Category: Data Science Central
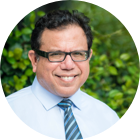
MMS • RSS
Article originally posted on Data Science Central. Visit Data Science Central
Introduction
Will china dominate AI?
When I spoke at the UK China business forum last month, I discussed this topic in response to an audience question.
In the current climate of nationalistic fervour, I see the same question asked in many guises.
For example, Politico present the problem of AI dominance as a threat perspective (a sputnik moment for the USA)
I summarise my views below.
As a disclosure, these are personal views. They are not associated with any organisation I am connected with (commercial or academic)
Background
Like many in Europe, I take the view of building bridges and of collaboration.
In many ways – the question of AI dominance is the wrong question
A view also echoed by others in Europe such as allai – there is no ai race
I have worked with Chinese AI companies (in robotics) and also enjoyed working with excellent Chinese students who I have mentored.
I am also familiar with the Chinese education system – such as the Gaokao
For anyone exploring AI and China, Kai Fu Lee’s book AI superpowers provides a great starting point
The key lessons from that book, as I see them, are:
- China is creating original ideas and applications (papers, patents etc)
- The availability of data in China gives an advantage to Chinese companies
- AI is more industrial revolution than a Cold War
- A divergence of skills will develop across geography (ex as Swiss and Japan for craftsmanship etc)
- China engages in bold experimentation when it comes to AI
All of this is true – for example – if current trends continue, China will overtake the US in the most-cited 50 percent of AI papers this year, the most-cited 10 percent next year, and the most-cited 1 percent by 2025. Via exponential view
China has a three-step plan for AI: firstly, it must be able to keep pace with all leading AI technology, and its application in general, by 2020. Part two is to make major breakthroughs by 2025, which is intended to lead to the third part of the plan – the establishment of China as the world leader in the AI field by 2030
Why is AI dominance the wrong question?
So, why is AI dominance the wrong question?
While every major country has national strategies for AI I believe that no country can dominate the complex AI space
To elaborate, here is an example from another domain
In early 2000s, with the start of 3G, NTT docomo and imode was expected to dominate
Everyone looked to Japan and Korea for the future of mobile
Indeed, Japanese and Korean mobile applications were rich media and cool
In fact, too rich media for world domination
In all other parts of the world, the networks were not advanced
And by the time they were, along came the iPhone and there was never a talk of a Korean or a Japanese company becoming the world leader in mobile (like the iPhone has become)
The early successes of iMode and other systems were due to deployment and standardization in specific geographies
standardization creates winners only in your local geography
By definition, that’s not world domination
Instead it is more relevant to focus on fundamental strengths and enable private companies to innovate
We are seeing the same in AI. Many of the advantages gained through Data for example are not translatable to other geographies (unless you can get hold of relevant data in those geographies – which is highly unlikely)
By this nuanced definition, there are many areas of excellence
- Engineering education – China, Israel, India
- Ecosystems and culture Canada – CIFAR
- Hubs – Waterloo, Oxford, Cambridge, London
- Favourable immigration policies for tech – currently Canada
- Investments from private companies – UK, India, Israel
- Education systems : GaoKao in China
- Specific areas – semiconductor industry in China
Obviously, China appears in many of these and in that sense will be a key player – but I do not expect a single dominant player to emerge.
Conclusions
- Core strengths matter more – and in that sense China will be a key player
- The question of who will dominate is misleading
- An open ecosystem will float all boats i.e. everyone will benefit by a free flow of ideas
Image source: Digital crew
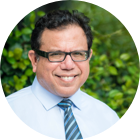
MMS • RSS
Article originally posted on Data Science Central. Visit Data Science Central
This is an interesting data science conjecture, inspired by the well known six degrees of separation problem, stating that there is a link involving no more than 6 connections between any two people on Earth, say between you and anyone living (say) in North Korea.
Here the link is between any two univariate data sets of the same size, say Data A and Data B. The claim is that there is a chain involving no more than 6 intermediary data sets, each highly correlated to the previous one (with a correlation above 0.8), between Data A and Data B. The concept is illustrated in the example below, where only 4 intermediary data sets (labeled Degree 1, Degree 2, Degree 3, and Degree 4) are actually needed. The numbers highlighted in red show how this chain of data sets is built.
We have the following correlations:
- Between Data A and Data B: -0.0044
- Between Degree 1 and Data A: 0.8232
- Between Degree 2 and Degree 1: 0.8293
- Between Degree 3 and Degree 2: 0.8056
- Between Degree 4 and Degree 3: 0.8460
- Between Data B and Degree 4: 0.8069
This is just a conjecture, and maybe the number of intermediary data sets or the 0.8 correlation must be fine-tuned and could depend on the size of the data set. But it makes for an interesting theoretical data science research project, for people with too much free time on their hands.
In some way, one could say that anything is related to everything, by a short path. Or that anything is caused by everything. This has of course been exploited in many news outlets to convey a political message, or to cause you to click on some random, worthless article, by using subject lines that seem implausible to attract your attention. .
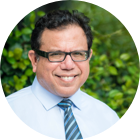
MMS • RSS
Article originally posted on Data Science Central. Visit Data Science Central
Monday newsletter published by Data Science Central. Previous editions can be found here. The contribution flagged with a + is our selection for the picture of the week. To subscribe, follow this link.
