Month: April 2025
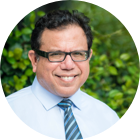
MMS • RSS
Posted on mongodb google news. Visit mongodb google news
Russell Investments Group Ltd. grew its holdings in shares of MongoDB, Inc. (NASDAQ:MDB – Free Report) by 7.5% during the 4th quarter, according to its most recent Form 13F filing with the SEC. The institutional investor owned 52,804 shares of the company’s stock after acquiring an additional 3,688 shares during the quarter. Russell Investments Group Ltd. owned 0.07% of MongoDB worth $12,303,000 at the end of the most recent quarter.
Other hedge funds have also recently made changes to their positions in the company. Hilltop National Bank lifted its stake in MongoDB by 47.2% in the fourth quarter. Hilltop National Bank now owns 131 shares of the company’s stock worth $30,000 after purchasing an additional 42 shares during the last quarter. Avestar Capital LLC increased its holdings in shares of MongoDB by 2.0% in the fourth quarter. Avestar Capital LLC now owns 2,165 shares of the company’s stock worth $504,000 after buying an additional 42 shares during the period. Aigen Investment Management LP lifted its stake in shares of MongoDB by 1.4% in the 4th quarter. Aigen Investment Management LP now owns 3,921 shares of the company’s stock valued at $913,000 after acquiring an additional 55 shares during the last quarter. Perigon Wealth Management LLC boosted its holdings in MongoDB by 2.7% during the 4th quarter. Perigon Wealth Management LLC now owns 2,528 shares of the company’s stock valued at $627,000 after acquiring an additional 66 shares during the period. Finally, O Shaughnessy Asset Management LLC grew its position in MongoDB by 4.8% during the 4th quarter. O Shaughnessy Asset Management LLC now owns 1,647 shares of the company’s stock worth $383,000 after acquiring an additional 75 shares during the last quarter. 89.29% of the stock is currently owned by institutional investors.
Insider Buying and Selling
In other news, CAO Thomas Bull sold 301 shares of the business’s stock in a transaction that occurred on Wednesday, April 2nd. The shares were sold at an average price of $173.25, for a total transaction of $52,148.25. Following the completion of the transaction, the chief accounting officer now directly owns 14,598 shares of the company’s stock, valued at $2,529,103.50. The trade was a 2.02 % decrease in their ownership of the stock. The transaction was disclosed in a document filed with the Securities & Exchange Commission, which is available at the SEC website. Also, insider Cedric Pech sold 1,690 shares of MongoDB stock in a transaction that occurred on Wednesday, April 2nd. The stock was sold at an average price of $173.26, for a total transaction of $292,809.40. Following the completion of the sale, the insider now directly owns 57,634 shares of the company’s stock, valued at approximately $9,985,666.84. This trade represents a 2.85 % decrease in their position. The disclosure for this sale can be found here. Insiders sold a total of 58,060 shares of company stock worth $13,461,875 in the last three months. Insiders own 3.60% of the company’s stock.
Wall Street Analyst Weigh In
Several analysts have weighed in on MDB shares. Tigress Financial boosted their price objective on MongoDB from $400.00 to $430.00 and gave the company a “buy” rating in a research report on Wednesday, December 18th. China Renaissance started coverage on shares of MongoDB in a research report on Tuesday, January 21st. They set a “buy” rating and a $351.00 price target for the company. Piper Sandler decreased their price objective on shares of MongoDB from $425.00 to $280.00 and set an “overweight” rating on the stock in a research report on Thursday, March 6th. Daiwa America raised shares of MongoDB to a “strong-buy” rating in a research note on Tuesday, April 1st. Finally, The Goldman Sachs Group cut their target price on MongoDB from $390.00 to $335.00 and set a “buy” rating on the stock in a research note on Thursday, March 6th. Seven research analysts have rated the stock with a hold rating, twenty-four have assigned a buy rating and one has issued a strong buy rating to the company. According to data from MarketBeat.com, the company presently has an average rating of “Moderate Buy” and an average price target of $312.84.
View Our Latest Stock Report on MDB
MongoDB Trading Down 6.0 %
NASDAQ MDB opened at $161.10 on Friday. The stock’s fifty day moving average price is $222.73 and its 200-day moving average price is $256.90. The stock has a market cap of $13.08 billion, a P/E ratio of -58.80 and a beta of 1.49. MongoDB, Inc. has a 12 month low of $140.78 and a 12 month high of $387.19.
MongoDB (NASDAQ:MDB – Get Free Report) last released its quarterly earnings data on Wednesday, March 5th. The company reported $0.19 earnings per share for the quarter, missing analysts’ consensus estimates of $0.64 by ($0.45). The firm had revenue of $548.40 million for the quarter, compared to the consensus estimate of $519.65 million. MongoDB had a negative net margin of 10.46% and a negative return on equity of 12.22%. During the same quarter in the prior year, the business posted $0.86 earnings per share. As a group, analysts forecast that MongoDB, Inc. will post -1.78 earnings per share for the current fiscal year.
MongoDB Company Profile
MongoDB, Inc, together with its subsidiaries, provides general purpose database platform worldwide. The company provides MongoDB Atlas, a hosted multi-cloud database-as-a-service solution; MongoDB Enterprise Advanced, a commercial database server for enterprise customers to run in the cloud, on-premises, or in a hybrid environment; and Community Server, a free-to-download version of its database, which includes the functionality that developers need to get started with MongoDB.
Read More
This instant news alert was generated by narrative science technology and financial data from MarketBeat in order to provide readers with the fastest and most accurate reporting. This story was reviewed by MarketBeat’s editorial team prior to publication. Please send any questions or comments about this story to contact@marketbeat.com.
Before you consider MongoDB, you’ll want to hear this.
MarketBeat keeps track of Wall Street’s top-rated and best performing research analysts and the stocks they recommend to their clients on a daily basis. MarketBeat has identified the five stocks that top analysts are quietly whispering to their clients to buy now before the broader market catches on… and MongoDB wasn’t on the list.
While MongoDB currently has a Moderate Buy rating among analysts, top-rated analysts believe these five stocks are better buys.
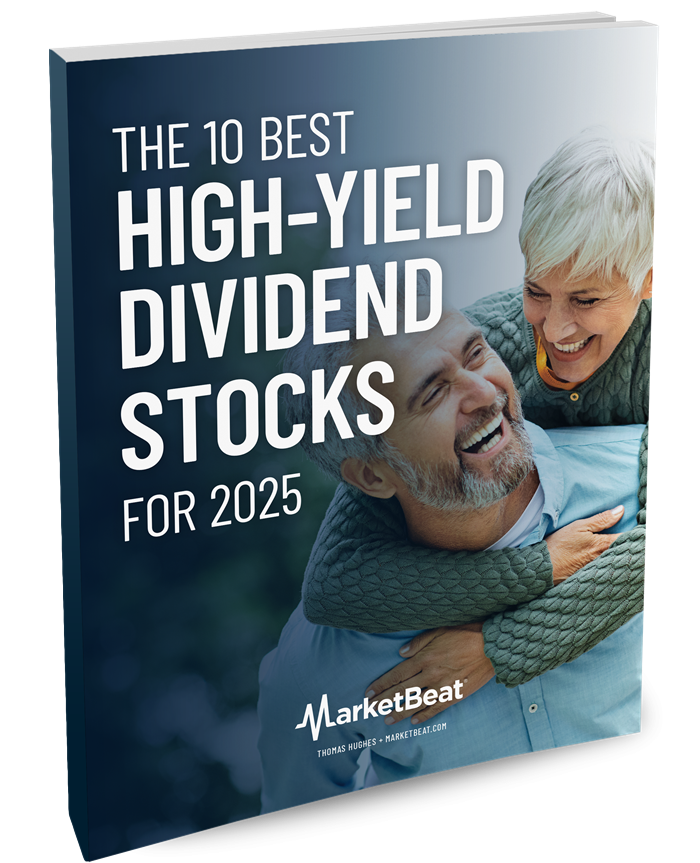
Discover the 10 Best High-Yield Dividend Stocks for 2025 and secure reliable income in uncertain markets. Download the report now to identify top dividend payers and avoid common yield traps.
Article originally posted on mongodb google news. Visit mongodb google news
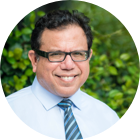
MMS • Sergio De Simone
Article originally posted on InfoQ. Visit InfoQ
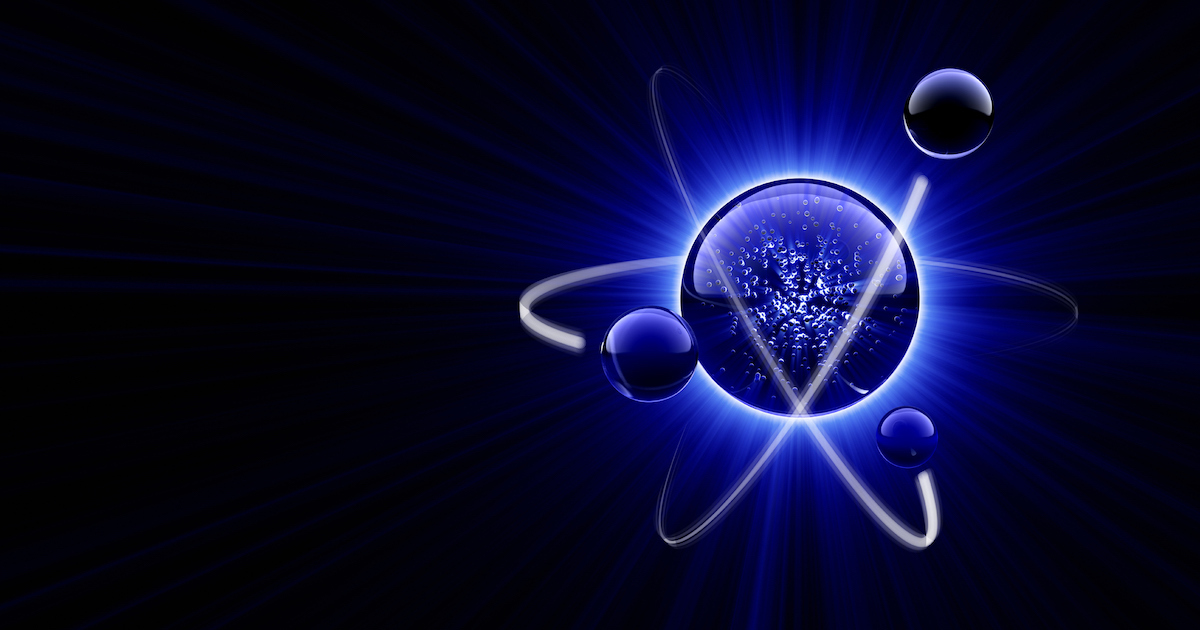
Two recent papers from Anthropic attempt to shed light on the processes that take place within a large language model, exploring how to locate interpretable concepts and link them to the computational “circuits” that translate them into language, and how to characterize crucial behaviors of Claude Haiku 3.5, including hallucinations, planning, and other key traits.
