Category: Uncategorized
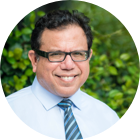
MMS • RSS
Posted on mongodb google news. Visit mongodb google news
EMC Capital Management trimmed its holdings in shares of MongoDB, Inc. (NASDAQ:MDB – Free Report) by 75.7% in the 4th quarter, according to its most recent disclosure with the SEC. The fund owned 1,920 shares of the company’s stock after selling 5,971 shares during the period. EMC Capital Management’s holdings in MongoDB were worth $447,000 as of its most recent SEC filing.
A number of other hedge funds have also recently made changes to their positions in the stock. Nisa Investment Advisors LLC raised its stake in MongoDB by 3.8% during the third quarter. Nisa Investment Advisors LLC now owns 1,090 shares of the company’s stock valued at $295,000 after buying an additional 40 shares during the last quarter. Hilltop National Bank raised its position in shares of MongoDB by 47.2% in the 4th quarter. Hilltop National Bank now owns 131 shares of the company’s stock worth $30,000 after purchasing an additional 42 shares during the last quarter. Tanager Wealth Management LLP boosted its stake in MongoDB by 4.7% in the 3rd quarter. Tanager Wealth Management LLP now owns 957 shares of the company’s stock worth $259,000 after purchasing an additional 43 shares in the last quarter. Rakuten Securities Inc. grew its position in MongoDB by 16.5% during the 3rd quarter. Rakuten Securities Inc. now owns 332 shares of the company’s stock valued at $90,000 after purchasing an additional 47 shares during the last quarter. Finally, Prime Capital Investment Advisors LLC increased its stake in MongoDB by 5.2% during the 3rd quarter. Prime Capital Investment Advisors LLC now owns 1,190 shares of the company’s stock valued at $322,000 after purchasing an additional 59 shares in the last quarter. 89.29% of the stock is owned by institutional investors.
Wall Street Analysts Forecast Growth
Several research analysts have recently issued reports on the stock. Barclays dropped their target price on shares of MongoDB from $400.00 to $330.00 and set an “overweight” rating on the stock in a research note on Friday, January 10th. Morgan Stanley increased their target price on MongoDB from $340.00 to $350.00 and gave the company an “overweight” rating in a research note on Tuesday, December 10th. Cantor Fitzgerald assumed coverage on MongoDB in a report on Friday, January 17th. They issued an “overweight” rating and a $344.00 target price for the company. KeyCorp upped their price target on MongoDB from $330.00 to $375.00 and gave the company an “overweight” rating in a report on Thursday, December 5th. Finally, JMP Securities reiterated a “market outperform” rating and issued a $380.00 price objective on shares of MongoDB in a report on Wednesday, December 11th. Two equities research analysts have rated the stock with a sell rating, four have assigned a hold rating, twenty-three have issued a buy rating and two have assigned a strong buy rating to the stock. According to MarketBeat, the company currently has a consensus rating of “Moderate Buy” and a consensus target price of $361.00.
Get Our Latest Analysis on MDB
Insider Buying and Selling at MongoDB
In other MongoDB news, CAO Thomas Bull sold 169 shares of the firm’s stock in a transaction that occurred on Thursday, January 2nd. The stock was sold at an average price of $234.09, for a total transaction of $39,561.21. Following the completion of the transaction, the chief accounting officer now owns 14,899 shares in the company, valued at $3,487,706.91. This trade represents a 1.12 % decrease in their position. The transaction was disclosed in a filing with the SEC, which is available through this link. Also, Director Dwight A. Merriman sold 1,000 shares of the company’s stock in a transaction on Tuesday, January 21st. The shares were sold at an average price of $265.00, for a total transaction of $265,000.00. Following the sale, the director now directly owns 1,116,006 shares in the company, valued at approximately $295,741,590. The trade was a 0.09 % decrease in their position. The disclosure for this sale can be found here. Insiders sold a total of 34,156 shares of company stock valued at $9,220,473 in the last three months. 3.60% of the stock is currently owned by corporate insiders.
MongoDB Stock Performance
MDB opened at $264.04 on Thursday. The stock has a market cap of $19.66 billion, a price-to-earnings ratio of -96.71 and a beta of 1.25. The firm’s fifty day moving average is $274.45 and its 200 day moving average is $269.62. MongoDB, Inc. has a fifty-two week low of $212.74 and a fifty-two week high of $509.62.
MongoDB (NASDAQ:MDB – Get Free Report) last announced its quarterly earnings data on Monday, December 9th. The company reported $1.16 EPS for the quarter, beating the consensus estimate of $0.68 by $0.48. MongoDB had a negative return on equity of 12.22% and a negative net margin of 10.46%. The business had revenue of $529.40 million for the quarter, compared to the consensus estimate of $497.39 million. During the same period in the previous year, the business earned $0.96 earnings per share. The business’s revenue was up 22.3% on a year-over-year basis. On average, equities research analysts predict that MongoDB, Inc. will post -1.79 earnings per share for the current year.
MongoDB Profile
MongoDB, Inc, together with its subsidiaries, provides general purpose database platform worldwide. The company provides MongoDB Atlas, a hosted multi-cloud database-as-a-service solution; MongoDB Enterprise Advanced, a commercial database server for enterprise customers to run in the cloud, on-premises, or in a hybrid environment; and Community Server, a free-to-download version of its database, which includes the functionality that developers need to get started with MongoDB.
Featured Stories
This instant news alert was generated by narrative science technology and financial data from MarketBeat in order to provide readers with the fastest and most accurate reporting. This story was reviewed by MarketBeat’s editorial team prior to publication. Please send any questions or comments about this story to contact@marketbeat.com.
Before you consider MongoDB, you’ll want to hear this.
MarketBeat keeps track of Wall Street’s top-rated and best performing research analysts and the stocks they recommend to their clients on a daily basis. MarketBeat has identified the five stocks that top analysts are quietly whispering to their clients to buy now before the broader market catches on… and MongoDB wasn’t on the list.
While MongoDB currently has a “Moderate Buy” rating among analysts, top-rated analysts believe these five stocks are better buys.
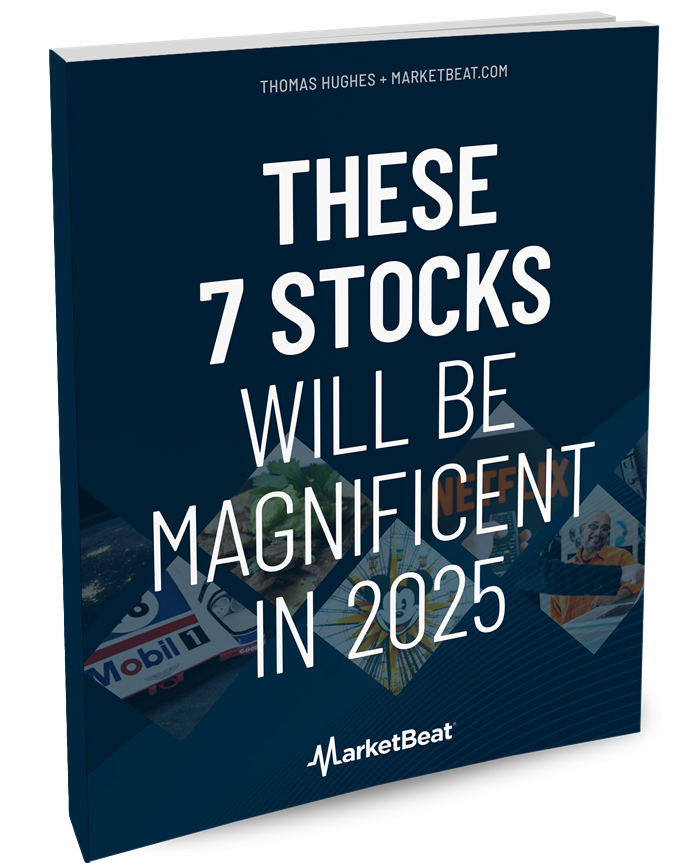
Discover the next wave of investment opportunities with our report, 7 Stocks That Will Be Magnificent in 2025. Explore companies poised to replicate the growth, innovation, and value creation of the tech giants dominating today’s markets.
Article originally posted on mongodb google news. Visit mongodb google news
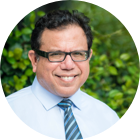
MMS • Cody Yu
Article originally posted on InfoQ. Visit InfoQ
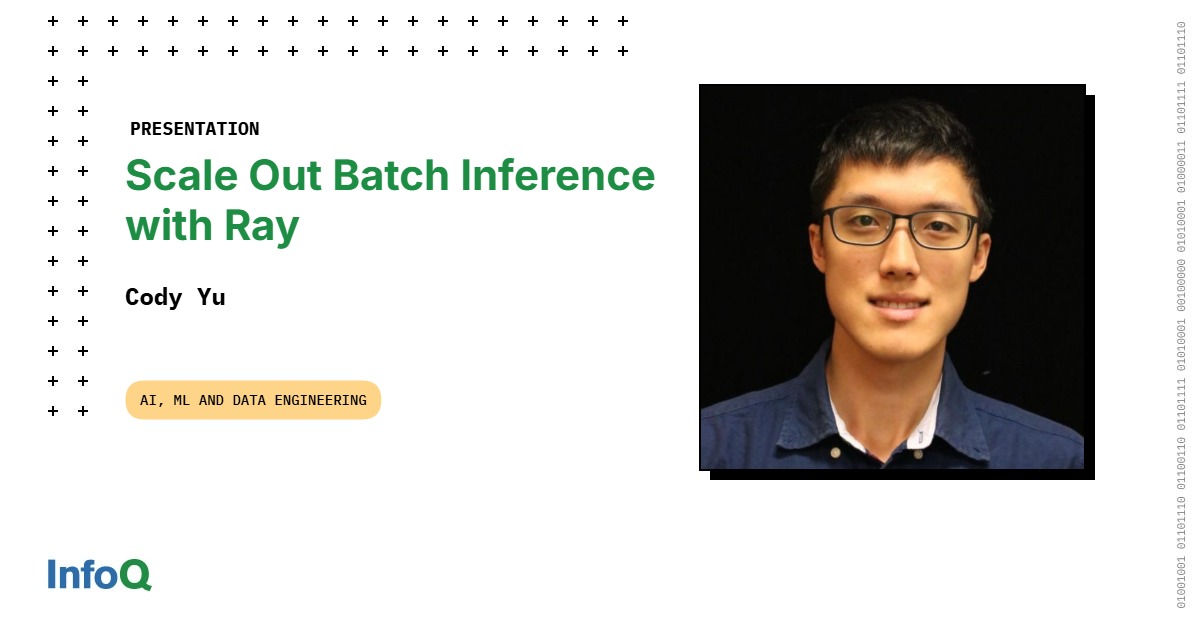
Transcript
Yu: I’m Cody. I’m a staff software engineer at Anyscale. I’m going to talk about how to scale out batch inference with Ray. I’m a staff software engineer and a tech lead for large language model performance at Anyscale. I’m also a vLLM, SGLang, and Apache TVM, which are our famous open-source projects committers. Before that, I was also the expert engineer at Boson AI. Also, a senior applied scientist at AWS AI.