Featured Resources and Technical Contributions
- Two New Deep Conjectures in Probabilistic Number Theory
- Machine Learning vs Statistics vs Statistical Learning in One Picture +
- Bayesian Machine Learning Part 5
- Jobs, Unemployment and 45’s Performance – Analysis done with Python
- Object Detection using Deep Learning: Faster R-CNN,YOLO,SSD
- Regularization in Machine Learning
- Three Books About the Mathematics of Data
- R Users’ Salaries from the 2019 Stackoverflow Survey
Featured Articles
- How GANs and Adaptive Content Will Change Learning, Entertainment and More
- Using NLP for Hidden Feature Extraction
- Common Errors in Machine Learning due to Poor Statistics Knowledge
- Tai Chi of Civilization
- Wrestling with Data Science “Second Surgeries”? Try MVE!
- Python as a tool benefiting data scientists in many ways
- Why is Data Scientist the most promising job of the future?
Picture of the Week
Source: article flagged with a +
To make sure you keep getting these emails, please add mail@newsletter.datasciencecentral.com to your address book or whitelist us. To subscribe, click here. Follow us: Twitter | Facebook.
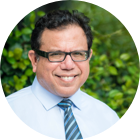
MMS • RSS
Article originally posted on Data Science Central. Visit Data Science Central
This is the next blog in my random series on better understanding some of these advanced Artificial Intelligence and Deep Learning algorithms. This “episode” takes on Generative Adversarial Networks (GANs). Hope you enjoy my “Deep Learning” learning journey.
I originally wrote in “Transforming from Autonomous to Smart: Reinforcement Learning Basics” how Reinforcement Learning was creating learning agents to beat games such as Chess, Go and Mario Bros. Reinforcement learning creates intelligent agents that learn via trial-and-error how to map situations to actions so as to maximize rewards. Reinforcement Learning is one of the more powerful Artificial Intelligence (AI) concepts because it is designed to learn and circumnavigate “situations” where you don’t have data sets with explicit known outcomes (which represents most real-life situations, like operating an autonomous vehicle).
However, creating Reinforcement Learning agents that can win at Chess, Go and Mario Bros is a (relative) piece of cake because each of these games operate within deterministic models where the output of the model is fully determined. We are now seeing Reinforcement Learning agents winning at games that operate under Stochastic models like StarCraft II and Texas Hold’em Poker where the agents need to make decisions and an opponent’s resources are unknown and historical information about previous game play is important to countering bluffing strategies. All of these advancements are moving us closer to a word of autonomous vehicles and (evil) robots.
Now add the ability of Deep Learning algorithms to create adaptive content based upon the human’s current capabilities and situational status. Using Generative adversarial networks (GANs), analytic-driven environments will be able to create adaptive content that can assist humans in resolving rapidly evolving, difficult situations, or push the envelope in providing a dynamic learning environment that is tailored to each individual’s experiences and strengths. The worlds of education, entertainment and healthcare (not to mention warfare) will be changed forever. Let’s explore further.
What Are Generative Adversarial Networks (GANs)?
Generative adversarial networks (GANs) are deep neural net architectures comprised of two neural nets, pitting one against the other (“adversarial”):
- The Generator is a neural network trained on a distribution of data (where a distribution of data might be images of dogs, cats, wolves) and extracts features from that distribution of data (mixed with some level of randomness) to try to fool the Discriminator into thinking the fake image is a real image. That is, the generator tries to create a fake cat image based upon the modeling (feature extraction) of real cats to fool the Discriminator into thinking that the fake cat image is a real cat image. Probably sounds better when someone else explains it…
- The Discriminator is a convolutional neural network that is trained to predict whether input images are real or fake. The discriminator outputs “1” if it thinks the image is real, and “0” if it thinks the image is fake. The discriminator tries to find a “decision boundary” or clusters that separate images into the appropriate classifications (dogs versus cats versus wolves). Then, to classify a new animal as either a dog or a cat or a wolf, it checks on which side of the decision boundary it falls and makes its prediction accordingly.
GANs can learn to mimic any data distribution (diseased plants, cancer, skin lesions, tumors, broken bones, mechanic parts abrasions) to create real-world like experiences. GANs can create something totally new yet life-like based upon adding randomness to a deep understanding of existing data distributions.
How Do Generative Adversarial Networks (GANs) Work?
After reading through 5 steps, see the illustration in Figure 1.
- Step 1: Like a traditional Neural Network, the Generator is trained (extracts features) on a large volume of a particular data distribution (dogs, cats, cars, plant diseases), then adds randomness to produce a fake data distribution based upon the aspects of the real images from which it learned.
- Step 2: The Discriminator then tries to separate the true data distribution from fake data distribution (clustering) and then calculates an error as to how close the fake data distribution is to the real data distribution.
- Step 3:Using Backpropagation, the Discriminative updates weights and biases to the neurons to decrease the classification error.
- Step 4:Then the Generator updates its weights by increasing the randomness on the key features and tries again to fool the Discriminator.
- Step Done:The GAN is considered successful when the generator can effectively trick the discriminator a pre-determined percentage of the time.
Figure 1: “Understanding Generative Adversarial Networks (GANs)”
At each iteration of the training process, the weights and biases of the generative network are updated in order to increase the classification error (error gradient ascent over the generator’s parameters) whereas the weights of the discriminative network are updated so that to decrease this error (error gradient descent over the discriminator’s parameters).
