Author: Anthony Alford
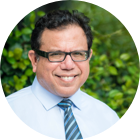
MMS • Anthony Alford
Article originally posted on InfoQ. Visit InfoQ
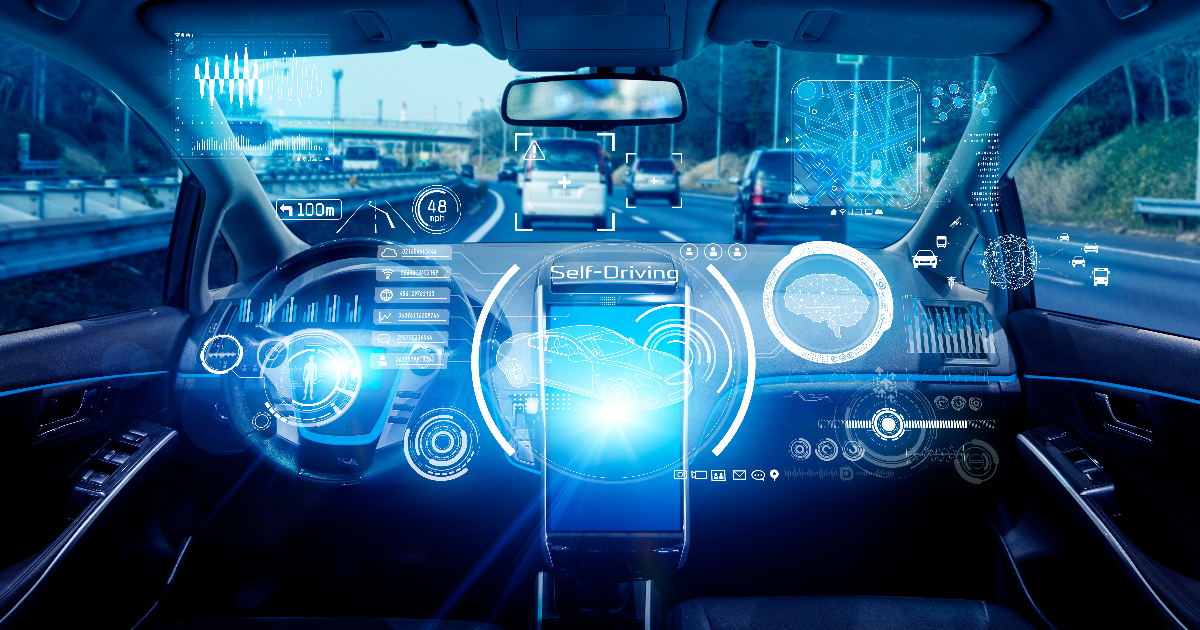
The recent Ai4 2023 conference featured a talk by Hussein Mehanna of Cruise titled “How Autonomous Vehicles Will Inform And Improve AI Model Testing.” Some key takeaways are that systems should handle the “long tail,” developers should measure model output quality, and developers should push their systems to fail.
Mehanna, Senior VP and Head of AI/ML at Cruise, began with the problem statement that while generative AI has great potential, its output is often unreliable; for example, language models are subject to hallucination. However, he believes that his company’s experience with deploying autonomous vehicles offers several lessons which can help improve the reliability of generative AI. Mehanna began by recounting some metrics about Cruise’s business—their cars have driven autonomously more than 3 million miles—then played a short video showing autonomous vehicles encountering and safely navigating several unexpected situations, such as pedestrians or cyclists darting in front of the vehicles.
Mehanna then shared several use cases of generative AI at Cruise. The company generates synthetic images to use as training data for their autonomous driving models; they generate both single frame scenes for their perception models as well as “adversarial” scenarios testing the autonomous behavior of the vehicle. In the latter instance, Mehanna gave the example of a pedestrian darting in front of the vehicle: with generative AI, Cruise can create a variation of that scenario where the pedestrian trips and falls. Mehnna also said that the vehicle software uses an equivalent of a language model to predict the motion of other vehicles.
He then delved into lessons for making generative AI more reliable. The first lesson is to handle the long tail; that is, to have an explicit strategy for when the model encounters very rare cases. He said that there is a misconception that human intelligence is more robust than AI because it has a better understanding of the world. Instead, he suggested that when encountered with an unexpected situation when driving, humans change their behavior, becoming more cautious. The key, then, is to know when the AI model encounters epistemic uncertainty; that is, to “know when they don’t know.”
The second lesson is to measure the quality of the model’s output. Mehanna admitted this is “much easier said than done,” but recommended against using simple aggregates. He gave the example of Cruise measuring their vehicles’ performance around pedestrians. On average, the performance is excellent; however, when measured by cohorts, that is, by age group of pedestrians, performance in the presence of pedestrian children is not good. He noted that it may take multiple iterations to find good quality measures. He also suggested that in many cases, so-called bias in AI models is actually a measurement problem of looking at aggregates instead of cohorts.
Finally, Mehanna encouraged developers to “push your system to the limits” and observe that quality metric in a lot of very different use cases. He mentioned that Cruise generates synthetic data for adversarial testing of their models. In these scenarios, they have control over all the objects and their behavior; they can, for example, add a pedestrian or make a human-driven car stop suddenly.
Mehanna concluded by saying, “You need to build a system of trust so that you can deploy generative AI—or any AI system—safely. And I believe we can learn a lot from autonomous vehicles.”
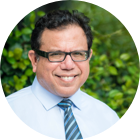
MMS • Anthony Alford
Article originally posted on InfoQ. Visit InfoQ
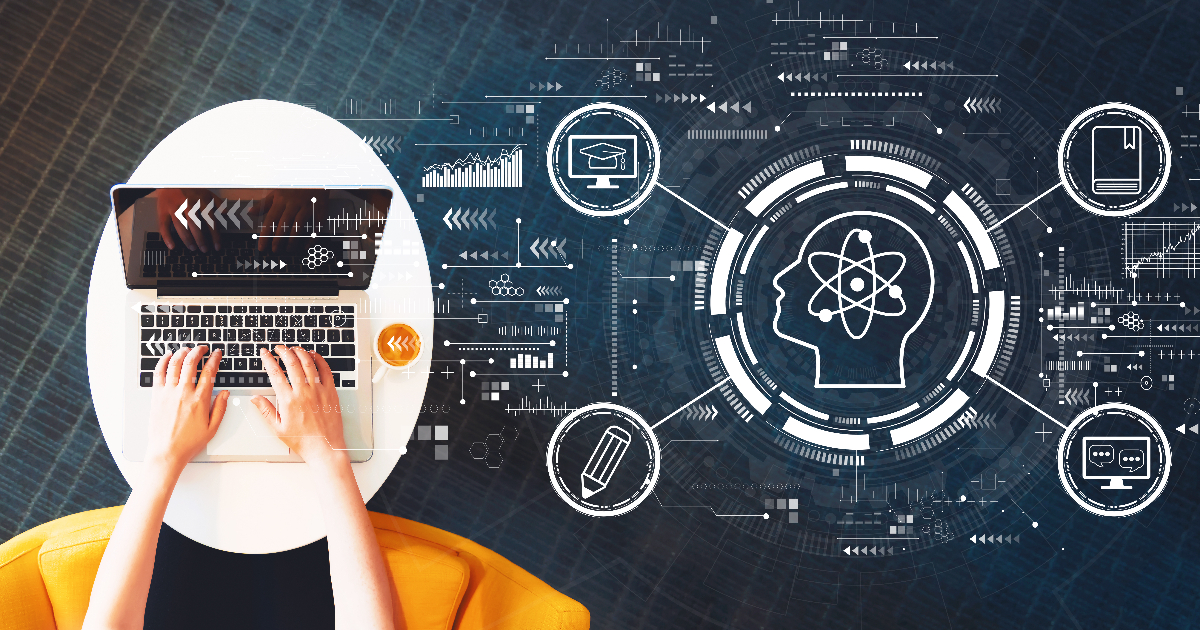
The recent Ai4 conference featured a panel discussion titled “Generative AI in Business and Society.” Some key takeaways are that generative AI offers many opportunities for operational efficiency and product personalization, that companies need to balance privacy concerns with personalization, and they need to understand how generative AI is used across their organization.