The GenAI Era
Before we dive into the batch inference for large language models with Ray, I want to first start with the statement that we are in the GenAI era. For example, you can see today a lot of people are talking to the chatbots, and some companies have already used a chatbot or large language model for their online customer services.
A more complicated situation or use case, could be using the large language model as the multi-agent to process a complicated task. We all know that the model today can actually generate not only the text, but also images, or even videos. Even the images from the slides were generated by OpenAI GPT-4. This illustrates how useful and important the applications are in this GenAI era.
Batch Inference
At the same time, the demand of the batch inference is also getting higher. This is mainly because we now have multi-modality data sources. You have cameras, mic, and sensors, and PDF files. Then, by processing these files, you will get different kind of raw data in different kinds of formats, which is unstructured or structured. For example, you can have the images extracted from the camera or sensor. You can have the audio, tabular data, or video, or even the raw text.
In order to make that data pretty useful for your real application and services in production, you will need either the embedding models or the large language models to help process this data. Then you can save the results back to the vector database, use for model training, classification, or extract the knowledge from that data for the various applications. In short, while a large language model becomes high on demand, the demand of the batch inference will go even higher. That’s why we want to dive into the batch inference and make it more cost effective and high throughput. Now scaling out batch inference actually will bring up certain challenges.
For example, the first thing you want to deal with is the scalability, because you can imagine those raw data can easily go up with hundreds of thousands of gigabytes or terabytes, or even more. Scalability becomes the biggest challenge. Come with high scalability, you have the reliability issue, because in order to save money, usually we will prefer to use the Spot Instances on the public cloud providers for cost saving. The Spot Instances will actually offer you the instances with, for example, just 10% of the original price, but it can be preempted anytime by the cloud provider in high peak hours.
In order to build a system on Spot Instances, we have to have a good reliability, that means you can deal with the failures due to the preemption, and you’ll be able to resume the failure jobs without any human in the loop. Of course, computing is one of the most important factors, because different stages in your data pipeline may require different hardware, which can be CPUs or GPUs, or different kinds of GPUs. Dealing with multiple stages in your data pipeline and heterogeneous computing also becomes a big challenge. Also, the flexibility, because you will need different models to process different data.
For simple models, you may need just simple object detection model. For complicated data, you may need a large language model to analyze the semantic or extract information, so the system, the batch inference pipeline also needs to deal with the flexibility of dealing with different OS models, or the custom models trained by yourself. Finally, SLAs. When you’re doing batch inference processing in production, you cannot just throw out the data and get a result without considering the cost. Either you will set the high throughput or cost or latency as your SLA before you get into the production to make sure you won’t bankrupt by processing those kinds of data.
Multi-Layer Approach
At Anyscale, we solve this problem by introducing a multi-layer approach. We basically have a three-layer approach. From the bottom up, we have the Ray Core which is a scalable general-purpose AI computing engine to deal with the scalability and reliability.
On top of that, we build Ray Data, which is an efficient and scalable data processing pipeline on Ray. Because Ray Core already deals with the scalability and reliability, so we can build Ray Data on top of that easily. I will show how we build it and how do we implement that later on. On top of that, we need a powerful large language model inference engine. We use the open-source vLLM, which is the most popular open-source large language model inference engine at the moment, and based on that, to construct our batch inference pipeline.
Ray – Scalable AI Compute Engine
Let’s start with Ray. Here’s a Ray overview. Ray is basically a distributed library to deal with large scale cluster and resource management and task allocation. On top of Ray, we already have a very plentiful ecosystem which are the application-level libraries built on top of Ray. For example, we have Ray Tune and Ray Train to deal with the training jobs. We have Ray Serve, to deal with the online model serving. RLlib, which is a reinforcement learning library built on Ray.
Also, the Ray Data, which I will be introducing very soon, is for data processing. All of those applications are implemented for different functionalities, but a common part of them is to deal with large scalability. At the bottom we have the remote functions and classes, we call those tasks and actors. Then the Ray Core, which is a general-purpose distributed execution layer to deal with all the task allocation scheduling and actor manipulations.
For example, for now, Anyscale has been using Ray to push the scalability to thousands of instances for running our workloads for our customers. In short, you can see we have the head nodes and the worker nodes. The worker node can be 1000. In each node, we have the driver and the worker. On the line, we have two important components, which is GCS and a dashboard server. For GCS, this is used for actor scheduling, placement group scheduling, node resource views. For dashboard server, this basically shows the dashboard metrics, system logs, job APIs.
We highlight those two components because we realize that when you’re scaling up to thousands of nodes, scalability, reliability are the important factors, but other than that, how do you make sure all the metrics and logs and the current status can be easily monitored and interpreted by users, are also becoming important factors. That’s why, in the recent development of Ray, we also focus on those GCS and dashboard server developments. We want to highlight that those two components have actually become much more robust on actual large clusters, and this is basically because we have optimized the event loop very carefully.
For example, we have the dedicated threads for high value and simple RPCs, and you also move expensive data fetching to the background threads, and break up expensive handlers. I won’t dive into too much details for the Ray Core, but if you’re interested in the details of Ray, welcome to check out the Anyscale documentation, or contact us for more details.
Ray Data – Scalable Data Preprocessing for Distributed ML
Once we have Ray to deal with the scalability, next we can build Ray Data, which could be a scalable data processing for distributed machine learning processing. Let’s start with the key challenges that Ray Data has solved. First is the heterogeneous computing. Like I mentioned in the beginning, in the different stages of your data pipeline, you may need different hardware in terms of CPUs and GPUs. In Ray Data, we enable streaming execution. That means we basically chunk the data into multiple blocks, and then we can process all the blocks in a streaming way.
In this way, we can make sure the resource on each stage can be maximized all the time. In this example, you can see we load and preprocess data on CPU and do the inference on GPU. We want to minimize the GPU idle time, because the GPU cost is usually like 4x of the CPU cost, or even more. The second challenge is, like I mentioned, reliability is an issue if we want to build a data pipeline on very cheap Spot Instances. Ray Data along with Ray Core, also make sure the reliability is there.
Specifically, we have the fault tolerance and checkpointing to make sure all the jobs can be done with the correct functionality and statements. Also, we have the job resumability so that whatever the instances are preempted by the cloud providers, we can make sure all the jobs will get executed only once. The third challenge is to deal with a complicated ecosystem. Now you have a thousand ways to process your data using various of the existing and mature systems, like HDFS, S3, those are the objective systems.
Also, obviously, Spark, Dask, those are the computing frameworks, and that’s for the data part. Then for machine learning and AI, of course, people usually use TensorFlow or PyTorch to do the inference for your model. Ray Data has already integrated all of those popular user frameworks, so that you can easily use Ray Data to integrate all of those components together to build your scalable data pipeline.
Let me dive into Ray Data implementation. First is the Ray Data Engine. In the example on the slides, we show a Ray Data batch inference example. In this example, we first read the image from S3 buckets and apply the custom transform function to process the data in any way you like. After that, we will send the processed data to inference. This inference is a class, and it will be hosting a model on GPUs. After data has been processed, we’ll basically upload the result back to the S3, and all this happens in a streaming way.
By writing this simple code, you already construct a highly reliable, highly scalable data pipeline using Ray Data. What’s happening under the hood is we will first construct a logical plan. This basically designs what to do with the data pipeline. You can see by this code snippet, we define the four stages in the CI pipeline: first read the data, transform data on CPU, perform inference on GPU, and then upload the result on CPU. However, you can imagine, although this data pipeline looks pretty simple and straightforward, it could be inefficient during the execution.
After this, Ray Data will actually compile this logical plan to generate the physical plan. The physical plan basically defines how to do with your data pipeline. In this simple example, we can see the read and transform are actually fused to a single MapOperator. That means we can save the data communication, data rewrite, Disk I/O by streaming the read and transform together.
Once we have the logical plan, during the actual execution, the data actually will be processed by Ray Tasks or Actors with different resource requirements. Like we mentioned, we can actually specify how many CPU cores you need to process the input data or how many GPUs you need to inference the data. By specifying different requests, what’s happening under the hood is Ray Data will do the scheduling for different tasks on different hardware in a dynamic way.
In this example, we have three nodes, two CPU nodes and one GPU node. Even for the GPU nodes, we have the CPU cores. Ray Data will basically just schedule the Ray Tasks on the CPU or GPU, based on the requirement. Of course, we’ll try to minimize the data movement by scheduling similar data to similar nodes. Like I mentioned, it’s important to have the streaming executor, so we’ll schedule tasks dynamically and manage the resources and handle the back-pressure. All of this is to ensure the GPU is consistently saturated. This becomes very important metrics when we’re evaluating the efficiency of the data pipeline with GPUs.
Talking about streaming execution, I do want to highlight one thing that plays an important role in efficiency, is about how do you communicate data between tasks and nodes? As you can imagine, because we can probably schedule the tasks on different stages, on different nodes, you will need an efficient way to communicate the data between nodes. We introduced an abstraction called the Ray Object Store, which is basically a storage or like a file system, so that the MapOperator can basically upload your temporary result to this object storage, and the next stage can consume that.
In order to achieve the best efficiency, this abstraction can be implemented in different ways. For example, between nodes and nodes, you can use share file system or AWS S3 object storage. With the same node, this can even be in, for example, the shared memory or local disk I/O. By optimizing and elegantly selecting the most suitable file system under the hood, we can optimize the data transfer between tasks.
Here is a case study using Ray Data. This example is the batch embedding generation. In this simple pipeline, we first load PDFs on CPU, and extract and clean text on CPU. Suppose this is streaming easy, in this example, the most difficult part is to extract embeddings for those text using the sentence transformer model. After this pipeline, we will have embedding factors for each PDF file, and this embedding can be used for data retrieval or the PDF search.
In order to generate such embeddings, we use Ray Data with 20 GPUs with 100% GPU utilization. These 2K PDFs, which include 30k pages, and we would like to generate 140k encodings, we use 20 GPUs, and this will take about just 4 minutes runtime to finish all the processing. By the trace on the right-hand side, we can see, once the task kicks off, all the GPUs are basically saturated all the time for the entire 4 minutes.
By doing so, you only need to pay less than $1 to finish the 2K PDF processing. Of course, we have some more examples which are available on the Anyscale blog post, feel free to check out. We do have more example case studies with much higher scalability. I do encourage you to check out and see other use cases.
Ray Data + LLM
I want to dive into the large language model part. The previous example I show, you can use Ray Data to construct a pipeline and basically leverage the machine learning model to process the PDF file. However, if we get into the large language model, you’ll bring up more challenges. First of all, what’s the motivation? Because we know that large language models are actually much more powerful to process the various tasks, you can let it do the summarization, tagging, or sentiment analysis, keyword extraction, or unstructured data processing. You can do much more things than the traditional machine learning models.
However, it also brings challenges. The most important challenge it brings up is large, because it’s called large language model, so it’s basically too large to achieve the high throughput in a very naive way. Here is one possibility of your data pipeline with large language model. Now in the data preprocessing stage, you are now just doing the data processing in the way you like. You also need to tokenize your input data. You probably also need to encode your image if your data has an image input. This is because a large language model doesn’t really take the text and image directly as the input.
