Category: Uncategorized
Presentation: Building SaaS From Scratch Using Cloud-Native Patterns: A Deep Dive Into a Cloud Startup
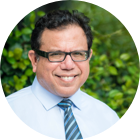
MMS • Joni Collinge
Article originally posted on InfoQ. Visit InfoQ
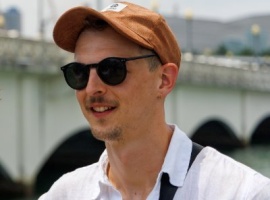
Transcript
Collinge: We’re going to be talking about building SaaS from scratch using cloud native patterns, a deep dive into a cloud startup. We’re going to start our session with a bit of a backstory. Our story begins back in late 2018 with Mark Fussell and Yaron Schneider. Now at this time, they’re working at Microsoft in a research and development team, and they’re incubating projects, one of which became the KEDA project, the Kubernetes Event Driven Autoscaler. They’re thinking about the problem of how to make enterprise developers more productive when it comes to writing distributed and cloud native applications. They come up with an idea that they start to ideate on. It gets a bit of traction. They start to prototype it, and eventually Microsoft publicly make this open source as the Dapr project, which stands for the Distributed Application Runtime. Who’s heard of the Dapr project? Is anyone actually running Dapr in production today? The Dapr project really started with the ambition of codifying best practices when it comes to writing distributed applications, so things like resiliency, common abstraction patterns, security, observability. Can we bake all of this into an easy way for developers to consume, that is cloud agnostic, that is language agnostic, and framework agnostic?
This is where I join the story. My name is Joni Collinge. At the time, I was also an engineer working at Microsoft, and I spent the last 8 years seeing enterprise developers solving the same problem time and again on the Azure platform. The value proposition of Dapr really resonated with me. I started becoming an open source maintainer of the project, and started contributing to it almost immediately. The project continued to develop and mature, and eventually Microsoft decided to move to an open governance model, where they donated it to the CNCF, and it became an incubating project. This is where we really start to see an uptick in enterprises adopting the project. Mark and Yaron decided that they were going to double down on this mission of empowering developers to build distributed systems and actually create a new company called Diagrid. For some reason, unbeknown to me, they decided to ask me to become a founding engineer at Diagrid to help build out the cloud services to deliver on this mission. After a little bit of convincing, I did just that and joined them at Diagrid. We had a vision to build two services off the bat, the first of which was called Conductor. Conductor was about remotely managing Dapr installations in users’ Kubernetes cluster. That was our first ambition. Our second ambition was building the Catalyst service, which would be fully serverless Dapr APIs, and we would turbocharge those as well with providing infrastructure implementations and a bunch of value-added features that you wouldn’t get through the open source project. That was the vision.
We’re going to start today’s presentation right there, and we’re going to look inside the box. Because I think a lot of the time we talk about clouds as just this black box, and we don’t really understand what’s going on inside those. A lot of these big cloud providers treat this as their secret sauce, when really, it’s just common patterns that are being applied across each of the cloud and really the secret sauce is the services that you’re exposing. Hopefully, this talk is insightful for anyone who’s going on this journey, and maybe encourage others to share how they’ve approached the same problems.
Why Do I Need a Cloud Platform to Do SaaS?
As a SaaS provider, why do you even care about a cloud platform? The cloud platform is all of the plumbing that actually delivers your service to your end users, and gives them a unified experience to adopt services across your portfolio. It includes things like self-service, multi-tenancy, scalability, and so on. There are many more things that I haven’t been able to list here. We’re just going to focus on the top five. I’m going to look at self-service, multi-tenancy, scalability, extensibility, and reliability. There might be some of you that are thinking, is this going to be platform engineering? Am I veering into a platform engineering talk? Although a lot of the problem space that we’re going to talk about and some of the technologies is common to both platform engineering and cloud engineering, I do want to make a distinction that it’s the end user of the cloud that is different. Internally, when you’re thinking about a platform engineering team, they are delivering a platform for your internal developers to build services, whereas the cloud platform I’m talking about is delivering a cloud to your end users so that they can adopt your services having a unified experience.
Most of you will probably think GCP, AWS, or Azure, or one of the other cloud providers that are out there when you think of a cloud platform. At Diagrid, our mission was to build a higher-level cloud that would serve the needs of developers rather than just being an infrastructure provider or service provider. We were going after these high-level patterns and abstractions and trying to deliver those directly to application developers so that they could adopt them from their code directly, rather than infrastructure teams provisioning Kafka or something like that. Obviously, as a startup, we’ve only got limited capacity. We’re going to be bootstrapping ourselves on top of existing cloud infrastructure. When you are adopting cloud infrastructure, obviously each of those are going to be providing different sets of APIs and abstractions to adopt. Some of those are common, things like Infrastructure as a Service, virtual machines. Even some platforms such as Kubernetes allow you to natively use those across the cloud. Then obviously you’ve got richer and higher-level proprietary services, things like Functions as a Service and container runtimes, things like that, which are bespoke to every cloud. At Diagrid, our strategy was to be cloud agnostic and portable. That might not be your strategy. If your strategy is to go all in on one particular cloud, then you can make slightly different tradeoffs. We decided to be cloud agnostic, and this meant that our abstractions were: we went for Kubernetes as our compute abstraction, we went for MySQL as our database abstraction, and we went for Redis as our caching and stream abstraction.
Just to think a little bit about the user journey of using a cloud, and I’m sure everyone has done this. You’ve sat at your laptop, you’ve got an SSH or an RDP session to some cloud VM running somewhere. What can we infer about that virtual machine? We know it’s running in some hypervisor, in some server, in some rack, in some data center, in something that a cloud calls a region. We’ve got this concept of regions that clouds are telling us about. How did that virtual machine get there? Or presumably you went to some centralized service that was hosted by that cloud provider, either via web page or via some form of CLI or potentially some SDK, and you ask that cloud provider to provision that virtual machine in that region. You would have had a choice of regions when you made that request, so you could have provisioned into various regions around the world that the cloud provider supports. Obviously, we can infer something about the cardinality here, that there is some global service that is able to provision resources into these regions at our demand. How does this actually look at Diagrid? Some terminology I want to set at this point is you can think about this centralized service offered by the cloud provider as a cloud control plane, and then we think about regional data planes which are configured by that cloud control plane. How does this look at Diagrid Cloud? For the Catalyst service that I talked about earlier, which is this serverless API service, it looks pretty much exactly like that model. We have a centralized control plane where we can provision infrastructure into regional data planes, which users can then consume from. For Conductor, where we’re actually managing infrastructure in users’ environments, the user is responsible for provisioning. We allow them to come and create something called a cluster connection. They can configure how they want that Dapr installation to look like. At the end of the day, it’s running in their Kubernetes cluster, so they are the ones that have to install it. We effectively give them an artifact to install, and from that point on, it connects back to our control plane and then can be remotely managed. There’s two slightly different use cases there that we have to support within Diagrid Cloud.
The big picture, we can think about admins managing cloud resources through some centralized control plane, which is in turn configuring data planes at the regional level to expose services for users to consume. As I said earlier, our compute platform was Kubernetes. This does mean, ultimately, that we’re going to have one or more Kubernetes clusters as part of our control plane, and then many data planes provisioned within regions spread across those. Just to touch a little bit on the multi-cloud story, because many people will say, I don’t care about multi-cloud. At the control plane, I think you’ve got more flexibility about making that choice, things like egress costs and stuff you might need to consider, but that’s becoming a bit of a non-issue, given some of the legislation changes. At the data plane, you might actually have customers, if you are working for enterprises, who are going to come to you and they’re going to say, I have a regulatory or a compliance reason that I need to only store data in this cloud provider and only in this region. If you’ve gone all in on one particular cloud provider, and they’re not portable and can’t even pretend to potentially move to an on-premise model, at the data plane, you might not be able to service those customers. Just something to consider is to keep your data plane as portable as possible. You might disagree with that, but that’s just one of my pieces of advice.
The Control Plane
We’re going to click into this control plane. How can we think about actually exposing this? Most clouds effectively have the same infrastructure to support this. Really that front door is some form of API gateway that’s going to be dressed up in many forms, but that API gateway basically has a bunch of centralized functionality that you don’t want in your control plane services, or you don’t want to repeat in your control plane services. It does things like authentication through some IDP. It does authorization. It does audit. Then it does some form of routing to control planes and control plane services. This is effectively a solved problem. I’m not going to spend too much time here, but API gateways, there’s many vendors. Take your pick. Then, what is that API gateway actually routing to? Sometimes we think about the control plane as just a big black box again. Is it just one monolithic service that’s servicing all of our different user experiences? I’ll break that down in a couple of slides. As you scale your control plane, you’re taking on more users, more resources, and you’re having to store more tenants. You might start to think about the cellular architecture. It’s basically partitioning your cloud. You’ll partition your control plane, and then book it different tenants into different instances of that control plane. They then map onto regions. You need to map onto regions given demand. You’re not mapping onto regions for scale. You only actually move between regions, possibly for some form of availability, but that’s handled at the AZ level. Mainly, it’s for data sovereignty reasons, or to serve particular customers with low latency. Really, you generally book it those cells and map them onto regions depending on your users.
What services do we actually have inside that control plane? I’ve just taken a couple of screenshots. These are just a couple of screenshots from our Catalyst product. I think the experiences that they’re exposing are fairly common to most cloud providers. We have the concept of configuring resources, and we’ll get into that. We have some visualizations that the cloud is providing to us. We have API logs and other telemetry, and we have graphs as well from the telemetry. There’s lots of other types of data that you’ll be interfacing with as a cloud provider, but these are just some common core functions that I think you need to think about as a cloud provider. We can think about breaking that down into types of services. I’m not saying these are the services like you need to go and write a resource service. I’m just saying these are the types of services you need to think about how you handle that data. We think about resources. Think about views, which is those visualizations that’s read only data that is built within the system to expose to your users. Then you have telemetry, which usually includes things like logs, metrics, and sometimes traces as well. There’s a bunch of other stuff that you also need to support. We’ll focus on resources and views for this session.