The panel was moderated by Aaron Rosenberg, partner at Radical Ventures. Panelists included Alon Yamin, CEO and co-founder of Copyleaks; Chrissie Kemp, chief data and digital product officer at Jaguar Land Rover; Mark Stutzman, CTO at AREA15; and Eiman Ebrahimi, CEO at Protopia AI. The panelists discussed questions about generative AI posed by Rosenberga.
Rosenberg began by asking Stutzman about the use of generative AI at his company, which provides immersive entertainment to its guests. Stutzman said that AREA 15 uses ChatGPT for “a lot of boring stuff,” such as their customer service chatbot, where it resolves “close to 85 to 90%” of guest questions. They also used a generative image AI to create images for theming a new restaurant at their venue, which he described as “like walking into a video game.” He also hinted at future plans for personalized interactive experiences generated dynamically by AI.
Next Rosenberg asked Kemp what adoption of generative AI looked like for a large enterprise such as Jaguar Land Rover. Kemp replied that they were being “cautious,” especially with respect to security and privacy. However, she said that adopting generative AI would allow them to deliver more personalized in-vehicle services, calling out the company’s partnership with NVIDIA. She also said that in the enterprise itself there would be “huge opportunities to drive productivity and efficiency.”
Rosenberg then asked Ebrahimi how his company, Protopia AI, and others like it are enabling enterprises to adopt generative AI. Ebrahimi noted that one of the biggest challenges is how to properly handle sensitive data. He called back to AREA 15 and Jaguar Land Rover both wanting to provide personalized experiences, but needing to balance collecting the personal data needed for that with privacy concerns. He referred to his company’s product, which transforms data into a form that’s not human-understandable, so that it can be used by machine learning algorithms while preserving privacy.
Rosenberg next asked Yamin what generative AI concerns he was seeing and how to address them. Yamin replied that he saw “amazing excitement” about opportunities in enterprises, along with worry about how to mitigate risks. He pointed out that many enterprises do not have a full picture of where generative AI is already being used within their organization. He recommended that companies define policies around the use of generative AI and build tools to enforce the policies. He also recommended they check the accuracy of the output of models even closer than they would human-created content.
Rosenberg then asked each panel member for “one piece of practical advice” on how organizations could experiment with generative AI. Stutzman said, “play with it, [but] be careful.” Yamin reiterated the need to know how generative AI was used across the organization, along with a need for clear policies. Kemp advised “invest your data,” as models are only as good as the data used to train them. Ebrahimi cautioned against hoping that “the legal system is going to come save us,” and instead recommended looking for technological solutions to privacy and compliance problems.
Finally, Rosenberg asked the panelists what they were most excited about when thinking about the future of generative AI. For Ebrahimi, it was personalized health care. Kemp predicted “another renaissance era in terms of creativity,” particularly in art. Yamin was excited about education, noting that there was already visible progress in the field. Stutzman seconded Ebrahimi’s excitement on health care, but added that he predicted a fully-automated marketing tech stack. Rosenberg concluded by sharing his own excitement about AI’s potential for advancing biology and physics.
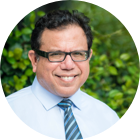
MMS • Anthony Alford
Article originally posted on InfoQ. Visit InfoQ
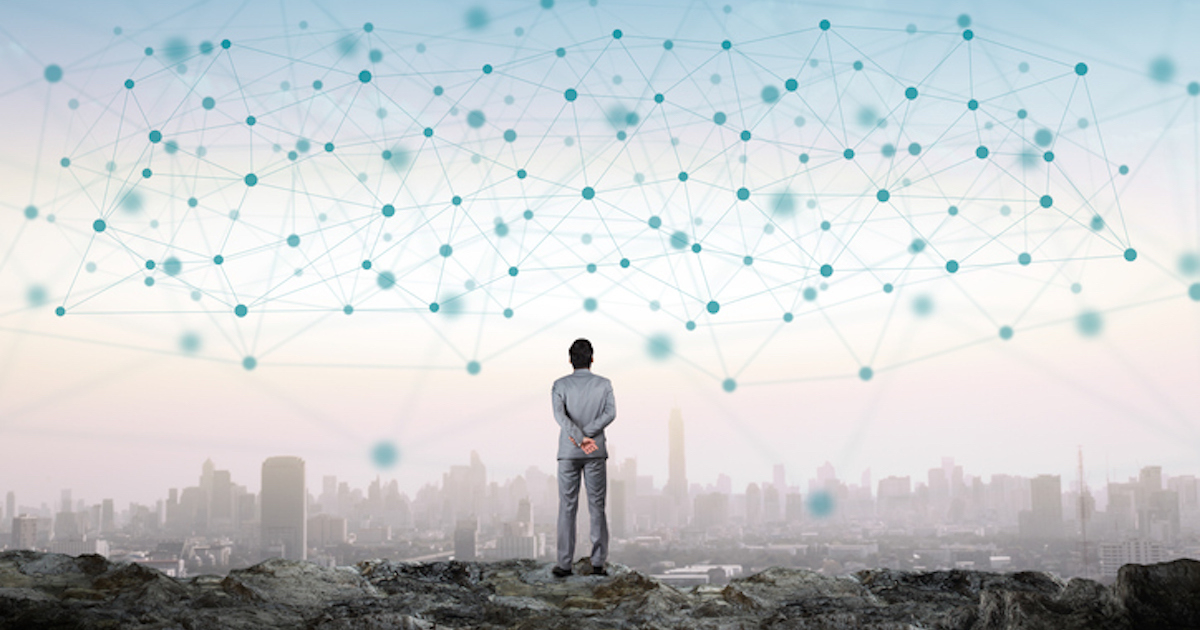
Day Two of Ai4 2023 conference was held on August 9th, 2023, at the MGM Grand hotel in Las Vegas, Nevada. This two-day event is organized by Fora Group and includes tracks focused on various industries, including automotive, financial, healthcare, and government. The day began with six mainstage presentations from leaders in AI technology.
The first talk was a fireside chat on “Navigating The Legal Complexities Of Cutting Edge AI,” between Che Chang, General Counsel at OpenAI and Ksenia Semenova, Founder and Editor-in-Chief of Turing Post. Semenova asked Chang several questions about the global legal landscape surrounding AI, and particularly generative AI. Chang noted that AI models, at their core, are simply using historical data to make a prediction; when looked at this way, “you understand…90% of what you need to think about from a regulatory perspective.” He also noted that “AI” is just another term for “any behavior that a machine can do, that a person can do,” and since there is no single law for regulating human behavior, it would not make sense to have a single regulation for machine behavior.
