Month: October 2022
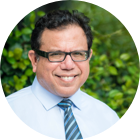
MMS • Nsikan Essien
Article originally posted on InfoQ. Visit InfoQ
AWS recently created a new template within the AWS Observability Accelerator project that provides an integrated telemetry solution for Elastic Kubernetes Service (EKS) workloads.
The AWS Observability Accelerator began as a series of infrastructure-as-code (Terraform) examples for customers to use as starting points for monitoring specific application types deployed on EKS. The project configures EKS workloads with AWS managed instances or distributions of tried and tested components of the modern observability stack: Prometheus, for scraping and storing time-series metrics; Grafana, for querying, visualisations and analysis; and OpenTelemetry, for generating, collecting and exporting telemetry data.
The newly released template provides a one-click solution that includes Prometheus and Grafana workspaces, OpenTelemetry collectors and IAM roles deployed in the architecture shown below:
Source: https://github.com/aws-observability/terraform-aws-observability-accelerator#readme
It also includes configured alerts and recording rules, resulting in a dashboard like this:
While preconfigured dashboards and alerts are a useful start for monitoring, observability of applications in environments as dynamic and complex as Kubernetes workloads require interrogatability. Jay Livens of Dynatrace writes in an exposition on observability:
In an observability scenario. . . you can flexibly explore what’s going on and quickly figure out the root cause of issues you may not have been able to anticipate.
The use of Grafana and its native integration with Prometheus for querying data gathered exposed via OpenTelemetry allows users of the AWS Observability accelerator to investigate unanticipated behaviours on their EKS workloads.
An often proposed alternative to components of the AWS Observability Accelerator would be Datadog. By deploying Datadog Agents in an EKS cluster and enabling its AWS integrations, it provides a unified platform for logs, traces and metrics which make up the three pillars of an observability solution.
According to a 2022 Gartner report on the Magic Quadrant of Application Performance Monitoring and Observability, DataDog sits as a leader in the field of Observability alongside Dynatrace. The Dynatrace offering also utilises a similar approach of an agent and integrations for routing the observability data to its Saas platform.
The AWS Observability Accelerator is maintained as an open-source project on GitHub by AWS Solutions Architects and the community.
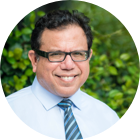
MMS • Sarah Wells Courtney Kissler Ann Lewis Nick Caldwell
Article originally posted on InfoQ. Visit InfoQ
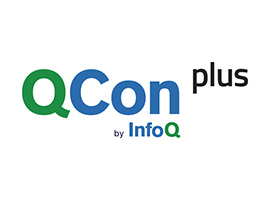
Transcript
Wells: I’m Sarah Wells. I’m a Technical Director at the Financial Times business newspaper. I’ve been there for nearly 11 years. I lead a group called engineering enablement, which is about seven different engineering teams that are building things for other engineers within the FT. Our customers are other engineers, and we are focused on trying to make sure that they can build products as quickly and easily as possible.
Caldwell: I’m VP of Engineering at Twitter, leading the consumer organization. Consumer, that’s just a fancy way to say the Twitter app, the website, and all the products that keep all the above safe. Previously was VPE at Reddit, and Chief Product Officer at Looker.
Lewis: My name is Ann Lewis. I am the Senior Advisor for technology in the Biden-Harris administration, currently embedded in the Small Business Administration government agency. I was previously the Chief Technology Officer of MUFON.
Kissler: Courtney Kissler. I’m CTO at Zulily, an online retailer. Prior to that worked at Nike, Starbucks, and Nordstrom.
Measuring Success from Improving Flow
Shoup: The title is Engineering Leadership Lessons for Improving Flow. I thought one way to start is, how do we know when we’re successful? When we improve flow, how do we measure that we’re successful.
Wells: Watching your talk, I realized that you’re really focused on the delivery. The Accelerate metrics, the DORA metrics are really relevant there. What I only just realized is that actually, at the FT, we got to the point where we were able to make small changes quite quickly a few years ago, and particularly for my part of the organization, the bit we’re most focused on is all the other stuff. If product engineering teams are having to spend a lot of time laboriously working out how to create a Lambda and connect something to something else, or to deploy a container into a Kubernetes platform or whatever, that also slows them down and stops them from making change. I’m not entirely sure where some of my metrics would be, which is why I’m interested to hear what other people think. Because once you improve some of your metrics, it’s very hard to prove that you should continue to invest. Because the metric doesn’t change. Apart from asking developers, is your life better because of the things we’ve built? I’m not entirely sure where else to go. I think, when you start the Accelerate metrics are absolutely great. If you are not releasing code as you finish it, and it going to production in hours, you’ve got a lot of benefit to gain from that. What happens after that?
Kissler: Plus 1000 to the Accelerate metrics and the DORA metrics. The one that doesn’t get touched upon as much but I think is critical to continuously understanding the health of the entire system is employee Net Promoter Score. Understanding how your teams feel. Maybe this goes to what you were saying Sarah, like we’ve done some improvements, we’ve seen the metric get better. Do we know if it’s really making a difference to our teams? I think that can be a way to really understand and learn. Because sometimes the underlying technology is important for minimizing burden and improving those metrics. There might be other things like what I have uncovered are ways of working, or something else has changed in the system and it doesn’t always become visible through those DORA metrics unless you’re also adding in employee Net Promoter Score.
Wells: It’s really interesting, because once you improve some things, people have different expectations. I was at the FT where it would take 20 minutes to build your code, and you went to production once a month. My newer colleagues at the FT are not happy when it takes them half an hour to release something. I think it is important to keep asking.