Instead, it takes tokens. Tokens are basically integer representations of the inputs. For example, an English word can be partitioned to one or multiple tokens. The large language model will solve the tokens and do the mathematic computation, like matrix multiplication and so on, to calculate the features and hidden states, and then determine the response. You have to do the tokenization to transform the text and images to the tokens and send it to the large language model.
After we got the response, the response is also in the token format. You have to do detokenization to convert those tokens back to the text. We can now see that we have a more complicated pipeline, and also this large language model, often, has to be executed on multiple GPUs instead of one. Those are the challenges we need to deal with.
vLLM + Ray Data – RayLLM-Batch
In summary, by the end of this talk, I want to introduce RayLLM-Batch. This is the solution we have by combining vLLM and Ray Data, to achieve the scalable and batch processing. Before that, I want to introduce vLLM. vLLM itself is the most popular large language model inference framework. It’s fully open source and it’s also part of the Linux Foundation. It now has more than 30k stars on GitHub, and we merge more than 200 PRs every month.
There are notable contributors, including Anyscale, AI21, Alibaba, AWS. You can see a lot of companies are actively contributing to vLLM to make it better and have more features for the large language model inference. There are also a lot of adopters that use vLLM in their product, including some open-source projects and companies.
I want to talk a bit about what’s the key features we used in vLLM and what we have to already contribute. Before that, I want to take a step back to briefly introduce how large language models do inference. Different from the traditional machine learning model that you simply send the input and do the model forwarding and get output, large language model uses so-called autoregressive decoding to do the inference.
This is basically because if you just run the model once, you will just generate one token. Like I mentioned, if you want X model to respond with text or the paragraph, you will need tons of tokens, and those tokens have to be generated one by one in an iterative fashion. That’s why we call that autoregressive, because, for example, we can see that you have the prompt, and then do the tokenization, it will be converted to the number of tokens.
Then doing the one forward, we just predict one next token and one next word. Then we will send this back to the model. This is called autoregressive. You will send the output, become the input of the next iteration and generate the next token. You have to do that iteratively to generate the complete response. You can see this is actually a very long latency process. If you use the ChatGPT or other chatbot providers, you can see the response is always output in a streaming way. That’s not only just because of the UX perspective, it’s because the model generates the output exactly in that way.
Now, from the production or the vendor point of view, we want to maximize the throughput in the server side to reduce the cost. We cannot just generate one response by fully utilizing the GPU resource at a time, we have to batch all the requests and generate requests together to minimize our cost and maximize the system throughput. One important technology being introduced is called continuous batching. Basically, we want to batch the requests coming from the users and decode them together. In this example, we have the first request coming in, and we first do the prefill to generate the KV-cache for the input prompt.
After that, we get into the decode stage to generate the response token one by one. Let’s say in this time period you got the second request coming in, because we first have the prefill-first policy, so we will pause the decode process and first do the prefill for the second request. After that, we will let this second request join the decode group to batch decode together. We can see, at this moment, our batch size becomes 2 because we’re decoding two requests together.
This is called continuous batching. We have two more requests coming in, so we pause the decode process again and do this prefill, and then let all the requests join together and decode together. This is the normal batch processing with continuous batching. We can see an obvious challenge for this algorithm to do the continuous batching.
First, you can see that the decode process has to be interrupted when the new request comes in. From the user point of view, you may see the model is generating some text and then pause for a while and then continue generating that. This is because of this behavior. The second part is you can see, if there is a long prompt, let’s say the request 3 has the input token 1.5k, this will slow down the entire batching of the request. You can see the latency between this token and this token is actually much longer than this token and this token.
In order to solve this problem, an important technology, or the feature that’s already introduced and integrated into the vLLM is called a chunked prefill. The basic idea is we want to split the long prompts to multiple chunks, and we want to batch chunked prompts and decode tokens together.
Again, in this example, when a new request comes in, instead of pausing the current decode, we actually let the current decode join the prefill batch, so we can do the prefill and decode together in the same batch. Let’s continue doing that. Now that request 3 is coming in, again, we don’t have to pause the decoding process of the existing two requests. We can directly introduce a new request coming in, and then we do the chunked prefill for the request 3, followed by request 4, and now we have all the decoding process together.
This becomes an ideal case that we can balance the batch sizes between all the time frames, and then we also make sure the decode process won’t be interrupted. This feature is basically contributed by Amey, he is the chunked prefill author and PhD student, and also SangBin from Anyscale. Here is an experimental result for enabling chunked prefill in your model serving, you can see that we basically have 1.4x ITL increment. ITL stands for inter-token latency.
From the user point of view, you will see the request coming out much faster than before. We’re also suffering from 30% TTFT slowdown. TTFT stands for time to the first token. This is easy to imagine, because now for the long prompts, you need multiple batches to process them all. This is being sacrificed by this technology. Because we have the much higher ITL improvement, the overall end-to-end latency is also reduced, so the overall system throughput is also improved by enabling chunked prefill.
The next feature I want to introduce is the prefix caching. This is basically very straightforward. We want to reuse the KV-cache from another request with the same batch so that we don’t have to compute the same hidden state for the same tokens again and again. There are two obvious examples that prefix caching can help a lot. The first one is called shared system prompt. You can imagine, if you’re hosting a service, a chatbot or something like that, you probably have the system instructions across all the requests.
From, for example, the OpenAI point of view, they will have a very long system instruction to tell the model you are used by an AI assistant, your response should follow this rule 1, 2, 3, and those are consistent across all the requests. We want to share that and avoid the computation of those instruction prompts. The second example is a multi-turn conversation. You can imagine when you are talking to the chatbot, when you respond to the model with a new question, the previous chatting history is actually reused from your previous round, so this is also a shared prefix. Because of that, we want to use prefix caching.
This in the vLLM, we have the hash based automatic prefix caching. The idea is pretty straightforward. We basically chunk your prompts into multiple blocks, and then we use the token ID as the hash key. When the new request comes in, we will use the token ID to see if we have already computed the blocks in the system. If so, we just directly reuse that block without recomputing that. This feature is basically led by Zhuohan from Berkeley and me from Anyscale. Another one is called speculative decoding.
Basically, the idea is also very straightforward. When you’re serving a large model, like 70B model, the latency is really pretty high. You may imagine that sometimes the model will respond with a very simple output, and in this case, using a small model can maybe fulfill your requirement already.
Speculative decoding is basically a case that we deploy both a small model and large model at the same time, and when the request is coming in, we use a small model to generate the speculative tokens and let the large model to verify that, because the verification cost is much lower than generating the token. If this small model can guess the token in a precise way, we can significantly reduce the latency for generating the response. This is also an important feature contributed by Cade and Lily from Anyscale.
The last feature I want to introduce is the pipeline parallelism. Like I mentioned in the beginning, the large language model may not be fitting into the single GPU due to the number of its parameters, so usually we will need more than one GPU to host one single replica for a model. That means you have to parallelize the model execution in some ways. There are two famous model parallelism strategies: you can do Tensor parallelism or pipeline parallelism. Tensor parallelism is a straightforward approach.
Basically, you just parallelize the execution of the matrix multiplication, and then aggregate the results after each layer. This implementation is extremely simple, but it also introduces very high communication overheads. It is good for improving the latency on a single node, or if your GPUs have a very high bandwidth inter-GPU communication, such as NVLink. On the other hand, the pipeline parallelism is a low communication overhead solution that basically partitions different decoding layers to different GPUs and pipeline the execution together. This is very good for throughput, because you can saturate all the GPUs on different pipeline stages.
This is often very useful for offline batching on the commodity GPUs which don’t have NVLink, so the inter-GPU communication has to go through the PCIe. We are already actively using pipeline parallelism in the vLLM for the batch inference. This feature was contributed by Murali from CentML, and SangBin from Anyscale. Here is an example of the pipeline parallelism. One thing I do want to highlight is, although pipeline parallelism is an intuitive solution to achieve high throughput for the batch inference, it’s not straightforward to achieve the best throughput out of the box.
For example, in this case, we show that we use 8 L4 GPUs to serve a single Llama 3.1-70B model. We can see TP8 stands for Tensor parallelism with eight GPUs, and PP8 stands for pipeline parallelism on eight GPUs. We can see that although pipeline parallelism should give us a better throughput, the throughput shown here is actually worse than Tensor parallelism. This is because we don’t really optimize the pipeline efficiency in this setting. Why?
First of all, we have to unbalance the pipeline stage execution time, because in the large language model, the basic architecture you can imagine is the repeated decoding layers, but actually in the beginning and the end of your model, you have the embedding layer. The first embedding layer will convert the tokens to the embedding factors, and the last layer will also convert hidden states back to the embedding factors for your vocabularies.
That means the first stage and the second stage in your pipeline must have higher execution time than other stages, if you partition the decoding layers evenly. We have to consider these factors when we partition the decoding layers. Specifically in this evaluation, we just allocate less decoding layers in the first and last stages to balance the execution time of all the pipeline stages. You can see by doing so, we have already achieved a similar throughput as the Tensor parallelism, but the same performance is not good either, because we expect a much higher throughput.
Another bottleneck is the unbalanced batch sizes between pipeline stages. Imagine you have the pipeline with eight stages in this example. In the first timestamp, you’re processing the prefill with 2K tokens, followed by a decode with just 10 tokens. Although the batch with 10 tokens can be processed much faster, there is a prefill in front of you to block your execution. This imbalance will create the pipeline bubbles and reduce the pipeline efficiency.
Our solution is to enable the chunked prefill, so we can make sure every batch has a similar number of tokens, so the execution time of this batch can be balanced across all the pipeline stages. You can see the result is pretty amazing. We basically get almost 2x throughput increment by simply enabling the chunked prefill. This is not the end. We actually can achieve much higher throughput by tweaking another configuration.
In this example, if we change the configuration to use two GPUs to do the Tensor parallelism and use four GPUs to do the pipeline parallelism, we can actually achieve a much higher throughput. This is because if we use the pipeline stage every four pipeline stages, we can actually balance the execution time in a better way given the number of decoding layers in this 70B model. You can imagine this configuration tuning is basically case by case, and there is no optimal solution across all the workloads and models.
RayLLM-Batch – Ray Data + vLLM
Finally, let me get into my final goal to combine vLLM, this is a powerful LLM inference engine, with Ray Data to construct a highly scalable data batch inference pipeline. RayLLM-Batch is an Anyscale library built for large scale, cost-optimized batch inference. The key features are, you can bring any open-source model, and it’s dealing with the fault tolerance. You can use the Spot Instances at scale to save money. We also have the optimizer for the custom workloads. There is a simple code snippet that’s being used by our customers.
Firstly, you just need to define your workload, for example, how you read the data, how you want to process the data. Then you just need to specify how many GPUs you have, and you want to use that for the batch inference. Then the rest of the thing will deal with RayLLM-Batch.
Other than that, we have the inference engine optimizer. Like I mentioned in the pipeline parallelism example, you have to optimize the engine configuration in terms of number of GPUs, number of pipeline stages, or even the batch sizes, whether you enable chunked prefill or something like that. This is actually pretty complicated. In the right-hand side, there’s a very scary usage example dumped from the vLLM open source. There are basically thousands of different configurations you can play with to achieve the optimal performance. This is actually pretty painful.