Next up was Aaron Cheng, Vice President of Data Science and Solutions at dotData, Inc., speaking on “The Million-Dollar Problem: How To Make My Data Ready For AI?” Cheng’s main thesis was that although raw data is very valuable, it is not ready for use in machine learning; he made the analogy of raw data to crude oil and “AI-ready” data to gasoline. The refining process used to get AI-ready data is feature engineering. He ended his talk with a case-study of a customer using his company’s feature engineering platform.
The third talk was “Harnessing AI For Education So All Students Benefit,” presented by Sal Khan, Founder and CEO of Khan Academy. Khan began with a recap of his company’s founding story, and then discussed Benjamin Bloom’s Two-Sigma Problem, which shows how one-on-one tutoring can give students a two standard deviation improvement in academic performance. Khan now believes that generative AI is almost good enough to approximate a one-on-one tutor, which could give every student this advantage. Although he was initially “bummed” by the news headlines about cheating which accompanied the release of ChatGPT at the end of 2022, he soon came to realize it was a positive development, since it “forced people to grapple with” the challenges of using generative AI in education. Khan concluded his talk with demo videos of Khan Academy’s new AI assistant, Khanmigo.
The next speakers were Jim Rowan, Principal at Deloitte, and Jatin Dave, Senior Manager at Deloitte, speaking on “Establishing An AI Center Of Excellence.” They noted that a majority of companies have not figured out how to generate value from their AI investments, and their thesis was that an AI Center of Excellence (CoE) would help companies achieve that value. They listed four standard operating principles: a plan for embedding AI in the core business; focus on observable business impact; a comprehensive view of the AI tech stack; and a lookout for external disruptions. They also listed four pitfalls: lack of shared vision across business units; lack of executive sponsorship; the AI CoE in a support role instead of leading; and incoherent metrics for the CoE.
Next up, Solmaz Rashidi, Chief Analytics Officer at The Estée Lauder Companies, spoke on “The Good, Bad, And Realities Of Deploying AI Projects Within Enterprises.” Rashidi began with statistics about AI initiatives and potential economic impact. She then shared a flowchart that executives could use to identify if a technology truly is AI. She concluded with an eight-point framework for enterprise AI deployments.
The final talk was another fireside chat, “The Past, Present, And Future Of Enterprise AI,” between Igor Jablokov, CEO of Pryon and Scott Pobiner, Head of UX Strategy, AI and Data Practice at Deloitte. Starting with the past, Jablokov recounted his previous efforts in AI, including developing technology used by Amazon Alexa and IBM Watson. He pointed out that the latest generative AI models are “nothing new,” they simply have finally gotten attention from normal people. He also lamented that internet search results, which used to return “innovative creations of other fellow human beings,” would soon become a “hall of mirrors” of AI-generated pages. He also cautioned against adoption of models such as Llama, which appear to be open-source, but do in fact have several restrictions on their use.
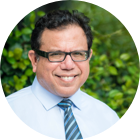
MMS • Anthony Alford
Article originally posted on InfoQ. Visit InfoQ
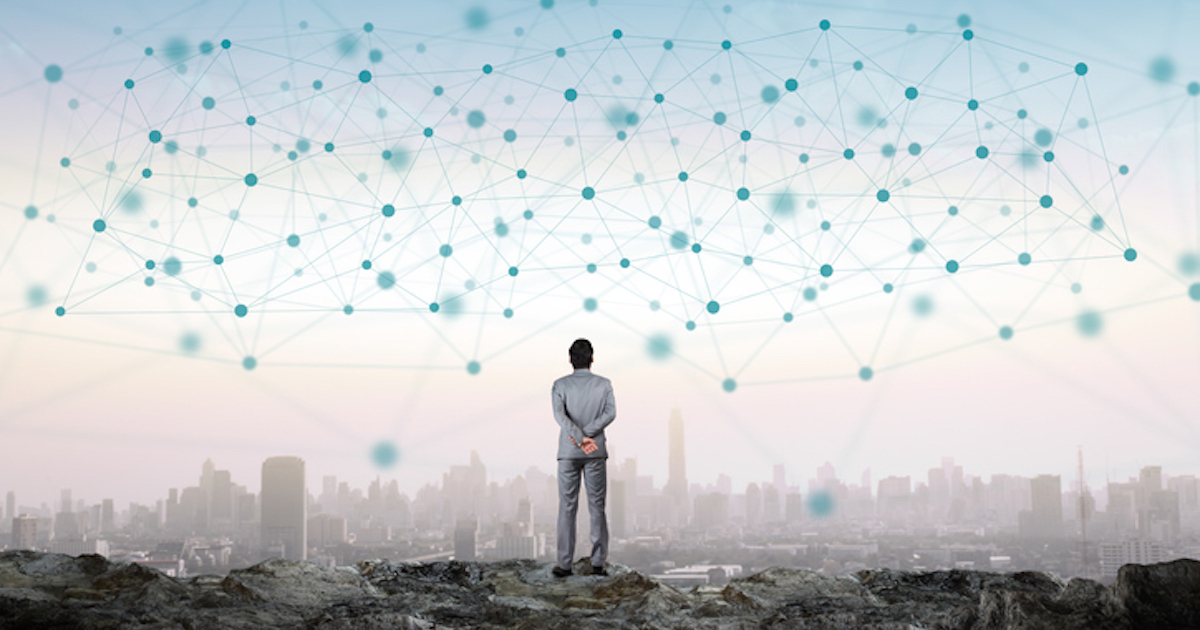
Day One of the Ai4 2023 conference was held on August 8th, 2023, at the MGM Grand hotel in Las Vegas, Nevada. This two-day event is organized by Fora Group and includes tracks focused on various industries, including automotive, financial, healthcare, and government. The day began with six mainstage presentations from leaders in AI technology.
The first speaker was Nikola Todorovic, Co-Founder and CEO of visual effects firm Wonder Dynamics. Todorovic’s talk was “AI Through The Lens Of Filmmaking,” which began with a short history of filmmaking, framing the theme that advances in the industry were often driven by increasing accessibility, to both the filmmakers and the audience. Todorovic’s company uses AI to advance that goal of increasing accessibility, reducing the cost for filmmakers to use CGI effects in their films by automating much of the “grunt work” these effects require, “up to 80 to 90% in some instances.”
Next up was Conor Jensen, Americas Field CDO at Dataiku. In his talk “Natural Selection In Everyday AI,” Jensen gave three examples of successful adaptations that companies make when successfully adopting AI, and three “evolutionary dead ends.” The successful adaptations are: building a “data science lifecycle” process of deciding what projects to work on; build an AI friendly culture, from both top-down and bottom-up in the organization; and investing in talent at all levels of the organization, which includes training front-line workers to interpret AI model output. The dead ends were: overly complex tech stacks; ineffective organizational structures; and siloed people and data.
Joann Stonier, EVP and Mastercard Fellow of Data and AI, followed with a talk on “Next Generation Innovation: A Responsible Road Map For AI.” Her roadmap consisted of eight components: principles, governance, data examination, analytics and risk, outcome assessment, interaction with LLMs, distance and evaluation, and review boards and committees. The fundamental principle of this roadmap is that “an organization’s data practices must be guided by the rights of individuals.” Furthermore, the higher the risk of a possible negative outcome from using an AI model, the more “distance” there should be between the model’s output and the actual outcome; she gave an example of a person being accused of a crime based solely on a facial recognition model output.