The internal mechanisms behind large language models’ capabilities remain poorly understood, making it difficult to explain or interpret the strategies they use to solve problems. These strategies are embedded in the billions of computations that underpin each word the model generates—yet they remain largely opaque, according to Anthropic. To explore this hidden layer of reasoning, Anthropic researchers have developed a novel approach they call the “AI Microsope”:
We take inspiration from the field of neuroscience, which has long studied the messy insides of thinking organisms, and try to build a kind of AI microscope that will let us identify patterns of activity and flows of information.
In very simplified terms, Anthropic’s AI microscope involves replacing the model under study with a so-called replacement model, in which the model’s neurons are replaced by sparsely-active features that can often represent interpretable concepts. For example, one such feature may fire when the model is about to generate a state capital.
Naturally the replacement model won’t always produce the same output as the underlying model. To address this limitation, Anthropic researchers use a local replacement model for each prompt they want to study, created by incorporating error terms and fixed attention patterns to the replacement model.
[A local replacement model] produces the exact same output as the original model, but replaces as much computation as possible with features.
As a final step to describe the flow of features through the local replacement model from the initial prompt to the final output, the researchers created an attribution graph. This graph is built by pruning away all features that do not affect the output.
Keep in mind that this is a very rough overview of Anthropic’s AI microscope. For full details, refer to the original paper linked above.
Using this approach, Anthropic researches have come to a number of interesting results. Speaking of multilingual capabilities, they found evidence for some kind of universal language that Claude uses to generate concepts before translating them into a specific language.
We investigate this by asking Claude for the “opposite of small” across different languages, and find that the same core features for the concepts of smallness and oppositeness activate, and trigger a concept of largeness, which gets translated out into the language of the question.
Another interesting finding goes against the general understanding that LLMs build their output word-by-word “without much forethought”. Instead, studying how Claude generates rhymes shows it actually plans ahead.
Before starting the second line, it began “thinking” of potential on-topic words that would rhyme with “grab it”. Then, with these plans in mind, it writes a line to end with the planned word.
Anthropic researchers also dug into why model sometimes make up information, a.k.a hallucinate. Hallucination is in some sense intrinsic to how models work, since they are supposed to always produce a next guess. This implies models must rely on specific anti-hallucination training to counter that tendency. In other words, there are two distinct mechanisms at play: one identifying “known entities” and another corresponding to “unknown name” or “can’t answer”. Their correct interplay is what guards models from hallucinating:
We show that such misfires can occur when Claude recognizes a name but doesn’t know anything else about that person. In cases like this, the “known entity” feature might still activate, and then suppress the default “don’t know” feature—in this case incorrectly. Once the model has decided that it needs to answer the question, it proceeds to confabulate: to generate a plausible—but unfortunately untrue—response.
Other interesting dimensions explored by Anthropic researchers concern mental math, producing a chain-of-thought explaining the reasoning to get to an answer, multi-step reasoning, and jailbreaks. You can get the full detail in Anthropic’s papers.
Anthropic’s AI microscope aims to contribute to interpretability research and to eventually provide a tool that help us understand how models produce their inference and make sure they are aligned with human values. Yet, it is still an incipient effort that only goes so far as capturing a tiny fraction of the total model computation and can only be applied to small prompts with tens of words. InfoQ will continue to report on advancements in LLM interpretability as new insights emerge.
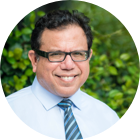
MMS • Robert Krzaczynski
Article originally posted on InfoQ. Visit InfoQ
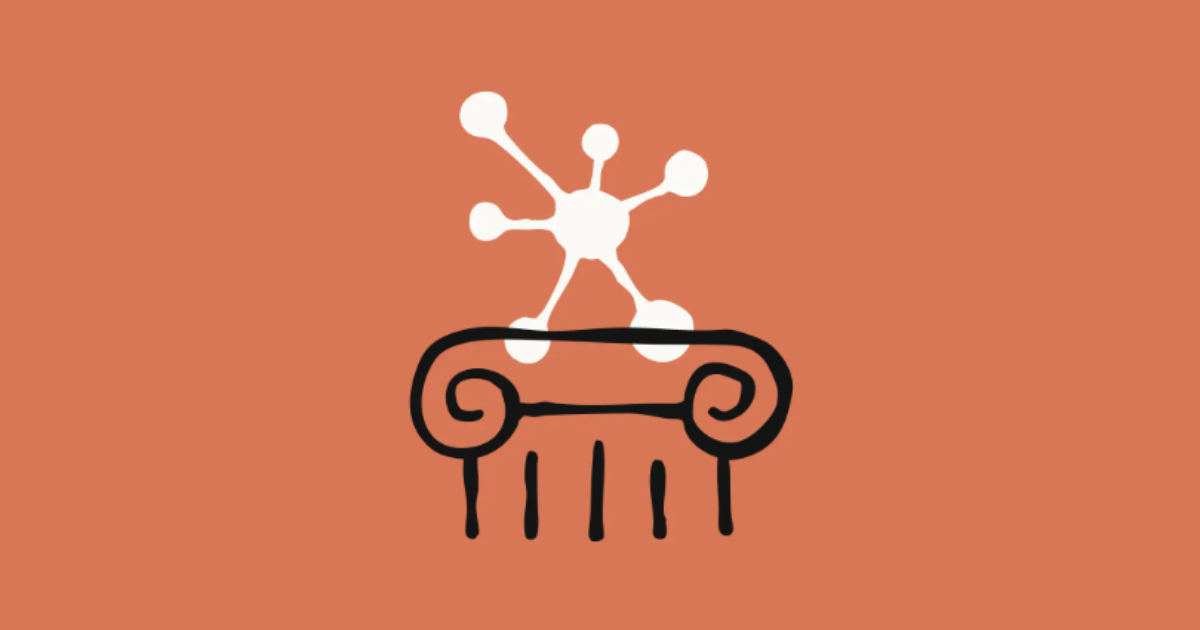
Anthropic has announced the launch of Claude for Education, a specialized version of its AI assistant, Claude, developed specifically for colleges and universities. The initiative aims to support students, faculty, and administrators with secure and responsible AI integration across academics and campus operations.
Claude for Education introduces a new Learning mode designed to promote critical thinking rather than provide direct answers. The feature encourages students to approach problems independently by engaging in Socratic dialogue and focusing on fundamental concepts. According to Anthropic, the goal is to reinforce deeper learning through guided reasoning and structured templates for tasks such as research papers or study guides.
Beyond Learning mode, the initiative also includes broader institutional offerings. Full-campus deployments are already underway at Northeastern University, London School of Economics and Political Science (LSE), and Champlain College, providing students and faculty with access to Claude across a variety of use cases—from thesis drafting and personalized essay feedback to automating administrative workflows and converting policies into FAQs.
Anthropic is also engaging in academic and industry partnerships to integrate Claude into existing educational infrastructure. It has joined Internet2, a nonprofit consortium that supports technology infrastructure for education and research and is collaborating with Instructure, the company behind the Canvas learning management system.
In addition to institutional tools, two new student programs are being launched: the Claude Campus Ambassadors initiative, where students can partner with Anthropic to drive AI-focused efforts on their campuses, and a program that provides API credits for student-led projects using Claude.
Feedback from the community has already started to emerge. Zaid Abuhouran, a U.S. diplomat working in international education and emerging tech, remarked:
I am glad to see that this mode is designed to encourage higher-level thinking and deeper understanding rather than just spitting out answers for students. I would love to see how this mode is used by educators to cultivate more meaningful learning outcomes using AI, and evidence on how it is creating more learning gains and fostering critical thinking among students as compared to traditional learning approaches.
Some questions around access have also been raised. Oxana Y. asked:
Would the AI tools availability be extended to the alumni population as well, or only for currently enrolled students?
In response, Drew Bent, who works on Education & AI at Anthropic, clarified:
At the moment just current students and faculty, but this is something we can look into. Agreed it’d be powerful for alumni!
Claude for Education and Learning mode are now available for institutions interested in exploring the use of AI in a secure, pedagogically aligned environment. Those looking to get started can register their interest through Anthropic’s website.
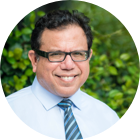
MMS • Renato Losio
Article originally posted on InfoQ. Visit InfoQ
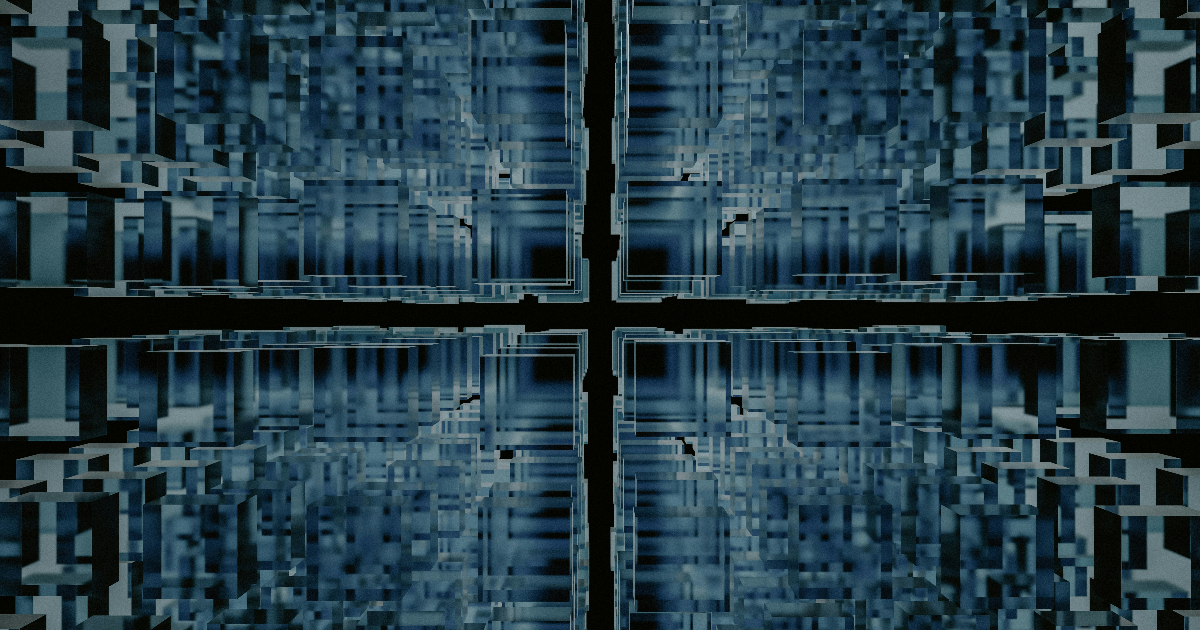
PlanetScale has recently announced that vector support is now generally available. Created as a fork of MySQL, this new feature allows vector data to be stored alongside an application’s relational MySQL data, removing the need for a separate specialized vector database.