Resources API
How should we design our control plane resources API? There is some prior art in this space. GCP, AWS, and Azure all have public APIs, and they’re all working quite well. You can have a look at their documentation. You can understand how they work. Thankfully for us, GCP have a design document about how they did go about designing their cloud APIs. It really boils down to these three very simple steps. We’re going to use declarative resources so that the consumer doesn’t care how that cloud provider actually works. Those resources can be modeled in a hierarchy, which tells us that there’s some relationships between those resources, and that can be nesting. Those resources can either be singular or they can be in a collection, like a list. Then we’ve got these standard methods which can be performed on every resource. We’ve got list, get, create, update, and delete. Anyone who’s thinking, this sounds an awful lot like RESTful API principles is absolutely bang on. This is just a REST API. All they’re saying is, you need to build a REST API over whatever domain objects your cloud wants to expose. One thing they don’t really tell us more about, and they’re all taking a slightly different approach here is, how should you actually shape those resources? What does a payload look like that you’re interfacing with? What does that mean for your system?
Is there something from the cloud native space that we can actually look for more inspiration here that gives us a more fully featured API design. This is where we introduce the Kubernetes resource model. The Kubernetes resource model is only the API part of Kubernetes effectively, and it’s designed in isolation from the rest of the system. It does have ramifications on the rest of the system, but it is its own design proposal. If you actually read the design proposal, it says that it is analogous to a cloud provider’s declarative resource management system. They’ve designed it from the ground up as a cloud resource management system. How do they expose their resources? As many of you probably know, Kubernetes uses this declarative YAML format where it has some common properties, such as API version, a kind which is effectively an API type, some metadata, and then a spec and a status. By having this common shape for all of its resources, it means that the Kubernetes API server has a bunch of API machinery, which only operates on generic objects. It has a bunch of code that it doesn’t need to rewrite for every single type in the system, it just handles generic objects. It doesn’t care about the specialization of that particular object. The specialization comes through the fields of the spec and the status. The spec is there to define the desired state of the world, the object. The status is the feedback mechanism from the system to report back a summary of the last observed status of this resource. Even by looking at the declarative resource, we can start to infer the type of system that we’re going to have to build to perform what we call reconciliation against this resource. A resource like this is mapped onto a HTTP path like that, which is fairly intuitive.
To look at a concrete example, this is a pod definition. The pod definition is clearly just saying that I want a container that is called explorer, and it uses this image. That API shape is defined by Kubernetes. This is one of their API types they’ve hardcoded into the API server. You can operate on these types of resources at these URL paths, and you can use these HTTP verbs. You can use GET, PUT, POST, PATCH, DELETE. It’s all fairly straightforward, fairly intuitive, and that’s what we want from our resource API. Why can’t we use the same exact approach for our own resource types? Why does it have to be a pod? Why can’t it be our own type, whatever we want to expose in our cloud? Why can’t we just use the same path structure and the same methods? There’s nothing stopping us. Just to touch on a slight tangent here, is that if you’re familiar with Azure, then you might be familiar with what are called ARM templates. If you’re familiar with AWS, you might be familiar with CloudFormation. These are a way of you basically composing resources and sending an entire unit towards the cloud, and the cloud then goes through that, parses it, and provisions all of the resources and manages the dependencies. As a cloud provider, do you think that you need something similar? If you look at KRM, it explicitly says that they don’t do that. They don’t bake in templating, but what they do do is something called resource composition, which means that you can implicitly define a higher-level resource which will ultimately break down into multiple lower-level resources. Or you could take the Crossplane approach, which is to have a resource type which explicitly defines those resources. It says, these are all the different resources, these are the dependencies. Then it’s up to the control loop, whatever that logic is, to parse that and process it. Or another alternative is to do something like Terraform or OpenTofu these days, and that is that you just defer this to the client. Terraform does not run on top of ARM templates or CloudFormation APIs. It runs on cloud primitive APIs, and it manages a dependency graph, and it manages the state, so you can always offload this to the client, and that might be a better experience than what you actually build natively in your cloud.
Just to summarize what I’ve covered so far. A cloud has a brain called a control plane, which configures many data planes. Authentication, authorization, audit, and routing can be provided via an API gateway. Cloud resources should be exposed via a REST like API. Kubernetes actually gives us a blueprint for how to build that API. High-level resources can compose low-level resources, which could avoid you doing things like templating.
Resources API Server
How do we actually expose that resource API? Many of you might be thinking, you’re running your control plane on Kubernetes, so you’ve got a Kubernetes API, why don’t we just expose that to the customers? Why don’t we just let users create objects in that Kubernetes API? I’m going to suggest that this is not a good idea, and that’s primarily because Kubernetes API is not multi-tenant, so you’re effectively going to be competing as a service provider with your own users. Your users are going to be creating objects in that API server. You’re going to be creating objects in that API server. Kubernetes can’t differentiate between you as a service provider and your users, and therefore will throttle you both accordingly. What we do want to do is we want to find another way of exposing a Kubernetes like API server. I’ve changed the terminology here to Kubernetes like, because I want you to think about this from the abstract sense. You want to think about the founding principles of what Kubernetes is exposing, the behaviors and the concept that it’s using, and seeing if there’s other ways that we can potentially get that that is not running Kubernetes, but it could potentially be running Kubernetes. I just want us to box ourselves into only thinking that Kubernetes is the solution. A couple of options you might be thinking about here is, just run Kubernetes on Kubernetes, and manage your own etcd server. I’m sure some people are doing it. It comes with overhead. You might even use something like the cluster API that’s exposing Kubernetes to provision managed clusters somewhere else that you’re going to use for your customers, or you might use technologies like vCluster or Capsule to try and build a multi-tenant model on top of the existing Kubernetes API server. I’m sure, again, you can build a system like this, where you’re provisioning independent API servers for your tenants and storing their resources, isolated inside that API server. There are a few projects to call out that are specifically built to try and solve this problem. One of them is KCP. KCP, I’m pretty sure, came out of Red Hat, maybe like 5 years ago, 6 years ago. It was a bit of an experiment. What they were trying to do is repurpose Kubernetes to literally build control planes. There’s lots of really good ideas and lots of good experiments that have gone into that project. Maybe going back two-and-a-half years ago, when we were building this cloud, the future of the project was a little uncertain, and it was basically just a bunch of promises and some prototypes. It’s definitely worth checking out if you are interested in this space. Basically, it has this concept of workspaces, which allows you to divvy up your API server and use it as a multi-tenant API server, which gives you stronger isolation than just namespaces, which is what you would get out of Kubernetes natively. Another technology you might have come across is Crossplane. This gives you rich API modeling abstractions, and it also gives you these providers that can spin up cloud infrastructure and various other systems. The problem with Crossplane is it needs somewhere to store those resources. You can’t just merely install Crossplane and then it runs. You need an API server in order to drive Crossplane. You have this bootstrapping problem where you still need to solve the API server problem. There are companies like Upbound who provide this as a managed API server. If you are interested in going down that road, check that out. Finally, there’s always the custom option, where we just learn from what these systems show us and try and build our own system.
I think in order to really make the decision of which way we want to go here, we really need to understand what those founding principles are. I’m just going to unpack the Kubernetes API server quickly, just so that we understand exactly what we’re going after in terms of the behavior we want to replicate. The Kubernetes like API server, as I’ve mentioned, is just a REST API, so I start up a HTTP server and start registering routes. How do those API types get into the API server? They can either be hardcoded or they can be registered through some dynamic mechanism. Then once a request comes in, you’re just going to perform the usual boilerplate stuff that you do in a REST API. You’re going to do some validation against some schema. You’re going to do defaulting and transformations. Ultimately, what you want to do is you want to store that resource somewhere. The reason you want to store that resource is you want to move from the synchronous world of the request to the asynchronous world of the processing. I’ve built systems. I’ve worked with people on systems that basically store this in all sorts of different types of storage. It could be a database, it could be a queue, could be a file system. I’ve even seen people modifying Git repositories. Basically, depends on the context of what you’re trying to solve. As a general-purpose control plane, I say the best choice here is to store it in a database. That’s what they’re good at. What you want from that database is you want to be able to enforce optimistic concurrency controls. What I mean by optimistic concurrency controls is that you can effectively get a global order of operations on any resource that’s stored in that database. The way you do that is through a sequence number. Every time you want to mutate a resource, let’s say you’ve got multiple requests that are going to be concurrently accessing a resource, and they all want to perform an update, if you took a system that does something like last write wins, you’re going to lose data. Because they’re all just going to start writing over each other. You need to enforce an order of operation to avoid data loss. With optimistic concurrency controls, when you first read the resource, you will get a sequence number with that. Let’s say the sequence number is 3. You then perform your update, and then you write it back to the database. On that write, if that sequence number has changed just to a value that you are not expecting, the database rather will reject that write, and you will now have to reread that resource, reapply the update, and write back to the database. This is really useful for these systems. Once the data is stored in the database engine, we then want to asynchronously through some eventing mechanism, trigger some controllers to perform the reconciliation.
