
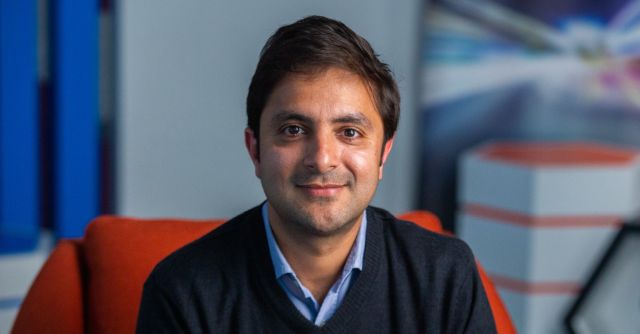
As Indian enterprises push forward with digital transformation, data modernization has become a central focus. Companies are moving to cloud-native systems, using real-time data, and beginning to build AI-driven applications.
MongoDB is working across the ecosystem, from startups and software vendors to large enterprises, to support this shift.
In this conversation, Sachin Chawla, VP for India & ASEAN at MongoDB, talks about how Indian enterprises are approaching data transformation, the challenges they face, and how MongoDB is adapting its strategy to meet these changing needs. Edited Excerpts:
What’s fundamentally changing in how Indian enterprises approach data and digital transformation, and how is that shaping your strategy in the region?
India is going through a significant phase in technology. While we’ll get to the challenges shortly, it’s worth highlighting the strong momentum we’re seeing in modern application development across the ecosystem.
This includes the startup space, where innovation is active, from early-stage companies like RFPIO to large-scale players like Zepto and Zomato. There’s also a robust ISV ecosystem here, which operates quite differently compared to markets like the US or EMEA. For instance, many Indian banks rely heavily on ISVs, often referred to as “bank tech”, for their software needs. Companies like CRMnext, Intellect AI, and Lentra are key examples, and many of them use MongoDB.
Beyond startups and ISVs, significant digital transformation is happening in large enterprises as well. Take Tata, for example, the Tata Neu app runs on MongoDB. So overall, there’s a lot of progress and activity in the ecosystem.
Now, on to the challenges. Broadly, they’re similar to those faced globally and can be grouped into three areas, first, improving developer productivity. Every organization is focused on how to help developers move faster and be more efficiently. Second, building modern AI applications. There’s growing pressure, both from the ecosystem and from leadership, to deliver AI-driven solutions.
Third, modernizing legacy applications. Many existing systems were built over years and aren’t designed to meet today’s demands. Users expect immediate, responsive digital experiences, and older systems can’t keep up. These are the key priorities: boosting developer productivity, adopting AI, and modernizing legacy systems.
What are the biggest misconceptions Indian enterprises still have about modern databases, and how do these hold back their digital transformation?
First, some organizations treat modernization as simply moving their existing systems from on-premises to the cloud. But lifting and shifting the stack without changing the application, the underlying infrastructure, or the database doesn’t actually modernize anything. It’s still the same application, just running in a different environment, and it won’t deliver new value just by being in the cloud.
Second, there’s the idea of using the best purpose-built database for each use case. In practice, this often leads to an overload of different databases within one organization. While each one might be optimal for its specific function, managing a large variety becomes a challenge. You end up spending more time and resources maintaining the system than actually innovating with it.
Third, when it comes to AI, many organizations lack a clear strategy. They often start building without clearly defined use cases or objectives, just reacting to pressure to “do something with AI.” Without a focused plan, it’s hard to deliver meaningful outcomes.
Which industries in India are making the boldest or most unexpected moves in digital transformation or data modernization, and why?
Every sector is adopting AI in its own way. Tech startups and digital-native companies tend to make faster, more visible moves, but even large enterprises are accelerating their efforts.
For example, we recently partnered with Intellect AI, an independent software vendor serving the global banking sector. They aimed to lead the way in building a multi-agent platform for banking clients to automate and augment operations in areas like compliance and risk, critical functions for many institutions.
We helped them develop this platform using MongoDB and MongoDB’s AI vector search. The result is called Purple Fabric, and it’s publicly available. This platform is now driving automation and augmentation in compliance and risk management.
One of their major clients is a sovereign fund managing $1.5 trillion in assets across around 9,000 companies. Using Purple Fabric, they automated ASC compliance processes with up to 90% accuracy.