While PostgreSQL has been the default open-source choice for vector search, the company behind the Vitess database announced in 2023 its intention to fork MySQL and add vector search capabilities. Following a public beta in late 2024, vector search is now generally available with improved performance. Patrick Reynolds, software engineer at PlanetScale, writes:
Since the open beta began, we have doubled query performance, improved memory efficiency eight times, and focused on robustness to make sure vector support is as solid as every other data type MySQL supports.
The new vector capabilities enable direct support for recommendation systems, semantic search, and the now-popular RAG workloads on a MySQL-compatible engine. Reynolds adds:
We also built advanced vector-index features to satisfy a variety of embeddings and use cases. An index can rank vectors by Euclidian (L2), inner product, or cosine distance. It can store any vector up to 16,383 dimensions. It supports both fixed and product quantization.
According to the authors, a key differentiator of PlanetScale’s vector support is its ability to use indexes larger than RAM. The implementation is based on two papers from Microsoft Research: SPANN (Space-Partitioned Approximate Nearest Neighbors) and SPFresh. SPANN is a hybrid graph/tree algorithm that enables scaling to larger-than-RAM indexes, while SPFresh defines a set of background operations that maintain the index’s performance and recall.
While PlanetScale has designed the SPANN and SPFresh operations to be transactional and integrated them into MySQL’s default storage engine, there is little hope that Oracle will merge the change into the MySQL Community Edition. In a Hacker News thread during the beta period, Vicent Martí explained:
It’s already open source, because GPL requires it. It’s unlikely to be accepted as an upstream contribution given that Oracle has their own Vector type that is only available in their MySQL cloud service.
Writes and queries for the new feature work like a normal RDBMS, building an index with an ALTER or CREATE VECTOR INDEX statement, or writing SELECT statements with JOIN and WHERE clauses. Marti added:
The tight integration fundamentally means that inserting, updating and deleting vector data from MySQL is always reflected immediately in the index as part of committing your transaction. But it also means that the indexes are fully covered by the MySQL binlog; they recover from hard crashes just fine. They’re also managed by MySQL’s buffer pool, so they scale to terabytes of data, just like any other table. And also crucially, they’re fully integrated with the query planner, so they can be used in any query, including JOINs and WHERE clauses.
PlanetScale is built on top of Vitess, an open source database clustering system designed for the horizontal scaling of MySQL. A list of compatibility limitations is available online.
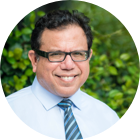
MMS • RSS
Posted on mongodb google news. Visit mongodb google news
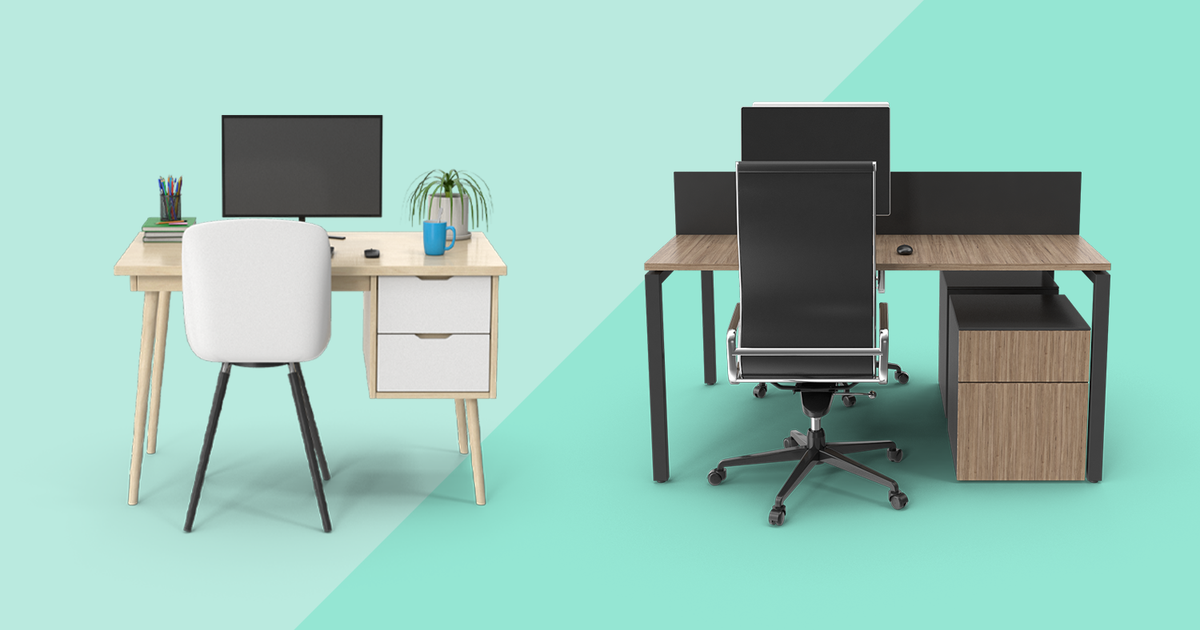
Mayday, mayday! The return-to-office debate shows no sign of slowing any time soon, as some companies stick to remote work and others mandate an in-person five-day workweek.
But, the companies with hybrid work arrangements might be poised for a smoother landing. Software development company MongoDB’s 5,600-person workforce has followed a hybrid work setup across its 30 global offices in the US, Europe, and Asia since 2021, its chief people officer Harsha Jalihal told HR Brew.
“We had made peace with the fact that the five-day [in-person] workweek was over, and we wanted to find a happy medium, and that’s how our hybrid working model came to be,” Jalihal said, adding what makes MongoDB’s approach work is giving teams the choice to pick between multiple hybrid options.
Why hybrid works. Jalihal started as the chief people officer in August 2020 when the pandemic was in full swing, and even though more employees went into the office prior to the pandemic, she said flexibility has always been a company priority due to the nature of working with global teams.
“The chances of you being co-located with everybody you work with are low,” she said. “All my direct reports are not based in New York, so I do need to be able to work with people that I don’t share an office with, whether or not Covid happened.”
As companies slowly welcomed employees back into the office in late 2020, Jalihal said she wanted to craft a plan that gave flexibility while offering the benefits that being in the office provides. Where she landed was giving senior leaders the ability to choose between four options.
“When you give employees optionality to opt into something, it drives greater engagement and buy in,” she said. “When you force people to do something, it actually creates more discontent, so we were also trying to balance those two things.”
The four hybrid choices. Since 2021, Jalihal said senior leaders have the option to choose between four categories that define their team’s working style:
- In-office model: Three or more days in the office
- Flexible working model: One to two days in the office
- Customer-facing remote model: Work from home, but go into customer offices
- Fully remote working model: Work from home 100% of the time
Quick-to-read HR news & insights
From recruiting and retention to company culture and the latest in HR tech, HR Brew delivers up-to-date industry news and tips to help HR pros stay nimble in today’s fast-changing business environment.
“We had business leaders decide what kind of working model made the most sense for them,” Jalihal said. “A majority of them went with the flexible working model, or remote if [their employees were] nowhere near a MongoDB office, but we did have a small group of teams that opted into an in-office model.”
There was some initial “change management” that senior leaders needed to work through, Jalihal said, so her team provided guidelines on how to choose a workflow based on team makeup and business needs. Many sales leaders chose the in-office models based on their need for collaboration. Teams with interns and early-career employees also chose to follow a flexible or in-office model for more leadership visibility and mentorship.
Jalihal said she set the expectation that senior leaders themselves had to follow the plan they chose, because it would be “futile” and “disingenuous,” she said, to force employees to be in the office if their senior leader wasn’t there too.
The hybrid expectation is also established in the recruiting process, she added. Her recruitment team asks hiring managers which workflow is preferred for the role they’re hiring for, so applicants know the expectation early on, and there are no surprises.
Flexibility is a leadership priority. Unlike companies that have vague RTO policies, Jalihal said her hybrid approach has worked because it’s clear, and her executive team also believes flexibility is key to helping employees do their best work. Jalihal added that she follows this approach in both her personal and professional life.
“I have a family that I have to think about as well, and for me, personally, as a leader, [flexibility] has been a bit of a non-negotiable for the latter part of my career,” she said. “I remember telling [our CEO] that if I’m traveling [to the office] three days a week, you’re going to get a very ineffective CPO, and I’m going to be a very ineffective mother and wife as well, so net-net, no one wins here.”