Our good friend Backpropagation (see the blog “What is Explainable AI and Why is it Needed?”) is how the measurement errors are fed back to the GAN to change the neuron weights and biases in order to gradually improve the “realness” accuracy of the fake images (see Figure 2).
Figure 2: Role of Backpropagation in GANs
The result is an entirely new yet real-looking reincarnation of a conglomeration of images for which the GAN has learned all the key aspects of those images and created something entirely new yet very life-like.
Using GANs to Create New Content
GANs are being used to recreate virtual levels and worlds, replicating very closely to how a human would operate. For example, we’re seeing GANs autonomously creating new levels, like in the game DOOM (DOOM is one of my favorites; hopefully Wolfenstein is next!).
Figure 3: Using GANs to create new Doom Levels
While using GAN to breathe new life into old games is cool, but there are even bigger opportunities for GANs in content generation.
VentureBeat reported that Disney is using GANs to accelerate the creation of storyboard animationsdirectly from scripts. Think about the massive volumes of animation images and content buried in the Disney vaults. Now image how Disney could apply GANs to accelerate the development of new animations more rapidly and at a fraction of the cost of producing an animation today (see Figure 4).
Figure 4: Disney Using GANs to Convert Scripts into Animations
The economics for content companies like Disney could be staggering! Frozen cost ~$150M to create, 4 years to create, and has netted Disney over $1.2B in box office receipts and another $5B in licensing revenues. The economics are pretty impressive, and one can certainly see why content providers like Disney would want to accelerate their ability to leverage their wealth of animation data distributions to accelerate these sorts of returns on investments.
Using GANs to Drive Adaptive Learning
While GANs appear to be very useful in creating new real-like content for the gaming, entertainment and advertising industries, the bigger win might be in the ability to create adaptive content that can accelerate training and education. Adaptive content is individualized content that can drive personalized interactions based upon an individual’s capabilities. experience, mood, and immediate goals.
In the area of education, GANs could create dynamic adaptive content that could be personalized for each individual student based upon their experience, areas of expertise, and current areas of struggle. The GAN-based educational system could dynamically create new content that could help the student in areas in which they are struggling and could push students into new areas more quickly if they are grasping concepts faster. GANs might play an even bigger role in the retraining of our workforces (especially when coupled with immersive technologies like Virtual Reality and Augmented Reality) as technology advances the nature of work. Learning will no longer be dependent upon the speed of the content and the expertise of the instructor!
And in the world of healthcare, the ability to dynamically create new experiences in training and re-certifying health care providers could ensure that all doctors are able to leverage the last learnings and developments and not be dependent upon having to attend the right conferences (snore) or churn through a stack of research studies to find learnings that are relevant for their current level of expertise. GANs could also provide a solution to the HIPAA privacy concerns by creating life-like data sets of severe medical situations without actually having to disclose any particular individual’s private data.
And in the area of warfare, well, all sorts of bad things can be conceived with adaptive content that can confuse enemies (and sway political elections).
Generative Adversarial Networks (GANs) Summary
The combination of deep reinforcement learning (to create AI-based assistants) and GANs (to create dynamic, adaptive content) could greatly accelerate the adoption and institutionalization of new technologies and accelerate business and society benefits in the process. Deep Reinforcement Learning could create AI-based assistants (agents) that aid teachers, physicians, technicians, mechanics, engineers, etc. while GANs could create new adaptive learning environments so that those assistants/agents are constantly learning – learning while the human in the process is doing more human-related activities like engaging with students, patients and customers.
Blog key points:
- Deep Reinforcement learning is driving rapid advancements in mastering games of increasing sophistication – starting with Chess, Go and Mario Bro with deterministic game frameworks to more stochastic games such as StarCraft II and Texas Hold’em Poker
- Generative adversarial networks (GANs) are deep neural net architectures comprised of two neural nets, pitting one against the other (thus the “adversarial”).
- GANs are playing an increasingly important role in content generation – creating life-like data distributions (cats, dogs, cars) based upon a deep understanding of the features of those data distributions.
- GANs are comprised of two neural networks – a generator which creates the fake images based upon a deep understanding of the features of real images, and a discriminator that tries to detect the fake images from the real images.
- The integration of Reinforcement Learning and GANs could yield dynamic, Adaptive Content that will greatly accelerate the worlds of entertainment, healthcare, education, security and unfortunately, warfare.
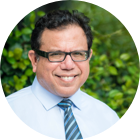
MMS • RSS
Article originally posted on Data Science Central. Visit Data Science Central
It is not unusual for a person to encounter hurdles or barriers in social processes – for example, to obtain financial support to do a post-secondary program. I drink decaffeinated coffee. Sometimes when I order coffee, perhaps due to lack of product demand I will receive a cup of coffee that is barely above room temperature. This situation likewise extends from some kind of social process – e.g. to get the order out although it is not quite what I ordered. In this blog, I refer to these common obstacles as “resistance.” In order to get something done that is dependent on social processes, it is usually necessary to develop processes that are structural in nature – and then to implement controls. Sometimes these processes and controls are the result of a deliberate plan or initiative; perhaps rather frequently, they exist as side-effects of attempts and efforts involving the interaction of people and systems. I describe these structural implements as forms of “conveyance.” A sensitive and observant individual might therefore be able to detect aspects of resistance and conveyance in socio-structural implements.