This is a really interesting point that we’ve been talking about Kubernetes and all of the patterns from Kubernetes, but you could build this system on AWS using serverless. You could use lambda as the API server. You could store your data in DynamoDB. You could use EventBridge to trigger your controllers, and those controllers could be lambdas. You use the context of your problem space and the decisions you’re making about what platforms you want to run on and what abstractions you want, to actually build the system, but just look at the founding principles that we’re trying to build the system on top of, and the behaviors that we’re going after. We sometimes refer to this as choreography, because it’s event based. That means that there’s clearly going to be the alternative, which we can talk about as orchestration. This might be that you basically predefine all your reconciliation logic, and you bundle it into some workflow engine, and the request comes in, and then you effectively offload that to the workflow engine to durably execute, and you expect the workflow engine to handle transient failures, do things like compensation during errors, and all the rest of it. Some technologies you might want to think of, if you’re going down this road, is something like Temporal or even Dapr workflows. My personal preference is to go with the database approach first, so write the resource to the database. The reason for that is you can then read it. Rather than going off and having some asynchronous workflow run, you have a resource that’s stored in the database that represents the latest version of that resource that you can quickly serve to your clients immediately. Then you have the eventing mechanism that triggers your controllers, and that eventing mechanism decouples the controllers from the resource, which means future use cases, as you bring them online, don’t have to reinvent everything. They can just simply subscribe to that eventing mechanism and start writing the logic. If those controllers themselves need to use some durable workflow to execute their logic, then go and do it, so be it. You can use both choreography and orchestration together to get the best of both worlds.
How does this actually work in Kubernetes? You’ve got the Kubernetes API server. It has some hardcoded types, things like pods, config maps, secrets, all that gubbins. It supports also custom API types via CRDs or custom resource definitions, and then it writes to its database, which is etcd. It uses optimistic concurrency control, and it uses a sequence number that’s called resource version. We’ve talked about all of that, and that makes sense. Now we’ve stored our resource in etcd, and it has this concept of namespaces, which allows you to isolate names of resources, because that’s all a namespace is. There’s no more isolation beyond literally just separating names with a prefix. Then, it has the concept of a watch cache. For every type of API that you bring to the API server, every CRD, you are going to get a watch cache that’s going to watch the keys in etcd. etcd has got this nice feature that does this natively. The API server is going to build these in-memory caches of all of your resources in order to efficiently serve clients. Some of those clients are going to be controllers, and controllers, you can build them a million different ways, using things like controller runtime or just client-go, or whatever. They all typically follow the same pattern of having this ListWatch interface. What that means is that when the controller comes online, it initially does a list. It says, give me all of the resources of this kind. Then, from that point on, it just watches for new resources. Then, periodically, it will do a list to see if it missed anything from those watch events. That basically is the whole engine that’s driving these controllers, running the reconciliation. As we know, Kubernetes was not invented to support CRDs out of the bat. What it was invented for was scheduling workloads onto nodes. You have the scheduler, and you also have all of these workload types that you might not actually need in your system, but you have because you’re using Kubernetes. You might want to consider that baggage for the use case that we’re talking about.
What did we do at Diagrid? People say, don’t build your own databases. They probably say, don’t build your own API servers either, but we did. Basically, we tried to take the simplest approach, which was that we did things like statically build in all of our API types into our API server. We used effectively the same API machinery as Kubernetes in order to handle our resources which were ultimately written to our database. Rather than using etcd, which is horrible to run, and no cloud provider offers a managed version, we just write it directly to our managed SQL database, and then we set up a watch. Rather than the watch cache building an in-memory buffer of all of these resources, we externalize the state to a Redis cache, and we also push onto a stream to trigger the controllers. This is like a change data feed that will drive the controllers. Notice where those controllers are. Those controllers are actually inside the API server, which means we install our API server, we get all of our types, and all of our control logic inside that single monolithic API server, which we can then scale horizontally, because all of our state is externalized. Then we also added support for remote controllers as well, which run outside of the API server, and they use the ListWatch semantics that we saw in Kubernetes as well. Just one thing to call out there is that you can efficiently scale your database by vertically partitioning by kind. Because in the Kubernetes world, you only ever access your resources by kind. You list pods. You list deployments. You don’t necessarily or very often go across resource types, so you can partition that way to efficiently scale.
If we dive in a little deeper into the API server to look at how that actually works internally? We’ve got all the REST gubbins that you would expect, and that’s the Kubernetes like API machinery, but that then interfaces with something we call resource storage. At the resource storage layer, we are using that generic object. All of that specialization of all the types and everything is basically lost at this point. We’ve done all the validation. We’ve done all the templating and all that stuff. We’re now just working with generic objects. That resource storage is abstracting us over the top of a transactional outbox pattern. When we write to our resources table, we are transactionally writing to an event log table at the same time, and that allows us to set up a watcher that is subscribed to that event log change, and then when it detects that there’s a change or an offset change, it will grab the relevant resource, update the cache, and then push an event onto the stream to signal the controllers. It does all of that using peek lock semantics so that it won’t acknowledge the offset change until it has both grabbed the resource, updated the cache, and pushed to the stream. What we’re getting from the stream is what we call level-based semantics, and this is the same as Kubernetes. What this means is, because we have ordered our changes at the resource in the database layer, we don’t have to operate on every single event, because we know the last event is already applied on top of every other resource change that has come before it. Effectively, you can compress 20, 30, 40, 100 changes if they happen in a quick time, into a single reconciliation at the controller level. These controllers have to run idempotently to support things like retries. They basically run until they succeed. Or, if they have some fatal error, they’ll dead letter, and this will feed back through to basically report a bug in the system at this point.
These controllers are clearly not Kubernetes controllers, and we’ve had to build our own framework for this. We have this generic base controller that is abstracting the interface to the cache and the stream, and it also performs a resync to basically do drift detection. When it detects that there is something that we need to reconcile, it will basically only call add or delete. An add is for a create or an update, and a delete is obviously for a delete. It calls that on the actual controller’s reconciliation logic, and that controller will then do whatever it needs to do to reconcile that resource. That logic is completely API specific, whatever that reconciliation looks like. There are some other things that our controllers do because they are so lightweight, is they just generate data. You don’t think about a Kubernetes controller that just writes a row to MySQL, you usually think about a Kubernetes controller that then goes and configures some cloud resources or updates things in Kubernetes, but why not use the same pattern to just drive database changes and business logic? We actually have these lightweight controllers that can do things like that, and they could just build things like materialized views. For instance, that visualization we talked about earlier, you could just have that as some reconciliation over a graph type or whatever. You can start to think about using this really generic pattern in lots of different ways. Once the reconciliation logic is completed, it effectively calls update status, which is the feedback mechanism to close out the full reconciliation. The system detects that, ok, we don’t need to do anything else, this resource is reconciled. For anyone who’s deeply interested in controllers and that logic, we do also use finalizers for orchestrating deletes. If you are interested, check that out on Kubernetes, because it’s well documented.
To summarize, try to isolate user resources from your internal resources, especially through some form of tenancy or a specific Kubernetes cluster. Evaluate the various ways that you can run a Kubernetes like API server against your use case. It’s not necessarily the only option to run Kubernetes. A system can support both choreography and orchestration, and they both have advantages and disadvantages, so use them wisely. Resource composition can satisfy some templating use cases.
The Data Plane
We’ve talked about the control plane, but the data plane is where actually things get configured to actually give a service to end users. I like to think about this in a few different models. There’s the centralized approach where all of the resources we’ve been talking about are being stored in an API server at the control plane level, and then that’s where the compute is running, or the controllers which are reconciling those resources into the data planes. You have all of this centralized management and all the reconciliation happening centrally, but it’s reaching into all of those regions and configuring the data planes. This approach might work well at a fairly low scale, and it does have some downsides, which I’ll get onto in future slides. The second approach I think about is decentralized control, and this is where you have resources stored at the control plane level, but you are synchronizing them down to the data planes at the regional level, which is actually where the controllers run to do the reconciliation. Obviously, the API servers are only synchronizing the particular resources that they need in that data plane. I’ll quickly just touch on KCP. This is similar to how KCP basically builds its model, which is that you can have these virtualized workspaces and API servers, but you then bind it to workload clusters, which is actually where the work happens. The last approach that I’ll quickly touch on is the federated control approach, which is that no resources are stored at the control plane at all. Basically, you’ve just got a big router. That router is directing you to whichever data plane you need in order to store that resource. Then the controllers continue to run in the data plane. By extension of this model, you could also think about a mesh model where basically all the API servers are in some form of mesh and can talk to each other, and can share resources among the regions. That’s a lot more complicated.
At Diagrid, we’ve followed the decentralized control model, which is similar to this, where you have a Kubernetes like API server in the control plane, and that’s where you store your resources. You need to somehow claim those resources from the data plane. You need to know which ones need to be synchronized down to the data plane. There is some form of claiming or binding which is assigning resources to a data plane. Then there’s a syncer, which is pulling those resources and updating the local API server, which then has the same logic we’ve already talked about, so that’s going to then drive the control loop, which will provision the end user services and shared infrastructure. One of the niceties about this approach is that that controller that is running in the data plane, can handle all the environment variance, because if you have a multi-cloud strategy, that could be running in AWS, it could be running in Azure, it could be running in GCP, it could be running in OpenShift, it could be running anywhere. Because that controller is running natively, it can use things like pod identity and it can use all of the native integrations with the cloud, rather than having some centralized controller having to second guess what that particular region needs. One of the things that we saw when we followed this approach is that you quickly start to bottleneck the API server, and if this is a managed API server from some cloud provider, you’re going to get throttled pretty quickly. That’s because you are synchronizing resources from the control plane into the API server, and then you have the controllers watching the API server, and then, in turn creating resources in the API server which also have controllers which are watching the API server, and so on. You end up really basically bottlenecking through your API server. We asked the question, could we go direct? Why are we using the API server at the data plane? Is it giving us any benefit? We basically summarize that we could go direct, but we would have to lose Kubernetes interoperability. We would lose the ability to use native Kubernetes controllers, and we would have to go it alone using our own custom approach. We did effectively build a model around this, which is that we have this syncer, which can rebuild state at any time from the Diagrid API server using that ListWatch semantics, which we talked about, and then it effectively calls an actor. There’s basically an actor per resource in the data plane. I’ll touch on this in the next slide a little bit more. This is all packaged in one of those remote controllers that we talked about earlier, which can talk to the Diagrid API server. All of these messages are going over a single, bidirectional gRPC stream, so we can efficiently pick up any changes in resources from the API server almost immediately and react to that without waiting for some 30-second poll or anything like that.