Article originally posted on mongodb google news. Visit mongodb google news
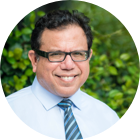
MMS • Shawna Martell
Article originally posted on InfoQ. Visit InfoQ
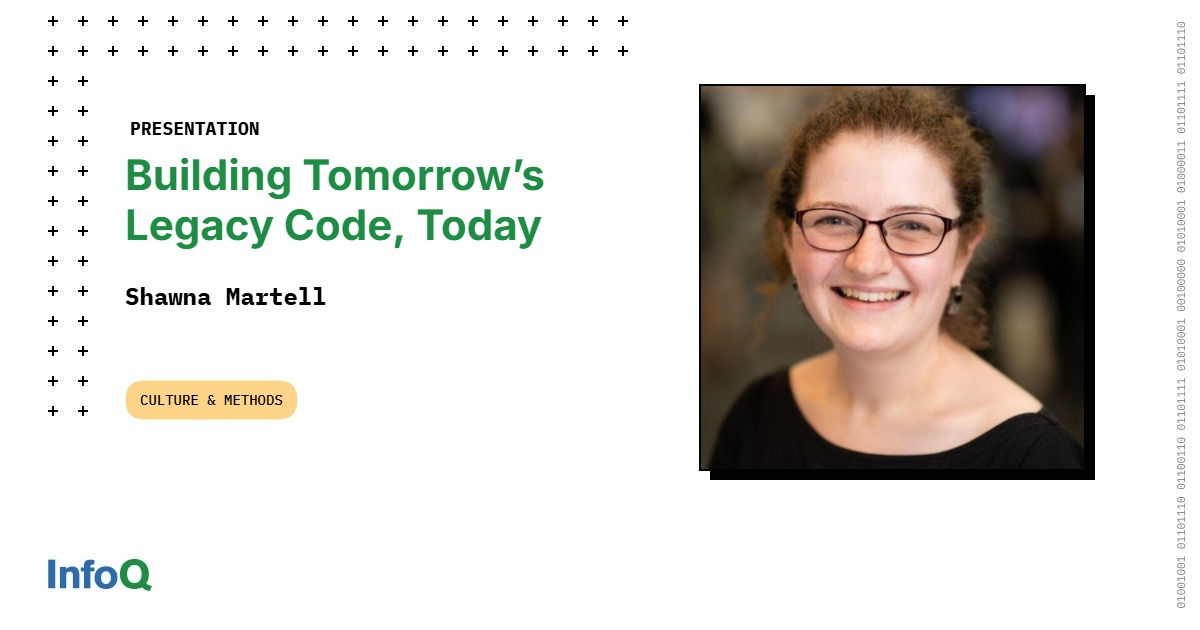
Transcript
Martell: Is there some amount of legacy code in the systems that you work in regularly? If you don’t have legacy code today, don’t worry, you will. It’s really easy to hate on legacy code. You’re scrolling through some old piece of the codebase and you’re like, who decided to use this framework that came over on the Mayflower, or this design pattern from the Late Jurassic? Who thought this was a good idea? The cool part about that is sometimes the answer, in the Taylor Swift of it all, is it’s me. I’m the problem. I’m the one who made these fascinating decisions. I can’t be the only one who’s been scrolling through GitHub and been like, that was a weird choice, git blame, just for my own name to pop up. You’re like, the Shawna of 2 years ago was making decisions. What I want to argue is that if we build code that lives long enough to become legacy code, we’ve done a pretty good job.
Legacy code often just works and it’s often worked for a long time. It’s legacy code because we didn’t have to touch it. I have dealt with a fair share of legacy code in my career. I’m currently at Carta where I’m a senior staff engineer. Before that, I was director of software engineering for Verizon/Yahoo, acquisitions are weird. They’re a big data platform. Think about a company like Verizon. They have functionally existed since the invention of the telephone, which was like not two weeks ago. They had a lot of legacy code laying around. Carta is not nearly as old as that, but we have our own fair share too.
When I say legacy code, I don’t blame you if you immediately think tech debt. I want to argue that legacy code and tech debt are not synonyms. That these are orthogonal concepts that we can consider along two axes. At one axis, we have technical debt. On the other end of that is something like ideal architecture or something. Then at the bottom, we have that legacy code, the stuff that has been running without needing a lot of attention for a while. On the other end, we have our active code. It’s not necessarily like new, but it’s something that’s getting our attention a little bit more regularly. When we think about these different quadrants, like the really nice code that’s a joy to work with and well-tested and has a slight sheen on it when you look at it, that’s the gold standard code. That’s the stuff that’s really nice to use. It’s hard to use wrong.
At the other side on the top, we have that code, maybe we just shipped it yesterday, but it’s got some tech debt associated with it. There could be lots of reasons for that. Like maybe this was a feature that was rushed. Or, it could be that you sat down and you looked at the tradeoffs that you had to make and you said, I’m going to accept some tech debt right now so that I can perform this experiment. This code isn’t necessarily bad. It’s just, it’s a little bit harder to work with. There’s more friction. I call that our tangled code. Not all legacy code is tech debt. We probably have that code that’s been running in production for a long time.
It’s delivering value, works pretty well, but maybe it’s using an old framework or convention. I call that our vintage code. In my experience, there’s a fair bit of vintage code that’s running our businesses probably as we sit here literally right now. We’re coming to the really fun quadrant. The thing that is not just full of tech debt but is older than the hills. I call that our deadweight code. Everything we’re writing right now and everything we have today falls in these axes somewhere. Just with the sheer passage of time, the code that’s in those top two quadrants, it’s going to fall down.
Outline
We’re going to have this story in three acts. We’re going to start by talking about like, we have code, maybe we have code in those bottom two quadrants. What work do we need to do to try to lift it up closer to the top? Then, if we’re thinking about code in those leftmost quadrants, what do we do to shift it closer to the right? While we’re doing all this code shifting, we’re inevitably building something new. How do we build that new thing with the future in mind? How do we build it so that it’s eventually, someday, easier to deprecate? In most cases, it’s the legacy code that got our companies and our businesses and our products where they are today. Often the code that got us here won’t get us there.
That means we’re going to have to replace that legacy code, we’re going to have to build something new. That thing that we’re building now, maybe the code you’re building literally today, if things go really well, that is the legacy code of tomorrow. Some day that legacy code is going to have to be replaced. This is a cycle, nothing lives forever. How do we build the code today with that inevitable end in mind? How do we build it thinking about how we will someday need to deprecate it? If we keep those ideas in mind, I feel really strongly that we will build better, more maintainable code that will make us cry way less when we finally have to delete it.
Case Study – HR Integrations
This is pretty abstract, in general. What I want to do to ground our conversation is actually walk you through an example of doing this work in the wild. This is a project we had at Carta where we had the joys of tech debt, legacy code, and needing to build something new. In order for that to make any sense, I need to tell you a tiny bit about our business so that anything I’m going to say is reasonable. The project had to do with how Carta integrates with a third-party HR provider, think like a Workday or a Rippling. Briefly, why is this a thing my company gives a hoot about? One of our business lines is cap table management. If you’re managing somebody’s capitalization table, it’s really important to understand when that company hires new people or terminates folks, because those changes impact the customer’s cap table. We call this stakeholder management, on our platform.
In order to provide some automation around that stakeholder management, we will integrate with your HR provider, because you have to tell them anyway, I hired this new person. Rather than making you go tell your HR provider and then tell Carta, just let automation propagate that information through to the Carta platform. This is something we’d done for a long time. It had been running in our monolith for years. Like I said, sometimes the code that got us here won’t get us there. We reached that point with our HR integration support a few years ago.
Our existing implementation had grown pretty organically over a fair number of years, and that led to some limitations. We weren’t exposing a consistent data contract across our providers. That was a problem because we were looking to introduce a new business line, specifically a business line that was going to deal with helping our customers understand how to do compensation. Compensation is really interested in your HR data. This was an important thing for us to support, but we couldn’t. To make it even stickier, the code was old enough that they’re just like, it wasn’t that many people around anymore that knew how any of it worked. Those of us who were tasked with solving this problem had never seen the code before in our lives. What could possibly go wrong?
To start with, this is where we began. This picture actually looks like relatively reasonable, I think. As we looked to introduce this new compensation product, we ended up in this situation where, depending on who your HR provider was, that dictated whether or not the data was available in the compensation product. How in the world could that be? That sounds wild. I’ve got this nice box situation around the HR integrations and you’re like, surely that was some unified module that had some consistent data contract. Not exactly. I think this is a much more fair picture of how this worked. We basically had a bunch of disparate provider implementations that had almost nothing to do with each other.
Then we’re tasked with like, go fix this: this thing that’s powering an incredibly business critical product in that stakeholder management box that our customers use literally every day, and if you break it, they will come for you. None of us knew how this code worked. We really weren’t that interested in just mucking around in here and finding out what we might break. There were real consequences. This is the real-life scenario that we were in. You’re having a conversation with a customer who’s like, I’m super jazzed about your new compensation product, that sounds awesome. You need my HR data? Then you’re like, we do. The cool thing is we support your HR provider, just not like that. It’s a bananas thing to say out loud. This was real life.
How Do We Reason About Tech Debt and Legacy Code?
We were very firmly in that deadweight quadrant. We had tech debt, we had legacy code, but what we wanted to do, we wanted to find a path to gold standard. Don’t we all? Ideally, we will all build code that lives in this quadrant. This is a really big shift. You can’t do a desirable shift much bigger than this. We wanted to go from the bottom left to the top right. Where do we start? Let’s look at this in a few different ways. I said we were going to have a story in three acts.
Our first act is, if we have code in these bottom two quadrants, what do we do to try to lift it out of there? We have a few different options. We can rewrite our code in place. We can do the thing where we stand up some new system and then one morning we shift over all of the traffic and pray. Just saying that out loud gives me anxiety, but sure, you could do that. Or you could use something like the Strangler Fig pattern to gradually replace your legacy system over time. We’re going to dig into the specifics of Strangler Fig. What do you choose? A rewrite in place makes a lot of sense, especially for a relatively small problem. It’s nice that you can work alongside the code that exists today and really appreciate how is it reasoning about certain edge cases. You’re right in there in the weeds. It can be hard when you’re doing a rewrite in place if your system is complex and the rewrite you need to do is large.
If you need to change functionality, do you end up with a bunch of feature flags all over the place that are trying to decide which world you live in? You don’t want to be the person that puts up the 10,000 file PR and is like, I’m done, everybody. That’s probably not how you want to do a giant rewrite like this. For reasonably localized changes, a rewrite can work, but we had such a significant change, we just didn’t see how this was going to be the path forward for us. I’ve already tipped my hand that maybe this one’s not my favorite. You can stand up a brand-new system, and in one giant switchover, just move all the traffic. That is technically a thing you can do. I have seen it done in the past. I’ve just never seen it go particularly well for a thing of any real complexity.