Consider a pool of funds intended to help the poor. Right at the outset, we can assume that these funds will not actually end poverty. If anybody has experience being able to spend resources to end poverty, by all means please correct me. The keywords here are “intended” and “help.” The social process set up to administer the program might be thought of a way to help the poor. From a slightly different perspective, the processes are meant to regulate and limit the flow of funds. No fancy system of administration is needed to prevent people from taking money from a bag of cash. The system is in place precisely to halt the free-flow of resources. How can this be done? Well, it is possible to impose eligibility criteria, income audits, reference requirements, essentially as many hoops as necessary. If somebody says a particular hoop is unfair, this doesn’t alter the fact that funds are limited; so it becomes necessary to install another hoop. The system is meant to contain all sorts of resistance; yet it is also true that funds must get distributed presumably through points of conveyance.
Resistance and conveyance are important aspects of our time. My perspective here is that the world is incapacitated. I am reluctant to use the term “overpopulated” – since there is actually a lot of physical room for bodies willing to move about. No, the planet has room for more – maybe not all at the same locations, of course. There is a lack of resources including government financial support. People talk about the injustice of having huge disparities between the rich and poor. What would happen if people actually gained lots of disposable income? They would spend money. They would have lots of children. Give this some thought. Everyone gets a house, two cars, four kids, four meals a day, possibly a cottage, new tablet and cellphone each year, and money to spare to buy whatever else they want. This scheme uses lots of energy and raw materials. I doubt that the planet can last long without the disparity in place. We encounter resistance and conveyance in socio-structural implements – because in discord there is harmony. The relative success of a person is greatly affected by their ability to exploit the dynamics between resistance and conveyance.
Resistance is evident in the presence of instrumentalism and disablement. Everyone has experienced disablement although they might not use the term. When we have little or no control over outcomes – when we can do nothing except stare at ourselves struggling to make it out of the tunnel – we are experiencing a type of disability. We literally have no “ability” to control the situation. Instrumentalism is when people are exploited or used as a means to an end. For example, a psychopath might kill somebody not so much because they have any strong feelings about that person personally but because they enjoy the rush from killing. Killing is merely a means to an end. I have nothing against chicken and hogs. But yes I might play with them if given the chance and eat them the next day. I suspect that many companies don’t genuinely care about their clients. They care about their client’s money. I know it is a peculiar connection for me to make – that instrumentalism and disablement are closely associated. The important point is that when instrumentalism and disablement exist, in all likelihood certain mechanisms are in place for the purpose of conveyance while other structures dismay people with enormous amounts of resistance.
When looking for a job, many people lug around all sorts of self-identity issues. When there is no response to an application or if an interview doesn’t lead to a job, they find fault in themselves. It is important to recognize that there might simply be a great deal of resistance. Odd as it might seem, at times there are also systems in place for the purpose of conveyance – for example, to fill available slots within a certain period of time. An excellent candidate might not get the desired job. The terrible candidate might get lucky. I am not describing a lottery exactly. People make the mistake of applying for a job rather than to fill the specified criteria. I guess it depends on whether the story is about the candidate or the job opening. As I mentioned earlier, it is necessary to identify what aspects of this social intersection might be met with resistance – and those aspects can contribute to conveyance. In a fast-moving creek, even a lifeless leaf can travel great distances. I imagine it takes some skill to land in the right body of water. Or it might be dumb luck. Or it might be “the force.”
I sometimes notice inanimate objects outside – kind of moving around as if they were alive. The convective currents in the wind toss about the debris. A monarch butterfly almost resembles a dead leaf even while fluttering to Mexico. We are carried by social winds also – flowing through time and space. We might blame things on Providence – e.g. the spirit of hat-tricks and miracles. Human life is actually fairly improbable, come to think of it, much like a string of hat-tricks. Consider the mathematics or geometry confronting human existence right here and now – as resources become depleted, species disappear, and ecosystems buckle under the weight of humankind. “What a beautiful, violent, and ultimately pointless story,” I am left thinking to myself. But actually the story doesn’t have to end. It just has to radically change. As the sun rises in the morning, I have no doubt that some kind “resistance” will rise up to ensure those changes occur. We are already feeling it now with precarious employment, automation, high living costs, and high levels of disparity between the rich and poor. We don’t need infinity stones or even special programs to quell the raging beast.
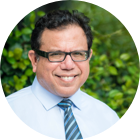
MMS • RSS
Article originally posted on Data Science Central. Visit Data Science Central
Bayesian Machine Learning (part -5)
Introduction: Expectation-Maximization
In this blog we are going to see how Expectation-maximization algorithm works very closely. This blog is in strict continuation of the previous blog. Previously we saw how probabilistic clustering ended up into chicken-egg problem. That is, if we have distribution of latent variable, we can compute the parameters of the clusters and vice-versa. To understand how the entire approach works we need to learn few mathematical tools, namely : Jensen’s inequality and KL divergence.
So, let’s start!!!