Caldwell: If you have a big enough company, or big enough team, they’ll have different metrics and different goals. Also, if you’re a platform team versus more of an edge team, it will be different. It’s all situational. There’s stuff I think that uniformly people care about when it comes to flow. If you need to invest in this, go ask your engineer about their release times, or how long it takes them to get a code review done. There are some real low hanging fruit that are measurable and can make a big impact on people’s lives. I think where it starts to get squishy, and I don’t know if anyone’s really solved this at scale, but when you start to aggregate the work of multiple developers, and then you’re trying to make measurements on team level output, it starts to get a little bit more squishy. We try and have tried to tackle this in lots of different ways. I think ultimately, when I’m thinking about flow, I’m thinking about for major, medium big rock items, what is the wall clock time from the inception of that idea to the delivery of the idea, maybe the first experiment that was run? Can we measure that? Then at the various stages in release, I go back and optimize it.
I find that sometimes people are getting tripped up on code. More often than not, people are getting tripped up on processes or maybe vestigial bureaucratic things that were meaningful at some point in the past, but no longer apply. You’re like trying to prune your process in order to increase your velocity. We don’t do that for every single project. I don’t know anyone who really has done that well, because it turns into like you’re clocking hours. You don’t want any engineer to clock hours, but you have to have some way to understand every phase of your release cycle and measure it. Then I just tie that to a few major projects and use them as exemplars for others.
Lewis: My answer would definitely have been different a year ago. Learning more about government bureaucracies, I think, in this context, where a great deal of implementation work is vendored out, and then the in-house career staff at an agency spend a lot of time trying to manage these vendors. Typically, these are folks who are guardians of huge amounts of budget and not subject matter experts in technology. I think one metric that I’ve started tracking at a high level is, can we actually implement any late breaking policy change without program disruption? Typically, a vendor will set up some agile system and then spend inordinate amounts of time trying to teach people what the user story is. They give up after a while, then they try to proceed forward. There’s some communication feedback loops between the decision makers and the implementers, if they can establish enough shared language. There’s also a concept of flow for who, so the vendor will try and manage their own internal flow that government liaisons are constantly disrupting. If you can get into a sense of shared flow, like at all, there’s any communication loop that doesn’t feel disruptive when you try and use it, then that’s a win for giant bureaucracies.
How to Get Buy-In From Engineers That Want To Re-Architect the System
Shoup: How do you get buy-in from engineers that want to re-architect the system because they believe that will make it faster?
Kissler: This is where I think leveraging a value stream map can be really powerful. Because if you do that, and you’re able to see where all the problems are, because I’ve been in this scenario where engineers say, “Just let us engineer our way out of this. We’re not moving fast enough, I know the answer. If we re-architect or we automate all the things, magic is going to happen.” In reality, what you might learn, is there are different bottlenecks in the system. It’s not always needing to move to microservices as the answer. In the case where I’ve applied value stream mapping to what many might call legacy technology, although I say that with a little bit of sarcasm, because in some cases, things get branded as legacy and they’re really not, like a mainframe. Some businesses run on a mainframe, and they’re not legacy technology. They’re a primary technology. You can apply these techniques regardless.
What you’ll learn is sometimes that it’s process related, or there’s insufficient information to go fast. You learn things that are not always just re-architecture. I’ve had engineers think that microservices is the answer. Then they go through a value stream mapping workshop, and they go, I had no idea that the real bottleneck is we’re asking for this information from our customer, and they don’t have it. Why are we asking for it? Or there’s a delay in our testing cycle, and so we need to do something different in how we’re doing unit tests, code coverage, or something. It’s not about the architecture of the system.
Caldwell: Microservices probably is not the default answer you should go. What the spirit of it is, is getting your teams to operate independently. Microservices can be a way to enable that, but can also introduce lots of other challenges that you have to account for. Then to the broader question of getting engineers to buy in to major re-architecture. I think I had actually the opposite problem, which is, engineers are always proposing some major re-architecture or wanting to spend five quarters on tech debt, or things like that. Getting them to treat those sorts of ideas and projects with the same level of strategic deliberation that we put into a product proposal, is what I tell them to do. That usually works out really well. If you can get a team to sit down and say, it’s not just like that moving off of our old web development framework is cool. Can you map to how that will enable better customer value, increase velocity. Really think through what the strategic bet would be. Either they’ll dissuade themselves of the idea or they’ll convince everybody. I’ve seen that happen multiple times. When I was at both Microsoft and Looker, we had a complete rewrite of the frontend tech stack. Reddit, the same thing, a complete rewrite of the frontend tech stack that came in part from the Eng team just explaining the velocity benefits that we would get. Think about it strategically. Sometimes there are fundamentally new technologies available like GCP, or ML is one now. They do need to be considered as strategic bets, as opposed to addressing tech debt or re-architecture.
Lewis: It’s helpful to dig into, why does the team want to re-architect? What problem are they trying to solve? Also, help everyone understand how to share ownership of the costs associated with it and make sure the cost of doing so justifies the value of whatever problem they’re trying to solve. There are a bunch of antipatterns in there. Sometimes engineers want to re-architect a system because someone else wrote it, and they want to do it their way. That’s usually not a good investment. Sometimes they do have a maintenance or sustainability or architecture problem that is worth solving. Sometimes engineers like to do that, because it’s a way of dealing with uncertainty to try and control something they actually can control, which is how a system is architected. I think it’s only generally worth it to re-architect when you need to rebalance what I like to think of as your complexity budget. You have a budget of time in your engineering capacity, budget of money of things you can spend money on. Then also, there’s always going to be some maximum amount of complexity across code bases that teams can reliably maintain. It’s easy to just build until you can only support things like 20% as well as everyone would like to. Then it’s good to resize, and that’s often a good opportunity to clean up tech debt, try out new architectures, and also get everyone aligned on why you’re doing this.
