Category: Uncategorized
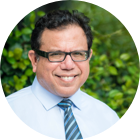
MMS • RSS
Posted on mongodb google news. Visit mongodb google news
MongoDB Inc (MDB, Financial), a leader in document-oriented database solutions, filed its 10-K on March 21, 2025, offering a comprehensive view of its financial health and strategic positioning. The company has seen a notable increase in revenue, with subscription services accounting for 97% of the total revenue, climbing from $1.24 million in 2023 to $1.94 million in 2025. Despite this growth, MongoDB Inc (MDB) reported a net loss of $(129,072) in 2025, although this is an improvement from the $(345,398) loss in 2023. The company’s commitment to innovation is evident in its substantial research and development expenses, which reflect its strategy to maintain and extend product leadership. This SWOT analysis delves into the strengths, weaknesses, opportunities, and threats as revealed by MongoDB Inc’s latest SEC filing.
Strengths
Brand Power and Market Position: MongoDB Inc (MDB, Financial) has established itself as a leading name in the database software market, known for its innovative document-oriented database platform. The company’s strong brand is built on a reputation for performance, scalability, flexibility, and reliability, which are critical factors in the database industry. MongoDB’s document-based architecture differentiates it from traditional relational databases, providing developers with a more natural and intuitive way to manage data. This strength has translated into significant revenue growth, particularly in subscription services, which have seen a year-over-year increase, demonstrating the company’s ability to attract and retain customers.
Research and Development Focus: MongoDB Inc (MDB, Financial) has consistently invested in research and development, which is a testament to its commitment to product innovation and leadership. In 2025, the company employed 1,327 individuals in its research and development team, underscoring its dedication to enhancing existing products and developing new offerings. This focus on R&D has led to the introduction of MongoDB version 8.0 and other features that keep the company at the forefront of database technology, catering to the evolving needs of developers and organizations.
Weaknesses
History of Net Losses: Despite its revenue growth, MongoDB Inc (MDB, Financial) has a history of net losses, which raises concerns about its long-term profitability. The company’s net loss decreased from $(345,398) in 2023 to $(129,072) in 2025, indicating an improvement in financial performance. However, the persistent losses highlight the challenges MongoDB faces in achieving profitability, particularly as it continues to prioritize growth and market expansion over immediate financial returns.
Intense Market Competition: MongoDB Inc (MDB, Financial) operates in a highly competitive database software market, where it faces stiff competition from legacy providers such as IBM, Microsoft, and Oracle, as well as cloud providers like AWS, GCP, and Microsoft Azure. These competitors have significant advantages, including established customer relationships, greater financial and technical resources, and broader product portfolios. MongoDB’s ability to compete effectively is crucial for its success, and the intense competition represents a significant weakness that the company must address.
Opportunities
International Expansion: MongoDB Inc (MDB, Financial) has identified significant opportunities to expand its platform’s use outside the United States. The company’s strategic focus on international growth can tap into new markets and diversify its revenue streams. By leveraging its strong product offerings and adapting to local market needs, MongoDB has the potential to increase its global footprint and capitalize on the growing demand for database solutions worldwide.
Strategic Partnerships and Developer Community: MongoDB Inc (MDB, Financial) has built a robust partner ecosystem and a large, engaged developer community, which presents opportunities for growth and innovation. The company’s partnerships with major cloud providers and expansion into new regions, such as China through collaborations with Alibaba Cloud and Tencent Cloud, can drive adoption and increase market presence. Additionally, fostering the MongoDB developer community can lead to increased brand awareness and advocacy, further propelling the company’s expansion.
Threats
Geopolitical Instability and Economic Conditions: MongoDB Inc (MDB, Financial) operates in a global market that is susceptible to geopolitical instability and economic fluctuations. Conflicts such as the Israel-Hamas conflict and Russia’s invasion of Ukraine, along with inflationary pressures and interest rate changes, can adversely affect the economy and MongoDB’s business. These external factors can disrupt global supply chains, increase costs, and impact customer spending, posing significant threats to the company’s financial stability and growth prospects.
Legal and Regulatory Challenges: MongoDB Inc (MDB, Financial) faces potential legal and regulatory challenges that could impact its operations and financial performance. The company is involved in ongoing litigation, such as the Baxter v. MongoDB securities action and shareholder derivative lawsuits, which could result in financial liabilities and damage its reputation. Additionally, MongoDB must navigate evolving regulatory landscapes, including privacy concerns and cybersecurity standards, which could impose additional compliance costs and operational constraints.
In conclusion, MongoDB Inc (MDB, Financial) demonstrates strong growth potential and market leadership, backed by its innovative technology and strategic investments in research and development. However, the company’s history of net losses and the intensely competitive landscape pose challenges that MongoDB must overcome. Opportunities for international expansion and strategic partnerships offer promising avenues for growth, while geopolitical and economic uncertainties, along with legal and regulatory issues, present threats that require careful management. Overall, MongoDB Inc (MDB) is well-positioned to leverage its strengths and opportunities to address its weaknesses and mitigate threats in the dynamic database market.
This article, generated by GuruFocus, is designed to provide general insights and is not tailored financial advice. Our commentary is rooted in historical data and analyst projections, utilizing an impartial methodology, and is not intended to serve as specific investment guidance. It does not formulate a recommendation to purchase or divest any stock and does not consider individual investment objectives or financial circumstances. Our objective is to deliver long-term, fundamental data-driven analysis. Be aware that our analysis might not incorporate the most recent, price-sensitive company announcements or qualitative information. GuruFocus holds no position in the stocks mentioned herein.
Article originally posted on mongodb google news. Visit mongodb google news
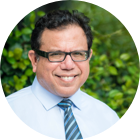
MMS • RSS
Posted on mongodb google news. Visit mongodb google news
Investing
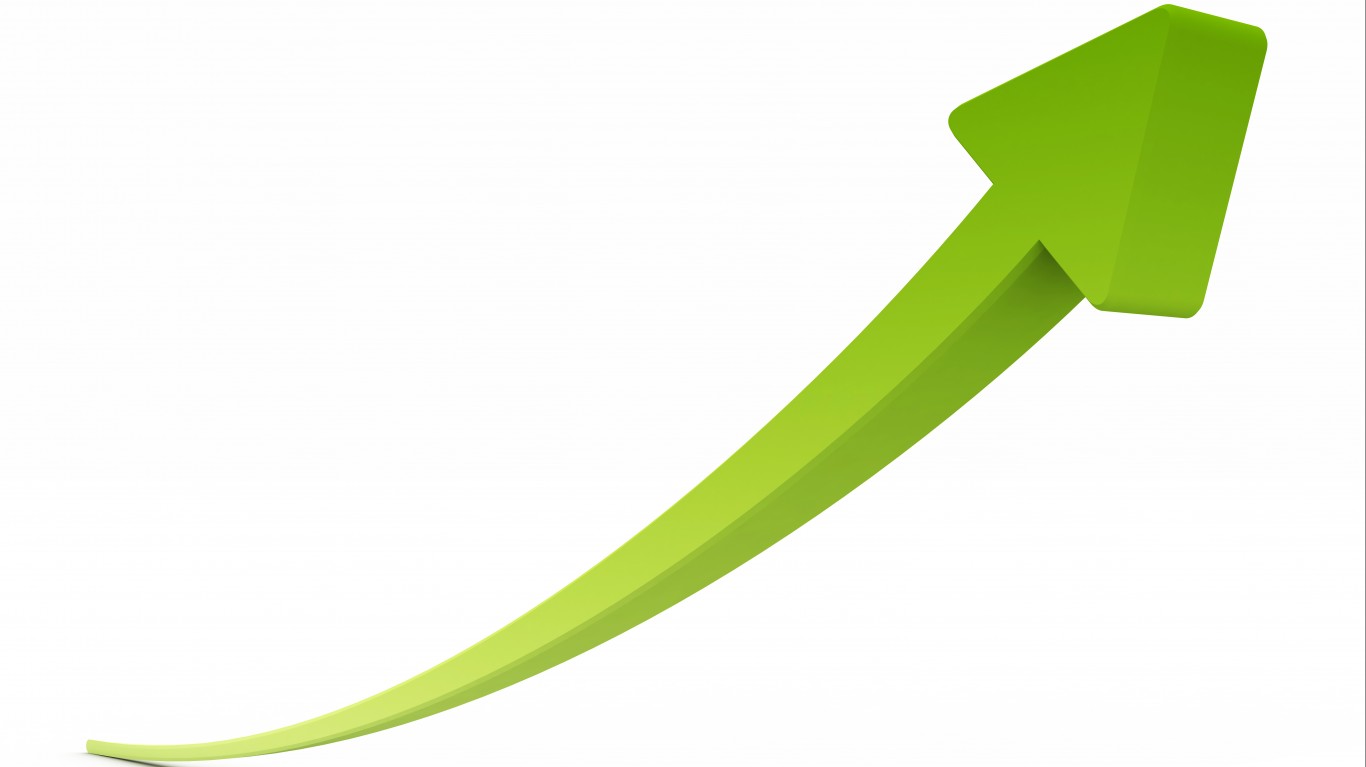
The stock market has recovered from its correction territory, but there’s still significant fear due to tariff-related uncertainty. This has caused stocks to trade sideways and make very little progress toward a sustained recovery. Most stocks remain expensive, especially if you look at tech stocks.
-
The stock market has recovered slightly from correction territory, but most stocks are far from fully recovering.
-
While many stocks are still expensive, a handful are now solidly undervalued.
-
Buying the dip in these stocks could help you realize triple-digit gains as they make a comeback.
-
Are you ahead, or behind on retirement? SmartAsset’s free tool can match you with a financial advisor in minutes to help you answer that today. Each advisor has been carefully vetted, and must act in your best interests. Don’t waste another minute; get started by clicking here here.(Sponsor)
But this shouldn’t deter you from investing entirely. Rather the opposite, since corrections tend to cause many companies to tumble below their intrinsic prices. For example, Warren Buffett pulls out his wallet during market turmoil. We haven’t seen much of that in the past two years as the market rallied, but it’s a good idea to swoop in and buy certain stocks that are trading cheaply.
MongoDB (MDB)
MongoDB (NASDAQ:MDB) is a database company. It sells database software to companies that need to handle lots of data. That aligns quite well with the machine learning, AI, and data center narrative, so you’d expect it to be sitting on top of a massive rally. Unfortunately, it has been quite the opposite over the past year as it has declined 47%.
You can blame it on the company’s Q4 FY2025 earnings. It wasn’t terrible as revenue still grew 20% year-over-year to $548.4 million, and the cloud service grew 24%. Guidance is what caused a selloff since MongoDB expects revenue between $2.24 billion and $2.28 billion for fiscal 2026. The midpoint is below the $2.3 billion expected by analysts. This may look like a small top-line guidance miss, but software companies are expected to continuously beat estimates, especially during the AI boom. EPS guidance of $2.44 to $2.62 also came in short of the $3.39 consensus.
Since then, the selloff has been more than enough to account for the disappointment in guidance. MDB currently trades at an earnings multiple of 70 times forward earnings, but EPS is expected to improve significantly after a 25.5% decline this year.
EPS is expected to grow from $2.73 for FY2026 all the way to $8 in FY2030. Moreover, analysts have paid much higher premiums for the stock historically, so if management manages to trounce estimates in Q1 FY2026, MDB stock could recover sharply. Its acquisition of Voyage AI for $220 million also boosted the company’s AI stack.
The consensus price target is at $320.7, implying a 67.91% upside potential. The highest price target of $430 implies up to 125% upside.
SPS Commerce (SPSC)
SPS Commerce (NASDAQ:SPSC) sells cloud-based software to help companies in the retail supply chain swap information more effectively. They also have extra analytics to track sales and inventory.
The stock was among the best-performing names since the start of 2018. SPSC stock returned around 250% in gains from February 2020 to February 2024. However, that’s around when it started plateauing. The stock pulled back sharply this year and SPSC is now down nearly 30% year-to-date.
The broader market has been jittery, and that’s partly to blame for the stock decline as it is highly involved in supply chains. However, its own numbers have spooked investors more than any broader market fears. Guidance for 2025 projects top-line growth at 19-20%. Solid, but considering how much investors were paying for this stock before it declined, it didn’t wow anyone.
SPSC seems worth buying the dip at current levels since the company has performed flawlessly, and every dip has proved to be a buying opportunity. This time is unlikely to be any different since SPS Commerce is still a cash cow with sticky recurring revenue and a network effect that’s tough to crack. It has over 120,000 companies hooked onto its platform.