Arijit Sengupta, Founder and CEO of Aible, and Daniel Lavender, Senior Director of Advanced Analytics Insights and Architecture at Ciena, gave a case study of Ciena’s adoption of Aible’s generative AI platform. Aible’s platform introduces the “information model,” the equivalent of the vector database for structured data. It also uses an explainable AI to “double-check” generated natural language statements against actual data, and presents users with charts of real data that are linked to natural language statements.
Next on the stage was a “fireside chat” on “Cracking An Outdated Legal System With AI” between Brandon Deer, Co-founder and General Partner at Crew Capital and Joshua Browder, CEO of DoNotPay. Browder’s company began as a simple repository of template letters to help users dispute traffic and parking citations; now the company uses generative AI agents to automate over 200 consumer rights processes. Browder noted that DoNotPay uses the open-source GPT-J model for generative AI because OpenAI “wouldn’t be happy” with some of their use cases. Browder drew applause toward the end of his talk when he mentioned that his company offers a product that can help users sue robo-callers.
The final talk was Luv Tulsidas, Founder and CEO of Techolution, on “Building The Enterprise Of Tomorrow With Real-World AI.” Tulsidas noted that according to Forbes, 91% of companies are investing in AI, but fewer than 1% of AI projects are providing RoI. To address this, Tulsidas offered five “secrets” of AI: any commercial AI product will only solve about 80% of your business use cases; you should focus only on specific-purpose AI projects; there are four categories of AI, from lab-only to fully-autonomous; that companies should create AI centers of excellence consisting of six core personas; and finally, that autonomous AI requires reinforcement learning with expert feedback (RHEF).
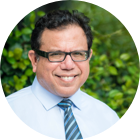
MMS • Anthony Alford
Article originally posted on InfoQ. Visit InfoQ
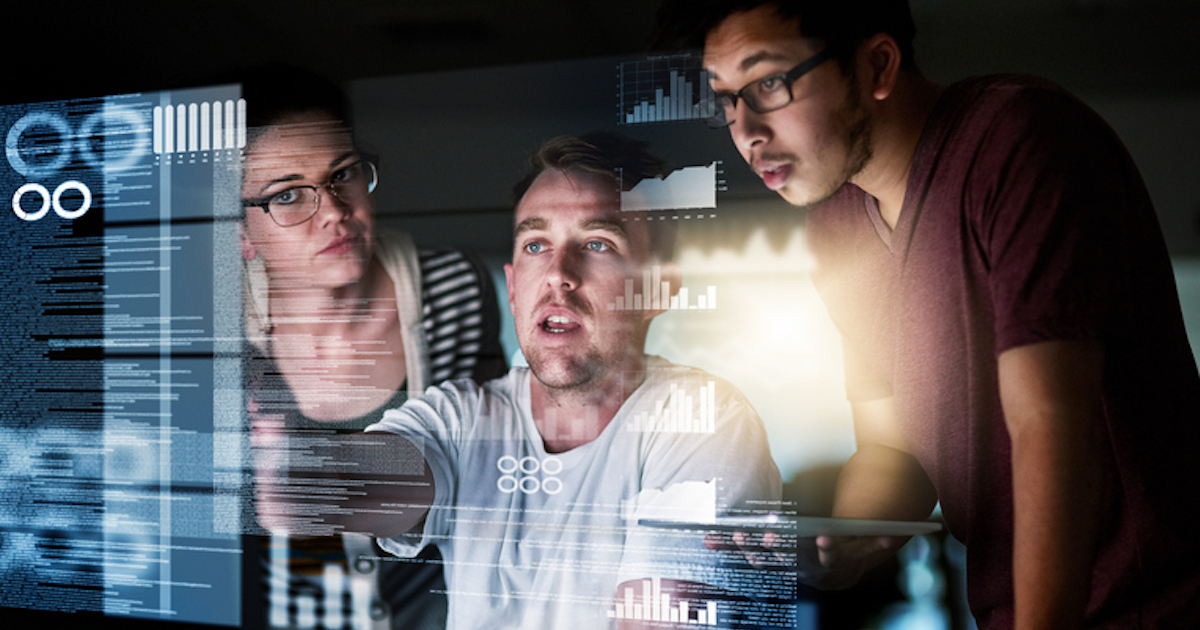
Researchers from Carnegie Mellon University (CMU) have published LLM Attacks, an algorithm for constructing adversarial attacks on a wide range of large language models (LLMs), including ChatGPT, Claude, and Bard. The attacks are generated automatically and are successful 84% of the time on GPT-3.5 and GPT-4, and 66% of the time on PaLM-2.
Unlike most “jailbreak” attacks which are manually constructed using trial and error, the CMU team devised a three-step process to automatically generate prompt suffixes that can bypass the LLM’s safety mechanisms and result in a harmful response. The prompts are also transferrable, meaning that a given suffix will often work on many different LLMs, even closed-source models. To measure the effectiveness of the algorithm, the researchers created a benchmark called AdvBench; when evaluated on this benchmark, LLM Attacks has an 88% success rate against Vicuna, compared to 25% for a baseline adversarial algorithm. According to the CMU team:
Perhaps most concerningly, it is unclear whether such behavior can ever be fully patched by LLM providers. Analogous adversarial attacks have proven to be a very difficult problem to address in computer vision for the past 10 years. It is possible that the very nature of deep learning models makes such threats inevitable. Thus, we believe that these considerations should be taken into account as we increase usage and reliance on such AI models.
With the release of ChatGPT and GPT-4, many techniques for jailbreaking these models emerged, which consisted of prompts which could cause the models to bypass their safeguards and output potentially harmful responses. While these prompts are generally discovered by experimentation, the LLM Attacks algorithm provides an automated way to create them. The first step is to create a target sequence of tokens “Sure, here is (content of query),” where “content of query” is the user’s actual prompt which is asking for a harmful response.
Next, the algorithm generates an adversarial suffix for the prompt by finding a sequence of tokens that is likely to cause the LLM to output the target sequence, using a Greedy Goordinate Gradient-based (GCG). While this does require access to the LLM’s neural network, the team found that by running GCG against many open-source models, the results were transferrable even to closed models.
In a CMU press release discussing their research, co-auth Matt Fredrikson said:
The concern is that these models will play a larger role in autonomous systems that operate without human supervision. As autonomous systems become more of a reality, it will be very important to ensure that we have a reliable way to stop them from being hijacked by attacks like these…Right now, we simply don’t have a convincing way to stop this from happening, so the next step is to figure out how to fix these models…Understanding how to mount these attacks is often the first step in developing a strong defense.
Lead author Andy Zou, a PhD student at CMU, wrote about the work on Twitter. He said:
Despite the risks, we believe it to be proper to disclose in full. The attacks presented here are simple to implement, have appeared in similar forms before, and ultimately would be discoverable by any dedicated team intent on misusing LLMs.
David Krueger, an Assistant Professor at the University of Cambridge, replied to Zou’s thread, saying:
Given that 10 years of research and thousands of publications haven’t found a fix for adversarial examples in image models, we have a strong reason to expect the same outcome with LLMs.
In a discussion of the work on Hacker News, one user pointed out:
Remember that a big point of this research is that these attacks don’t need to be developed using the target system. When the authors talk about the attacks being “universal”, what they mean is that they used a completely local model on their own computers to generate these attacks, and then copied and pasted those attacks into GPT-3.5 and saw meaningful success rates. Rate limiting won’t save you from that because the attack isn’t generated using your servers, it’s generated locally. The first prompt your servers get already has the finished attack string included — and researchers were seeing success rates around 50% success rate in some situations even for GPT-4.