In Anyscale, we have the inference engine optimizer, which is pretty straightforward. We have the autotuner to explore those possible configurations, and then evaluate those configurations on all GPU clusters. Because of Ray which is already very scalable and reliable, we simply build this optimizer on top of Ray so that we can tune a lot of configurations in a very short period of time.
Case Studies
Finally, is a case study. We use the synthetic dataset with 320 million tokens, and then we enable chunked prefill, prefix caching, and pipeline parallelism like I introduced before. From this result, we can see the engine optimizer can help reduce the total processing time by up to 34% as shown in the right-hand side.
Also, the prefix caching can help reduce the total process time by up to 64% with the 80% shared prefix. We can dive into this figure, in the left-hand side, we have the Llama 3.1-8B on L40S GPUs, and the right-hand side is the 70B. We can see that by enabling the engine optimizer and the prefix caching, we can reduce the cost by almost an order of magnitude. Then this also, again illustrates that different workloads and different models will require different optimizations.
Takeaways
We mentioned that the large-scale batch inference actually becomes a high demand workload in the AI era, and Ray Data can actually push the scalability of the batch inference to thousands of nodes. Large language models actually make batch inference even more practical. vLLM has enabled most features for high throughput LLM inference, and it works out of the box. If you combine vLLM with Ray Data to become RayLLM-Batch, that will be an ideal solution for large scale LLM batch inference.
Questions and Answers
Participant 1: Are there any examples of what you’ve used RayLLM-Batch to build, for example?
Yu: Yes, we do have customer examples. Some examples I can probably share, high level, is we have a customer that uses RayLLM-Batch to process their customer data, and generating a possible next action item. Like, how do we deal with this customer? Where we should give them the discount, or we should greet them more frequently, or something like that. They process millions of customer data using RayLLM-Batch.
Another example is probably, there’s our customer, they have the customer purchase history, there’s like grocery customers. They have the customer purchase history. They want to have some insight from the processing history. They threw the purchase history with some system prompt and asked the model to analyze those purchase history and do the recommendation and probably just get a sense about what items are getting popular across all the customers. Those are the obvious examples that actively use RayLLM-Batch, at the moment. We believe there are a lot more applications, and we’re looking forward to see what else people can do.
Participant 2: With vLLM, you went over several different inference optimization techniques that are being used. Which one do you think might contribute more towards inference optimization?
Yu: In order to answer this question, you have to make a lot of assumptions about your workloads and models. For example, if your model is 8 billion parameters, it can be usually fit into a single GPU. The most important feature could be chunked prefill and prefix caching, and probably floating points, FP8 execution and quantization. If you want to serve a much larger model, like 70 billion, or even 4 billion or 5 billion from Llama 3, then you only need multiple GPUs.
In this case, the most important feature could be the pipeline parallelism. It really depends on your use case and workload. For example, if your workload doesn’t have any prefix sharing, every of your texts are having different prompts, or even the prompt is actually pretty short, then prefix caching may not be the one you want. On the other hand, if you have 80% of the shared prompts, then prefix caching plays the most important role.
Participant 3: The tuner you are using, is it more of a grid search, or do you use some size of activation, size of weight, and do just educated guess first, and they start from there.
Yu: For our tuner, the style is configured in a flexible way. We would use different search algorithms based on real use cases. For example, for certain cases that we already manually shrink the tuning space to a very small size, like 10 or 20 configurations, in this case, we’ll just use the grid search or exhaustive grid search.
For more broad use cases, which we may have thousands of configuration points, in this case, we’re experimenting with different search algorithms. Currently in our radar could be the Bayesian optimization or the tree-based structured search, or even the reinforcement learning based search algorithms. There are a lot of tuning libraries off-the-shelf, so we’re basically just using that. We’re not inventing the new search algorithm, but basically just see which algorithm is the most suitable one for our use cases. We want to make that more efficient.
Participant 3: On the same line, for a model that either fits in a GPU or two GPUs, is there any point on going more in terms of Tensor parallel, because it’s just going to add the overhead. Unless you’re looking for lower latency, is there any point on going on the Tensor parallel route? Let’s say my model fits in two GPUs, and I’m happy with latency, is there any point in going Tensor parallel on four GPUs?
Yu: This is very correct. Tensor parallelism has two benefits. One is, it should lower the latency, given that you parallelize the matrix multiplication on two GPUs. The second is, basically you balance the workloads, because, like I mentioned, in order to make the pipeline parallelism efficient, you have to deal with the pipeline bubbles, and you want to make sure the execution time of each pipeline stage is balanced. For Tensor parallelism, we don’t have this problem, because you naturally have to balance the workload across all GPUs. That’s why we call that simple and easy to optimize.
Participant 3: On the chunked prefilling, what happens? Let’s say you have a continuous batching, max batch size is 128, and then after some time, let’s say 16 of my prompts are done. They have reached the stopping condition, but the rest of the prompts are still active. Does the batch have to waste computation for those 16 queries that are already done.
Yu: This is naturally handled by continuous batching with or without the chunked prefill. We can see that in each timestamp, we actually reconstruct a batch on the fly. If, for example, the request 1 is down in a certain time, it will just be kicked out to dispatch, and then directly return to the user. It doesn’t have to be staying here until all the requests are done. This is the flexibility of the continuous batching.
Participant 1: For companies or organizations that are getting started with this, you mentioned some overhead tradeoffs. Is it easy for, let’s say like a startup, to get started? What are the main maybe challenges if they’re doing that?
Yu: Based on all the customers we have been dealing with and the people we are collaborating with in the open-source community, it’s actually straightforward if you want to start with open-source solutions and tweak that by yourself to face those tradeoffs. Usually, things get more challenging when you’re scaling out with hundreds of thousands of nodes.
Usually our experience, we see from our customers, is they have the prototyping with a small scale, like five nodes or so. Once they want to get into a higher scalability, they probably just seek for help from us. That’s the way they’re dealing with that. Surely, tweaking the performance for the small-scale problems could be straightforward if you spend time on it.
Participant 4: I’d be interested to hear the challenges you have with this prefix batching. Does it lead to hallucinations? Have you seen accuracy problems once you start batching or caching the prefix?
Yu: In terms of the output, the prefix caching will guarantee exactly the same output as before, because we just avoid recomputation. We’re not using approximation or anything like that. As long as you prefix cache in the right way, the output will guarantee to be the same. Of course, there are some more advanced technologies, like approximate prefix cache. I only have a slight difference compared to the previous prompt, can I directly use his result? In this way, you may still get some reasonable response, but the accuracy may be changed.
Participant 4: Because I know proximity matters, and it’s long tail as it goes off, it matters less. Is it automatically determined, or are you manually defining that?
Yu: In the very end, we have automatic prefix caching. We chunk the prompts in several blocks, and then basically we just see how many blocks you can match from the beginning. If you can match 50% of your blocks, then you have the 50% computing reduction. If you can only match like 30% you can save only 30%. It depends on two things. One is whether your prompt is actually shared with the previous request, and the second whether the compute results is still in the cache. It may be evicted if you have so many requests with different prompts.
See more presentations with transcripts
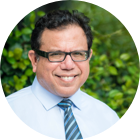
MMS • John Heintz
Article originally posted on InfoQ. Visit InfoQ
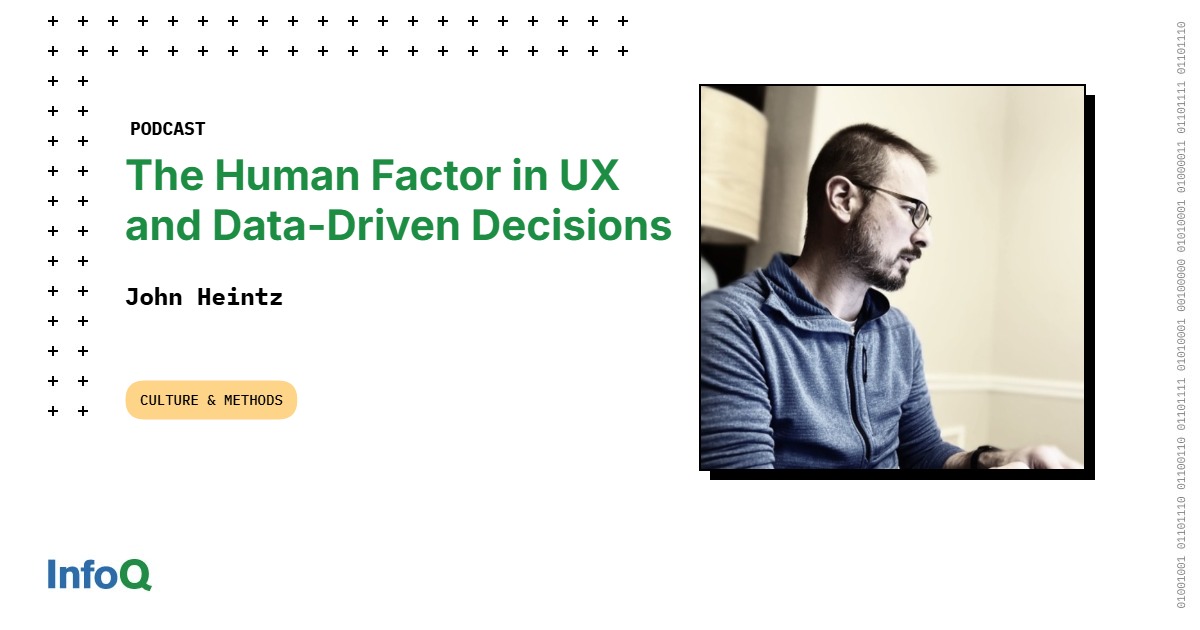
Transcript
Shane Hastie: Good day, folks. This is Shane Hastie for the InfoQ Engineering Culture podcast. Today I’m sitting down with John Heintz. John, thanks for taking the time to talk to us today.
John Heintz: Thank you, Shane. I appreciate the invitation. It’s great to be here.
Shane Hastie: My normal starting point is who’s John?
Introductions [00:49]
John Heintz: John is a technologist that learned how to do a lot of agile and test automation and DevOps, and eventually learned how to do more of the people side of things, understanding how to organize bigger systems, became a co-founder of a product company, an AI system. I’ve done some consulting in my career. I’ve done some technology leadership in my career. I have managed to co-found and sell one business.
Shane Hastie: Cool. What got us talking today was a talk that you gave talking about how does this data make me feel? Why should I care how the data makes me feel?
Why we should care about emotions when conveying data [01:33]
John Heintz: Well, that’s a great question. We all as humans need to care how data makes us feel so that we can make better decisions with the data. The way that I got to framing the topic of the presentation that you saw about was we were trying to create an AI forecasting system that was producing a lot of data, really interesting data, and as a technologist, and I was being mentored by my co-founder in some really interesting aspects of data science and data processing. I loved the data. It was all really cool.
However, the intended users of our system were not able to understand the data the same way we did. That began a journey in us not just creating really cool math and really cool algorithms, but in figuring out how do we make this data work for our end users and help them understand what they should do and make better decisions with it. That course took me through some psychology into UX and we learned some interesting lessons. The way that I ended up conveying that is, “How does this data make me feel”, is the important kind of phrase that we started with for how to deal with that system.