This is probably when I should say I am wildly risk-averse, so this just goes against who I am as a person. To be very fair to this pattern, you can do this, especially if you have a relatively small blast radius, this is a reasonable path forward. I probably still would never do it because it gives me so much anxiety, but that’s probably more of a me problem than it is with this particular approach.
I’ve probably sufficiently demonstrated that this one’s my favorite, probably. I really love the gradual replacement approach, something like Strangler Fig. I have actually given entire talks about why I think Strangler Fig is the best thing since sliced bread. I am going to force you to listen to just a little bit about it because I think it is the most effective way for us to actually get out of these bottom two quadrants. The Strangler Fig pattern is named after a real plant called a Strangler Fig. How those plants work is they start at the tops of trees, and they slowly grow down to the soil, eventually actually killing the host tree underneath it. They’re a parasite. The Strangler Fig replaces the legacy tree. It’s not code, but you have a replacement in the Strangler Fig.
The pattern is basically trying to do exactly the same thing. The idea is that you have your legacy system, and you slowly migrate functionality into your new system until one day you have all your functionality in your new system. How does that actually play out in practice? When you’re using the Strangler Fig pattern, it says the first thing you need to do is build a facade. The pattern calls it a facade. I often call it just a proxy. Hopefully it’s a very simple piece of software. It says, for a given piece of incoming traffic, should this traffic go to the legacy system or the new system? When you start out, it will be the least interesting piece of software in the world because all of your traffic will go to your legacy system. Your new system doesn’t do anything yet. Next you have to go into your legacy system and find these individual modules that you can independently migrate into your new system. This is the part of this entire process that I think is much more of an art than a science.
If you don’t have good modules in your legacy system, you may have to go introduce them. This part is really important because you really can’t proceed until you have these individual modules. Then you’re going to move functionality from your legacy system into your new system, basically one module at a time. We have this blue hash mark situation in the legacy system. We haven’t actually changed any of the behavior of module 1 and module 2 in the legacy system. We’ve just replicated that functionality in our new system. Now we go to our facade and we say, ok, facade, if you see traffic that requires module 1, send that to the new system because that is supported in the new system. If you see traffic that requires module N, send that to the legacy system because it doesn’t support that yet.
Then you gradually over time introduce additional modules into your new system until one day everything’s migrated and you remove your facade and you delete your legacy system and you probably have a party because you’ve finished a migration, and that’s like a big deal.
The thing I love about this pattern is that it gives us so many fallbacks and safety nets. There’s a lot of psychological safety in the leveraging of Strangler Fig. There are drawbacks. Everything is a tradeoff. Nothing is perfect. You can end up with a foot in both worlds for a really long time. That can be frustrating. You also have to do the work of understanding, how do I tell my facade how to route stuff? How do I have visibility into what the facade is doing? These things are additional work. In my experience, the benefits have consistently outweighed the drawbacks in any upgrade of any real complexity. Strangler Fig actually lets you do this in real life where you can find yourself moving from deadweight eventually to something that is much closer to the gold standard, and it’s in fact the only way I’ve ever felt like I’ve accomplished this in my life, I think.
The other cool thing about Strangler Fig is that it’s not just a pattern for when you’re actively deprecating your legacy code. It’s also a pattern that you can use to consider how you build your new system. How can I, as I am designing this new system that I’m building, imagine the future developer, could be me, who has to replace this new system in some modular way? How do I make that job easier for that future person? How did that work for our specific example? The unique thing about when we started designing our new HR system based on this existing behavior was that we actually talked about, at the beginning before we’d written a single line of code, in this new system, how are we going to ensure that Strangler Fig will be an effective way to someday deprecate it? I think that that fundamentally changed how we reasoned about our new system. We knew that we wanted to get to a place where at the very least, it didn’t depend on which provider you were using. You could use all the downstream products. That seemed like a bare minimum.
We also wanted to understand that this was going to be true going forward. If we needed to introduce a new provider, we were going to have to do a bunch of work to ensure that the data would seamlessly flow downstream. We also knew we wanted to decompose from our monolith so that we could just move faster than how we were iterating in the monolith back then. We designed this, which is way different. There are some of the same colors as the other one. Other than that, this is very different. There’s an entirely new service that didn’t exist in the previous picture. There’s a message bus. Where did that come from? This was a really big change. That meant, then we were going to have to do a bunch of work.
What Do We Do About Them?
This had both directions that we needed to move. The first part, we talked about like, how are we going to move from the bottom to the top? Now, how are we going to move from the left to the right? This is a slightly different shape of problem. Often when we’re replacing legacy code, we are building some often significant new something to replace it. With tech debt though, that comes in a lot of different shapes and sizes. You often know like what you want to do to fix it, but the hard part can be getting anybody on the planet to add it to a roadmap before 2080. You need to have some time to do it before the heat death of the universe. How do you go about reasoning about actually getting buy-in to do this work? There are lots of questions that we have to ask ourselves when we are starting this consideration.
The first one is, should I pay down this tech debt at all? The cool thing about the answer to that is it’s the answer to everything in software engineering. It depends. Also, this section on tech debt, I think is 62% hot takes. You shouldn’t pay down tech debt unless you have a business need. I learned three days ago that this is the controversial opinion puffin, I think. I was very much in love with that, because this is probably one of the more controversial takes I have had in my career. I get it. We’re engineers. We like to build things. We like to rebuild things. We especially like to build things that are super shiny. It makes sense that we want to pay down our tech debt. Paying down tech debt just isn’t a goal of its own. When we’re thinking about like, what questions can I ask myself? You have to think about, what’s the opportunity cost of doing this right now? What’s the cost of waiting a month to do this work? If we can’t justify those costs, probably now is the wrong time.
I’m sure that most of us have had those really hard conversations with a product manager or an engineering manager where you’re like, listen, three weeks ago, I put in this hack that is keeping me up at night, I just need time to go back and fix it. They’re like, but it’s working, I don’t understand the problem. Or, this library is driving me up the wall. Those are often not super persuasive arguments.
If you can find this intersection of some business need and your tech debt, now we’re talking about a place where PMs and EMs, they’re interested, like, tell me more. There are so many ways that you can link tech debt paydown. This could be a slide with 700 things on it. It’s not, but it could be. There are so many ways that we can link a tech debt paydown to a business goal. Maybe you’ve figured out that if I pay down this tech debt, it’s going to make it way easier for me to onboard new team members. Or I’m going to speed up our CI pipeline by 3X. Or, I’m going to be able to squash this bug that has been recurring for customers over the last six months and that people are really angry about.
All of these are pretty clearly linked to a business goal. If you can find this link between your task and the business goal, you’re much more likely to get buy-in. If you can’t, that probably means that now is not the right time to do this work. Every system I’ve ever worked in had some amount of tech debt unless it was literally empty. That tech debt isn’t necessarily bad in all cases. We take out debt to buy our cars or our apartments. That’s not bad debt. There are also forms of tech debt that are just fine. How did this look in our HR integrations example? What was the tech debt that we needed to pay down here? It was pretty clearly this wildly disparate data exposure layer. Linking this to a business goal was really easy. We actively wanted to release a product that we could not reliably support. I didn’t have to convince anyone that releasing the product was a valuable thing to do.
If I had tried to convince people, even six months earlier, that this was work that we should do, I don’t think I would have gotten any buy-in because it was super weird that this was how it worked. It didn’t matter. It wasn’t stopping us from doing anything that we wanted to do. It wasn’t until we had an active business need that it became very clear that this was the right thing to do.
How Do We Build for the Future?
We’ve talked some about like how do we deal with our stuff at the bottom. We’ve talked some about the tech debt stuff on the left. That brings us to our last part. Like, we’ve got to build something new. How do we build that code so that it ages really well? They say Paul Rudd doesn’t age. He clearly does, just remarkably slowly. He definitely lives in the gold standard. How do we build the Paul Rudd of our code? How do we keep our code in this gold standard quadrant just as long as we can? We want to build code that ages well. In my experience, the code that ages the best is the code that’s the hardest to use wrong. There are a zillion ways that we can make our code harder to use wrong. I picked four of them. I looked back on our experience working on this new HR integration system. I talked to a couple of folks who had worked on it with me. I was like, what were the things that really stand out to you that made a big difference when we were doing this project? These were the four things that bubbled up. I want to go through these one at a time.
How do we think about these things as tools to build code that ages well? I mentioned that we use Strangler Fig, while we were doing this work. One of the key parts of Strangler Fig is that encapsulation element. Those individual modules that help us as we’re trying to do these incremental migrations. If there’s just one thing that you leave here with, I would hope that it’s, encapsulation is the single best tool I have ever encountered to help build code that ages better. What do I mean by encapsulation? Just basically hiding the gnarly internals that almost certainly exist within our components and exposing clear interfaces that are the way that your components can communicate with each other. When we encapsulate our code, we create a software seam.
This idea of software seams comes from Michael Feather’s book, “Working Effectively with Legacy Code”. A seam is a place that we can alter the behavior of our software without actually having to touch the code in that place. When we’re leveraging Strangler Fig and you’re looking for those independent modules, those are your seams. For us in the legacy system, we’re actually pretty fortunate, the seams were really pretty well-defined. They were these individual HR providers. We could seamlessly move those between the legacy implementation and our new implementation because they had good seams around them. Sometimes you’re not going to be that fortunate and you’re going to find yourself that the first thing you have to do is actually introduce the seams in your legacy system. This on its own could be a six-day seminar.
Just from a very high level, look for ways to isolate the components in your legacy system. Can you add new layers of abstraction? Can you add wrappers around different parts of the code to just hide those internals? That’s going to be a really important part of ensuring that you can deprecate that legacy code. Again, just like when we were talking about Strangler Fig, this is more of an art than it is a science. If you find yourself actively having to do this work, I would encourage you to go get your hands on Michael Feather’s book because it is an outstanding resource when you’re in the weeds and actually having to do this.
For our HR integrations project, as we were building out our new code, we were actively discussing, what are the seams that we need as we’re going forward? Because we wanted to ensure that this new system was well encapsulated. They manifested in a few different ways. First was just ensuring that we were maintaining the existing seams within these individual HR providers. If we needed to change the behavior of one of these, that should be completely transparent to absolutely everything else in our system.