This example shows that while enterprises may seem slower, companies like Intellect AI are enabling them to move quickly by building powerful tools tailored for complex environments.
What recurring data architecture issues do you see in enterprise AI projects, and how does your company help address them?
When you look at AI applications, it’s important to understand that the data used to build them is mostly unstructured. This data is often messy, comes from various sources, and appears in different formats such as video, audio, and text. Much of it is interconnected, and the overall volume is massive. Additionally, the data changes rapidly.
These are the three core characteristics of AI data: it’s unstructured, high in volume, and constantly evolving. As a result, if you look at an AI application a year after it’s built without any updates, it’s likely already outdated. Continuous updates are essential.
MongoDB stores data in a document format, unlike traditional systems that use rows and columns. Trying to store large volumes of unstructured and fast-changing data in a tabular format becomes unmanageable. You’d end up with thousands of tables, all linked in complex ways. Any change in one table could affect hundreds or thousands of others, making maintenance difficult.
This is why many modern applications are built on MongoDB rather than on legacy systems. For example, Intellect AI uses MongoDB, as does DarwinBox, which uses AI to power talent search queries like finding the percentage of top-rated employees. Previously, this kind of semantic search would take much longer.
Another example is Ubuy, an e-commerce platform with around 300 million products. They switched from a SQL database to MongoDB with vector search. Search times went from several seconds to milliseconds, enabling efficient semantic search.
RFP.io is another case. It uses vector search to process and understand RFP documents, identifying which sections relate to topics like security or disaster recovery. This simplifies the process of responding to RFPs.
As enterprises shift to unstructured data, vector search, and real-time AI, how is MongoDB adapting, and what key industry gaps still remain?
The first step is collecting and using data in real time. For that, you need the right database. A document model is a natural fit for the scale and structure of this data.
Once you have the data, the next step is using it effectively. That starts with full-text search, similar to how you search on Google. Most applications today rely on this kind of search functionality.
But if you’re building AI applications, you also need to vectorize the data. Without vectorization, you can’t perform semantic searches or build meaningful AI features.
At this point, companies usually face a choice. They often have data spread across multiple databases. To enable full-text search, they might add a solution like Elasticsearch. For semantic search, they bring in a vector database such as Pinecone. If they want to train or fine-tune models using internal data, they also need an embedding model. So now they’re managing a database, a full-text search engine, a vector search system, and an embedding model, each a separate component.
The integration work required to get all these systems to operate together can consume a large amount of development and management time, pulling focus away from innovation.
In contrast, our platform simplifies this. It uses a single document database to store all types of data. It includes Atlas Search for full-text search, built-in vector search, and now, with our acquisition of Voyage, integrated embedding capabilities. You don’t need separate systems for each function.
With everything in one place, there’s no need for complex integration. You can run full-text and semantic (hybrid) search from the same platform. This reduces cost, simplifies management, and frees up time for innovation. Customers tell us this is the biggest advantage—they don’t need to stitch multiple tools together, which can be very hard to manage.
What’s next for MongoDB in India, are you focusing on AI, edge deployments, cloud-native capabilities, or something else?
Our focus will remain on three main areas. First, we’ll continue working with developers to help them improve their productivity. Second, we’ll collaborate across the ecosystem and with enterprises to build modern applications. Third, and most significantly, we’ll support large enterprises as they modernize their applications, whether by creating new ones or upgrading legacy systems. This includes helping them reduce technical debt, move away from outdated applications and databases like Oracle and SQL, and transition to more modern architectures that align with their goals. These are our three key priorities.
Where do you see AI heading in the data modernization space over the next three to five years?
In my view, we’re at a stage similar to the 1960s when computers and operating systems were just emerging. I see large language models (LLMs) as the new operating systems. We’re in the early phase, and what comes next is the development of applications built on top of them. As this evolves, more advanced and diverse applications will emerge.
Building applications is becoming much easier. For example, there’s a concept called white coding, where even young children, eight or nine years old—can create apps. If a computer can guide you step by step, almost anyone can build one. That’s where we’re heading: a world where millions of applications can be developed quickly and easily.
We see ourselves as a natural platform for these applications because we make it simple to store data. So, over the next few years, we expect a surge in development activity. A lot is going to change, and I think we’ll all be surprised by just how much.

TRENDING STORIES
Next Article