Shane Hastie: Let’s dig into some of those lessons. How should the data make me feel?
John Heintz: I suppose the simplest answer is that data that indicates good positive things should make us feel good, and data that indicates worrying, troublesome warning signs should make us feel more cautious or more nervous or not good, bad. The intuitive reaction that we have to data can help guide our responses to what we see. If we’re looking at a speedometer on a car and we look at the speedometer and the speedometer is way up really high, where the RPMs are way high into the red zone, that can give us a cause for concern. We might be worried about the health of the engine or the speed that we’re going. Data can make us feel good or bad, positive or negative. Leaning into that and accentuating that in our data systems is a very useful tool to help convey to our users, to ourselves what we’re looking at.
Shane Hastie: As a technologist, building user interfaces where I’m trying to communicate something to the end user, how do I make this useful for me?
The implications for interface design [03:57]
John Heintz: The first step is understanding what’s important to your users. That sounds really boring and really mundane in many senses, but it’s actually very, very important. Really, truly understanding what does good news and what does bad news look like for your customers means that you understand how to build a user interface that can convey that to them. The systems that we were designing for were showing project forecast and schedule forecasting. That’s not a super exciting user interface all the time, but if your project is in the green and you’re on schedule, that means very important good things.
If your project is in the red, it means important negative things that need the users to come in and take different actions, be able to make some corrective measures to get back on track or to communicate about the changes and the updates, and so understanding what do users need to know, and then framing the system to generate the information that they need to know at an intuitive level.
I can stop for just a second and say about the psychology that we were leaning into at that time was Thinking, Fast and Slow by Kahneman. Our brains have at least two different modes of thinking. One mode is fast thinking, where we’re doing intuitive natural reactions to things. If we think we’re going to step on something that looks like a stick or that looks like a snake, our brain will react very differently. If we think there’s a snake there, we’ll jump back real fast. Our brain is giving us a very strong intuitive impulse to back away from something that could be dangerous to us. Thinking slow is doing algebraic formulas by longhand on paper, that slow thinking, engaging all the faculties of the brain. What we realized is that we needed our user interfaces to communicate to the fast thinking part of the brain that would have a very quick intuitive natural reaction.
We wanted to give that fast thinking part of our user’s brains the right information, the right signal. If the system is indicating that there’s a risk to the project, then we want to give our users some visual indications that trigger that fast thinking so that they have a feeling about the data that is negative or worrisome and that it triggers them to take some actions. If the system is indicating and predicting that the system is on track and everything’s good, we want to give our users this fast thinking positive indication that everything is good. The feeling of the data is do I feel that the state is good or bad and do I need to feel positive and successful right now about it? Or do I need to take action and do something because I’m spurred to fix some problem? Those are some of the psychology that we were using was thinking fast and slow.
Shane Hastie: Is this as simple as red and green?
John Heintz: It can be that simple, but in our situation, and we’re on a podcast so you can’t even see me waving my hands around, but it would be as simple as red and green if we were talking about a very simple problem space, but we’re not. We’re talking about complex system visualization. If you imagine any kind of complex chart or interpretation system that you or your stakeholders or your customers need to look at and understand, often there’s time involved, like what’s happening over time this week, things might be in one state last week in a different state. There’s trending, are we trending in the right direction or re-trending in the wrong direction for things? Being able to convey things with temporal components and being able to convey a broad set of things all at the same time are much more complicated than just red or green, although yet it does boil down to that might be the simplest thing.
If there’s a red warning light, you should look under the hood. But what do you see when you look there at the next level of dashboard? In the situation that we were working in, we were building a burn-down chart that was a risk burn-down chart. The idea is at the beginning of a project, there’s some amount of unknowns and risks and uncertainty, and that’s very natural. That’s fine. When you’re building something new, there’s always uncertainties involved in it. But by the end of the project, as you get to be right before delivery or finishing this effort, the number of unknowns should be gone and everything else should be all good. Tomorrow we’re going to release this, we’re going to give this to our customers, and everything is fine.
Over time, that has to go down. How far below the risk burn-down line you are is one aspect of it. Or if you’re above and you’re in the red zone, how far above is also an important part of this. When you’re looking at a dashboard, you want to be able to have the dashboard give you an indication of positive and negative as well as how severely positive or how severely negative this is. Do we celebrate or do we jump into action right now would be the other aspect of it.
Shane Hastie: How do we bring in… Going beyond that thinking fast, thinking slow, how do we bring in elements of usability and accessibility in this?
Including usability and accessibility [09:15]
John Heintz: That’s a good question. The situation that we were in, we were three engineers. My co-founder had a PhD in math. Our lead engineer was a super seasoned software developer. I’d been a software developer for most of my career. We were the classic anti-pattern of engineers trying to build a user interface. We hilariously created some things that were not that great. We realized we were trying to go for these principles of thinking fast and slow. We knew we were trying to trigger the fast thinking part of the brain. We didn’t succeed at creating our own user interfaces to do that very well. What we did is actually reach out, get a good recommendation for a good UX group that was able to work with us. As a startup, we paid money for this. We really invested in this literally, both our time and some of our money.
We ended up working with that group to help build this interface with our users in mind. They did a great job of taking all of the things that we were trying to do and they distilled them down into a type of visualization that, in hindsight really was obvious. We could kick ourselves for not having thought of it without having an extra group, but we didn’t. Like all good innovations, everything in hindsight should seem pretty obvious and this one does, too, but I don’t regret it at all. We definitely needed their help. We brought in some UX experts for the piece of this puzzle that we were not able to figure out ourselves. We knew we hadn’t figured it out, because our end users, no matter how much we wrote or created documentation or talked with them and explained the system, that training just didn’t work.
They just didn’t get it. They didn’t like it. They didn’t appreciate what we were trying to show them. They liked what we interpreted and told them was true about the system and what was going on. They liked the information, but the presentation, the UX that we had built was definitely not up to par. After we worked with this group, the new version of the system was much more quickly adopted, training took less than an hour and it was all said and done.
Shane Hastie: What are some of the other psychological lessons that you’ve learned that as technologists we should think about?
Psychological nudges applied to software design [11:39]
John Heintz: Well, there was one more specific area of psychology that we did bring into this, and I think it’s applicable generally for all kinds of technologists. This is a book called Nudge by Thaler and Sunstein. The book is about human psychology. It’s often used in advertising and big organizations will often push user interfaces and different systems that will give humans the nudge to buy the products they’re recommending, the way that they’re recommending them. This applies for humans in general. All of us, when we’re shopping on Amazon or any other big site, we are being nudged all the time. The best buy, the recommended box, the most people in your network use this box, all of those are nudges. A nudge works by looking like the default most common option. Humans are wired so that we tend to look at those in our social group.
We look at what’s working for our neighbors and we generally assume that it’s a safe choice. Well, if we go back to our tribal heritage and wanderers and gatherers, this was very true. Everybody around you was surviving and they were doing things a certain way. They’d learned some things. We adopted and began to use that as a checkbox to say, “This is a safe way to make choices. This is a safe way to live. We can use it”. Our brain psychologically assumes that the default choice is a safe choice and we often will go with it. Well, this can be used for good or bad.
When advertisers are convincing us to buy stuff we don’t need, we don’t think that that’s necessarily a great thing for me or for society, but the fact is that’s how the psychology in our brains does work. What we were very aware of and what I would say all technologists should consider is all of the systems that we build that do any descriptive or predictive analysis are giving our users something at the top of the list as “This is probably the thing that is the most important, the most likely”, whatever it is that’s the most. We’re giving our users lists and the ordering of those lists is psychologically very important. Whatever we put at the top of the list and say, “This is the most likely answer”, will have a very strong psychological nudge that our users will go with it.
To come back to the system that we were building, we were building a project schedule and risk system that was identifying what was causing risks to delivering a project on schedule. The probabilities that we were assigning, things could be calculated as the odds or the number of days likely that this will cause an impact. What we wanted to do was very carefully give our users a recommendation engine to say, “If your project has gone into the red, here’s the top one, or two, or three things that are causing the most likelihood that this is the issue and the problem”. The important aspect of our system was to provide recommendations that had the highest chances of improving and making the system better.
Shane Hastie: Stepping away from the psychology of the design of the products and maybe thinking a little bit about the psychology of people and teams, a common challenge for technical leaders is how much currency do I need with the technology that I work with?
Staying current as a technical leader [15:25]
John Heintz: That’s a good question. I think that being able to understand the technologies that exist today and the trending, I’ve got a trick that I try to use to understand how much do I need to know about what’s going on today with any given technology, and whether that’s understanding the psychology with humans, whether that’s understanding a new technology for integrating distributed systems. What I try to do is I try to look at what was true in the earliest literature where things were initially talked about and published. The benefit of this is back when any given field is young, the number of publications is much smaller, and so you can at least understand how many there are and read some of them. As opposed to later, in any given life cycle of psychology or technology, the number of publications is just daunting.
What I will tend to do is I will look back at the early stages of any given field, understand what are some of the pivotal seminal publishings and topics that were true at the beginning of it, and then do a quick survey of what’s still true today and whatever’s consistent, whatever carried forward from the early days is likely super, super important forever is my assumption. What I try to do is I try to stay connected with the founding principles of whatever field it is, and then I try to pick something that’s still present today that connects to that and practice with that. That way I feel the most connected to the original ideas of something as well as to what’s current and relevant today. In my own work, my coding, I’m not full stack. I don’t code everywhere anymore, but my own coding now, I’m doing Python, I’m doing data and numerical analysis and Bayesian analysis and techniques.
I’m still building some predictive systems. I’m still coding, but I’m not trying to learn everything. I’m not trying to be everywhere at once. The other technique that I will adopt is I try to have really good friends and colleagues that are experts in these other areas. When a question comes up that I know that I don’t have a deep experience in, I will ask somebody a question and I’ve got a number of people in different areas where I can really trust their answer will put me on the right track much faster than I would be able to on my own.
Shane Hastie: We were chatting before we started about something that’s been around in our industry for a long, long time and it’s popping up, and popping up, and popping up all the time. Conway’s Law, and you have a story of an organization where you’re dealing with that.
Applying Conway’s Law in practice [18:09]
John Heintz: Yes. I find Conway’s Law is often talked about in a negative term as a warning, as a hammer that you’re going to get hit with. My own perception of Conway’s Law is it’s actually an opportunity. It gives us a chance to look at the design of our software systems, and it gives us an opportunity to look at the design of our human systems, our organization structures, and both of them are flexible in different ways. Conway’s Law basically says that both of those designs of human and software systems need to relate together in healthy effective ways. The number of times that these types of problems pop up, these types of issues where there’s a mismatch between the software and the organization is something that does just keep popping up in our industry again and again and again.
I think it’s a result of our human systems and the struggle we always have to try and design computer systems from human systems without always paying attention to both of them at the same time, equally. There’s a team and a group that I’m working with, they’ve got a distributed system, and one of the things that just became really relevant and obvious with them is that the design of their distributed system isn’t as clear, isn’t as coherent as they thought it was going to be at this point. The building blocks are really good.
The technology pieces, the way they do the event integration between the services, all really cool tech, all really good and effective, but the big picture is not quite as obviously present right now. That’s the piece that we’re all looking at and recognizing that some of the team organization structures have started creating extra services in different areas that were a surprise from the technological perspective.