Wells: I was an engineer. This is me saying something that’s true of me. If you’ve come across the Spotify Team Health Check, the idea is you do a traffic light on a bunch of different categories, one of the categories is the health of your code base. If I had a team that was reliably green on that, I would think we were actually in trouble, because developers are never happy with the health of their code base. They always want to improve it. We talk sometimes about making sure we’re not building something in CV++. Is it there because you want it on your resume? We’ve tried two different things for this, and they’re the opposite, actually. I like Nick’s suggestion of, can you write a proposal about why we should do this, bring it to a forum. We have a pretty lightweight tech governance group, if you can bring something there and explain it, then, yes, we can endorse it. The other thing we did was having 10% time where people could scratch whatever itch, and I was leading the team. Anytime someone said to me, we don’t like Docker, we should move to Rocket. We could say, fine, do a 10% day thing on it. You could do whatever you liked, you had to present the next day on what you found. Very often people would say, it wasn’t as exciting as I thought it was going to be.
Connecting Code Quality and Delivery Speed
Shoup: Do you have any way to connect the quality of code with the speed to deliver, or are we mostly relying on the DORA metrics for that?
Kissler: It’s absolutely connected. The thing that’s awesome about the DORA metrics is that you’re not compromising quality, if you’re looking at that balance point of view. If your lead time is not good, sometimes that’s an indicator that quality is like you’re doing rework, or you’re finding defects late. The other one is the percent change failure rate. If every time you’re deploying to production, you’re having to roll it back, or you are uncovering an issue once you go to production, that’s an indicator of quality. I believe that you can use those to understand quality in addition to flow of value, and speed.
Wells: For me, the key thing is the small changes. If you’re doing lots of changes, those small changes, you can look at what actually changed and understand the difference between here’s a commit that’s going live and I can read it, versus here’s 4 weeks’ worth of work in one big release. When it goes wrong, we’re trying to work out which of those changes went wrong. It inevitably improves quality.
Lewis: Plus-plus to DORA metrics, but also overlaid with a sense of how decoupled the code base is for frontline systems are or need to be. It’s rolled up in some of the DORA metrics. One of the biggest ways to empower your teams to move faster is to make sure that their systems are decoupled in the right ways.
How Delivery Speed Is Affected by Changes in People and Process vs. Changes in Tech
Shoup: Based on your experiences, how much of the benefit of going faster is derived from changes relating to people and process and how much is derived from changes in technology?
Caldwell: Almost all of it is getting rid of red tape processes. There have been a few instances where it was tech. I’ll give you very concrete examples that they tend to be more generational changes, like the move to GCP and big data. If you’ve never deployed Kubernetes as part of your Ops system, everywhere I’ve used that has resulted in an immediate transformative step-up. Certain modern JavaScript frontend libraries, similar sorts of things. In general, if I’m going to debug a team that seems to be going slower, getting stuck in the mud. It’s things like, we’ve got a 50-page security compliance thing and there’s no easy way to checklist through it. The person whose job it used to be to steward people through that thing has left, and now they’re stuck, or things of that nature. It’s definitely awesome to have good tools and good technology, and occasionally you do get step function improvement here. If you’re talking about, what do I spend most of my time doing? It’s not that. It’s cutting red tape and helping people find a safe path through that maze of past decisions, so that they can try out a new course and create problems for some future person.
Wells: I tend to agree, it’s about the people and process, almost always, with the exception that there are certain foundational things about the technology you use. It’s not what technology. It’s, is it a decoupled architecture where people can make changes in one part of your system without affecting the other? The reason for things like that is we couldn’t do zero downtime deployments while I first worked at the Financial Times, which means you have to do deployments at a point where there is no news happening. Luckily, we’re a business newspaper, so we can do that on a Saturday quite often. That change to having an architecture where we can deploy to multiple instances sequentially, and we’re not doing big schema changes on a relational database that could take you out for several hours, that is a technology change. It does stop you from going fast. It’s probably easier to change than all of the stuff around people and process, and the fact that people think a change advisory board is essential, someone has to sign this off. The reporting, the fact that the Accelerate book says, change advisory boards don’t mean you have less failure, it just means it takes longer to fail. That stuff is really useful.
Lewis: I agree that it’s mostly people and process. Unfortunately, even though we want it to be the beautiful, and the shining quality of the code that we all write. It’s more about people being able to work within a system with enough shared language to be able to move quickly. I think in medium-sized organizations, there’s also an aspect of measuring onboarding time to new systems and trying to drive that down. Some of that is the system and some of that is the teams. Typically, teams that have established what Google calls psychological safety are faster at that for people and process reasons. Plus one to that. Government is a great example of how red tape intentionally slows processes down. If you were to try and measure that, measure the number of people who hold jobs where their only role or output they’re tracked against is controlling the throughput of other people for a bunch of reasons, some of which are good reasons. The way that bureaucracies work, sometimes there’s some strategic advantage to try and to slow some particular team and program down, however terrible that sounds. Most of the time, it makes everything slower, and it should be avoided. Everyone’s job should be to deliver on outcomes and not control other people.
Kissler: I talk about deploy on day one, which I think is related to your speed of onboarding, which sometimes will highlight technology investment required in order to achieve that. What I’ve seen that is a trap is just focus on getting a tool, figure out how to get CI/CD working, do automation, do pipeline automation, versus looking at what is it really taking for us to deploy? Where do we have opportunities? Then that typically shows, is it really a tech problem or not? If so, then we can focus on that, but leading with the people and process part, I think gets to better outcomes for sure.