Jensen’s Inequality
Jensen’s inequality states that, for a convex function f(x) , if we select points as x = a and x = b, also we take α,β such that α + β = 1 ,then
If we consider x as a random variable and it takes the values a, b with probability α, β (as we know α + β = 1)., then the expression αa + βb is the expectation of x –> E(x), and the expression αf(a) + βf(b) — > is E(f(x)). Thus, the expression of Jensen’s inequality can be re-written as:
This above expression will be used in further analysis.
Kullback–Leibler divergence
This is the method of computation of divergence between two probability distribution functions. The mathematical expression is as follows:
Where q(x) and p(x) are pdf. functions on a random variable x. KL(q||p) is always ≥ 0.
Expectation – Maximization Algorithm
Now from our previous blog discussion, we know that marginalized posterior distribution is as follows :
Now we need to maximize the above expression w.r.t θ. The problem here is that maximization of the above expression is not simple, and function usually has many local maxima. We can use gradient – decent here but the process will become too lengthy. To solve this, we use a different approach here all together. We will try and construct a lower bound of the above expression. We will use Jensen’s inequality. And once we build our lower bound, we will try and maximize it.
the lower bound is also called variational lower bound
here we will try to apply Jensen’s inequality to compute the lower bound
consider the log as our function f and let us introduce a new function q(t=c, θ), and multiply it in denominator and numerator. So the new equation looks like:
Now consider the ratio (P(XI, t=c | θ)/ q(t=c, θ)) as random variable and q(t=c, θ) as the probabilistic coefficient as ∑3c=1 q(t=c, θ) = 1
Now from Jensen’s inequality, we know that:
Graphically
All the above small curves are the family from lower bound L(θ, q).
Expectation Step
So, one thing is clear that Lower bound will always be beneath the actual function, and we need to maximize the lower bound to be as much closer to actual function as possible. In expectation step we fix our θ and try to maximize the lower bound. We do it by reducing the gap between actual function and the lower bound. The equation is as follows :
putting in some linear algebra, the equation ends up in the expression of KL divergence between q and posterior t
the solution of expectation step is :
q(t=c) = P(t=c | Xi ., θ)
Maximization step
Once we get the gap reduced between the variational lower function L(θ, q) and log(P(X |θ)), we maximize our lower bound function w.r.t θ.
The second component of above equation is const. w.r.t θ. Therefore, we drop it, the new equation remains is:
In the next post we will continue our discussion with Gaussian mixture model and try to implement Expectation – Maximization algorithm to perform clustering.
Thanks for reading !!!
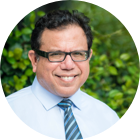
MMS • RSS
Article originally posted on Data Science Central. Visit Data Science Central
The material discussed here is also of interest to machine learning, AI, big data, and data science practitioners, as much of the work is based on heavy data processing, algorithms, efficient coding, testing, and experimentation. Also, it’s not just two new conjectures, but paths and suggestions to solve these problems. The last section contains a few new, original exercises, some with solutions, and may be useful to students, researchers, and instructors offering math and statistics classes at the college level: they range from easy to very difficult. Some great probability theorems are also discussed, in layman’s terms: see section 1.2.
The two deep conjectures highlighted in this article (conjectures B and C) are related to the digit distribution of well known math constants such as Pi or log 2, with an emphasis on binary digits of SQRT(2). This is an old problem, one of the most famous ones in mathematics, still unsolved today.
1, A Strange Recursive Formula
Let us consider the following recurrence system:
If z(n) < 2y(n) Then
- y(n + 1) = 4y(n) – 2z(n)
- z(n + 1) = 2z(n) + 3
Else
- y(n + 1) = 4y(n)
- z(n + 1) = 2z(n) -1
Now, let us consider the following limit:
The limit may or may not exist, and it may or may not depend on the initial conditions. Let us assume that the initial values y(1) and z(1) are strictly positive integers. If for some value of n, either y(n) = 2z(n) or 2y(n) = z(n), we call it the non-standard case. Otherwise, we call it the standard case. The first result below is about the limit mentioned earlier. However this is not one of the two deep conjectures featured in this article, but rather an interesting intermediary result.
Conjecture A
In the standard case, the above limit is always equal to 1. In the non-standard case, it is always equal to 3.
Now let’s the fun begin. Let d(n) = (z(n) − 2z(n−1) + 1) / 4. The sequence d(n) represents the binary digits of some unknown number x, a number that depends on the initial conditions. In the standard case, x is irrational while in the non-standard case, x is a rational number. It turns out that if y(1) = 2, and z(1) = 5 then that number is x = SQRT(2). You may ask: so what? Here is where it becomes fascinating: Proving Conjecture A for y(1) = 2 and z(1) = 5 (this is the standard case) amounts to proving that exactly 50% of the binary digits of SQRT(2) are equal to one.
Generally speaking, the above limit is equal to 4p − 1 where p is the proportion of binary digits equal to one for the number x in question: this fact is easy to prove, almost trivial, as the formula in the limit was built with that goal in mind.
An article about this recursion for SQRT(2), with the connection to its digit distribution, can be found here. An application to the gaming industry can be found in section 2.1 in the following article. The source code that I used in my computations is accessible here (Perl code using the Bignum library for exact arithmetic.)