It’s not that it’s wrong in any case, it’s just this is a really good opportunity to step back, understand the architecture of this distributed system and look at the design of the human system team structures and the communication patterns and rethink what should they both look like. Conway’s Law is the perfect framing for that because both of these systems have different shapes and structures, and there’s a couple of places where there’s obviously going to be a bit a mismatch and some scribble. The opportunity to use Conway’s Law as a way of thinking about both of these positively at the same time I think is a really wonderful thing.
Shane Hastie: At a practical level, how do we do that?
John Heintz: That’s a great question. Practically what I usually like to do is look at the nature of the system, look at the design of the products that we’re trying to create, and naturally what underlying software systems, components, and architectures should support those products as my first step in this. Then my second step, after understanding the vision of the systems that we want to create, then we pull back and look at the human organizations that are the right shape and structure and grouping to support creating those systems.
I start with, I practically, and this is still a little bit abstract, but I start with understanding the nature of the systems first and then working back to what would be an optimum human organization structure for those systems. Even more practically than that, every given organization will have structures, and those structures will never perfectly match the software systems. Humans are not as fungible or spin-upable as computer software is.
I think practically the other aspect to that is understanding wherever there’s a mismatch between the design of the software systems and the structure of the humans creating virtual teams and putting together, I like Team of Teams, that’s another book reference, but I like that reference because it does a much more effective job of horizontal communication structures.
The key takeaway from that is if you’ve got two different teams that do need to collaborate, one person from team A joins many of the team B meetings and status and communication structures. One person from team B joins many of the team A meetings as well. You have at least one individual from both sides being very aware and in tight communication with the other team. You don’t have one single massive team, you still have two separate teams, but what you’ve done is you’ve cross-pollinated to a very significant degree. I like that as a way of dealing with some of the natural frictions and structures that might occur when the team structures and the system structures don’t match exactly.
Shane Hastie: John, a lot of interesting stuff there. If people want to continue the conversation, where can they find you?
John Heintz: Well, my LinkedIn profile is a great place to find me, John Heintz, LinkedIn. I’m sure with that search you’ll be able to find me or I might be in a link somewhere below on this podcast.
Shane Hastie: You will, indeed. Thanks so much for taking the time to talk to us today.
John Heintz: Absolutely. Thank you, Shane. I appreciate the invite and it was great getting a chance to catch up.
Mentioned:
.
From this page you also have access to our recorded show notes. They all have clickable links that will take you directly to that part of the audio.
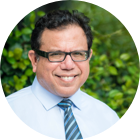
MMS • RSS
Posted on mongodb google news. Visit mongodb google news
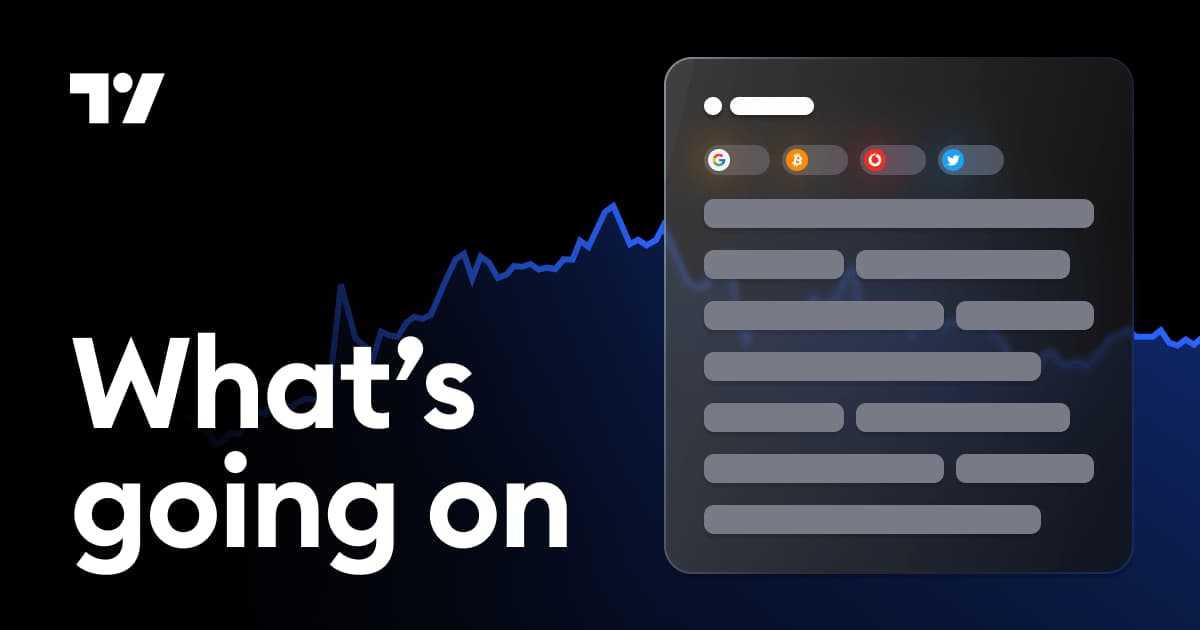
Reporter Name | Ittycheria Dev |
Relationship | President & CEO |
Type | Sell |
Amount | $2,333,757 |
SEC Filing | Form 4 |
MongoDB President & CEO, Dev Ittycheria, reported selling 8,335 shares of Class A Common Stock on January 28, 2025, under a Rule 10b5-1 trading plan. The transactions were executed at prices ranging from $264.97 to $286.09, with each transaction price being a weighted average. The total sale amount was $2,333,757. Following these transactions, Ittycheria directly owns 217,294 shares of MongoDB.
SEC Filing: MongoDB, Inc. [ MDB ] – Form 4 – Jan. 30, 2025
Article originally posted on mongodb google news. Visit mongodb google news
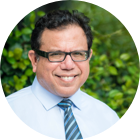
MMS • RSS
Posted on mongodb google news. Visit mongodb google news
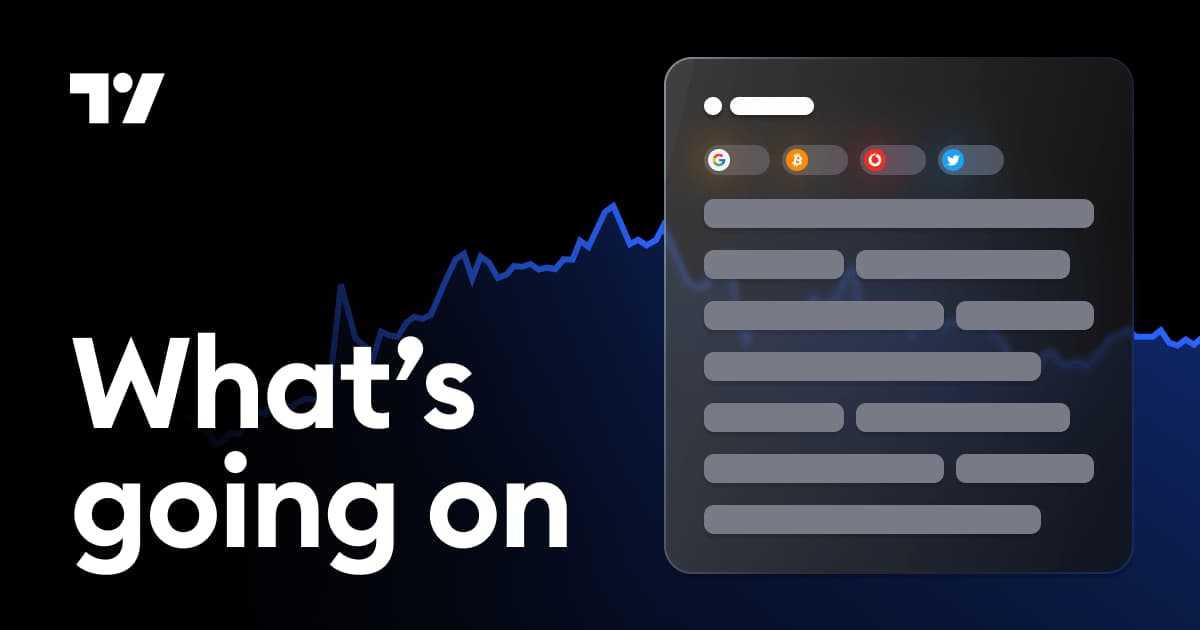
Reporter Name | Ittycheria Dev |
Relationship | President & CEO |
Type | Sell |
Amount | $2,333,757 |
SEC Filing | Form 4 |
MongoDB President & CEO, Dev Ittycheria, reported selling 8,335 shares of Class A Common Stock on January 28, 2025, under a Rule 10b5-1 trading plan. The transactions were executed at prices ranging from $264.97 to $286.09, with each transaction price being a weighted average. The total sale amount was $2,333,757. Following these transactions, Ittycheria directly owns 217,294 shares of MongoDB.
SEC Filing: MongoDB, Inc. [ MDB ] – Form 4 – Jan. 30, 2025
Article originally posted on mongodb google news. Visit mongodb google news
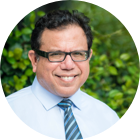
MMS • RSS
Posted on mongodb google news. Visit mongodb google news
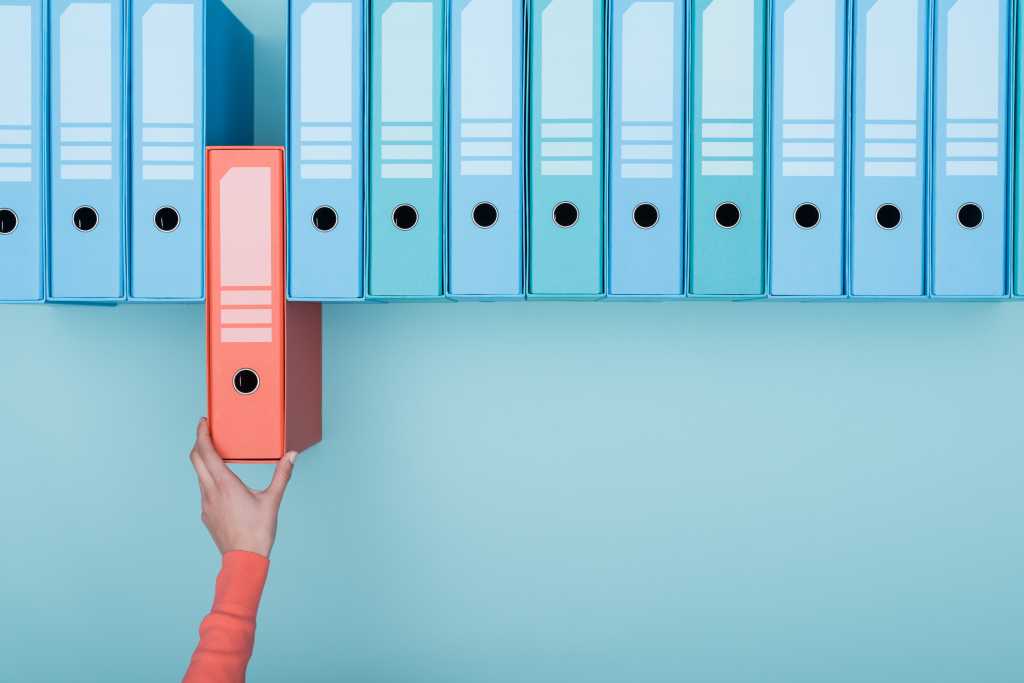
From the shell, you can embed API calls in select statements. This allows you to experiment with operations before adding them to calls from your code. The shell lets you build collections, add items, and experiment with CRUD operations. Other operations apply filters and support queries, as well as building indexes across one or more fields in a collection. You can find a lengthy list of documented API functions in the project wiki, grouped into common sets of operations.