Experimentation in Value Stream Mapping to Get Org Buy-In
Shoup: If an organization is hesitant to invest a lot into value stream mapping, is there a way to get the ball rolling with a smaller effort and demonstrate the goodness?
Caldwell: I think my approach is to always allow for experimentation of changes to process, tech, culture, anything you might imagine, if your company is large enough to support it. I assume that at any given moment, my culture is only 85% right, and to close the gap on the other 15%, I’d better have either a smart acquisition strategy, or just allow people within the company to experiment with different things. If they catch fire, I’ll pick them up and try and distribute them more broadly. You never want to fall into a state of being stagnant or just assuming that you’re doing such a good job you don’t need to improve. This applies to everything: management, technology, processes. All of it, you should try and disrupt yourself. You should set up situations whereby the smartest people within your organization who tend to be on the lowest levels of the org chart are put in positions of power to allow for that disruption.
Org Structure Change to Improve People and Process
Shoup: To improve the people and process, did you have to change the organizational structure, such as merging the product department with the engineering department?
Wells: Yes, you do have to change organizational structure. It’s the bingo Conway’s Law, you ship your organizational structure. That means that you have to look at where there is something, a boundary that makes the wrong split in the organization. It’s when your culture change needs to have that. Sometimes it’s big, so we’re moved away from having separate operations and development. You’re doing it everywhere, potentially. Sometimes it’s smaller. The easiest way to change culture is to change the structure of some part of your organization to do that. Recently, at the Financial Times, we moved some teams around, so that we ended up with my group being every team that focused in on engineers. Previously, some of the teams were in a group where there was some people building stuff for FT stuff on the whole. You want that focus on a customer, it’s really helped us to know what we’re trying to do.
Lewis: Wholeheartedly agree with that, especially the Conway’s Law bit. I think when you’re changing structures, you’re trying to solve for not how to take these teams and smash them together, or pull them apart again if they’re complaining about the kinds of interactions they’re having with each other, but ready to figure out how to create the right kinds of ownership. Sometimes you need product to sit closer to tech, because tech is building and prioritizing problems that affect tech and not necessarily your user base. Sometimes it’s helpful to split them apart, so that product can run ahead on user research that will be built into the next generation of your product that your tech team is not in place to be able to deliver on yet. Thinking through, how does ownership work right now? What’s working? What’s broken? What do you want to fix? I think that’s a good first step before thinking about org chart evolution.
Caldwell: I’m going to hit the Conway’s bingo square again. Conway’s Law, it tells you right up front, you will ship your org chart. You then need to think through, then do I have the right org chart? You should be continually changing your org chart to match the strategy for whatever you’re trying to deliver to the world. Embrace Conway’s Law. You can have some agility and flexibility. If you can bake that into the culture of your team, that we’re going to be continually moving around to best shape ourselves to suit whatever our business need is or whatever strategy we’re trying to pursue. If you can get people comfortable with that, you end up with a knowledge sharing, you end up with more effective ability to deliver for your customers. Lots of good things happen if you’re able to build out that culture. I also acknowledge that it’s very hard to do that. People don’t like being moved around a lot.
Kissler: I think that one of the additional critical components of structure changes is also having a system to validate that the org structure changes are actually getting you a better outcome. Because I’ve been in scenarios where organizations just say, if we just reorganize, things will be better, and sometimes don’t take that real critical lens on what is broken. What I’ve found often is, what tends to be broken is lack of alignment on shared outcomes. Even if you change your org structure, you might still need that. Because often, even when you’re trying to create as much autonomy as possible and ownership, there’s often dependencies outside of that org structure in order to get work done. If you can get to alignment on shared outcomes, and the structure to stay aligned on those, I think then orgs should be fluid, and often need to be. In the absence of having the system that also creates the right ownership model, I think it can break down and then it just becomes whiplash.
How to Deal with Services and Repos without Ownership
Shoup: Jorge asked a question about, his company went through a bunch of reorgs in the last year. Sometimes the bottleneck is collaborating in repos and services owned by another team or without any owner, and other teams might not be able to help. Any special way we can deal with that? That’s a very real problem.
Lewis: I think it helps to make it explicit, which teams are supporting frontline products and which teams are supporting other teams? Because the team supporting other teams are the ones who tend to get overloaded most quickly, and then can’t help the n+1 request or have these orphan code bases. I’d do resource planning with them and capacity planning with them and try and establish some shared language about how do the frontline teams ask the service teams for requests, and what visibility is there into when one of those teams runs out of capacity. Because typically, when that happens it sometimes means more budget, and in government, everyone’s solution is we need more resources. Then that sometimes helps the problem. Sometimes it just reinforces the problem. Just giving everyone leverage of negotiation, and turning that into a shared problem can be helpful too. Because if 10 frontline teams are all asking the same scarce resource team for something that they can’t all get, maybe it means they need something other than the request that they’re asking for.
Wells: On the subject of ownership of stuff, I think you have to get agreement that everything should be owned, and should be owned by a team. We’ve got a central registry of all of our systems, and the system is linked to a team. It might not be that that team really knows it. If their name is against it, and they’re the ones that will get called if it breaks, it makes people think, yes, that could be a problem. It’s still difficult, but at least you have someone to talk to, and at least they have a sense of I have got these 20 systems, I ought to have some idea of how they work.