Let’s look at these actors a little bit more. This is not strictly an actor from some formal actor definition, but basically what it is, is it’s an object that represents something in the data plane. We think about things like projects or application identities or Pub/Subs, and things like that, as resources. This actor is like something that’s in the memory of the process, and it’s basically listing on an inbox for differential specifications. Changes to the specification are getting pushed to it through the inbox, and then when it detects that change, it updates its internal state of what that specification looks like, and then reruns a reconciliation loop, which is just using a provisioner abstraction to configure things in Kubernetes through either natively Kubernetes, or Helm, or a cloud provider. Throughout that process, it’s flushing status updates back up to the control plane, so you as a user, you can see it transitionally going through all of these states as it’s provisioning infrastructure and managing the various things that it needs to do. The reason I say it’s not strictly an actor is because there’s no durability. Our state can be rebuilt on demand, so we are not using any persistence for this actor. This actor is literally something that’s in memory. There’s no messaging between this actor and any other actor, which means there’s no placement, and there’s no activation. There’s none of that stuff. If you’re deeply familiar with actors and very strict on that, then me using the actor term is probably not correct, but it does give us the sense of the concurrency controls that we’re using, which is that we are blocking on an inbox channel and we are pushing through an outbox channel. In reality, this is actually leveraging Go’s concurrency primitives. This is actually a goroutine. This goroutine is listing to a Go channel, which is the inbox, and it’s writing on a Go channel on the outbox. The Go runtime is optimized to basically schedule these goroutines efficiently. They are virtual threads, green threads, whatever you want to call them, and you can have tens of thousands, if not hundreds of thousands of these in a single process, using very little memory and CPU. Because these actors are mostly idle, or they’re doing I/O bound work talking over the network, we can really efficiently context switch between many actors and do concurrent processing at the same time.
Just coming back to the top-level picture, the last thing I wanted to talk about here is the ingress path. How are the users actually talking to these end services? At the data plane level, you need to be exposing some form of public load balancer and ingress. You need to provide some way of them routing to these services. Typically, you might, like in this instance, use a Kubernetes ingress server with a public load balancer, and then use some wildcard DNS record to do the routing. Your user will have a credential that they will have got when they provisioned whatever resource it was through the control plane. You will either give them a connection string, an API token, or, preferably, an X.509 certificate. They then provide that to you on the data plane API, and then you perform the routing to whichever service is the one that they’re assigned to. A couple of things to think about here is that you will need to have variable isolation and performance levels at the services. It is just expected these days that if you are providing a cloud service that you can configure the performance so you can request more CPU, more memory, more throughput, lower latency, all of that stuff needs to be a scale. You need to build the system so that your actors can reconcile different types of system. They need to be able to say, I’m going to provision this inside some types of virtualization because I need stricter isolation, or I’m going to provision this using some external service because it gets higher throughput. You need to build in all of this variability into your data plane to support your end users.
Lastly, to summarize, clouds can use a centralized, decentralized, federated, or mesh approach to data plane resource propagation. Try not to set fire to your API server, because it’s quite hard to put out once it is. Consider how to handle environment variants in your data plane if you’re doing multi-cloud. Provide tiers of isolation and performance at the data plane. One size is not fits all when it comes to cloud resources.
Timeline (Dapr and Diagrid)
October 2019 is when Dapr was first open sourced and made public on GitHub. It was donated to CNCF in November 2021. I joined the project about a month after it was first announced in November 2019. Diagrid was span out in December 2021. We set out to build Conductor in about 7 or 8 months, which we pretty much did, and we did it with two backend engineers, one frontend engineer, and one infra engineer. That now serves hundreds of Dapr clusters and thousands of Dapr applications and millions of metrics per day. That’s now in production, and it’s actually free. Then the second service is Catalyst, and that went into private preview in November 2023. Again, we built that with a fairly lightweight team. We had four backend engineers, two frontend engineers, and two infra engineers, and we were also still working on Conductor and our open source project of Dapr. That also runs hundreds of Dapr clusters, they just happen to now be internal, thousands of Dapr applications, but also now has to process millions of requests per day as obviously, it’s an API as a service.
Questions and Answers
Participant 1: If you were to rewrite what you’ve done in the last 2 years, are there bits that you’re not happy or you would change, or has anything changed in the last 2, 3 years?
Collinge: Yes. We had to iterate a few times on this. Basically, the first way we built this was we had the Diagrid API server, and then we had more logic in the control plane that was effectively copying these resources down to a different database table, and then another gRPC API that then exposed it to the agent that ran in the data plane cluster. We realized we were just basically copying data all the way down, probably three times to get it into the data plane. Then we just had this light bulb moment where we were like, why don’t we just run this as a remote controller and just use a gRPC stream, because the previous model was built on polling, and it basically left us taking minutes to provision resources. Although users are pretty familiar with waiting minutes to create a virtual machine, if you want to build the next level UX for these things, being able to provision application identities and APIs in seconds is really what you’re after. Moving to this model allows us to reduce that time massively.
Participant 2: I saw that you’ve basically mimicked a lot of Kubernetes logic and functionality. Was it a conscious decision to not use Kubernetes as like a product decision to decouple yourselves from the scheduling system and be agnostic so you can run on any cloud, even ones that don’t offer any managed Kubernetes solution. Why didn’t you just go with Kubernetes from the beginning?
Collinge: Kubernetes has a lot of interesting characteristics, which we’re trying to gain. It wasn’t designed to run business logic. It wasn’t designed for running lightweight controllers. In fact, it wasn’t even designed for running controllers and CRDs. It was built for the kubelet to provision pods, and it’s just been extended, and people have repurposed it for these new use cases, because they like the extensibility of the API. When we wanted to build Conductor initially, we had jobs that were literally just generating YAML and writing them to like a file in S3 or in GCS. You think about all of the plumbing that goes into writing a Kubernetes controller for it to just do a simple job of generating some YAML and stick it in a file, you start thinking of all the overhead that you’re buying into with Kubernetes. Basically, it came down to what I said at one point, which is, if you limit the solution space to Kubernetes, Kubernetes has lots of constraints, and you start limiting yourself more. If you just expose that and just think about the more founding principles, I think you’ve got a lot more flexibility to explore other options. Like I said, you could. We couldn’t, because we needed to be cloud agnostic. You could build all this on serverless, for sure, and it would be a lot simpler than some of the stuff that I’ve talked about, but we didn’t have that luxury.
Participant 3: The way I understood this is basically that you created this Kubernetes like API to a bit go around the specificities that the different Kubernetes and the different cloud providers may have. Like Kubernetes in AKS is not one in AKS, and like on Azure and AWS, you may have some differences. Now, like for a data platform team that would need to build some service in a given cloud provider, let’s say you build something on AWS and you want to build some kind of well interfaced services, would you now take that road of building a “simple” API with a controller on the back and deal with that yourself. Or would you, in this more constrained context of one cloud provider, pick one of AKS, or one of the provided Kubernetes and build a controller on top of it?
Collinge: I think this touches a little bit more on the platform engineering side of things. It is a bit muddy and a bit vague, which is that we didn’t have a platform team. We were three engineers, so to think about platform engineering is a bit nonsensical. You can build a cloud without using all of these cloud principles, to actually provision your infrastructure internally. If you do want to get into the world of platform engineering, then on the infrastructure side, I would definitely not custom build stuff, basically. For provisioning your services, for provisioning like data platform teams and all that stuff, I would stick to Kubernetes and traditional workloads and integrations, and use everything off the shelf as I could, and tools. The reason we built this cloud is for serving our end users efficiently and giving the experience we wanted to our end users, but all they’re doing is provisioning resources. They’re not building platforms on top of our cloud.
Participant 3: I also think you are probably doing some platform engineering for it, but as a SaaS. It’s fairly similar, but indeed the fact that you have a product and everything on top of that makes some kind of customization worthy.
Collinge: The closest thing is like Upbound, I think, to building a system like this as a SaaS, like a full SaaS cloud as a service thing, but they are still very infrastructure focused. I think that there probably is an opportunity to build cloud as a service thing which is a bit more flexible and supports more lightweight business logic, because you might just want to create an API key. Why do you need all this logic to create an API key?
See more presentations with transcripts
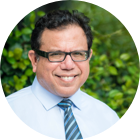
MMS • RSS
Posted on mongodb google news. Visit mongodb google news
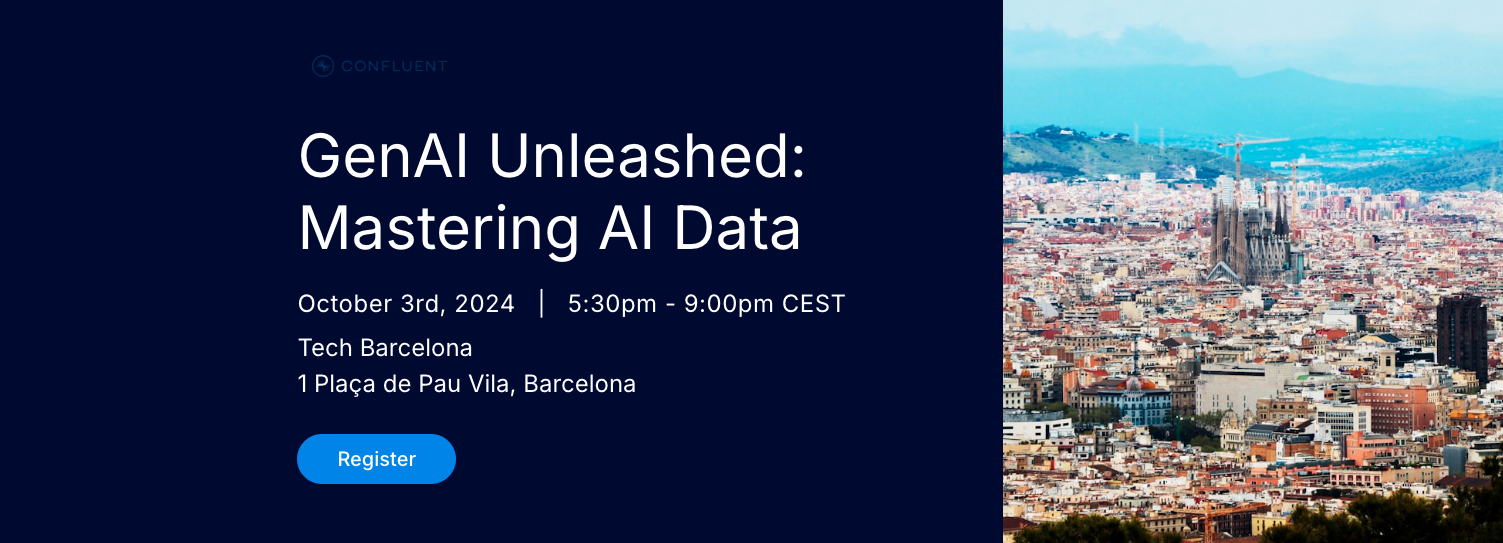
The foundation of a thriving, #AI-driven business is no longer static, outdated data architectures. Today, the future belongs to those who build a dynamic real-time data framework.