For now, the GitHub wiki is the main source of documentation for DocumentDB. It’s a little on the thin side and could do with more examples. However, DocumentDB is currently intended for developers who want an alternative to MongoDB, one that’s available with an open source license rather than a source-available license. For now, as there’s no SDK, you’ll need to build your own calls to the API. These are based on MongoDB, so porting applications shouldn’t be too complex.
Why this? Why now?
The reasoning behind the DocumentDB project seems to be the big ambition to deliver a standard NoSQL API and engine, much like that developed for SQL. Microsoft has a lot of experience working in standards bodies, especially building and delivering the essential tests needed to make sure that any implementation of the resulting standard meets the necessary requirements.
Article originally posted on mongodb google news. Visit mongodb google news
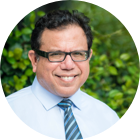
MMS • RSS
Posted on mongodb google news. Visit mongodb google news
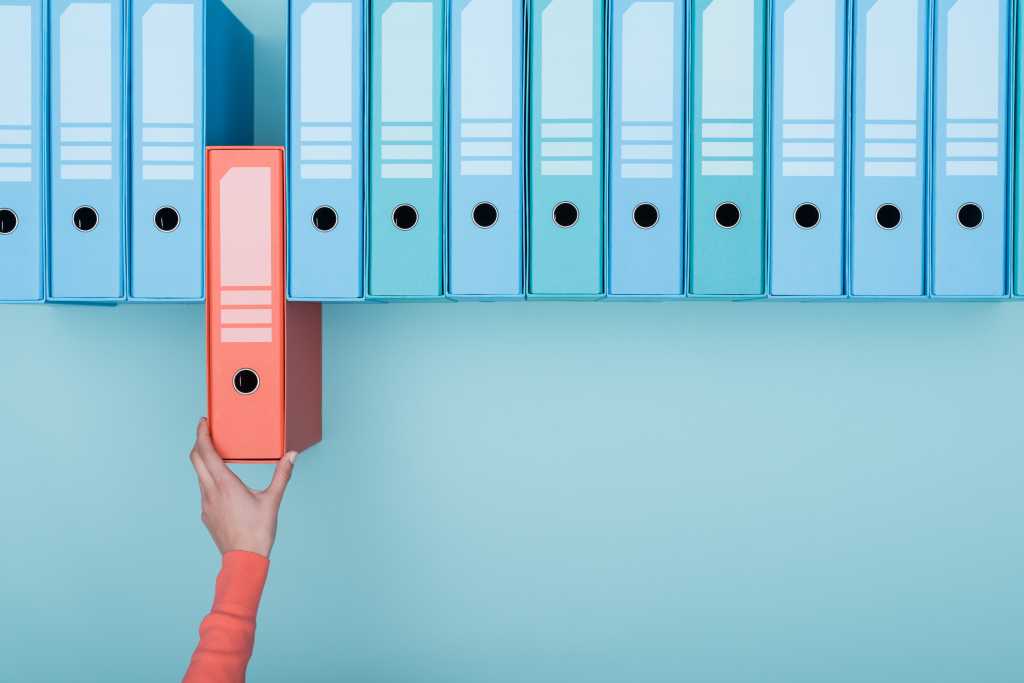
From the shell, you can embed API calls in select statements. This allows you to experiment with operations before adding them to calls from your code. The shell lets you build collections, add items, and experiment with CRUD operations. Other operations apply filters and support queries, as well as building indexes across one or more fields in a collection. You can find a lengthy list of documented API functions in the project wiki, grouped into common sets of operations.
For now, the GitHub wiki is the main source of documentation for DocumentDB. It’s a little on the thin side and could do with more examples. However, DocumentDB is currently intended for developers who want an alternative to MongoDB, one that’s available with an open source license rather than a source-available license. For now, as there’s no SDK, you’ll need to build your own calls to the API. These are based on MongoDB, so porting applications shouldn’t be too complex.
Why this? Why now?
The reasoning behind the DocumentDB project seems to be the big ambition to deliver a standard NoSQL API and engine, much like that developed for SQL. Microsoft has a lot of experience working in standards bodies, especially building and delivering the essential tests needed to make sure that any implementation of the resulting standard meets the necessary requirements.
Article originally posted on mongodb google news. Visit mongodb google news
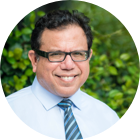
MMS • RSS
Posted on mongodb google news. Visit mongodb google news
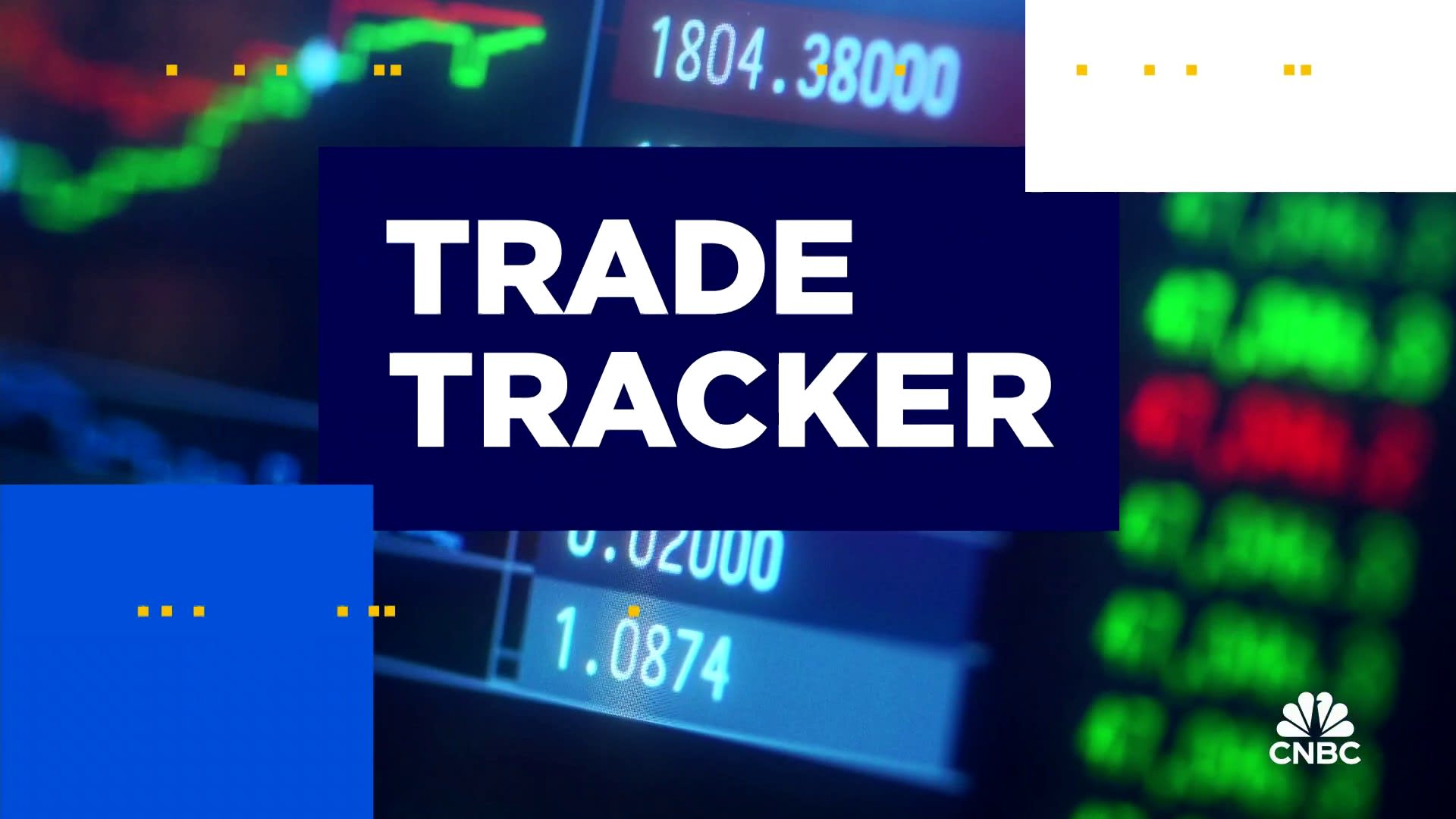
Kevin Simpson, Capital Wealth Planning founder and CIO, joins CNBC’s “Halftime Report” to detail his latest portfolio moves.
03:17
Thu, Jan 30 20251:22 PM EST
Article originally posted on mongodb google news. Visit mongodb google news
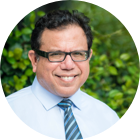
MMS • RSS
Posted on mongodb google news. Visit mongodb google news
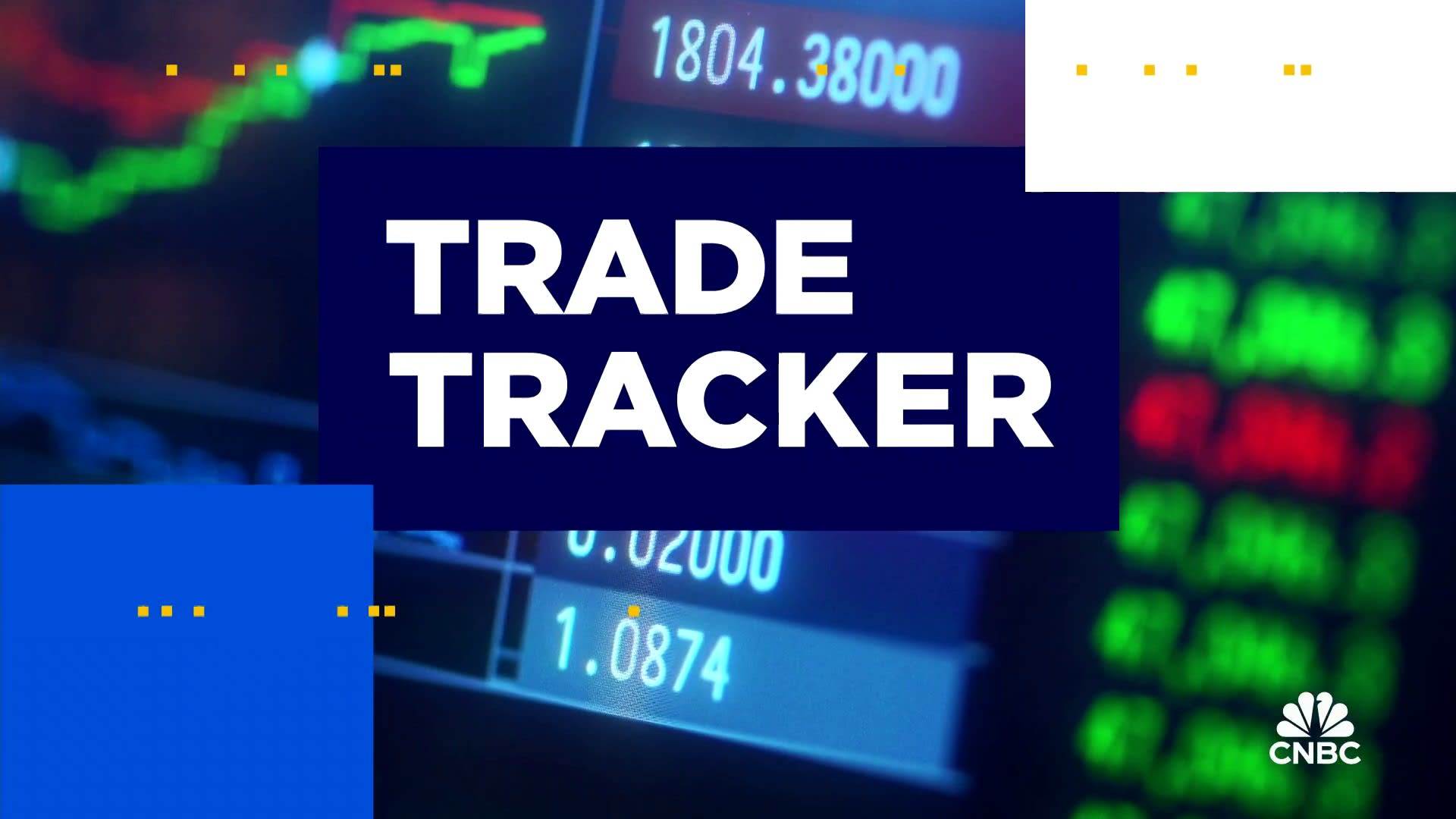
Kevin Simpson, Capital Wealth Planning founder and CIO, joins CNBC’s “Halftime Report” to detail his latest portfolio moves.