The convergence to 1, for the standard case and in particular for x = SQRT(2), is backed by empirical evidence. This also works even if y(1) or z(1) are not integers. Indeed, non-integer initial values could be investigated to get deeper insights and try to prove conjecture A. For instance, it might be worth investigating what happens when y(1) tends to 2, and z(1) tends to 5. Below is a chart slowing the rather slow convergence for the limit, of the order 1 / SQRT(n) — the same speed (not a coincidence!) as for the central limit theorem.
Figure 1: Convergence of the limit to 1, for x = SQRT(2), based on the first n = 10,000 iterations
1.1. A deeper result
The slow convergence, mimicking the central limit theorem based on empirical evidence, led me to issue the following deeper result.
Then E(n) = SQRT(n) * (L(n) – 1) = O(1). In other words, E(n) is bounded as n tends to infinity. The first deep result below (Conjecture B) is equivalent (actually a little stronger) to the statement just made if x = SQRT(2):
Conjecture B
Let q(n) denotes the proportion of binary digits of SQRT(2), or any other normal number (also called good seed, for instance Pi or log 2) that are equal to one, among their first n digits. Then we have:
This is a much stronger statement than the best result proved so far. The best formally proved result states that among the first n binary digits of SQRT(2), at least SQRT(2n) of them are equal to one as n tends to infinity (reference: here) but everyone “knows” that this proportion actually tends to n / 2.
My conjecture is confirmed by empirical evidence, pictured in the chart below.
Figure 2: SQRT(n) * |q(n) – 1/2| for n up to 1,000,000;
q(n) is the proportion of binary digits of SQRT(2) equal to one, among its first n digits
The time series in Figure 2 looks like a Brownian motion at first glance, but it is not. The variance of a Brownian motion is strictly increasing, and becomes infinite over time, but here we are dealing with a bounded Brownian motion.
1.2. Connection to the Berry-Esseen theorem
The Berry-Esseen theorem provides a second-order approximation to the central limit theorem (itself a first order approximation.) Higher order approximations are available, see here. If the random variables are Bernoulli, better approximations are available, this is a topic of active research. Let me mention a recent result, which is the topic of a PhD thesis, which is where Conjecture B is derived from (the full thesis available here):
If the binary digits of normal numbers were distributed as i.i.d Bernouilli variables of parameter 1/2, as it very, very, very much seems to be the case, then Conjecture B would be true as a result of the above theorem. As of today, it is still one of the biggest mysteries of mother nature. Millions of smart mathematicians have worked on this problem without success for hundreds of years.
1.3. Potential path to solving this problem
On a positive note, there are a few promising approaches. In this section, I describe a possible path to a solution. The recurrence system described at the very beginning of this article, yielding the digits d(n) of some unknown real number x, with one of them being x = SQRT(2), has the following nice features:
- It is ergodic as far as the sequence d(n) is concerned
- It does not depend on the initial values y(1) and z(1), for its limiting distribution
- It does not involve SQRT(2) at all, as far as asymptotics are concerned
- It only has two statistical solutions (called attractors in chaos theory): the standard and non-standard cases, and SQRT(2) belongs to the standard case
In short, proving for the standard case that being a normal number is the only solution (the attactor) to the underlying discrete stochastic equation attached to the recurrence system, may be the way to go. This is equivalent to proving that the unique attractor is the uniform distribution, for the digit sequence d(n).
To the contrary, the classical approach relies on the recursion x(n + 1) = bx(n) – INT(bx(n)) where b is the base (here b = 2) and x(1) = x. Then d(n) = INT(bx(n)). It yields the stochastic integral equation P(Y < y) = P( bY – INT(bY) < y) with infinitely many solutions (statistical distributions.) Most of these solutions, for non-normal numbers, are pretty wild: see here and here. In addition, in the classical approach, the solution depends on the initial value: the number x.
Instead, using the discrete recurrence system featured in the introduction, eliminates these problems, at least for the number x = SQRT(2) in base b = 2.
Note: The recursion mentioned here is identical to the one featured in section 2.1 in this article after the change of variable z(n) = 4x(n) + 1. An additional change of variables, w(n) = z(n) − 2y(n), could prove useful.
2. Potential Solution Based on Special Rational Number Sequences
Here we follow a totally different approach to study the binary digits of SQRT(2). We start with the following sequence:
The brackets represent the fractional part function. It is easy to prove that x(n) is a rational number, and that its period in its base 2 expansion is equal to 2 * 3^(n -1). What’s more, the proportion of zeros and ones in the binary expansion of any x(n) is exactly 50/50 (see here). The median value M(n) computed on x(1), …, x(n) is one of these numbers, if n is odd: it is the middle value once x(1), …, x(n) have been sorted in numerical order. Thus the proportion of zeros and ones, in M(n), is always 50/50 if n is odd, regardless of n. At the limit as n tends to infinity, M(n) tends to SQRT(2)/2. This is due to the fact that x(n) has the distribution of 1 / 2^X where X is uniform on [0, 1], because {n log 3 / log 2} is equidistributed mod 1, itself a consequence of the fact that log 3 / log 2 is irrational.
It is tempting to conclude that the binary digits of SQRT(2) are thus 50/50 zeros and ones, and that this ratio is exact, not an approximation. It is too good to be true though, the situation is actually more complicated than that. If you look at the minimum or the maximum rather than the median, it does not work. Even if looking at the median, but with different x(n)’s, say x(n)’s that are perfect random numbers uniformly distributed on [0, 1], while M(n) always has 50/50 zeros and ones, at the limit as n tends to infinity, it is no longer true: M(n) tends to 1/2, the worst non-normal number!