Caldwell: I don’t know how you build your backlog, or how you determine what you’re going to work on for the quarter. One thing I’ve seen work for these support teams that get a lot of dependencies is to break the dependencies into two classes, like one is things that should be converted into platform features, or reusable components. Those can take a longer time to land, because in the long run they’ll enable everyone to move quickly. Then things that are more directly like, unless we hit this dependency, we won’t be able to ship some new feature within that quarter. Then if you tease those two apart, you can set clear expectations on timelines. For a long running platform component, for example, you should never have an edge team take a dependency on that work, even if they might benefit from it in the long term, because it will slow everybody down. Knowing that it is a long running dependency, you can then say, here’s a mitigating step for the short term. Then we’ll commit to picking up the conversion to the shared component or wherever it might be in the long term. This is a long-winded way of saying that I treat teams that we know are going to get a ton of dependencies during a special time period during our planning process, because that is usually where all the hiccups in terms of our predictability show up.
What’s Coming Next?
Shoup: It feels like a lot of what we’ve heard is the same over the last few years. What’s new and exciting to you that’s coming next?
Caldwell: I have a very abstract answer to it. I think collaboration tools have radically improved during COVID. We were just talking a lot about org charts and structure. If we enter in a world where our collaboration becomes order of magnitude easier, do we have to lean as much as we did previously on an org chart and managerial hierarchy? Then in correspondence to that, someone else was talking about microservice architecture, and we spend a lot of time talking about removing dependencies. In a world where the technology itself also supports more independent teams, where do we end up in the future? Are we going to start chopping levels out of the org chart? How are we going to get teams to trust each other? I think it’s a very interesting world that we’re moving toward, because flatter, less hierarchy, with better tooling should allow us all to move with better flow more quickly. We’re figuring it out in real time, like how it’s supposed to work, which is exciting, I think for managers.
Wells: It’s really interesting, because I think that the change from when I first started as an engineer, and you had basically got monolithic applications and slow release cycles, and considerably less complexity operationally, because you deployed your app onto a Tomcat server, and it had an Apache in front of it. We’ve got empowered autonomous teams, and everything seems more complicated. People have to know a lot more, and it takes a lot longer to necessarily get things going. That might just be partly where I’m working. Things got to get simpler. I don’t think everyone should have to run something like a Kubernetes platform. For most companies, that is overkill. I would like it to be really easy to write some code and just give it to someone. You look at companies like Spotify or Monzo, where they’ve got that framework, just starting to go back to engineers just being able to concentrate on writing the code. Whereas I think in the meantime, it’s got very broad. I’m going to say this, because it’s my job, working on the stuff that provides a platform and enables other engineers to the point where they don’t even have to think about it, seems to me to be something where we could invest and get a benefit for our companies.
Lewis: I’m going to go out on a limb with maybe an antagonist answer, which is that process optimization should never be exciting, especially in government, but probably in general. I think it’s exciting about this kind of engineering work at scale is the impact. Government tech, very boring, using at least 10-year-old tools, sometimes 30-year-old tools, but the agency I’m embedded within delivered a trillion dollars of economic aid into the economy at a time when we were facing economic disasters. Who cares if no one’s ever heard about Kubernetes and will never accept cloud into their heart? The impact still matters.
Kissler: My excitement is around, I truly believe that the focus on improving flow, and even today, it’s like we’re all in engineering leadership roles. My passion is, this is not an engineering or a technology problem. This is a business/company problem. Trying to bring the broader organization along for the value of improving flow is where I tend to get excited, because I think we’re getting to the point now, and some organizations are already there, where their business partners are in. I think many organizations still think that this is a problem for technology to solve. For me, the next evolution is to bring the broader company and business partners along.
See more presentations with transcripts
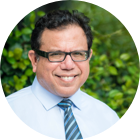
MMS • Liz Rice
Article originally posted on InfoQ. Visit InfoQ
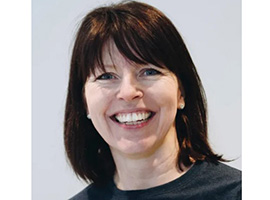
Transcript
Rice: My name is Liz Rice. I’m the Chief Open Source Officer at Isovalent. I’m also an ambassador and board member for OpenUK. Until recently, I was chair of the Technical Oversight Committee at the Cloud Native Computing Foundation. I joined Isovalent just over a year ago, because that team has so much expertise in eBPF, which is the technology that I’m talking about. I’ve been excited about eBPF for a few years now. From my CNCF work, I’ve seen some of the really incredible range of things that eBPF can enable. I want to share some of the reasons why I’m so excited about it, and specifically talk about the ways that eBPF can help us build more resilient deployments.
What is eBPF?
Before we get to that, let’s talk about what eBPF is. The acronym stands for Extended Berkeley Packet Filter. I don’t think that’s terribly helpful. What you really need to know is that eBPF allows you to run custom code in the kernel. It makes the kernel programmable. Let’s just pause for a moment and make sure we’re all on the same page about what the kernel is. Kernel is a core part of your operating system, which is divided into user space and the kernel. We typically write applications that run in user space. Whenever those applications want to interface with hardware in any way, whether they want to read or write to a file, send or receive network packets, accessing memory, all these things require privileged access that only the kernel has. User space applications have to make requests of the kernel whenever they want to do any of those things. The kernel is also looking after things like scheduling those different applications, making sure that multiple processes can run at once.