Code for reproducing the LLM Attacks experiments against the AdvBench data is available on GitHub. A demo of several adversarial attacks is available on the project website.
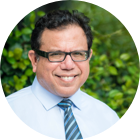
MMS • Anthony Alford
Article originally posted on InfoQ. Visit InfoQ
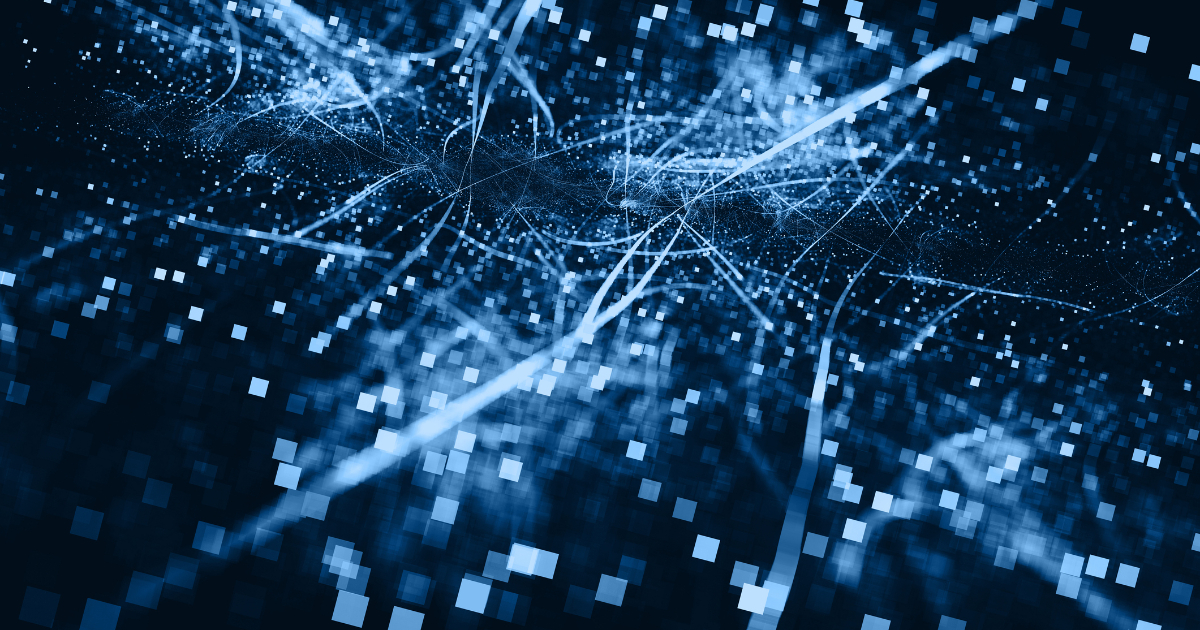
Meta recently announced Voicebox, a speech generation model that can perform text-to-speech (TTS) synthesis in six languages, as well as edit and remove noise from speech recordings. Voicebox is trained on over 50k hours of audio data and outperforms previous state-of-the-art models on several TTS benchmarks.
Unlike many TTS models which are autoregressive, Voicebox is based on a newer architecture called flow-matching. The model is trained to predict masked sections in an audio input, which allows it to perform infilling tasks, such as removing environmental noise from speech recordings or correcting mispronounced words. It can also perform tasks that it was not specifically trained to do, such as cross-lingual style transfer. Voicebox is trained on audiobook recordings paired with their text, recorded in English, French, German, Spanish, Polish, and Portuguese. Although Meta recently open-sourced another multilingual TTS model, the researchers have not done so with Voicebox, citing safety concerns:
There are many exciting use cases for generative speech models, but because of the potential risks of misuse, we are not making the Voicebox model or code publicly available at this time. While we believe it is important to be open with the AI community and to share our research to advance the state of the art in AI, it’s also necessary to strike the right balance between openness with responsibility. With these considerations, today we are sharing audio samples and a research paper detailing the approach and results we have achieved.
Similar to large language models (LLM), Voicebox was trained to predict a masked section of its input. In the case of Voicebox, the input includes a segment of speech audio and its text transcript. A portion of the audio is masked, but the text is not; thus given the text, the model learns to synthesize the audio of the masked words in a way that matches the surrounding audio.
Although Voicebox was trained only on this one task, as with LLMs it can perform in-context learning to perform other tasks. For example, it can perform style transfer: given audio and its transcript, plus additional text to synthesize, it will use the style of the given audio. It can also remove noise: given audio of speech and its associated transcript, along with a mask indicating the noisy section of the audio, the model can resynthesize that section. The Meta team evaluated Voicebox’s performance on several of these tasks, including zero-shot TTS, cross-lingual zero-shot TTS, and text guided denoising. When measuring the mode’s word error rate (WER) and audio similarity, Voicebox outperformed the previous state-of-the-art models VALL-E and A3T.
In an effort to minimize the potential safety risks of the Voicebox model, Meta also developed a classifier model trained to detect synthesized speech. When tested on the Librispeech benchmark, the classifier could “trivially” distinguish the original audio from the benchmark data, vs speech synthesized by Voicebox from the text transcript.
In a Hacker News discussion about Voicebox, one user pointed out Meta’s decision not to release the model, and wondered how difficult it would be to replicate given the size of the training data. Another user replied
Assuming 10 hours [each], 6k books feels a very achievable dataset. Even Librivox claims 18k books (with many duplicates and hugely varying quality levels). If you wanted to get expansive, you could dig into the podcast archives of BBC, NPR, etc which could potentially yield millions of hours.
InfoQ recently covered Massively Multilingual Speech (MMS), a speech model that Meta did open-source. MMS can perform ASR and TTS in over 1k languages; however, it cannot perform tasks such as editing and style transfer that Voicebox can. InfoQ also covered Google’s AudioPaLM model which can perform ASR, TTS, and speech-to-speech translation (S2ST) with voice transfer.
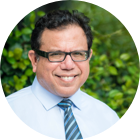
MMS • Anthony Alford
Article originally posted on InfoQ. Visit InfoQ
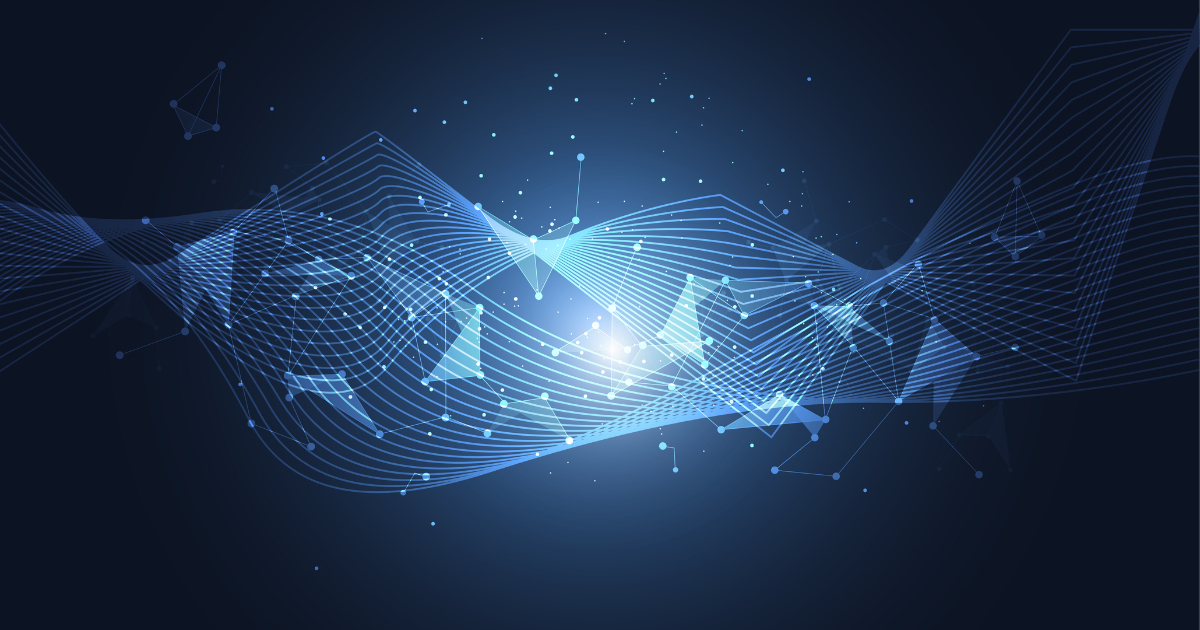
Researchers from UC Berkeley and Microsoft Research have open-sourced Gorilla, a large language model (LLM) that can write code to call APIs. In experiments measuring generated code accuracy, Gorilla outperforms several baseline models, including GPT-4.