On October 3, join MongoDB, Confluent, and Amazon Web Services in Barcelona for a roundtable discussion and happy hour where you will learn how to unlock new #genAI opportunities.
This event is aimed at architects, software developers and engineers from enterprise-level & Digital Native companies that love to leverage cutting edge technologies to build amazing AI powered applications”.
Article originally posted on mongodb google news. Visit mongodb google news
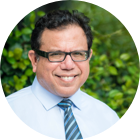
MMS • RSS
Posted on mongodb google news. Visit mongodb google news
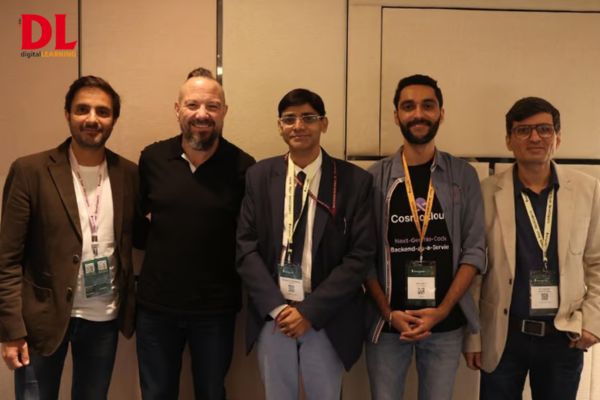
MongoDB has joined forces with the All-India Council for Technical Education (AICTE) under the Ministry of Education to upskill 500,000 students across India. This partnership, part of MongoDB’s “MongoDB for Academia” initiative, aims to provide in-demand full-stack development skills to students through a 60-hour virtual internship. This training includes experiential learning, boot camps, project work, and exposure to corporate-style environments.
The collaboration is backed by SmartBridge’s SmartInternz platform, offering over 150,000 students access to virtual internships and hands-on experience with MongoDB Atlas, a leading multi-cloud developer data platform. Launched in September 2023, the program provides educators with curriculum resources, students with free credits to use MongoDB tools, and certifications to jump-start careers in technology.
To further expand its reach, MongoDB has also partnered with GeeksforGeeks, a well-known computer science learning platform in India. This collaboration will offer MongoDB’s Developer Learning Path to 25 million GeeksforGeeks users, reaching over 100,000 aspiring developers through both online and offline centres.
Dr Buddha Chandrasekhar, CEO of Anuvadini AI and Chief Coordinating Officer at AICTE, emphasised that the ongoing wave of AI and modern technologies presents India with vast opportunities. He highlighted the importance of equipping developers with the right skills to capitalise on this potential.
Also Read: Literacy Extends Beyond Basic Skills Of Reading & Writing
MongoDB has already made substantial strides in India, with over 200 partnerships across educational institutions, training more than 100,000 students, and completing over 450,000 hours of learning. According to Sachin Chawla, Area Vice President of MongoDB India, the initiative is a testament to MongoDB’s commitment to nurturing the next generation of tech talent in the country.
This initiative positions MongoDB and AICTE as key contributors to India’s tech education ecosystem, offering unparalleled resources to students eager to develop cutting-edge skills.
Be a part of Elets Collaborative Initiatives. Join Us for Upcoming Events and explore business opportunities. Like us on Facebook , connect with us on LinkedIn and follow us on Twitter , Instagram.
Article originally posted on mongodb google news. Visit mongodb google news
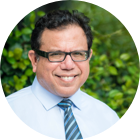
MMS • RSS
Posted on mongodb google news. Visit mongodb google news
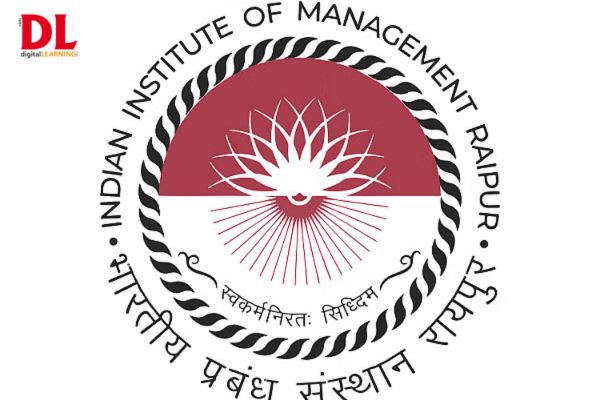
IIM Raipur is set to launch its much-anticipated Management Development Programs (MDPs) on September 20, 2024, offering professionals a unique opportunity to upgrade their managerial skills and advance their careers. Running for just over a month until October 23, 2024, these programs are tailored to meet the needs of professionals across industries, helping them navigate today’s complex business environment.
The Indian Institute of Management (IIM) Raipur has designed six Management Development Programs that cover essential subjects, including Healthcare Management, Business Analytics, and Public-Private Partnerships in General Management. These courses aim to equip professionals with the expertise needed to drive growth and efficiency within their organisations.
According to IIM Raipur, the MDPs also include specialised training in Project Appraisal, Financing, and Project Management, as well as Financial Risk Management. Participants will also explore Innovation and Technology Management within the Strategic Management domain, ensuring they gain cutting-edge insights into managing modern business challenges.
Also Read: MongoDB and AICTE Partner to Upskill 500,000 Indian Students.
The curriculum employs a range of practical learning methodologies, such as:
- Case studies, simulations, and role-playing exercises
- Critical incident techniques and In-Basket exercises
- Interactive group discussions and program-specific assignments
- Classroom lectures led by expert faculty
IIM Raipur’s MDPs are designed to offer professionals hands-on experience with real-world scenarios, enabling them to apply contemporary strategies and tools to manage their organisations effectively. Whether you’re looking to specialise in finance, project management, or strategic management, these programs provide the advanced knowledge needed to excel in today’s fast-changing business landscape.
Be a part of Elets Collaborative Initiatives. Join Us for Upcoming Events and explore business opportunities. Like us on Facebook , connect with us on LinkedIn and follow us on Twitter , Instagram.
Article originally posted on mongodb google news. Visit mongodb google news
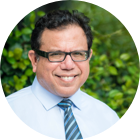
MMS • Ben Linders
Article originally posted on InfoQ. Visit InfoQ
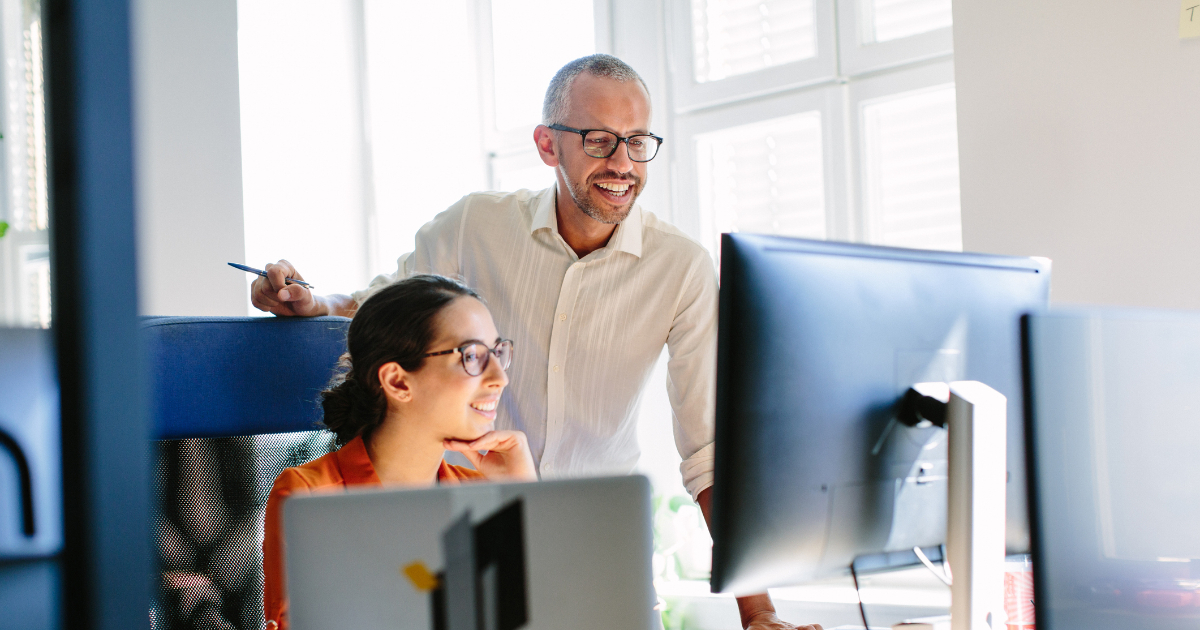
Resolving impediments to flow and removing unnecessary sources of cognitive load can make culture issues disappear in organisations, Nigel Kersten argued. Start with a clear strategy that is easy to communicate, then follow the path to creating stream-aligned teams and platform teams, he suggested.