The consensus price target of $207.11 implies 60.4% upside potential. Price targets go as high as $240, and even the lowest price target of $175 implies solid upside.
Datadog (DDOG)
Datadog (NASDAQ:DDOG) makes its money by selling a cloud-based monitoring and analytics platform to its businesses. Investors were raking in the dough until late 2024, when things started going wrong. DDOG stock is now down 27.8% year-to-date.
In Q4 2024, Datadog reported Q4 2024 earnings. Revenue climbed 25% to $738 million, and adjusted EPS hit $0.49 per share. Both beat expectations, but investors were not happy with the 2025 guidance. Datadog projected revenue growth slowing to 18-19% in the range of $3.175 to $3.195 billion, with an EPS decline of $1.65 to $1.7 per share from $1.82 in 2024.
Wall Street analysts were expecting $3.24 billion in revenue and stable or growing profits. That gap and the shrinking margins sent the stock tumbling. Recent broader market fears haven’t helped either. Companies are cutting budgets on some cloud services, and some big clients have optimized their usage. Some clients are now negotiating volume discounts or scaling back, and Datadog is ramping up spending to offset any revenue decline.
The dip looks like an overreaction to a temporary margin squeeze. Datadog’s EPS is expected to decline 7% this year and then continue growing at a solid pace from $1.7 in 2025 to over $6 through 2030. If they hit even the low end of guidance ($3.175 billion) and trade back to a 15x P/S ratio (not unreasonable for a 20%+ grower), that’s still a $47 billion market cap.
The consensus price target of $158.7 implies 53.5% upside potential. The highest price target is at $200.
Get Ready To Retire (Sponsored)
Start by taking a quick retirement quiz from SmartAsset that will match you with up to 3 financial advisors that serve your area and beyond in 5 minutes, or less.
Each advisor has been vetted by SmartAsset and is held to a fiduciary standard to act in your best interests.
Here’s how it works:
1. Answer SmartAsset advisor match quiz
2. Review your pre-screened matches at your leisure. Check out the advisors’ profiles.
3. Speak with advisors at no cost to you. Have an introductory call on the phone or introduction in person and choose whom to work with in the future
Thank you for reading! Have some feedback for us?
Contact the 24/7 Wall St. editorial team.
Article originally posted on mongodb google news. Visit mongodb google news
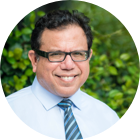
MMS • Alexandru Ene
Article originally posted on InfoQ. Visit InfoQ
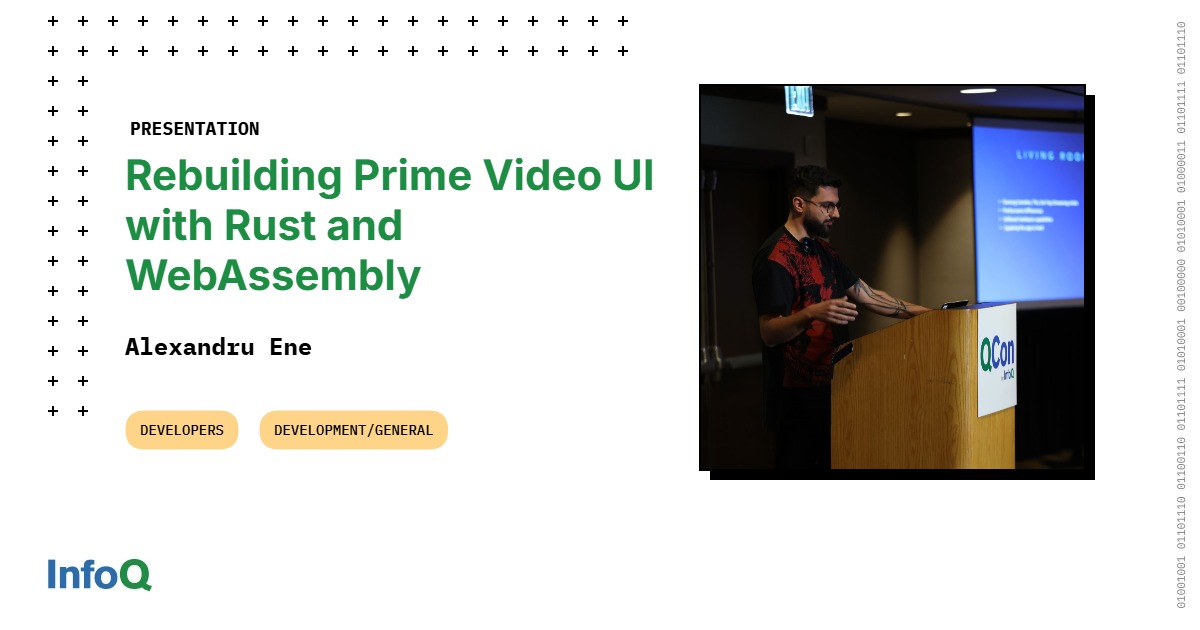
Transcript
Ene: We’re going to talk about how we rebuilt a Prime Video UI for living room devices with Rust and WebAssembly, and the journey that got us there. I’m Alex. I’ve been a principal engineer with Amazon for about eight years. We’ve been working with Rust for a while actually in our tech stack for the clients. We had our low-level UI engine in WebAssembly and Rust for that log. Previously I worked on video games, game engines, interactive applications like that. I’ve quite a bit of experience in interactive applications.
Content
I’ll talk about challenges in this space, because living room devices are things like set top boxes, gaming consoles, streaming sticks, TVs. People don’t usually develop UIs for these devices, and they come with their own special set of challenges, so we’re going to go through those. Then I’ll show you how our architecture for the Prime Video App looked before we rewrote everything in Rust. We had a dual tech stack with the business code in React and JavaScript, and then low-level bits of the engine in Rust and WebAssembly, a bit of C++ in there as well. Then I’ll show you some code with our new Rust UI SDK and how that looks, which is what we use right now in production. We’re going to talk a little bit of how that code works with our low-level existing engine and how everything is organized. At the end, we’re going to go a little bit to results, lessons learned.
Challenges In This Space
Living room devices, so as I said, these are gaming consoles, streaming sticks, set top boxes. They come with their own challenges, and some of them are obvious. There’s performance differences that are huge. We’re talking about a PlayStation 5 Pro, super nice gaming console, lots of power, but also a USB power streaming stick. Prime Video, we run the same application on all of these device types. Obviously, performance is really important for us. We can’t quite have teams per device type, so one team that does set top boxes, then another team does gaming consoles, because then everything explodes. When you build a feature, you have to build it for everything. We were building things once and then deploying on all of these device categories here. We don’t deploy this application that I’m talking about on mobile devices, like iPhone, iOS, mobile devices don’t have this. This is just living room devices. Again, a huge range of performance. We’re trying to write our code as optimal as possible.
Usually, high performant code is code that you compile natively. Let’s say Rust compiled to native, C++ compiled to native, but that doesn’t quite cut it in this space and we’ll see why. Another pain point and challenge is hardware capabilities between these devices is a pain. As SDK developers, we need to think a lot about what are reasonable fallbacks that application developers who write the app code and the app behavior, they don’t need to think about when they write that code and every little hardware difference. We try to have some reasonable defaults. That’s not always possible, so we use patterns like feature flags and things like that to let them have a bit more control. It’s a fairly challenging thing.
Another thing is we’re trying to make this application as fast as possible with as many features as possible to every customer, but then updating native code on these device types is really hard. Part of that is these devices don’t even have app stores, most of them. Maybe it goes up with a firmware update. That’s a pain. It requires a manual process interacting with a third party that owns the platform. Even on places that do have an app store, if you try to update an app on an app store, it’s quite a challenge as well. You need to wait. It’s highly likely a manual process. We’re having this tension between code that we’re downloading over the air, like JavaScript, WebAssembly, and so on, fairly easy, and then code that works on a device that is very fast, but then really hard to update. We want to have this fast iteration cycle. Updating the app in a short amount of time is huge for us.
Again, this is how the application looks like today. I’ve been there eight years and we changed it many times. I’m sure it’s going to change again sometime as it happens with the UIs. We’ve added things to it like channels, live events, all sorts of new features that weren’t there in the starting version. Part of us being able to do that was this focus on updatability that we had all the way in the beginning. Most of these applications were in a language like JavaScript that we can change basically everything on it and add all of these features almost without a need to go and touch the low-level native code. I’ll show you the architecture and how it looks like.
Today, if a developer adds some code, changes a feature, fixes a bug, does anything around the UI, that code goes through a fully CI/CD pipeline, no manual testing whatsoever. We test on virtual devices like Linux and on physical devices where we have a device farm. Once all of those tests pass, you get this new experience on your TV in your living room. That is way faster than a native app update for that platform.
Right now, you’ll see it working and you’ll see a couple of features. This is a bunch of test profiles I was making because I was testing stuff. We have stuff like layout animation, so the whole layout gets recalculated. This is the Rust app in production today. Layout animations are a thing that was previously impossible with JavaScript and React, and now they just work. When you see this thing getting bigger, all the things get reordered on the page. These are things that are just possible right now due to the performance of Rust. Almost instant page transitions as well are things that weren’t possible with TypeScript and React due to performance constraints. This is live today and this is how it looks like, so you have an idea on what is going on in there. We’re going to get a little bit back to layout animations and those things later. For people who are not UI engineers, or don’t quite know, this is the slide that will teach you everything you need to know about UI programming.
Basically, every UI ever is a tree of nodes, and the job of a UI SDK is to manipulate as fast as possible this tree as a reaction to user inputs or some things that happen like some events. You either change properties on nodes, like maybe you animate a value like a position, and then the UI engine needs to take care of updating this tree and creating new nodes, deleting new nodes, depending on what the business logic code tells you to do. Those nodes could be view nodes that are maybe just a rectangle. Then, text nodes are quite common, and image nodes, those type of things. Nothing too complicated. It’s really annoying that it’s a tree, but we’re going to move on because we’re still having a tree, even in our Rust app we didn’t innovate there, but it’s just important to have this mental model. We call this in our UI engine, a scene tree, browsers call it a DOM, but it’s basically the same thing everywhere.
High-Level Architecture
This is the high-level architecture before we rewrote everything in Rust. As you can see, we already added Rust, I think two years, three years ago, we already had it there for the low-level UI engine. There’s a QCon talk about this journey. There’s another part which is saying JavaScript here, but actually developers write TypeScript, that has the business logic code for the Prime Video App. This is the stuff we download. This is downloaded every time the application changes. This is what we output at the end of that full CI/CD pipeline. It’s a bundle that has some WebAssembly compiled Rust code and some JavaScript that came from TypeScript and got transpiled to JavaScript. It maybe changes once per day, sometimes even more, sometimes less, depending on our pipelines and if the tests pass or not, but it’s updated very frequently on all of the devices that I spoke about, the device categories.
Then we have the stuff on device in our architecture. We’re trying to keep it as thin as possible because it’s really hard to update, so the less we touch this code, the better. It has a couple of virtual machines, some rendering backend, which mainly connects the higher-level stuff we download to things like OpenGL and other graphics APIs, networking. This is basically cURL. Some media APIs and storage and a bunch of other things, but they’re in C++. We deploy them on a device and they sit there almost untouched unless there’s some critical bug that needs to be fixed or some more tricky thing. This is how things work today.
Prime Video App, (Before) With React and WebAssembly
You might wonder, though, these are two separate virtual machines, so how do they actually work together? We’re going to go through an example of how things worked before with this tech stack. The Prime Video App here takes high-level decisions, like what to show the user, maybe some carousels, maybe arrange some things on the screen. Let’s say in this example, he wants to show some image on your TV. The Prime Video App is built with React. We call it React-Livingroom because it’s a version of React that we’ve changed and made it usable for living room devices by pairing them some features, simplifying them, and also writing a few reconcilers because we have this application that works on this type of architecture, but also in browsers because some living room devices today have just an HTML5 browser and don’t even have flash space big enough to put our native C++ engine. We needed that device abstraction here. Prime Video App says, I want to put an image node. It uses React-Livingroom as a UI SDK.
Through the device abstraction layer, we figure out, you have a WebAssembly VM available. At that point in time, instead of doing the actual work, it just encodes a message and puts it on a message bus. This is literally a command which says, create me an image node with an ID, with a URL where we download the image from, some properties with height and position, and the parent ID to put it in that scene tree. The WebAssembly VM has the engine, and this engine has low-level things that actually manage that scene tree that we talked about.