Described as “an API appstore for LLMs,” Gorilla is based on the LLaMA open-source LLM. The LLM is finetuned on APIBench, a new dataset of API descriptions of ML models hosted on HuggingFace, TorchHub, and TensorHub. Gorilla can also call out to an external document database of API definitions, which allows it access to new APIs without re-training. Using Gorilla, developers can create natural language descriptions of a problem, such as “Invoke an image classification model that uses less than 10M parameters, but maintains an ImageNet accuracy of at least 70%.” Gorilla would then output the Python code to invoke the appropriate ML model with the proper options. According to the authors,
LLMs are swiftly gaining popularity across diverse domains. In our study, we spotlight techniques designed to enhance the LLM’s ability to accurately identify the appropriate API for a specific task—a significant but often overlooked aspect in the advancement of this technology. Since APIs function as a universal language enabling diverse systems to communicate effectively, their correct usage can boost the ability of LLMs to interact with tools in the wider world.
LLMs like GPT-4 have excellent performance on a wide range of tasks, including generating code. However, their knowledge of APIs is “frozen” at training time, so that they cannot generate code to call newer APIs. Further, they often hallucinate—in the case of code generation, they might output a call to an API that does not exist. InfoQ has covered several recent efforts to address these issues; for example, Meta’s Toolformer which can invoke external service APIS, and ChatGPT’s plugin system that augments the LLM with external resources.
The Berkeley team points out, however, that these approaches are based on prompting the LLM with examples of API calls. By contrast, the Gorilla approach focuses on “systematic evaluation and building a pipeline for future use.” The researchers began by assembling the APIBench dataset. The team first collected all the model cards from the HuggingFace model hub, PyTorch hub, and TensorFlow hub. After filtering, this produced a collection of 1,645 API calls. For each of those, the researchers used GPT-4 to generate a dataset of instruction-api pairs for fine-tuning Gorilla.
A major challenge in evaluating Gorilla’s output was to identify hallucinations. First, the team defined a hallucination as any model output that calls an API not in the model’s external database of API definitions. This is contrasted with an error, which is an output that simply calls a “real” API incorrectly. The team used the abstract syntax tree (AST) of the generated code to match with APIs in the database and test set for evaluation purposes. Using this AST accuracy metric on zero-shot tasks, Gorilla performed 20.43% better than GPT-4.
Gorilla’s lead author Shishir Patil joined a Hacker News discussion about the work, answering several questions. When asked whether the model’s license allowed commercial use, Patil pointed out that there are three versions of Gorilla. The one based on LLaMA is not licensed for commercial use, but the ones based on MPT-7 base and Falcon-7B are. Another user asked how Gorilla compared to LangChain; Patil replied:
Langchain is a terrific project that tries to teach agents how to use tools using prompting. Our take on this is that prompting is not scalable if you want to pick between 1000s of APIs. So Gorilla is a LLM that can pick and write the semantically and syntactically correct API for you to call! A drop in replacement into Langchain!
The Gorilla code and model files are available on GitHub. There is also a Google Colab notebook demo of the model.
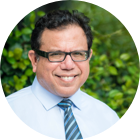
MMS • Anthony Alford
Article originally posted on InfoQ. Visit InfoQ
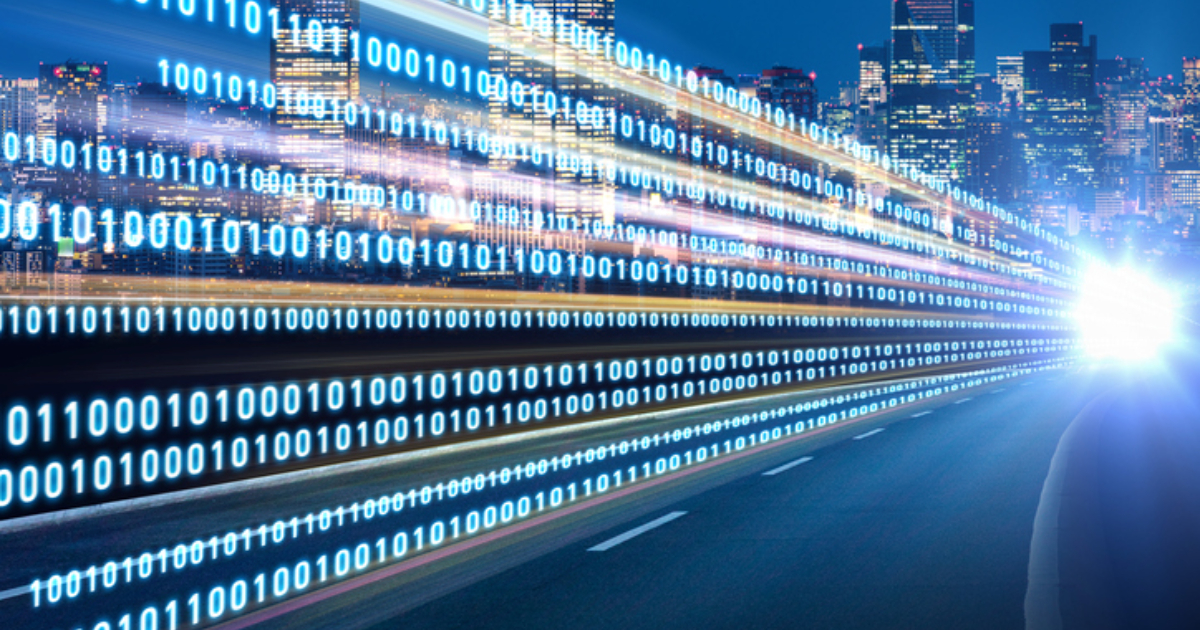
The recent QCon New York conference featured a panel discussion titled “Navigating the Future: LLM in Production.” Some key takeaways are that there are two trends in LLMS: closed models behind APIs and open-source models, and that organizations using LLMs will need to think deeply about testing and evaluating the models themselves, with a strong emphasis on risk mitigation.
The panel was moderated by Bozhao (Bo) Yu. Panelists included Sherwin Wu, a member of technical staff at OpenAI; Hien Luu, Sr. engineering manager at DoorDash; and Rishab Ramanathan, co-founder & CTO of Openlayer. The panelists discussed questions about large language models (LLMs) posed by Yu and fielded a few more from the audience at the end of the session.