Nigel Kersten spoke about enabling fast flow in software organizations at FlowCon France 2024.
For a long time, DevOps was useful just to make it clear that you wanted to change the traditional relationship between the teams that built software, and the teams that were responsible for maintaining and running that software, and that you wanted to implement more sophisticated automation, however Kersten says he doesn’t believe that’s the case anymore.
He advised not to focus on “fostering culture” or “fostering DevOps” as a primary goal. Instead, focus on improving fast flow and low cognitive load for your teams involved in software delivery. As you resolve impediments to flow and unnecessary sources of cognitive load, then the “cultural” issues will start to disappear, he argued:
Delivery will become increasingly more predictable, friction between teams will be reduced, and teams will have more time to invest in new skills, automating processes, and implementing self-service interfaces, all of which will continue to free up more time to create an environment of continuous improvement.
Technological improvements like containers, VMs, infrastructure-as-code, software-defined-networking, collaborative version control, and CI/CD can make it possible to fix cultural issues around organisational dynamics and bad product delivery, as Kersten explained in how technology can drive culture change in software organisations.
One of the primary drivers of the early DevOps movement was a complete lack of alignment between the people who built software, and the people who were responsible for running it in production, so we all spent a lot of time focusing on ways to increase collaboration in order to improve software delivery, Kersten said. This was great, but over time collaboration itself started to become the goal, and that’s just not a productive way to run a software organisation, he added.
Kersten referred to Team Topologies and the increased emphasis on platform engineering that we’ve seen emerge over the last few years, which both recognise that collaborative interactions are not universally appropriate:
Collaboration between functions like development and security is great at the design phase for a new product, but the goal should be to move towards X-as-a-service interactions and minimise collaboration over time.
Before you start hunting around for an internal developer platform solution, or reorganising people into stream-aligned teams, make sure that you have a clearly defined strategy that is easy to communicate, Kersten explained:
First, this is more important to get right than your organisation structure and platform team adoption, and secondly, it’s necessary in order to define the right value streams, decide whether you should have a platform team, and define the high-level goals for success.
Kersten referred to the book Good Strategy, Bad Strategy and the model used to define a strategy: a diagnosis of the situation, some guiding principles for addressing the situation, and a set of concrete actions. Strategy without an action plan is just a vision statement, he said.
If you’ve got your strategy and actions in place, then the path to creating stream-aligned teams and platform teams will be reasonably obvious, Kersten concluded. He suggested focusing on creating and amplifying feedback loops, both internal, and external:
Ask yourself: are your developers able to experiment quickly, and share those experiments easily with colleagues and stakeholders? Are your stream-aligned teams aware of upcoming changes to the platform itself? Does your platform team treat their stream-aligned teams as customers? Do they do product discovery and user research to guide their roadmap?
If the answer to these questions is no, then focus on removing whatever barriers are in the way of a fast feedback loop and user outcome-driven culture, Kersten concluded.
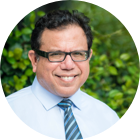
MMS • RSS
Posted on mongodb google news. Visit mongodb google news
Headlands Technologies LLC acquired a new position in shares of MongoDB, Inc. (NASDAQ:MDB – Free Report) during the 2nd quarter, according to its most recent disclosure with the SEC. The institutional investor acquired 2,488 shares of the company’s stock, valued at approximately $622,000.
Other hedge funds have also modified their holdings of the company. Vanguard Group Inc. raised its holdings in MongoDB by 2.9% during the 4th quarter. Vanguard Group Inc. now owns 6,842,413 shares of the company’s stock valued at $2,797,521,000 after buying an additional 194,148 shares during the period. Atalanta Sosnoff Capital LLC raised its holdings in MongoDB by 24.7% during the 4th quarter. Atalanta Sosnoff Capital LLC now owns 54,311 shares of the company’s stock valued at $22,205,000 after buying an additional 10,753 shares during the period. Fiera Capital Corp raised its holdings in MongoDB by 0.8% during the 4th quarter. Fiera Capital Corp now owns 224,293 shares of the company’s stock valued at $91,702,000 after buying an additional 1,695 shares during the period. Artisan Partners Limited Partnership purchased a new stake in MongoDB in the fourth quarter worth $10,545,000. Finally, Prudential PLC increased its stake in MongoDB by 2.4% in the fourth quarter. Prudential PLC now owns 21,169 shares of the company’s stock worth $8,655,000 after purchasing an additional 489 shares during the period. 89.29% of the stock is owned by hedge funds and other institutional investors.
MongoDB Stock Down 0.5 %
NASDAQ MDB traded down $1.51 during trading on Thursday, hitting $295.18. The company’s stock had a trading volume of 922,180 shares, compared to its average volume of 1,503,565. The company has a debt-to-equity ratio of 0.84, a current ratio of 5.03 and a quick ratio of 5.03. The company has a market cap of $21.80 billion, a PE ratio of -105.67 and a beta of 1.15. The business’s 50 day simple moving average is $254.78 and its 200-day simple moving average is $305.40. MongoDB, Inc. has a fifty-two week low of $212.74 and a fifty-two week high of $509.62.
MongoDB (NASDAQ:MDB – Get Free Report) last issued its quarterly earnings results on Thursday, August 29th. The company reported $0.70 earnings per share for the quarter, topping the consensus estimate of $0.49 by $0.21. The business had revenue of $478.11 million during the quarter, compared to the consensus estimate of $465.03 million. MongoDB had a negative return on equity of 15.06% and a negative net margin of 12.08%. The company’s quarterly revenue was up 12.8% on a year-over-year basis. During the same quarter last year, the company earned ($0.63) earnings per share. On average, equities analysts anticipate that MongoDB, Inc. will post -2.46 EPS for the current fiscal year.
Wall Street Analysts Forecast Growth
A number of analysts have weighed in on the company. UBS Group increased their target price on MongoDB from $250.00 to $275.00 and gave the stock a “neutral” rating in a research note on Friday, August 30th. Royal Bank of Canada reiterated an “outperform” rating and issued a $350.00 target price on shares of MongoDB in a research note on Friday, August 30th. Oppenheimer increased their target price on MongoDB from $300.00 to $350.00 and gave the stock an “outperform” rating in a research note on Friday, August 30th. Morgan Stanley increased their target price on MongoDB from $320.00 to $340.00 and gave the stock an “overweight” rating in a research note on Friday, August 30th. Finally, Robert W. Baird dropped their price target on MongoDB from $450.00 to $305.00 and set an “outperform” rating on the stock in a research report on Friday, May 31st. One investment analyst has rated the stock with a sell rating, five have given a hold rating and twenty have assigned a buy rating to the stock. According to data from MarketBeat, the stock currently has an average rating of “Moderate Buy” and a consensus price target of $337.56.
View Our Latest Stock Report on MongoDB
Insiders Place Their Bets
In other news, CRO Cedric Pech sold 273 shares of the firm’s stock in a transaction dated Tuesday, July 2nd. The stock was sold at an average price of $265.29, for a total value of $72,424.17. Following the completion of the transaction, the executive now directly owns 35,719 shares in the company, valued at $9,475,893.51. The sale was disclosed in a document filed with the SEC, which is available at the SEC website. In other news, CRO Cedric Pech sold 273 shares of the firm’s stock in a transaction dated Tuesday, July 2nd. The stock was sold at an average price of $265.29, for a total value of $72,424.17. Following the completion of the transaction, the executive now directly owns 35,719 shares in the company, valued at $9,475,893.51. The sale was disclosed in a document filed with the SEC, which is available at the SEC website. Also, CAO Thomas Bull sold 138 shares of MongoDB stock in a transaction that occurred on Tuesday, July 2nd. The shares were sold at an average price of $265.29, for a total value of $36,610.02. Following the transaction, the chief accounting officer now owns 17,222 shares of the company’s stock, valued at $4,568,824.38. The disclosure for this sale can be found here. Over the last 90 days, insiders sold 33,179 shares of company stock valued at $8,346,169. 3.60% of the stock is currently owned by corporate insiders.
MongoDB Company Profile
MongoDB, Inc, together with its subsidiaries, provides general purpose database platform worldwide. The company provides MongoDB Atlas, a hosted multi-cloud database-as-a-service solution; MongoDB Enterprise Advanced, a commercial database server for enterprise customers to run in the cloud, on-premises, or in a hybrid environment; and Community Server, a free-to-download version of its database, which includes the functionality that developers need to get started with MongoDB.
Read More
Before you consider MongoDB, you’ll want to hear this.
MarketBeat keeps track of Wall Street’s top-rated and best performing research analysts and the stocks they recommend to their clients on a daily basis. MarketBeat has identified the five stocks that top analysts are quietly whispering to their clients to buy now before the broader market catches on… and MongoDB wasn’t on the list.
While MongoDB currently has a “Moderate Buy” rating among analysts, top-rated analysts believe these five stocks are better buys.