The idea to make this work is to remove infinitely many x(n)’s, in order words, to consider a subsequence x(t(n)) with t(n) being increasingly large integers. If the thinning process used to carefully remove selected x(n)’s is in some sense uniform, then the distributions of x(t(n)) ,and x(n) will be identical and thus the limiting medians, will be unchanged. The new x(n) can be defined as follows:
Here, t(n) is a strictly increasing sequence of integers. If t(n) is a polynomial in n, with the leading coefficient equal to one, then {t(n) log 3 / log 2} is still equidistributed modulo 1 (see why here), and thus the distribution of the new x(n)’s and its limiting median is unchanged. If any time you remove an original x(n) smaller than SQRT(2), your remove one that is larger than SQRT(2)/2, and conversely, then the limiting median is also unchanged, equal to SQRT(2)/2. Or if t(n) is the arrival time of the n-th minimum record of |x(k) – SQRT(2)/2| computed on the first original x(k), k = 1, …, n, then the limit of the new sequence x‘(n) = x(t(n)), as n tends to infinity, will be SQRT(2)/2: this is discussed in details here. This latter approach is mathematically elegant: it involves the convergents of the continued fraction of log 3 / log 2, and has some connections with music theory.
I haven’t found so far a sequence t(n) that leads to a fool-proof solution. One approach that is promising, is discussed in section 2.1. It also leads to our second deep conjecture, Conjecture C.
2.1. Interesting statistical result
Let N(m, x) be the number of binary digits equal to 1, among the first m binary digits of x, and assume x is in [0, 1]. Using the sequence x(n) defined at the beginning of section 2, we say that the term x(n) is a bad number if and only if:
for at least one value of m between m = 1 and m = n. Also we say that x is an irregular number if the same condition is satisfied for at least one value of m, however large or small.
Now let’s remove all the bad numbers from the sequence x(n). This will remove a very tiny proportion of the x(n)’s, less than one out of 2^15 terms, but a strictly positive proportion nevertheless. Any term x(n) starting with 20+ successive digits equal to one, in its binary expansion, will be removed. Will the limiting median SQRT(2)/2 be preserved if we do this? Very likely, but no one knows. Among the standard mathematical constants, none are expected to be irregular numbers if Conjecture B is correct. This leads us to the following, even deeper conjecture:
Conjecture C
The number SQRT(2) is not an irregular number. Indeed, none of the top 20 most popular mathematical constants (Pi, e, log 2 and so on) is an irregular number.
Of course after removing the bad terms in the sequence x(n), the limiting median will have 50% zeros and 50% ones in its binary expansion, by construction, whatever that new median is. But the median might have been shifted by this process. For instance, the minimum won’t tend to 1/2 anymore, the maximum won’t tend to 1 anymore. But what about the median? Yet Conjecture C gives very strong bounds about the binary digit distribution for SQRT(2), and knowing these bounds might help solving the problem.
2.2. Another curious statistical result
Let’s get back to the original sequence x(n) defined at the beginning of section 2. Let’s look at the m-th binary digit of x(n). It’s average value computed over all x(n), n = 1, 2, and so on is denoted as m(m). We have:
Also,
The number 1 / (2 log 2) is the average value of x(n), computer over all the terms of the sequence x(n). Finally m(m) converges exponentially fast to 1/2 as m tends to infinity, and the sequence m(m) is strictly increasing. As a side note, the number v(m) consisting of the successive m-th binary digit of x(n) for n = 1, 2, and so on, is irrational: its proportion of binary digits equal to one is m(m), and since m(m) is irrational, that number v(m) must also be irrational, and of course, non-normal regardless of m. For more details, see here.
3. Exercises
The material presented in sections 1 and 2 represents only a tiny part of my research on this topic. The exercises below explore some of the interesting issues not covered in the main part of this article.
[1] This a starter, easy to prove, even though the result sounds intriguing. Let d(n) be the n-th binary digit of a number x in [0, 1]. Prove that
[2] More difficult. Define R(n) as
where h(n) is an increasing sequence of positive integers, with h(n)/n tends to 1 as n tends to infinity. Prove that R(n) tends to log 2 as n tends to infinity. If h(n) = n, then R(n) = H(2n) – H(n) where H(n) is the n-th harmonic number. These fractions have been well studied, and could potentially lead to a proof that log 2 is a normal number, especially if h(n) is carefully chosen.
[3] Using the equidistribution theorem, prove that the proportion of binary digits equal to one, for an irrational number x in [0, 1], is equal to
The change of variable z = 2^y can help.
[4] More difficult. Prove that if if x(n) = { nq } and y(n) = { nq * 12 / 35 }, then the correlation between the two sequences is 1 / (12 * 35). The brackets represent the fractional part function. Replace 12 and 35 by any pair of integers that do not share a common divisor, and as long as q is irrational, this result easily generalizes. For a proof, see here.
[5] Let us consider the 2^n possible sequences of n binary digits. We define a run of length k as being a sub-sequence of k identical digits. How many of these 2^n n-digits “words” have a maximum run of length k? Start with k = 2. The answer, for k = 2, is F(n), the n-th Fibonacci number. See here the solution to this problem. More generally, the longest run is of order log n. See here for details, this is a pretty deep result. See also this article.