Yu began by asking panelists their opinions of the future of LLMs in production and how they will be used. Ramanathan predicted that there would be two broad categories of use: low-risk scenarios, such as internal document retrieval; and higher risk scenarios, where LLMs would likely be used as a “copilot” rather than acting autonomously. Luu referred to a recent blog post by DoorDash’s Head of AI, which identified five usage areas; Luu elaborated on the use case of LLMs as digital assistants. Wu posited that there would be a mix of use cases: calling out to APIs for “closed” foundation models vs. running self-hosted open-source models.
Yu next posed the question of whether operating LLMs (LLMOps) would continue to be a part of MLOps, or if it would be a new discipline. Luu, who manages an MLOps team, thought it would be an extension of MLOps, pointing out that the goal of MLOps is to allow an organization to use ML “quickly, easily and efficiently.” Ramanathan agreed, but thought that there would be components of MLOps that might not be as important.
The next question was what parts of the ML workflow would be kept and what parts might need rethinking, in particular due to the challenges of serving very large models. Luu praised the efforts of the open-source community in researching ways to distribute models across GPUs. Wu suggested the focus would be on the input and output of the pipeline: the input being the data needed to fine-tune models, and the output being careful evaluation of the model output. Ramanathan seconded the need for evaluation, pointing out that consumers of an LLM’s output should “think deeply about testing it and evaluating it themselves.”
Yu concluded by asking the panelists for their “wish list” of LLM developments. Ramanathan, who had previously worked at Apple, wished for assistants such as Siri to gain abilities on par with ChatGPT. Wu wished for more progress on multimodal models as well as improvements in AI safety.
The panelists then answered several questions from audience members. One asked about whether prompt engineering would be a long-term need, or whether models would improve to the point where it was not needed. Wu agreed it was an open question, but speculated prompt engineering would be needed for at least five more years. Ramanathan pointed out that there were open-source libraries to help with prompt generation.
Several audience members asked questions about privacy and regulation, especially in light of the recent EU AI Act. Wu said that OpenAI’s perspective is that they would always follow the law, and would work to improve or fix their models to “have as far reach as possible.” Ramanathan followed up by pointing out that the new Act would require transparency of training datasets; he noted however that the law was rather “handwavy.”
Voxel51 Open-Sources Computer Vision Dataset Assistant VoxelGPT – Q&A with Jason Corso
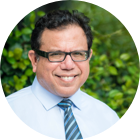
MMS • Anthony Alford
Article originally posted on InfoQ. Visit InfoQ
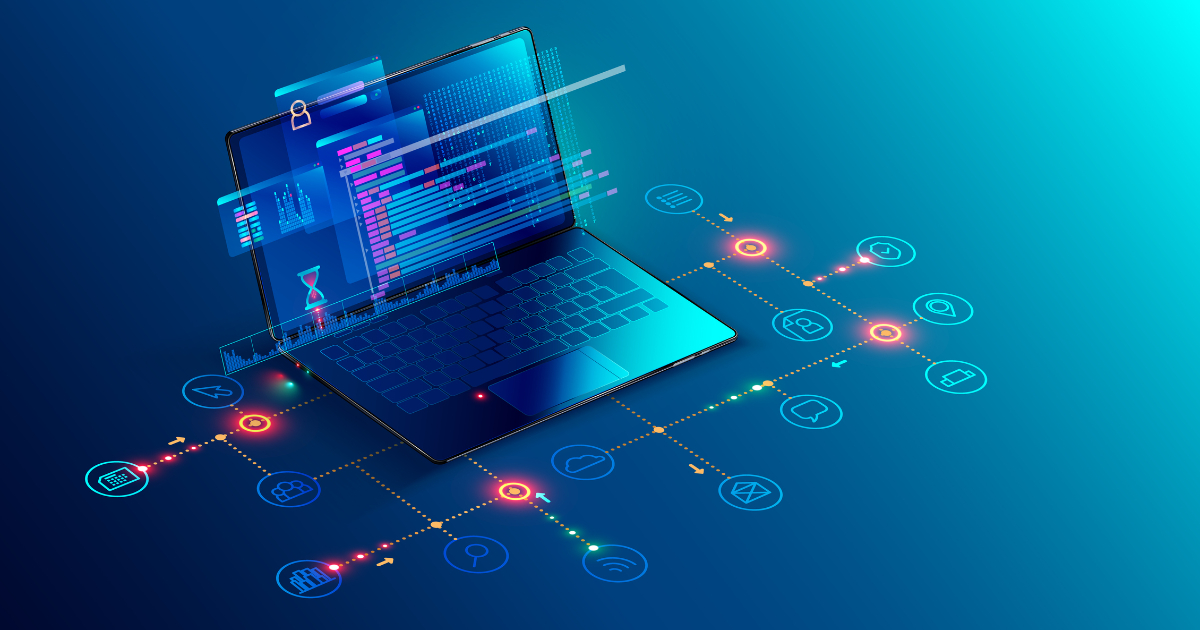
Voxel51 recently open-sourced VoxelGPT, an AI assistant that interfaces with GPT-3.5 to produce Python code for querying computer vision datasets. InfoQ spoke with Jason Corso, co-founder and CSO of Voxel51, who shared their lessons and insights gained while developing VoxelGPT.
In any data science project, data exploration and visualization is a key early step, but many of the techniques are intended for structured or tabular data. Computer vision datasets, on the other hand, are usually unstructured: images or point clouds. Voxel51’s open-source tool FiftyOne provides a query language for exploring and curating computer vision datasets. However, it can be challenging for casual users to quickly and reliably use tools like FiftyOne.
VoxelGPT is a plug-in for FiftyOne that provides a natural language interface for the tool. Users can ask questions about their data, which are translated into Python code that leverages FiftyOne to produce the answers. VoxelGPT uses LangChain and prompt engineering to interact with GPT-3.5 and output the Python code. InfoQ spoke with Jason Corso, co-founder and CSO of Voxel51, about the development of VoxelGPT.
InfoQ: It’s clear that while LLMs provide a powerful natural language interface for solving problems, getting the most out of them can be a challenge. What are some tips for developers?
Jason Corso: A key learning we had while developing VoxelGPT is that expecting one interaction with the LLM to sufficiently address your task is likely a bad idea. It helps to carefully segment your interaction with the LLM to sufficiently provide enough context per interaction, generate more useful piecemeal results, and later compose them together depending on your ultimate task.
A few other lessons learned:
- Start simple, gain intuition, and only add layers of complexity once the LLM is acting as you expect.
- LangChain is a great library, but it is not without its issues. Don’t be afraid to “go rogue” and build your own custom LLM tooling wherever existing tools aren’t getting the job done.
InfoQ: Writing good tests is a key practice in software engineering. What are some lessons you learned when testing VoxelGPT?
Corso: Testing applications built with LLMs is challenging, and testing VoxelGPT was no different. LLMs are not nearly as predictable as traditional software components. However, we incorporated software engineering best practices into our workflows as much as possible through unit testing.
We created a unit testing framework with 60 test cases, which covered the surface area of the types of queries we’d expect from usage. Each test consisted of a prompt, a FiftyOne Dataset, and the expected subset of the dataset resulting from converting the prompt to a query in FiftyOne’s domain-specific query language. We ran these tests each time we made a substantial change to the code or example set in order to prevent regression.