For example, the scene and resource manager will figure out, there’s a new message. I have to create a node, put it in the tree. It’s an image node, so it checks if that image is available or not. It issues a download request. Maybe it animates some properties if necessary. Once the image is downloaded, it gets decoded, uploaded to the GPU memory, and after that, the high-level renderer here, from the scene tree that could be quite big, it figures out what subset of nodes is visible on the screen and then issues commands, the C++ layer, that’s with gray, to draw pixels on the screen. At the end of it all, you’ll have The Marvelous Mrs. Maisel image in there as it should be.
This is how it used to work. When we added Rust here, we had huge gains in animation fluidity and these types of things. However, things like input latency didn’t quite improve, so the input latency is basically the time it takes from when you press a button on your remote control, in our case, until the application responds to your input. That’s what we call input latency. That didn’t improve much or at all because, basically, all of those decisions and all that business logic, like what happens as a response to an input event to the scene tree, is in JavaScript. That’s a fairly slow language, especially since some of this hardware can be as, maybe, dual-core devices with not even 1 gigahertz worth of CPU speed and not much memory.
Actually, those are medium. We have some that are even slower, so running JavaScript on those is time-consuming. We wanted to improve this input latency, and in the end, we did, but we ended up with this architecture. The engine is more or less the same, except we added certain systems that are specific to this application. For example, focus management, layout engine is now part of the engine. I didn’t put it on this slide because it goes into that scene management. On top of it, we built a new Rust UI SDK that we then use to build the application. Everything is now in Rust. It’s one single language. You don’t even have the message bus to worry about. That wasn’t even that slow anyway. It was almost instantaneous. The problem was JavaScript, so we don’t have that anymore. We’re actually not quite here because we are deploying this iteratively, page by page, because we wanted to get this out faster in front of customers, but we will get here early next year.
UI Code Using the New Rust UI SDK
This is going to be a bit intense, but here’s some UI code with Rust, and this is actually working UI code that our UI SDK supports. I’m going to walk you through it because there’s a few concepts here that I think are important. When it comes to UI programming, Rust isn’t known for having lots of libraries, and then the ecosystem is not quite there. We had to build our own. We’d use some ideas from Leptos, like the signals that I’m going to talk about, but this is how things look like today. If you’re familiar with React and SolidJS and those things, you’ll see some familiar things here.
First, you might notice, is that Composer macro over there, that gets attached to this function here that returns a composable. A composable is a reusable piece of tree, of hierarchy of nodes that we can plug in with other reusable bits and compose them, basically, together. This is our way of reusing UI. This Composer macro actually doesn’t do that much except generate boilerplate code that gives us some nicer functionality in that compose macro you see later down in the function. It allows us to have named arguments as well as nicer error messages and optional arguments that might miss for functions.
This is some quality-of-life thing. Then our developers don’t need to specify every argument to these functions, like this hello function here that just takes a name as a string. In this case, the name is mandatory, but we can have optional arguments with optional values that you don’t need to specify. Also, you can specify arguments in any order as long as you name them, and we’ll see that below. It’s just super nice quality-of-life thing. I wish Rust supported this out of the box for functions, but it doesn’t, so this is where we are.
Then, this is the core principle of our UI SDK. It uses signals and effects. The signal is a special value, so this name here will shadow the string above. Basically, this name is a signal, and that means when it changes, it will trigger effects that use it. For example, when this name changes, it will execute the function in this memo, which is a special signal, and it creates a new hello message with the new value the name has been set to. It executes the function you see here. It formats it. It concatenates, and it will give something like Hello Billy, or whatever. Then hello message is a special signal that also will trigger effects. Here you see in this function, we use the hello message.
Whenever the hello message is updated, it will trigger this effect that we call here with create effect. This is very similar to how SolidJS, or if you’re familiar with React, works. Actually, this is quite important because this is also what helps UI engineers be productive in this framework without knowing much Rust actually. The core of our UI engine is signals, effects, and memos, which are special signals that only trigger effects if the values that they got updated to are different from the previous value. By default, they just trigger the effect anyway.
Then, we have this other macro here, which is the compose macro, and this does the heavy lifting. This is where you define how your UI hierarchy looks like. Here we have a row that then has children, which are label nodes. You see here the label has a text that is either a hardcoded value with three exclamation marks as a string, or it can take a signal that wraps a string. The first label here will be updated whenever hello message gets updated. Without the UI engineer doing anything, it just happens automatically that hello message gets updated, the label itself will render the new text, and it just works. If you’re a UI engineer, this is the code you write. It’s fairly easy to understand once you get the idea. Here we have some examples, for example, ChannelCard and MovieCard are just some other functions that allow you to pass parameters like a name, a main_texture, and maybe a title, a main_texture, and so on.
Again, they could have optional parameters that you don’t see here. You can even put signals instead of those hardcoded values. It doesn’t quite matter, it’s just these will be siblings of those two labels. All the way down we have button with a text, that says Click. Then it has a few callbacks on select, on click, and stuff like that, that are functions that get triggered whenever those events happen in the UI engine. For example, whenever we select this button, we set a signal. That name gets set to a new name. This triggers a cascade of actions, hello message gets updated to hello new name. Then, the effects gets trigger because that’s a new value, so that thing will be printed.
Then, lastly, the first label you see here, will get updated to a new value. Lastly, this row has properties or modifiers, so we can modify the background color. In this case, it’s just set to a hardcoded value of blue. However, we support signals to be passed here as well. If you have a color, that’s a signal of a color. Whenever that gets set, maybe on a timer or whatever, the color of the node just gets updated and you pass it here exactly like we set this parameter. That’s another powerful way where we get behavior or effects as a consequence of business logic that happens in the UI code. This is what your engineers deal with, and it’s quite high-level, and it’s very similar to other UI engines, but it’s in Rust this time.
When we run that compose macro, this is how the UI hierarchy will look like in the scene tree. You have the row, and then it has a label. Then labels are special because they’re widgets. Composables can be built out of widgets, which are special composables our UI SDK provides to the engineers, or other composables that eventually are built out of widgets. Widgets are then built out of a series of components. This is fairly important because we use entity component systems under the hood. Components, for now, you can think of them as structures without behavior, so just data without any behavior. The behavior comes from systems that operate on these components.
In this case, this label has a layout component that helps the layout system. A base component, let’s say maybe it has a position, a rotation, things like that. RenderInfo components, this is all the data you need to put the pixels on the screen for this widget once everything gets computed. A text component, this does text layout and things like that. Maybe a text cache component that is used to cache the text in the texture so we don’t draw it letter by letter.
The important bit is that widgets are special because they come as predefined composables from our UI SDK. Then, again, composables can be built out of other composables. This row has a few children here, but eventually it has to have widgets as the leaf nodes because those are the things that actually have the base behavior. Here maybe you have a button and another image, and the button has, all the way down, a focus component. This allows us to focus the button, and it gets automatically used by that system. The image component, again, just stores a URL and maybe the status, has this been downloaded, uploaded to GPU, and so on. It’s fairly simple. Basically, this architecture in our low-level engine is used to manage complexity in behavior. We’ll see a bit later how it works. Then we had another Movie Card in that example and, again, it eventually has to be built out of widgets.
Widgets are the things that our UI SDK provides to UI developers out of the box. They can be row, columns, image, labels, stack, rich text, which is special text nodes that allows you to have images embedded and things like that. Stacks, row list, and column list, and these are scrollable either horizontally or vertically. I think we added grid recently because we needed it for something, but basically, we build this as we build the app. This is what we support now. I think button is another one of them that’s just supported here out of the box that I somehow didn’t put. Then, each widget is an entity ID. It has an ID and a collection of components. Then, the lower-level engine uses systems to modify and update the components. ECS is this entity component system. It’s a way to organize your code and manage complexity without paying that much in terms of performance. It’s been used by game engines, not a lot, but for example, Overwatch used it.
Thief, I think, was the first game in 1998 that used it as a piece of trivia. It’s a very simple idea, which is, you have entities, and these are just IDs that map to components. You have components that are data without behavior. Then you have systems, which are basically functions that act on tuples of components. It always acts on more things at the time, not on one thing at a time. It’s a bit different than the other paradigms. It’s really good to create behavior, because if you want a certain behavior for an entity, you just add the component and then the systems that need that component automatically will just work because the component is there.
Here is how it might work in a game loop. For example, these systems are on the left side and then the components are on the right side. When I say components, you can basically imagine those as arrays and entity IDs as indices in those arrays. It’s a bit more complicated than that, but that’s basically it. Then the things on the left side with the yellow thing, those are systems, and they’re basically functions that operate on those arrays at the same time. Let’s say the resource management system needs to touch base components, image components, and read from them. This reading is with the white arrow, and it will write to RenderInfo components. For example, it will look where the image is, if it’s close to the screen, look at the base component. It looks at the image component that contains the URL. It checks the image status that will be there. Is it downloaded? Has it been uploaded to the GPU? If it has been decoded and uploaded to the GPU, we update the RenderInfo components so we can draw the thing later on the screen.
For this system, you need to have all three components on an entity, at least. You can have more, but we just ignore them. We don’t care. This system just looks at that slice of an object, which is the base components, the image components, and RenderInfo components. You have to have all three of them. If you have only two without the third one, that entity just isn’t touched by this system and it does nothing, the system widget. Then we have the layout system. Of course, this looks at a bunch of components and updates one at the end. It’s quite complicated, but layout is complicated anyway. At least that complication and that complexity sits within a file or a function. You can tell from the signature that this reads from a million things, writes to one, but it is what it is. You can’t quite build layout systems without touching all of those things. Maybe we have a text cache component that looks at text components and writes to a bunch of other things.
Again, you need to have all three of these for an entity such that is updated by this system. All the way at the end, we have the rendering system that looks at RenderInfo components, reads from them. It doesn’t write anywhere because it doesn’t need to update any component. It will just call the functions in the C++ code in the renderer backend to put things on the screen. It just reads through this and then updates your screen with new pixels. It sounds complicated, but it’s a very simple way to organize behavior. This has paid dividends organizing our low-level architecture like this for reasons that we’ll see a little bit later, how and why. Not only for the new application, but also the old application because they use the same low-level engine.
Again, going back to the architecture, this is what we have, Prime Video App at the top. We’ve seen how developers write the UI with composables using our UI SDK. Then we’ve seen how the UI SDK uses widgets that then get updated by the systems, and have components that are defined in the low-level engine. This is again, downloaded. Every time we write some new code, it goes through a pipeline, it gets built to WebAssembly, and then we just execute it on your TV set top box, whatever you have in your living room. Then we have the low-level stuff that interacts with the device that we try to keep as small as possible. This is what we shipped, I think, end of August. It’s live today.
The Good Parts
Good parts. Developer productivity, actually, this was great for us. Previously, when we rewrote the engine, we had a bunch of developers who knew C++ and switched to Rust, and we had good results there. In this case, we switched people who knew only JavaScript and TypeScript to Rust, and they only knew stuff like React and those frameworks. We switched them with our Rust UI SDK with no loss in productivity. This is both self-reported and compared with. Whenever we build a feature, we have other clients that don’t use this, so, for example, like the mobile client or the web client and so on. The Rust client, actually, when we were discussing some new features to be built now on all of the clients, was, I think, the second one in terms of speed, behind web. Then even mobile developers had higher estimations than we did here. Also, we did this whole rewrite in a really short amount of time. We had to be productive. We built the UI SDK and a large part of the app quite fast.
The reason why I think this is true is because we did a lot of work in developer experience with those macros that maybe look a bit shocking if you don’t know UI programming, but actually they felt very familiar to UI engineers. They could work with it right off the bat, they don’t have to deal with much complexity in the borrow checker. Usually, in the UI code, you can clone things if necessary, or even use a Rc and things like that. You all know, this is not super optimal. Yes, we came from JavaScript, so this is fine, I promise. The gnarly bits are down in the engine, and there we take a lot of care about data management and memory and so on. In the UI code, we can afford it easy. Even on the lowest level hardware, I have some slides that you’ll see the impact of this.
Another thing in the SDK, as the SDK and engine team, we chose some constraints and they helped us build a simpler UI SDK and ship it faster. For example, one constraint our UI engine has, I might show it to you, is that when you define a label or a widget or something like that, you cannot read properties from it unless you’ve been the one setting properties. It’s impossible to read where on the screen an element ends up after layout from the UI code. You never know. You just put them in there. We calculate things in the engine, but you can’t read things unless you’ve been the one saying, this is your color, blue. Then you’re like, yes, it’s in my variable. I can read it, of course. Things like that, you can’t read. This simplified vastly our UI architecture and we don’t have to deal with a bunch of things, and slowness because of it. It seems like a shocking thing. Maybe you need to know where on the screen. No, you don’t, because we shipped it.