[6] At the beginning of section 2, we defined a specific sequence x(n) that has this property: all x(n)’s are rational numbers with (in binary representation) a period equal to 2 * 3^(n-1). The denominator, for these rational numbers is always 3^n. If we want rational numbers with a bigger period, indeed with the maximum possible period, we should look at denominators that are reptend primes: see here and here. See also my question posted on StackExchange, here. Would it be easier to work with these special primes, rather than with my original sequence x(n)?
[7] It is possible to get an incredibly good approximation for the sum of the first n terms {k log 3 / log 2}, k = 1, 2, …, n. As usual, the brackets represent the fractional part function. More specifically:
Prove this result. See solution here.
[8] Consider the following recursion: x(1) = 1, x(n+1) = ax(n) + bn, y(n) = −1 + x(n)/a^n. This recursion seems quite intractable, but it has some interesting features and it is much more friendly than it looks at first glance. Here a, b are two positive parameters, with a > 1. Let g(a, b) be the limit of y(n) as n tends to infinity. Prove that if a and b are rational numbers, then g(a, b) is also a rational number. See solution here. Unfortunately, it means that this recursion is of no use to prove the normalcy of standard mathematical constants, contrarily to what I initially thought.
[9] Difficult. Let x(n) = { b^n x} with the brackets representing the fractional part function. Does the auto-correlation structure of this sequence characterizes a good seed: if correl(x(n), x(n+k)) = 1 / b^k, does it mean that x is a good seed (that is, a normal number) in base b? The definition of this correlation coefficient is posted here: see section “definition of correlation”. There is no solution to this day.
WebAssembly Source Code Can Now Be Debugged Outside the Browser With GDB and LLDB
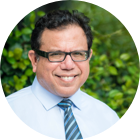
MMS • RSS
Article originally posted on InfoQ. Visit InfoQ
Mozilla recently demonstrated debugging of WebAssembly binaries outside the browser, with standard debuggers like GDB and LLDB. Debugging WebAssembly code in the same execution environment used in production allows developers to catch and diagnose bugs that may not arise in a native build of the same code.
WebAssembly, in connection with WASI made strides to be used outside of the browser, and realize the Write Once, Run Anywhere promise.
While running WebAssembly programs outside the browser is already possible, in particular with wasmtime
, the debugging story presented some difficulties. While WASI allows developers to use console
logging to report on the execution of the program, it was not possible to debug the original source. Taking the example of a Rust source compiled to WebAssembly, rustwasm
documentation explains the issue:
Debuggers currently provide limited utility, and we end up stepping through raw WebAssembly instructions emitted by the compiler, rather than the Rust source text we authored.
Source-level debugging of .wasm
files using traditional tools like GDB and LLDB is now possible.
In a recently published screencast, Mozilla illustrates the debugging with LLDB of a Rust-generated WebAssembly program which implements a solution to the FizzBuzz problem. The screencast first showcases compiling Rust code with cargo
to WebAssembly, and running the generated wasm code with wasmtime
. The screencast continues with instrumenting the wasm code with debugging information, using LLDB:
lldb -- wasmtime -g target/wasm32-wasi/debug/fizz.wasm
Once this is done, developers can use breakpoints (for instance breakpoint set -name fizzbuzz
), source-level navigation, inspect and modify variables during the interrupted execution of the program, view and navigate stack frames, and perform other typical debugging functions with the command line interface or the graphical user interface of LLDB:
Mozilla explains how source-level debugging may help WebAssembly developers catch otherwise obfuscated bugs:
Wasmtime’s debugging support makes it easier to catch and diagnose bugs that may not arise in a native build of the same code. For example, the WebAssembly System Interface(WASI) treats filesystem access more strictly than traditional Unix-style permissions. This could create issues that only manifest in WebAssembly runtimes.
The LLVM Project is a collection of modular and reusable compiler and toolchain technologies. The LLDB project builds on libraries provided by LLVM and provides a native debugger.
GDB (GNU Project debugger) is a debugger which supports the following languages: C++, Rust, Go, Objective-C, Ada, Assembly, C, D, Fortran, OpenCL, Modula-2, and Pascal.
WASI (WebAssembly System Interface) is a modular system interface for WebAssembly focused on security and portability, which is on a standardization path. WASI aims at enabling developers to run WebAssembly programs on any devices, computers, or operating systems.
Wasmtime is a standalone JIT-style runtime for WebAssembly. The Wasmtime runtime’s tutorial contains examples for how to target WASI from C and Rust. The resulting .wasm
modules can be run in any WASI-compliant runtime, such as Wasmtime or Fastly’s Lucet.
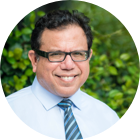
MMS • RSS
Article originally posted on Data Science Central. Visit Data Science Central
Statistics, Statistical Learning, and Machine Learning are three different areas with a large amount of overlap. Despite that overlap, they are distinct fields in their own right. The following picture illustrates the difference between the three fields.
References
An Introduction to Statistical Learning
VERY BASIC OVERVIEW OF STATISTICS AND MACHINE LEARNING
MACHINE LEARNING VS. STATISTICS
Machine Learning vs. Statistics
The Actual Difference Between Statistics and Machine Learning