Normally, we’re writing applications that run in user space. eBPF is allowing us to write kernels that run within the kernel. We load the eBPF program into the kernel, and we attach it to an event. Whenever that event happens, it’s going to trigger the eBPF program to run. Events can be all sorts of different things. It could be the arrival of a network packet. It could be a function call being made in the kernel or in user space. It could be a trace point. It could be a perf event. There are lots of different places where we can attach eBPF programs.
eBPF Hello World
To make this a bit more concrete, I’m going to show an example here. This is going to be the Hello World of eBPF. Here is my eBPF program. The actual eBPF program are these few lines here. They’re written in C, the rest of my program is in Python. My Python code here is going to actually compile my C program into BPF format. All my eBPF program is going to do is write out some tracing here, it’s going to say hello QCon. I’m going to attach that to the event of the execve system call being made. Execve is used to run a new executable. Whenever a new executable runs, execve is what causes it to run. Every time a new executable started on my virtual machine, that’s going to cause my tracing to be printed out.
If I run this program, first of all, we should see we’re not allowed to load BPF unless we have a privilege call CAP BPF which is typically only reserved for root. We need super-user privileges to run. Let’s try that with sudo. We start seeing a lot of these trace events being written out. I’m using a cloud VM. I’m using VS Code remote to access it. That it turns out is running quite a lot of executables. In a different shell, let’s run something, let’s run ps. We can see the process ID, 1063059. Here is the trace line that was triggered by me running that ps executable. We can see in the trace output, we don’t just get the text, we’re also getting some contextual information about the event that triggered that program to run. I think that’s an important part of what eBPF is giving us. We get this contextual information that could be used to generate observability data about the events that we’re attached to.
eBPF Code Has to be Safe
When we load an eBPF program into the kernel, it is crucial that it’s safe to run. If it crashes, that would bring down the whole machine. In order to make sure that it is safe, there’s a process called verification. As we load the program into the kernel, the eBPF verifier checks that the program will run to completion. That it never dereferences a null pointer. That all the memory accessing that it will do is safe and correct. That ensures that the eBPF programs we’re running won’t bring down our machine and that they’re accessing memory correctly. Because of this verification process, sometimes eBPF is described as being a sandbox. I do want to be clear that this is a different kind of sandboxing from containerization, for example.
Dynamically Change Kernel Behavior
What eBPF is allowing us to do is run custom programs inside the kernel. By doing so, we’re changing the way that the kernel behaves. This is a real game changer. In the past, if you wanted to change the Linux kernel, it takes a long time. It requires expertise in kernel programming. If you make a change to the kernel, it then typically takes several years to get from the kernel into the different Linux distributions that we all use in production. It can be quite often five years between a new feature in the kernel arriving in your production deployments. This is why eBPF has suddenly become such a prevalent technology. As of the last year or so, almost all production environments are running Linux kernels that are new enough to have eBPF capabilities in them. That means pretty much everyone can now take advantage of eBPF and that’s why you’ve suddenly seen so many more tools using it. Of course, with eBPF, we don’t have to wait for the Linux kernel to be rolled out. If we can create a new kernel capability in an eBPF program, we can just load it into the machine. We don’t have to reboot the machine. We can just dynamically change the way that that machine behaves. We don’t even have to stop and restart the applications that are running, the changes affect the kernel immediately.
Resilience to Exploits – Dynamic Vulnerability Patching
We can use this for a number of different purposes, one of which is for dynamically patching vulnerabilities. We can use eBPF to make ourselves more resilient to exploits. One example that I like of this dynamic vulnerability patching is being resilient to packets of death. A packet of death is a packet that takes advantage of a kernel vulnerability. There have been a few of these over time where the kernel doesn’t handle a packet correctly. For example, if you put a length field into that network packet that’s incorrect, maybe the tunnel doesn’t handle it correctly and perhaps it crashes, or bad things happen. This is pretty easy to mitigate with eBPF, because we can attach an eBPF program to the event that is the arrival of a network packet. We can look at the packet, see if it is formed in the way that would exploit this vulnerability, the packet of death. Is it the packet of death? If it is, we can just discard that packet.
Example – eBPF Packet Drop
As an example of how easy this is, I’m just going to show another example of a program that will drop network packets of a particular form. In this example, I’m going to look for ping packets. That’s the protocol ICMP. I can drop them. Here’s my program. Don’t worry too much about the details here, I’m essentially just looking at the structure of the network packets. I’m identifying that I’ve found a ping packet. For now, I’m just going to allow them to carry on. XDP_PASS means just carry on doing whatever you would have done with this packet. That should emit whatever tracing you get. This is actually a container called pingbox. I’m going to start sending pings to that address and they’re being responded to. We can see the sequence number here ticking up nicely. At the moment, my eBPF program is not loaded. I’m going to run a makefile that will compile my program, clean up any previous programs attached to this network interface, and then load my program. There’s make running the compile, and then attaching to the network interface eth0 here. You see immediately it’s started tracing out, Got ICMP packet. That hasn’t affected the behavior, and my sequence numbers are still just ticking up as before.
Let’s change this to say, drop. We’ll just make that. What we should see is the tracing here is still being generated. It’s continuing to receive those ping packets. Those packets are being dropped, so they never get responded to. On this side here, the sequence numbers have stopped going up, because we’re not getting the response back. Let’s just change it back to PASS, and make it again. We should see, there’s my sequence numbers, there were 40 or so packets that were missed out, but now it’s working again. What I hope that illustrates is, first of all, how we can attach to a network interface and do things with network packets. Also, that we can dynamically change that behavior. We didn’t have to stop and start ping. We didn’t have to stop and start anything. All we were doing was changing the behavior of the kernel live. I was illustrating that as an illustration of how handling packet of death scenarios would work.