There was no need to know where you are on the screen, and there was no need to read a property that you haven’t set. There are certain cases where we do notify UI developers through callbacks where they can attach a function and get notified if something happens. It’s very rare. It happens usually in case of focus management and things like that. You will get a function call that you’re focused, you’re not focused anymore, and that works fine. Again, it’s a tradeoff. It has worked perfectly fine for us. That’s something that I think also has helped productivity. We only had one instance where developers asked to read a value of layout because they wanted something to grow, and maybe at 70% of the thing, they wanted something else to happen. Just use a timer and that was fixed.
Another good thing is that we iteratively shipped this. This is only because we used, I think in my view, entity component systems as the basis of our lower-level engine. That low-level engine with the systems it has and the components it has, currently supports JavaScript pages. By pages, I mean everything on the screen is in Rust or everything on the screen is in JavaScript. For example, we shipped the profiles page, which is the page you select the profile. The collections page, that’s the page right after you select the profile and you see all of the categories, all of the movies and everything. The details page, which is, once you choose something to watch, you can go to that place and see maybe episodes or just more details about the movie, and press play. We still have to move the search page, settings, and a bunch of other smaller pages. Those are still in JavaScript. This is work in progress, so we’re just moving them over. It’s just a function of time. We only have 20 people for both the UI SDK and the application. It takes a bit to move everything. It’s just time.
Another reason, it’s just work in progress. We think it was good. That entity component system managed perfectly fine to have these two running side-by-side. I don’t think we had one bug because of this. We only had to do some extra work to synchronize a bunch of state between these things, like the stack that you used to go back, the back stack and things like that, but it was worth it in the end. We got this out really fast. We actually first shipped the profiles page and then added the collections page and then the details page and then live and linear and whatnot. That’s nice.
Another good part is, in my opinion, we built tools as part of building this UI SDK. Because we built an SDK, so we had to build tools. I think one winning move here was, it’s really easy in our codebase to add a new tool, mostly because we use egui, which is this Rust immediate mode UI library. You see there like the resource manager just appears on top of the UI. This is something a developer built because he was debugging an issue where a texture wasn’t loading and he was trying to figure out, how much memory do we have? Is this a memory thing? The resource manager maybe didn’t do something right. It just made it very easy to build tools. We built tools in parallel with building the application and the UI SDK.
In reality, these are way below what you’d expect from browsers and things like that, but with literally 20% of the tools, you get 80% done. It’s absolutely true. You just need mostly the basics. Of course, we have debugger and things like that that just work, but these are UI specific tools. We have layout inspectors and all sorts of other things, so you can figure out if you set the wrong property. Another cool thing, in my opinion, so we built this, which is essentially a rewrite of the whole Prime Video App. Obviously, we’re against these things without a lot of data. One thing that really helped us make a point that this is worth it is to make a prototype that wasn’t cheating, that we showed to leadership around, this is how it feels on the device before what we did, and this is with this new thing.
Literally, features that were impossible before, like layout animations, are just super easy to do now. You see here, things are growing, layout just works, it rearranges everything. Things appear and disappear. Again, this is a layout animation here. Of course, this is programmer art, but has nothing to do with designers. We are just showcasing capabilities on a device. As you can see, things are over icons and under, it’s just a prototype, but it felt so much nicer and responsive compared to what you could get on a device that it just convinced people instantly that it’s worth the risk of building a UI in Rust and WebAssembly. Because even though we added Rust and it was part of our tech stack, we were using it for low-level bits, but this showed us that we can take a risk and try to build some UI in it.
Here are some results. This is a really low-end device where input latency for the main page for collection page was as bad as 247 milliseconds, 250 milliseconds, horrible input latency, with the orange color, this is in JavaScript. With Rust in blue, 33 milliseconds, easy. Similarly, details page, 440 milliseconds. This also includes layout time, because if you press a button as the page loads and we do layout, you might wait that much. This is max. The device is very slow. Again, 30 milliseconds, because layout animations means we need to run layout as fast as an animation frame, which is usually 16 milliseconds or 30 milliseconds at 30 FPS. It’s way faster and way more responsive. Again, that line is basically flat. It was great. Other devices have been closer to those two lines, but I picked this example because it really showcases even on the lowest-end device, you can get great results. The medium devices were like 70 milliseconds, and they went down to 16 or 33, but this is like the worst of them all. We have that.
The Ugly Parts
Ugly parts. WebAssembly System Interface is quite new. WebAssembly in general is quite new. We’re part of the W3C community. We’re working with them around features, things like that. There are certain things that are lacking. For example, we did add threads, but also there’s things that happen in the ecosystem that break our code sometimes because we use something that’s not fully standardized in production for a while. One such example was recently Rust 1.82, enabled some feature by default for WebAssembly WASI builds, that basically didn’t work on older WebAssembly virtual machines that we had in production. We basically now have a way to disable it, even if you have a new default and things like that. It’s worth it for us. That’s something to think about.
Also, WebAssembly System Interface keeps evolving and adding new features, and we’re trying to be active as part of that effort as well. It requires engineering effort. We can’t just quite take a dependency, or specifically on WebAssembly, and just be like, let’s see where this ship goes. You need to get involved in there and help with feedback, with work on features and so on. Another one we found out is panic-free code is really hard. Of course, exceptions should be for exceptional things, but that’s not how people write JavaScript. When the code panics in our new Rust app, the whole app gets just basically demolished, it crashes. You need to restart it from your TV menu. It’s really annoying. Panics shouldn’t quite happen. It’s very easy to cause a Rust panic, just access an array with the wrong index, you panic, game over. Then, that’s it. If you’re an engineer who only worked in JavaScript, maybe you’re familiar with exceptions, you can try-catch somewhere.
Even if it’s not ideal, you can catch the exception and maybe reset the customer at some nice position, closer to where they were before or literally where they were before. It’s impossible with our new app, which is really annoying. We, of course, use Clippy to ban unwraps and expect and those things. We ban unsafe code, except in one engine crate that has to interact with the lower-level bits. Again, it required a bit of education for our UI engineers to rely on this pattern of using the result type from Rust and get comfortable with the idea that there is no stack unwinding, especially there is no stack unwinding in WebAssembly, which is tied to the first point. You can’t even catch that in a panic handler. It just aborts the program. Again, this pretty big pain point for us.
In the end, obviously we shipped, so we’re happy. We almost never crashed, but it requires a bunch of work. This also generated a bunch of work on us because we were depending on some third-party libraries that were very happily panicking whenever you were calling some functions in a bit of a maybe not super correct way. Again, we would rather have results instead of panics for those cases. It led to a bit of work there that we didn’t quite expect. That’s something to think about especially in UI programming, or especially if you go, like we did, from JavaScript to Rust and WebAssembly.
The Bytecode Alliance
The Bytecode Alliance is this nonprofit organization we’re part of, a bunch of companies are part of it, and builds on open-source standards like WebAssembly, WebAssembly System Interface. Then, the WebAssembly Micro Runtime, which is the virtual machine we use, is built over there, as well as Wasmtime, which is another popular Rust one, implemented in Rust this time. WebAssembly Micro Runtime is C. It’s a good place to look at if you’re interested in using Rust in production, and especially using WebAssembly in production more specifically. In our case, it comes with Rust and everything.
Questions and Answers
Participant: You mentioned you don’t use this for your web clients. Would you think that something like this could work with using WebGL as the rendering target?
Ene: We did some comparisons on devices. There’s a bunch of pain points. First pain point is on the ones we do have to use a browser, because there’s no space on the flash, on some set top boxes. The problem is those are some version of WebKit that has no WebAssembly. That’s the big hurdle for us there. It could be possible. We did some experiments and it worked, but you do lose a few things that browsers have that we don’t. Today, it’s not worth it for us because those have very few customers. They work fairly ok in terms of comparing them to even the system UI. Even though they don’t hit these numbers, it would be a significant amount of effort to get this SDK to work on a browser.
Right now, it’s just quite simple because it has one target, the one that has the native VM. It requires a bunch of functions from the native VM that we expose that aren’t standard. Getting those would probably require to pipe them to JavaScript. Then you’re like, what’s going on? You might lose some performance and things like that. It’s a bit of a tricky one, but we’re keeping an eye on it.
See more presentations with transcripts
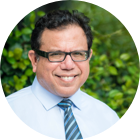
MMS • RSS
Posted on mongodb google news. Visit mongodb google news
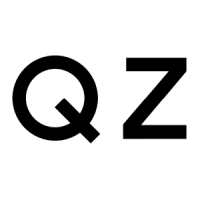
In This Story
MongoDB Inc. (MDB+0.51%) has submitted its Form 10-K filing for the fiscal year ended January 31, 2025.
The filing reports total revenue of $2,006.4 million, a 19% increase from $1,683.0 million in the previous fiscal year. This growth was primarily driven by a 19% increase in subscription revenue, which totaled $1,943.9 million.
MongoDB Atlas, the company’s database-as-a-service offering, represented 70% of total revenue, up from 66% in the prior year. The company noted continued growth in both self-serve and direct sales customers for MongoDB Atlas.
Advertisement
The company reported a net loss of $129.1 million, an improvement from a net loss of $176.6 million in the previous year. This was attributed to increased revenue and cost management.
Advertisement
Operating expenses increased to $1,687.2 million, up from $1,492.3 million in the previous year, with sales and marketing expenses accounting for the largest portion at $871.1 million.
Advertisement
Research and development expenses rose to $596.8 million, reflecting continued investment in product development and innovation.
The company ended the fiscal year with $2.3 billion in cash, cash equivalents, and short-term investments, providing a strong liquidity position.
Advertisement
MongoDB highlighted its ongoing focus on expanding its customer base, with over 54,500 customers as of January 31, 2025, compared to over 47,800 customers the previous year.
The filing also detailed the company’s efforts to increase sales within its existing customer base, noting a net ARR expansion rate of 118% as of January 31, 2025.
Advertisement
MongoDB continues to invest in its global reach, with 46% of total revenue generated outside the United States.
The company addressed risks related to macroeconomic conditions, including inflation and geopolitical instability, which could impact future growth and financial performance.
Advertisement
This content was summarized by generative artificial intelligence using public filings retrieved from SEC.gov. The original data was derived from the MongoDB Inc. annual 10-K report dated March 21, 2025. To report an error, please email earnings@qz.com.
Article originally posted on mongodb google news. Visit mongodb google news
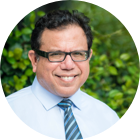
MMS • RSS
Posted on mongodb google news. Visit mongodb google news
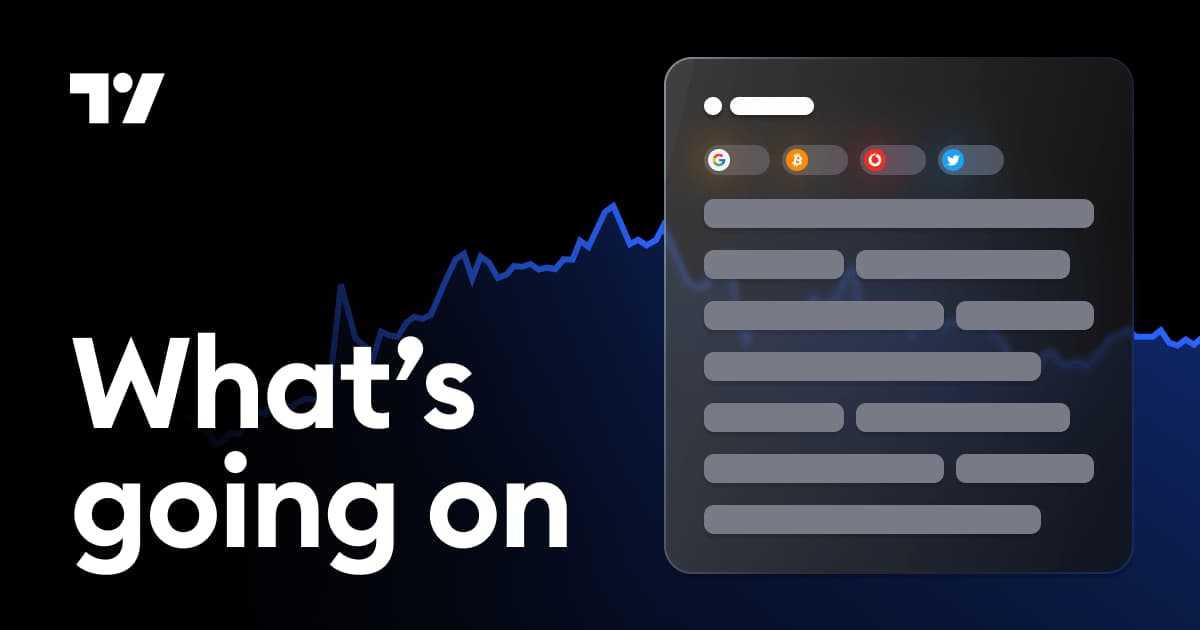
MongoDB, Inc., a leading provider of modern, general-purpose database platforms, has released its annual 10-K report for the fiscal year ended January 31, 2025. The report details the company’s financial performance, business operations, strategic initiatives, and the challenges it faces in the competitive database software market. MongoDB continues to expand its market presence with significant growth in revenue and customer base, while also navigating various risks and investing in future growth.