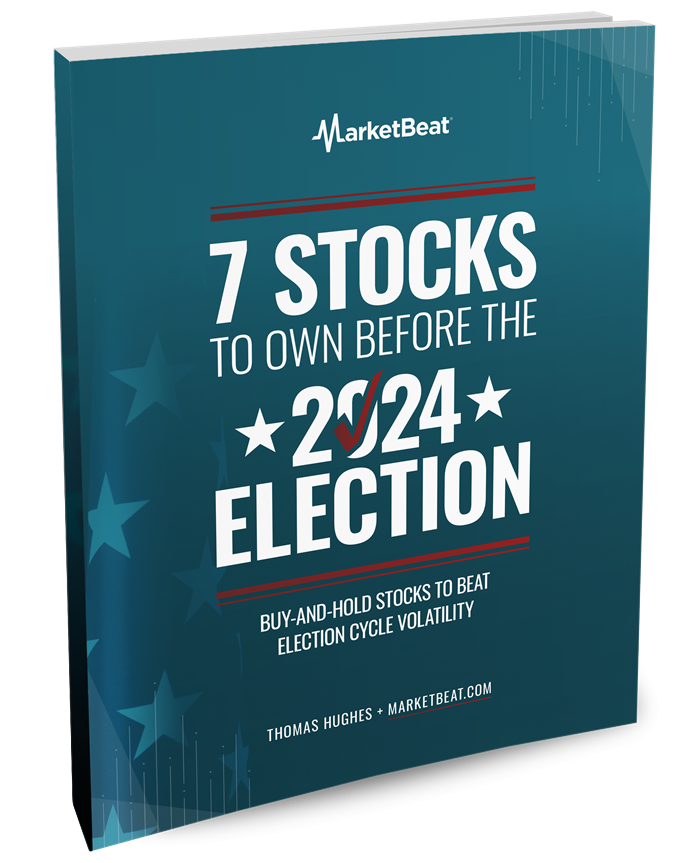
Looking to avoid the hassle of mudslinging, volatility, and uncertainty? You’d need to be out of the market, which isn’t viable. So where should investors put their money? Find out with this report.
Article originally posted on mongodb google news. Visit mongodb google news
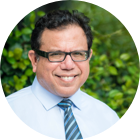
MMS • RSS
Posted on mongodb google news. Visit mongodb google news
National Bank of Canada FI reduced its stake in MongoDB, Inc. (NASDAQ:MDB – Free Report) by 72.7% during the second quarter, according to its most recent 13F filing with the Securities and Exchange Commission (SEC). The institutional investor owned 7,243 shares of the company’s stock after selling 19,254 shares during the period. National Bank of Canada FI’s holdings in MongoDB were worth $1,909,000 at the end of the most recent reporting period.
A number of other hedge funds have also added to or reduced their stakes in MDB. Norges Bank bought a new position in shares of MongoDB during the fourth quarter valued at approximately $326,237,000. Jennison Associates LLC boosted its stake in shares of MongoDB by 14.3% during the first quarter. Jennison Associates LLC now owns 4,408,424 shares of the company’s stock valued at $1,581,037,000 after purchasing an additional 551,567 shares in the last quarter. Swedbank AB boosted its stake in shares of MongoDB by 156.3% during the second quarter. Swedbank AB now owns 656,993 shares of the company’s stock valued at $164,222,000 after purchasing an additional 400,705 shares in the last quarter. Axiom Investors LLC DE bought a new position in shares of MongoDB during the fourth quarter valued at approximately $153,990,000. Finally, Clearbridge Investments LLC boosted its stake in shares of MongoDB by 109.0% during the first quarter. Clearbridge Investments LLC now owns 445,084 shares of the company’s stock valued at $159,625,000 after purchasing an additional 232,101 shares in the last quarter. 89.29% of the stock is currently owned by institutional investors.
Wall Street Analyst Weigh In
A number of equities analysts have recently issued reports on MDB shares. Scotiabank increased their price objective on shares of MongoDB from $250.00 to $295.00 and gave the company a “sector perform” rating in a research note on Friday, August 30th. Robert W. Baird dropped their price objective on shares of MongoDB from $450.00 to $305.00 and set an “outperform” rating on the stock in a research note on Friday, May 31st. Needham & Company LLC increased their price objective on shares of MongoDB from $290.00 to $335.00 and gave the company a “buy” rating in a research note on Friday, August 30th. UBS Group raised their target price on shares of MongoDB from $250.00 to $275.00 and gave the company a “neutral” rating in a research note on Friday, August 30th. Finally, JMP Securities restated a “market outperform” rating and issued a $380.00 target price on shares of MongoDB in a research note on Friday, August 30th. One investment analyst has rated the stock with a sell rating, five have given a hold rating and twenty have assigned a buy rating to the company’s stock. Based on data from MarketBeat.com, MongoDB currently has a consensus rating of “Moderate Buy” and a consensus price target of $337.56.
Get Our Latest Stock Report on MDB
Insider Buying and Selling at MongoDB
In other news, Director John Dennis Mcmahon sold 10,000 shares of MongoDB stock in a transaction dated Monday, June 24th. The shares were sold at an average price of $228.00, for a total value of $2,280,000.00. Following the sale, the director now owns 20,020 shares in the company, valued at $4,564,560. The sale was disclosed in a document filed with the SEC, which can be accessed through the SEC website. In other news, Director John Dennis Mcmahon sold 10,000 shares of MongoDB stock in a transaction dated Monday, June 24th. The shares were sold at an average price of $228.00, for a total value of $2,280,000.00. Following the sale, the director now owns 20,020 shares in the company, valued at $4,564,560. The sale was disclosed in a document filed with the SEC, which can be accessed through the SEC website. Also, Director Dwight A. Merriman sold 1,000 shares of MongoDB stock in a transaction dated Thursday, June 27th. The shares were sold at an average price of $245.00, for a total transaction of $245,000.00. Following the completion of the sale, the director now owns 1,146,003 shares in the company, valued at approximately $280,770,735. The disclosure for this sale can be found here. Over the last quarter, insiders sold 33,179 shares of company stock valued at $8,346,169. Corporate insiders own 3.60% of the company’s stock.
MongoDB Trading Up 1.8 %
Shares of MongoDB stock opened at $296.69 on Thursday. The company has a debt-to-equity ratio of 0.84, a current ratio of 5.03 and a quick ratio of 5.03. The firm has a fifty day moving average price of $254.78 and a 200 day moving average price of $305.40. MongoDB, Inc. has a 1 year low of $212.74 and a 1 year high of $509.62. The stock has a market cap of $21.92 billion, a PE ratio of -105.58 and a beta of 1.15.
MongoDB (NASDAQ:MDB – Get Free Report) last issued its earnings results on Thursday, August 29th. The company reported $0.70 earnings per share (EPS) for the quarter, topping the consensus estimate of $0.49 by $0.21. MongoDB had a negative net margin of 12.08% and a negative return on equity of 15.06%. The firm had revenue of $478.11 million during the quarter, compared to analysts’ expectations of $465.03 million. During the same quarter last year, the company posted ($0.63) earnings per share. The firm’s revenue was up 12.8% compared to the same quarter last year. Equities research analysts predict that MongoDB, Inc. will post -2.46 earnings per share for the current year.
MongoDB Profile
MongoDB, Inc, together with its subsidiaries, provides general purpose database platform worldwide. The company provides MongoDB Atlas, a hosted multi-cloud database-as-a-service solution; MongoDB Enterprise Advanced, a commercial database server for enterprise customers to run in the cloud, on-premises, or in a hybrid environment; and Community Server, a free-to-download version of its database, which includes the functionality that developers need to get started with MongoDB.
Further Reading
Want to see what other hedge funds are holding MDB? Visit HoldingsChannel.com to get the latest 13F filings and insider trades for MongoDB, Inc. (NASDAQ:MDB – Free Report).
Receive News & Ratings for MongoDB Daily – Enter your email address below to receive a concise daily summary of the latest news and analysts’ ratings for MongoDB and related companies with MarketBeat.com’s FREE daily email newsletter.
Article originally posted on mongodb google news. Visit mongodb google news
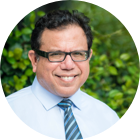
MMS • RSS
Posted on mongodb google news. Visit mongodb google news
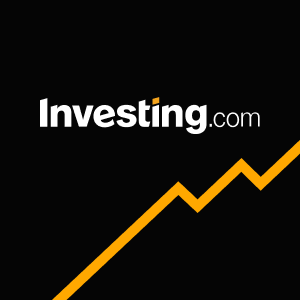
The transaction was executed under a Rule 10b5-1 trading plan, which allows company insiders to set up a predetermined plan to sell stocks at a specific time. This can help executives avoid accusations of insider trading by selling shares at predetermined times and prices, regardless of any non-public information they may have.
Following the sale, Thomas still holds a significant number of shares in the company, with 16,222 shares of MongoDB’s Class A Common Stock remaining in his possession. This indicates a continued investment in the company’s future despite the recent sale.
MongoDB has not made any additional comments regarding the transaction. Investors often monitor insider sales as they may provide insights into an executive’s view of the company’s current valuation or potential future performance. However, it is also not uncommon for executives to sell shares for personal financial reasons that are not related to their outlook on the company.
As with any insider transaction, investors are encouraged to consider the context of the sale and to look at the company’s performance and potential as part of their broader investment strategy.
In other recent news, MongoDB has been the subject of several positive analyst reviews following its strong second quarter performance. DA Davidson, KeyBanc Capital Markets, and Oppenheimer have all raised their price targets for MongoDB, reflecting the company’s robust performance and business stabilization. MongoDB’s Q2 results showcased a 13% year-over-year revenue increase, totaling $478 million, largely driven by the success of its Atlas (NYSE:ATCO) and Enterprise Advanced offerings. The company added more than 1,500 new customers during the quarter, bringing its total customer base to over 50,700. Looking ahead, MongoDB’s management anticipates Q3 revenue to be between $493 million to $497 million, with full fiscal year 2025 revenue projected to be between $1.92 billion to $1.93 billion. These projections are based on MongoDB’s recent performance and the analyst firms’ expectations. The company’s updated guidance for fiscal year 2025 was also a contributing factor to the analysts’ revised outlook. Despite management not adjusting their consumption growth forecast for the second half of fiscal year 2025, the analysts suggest that a repeat of the second fiscal quarter’s consumption growth could lead to further upside.
MongoDB, Inc.’s (NASDAQ:MDB) recent insider stock sale by Chief Accounting Officer Bull Thomas has drawn attention to the company’s financial health and future prospects. The InvestingPro platform provides in-depth analysis and metrics that can offer additional insight into MongoDB’s position in the market.