Resilience to Exploits – BPF Linux Security Module
We can be resilient to a number of other different exploits using BPF Linux security modules. You may have come across Linux security modules, such as AppArmor, or SELinux. There’s a Linux security module API in the kernel which gives us a number of different events that something like AppArmor can look at and decide whether or not that event is in or out of policy and either allow or disallow that particular behavior to go ahead. For example, allowing or disallowing file access. We can write BPF programs that attach to that same LSM API. That gives us a lot more flexibility, a lot more dynamic security policies. As an example of that, there’s an application called Tracee, that’s written by my former colleagues at Aqua, which will attach to LSM events and decide whether they are in or out of policy.
Resilience to Failure – Superfast Load Balancing
We can use eBPF to help us be resilient to exploits. What other kinds of resiliency can we enable with eBPF? One other example is load balancing. Load balancing can be used to scale requests across a number of different backend instances. We often do it not just for scaling, but also to allow for resilience to failure, high availability. We might have multiple instances so that if one of those instances fails in some way, we still have enough other instances to carry on handling that traffic. In that previous example, I showed you an eBPF program attached to a network interface, or rather, it’s attached to something called the eXpress Data Path of a network interface. eXpress Data Path is very cool, in my opinion. You may or may not have a network card that allows you to actually run the XDP program, so run the eBPF program on the hardware of your network interface card. XDP is run as close as possible to that physical arrival of a network packet. If your network interface card supports it, it can run directly on the network interface card. In that case, the kernel’s network stack would never even see that packet. It’s blazingly fast handling.
If the network card doesn’t have support for it, the kernel can run your eBPF program, again, as early as possible on receipt of that network packet. Still super-fast, because there’s no need for the packet to traverse the network stack, certainly never gets anywhere near being copied into user space memory. We can process our packets very quickly using XDP. We can make decisions like, should we redirect that packet. We can do layer-3, layer-4 load balancing in the kernel incredibly quickly, possibly not even in the kernel, possibly on a network card to decide whether or not we should pass this packet on up to the network stack and through to user space on this machine. Or perhaps we should be load balancing off to a different physical machine altogether. We can redirect packets. We can do that very fast. We can use that for load balancing.
The Kube-proxy
Let’s just briefly turn our thoughts to Kubernetes. In Kubernetes, we have a load balancer called the kube-proxy. The kube-proxy balances or allows load balancing or tells pod traffic how to reach other pods. How can a message from one pod get to another pod? It acts as a proxy service. What is a proxy if not essentially a load balancer? With eBPF we have the option not just to attach to the XDP interface close to the physical interface as possible. We also have the opportunity to attach to the socket interface, so as close to the application as possible. Applications talk to networks through the socket interface. We can attach to a message arriving from a pod and perhaps bypass the network stack because we know we want to send it to a pod on a different machine, or we can bypass the network stack and loop straight back to an application running on the same physical machine or the same virtual machine. By intercepting packets as early as possible, we can make these load balancing decisions. We can avoid having to go through the whole kernel’s network stack, and it gives us some incredible performance improvements. Kube-proxy replacement performance compared to an iptables based Kube-proxy can be dramatically quicker.
eBPF Enables Efficient Kubernetes-Aware Networking
I want to now dive a little bit more into why eBPF can enable this really efficient networking particularly in Kubernetes. In that previous diagram, I just showed the kernel network stack as one box. Networking stack is pretty complicated. Typically, a packet going through the kernel’s network stack goes through a whole bunch of different steps and stages, as the kernel decides what to do with it. In Kubernetes, we have not just the networking stack on the host, but we typically run a network namespace for every pod. Each pod, by having its own network namespace has to run its own networking stack. Imagine a packet that arrives on the physical eth0 interface. It traverses the whole kernel’s networking stack to reach the virtual Ethernet connection to the pod where it’s destined to go. Then it goes through the pod’s networking stack to reach the application via a socket. If we use eBPF, and particularly if we know about Kubernetes identities and addresses, we can bypass that stack on the host. When we receive a packet on that eth0 interface, if we already know whether that IP address is associated with a particular pod, we can essentially do a lookup and just pass that packet straight to the pod where it then goes through the pod’s networking stack, but doesn’t have to go through all the complication of everything happening on the host’s networking stack.
Using an eBPF enabled networking interface for Kubernetes like Cilium, we can enable this network stack shortcutting because we’re aware of Kubernetes identities. We know what IP addresses are associated with which pods but also which pods are associated with which services, with namespaces. With that knowledge, we can build up these service maps showing how traffic is flowing between different components within our cluster. eBPF is giving us visibility into the packet. We can see, not just the destination IP address and port, we can route through a proxy to find out what HTTP type of request it is. We can associate that flow data with Kubernetes identities.
In a Kubernetes network, IP addresses change all the time, pods come and go. An IP address one minute may mean one thing, and two minutes later, it means something completely different. IP addresses are not terribly helpful for understanding the flows within a Kubernetes cluster. Cilium can map those IP addresses to the correct pod, the correct service at any given point in time and give you much more readable information. It is measurably faster. Whether you’re using Cilium or other implementations of eBPF networking, that ability to get the networking stack on the host gives us measurable performance improvements. We can see here that the blue line on the left is the request-response rate for number of requests per second that we can achieve without any containers at all, just directly sending and receiving traffic between nodes. We can get performance that’s nearly as fast using eBPF. Those yellow and green lower bars in the middle show us what happens if we don’t use eBPF, and we use the legacy host routing approach through the host network stack, it’s measurably slower.
eBPF Network Policy Decisions
We can also take advantage of having that knowledge of Kubernetes identities and the ability to drop packets to build very efficient network policy implementations. You saw how easy it was to drop packets. Rather than just inspecting the packet and deciding that it was a ping packet, can compare the packet to policy rules and decide whether or not they should be forwarded or not. This is quite a nice tool that we have. You can find this at networkpolicy.io to visualize Kubernetes network policies. We talked about load balancing, and how we can use load balancing within a Kubernetes cluster in the form of kube-proxy. After all, Kubernetes gives us a huge amount of resiliency. If an application pod crashes, it can be recreated dynamically without any operator intervention. We can scale automatically without operator intervention.