Financial Highlights
Total Revenue: $2,006.4 million, reflecting a 19% increase from the previous year driven by increased demand for the company’s platform and related services.
Gross Profit: $1,471.1 million, representing a gross margin of 73%, with a slight decline due to increased third-party cloud infrastructure costs.
Loss from Operations: $(216.1) million, an improvement from the previous year, primarily due to higher sales and marketing spend and research and development costs.
Net Loss: $(129.1) million, a reduction in net loss compared to the previous year, driven by higher interest income and improved operating cash flow.
Net Loss Per Share: $(1.73), showing an improvement from the previous year’s $(2.48) per share, reflecting the company’s efforts in cost management and revenue growth.
Business Highlights
Revenue Segments: MongoDB Atlas, the company’s multi-cloud database-as-a-service offering, represented 70% of total revenue for the fiscal year ended January 31, 2025, up from 66% in the prior year. MongoDB Enterprise Advanced, the self-managed commercial offering, accounted for 24% of subscription revenue.
Geographical Performance: Revenue generated outside of the United States was 46% of total revenue for the fiscal year ended January 31, 2025, consistent with the previous year, indicating stable international market performance.
New Product Launches: In 2023, MongoDB announced the general availability of Relational Migrator, a tool designed to facilitate the migration of applications from legacy relational databases to MongoDB.
New Product Launches: During 2024, MongoDB introduced version 8.0 of its platform, enhancing performance, security, and availability. New features included improvements to Queryable Encryption and the addition of Atlas Stream Processing.
Sales Units: As of January 31, 2025, MongoDB had over 54,500 customers across more than 100 countries, with over 7,500 customers sold through direct sales and channel partners, accounting for 88% of subscription revenue.
Future Outlook: MongoDB plans to continue investing in product development, sales, and marketing to expand its customer base and increase market penetration, with a focus on maintaining product leadership and developer mindshare. The company anticipates continued macroeconomic headwinds impacting the growth rate of existing MongoDB Atlas applications in the short term but remains committed to leveraging its large market opportunity.
Strategic Initiatives
Strategic Initiatives: MongoDB is focused on expanding its customer base and global reach, having increased its customer count to over 54,500 across more than 100 countries. The company is investing in its sales and marketing efforts, as well as in its developer community outreach, to drive customer acquisition. Additionally, MongoDB is committed to extending its product leadership by investing in research and development, having spent $2.5 billion since inception to enhance its developer data platform and introduce new features such as MongoDB version 8.0 and Atlas Stream Processing.
Capital Management: MongoDB’s capital management activities include the settlement of Capped Calls associated with the 2024 Notes, resulting in a cash inflow of $170.6 million. The company also issued a notice of redemption for its 2026 Notes, leading to the conversion of approximately $1.1 billion aggregate principal amount into common stock. MongoDB’s principal sources of liquidity as of January 31, 2025, include cash, cash equivalents, short-term investments, and restricted cash totaling $2.3 billion. The company has generated positive operating cash flows in recent years, with $150.2 million in operating cash flow for the year ended January 31, 2025.
Future Outlook: MongoDB plans to continue investing in growth and scaling its business, focusing on long-term revenue potential. The company expects to incur operating losses and may experience negative cash flows from operations in the future as it invests in strategic initiatives. MongoDB is also exploring opportunities for additional equity or debt financing to support its growth strategy. The company remains vigilant in monitoring macroeconomic conditions and their impact on its business, adjusting its practices as necessary to maintain financial stability and capitalize on market opportunities.
Challenges and Risks
Challenges and Risks: The company faces several significant risks that could impact its business operations and financial results. Key risks include unfavorable conditions in the industry or global economy, which could limit growth and adversely affect results. The company’s reliance on customer renewals and expansion of software usage is critical, and any decline in renewals could harm the business. The company also has a limited operating history, making future results difficult to predict, and has a history of losses, which may continue as costs increase.
The company derives a majority of its revenue from MongoDB Atlas, and any failure to meet customer demands could adversely affect business prospects. Intense competition in the database software market, including from larger, more established companies, poses a significant threat. The company’s decision to offer Community Server under the Server Side Public License (SSPL) may impact adoption negatively, and the enforceability of open source licenses like the AGPL and SSPL is uncertain.
Operational risks include the need to effectively expand the sales and marketing organization to add new customers and increase sales. The company has experienced rapid growth, and failure to manage this growth effectively could impair business operations. Security breaches or incidents, particularly involving third-party service providers, could damage the company’s reputation and lead to significant liability.
Regulatory risks are also prominent, with stringent and evolving data privacy and security laws in the U.S. and abroad. Compliance with these laws is complex and costly, and failure to comply could lead to regulatory actions and reputational harm. The company also faces risks related to intellectual property, including potential litigation and the need to protect its intellectual property rights.
Management acknowledges the challenges posed by the competitive landscape and the need to continue investing in sales and marketing to drive growth. The company is focused on enhancing its product offerings and expanding its customer base to mitigate these risks. Management also highlights the importance of maintaining high-quality support to ensure customer satisfaction and retention.
The company is exposed to market risks, including fluctuations in foreign currency exchange rates, which could adversely affect financial results. Economic uncertainties, such as inflation and interest rate changes, also pose risks to the company’s financial stability. Management is monitoring these risks closely and is prepared to adjust strategies as needed to mitigate potential impacts.
SEC Filing: MongoDB, Inc. [ MDB ] – 10-K – Mar. 20, 2025
Article originally posted on mongodb google news. Visit mongodb google news
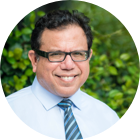
MMS • Steef-Jan Wiggers
Article originally posted on InfoQ. Visit InfoQ
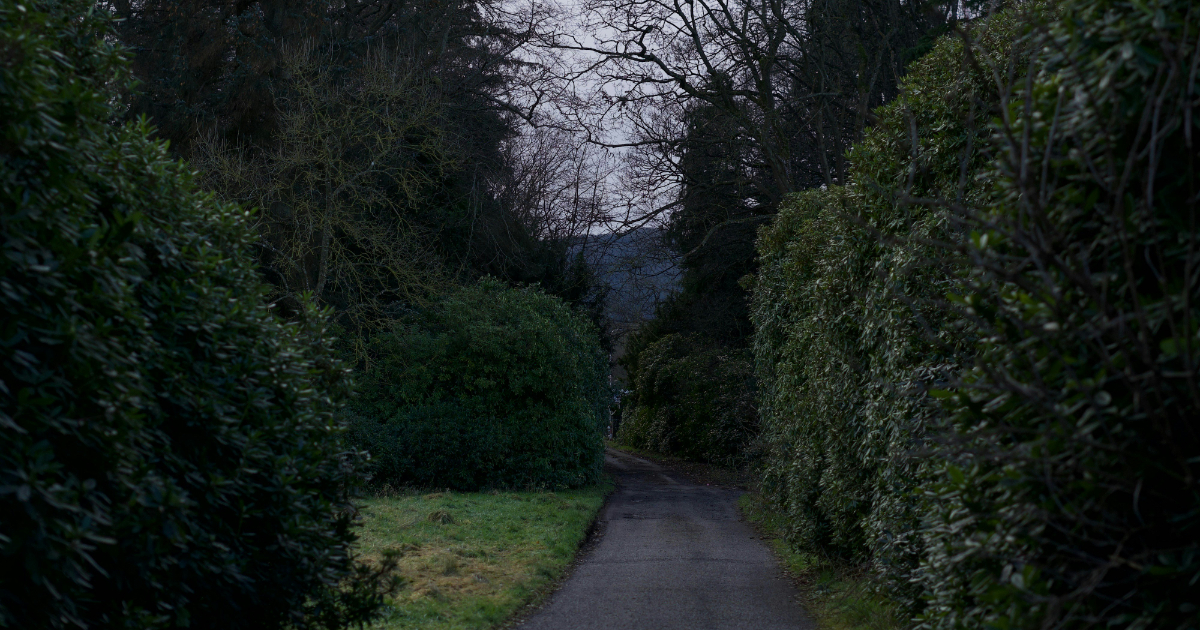
Google Cloud has unveiled its new A4 virtual machines (VMs) in preview, powered by NVIDIA’s Blackwell B200 GPUs, to address the increasing demands of advanced artificial intelligence (AI) workloads. The offering aims to accelerate AI model training, fine-tuning, and inference by combining Google’s infrastructure with NVIDIA’s hardware.
The A4 VM features eight Blackwell GPUs interconnected via fifth-generation NVIDIA NVLink, providing a 2.25x increase in peak compute and high bandwidth memory (HBM) capacity compared to the previous generation A3 High VMs. This performance enhancement addresses the growing complexity of AI models, which require powerful accelerators and high-speed interconnects. Key features include enhanced networking, Google Kubernetes Engine (GKE) integration, Vertex AI accessibility, open software optimization, a hypercompute cluster, and flexible consumption models.
Thomas Kurian, CEO of Google Cloud, announced the launch on X, highlighting Google Cloud as the first cloud provider to bring the NVIDIA B200 GPUs to customers.
Blackwell has made its Google Cloud debut by launching our new A4 VMs powered by NVIDIA B200. We’re the first cloud provider to bring B200 to customers, and we can’t wait to see how this powerful platform accelerates your AI workloads.
Specifically, the A4 VMs utilize Google’s Titanium ML network adapter and NVIDIA ConnectX-7 NICs, delivering 3.2 Tbps of GPU-to-GPU traffic with RDMA over Converged Ethernet (RoCE). The Jupiter network fabric supports scaling to tens of thousands of GPUs with 13 Petabits/sec of bi-sectional bandwidth. Native integration with GKE, supporting up to 65,000 nodes per cluster, facilitates a robust AI platform. The VMs are accessible through Vertex AI, Google’s unified AI development platform, powered by the AI Hypercomputer architecture. Google is also collaborating with NVIDIA to optimize JAX and XLA for efficient collective communication and computation on GPUs.
Furthermore, a new hypercompute cluster system simplifies the deployment and management of large-scale AI workloads across thousands of A4 VMs. This system focuses on high performance through co-location, optimized resource scheduling with GKE and Slurm, reliability through self-healing capabilities, enhanced observability, and automated provisioning. Flexible consumption models provide optimized AI workload consumption, including the Dynamic Workload Scheduler with Flex Start and Calendar modes.
Sai Ruhul, an entrepreneur on X, highlighted analyst estimates that the Blackwell GPUs could be 10-100x faster than NVIDIA’s current Hopper/A100 GPUs for large transformer model workloads requiring multi-GPU scaling. This represents a significant leap in scale for accelerating “Trillion-Parameter AI” models.
In addition, Naeem Aslam, a CIO at Zaye Capital Markets, tweeted on X:
Google’s integration of NVIDIA Blackwell GPUs into its cloud with A4 VMs could enhance computational power for AI and data processing. This partnership is likely to increase demand for NVIDIA’s GPUs, boosting its position in cloud infrastructure markets.
Lastly, this release provides developers access to the latest NVIDIA Blackwell GPUs within Google Cloud’s infrastructure, offering substantial performance improvements for AI applications.
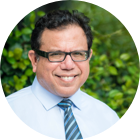
MMS • RSS
Posted on mongodb google news. Visit mongodb google news
William Blair Investment Management LLC cut its stake in shares of MongoDB, Inc. (NASDAQ:MDB – Free Report) by 6.5% in the 4th quarter, according to the company in its most recent disclosure with the Securities and Exchange Commission (SEC). The firm owned 25,840 shares of the company’s stock after selling 1,807 shares during the quarter. William Blair Investment Management LLC’s holdings in MongoDB were worth $6,016,000 as of its most recent SEC filing.