One of the key InvestingPro Tips for MongoDB is the company’s strong liquidity position, as it holds more cash than debt on its balance sheet. This is a positive sign for investors, indicating that the company has a solid financial foundation and resilience in managing its capital structure. Additionally, analysts have revised their earnings upwards for the upcoming period, which suggests optimism about MongoDB’s earning potential in the near future.
From the real-time data available on InvestingPro, MongoDB has a market capitalization of $21.95 billion, reflecting its significant presence in the industry. Despite a negative P/E ratio of -97.93, which indicates that the company is not currently profitable, there is an expectation of growth, with a revenue increase of 22.37% over the last twelve months as of Q1 2023.
Investors may also note the company’s price performance, with a strong return over the last month of 24.09% and over the last three months of 30.25%. This recent uptick could be a signal to some investors of potential momentum, despite the past year’s overall negative return of -26.07%.
For those interested in further insights and tips, InvestingPro offers additional analysis and metrics for MongoDB, which can be found at: https://www.investing.com/pro/MDB. There are currently 12 more InvestingPro Tips available that provide a comprehensive view of the company’s financial health and market performance.
This article was generated with the support of AI and reviewed by an editor. For more information see our T&C.
Article originally posted on mongodb google news. Visit mongodb google news
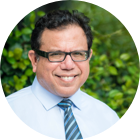
MMS • RSS
Posted on mongodb google news. Visit mongodb google news
MongoDB, Inc. (NASDAQ:MDB – Get Free Report) CAO Thomas Bull sold 1,000 shares of the stock in a transaction dated Monday, September 9th. The stock was sold at an average price of $282.89, for a total value of $282,890.00. Following the sale, the chief accounting officer now owns 16,222 shares of the company’s stock, valued at approximately $4,589,041.58. The transaction was disclosed in a document filed with the Securities & Exchange Commission, which is available at this link.
Thomas Bull also recently made the following trade(s):
- On Tuesday, July 2nd, Thomas Bull sold 138 shares of MongoDB stock. The stock was sold at an average price of $265.29, for a total value of $36,610.02.
MongoDB Trading Up 1.8 %
Shares of NASDAQ:MDB traded up $5.20 on Wednesday, hitting $296.69. 1,123,702 shares of the company’s stock were exchanged, compared to its average volume of 1,506,879. The business’s 50 day simple moving average is $254.14 and its two-hundred day simple moving average is $305.46. The company has a debt-to-equity ratio of 0.84, a current ratio of 5.03 and a quick ratio of 5.03. The stock has a market capitalization of $21.92 billion, a price-to-earnings ratio of -103.73 and a beta of 1.15. MongoDB, Inc. has a 12 month low of $212.74 and a 12 month high of $509.62.
MongoDB (NASDAQ:MDB – Get Free Report) last released its quarterly earnings data on Thursday, August 29th. The company reported $0.70 earnings per share (EPS) for the quarter, beating the consensus estimate of $0.49 by $0.21. The company had revenue of $478.11 million during the quarter, compared to analysts’ expectations of $465.03 million. MongoDB had a negative net margin of 12.08% and a negative return on equity of 15.06%. The firm’s quarterly revenue was up 12.8% on a year-over-year basis. During the same period in the previous year, the company earned ($0.63) EPS. Analysts anticipate that MongoDB, Inc. will post -2.46 EPS for the current fiscal year.
Analyst Upgrades and Downgrades
Several research firms have recently commented on MDB. Royal Bank of Canada reissued an “outperform” rating and issued a $350.00 target price on shares of MongoDB in a report on Friday, August 30th. Loop Capital dropped their price objective on shares of MongoDB from $415.00 to $315.00 and set a “buy” rating for the company in a report on Friday, May 31st. DA Davidson lifted their target price on MongoDB from $265.00 to $330.00 and gave the stock a “buy” rating in a report on Friday, August 30th. Bank of America increased their price target on MongoDB from $300.00 to $350.00 and gave the company a “buy” rating in a research note on Friday, August 30th. Finally, Scotiabank lifted their price objective on MongoDB from $250.00 to $295.00 and gave the stock a “sector perform” rating in a research note on Friday, August 30th. One analyst has rated the stock with a sell rating, five have given a hold rating and twenty have assigned a buy rating to the company. Based on data from MarketBeat, MongoDB presently has a consensus rating of “Moderate Buy” and a consensus target price of $337.56.
Institutional Inflows and Outflows
Several institutional investors have recently bought and sold shares of MDB. Vanguard Group Inc. lifted its holdings in shares of MongoDB by 1.0% in the 1st quarter. Vanguard Group Inc. now owns 6,910,761 shares of the company’s stock worth $2,478,475,000 after buying an additional 68,348 shares during the period. Jennison Associates LLC increased its position in MongoDB by 14.3% during the 1st quarter. Jennison Associates LLC now owns 4,408,424 shares of the company’s stock valued at $1,581,037,000 after purchasing an additional 551,567 shares during the period. Norges Bank bought a new stake in shares of MongoDB in the 4th quarter valued at about $326,237,000. Swedbank AB lifted its position in shares of MongoDB by 156.3% in the 2nd quarter. Swedbank AB now owns 656,993 shares of the company’s stock worth $164,222,000 after purchasing an additional 400,705 shares during the period. Finally, Champlain Investment Partners LLC increased its holdings in MongoDB by 22.4% during the first quarter. Champlain Investment Partners LLC now owns 550,684 shares of the company’s stock valued at $197,497,000 after buying an additional 100,725 shares during the period. 89.29% of the stock is currently owned by hedge funds and other institutional investors.
MongoDB Company Profile
MongoDB, Inc, together with its subsidiaries, provides general purpose database platform worldwide. The company provides MongoDB Atlas, a hosted multi-cloud database-as-a-service solution; MongoDB Enterprise Advanced, a commercial database server for enterprise customers to run in the cloud, on-premises, or in a hybrid environment; and Community Server, a free-to-download version of its database, which includes the functionality that developers need to get started with MongoDB.
See Also
This instant news alert was generated by narrative science technology and financial data from MarketBeat in order to provide readers with the fastest and most accurate reporting. This story was reviewed by MarketBeat’s editorial team prior to publication. Please send any questions or comments about this story to contact@marketbeat.com.
Before you consider MongoDB, you’ll want to hear this.
MarketBeat keeps track of Wall Street’s top-rated and best performing research analysts and the stocks they recommend to their clients on a daily basis. MarketBeat has identified the five stocks that top analysts are quietly whispering to their clients to buy now before the broader market catches on… and MongoDB wasn’t on the list.
While MongoDB currently has a “Moderate Buy” rating among analysts, top-rated analysts believe these five stocks are better buys.
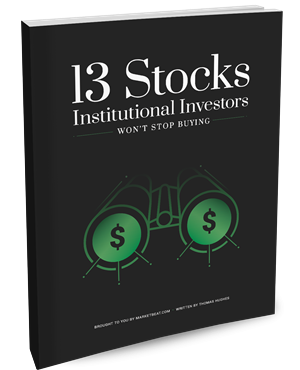
Which stocks are major institutional investors including hedge funds and endowments buying in today’s market? Click the link below and we’ll send you MarketBeat’s list of thirteen stocks that institutional investors are buying up as quickly as they can.
Article originally posted on mongodb google news. Visit mongodb google news
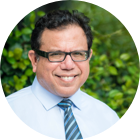
MMS • RSS
Posted on mongodb google news. Visit mongodb google news
MongoDB Inc (NASDAQ:MDB), a leading database platform provider, saw a notable insider transaction recently. Thomas Bull, the Chief Accounting Officer, sold 1,000 shares of the company on September 9, 2024. The transaction was documented in an SEC Filing. Following this sale, the insider now owns 16,222 shares of MongoDB Inc.
MongoDB Inc is known for its general purpose, document-based, distributed database built for modern application developers and for the cloud era. This technology is widely recognized for its capabilities to manage large scale databases flexibly and efficiently.
On the day of the sale, shares of MongoDB Inc were priced at $282.89, valuing the company with a market cap of approximately $21.93 billion. This valuation places the company in a significant position within the tech sector, reflecting its substantial market influence and financial stability.
The sale by Thomas Bull is part of a broader trend of insider transactions at MongoDB Inc. Over the past year, there have been 60 insider sells and no insider buys. The insider, Thomas Bull, has sold a total of 1,826 shares over the past year and has not purchased any shares.
Regarding the company’s valuation metrics, MongoDB Inc currently shows a price-to-GF Value ratio of 0.63, indicating that the stock is Significantly Undervalued according to GuruFocus’s GF Value calculation. The GF Value is a proprietary measure reflecting expected performance and historical trading multiples.
The GF Value of $450.20 suggests a potential for price increase if the market adjusts to the intrinsic value calculated by GuruFocus. This valuation is supported by historical trading multiples such as the price-earnings ratio, price-sales ratio, price-book ratio, and price-to-free cash flow, along with adjustments based on past performance and future business expectations.
This insider sale might draw attention from investors monitoring insider behaviors as indicators of company health and future stock performance. The ongoing sales by insiders, coupled with the company’s current valuation status, could influence investor sentiment and market movements related to MongoDB Inc’s shares.
This article, generated by GuruFocus, is designed to provide general insights and is not tailored financial advice. Our commentary is rooted in historical data and analyst projections, utilizing an impartial methodology, and is not intended to serve as specific investment guidance. It does not formulate a recommendation to purchase or divest any stock and does not consider individual investment objectives or financial circumstances. Our objective is to deliver long-term, fundamental data-driven analysis. Be aware that our analysis might not incorporate the most recent, price-sensitive company announcements or qualitative information. GuruFocus holds no position in the stocks mentioned herein.
This article first appeared on GuruFocus.
Article originally posted on mongodb google news. Visit mongodb google news