InfoQ: AI safety is a major concern. What were some of the safety issues you confronted and how did you solve them?
Corso: Yes, indeed AI safety is a key element to consider when building these systems. When building VoxelGPT, we were intentional about addressing potential safety issues in multiple ways.
Input validation: The first stop on a prompt’s journey through VoxelGPT is OpenAI’s moderation endpoint, so we ensure all queries passed through the system comply with OpenAI’s terms of service. Even beyond that, we run a custom “intent classification” routine to validate that the user’s query falls into one of the three allowed classes of query, is sensible, and is not out of scope.
Bias mitigation: Bias is another major concern with LLMs, which form potentially unwanted or non inclusive connections between concepts, based on their training data. VoxelGPT is incentivized to infer as much as possible from the contextual backdrop of the user’s FiftyOne Dataset, so that it capitalizes on the base LLM’s inference capabilities without being mired in its biases.
Programmed limitations: We purposely limited VoxelGPT’s access to any functionality involving the permanent moving, writing, or deleting of data. We also prevent VoxelGPT from performing any computationally expensive operations. At the end of the day, the human working with VoxelGPT (and FiftyOne) is the only one with this power!
InfoQ: What was one of the most surprising things you learned when building VoxelGPT?
Corso: Building VoxelGPT was really quite fun. LLMs capture a significant amount of generalizable language-based knowledge. Their ability to leverage this generalizability in context-specific ways was very surprising. What do I mean? At the heart of FiftyOne is a domain-specific language (DSL), based in Python, for querying schema-less unstructured AI datasets. This DSL enables FiftyOne users to “semantically slice” their data and model outputs to various ends like finding mistakes in annotations, comparing two models, and so on. However, it takes some time to become an expert in that DSL. It was wildly surprising that with a fixed and somewhat limited amount of context, we could provide sufficiently rich “training material” for the LLM to actually construct executable Python code in FiftyOne’s DSL.
The VoxelGPT source code is available on GitHub. There is also an online demo available on the FiftyOne website.
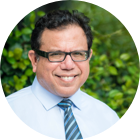
MMS • Anthony Alford
Article originally posted on InfoQ. Visit InfoQ
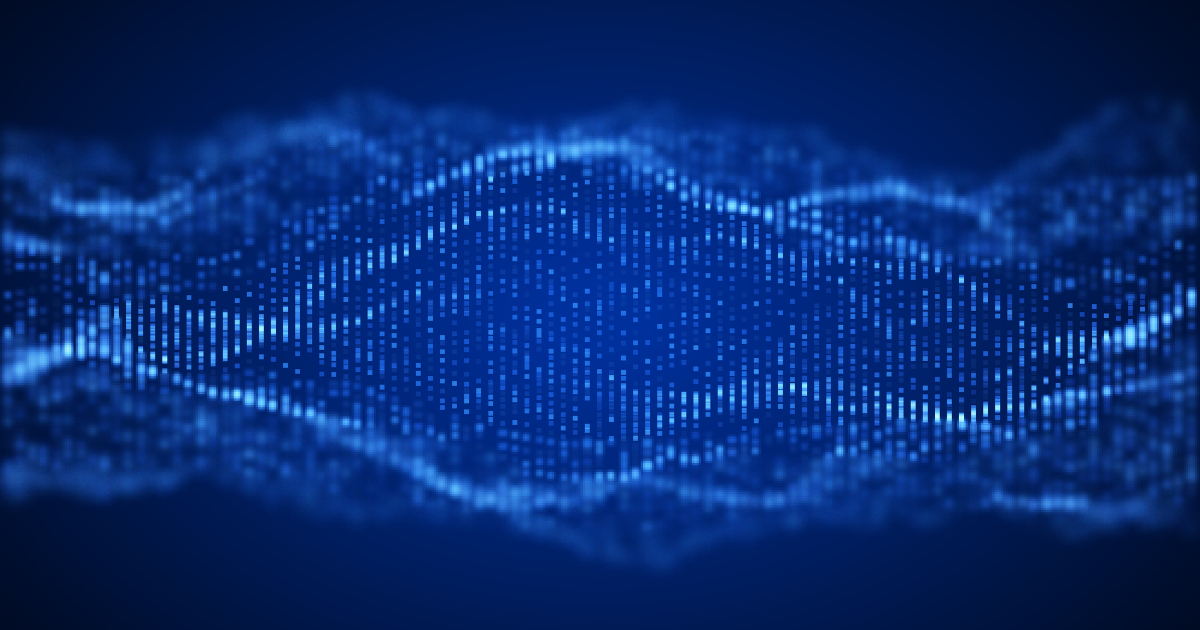
Meta AI open-sourced the Massively Multilingual Speech (MMS) model, which supports automatic speech recognition (ASR) and text-to-speech synthesis (TTS) in over 1,100 languages and language identification (LID) in over 4,000 languages. MMS can outperform existing models and covers nearly 10x the number of languages.
MMS is based on the wav2vec model and is pre-trained on a dataset containing 491K hours of speech in 1,406 languages, which is based on existing cross-lingual datasets as well as a new dataset of 9,345 hours of unlabelled recordings of religious text readings, songs, and other speech in 3,860 languages. To fine-tune the ASR and TTS models, Meta used recordings of Bible readings in 1,107 languages, which provided labeled cross-lingual speech data. The fine-tuned MMS models can perform ASR and TTS in those 1,107 languages as well as LID in 4,017 languages. According to Meta,
Many of the world’s languages are in danger of disappearing, and the limitations of current speech recognition and speech generation technology will only accelerate this trend. We envision a world where technology has the opposite effect, encouraging people to keep their languages alive since they can access information and use technology by speaking in their preferred language.
Training speech processing AI models using supervised learning requires large datasets of labeled speech data—usually audio recordings paired with transcripts. For many languages such as English, such datasets are readily available; however, for low-resources languages with very few native speakers, collecting a large dataset might be impossible. Meta’s previous research on XLS-R and NLLB showed that a single cross-lingual model combined with self-supervised pre-training can, after fine-tuning on small amounts of data, perform well on approximately 100 languages, even on low-resource ones. More recently, InfoQ covered OpenAI’s Whisper and Google’s USM, which also support around 100 languages each.
To scale their model to handle thousands of languages, Meta needed an audio dataset with more languages. The team chose to use audio recordings of the Christian New Testament; this provided labeled audio data in over 1,000 languages, with an average of 32 hours per language. Although each language’s recording was a single speaker, usually male, the researchers found that this introduced very little bias in the final models: the models performed similarly in female and male benchmark audio. They also did not find any bias due to the model being trained largely on religious texts.
Meta’s chief AI scientist Yann LeCun called out several highlights of MMS on Twitter, noting in particular it has “half the word error rate of Whisper.” Several users pointed out that the model’s usefulness was limited by its non-commercial license. Another user pointed out other drawbacks, and questioned whether it was indeed better than Whisper:
In my testing, it performs worse than Whisper for transcription to text, mis-hearing words and not hearing implied punctuation. Also it’s about 10x slower than Faster-Whisper. [MMS] uses 20 GB of RAM, while Whisper uses about 1 GB. For these reasons and others this is fairly impractical for people to use for a real application. Also note that you need to specify the language being spoken while Whisper will identify it for you. Hope these issues get resolved over time and OpenAI has a competitor eventually in this area.
The MMS code and pretrained model files are available on GitHub. A list of the supported languages for each task (ASR, TTS, and LID) is available online.