Other hedge funds have also recently modified their holdings of the company. Hilltop National Bank lifted its stake in shares of MongoDB by 47.2% in the 4th quarter. Hilltop National Bank now owns 131 shares of the company’s stock worth $30,000 after acquiring an additional 42 shares during the period. Brooklyn Investment Group acquired a new stake in MongoDB in the third quarter valued at about $36,000. Continuum Advisory LLC lifted its position in MongoDB by 621.1% in the third quarter. Continuum Advisory LLC now owns 137 shares of the company’s stock valued at $40,000 after purchasing an additional 118 shares during the period. NCP Inc. acquired a new stake in shares of MongoDB in the 4th quarter valued at approximately $35,000. Finally, Wilmington Savings Fund Society FSB acquired a new stake in shares of MongoDB in the 3rd quarter valued at approximately $44,000. 89.29% of the stock is owned by institutional investors and hedge funds.
MongoDB Stock Performance
Shares of MDB stock opened at $190.06 on Thursday. MongoDB, Inc. has a one year low of $173.13 and a one year high of $387.19. The company has a 50 day moving average price of $253.21 and a 200 day moving average price of $270.89. The firm has a market capitalization of $14.15 billion, a price-to-earnings ratio of -69.36 and a beta of 1.30.
MongoDB (NASDAQ:MDB – Get Free Report) last released its quarterly earnings data on Wednesday, March 5th. The company reported $0.19 EPS for the quarter, missing analysts’ consensus estimates of $0.64 by ($0.45). The firm had revenue of $548.40 million for the quarter, compared to analyst estimates of $519.65 million. MongoDB had a negative return on equity of 12.22% and a negative net margin of 10.46%. During the same quarter in the previous year, the company earned $0.86 earnings per share. As a group, equities analysts forecast that MongoDB, Inc. will post -1.78 EPS for the current fiscal year.
Insiders Place Their Bets
In other news, insider Cedric Pech sold 287 shares of the stock in a transaction on Thursday, January 2nd. The shares were sold at an average price of $234.09, for a total value of $67,183.83. Following the completion of the sale, the insider now owns 24,390 shares of the company’s stock, valued at $5,709,455.10. This represents a 1.16 % decrease in their ownership of the stock. The transaction was disclosed in a legal filing with the Securities & Exchange Commission, which is available at this hyperlink. Also, CAO Thomas Bull sold 169 shares of the stock in a transaction on Thursday, January 2nd. The stock was sold at an average price of $234.09, for a total transaction of $39,561.21. Following the completion of the sale, the chief accounting officer now directly owns 14,899 shares of the company’s stock, valued at $3,487,706.91. The trade was a 1.12 % decrease in their position. The disclosure for this sale can be found here. Insiders sold 43,139 shares of company stock worth $11,328,869 over the last three months. 3.60% of the stock is owned by insiders.
Analysts Set New Price Targets
A number of equities research analysts have commented on the company. Bank of America reduced their target price on MongoDB from $420.00 to $286.00 and set a “buy” rating for the company in a report on Thursday, March 6th. Tigress Financial raised their price objective on shares of MongoDB from $400.00 to $430.00 and gave the company a “buy” rating in a research note on Wednesday, December 18th. KeyCorp cut shares of MongoDB from a “strong-buy” rating to a “hold” rating in a research note on Wednesday, March 5th. Oppenheimer reduced their price target on shares of MongoDB from $400.00 to $330.00 and set an “outperform” rating for the company in a research report on Thursday, March 6th. Finally, Guggenheim upgraded shares of MongoDB from a “neutral” rating to a “buy” rating and set a $300.00 price target for the company in a research report on Monday, January 6th. Seven analysts have rated the stock with a hold rating and twenty-three have assigned a buy rating to the company. Based on data from MarketBeat, the stock currently has an average rating of “Moderate Buy” and a consensus target price of $320.70.
Check Out Our Latest Report on MongoDB
MongoDB Company Profile
MongoDB, Inc, together with its subsidiaries, provides general purpose database platform worldwide. The company provides MongoDB Atlas, a hosted multi-cloud database-as-a-service solution; MongoDB Enterprise Advanced, a commercial database server for enterprise customers to run in the cloud, on-premises, or in a hybrid environment; and Community Server, a free-to-download version of its database, which includes the functionality that developers need to get started with MongoDB.
See Also
Want to see what other hedge funds are holding MDB? Visit HoldingsChannel.com to get the latest 13F filings and insider trades for MongoDB, Inc. (NASDAQ:MDB – Free Report).
This instant news alert was generated by narrative science technology and financial data from MarketBeat in order to provide readers with the fastest and most accurate reporting. This story was reviewed by MarketBeat’s editorial team prior to publication. Please send any questions or comments about this story to contact@marketbeat.com.
Before you consider MongoDB, you’ll want to hear this.
MarketBeat keeps track of Wall Street’s top-rated and best performing research analysts and the stocks they recommend to their clients on a daily basis. MarketBeat has identified the five stocks that top analysts are quietly whispering to their clients to buy now before the broader market catches on… and MongoDB wasn’t on the list.
While MongoDB currently has a Moderate Buy rating among analysts, top-rated analysts believe these five stocks are better buys.
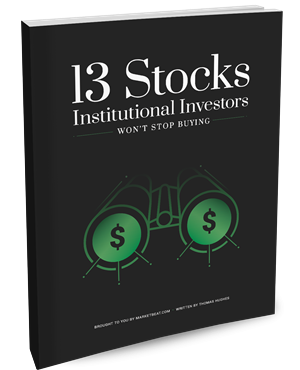
Which stocks are hedge funds and endowments buying in today’s market? Enter your email address and we’ll send you MarketBeat’s list of thirteen stocks that institutional investors are buying now.
Article originally posted on mongodb google news. Visit mongodb google news
Podcast: Claire Vo on Building High-Performing, Customer-Centric Teams in the Age of AI
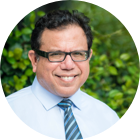
MMS • Claire Vo
Article originally posted on InfoQ. Visit InfoQ
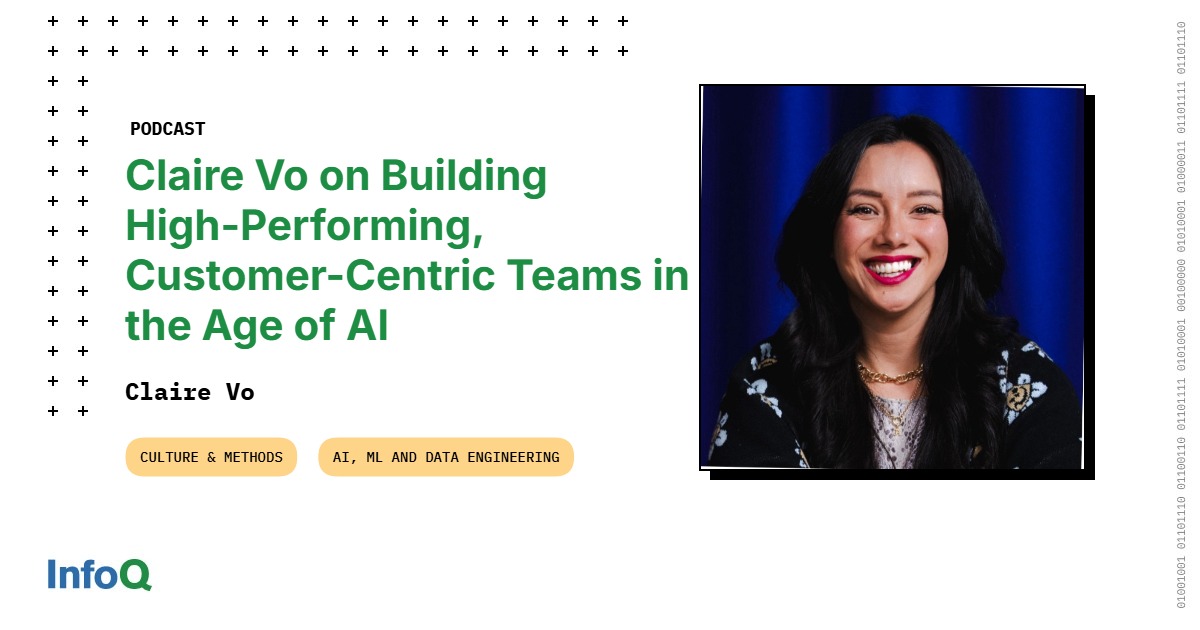
Transcript
Shane Hastie: Good day, folks. This is Shane Hastie from the InfoQ Engineering Culture Podcast. Today I’m sitting down with Claire Vo from LaunchDarkly. Claire, welcome. Thanks for taking the time to talk to us today.
Claire Vo: Thanks so much for having me.
Shane Hastie: My normal starting point in these conversations would be who’s Claire?
Introductions [00:48]
Claire Vo: That’s maybe a question I need to ask myself on a daily basis. Who is Claire? In the context of this conversation, I am Claire Vo. I’m the chief product and technology officer at LaunchDarkly. I lead our entire technology organization, so not just engineering, but product management, design, data. I even, pretty surprisingly, have a couple sellers on the team for our new incubation product. I have a pretty broad remit.
Functionally, my job is to build a really high performing team where we can make great investments in R&D, and bring great products to our customers, and great value for the company.
Shane Hastie: What is a high performing team today?
What is a high-performing team today? [01:29]
Claire Vo: It’s a really great question. For me, I think about performance from the lens of our ultimate goal. To me, our ultimate goal is to solve hard problems for customers that have value to those customers, that have commercial markets for them, and that can build enterprise value for the company. To me, a team that is building towards our ultimate goal, which is really customer-centric and value-driven, is what I like to see engineering organizations.
Of course, we want technical excellence. Of course, we want camaraderie, and culture, and engagement. Of course, we want innovation. And of course, we want to attract competitive talent. But at the end of the day, that is a means to the end. We’d really like to say customer-centric in what we’re trying to achieve for our colleagues and the business.
Shane Hastie: Customer-centric. As an engineer, how do I become customer-centric?
Engineers need to be customer-centric [02:29]
Claire Vo: Well, lucky for us, our customers are engineers. We’re in a really privileged spot. LaunchDarkly is the leading feature management experimentation platform in the market. We serve engineers, that’s our job. We build dev tools for engineers. Be build for builders. That’s actually one of the things that attracted me to LaunchDarkly, is it’s really candidly fun to be able to build for builders, and building technical tools for technical folks is very interesting.
One, whether you eat your own dog food or drink your own champagne, we are power users of LaunchDarkly at LaunchDarkly. We use LaunchDarkly to release, test, optimize our own code in production. We use it for small things, for big things. We use all the features. We take advantage of the full platform. One of the ways we become customer-centric as an organization is we act as customers.
That’s not just the engineering organization. Product managers are expected to use the product. Our designers are expected to use the product. The product is not just the UI and the web app, it’s our SDKs, it’s our data export tools, all those things that make up the whole platform. I would say that’s one primary way we’re a little advantaged in being customer-centric.
But the other way is we stay very close to customers. My expectation in my organization is that everybody’s having customer conversations. Live, real human-to-human customer conversations every week. That’s not just the realm of our salespeople or our customer success team. Engineers need to know their customers that they go to to discuss their most challenging skill issues. Product managers need to have a group of customers they can rely on for early feedback on products. We really stay close to customers.
Then I think the third thing is we really bring a beginner’s mindset to our product. LaunchDarkly has been in the market for over 10 years. In those 10 years, you can build up a lot of tolerance for the sharp edges in your product, or the things that you’ve gotten used to clicking around or hacking around. I really think it’s important for folks to come to their own products with a beginner mindset, look at it with fresh eyes, and think, “If I knew nothing about LaunchDarkly, would this be easy to do?” Those are just a couple ways that we’re really customer-centric in my organization.
Shane Hastie: You said make a great culture. What does that feel like? What does that look like?
What makes a great culture? [05:01]
Claire Vo: Yes. It’s really funny. I have this fancy, C-level title, and tend to go into growth and scale stage startups, so much later stage startups. Everybody thinks that I get hired to make teams act like a big company. I come in as the grownup and I say, “Okay, this is how you’re supposed to run an engineering organization at scale, or a product organization at scale”, et cetera. Of course, my leaders and my team across the board want to do things to professionalize the way we operate, and make sure that we’re showing up for customers with stability and pace. All those things are great. And I get hired to remind the team to operate like a startup.
To me, a great culture is one that is very close to customers, that moves fast. Where there are builders in the team who want to bring their technical, and intellectual, and creative skills to problems people have. I think a culture that is very close to how a startup would operate. Lower process, lower BS, lower politics, focus on the customer, focus on building, have fun. Those, to me, are the cultures I thrive in. Whether or not that’s the best culture or not, that is the culture that I prefer to operate in. Then the non-negotiable is, as I said up front, high performance. All of that does not matter unfortunately unless we build great products that have market acceptance that can grow the company to the ambitions that we have.