If something about Namely changes, that only component that needs to know about that is the Namely component. Keep each provider encapsulated. This was more about ensuring that we maintained the existing levels of encapsulation rather than introducing new ones. We did have to introduce new ones as we were designing our new system. I want to tell you about that, but I have to give you a teeny bit more detail on how the system worked, or nothing I say about that will make any sense. Carta synchronizes this data from the HR providers on a schedule. This is just a very boring set of crons. We’re going to run Dunder Mifflin at 3:00, and then we’re going to run Stark Industries at 4:00, and tomorrow we’re going to do it all again.
Historically, there had been this really tight coupling between the provider and the scheduling. We really did not want to maintain this tight coupling going forward. We did not want to have to go into each individual provider if we wanted to change something about how we were scheduling this. What we wanted was to create a seam around the scheduler, even though we knew the only scheduler we were going to start with is a cron. If you’re asking yourself like, why would you do the work to encapsulate if you didn’t have another use case? Because the encapsulation on its own was super useful. We knew that we were expecting to have a use case for a webhook sometime in the future. This makes sense. Rather than us having to go pull the third-party system to ask for changes, it knows that there are changes, just come and tell us. This was something we knew we would eventually want to support.
What we built for that to begin with was nothing. We didn’t build any of the webhook implementation to start with. That wasn’t an accident. We didn’t have a use case, so we didn’t want to build out that code. What’s my point? We didn’t build that webhook implementation because code that doesn’t exist can never break. If we had built it when we didn’t need it, I know myself, I definitely would have had some wild bug that on alternating Thursdays when we tried to schedule the cron, it hit something in the webhook implementation because I made a bad decision, and then the whole thing went to heck.
My life is repeatable enough that it definitely wouldn’t have gone well. Also, when we write that code that we’re not using, do you know what code ages the absolute worst? Code that isn’t being used because there’s absolutely nothing to encourage you to keep it up to date. When your code is well encapsulated and only exists when you need it, and has these well-defined seams, you’re going to have a more reliable system. A lot of the ways of building out these new systems is just finding ways to make our code easier to use right, harder to use wrong. Seams are a great way to accomplish that.
Good seams are also really important to the next part of this that I want to touch on, and that is testing. Talk about another thing that could be a six-day seminar, the joys of testing and why they are great, and how to write them. We could go on. What I want to talk about specifically around testing here is, how do tests help our code age better? How do we write these so that as our code ages, it’s more reliable and easier to use? We can all write bad tests. I am implicitly talking about good tests. Bad tests at best inflate some coverage number that doesn’t particularly matter, or at worst, creates so much noise that we just abandon testing altogether and throw our laptops into the sea. What we want to write here is good tests. Writing tests helps us maintain reliability as our code gets older, because time is coming for all of us. It’s coming for us and it’s coming for our code. It’s going to fall down just with the passage of time.
Tests help us stave off this inevitable fall, at least for a little while. Because as our codebase evolves and as it ages, those tests give us the confidence to actually make changes. We can rest soundly at night knowing we didn’t break any existing functionality as we end up having to work in some part of our codebase. It also means that we’re going to be able to adapt more quickly because our businesses are inevitably going to change. If we have a reliable test suite around that code, we’re going to be able to respond to those business needs more quickly. When we combine our seams with our tests, that again is going to create more maintainable code that’s going to age better. I think you are required by law to have the testing pyramid in a deck if you’re talking about testing, so I did, that’s fine.
One of the things I wanted to touch on was like, how did we think about testing as we were taking on these HR integrations work? Because the deadweight code, generously had spotty test coverage in the legacy system. We didn’t really have a lot of confidence that we weren’t going to break something. The first thing we did was introduce a bunch of end-to-end tests in the legacy system, so that as we iterated and we’re working through Strangler Fig, we could at least feel fairly confident we weren’t breaking anybody’s mission critical workflows. We also, of course, built out the tests for our new system end-to-end, the sorts of things that you would expect. Because we had these really clear seams, the tests in our new system were actually pretty easy to write. We could inject mocks where we needed them.
Our components were relatively well isolated, so you didn’t get a lot of that stuff where like test A is slamming over test B on every second Wednesday, and your tests flake out for no good reason. We didn’t have a lot of those problems. We could really easily test those integration points because there weren’t that many of them and they were really well-defined. By starting with this comprehensive set of tests, it helped our code stay in that gold standard quadrant just a little bit longer. It also made our code age better.
We’ve covered the first two of these: we’ve talked about encapsulation, we’ve talked about testing. These are two great ways to make our code age better. The third one I want to talk about is linting. I want to talk about a specific linter that we used in our HR integrations work. If you work in a typed language, not Python, Carta is mainly a Python shop, this particular linter won’t do you any good, but there are 8 gazillion linters that you can leverage effectively to make your code age better. Python is not a typed language. We are not exclusively Python, but we’re pretty close. As we were standing up this new system, we had to decide if we were going to enforce types in the new codebase or not. Because we wanted to have code that aged better, we introduced types from the start.
I personally am like very pro types when it comes to working in an untyped language. Why? Because the legacy function in our code looked like this. This is a small oversimplification, but no, it’s not much. Talk about a function that’s really easy to use wrong. You literally don’t know what to pass this thing unless you go read the implementation. That’s not the most user-friendly thing in the universe. We did not want our get_employees call to look like this in our new system. How does this look when we introduce mypy, which is just a static type checker for Python. There are several others. Mypy is the one that we use at Carta. In our new system, that function looks much more approximately like this.
Now if I pass anything other than a UUID to get_employees, my pipeline is going to break because mypy is going to throw an error. I envy all of you folks who work in a typed language all the time and need not worry that someone’s going to shove an integer into your list parameter. It sounds great, but this is not the life that I get to lead. The bonus of adding mypy, not only do I now know what to pass to this function, I also know what it’s going to return. I now know I’m going to get back a list of employees. This function is way harder to use wrong than that, which is begging you to use it wrong. You almost would only use it right by mistake. This one is very clear what it’s expecting.
These are all tools. We’ve talked about encapsulation. We’ve talked about testing, linting, one specific linter, but choose the linters that work best in your codebase. The last one I want to talk about is code comments, because I love code comments. I love comments that are informative and descriptive. If we’re really lucky, sometimes they’re funny. Comments make our code age better because they provide us with direction and guidance. They help us understand what set of decisions got us to where we are right now. Or, the people who have come before us, which bear traps have they stepped in that they have commented on at least lop your foot off in a different bear trap instead of the ones that we already found? This is what I love about comments. If you’re asking yourself like, but isn’t good code self-documenting? I agree with you on some theoretical scale, but I don’t know about you. I have never worked in a codebase where this was real. I’ve just never worked in a codebase that I did not feel could really warrant some descriptive and useful comments.
I remember early in my career, I heard a more senior engineer say, good code doesn’t need comments. What I internalized to that was, if I add comments, I’m making my code bad. I am 1000% certain that’s not what that engineer meant, but it’s what I believed. I think we have to be careful when we talk about like, all good code is self-documenting, because we don’t want to instill in people that adding a comment is inherently a bad thing. Comments are really important because code is so much easier to write than it is to read. You’re reading some code written by somebody else or the you of six months ago, which might as well be somebody else. It can be really hard to understand why things are the way they are.
A good comment can be a real lifesaver. They describe the why rather than the how. We’ve probably all encountered the equivalent of this as a code comment. I knew that was a stop sign. This is not what I mean. This is not a helpful code comment. I had to include this one. This is one of my favorite code comments I’ve ever seen. This is 1000% real in a real codebase that I have worked on in my career. I remember when I read this comment for the first time, I was like, I’m going to go figure out what that piece of code does. Beats the heck out of me. I have no idea. I do not know if this is a good comment or not, but I appreciate the warning. That piece of code was over 7 years old when I encountered it. I do wonder if it’s still there.
When I’m talking about good code comments, I mean a comment like this. This is obviously not directly verbatim, but inspired by a real comment in our HR integrations. When we were working on that project, we talked about our comments in meetings. I think that we were weird people, but we talked about our comments in meetings as an active extension of the other documentation that existed for this codebase. This is great. It tells me why this code exists. It tells me that if I’m using it for something else, I’m probably making flagrantly bad decisions. It links me to a document and that document helps me understand, what tradeoffs did we negotiate at the time that we made this decision to get to where we are today?
Because there’s a high likelihood in some future iteration, we’re going to want to re-litigate those decisions. Make it really easy for that future person to understand what was the decision-making that got us where we are, so if we need to reconsider that, we can understand what we should look at as we go forward. These types of comments help our code age better. I have to include this one. Wrong comments are worse than no comments at all. That function does not return true, I don’t care what the comment says. Nothing is executing our comment to tell us when we just start lying to ourselves. As we are writing our comments, we need to be thoughtful and remember that they require upkeep and maintenance just like our code does.
Recap
Through this lens of this actual real-life work we did for Carta’s HR integrations migration, we’ve looked at some ways that we can move our code from those bottom two quadrants closer to the top. We’ve looked at how we can pay down our tech debt, how can we take code in those leftmost quadrants, move it closer to the right, and how do we build the Paul Rudd of code. I didn’t really tell you like, how did it go? I’m saying we did this HR integrations thing and maybe it blew up two weeks after we launched it.
Thankfully, no. It’s one of our more reliable systems at Carta even still now, and it’s been several years. This code has changed hands several times, moved between different teams, but that resilience has pretty consistently remained. I think a lot of it has to do with the fact that as we were actively designing the system, we were consistently talking about its inevitable demise. How did we use the understanding that someday some poor person was going to have to replace this in order to inform the decision-making of designing it upfront? This is a project I am really excited to be able to say that I had a part in. It’s been quite a few years now. I think that I probably have to call this code vintage at this point. It’s pretty good, I think. I said at the top that the code we’re building today is the legacy code of tomorrow, and that’s true.
If we keep in mind that future developer who someday is going to have to deprecate whatever we’re building today, we can be the author of the vintage code that’s actually pretty nice to work with rather than that deadweight code that’s unwieldy and confusing. You never know. The future developer whose day you might be saving, it just might be you.
Questions and Answers
Participant 1: I really agree with the point you made about wrong comments being worse than no comments. I was just wondering if you had any suggestions for tools or processes that could help keep comments accurate and up to date?