03:17
Thu, Jan 30 20251:22 PM EST
Article originally posted on mongodb google news. Visit mongodb google news
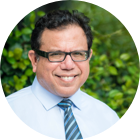
MMS • RSS
Posted on mongodb google news. Visit mongodb google news
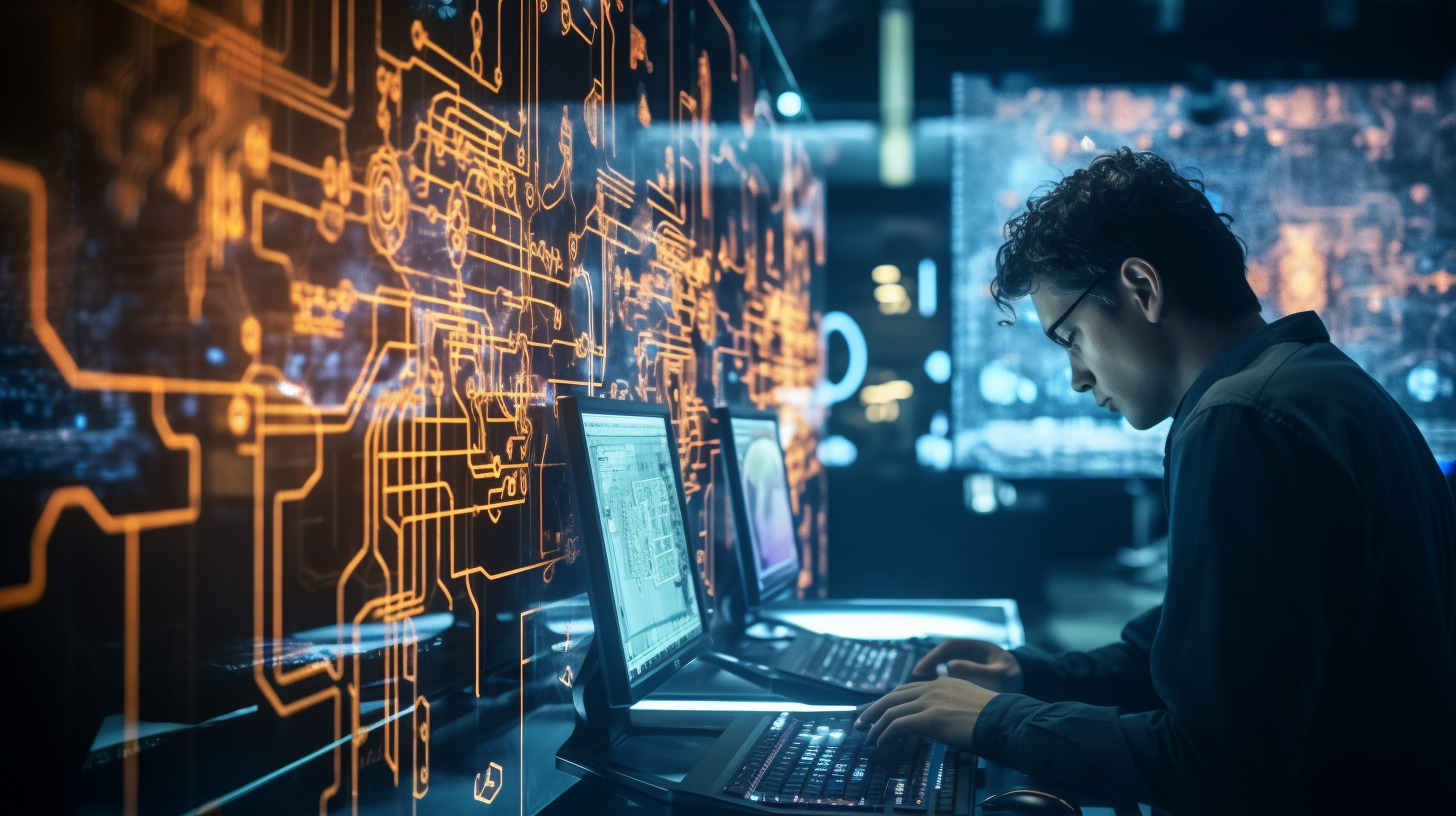
Artificial intelligence is the greatest investment opportunity of our lifetime. The time to invest in groundbreaking AI is now, and this stock is a steal!
The whispers are turning into roars.
Artificial intelligence isn’t science fiction anymore.
It’s the revolution reshaping every industry on the planet.
From driverless cars to medical breakthroughs, AI is on the cusp of a global explosion, and savvy investors stand to reap the rewards.
Here’s why this is the prime moment to jump on the AI bandwagon:
Exponential Growth on the Horizon: Forget linear growth – AI is poised for a hockey stick trajectory.
Imagine every sector, from healthcare to finance, infused with superhuman intelligence.
We’re talking disease prediction, hyper-personalized marketing, and automated logistics that streamline everything.
This isn’t a maybe – it’s an inevitability.
Early investors will be the ones positioned to ride the wave of this technological tsunami.
Ground Floor Opportunity: Remember the early days of the internet?
Those who saw the potential of tech giants back then are sitting pretty today.
AI is at a similar inflection point.
We’re not talking about established players – we’re talking about nimble startups with groundbreaking ideas and the potential to become the next Google or Amazon.
This is your chance to get in before the rockets take off!
Disruption is the New Name of the Game: Let’s face it, complacency breeds stagnation.
AI is the ultimate disruptor, and it’s shaking the foundations of traditional industries.
The companies that embrace AI will thrive, while the dinosaurs clinging to outdated methods will be left in the dust.
As an investor, you want to be on the side of the winners, and AI is the winning ticket.
The Talent Pool is Overflowing: The world’s brightest minds are flocking to AI.
From computer scientists to mathematicians, the next generation of innovators is pouring its energy into this field.
This influx of talent guarantees a constant stream of groundbreaking ideas and rapid advancements.
By investing in AI, you’re essentially backing the future.
The future is powered by artificial intelligence, and the time to invest is NOW.
Don’t be a spectator in this technological revolution.
Dive into the AI gold rush and watch your portfolio soar alongside the brightest minds of our generation.
This isn’t just about making money – it’s about being part of the future.
So, buckle up and get ready for the ride of your investment life!
Act Now and Unlock a Potential 10,000% Return: This AI Stock is a Diamond in the Rough (But Our Help is Key!)
The AI revolution is upon us, and savvy investors stand to make a fortune.
But with so many choices, how do you find the hidden gem – the company poised for explosive growth?
That’s where our expertise comes in.
We’ve got the answer, but there’s a twist…
Imagine an AI company so groundbreaking, so far ahead of the curve, that even if its stock price quadrupled today, it would still be considered ridiculously cheap.
That’s the potential you’re looking at. This isn’t just about a decent return – we’re talking about a 10,000% gain over the next decade!
Our research team has identified a hidden gem – an AI company with cutting-edge technology, massive potential, and a current stock price that screams opportunity.
This company boasts the most advanced technology in the AI sector, putting them leagues ahead of competitors.
It’s like having a race car on a go-kart track.
They have a strong possibility of cornering entire markets, becoming the undisputed leader in their field.
Here’s the catch (it’s a good one): To uncover this sleeping giant, you’ll need our exclusive intel.
We want to make sure none of our valued readers miss out on this groundbreaking opportunity!
That’s why we’re slashing the price of our Premium Readership Newsletter by a whopping 70%.
For a ridiculously low price of just $29.99, you can unlock a year’s worth of in-depth investment research and exclusive insights – that’s less than a single restaurant meal!
Here’s why this is a deal you can’t afford to pass up:
• Access to our Detailed Report on this Game-Changing AI Stock: Our in-depth report dives deep into our #1 AI stock’s groundbreaking technology and massive growth potential.
• 11 New Issues of Our Premium Readership Newsletter: You will also receive 11 new issues and at least one new stock pick per month from our monthly newsletter’s portfolio over the next 12 months. These stocks are handpicked by our research director, Dr. Inan Dogan.
• One free upcoming issue of our 70+ page Quarterly Newsletter: A value of $149
• Bonus Reports: Premium access to members-only fund manager video interviews
• Ad-Free Browsing: Enjoy a year of investment research free from distracting banner and pop-up ads, allowing you to focus on uncovering the next big opportunity.
• 30-Day Money-Back Guarantee: If you’re not absolutely satisfied with our service, we’ll provide a full refund within 30 days, no questions asked.
Space is Limited! Only 1000 spots are available for this exclusive offer. Don’t let this chance slip away – subscribe to our Premium Readership Newsletter today and unlock the potential for a life-changing investment.
Here’s what to do next:
1. Head over to our website and subscribe to our Premium Readership Newsletter for just $29.99.
2. Enjoy a year of ad-free browsing, exclusive access to our in-depth report on the revolutionary AI company, and the upcoming issues of our Premium Readership Newsletter over the next 12 months.
3. Sit back, relax, and know that you’re backed by our ironclad 30-day money-back guarantee.
Don’t miss out on this incredible opportunity! Subscribe now and take control of your AI investment future!
No worries about auto-renewals! Our 30-Day Money-Back Guarantee applies whether you’re joining us for the first time or renewing your subscription a year later!
Article originally posted on mongodb google news. Visit mongodb google news