I do bring to great cultures a focus on what really is our job. Because I think a lot of times, teams lose sight of that. They think their job is to do the work that gets them promoted, or their job is to do the work to make them look good in front of executives. None of that is actually their job. Their job is to help us build a great business and have an impact on users. I think that centricity about the job to be done is really important in great cultures.
Shane Hastie: You also, when we were chatting earlier, spoke about a culture of experimentation. How does that play out?
Encouraging a culture of experimentation [07:06]
Claire Vo: Yes. What I really think is to build great products, you have to be quite hypothesis-driven. You have to build, test, iterate over time.
One of my things that I say commonly is no genius product managers. What I mean by no genius product managers is product managers can hone product sense, and build intuition, and have experience that helps them narrow the surface area of the right problem to solve. But the reality is we have to remain humbled by users and be able to be humbled by the market. It is less important to be right out the gate, and more important to be fast out the gate and listen to what users say.
I really do think it’s important that we approach every build as a hypothesis. “I believe that there’s a great market here because what I know is that this data tells us there’s an opportunity, and I think we can do X by building Y”. That’s a perfectly valid hypothesis. Then you have to have two things to make this culture of experimentation work. You have to have a real intellectual honesty accountability. “We had a hypothesis. Are we really holding ourselves accountable to that outcome?”
I’m sure you, like I have, have been in teams where everybody says, “Yes! We’re going to hit this OKR or this goal, and I have this beautiful strategy and plan to do it”. Then you build the beautiful strategy and the plan. In the end, you don’t really hit the OKR. People say, “Oh, well”, there’s this excuse and that excuse. You really have to have a culture of accountability. “We said we were going to do something. Did we do it? If we didn’t do it, let’s understand the why”.
The second thing you really have to have is a tolerance for failure. Which is the organization has to be resilient enough to tolerate getting it wrong. That means people have to be okay with being wrong. They have to be okay with saying, “My hypothesis was incorrect”. And the organization itself actually has to be embrace people that can do that, because those are the folks that have the iterative, scrappy mindset that’s really going to get you moving in the right direction with setbacks.
I think those two things. Of being intellectually honest and accountable, coupled with a high tolerance of failure and a hypothesis mindset, in a team that moves fast, you get to the right answer quickly and then you’re pretty unstoppable.
Shane Hastie: You have both product and engineering under one umbrella.
Claire Vo: Yes.
Shane Hastie: Most organizations don’t do that.
Having engineering and product under a single leader [09:32]
Claire Vo: More and more are, but the CPTO role is relatively rare. I’ve actually done it twice now. I’ve had a couple revs at this particular role.
Here’s where I think it works well. I think the technology team, or at least that’s what we call it. Some folks call it EPD, we call it technology. I’ve worked at other companies that have cute, little names for it. But that triad of functional teams, having a singular identity, I think is really healthy for culture. The idea that engineering and product are inherently at odds, that design is always an afterthought, I don’t think that’s necessary. I think it is often a reflection of how organizations are run under siloed executives.
I really believe that, whether or not it’s reporting to me, a singular identity as an organization that represents the R&D investment in a company is very healthy. It creates better collaboration. It creates more innovation. It allows you to identify and overcome friction within teams. It sets a standard talent bar across all those orgs. I think it’s very healthy and I’ve seen it play out.
I also think, in certain organizations, it can be really helpful for the CEO, as I say, to have a single person to hold accountability for R&D. Especially if, like at LaunchDarkly, we have a CEO that is not the founder, it can be very, very helpful to have an executive that represents the full investment in R&D, the team, the technology, and the product at the executive level. There’s full accountability across that investment, which in most software teams, is the biggest investment in the company. I think from executive organization perspective, it can be useful.
But I think the skillsets are pretty rare and it takes a certain type to be able to do it. I have a technical background. I’m a classic generalist. I’ve been a founder. I’ve actually done revs in all these organizations because I myself have the personality of curiosity and learning. I have worked in startups, which means you get exposed to a lot of things. I’ve built up the scale operational experience, technical expertise, and strategy experience to be able to operate across all these functions. Then of course, I have amazing leaders across those teams. But I have people that report to me that say, “I never want your job. I don’t want the whole thing. That seems hard”. There’s definitely folks that love the specialty, SVP Product, SVP Engineering role.
I do think from an identity perspective, from an R&D investment perspective, and for certain types, it can be a really functional structure for a software company.
Shane Hastie: Down at the place where the work gets done. How do we get the product and engineering folks working well together?
Getting product, design and engineering working together [12:30]
Claire Vo: I think there’s a couple things. And you forget one, design. Everybody always forgets design. That’s the other thing that I always do. I’m like, “D is part EPD”.
I think there’s been a lot of thought around this classic EPD triad, engineering, product, and design as shepherds for building a product experience. I think that continues to be true. But down at the team level, how do you actually make that happen? Well, one, I do think you have to have true triad-level accountability and parity on teams. Product doesn’t lead everybody else. Product, engineering, and design jointly are the leads for an initiative.
Then the second thing that I think folks forget that we do culturally is that doesn’t mean they’re siloed. That means they’re a cohesive unit. We have an operating principle in our technology organization called There Are No Lanes. What do we mean by there are no lanes? You don’t stay in your lane, and you don’t expect others to stay in their lane. There are no lanes. We have a shared goal. We are a team. We have skills that vary. If it makes sense for me to help in your lane, I have permission and accountability to do so.
There’s some pretty interesting examples of this in this new world of AI where designers can start to build code and prototypes. And engineers can start to generate product requirements. And product managers can start to wire frame and do some really interesting design work with these AI tools. Rather than saying, “No, that’s design’s job, or no, that’s product’s job”, what we’re embracing is this flexible creativity and skillset in our teams which allows us to move faster and build ultimately better products.
Those are the two things. I think parity at the lead level, and then real embrace of this one team mindset.
Shane Hastie: You touched on AI.
Claire Vo: Yes.
Shane Hastie: What is happening with the adoption of … And we’re in many ways very early in the AI revolution. But what’s happening in that team level you mentioned in using some of the tools?
The adoption of AI in development [14:38]
Claire Vo: It’s a combination of tremendous excitement and a slow pace of adoption. I have a very unique point of view on this. I am probably what one would call an AI maximalist. I am not very risk averse around this topic. I think, in fact, most teams are moving too slow against becoming more AI-driven or AI native. We’ve been fairly aggressive in our adoption and experimentation, and again there’s that word experimentation, our experimentation with different tools, because I really believe that fundamentally how software is built is changing. And I don’t mean over 10 years, I mean over 18 months. It is just night and day. The cost of building is collapsing. The way we build is collapsing. The tools we use are changing.
I really take the approach that I should not build the, for example, engineering organization of January 2025. I should not build that team. I should think, cast forward five or 10 years, “What is this team actually going to look like? How are they going to operate? What is the world going to look like?” And work back into what do we need to do now to be world-class then? I’m really, really very much on the edge.
I think what’s happening at the ends, at the leaf, is experimentation and pilots. I think there is increasing acknowledgement that, for example, coding copilots and coding agents have a place in the stack. There is a risk and compliance framework that can accept these as part of scaled engineering organizations. You can get over that hurdle, both from a security and an IP perspective, relatively well at this point, especially with the larger foundation model providers. Then it’s, well, what the heck do I do with this thing and how does it change how we work?
My general approach has been to, one, allocate budget to it. I don’t think you can experiment without money, so we put aside money for it. Even if it gets wasted, it’s worthwhile because we learned. Two, name people accountable for every experiment and have them articulate what the outcome of that experiment would be. Then three, it’s funny to say internally, but build in public. Which is we are very open with our experimentation in using these AI tools. We show the good, we show the bad. We have a Slack channel called Project Building with AI. People post, “I tried to have Cursor do this and it was amazing. I tried to have Devon do that and it failed. I tried to use V0 to do this and it was amazing. I tried to have ChatGPT do that and it failed”.
That very open, and again intellectually honest assessment, allows us to identify opportunities to really transform how we build products here at LaunchDarkly. I think there will be increasing excitement. I am definitely driving it, both tops down and bottoms up. We’re seeing real value, so there’s a there there.
Shane Hastie: You mentioned building the team for three, five, 10 years.
Claire Vo: Yes.
Shane Hastie: What is that team going to be?
What will teams look like in the future? [17:38]
Claire Vo: Oh, I have so many opinions on it. My real hypothesis is that teams will largely be led by what I’m calling the AI-powered triple threat. Somebody who is fluent enough across technology, product, and design to actually take those three roles and collapse them into one, and be a singular leader for a team or a business unit. I think that model is really starting to show up in my mind and it’ll be interesting to see if it actually shows up in organization charts. I think you will have teams that are combinations of humans and agents orchestrating across workflows. I think those teams will be building a lot of product.
I actually, in addition to being an AI maximalist, I’m an optimist. I think technology has generally only pushed us forward in the world. In building an amazing world with amazing product and amazing economic impact, and therefore amazing human impact. I think we’ll see a lot of really, really neat things be built. But I think the shapes of the teams are going to look so different.
Shane Hastie: What do we need to do to help the people of today become those team members?
The need to invest in learning and development [18:44]
Claire Vo: One, we need to invest in learning and development. This is a real upskilling moment. It’s really funny to take teams where folks have spent a tremendous amount of time in their academic training, and then their professional training. These are senior engineers, and staff engineers, and principle product managers who are so adept in what they do. And say, “Guess what? You are actually not going to know how to do your job in two years and I need to reskill you in how this is actually going to look”. I do think there’s this learning and development motion that needs to happen.
Then I think there is cultural things that, if you don’t have, it will make it very hard for you to get here. Again, they’re things that I have said are important before and I’ll continue to say. You have to have a culture of experimentation. You have to be willing to try things. You have to have a willingness to fail, and admit where things are working and where they’re not. Then you have to, I think in general, have an optimistic outlook. People can get very afraid about how changes will impact their jobs, their livelihoods. I think it is naive to ignore that and claim that that is not something that is on people’s minds.
And at the same time, I think leaders have the opportunity to frame and build companies that have tremendous upside for the individuals that participate in them. Professional, personal, economic, just core satisfaction. But I don’t think, if leaders ignore that piece of it, they will be very successful.
Shane Hastie: You mentioned reskill. You mentioned the culture. What are the skills?
New skills to learn [20:20]
Claire Vo: There’s a couple things that I think about. One. There is actually, from a technical perspective, you were talking about engineering, understanding how these models operate, how they operate inside products, what their tool sets are. There’s functionally this whole world of AI engineering. That I think is a skill that technically needs to be built in actually product, engineering, and design teams. That is just a whole new surface area of how we build software. It’s very different.
The second skill is how do I build alongside copilots, agents, and AI-enabled tools? What’s really interesting is it is muscle memory you have to break, not value you have to prove. What I mean by that is, a simple example. Everybody knows if they go to ChatGPT, they can accelerate some part of their day. I think people have generally accepted that, they have proven it to us all. They just forget to go to ChatGPT. They forget to make that part of their workflow because they’re so used to, when I have a task to do, this is the place I go to do it. Then I’m 75% down the path and I’m almost done, so I’ll just do it. I do think there’s this workflow operations model that you really have to, somebody when I went to lunch called it whole team behavior modification. You really have to get the whole team to start doing handoffs and work in a very different way. I think that’s pretty interesting.
Then the third thing is there are these tools themselves that have a learning curve. Let’s just take, for example, one of these AI-powered IDEs like Cursor. People get bad outcomes from Cursor because they don’t use Cursor well because they have not learned to use a conversational coding copilot well. There’s setup you have to do. There’s practices you have to do. I think there is actually learning the tools themselves enough to get value out of them that I also think is very important.
Shane Hastie: But those tools are changing so fast.
Claire Vo: Yes, but I feel like the skills are replicable across tools.
Shane Hastie: A lot of good stuff there. What haven’t I asked you that I should have done?
Engineering crossing time and space [22:23]
Claire Vo: One of the things that I don’t think we talked about is maybe how it’s going to change geography and how teams are composed across timezones and what it means. You and I are speaking from quite a distance. Post-COVID, we’ve gone through this radical reimagining that what work looks like. I think that AI itself will have impacts on who you hire, where you hire, when they work, how they work.
