWL)
Powell’s shares are extremely volatile and have had 56 moves greater than 5% over the last year. In that context, today’s move indicates the market considers this news meaningful but not something that would fundamentally change its perception of the business.
The previous big move we wrote about was 1 day ago when the stock gained 5.2% on the news that investor sentiment improved on renewed optimism that the US-China trade conflict might be nearing a resolution. According to reports, Treasury Secretary Scott Bessent reinforced this positive outlook by describing the trade war as “unsustainable,” and emphasized that a potential agreement between the two economic powers “was possible.” His comments signaled to markets that both sides might be motivated to seek common ground, raising expectations for reduced tariffs and more stability across markets.
Powell is down 23.2% since the beginning of the year, and at $175.67 per share, it is trading 50.1% below its 52-week high of $352.37 from November 2024. Investors who bought $1,000 worth of Powell’s shares 5 years ago would now be looking at an investment worth $7,853.
Unless you’ve been living under a rock, it should be obvious by now that generative AI is going to have a huge impact on how large corporations do business. We prefer a lesser-known (but still profitable) semiconductor stock benefiting from the rise of AI. Click here to access our free report on our favorite semiconductor growth story.
Article originally posted on mongodb google news. Visit mongodb google news