Martell: I have seen a linter in my life that I think was at best directionally correct, but specifically wrong. I do not know of any tooling other than code reviews. The other tool I think about is like, write a comment that’s hard to become a lie. If you’re describing why you’re doing what you’re doing, unless you change the why, then that’s still true. I think it’s much harder when you have a comment that describes the how, because you might change the how seven times, and that’s harder to keep that comment up to date. If you write a comment that’s not particularly interested in the specific implementation, it’s just going to age better.
Participant 2: I wanted to see what you feel about to-do comments. Because they pop up a lot, and if you check legacy code, obviously it’s riddled with to-do codes, 7-year, 8-year to-do codes. How do you feel it’s the best approach to those to-do comments. Is it worth revisiting? Is it worth removing? Because they do have some history attached to them.
Martell: To a certain degree, it does depend. It depends on the culture of your organization, and like, how much are you lying to yourself? Every to-do comment is a little bit of a lie. It’s just a spectrum of how much of a lie it is. I think that ideally what we leave for ourselves is an artifact to help us understand why we got here and what potential future might look like more than a specific to-do comment. I have seen some organizations that are quite studious about their to-do comments and have linters that figure out how old they are and send you a nasty Slack message to you and your boss, and then eventually to the CEO that’s like, “Shawna said two weeks ago that she was going to do this and she didn’t and she’s in trouble now”. Does that work everywhere? Almost certainly not. Does it work some places? Probably yes. I think the important part when we’re thinking about what artifact are we leaving in our code to help future us, is that whatever your organization is going to find the most useful is the right thing. The day that your to-do comment can go to kindergarten, let’s just delete it and move on with our lives. I think we’re lying to ourselves at that point.
See more presentations with transcripts
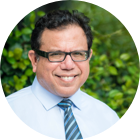
MMS • Ben Linders
Article originally posted on InfoQ. Visit InfoQ
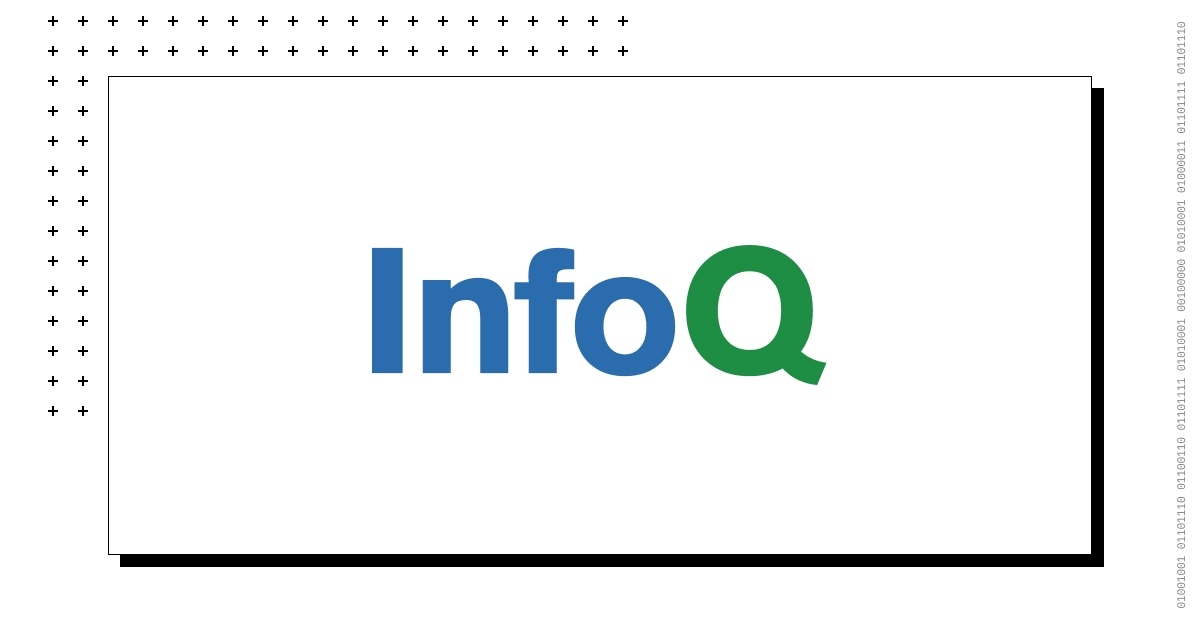
A rigid hierarchical dynamic between senior and junior software engineers can stifle innovation, discourage fresh perspectives, and create barriers to collaboration. According to Beth Anderson, senior engineers can actively learn from their junior counterparts. She suggests creating an environment of mutual growth, psychological safety, and continuous learning.
Beth Anderson spoke about how senior software engineers can learn from juniors at QCon London.
Often power dynamics focus on senior engineers passing information to more junior engineers, expecting them to approach engineering tasks similarly as they do, Anderson said. Passing information along is a potentially missed opportunity for a more meaningful conversation between senior and junior engineers, where senior engineers can learn new approaches and consider issues and solutions from juniors:
A high power distance can create an environment in which junior engineers are afraid of speaking up when they see an issue that causes a much larger problem, where junior engineers aren’t listened to and don’t feel valued.
Seniors can learn from junior engineers, who are often very highly motivated, and have a fresh perspective and an up-to-date set of skills. We shouldn’t have a fixed idea of who we can learn from, based on a hierarchy or on seniority, but instead celebrate curiosity and create an environment where seniors focuses on supporting junior engineers, not controlling them or shutting them down, Anderson said.
To cultivate an inclusive engineering culture, Anderson suggested active listening, amplifying people’s voices, and valuing their input. Senior engineers need to constantly be aware of how they’re interacting with junior engineers and ensuring they lift them up, not keep them down:
Curating a place of psychological safety in which junior engineers feel comfortable and empowered to speak up and ask questions is critical.
To create an environment where engineers can learn and grow, and feel psychological safety, Anderson suggested implementing “reverse feedback”, allowing junior engineers to speak up and provide feedback about how the senior engineer is communicating with them.
She mentioned asking junior engineers how they prefer to learn and communicate and listen to their ideas with an open and willing mind.
It’s more difficult for junior engineers to affect culture in an organization, although being open with how you prefer to learn is a good way to start, Anderson suggested:
Juniors can ask to review senior engineers’ code and/or pull requests as a learning tool, providing feedback to senior engineers.
Anderson advised junior engineers to be mindful of behaviors they’ve learned from others, and avoid repeating the ones they found difficult as juniors. Seniority isn’t about power, it’s an opportunity to create positive change.
Regardless of their level of work experience, each person has a unique insight and something to teach you if you’re prepared to listen actively and give them an environment in which they feel comfortable and valued. Junior engineers are the perfect people to ask why things are done the way they are, and for senior engineers to take that as an opportunity to reflect, Anderson concluded.
InfoQ interviewed Beth Anderson about learning from junior engineers.
InfoQ: What issues can arise from a high power distance between engineers?
Beth Anderson: At my first company, I remember making a mistake and being berated by a senior engineer. At the time I felt like I’d failed, although there was no way I could have known how to do it differently.
Had the senior engineer understood our different experiences, the outcome could have been a positive learning exercise.
InfoQ: How can we cultivate an empowering engineering culture?
Anderson: Think back to our early days as engineers and remember how we had ideas but looked for some help implementing them. A bad senior engineer would dismiss ideas whereas a great senior engineer would listen, value, and empower that junior colleague.
While I have learned from every senior engineer I’ve worked with, the engineers who have helped me improve the most have been people who listened to what I had to say with an open and willing mind.
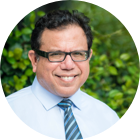
MMS • RSS
Posted on mongodb google news. Visit mongodb google news
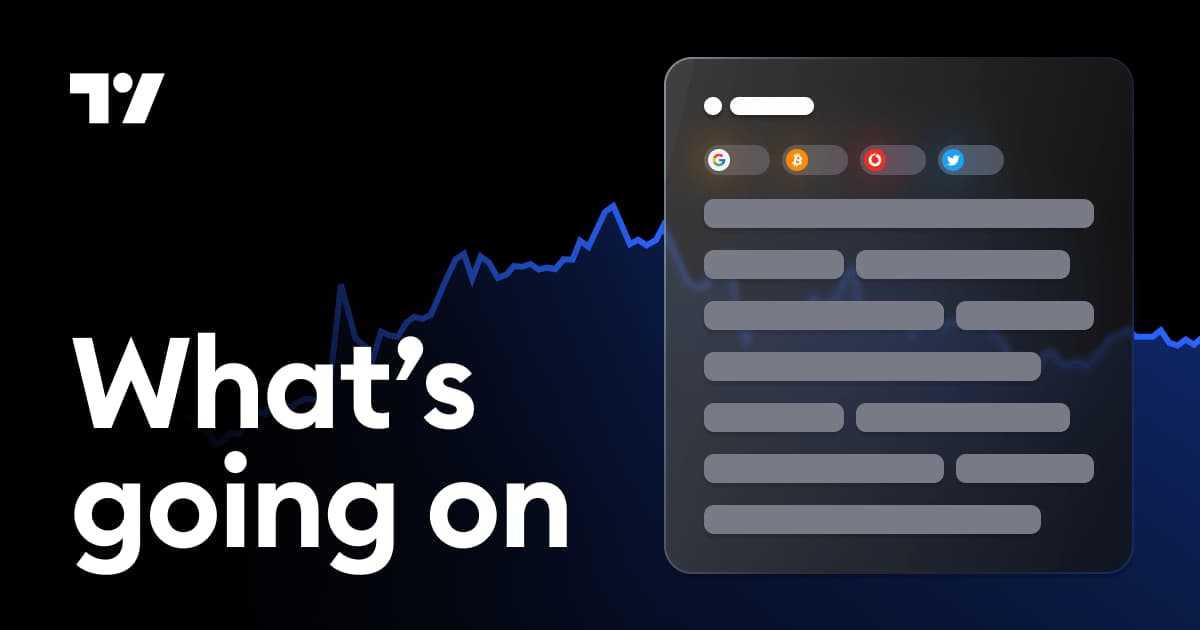
MongoDB MDB shares have plunged 54.1% in the trailing 12 months, underperforming the Zacks Computer and Technology sector, the S&P 500 index and the Zacks Internet – Software industry’s return of 3.1%, 6.9% and 10.4%, respectively.