I actually had a moment this morning. I’m pretty experimental and I use Cognition Labs’ Devon copilot. This morning, it was so funny because it works synchronously. It does planning, and it’s an agent so it works synchronously. It’s working and clicking off tasks. But it is a copilot in some extent. Occasionally, Devon will ask me, “Hey, can you review this PR? Or I need this environment variable set up”, all those sorts of things. I was thinking there’s this challenge of this agent can work in infinite time space, and I myself am limited by my body.
I actually said to Devon this morning, it was so funny. I said, “Hey, just so you know, I’m going to go grab a cup of coffee. I’ll be back later. If you need something, I’m not going to be here”. It got my brain thinking that I think organizations are not prepared for how operationally this will cascade out across their orgs. They’re not playing those game theory games of if 10% of my engineering workforce actually becomes an agent, do I need follow the sun coverage on my human engineering team to keep that agent team productive?
Maybe I push it really far and I think about the future more than other folks do. But if you’re, for example, San Francisco-based or a US-based team, how you set up your team to operate alongside agents can be a competitive advantage and a strategic advantage that I don’t know if enough technology leaders are thinking through. I know you’re the people person, and soon enough you will be the people plus agent person, I’m presuming. Because you said, “I spend my time with the humans”. You’re going to spend your time with the humans and the prototype humans. But it is really something very interesting to think about, the human interactions both from a collaboration perspective, as just candidly, the operational perspective is something I think leaders need to consider.
Shane Hastie: Wow. Claire, thanks very much. If people want to continue the conversation, where do they find you?
Claire Vo: I am on X. And of course, I am on LinkedIn, Claire Vo.
Shane Hastie: Thank you so much.
Claire Vo: Thank you.
Mentioned:
.
From this page you also have access to our recorded show notes. They all have clickable links that will take you directly to that part of the audio.
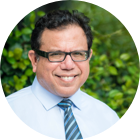
MMS • Aditya Kulkarni
Article originally posted on InfoQ. Visit InfoQ
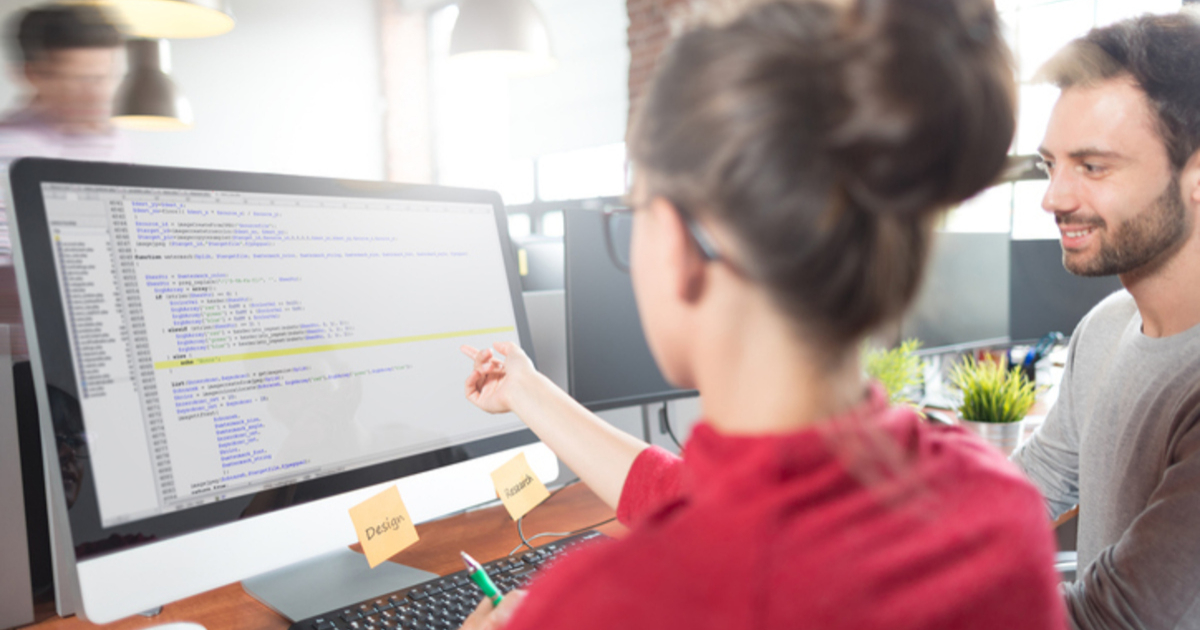
HashiCorp Cloud Platform (HCP) recently elaborated on automating the Terraform workspace creation using a TFE provider and building an onboarding module. This approach addresses the challenge of manual workspace creation, which has been a bottleneck for teams scaling their operations.
Emmanuel Rousselle, Staff Solutions Architect at HashiCorp, discussed the approach in a blog post. To illustrate how to automate the creation of HCP Terraform workspaces for an application team, Rousselle used an example of a fictitious tech company, HashiCups.
Associating with a common real-world scenario, HashiCups has successfully built its initial cloud landing zones using HCP Terraform. As the platform team begins onboarding an application team, they recognize the need to simplify the process. The traditional method of manually creating workspaces proves inefficient and prone to errors, prompting the team to explore automation solutions.
The process starts with a thorough assessment of requirements. The platform team meets with the application team to understand their familiarity with HCP Terraform workspaces, the environment landscape, and who should have permission to modify infrastructure configurations. Workspaces in HCP Terraform are isolated environments where teams manage specific infrastructure resources, each maintaining its state file for tracking changes.
The application team then adopts a three-environmental landscape, which requires the creation of three workspaces. The platform team also outlines additional requirements for HCP Terraform workspace default settings. Each application team is to have two distinct groups: one responsible for workspace administration and another with the necessary permissions to use the workspaces.
The onboarding module creation begins with developing key configuration files. The variables.tf
file defines essential variables, including application_id
, admin_team_name
, user_team_name
, and environment_names
. The environment_names
variable is validated to include a prod
environment, aligning with organizational requirements.
Next, the main.tf
file is used to define workspaces and team permissions.
resource "tfe_workspace" "workspace" {
for_each = toset(var.environment_names)
name = "${lower(var.application_id)}-${lower(each.value)}"
description = "Workspace for the ${each.value} environment of application ${var.application_id}"
allow_destroy_plan = each.value == "prod" ? false : true
}
data "tfe_team" "admin_team" {
name = var.admin_team_name
}
data "tfe_team" "user_team" {
name = var.user_team_name
}
resource "tfe_team_access" "admin_team_access" {
for_each = toset(var.environment_names)
workspace_id = tfe_workspace.workspace[each.value].id
team_id = data.tfe_team.admin_team.id
access = "admin"
}
resource "tfe_team_access" "user_team_access" {
for_each = toset(var.environment_names)
workspace_id = tfe_workspace.workspace[each.value].id
team_id = data.tfe_team.user_team.id
access = "write"
}
An example of main.tf file (Source)
For the production environment, the workspace is configured to prevent destroying plans adhering to organizational policies. The workspace naming follows a specific convention using string interpolation. The configuration utilizes data sources to fetch team information, though an alternative approach involves using team IDs for input variables, which can simplify the code but may reduce readability.
The Terraform module can accommodate different teams’ environments using variable fields instead of hardcoded values. Additionally, Rousselle has elaborated on the Terraform tests, which reside under the tests
directory to validate environment names and enforce consistent naming conventions. These tests ensured workspace names were in lowercase and critical environments like production were always included.
Furthermore, test suites are created for several key functions. They start by providing a valid TFE provider configuration and ensuring all necessary prerequisites are in place. The tests then verify that invalid environment landscapes, such as those missing the prod
environment or using incorrect names like production
, are correctly detected and cause the plan operation to fail.
As a side, HashiCorp has also announced the general availability of Terraform 1.11, which is ready to be used with HCP Terraform. This latest version introduces write-only arguments, allowing users to utilize ephemeral values in specific managed resource arguments, enhancing flexibility and functionality.
Finally, two essential files support the module’s documentation: README.md
and CHANGELOG.md
. The README.md
file gives users detailed instructions on the module’s purpose, usage, input variables, outputs, and specific requirements or dependencies. Meanwhile, the CHANGELOG.md
file tracks all significant changes and version updates over time, ensuring transparency and ease of maintenance for future users.
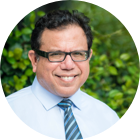
MMS • Bruno Couriol
Article originally posted on InfoQ. Visit InfoQ
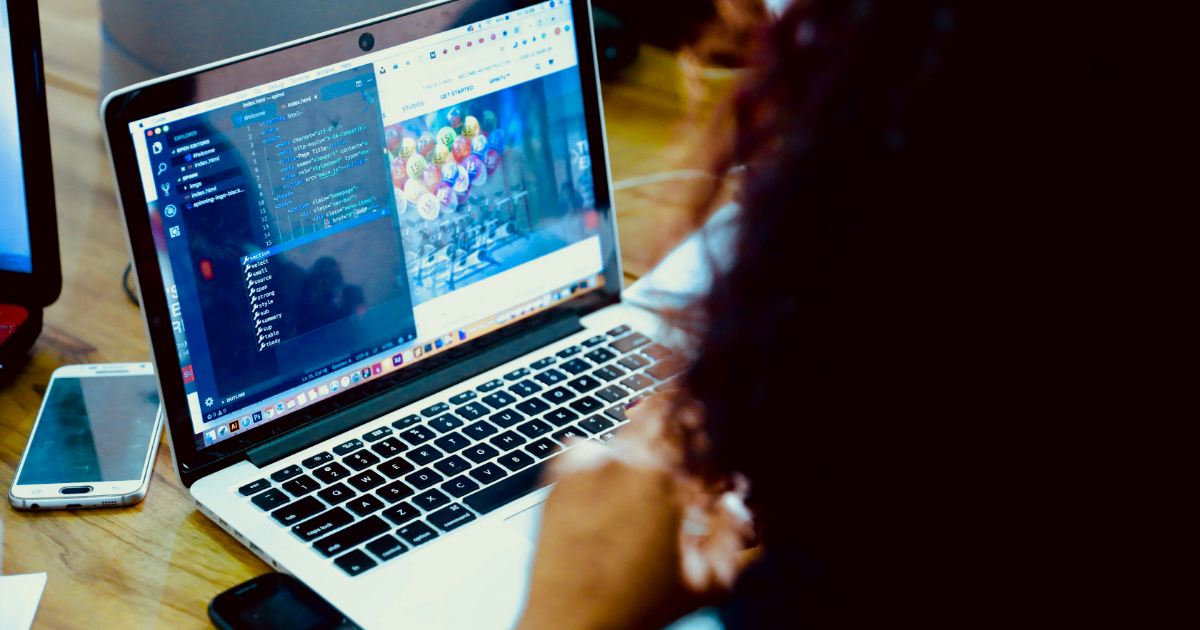
Following up on plans to turn ESLint into a general-purpose linter, the ESLint team recently announced official support for the CSS language. The support comes in addition to recently added support for JSON and Markdown linting.
The built-in CSS linting rules include checking against duplicate @import
rules, empty blocks, invalid at-rules, invalid properties, and enforcing the use of @layer
. CSS layers are a new addition to the CSS standard (see CSS Cascading and Inheritance Level 5) that give designers more control over the cascade so the resulting styles are predictably applied instead of being unexpectedly overridden by other rules in other places. A 2020 survey by Scout APM measured that developers spend over 5 hours per week on average debugging CSS issues with cascade/specificity bugs poised to be a major contributing factor.
However, the key lint rule addition is arguably the require-baseline
rule, which lets developers specify which CSS features to check against, depending on their level and maturity of adoption across browsers.
Baseline is an effort by the W3C WebDX Community Group to document which features are available in four core browsers: Chrome (desktop and Android), Edge, Firefox (desktop and Android), and Safari (macOS and iOS). As part of this effort, Baseline tracks which CSS features are available in which browsers. Widely available features are those supported by all core browsers for at least 30 months. Newly available features are those supported by all core browsers for less than 30 months. An example of linter configuration for a widely available baseline is as follows:
import css from "@eslint/css";
export default [
{
files: ["src/css/**/*.css"],
plugins: {
css,
},
language: "css/css",
rules: {
"css/no-duplicate-imports": "error",
"css/require-baseline": ["warn", {
available: "widely"
}]
},
},
];
CSS linting is accomplished using the @eslint/css
plugin:
npm install @eslint/css -D
Developers are encouraged to review the release note for a fuller account of the features included in the release together with technical details and code samples.
ESLint is an OpenJS Foundation project and is available as open-source software under the MIT license. Contributions are welcome via the ESLint GitHub repository. Contributors should follow the ESLint contribution guidelines.