The company is facing a challenging near-term landscape due to an uncertain macroeconomic climate and rising competitive pressures. The stock has also been swept up in the broader market sell-off, driven by concerns over reduced consumer demand and the trade war. Let’s take a closer look at the key factors weighing on the stock, which should serve as a warning for investors.
MDB’s Competition is a Challenge
MongoDB faces tough competition from companies like Amazon’s AMZN DynamoDB, Couchbase BASE and Oracle ORCL, among others. Amazon DynamoDB is a fast NoSQL database, ideal for apps needing quick reads and writes with real-time data access. Oracle Database offers strong indexing and query optimization, making it great for fast and efficient data retrieval. Couchbase performs well in high-speed, offline, and mobile-first environments. It also has built-in caching for faster data access and uses an SQL-like query language. Shares of Oracle have gained 10.1% in the trailing 12 months, while Amazon and Couchbase have lost 2.7% and 40%, respectively.
This competitive landscape puts pressure on MongoDB’s market share and growth potential, especially as rivals continue to enhance performance and integration features. With stronger-performing peers gaining market share, MDB’s positioning appears increasingly vulnerable.
MongoDB, Inc. Price and Consensus
MongoDB, Inc. price-consensus-chart | MongoDB, Inc. Quote
MDB’s Margins and Growth Trajectory Raise Concerns
MongoDB’s operating margin is expected to decline from 15% in fiscal 2025 to 10% in fiscal 2026, signalling a weakening profitability trend. The drop is largely due to the $50 million high-margin, multiyear license revenues that were incurred in fiscal 2025 and won’t repeat again, and increased spending on R&D and marketing initiatives. These investments, including the $220 million Voyage AI acquisition, are unlikely to drive immediate returns.
Moreover, the company’s gross margin slipped from 77% to 75% in fiscal 2025, as Atlas, MongoDB’s lower-margin cloud product, now accounts for 71% of revenues. Despite its scale, Atlas’ growth is expected to remain flat year over year. Meanwhile, the non-Atlas business continues to decline, adding to growth headwinds in fiscal 2026.
MDB Offers Disappointing Fiscal 2026 Guidance
For fiscal 2026, the company projects revenues between $2.24 billion and $2.28 billion, reflecting a modest 12.4% year-over-year growth at the midpoint, down sharply from 19% year-over-year growth recorded in fiscal 2025. The Zacks Consensus Estimate for revenues is pinned at $2.26 billion, implying year-over-year growth of 12.88%.
The consensus mark for earnings is pegged at $2.66 per share, which has been revised downward by 2.2% over the past 30 days, indicating a year-over-year decline of 27.32%.
MDB beat the Zacks Consensus Estimate for earnings in each of the trailing four quarters, with the average surprise being 62.04%.
Find the latest EPS estimates and surprises on Zacks Earnings Calendar.
Conclusion: Time to Sell MDB Stock
Given the intense competition, pressured margins, weaker revenue guidance and broader market pressures, it appears prudent for investors to sell MongoDB stock for now.
The company’s non-Atlas revenues are declining, free cash flow has nearly halved, and operating margins are under pressure. With high R&D spending offering limited short-term returns and Atlas growth stalling, MongoDB faces too many headwinds to justify holding the stock in the near term. MDB currently carries a Zacks Rank #4 (Sell), suggesting that it may be wise for investors to stay away from the stock for the time being.
You can see the complete list of today’s Zacks #1 Rank (Strong Buy) stocks here.
This article originally published on Zacks Investment Research (zacks.com).
Zacks Investment Research
Article originally posted on mongodb google news. Visit mongodb google news
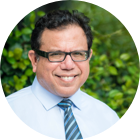
MMS • RSS
Posted on mongodb google news. Visit mongodb google news
Summary:
- Stifel maintains a Buy rating for MongoDB (MDB, Financial) with a revised target price of $275.
- Analysts predict a significant upside for MDB, with an average target price of $297.36.
- GuruFocus estimates suggest a 168.2% upside based on the GF Value metric.
Stifel has reaffirmed its Buy rating on MongoDB (MDB), although it has adjusted the target price to $275, citing increased competition from PostgreSQL. Despite this competitive pressure, a substantial 80% of surveyed customers anticipate maintaining consistent growth in their database usage, utilizing both technologies for different workloads. MongoDB’s valuation presents an attractive opportunity for long-term investors.
Wall Street Analysts’ Forecast
According to price targets from 34 analysts, MongoDB Inc (MDB, Financial) is expected to reach an average price target of $297.36 within a year. This includes a high estimate of $520.00 and a low of $180.00. The average target implies a robust upside of 84.16% from the current market price of $161.47. For more detailed projections, please visit the MongoDB Inc (MDB) Forecast page.
Consensus from 38 brokerage firms places MongoDB Inc (MDB, Financial) at an average recommendation of 2.0, signaling an “Outperform” status. The rating system ranges from 1 to 5, where 1 is a Strong Buy and 5 is a Sell.
According to GuruFocus, the estimated GF Value for MongoDB Inc (MDB, Financial) is projected to be $433.07 in one year. This indicates a potential upside of 168.2% from the current price of $161.47. The GF Value metric reflects GuruFocus’ valuation of the stock, derived from historical trading multiples, past growth, and future business projections. Additional information is available on the MongoDB Inc (MDB) Summary page.
Article originally posted on mongodb google news. Visit mongodb google news
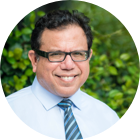
MMS • Craig Risi
Article originally posted on InfoQ. Visit InfoQ
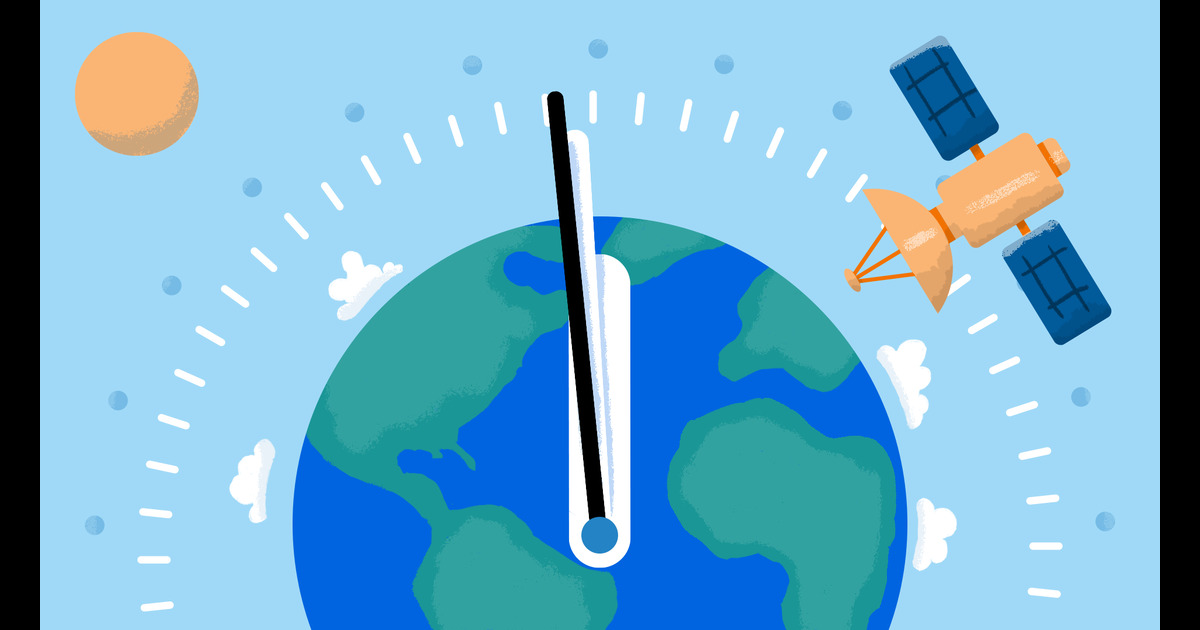
Many systems rely on precise and consistent timekeeping for coordination, logging, security, and distributed operations. Even a one-second discrepancy can cause failures in time-sensitive processes such as financial transactions, database replication, and scheduled tasks. For systems that require strict synchronization—like distributed databases, telemetry pipelines, or event-driven architectures—handling leap seconds incorrectly can lead to data loss, duplication, or inconsistencies. As such, managing leap seconds accurately ensures system reliability and consistency across environments that depend on high-precision time.
For those unfamiliar with the concept leap seconds are periodic adjustments added to Coordinated Universal Time (UTC) to account for irregularities in Earth’s rotation, ensuring that atomic time remains synchronized with astronomical time. While necessary for precise timekeeping, these adjustments can pose challenges for systems requiring high-precision synchronization, such as those utilizing the Precision Time Protocol (PTP). PTP is designed to synchronize clocks within a network to sub-microsecond accuracy, making the handling of leap seconds particularly critical.
Traditional methods of handling leap seconds, such as smearing—where the extra second is spread over a period to minimize disruption—are often employed in Network Time Protocol (NTP) systems. However, applying similar techniques in PTP systems is problematic due to their higher precision requirements. Even minimal adjustments can lead to synchronization errors, violating the stringent accuracy standards PTP aims to maintain.
To address this, Meta has developed an algorithmic approach that is integrated into their PTP service. This method involves a self-smearing technique implemented through the fbclock library, which provides a “Window of Uncertainty” (WOU) by returning a tuple of time values representing the earliest and latest possible nanosecond timestamps. During a leap-second event, the library adjusts these values by shifting time by one nanosecond every 62.5 microseconds. This stateless and reproducible approach allows systems to handle leap seconds automatically without manual intervention.
This self-smearing strategy offers several benefits, including seamless handling of leap seconds and maintaining the high precision required by PTP systems. However, it also introduces trade-offs. For instance, discrepancies can arise when integrating with systems that use different smearing methods, such as NTP’s quadratic smearing, potentially leading to synchronization issues during the smearing period.
Managing leap seconds in high-precision environments like those utilizing PTP requires innovative solutions to maintain synchronization accuracy. Meta’s algorithmic approach exemplifies how tailored strategies can effectively address the challenges posed by leap seconds, ensuring the reliability and precision of time-sensitive systems.