Resilience to Failure – ClusterMesh
What about the resiliency of the cluster as a whole, if your cluster is running in a particular data center and you lose connectivity to that data center? Typically, we can use multiple clusters. I want to show how eBPF can make the connectivity between multiple clusters really very straightforward. In Cilium, we do this using a feature called ClusterMesh. With ClusterMesh, we have two Kubernetes clusters. The Cilium agent running in each cluster will read a certain amount of information about the state of other clusters in that ClusterMesh. Each cluster has its own database of configuration and state stored in etcd. We run some etcd proxy components that allow us to just find out about the multi-cluster specific information that we need, so that the Cilium agents on all the clusters can share that multi-cluster state.
What do I mean by multi-cluster state? Typically, this is going to be about creating highly available services. We might run multiple instances of a service on multiple clusters to make them highly available. With ClusterMesh, we simply mark a service as global, and that connects them together such that a pod accessing that global service can access it on its own cluster, or on a different cluster, should that be necessary. I think this is a really nice feature of Cilium, and remarkably easy to set up. If the backend pod on one cluster is destroyed for some reason, or indeed if the whole cluster goes down, we still have the ability to route requests from other pods on that cluster to backend pods on a different cluster. They can be treated as a global service.
I think I have an example of this. I have two clusters. My first cluster is up, we can see cm-1, standing for ClusterMesh 1, and a second cluster, cm-2. They are both running some pods. We quite often in Cilium do some demos with a Star Wars theme. In this case, we have some X-wing fighters that want to be able to communicate with the Rebel base. We also have some similar X-wings and Rebel bases on the second cluster. Let’s just take a look at the services. In fact, let’s describe that Rebel base, service rebel-base. You can see it’s annotated by Cilium as a global service. It’s been annotated by me as part of the configuration to say I want this to be a global service. The same is true if I look on the second cluster there. They’re both described as global. What that means is, I can issue requests from an X-wing on either cluster, and it will receive responses from a load balanced across those two different clusters, across backends on those two different clusters. Let’s try that. Let’s run it in a loop. Let’s exec into an X-wing. It doesn’t really matter which X-wing. We want to send a message to the Rebel base. Hopefully, what we should see is, we’re getting responses from sometimes it’s cluster 1, sometimes it’s cluster 2, at random.
What if something bad were to happen to the Rebel base pods on one of those clusters? Let’s see which nodes are on the code. Let’s delete the pods on cluster 2. In fact, I’ll delete the whole deployment of Rebel base on the second cluster. What we should see is that all the requests are now handled by cluster 1. Indeed, you can see, it’s been cluster 1 now for quite some time. That resiliency where we literally just have to mark our services as global, it’s an incredibly powerful way of enabling that multi-cluster high availability.
Visibility into Failures – eBPF Observability Instrumentation
Lest I give you the impression that eBPF is just about networking, and advantages in networking, let me also talk a bit about how we can use eBPF for observability. Which is, after all, incredibly important, if something does go wrong. We need observability so that we can understand what happened. In a Kubernetes cluster, we have a number of hosts, and each host has only one kernel. However many user space applications we’re running, however many containers we’re running, they’re all sharing that one kernel per host. If they’re in pods, still only one kernel however many pods there are. Whenever those applications in pods want to do anything interesting, like read or write to a file, or send or receive network traffic, whenever Kubernetes wants to create a container. Anything complicated involves the kernel. The kernel has visibility and awareness of everything interesting that’s happening across the entire host. That means if we use eBPF programs to instrument the kernel, we can be aware of everything happening on that whole host. Because we can instrument pretty much anything that’s happening in the kernel, we can use it for a wide variety of different metrics and observability tools, different kinds of tracing, they can all be built using eBPF.
As an example, this is a tool called Pixie, which is a CNCF sandbox project. It’s giving us with this flamegraph, information about what’s running across the entire cluster. It’s aggregating information from eBPF programs running on every node in the cluster to produce this overview of how CPU time is being used across the whole cluster with detail into specific functions that those applications are calling. The really fun thing about this is that you didn’t have to make any changes to your application, you don’t have to change the configuration even to get this instrumentation. Because as we saw, when you make a change in the kernel, it immediately affects whatever happens to be running on that kernel. We don’t have to restart those processes or anything.
This also has an interesting implication for what we call the sidecar model. In a lot of ways, eBPF gives us a lot more simplicity compared to the sidecar model. In the sidecar model, we have to inject a container into every pod that we want to instrument. It has to be inside the pod because that’s how one user space application can get visibility over other things that are happening in that pod. It has to share namespaces with that pod. We have to inject that sidecar into every pod. To do that, that requires some YAML be introduced into the definition of that pod. You probably don’t write that YAML by hand to inject the sidecar. It’s probably done perhaps in admission control or as part of a CI/CD process, something will likely automate the process of injecting that sidecar. Nevertheless, it has to be injected. If something goes wrong with that process, or perhaps you didn’t mark a particular pod as being something you want to instrument, if it doesn’t happen, then your instrumentation has no visibility into that pod.
On the other hand, if we use eBPF, we’re running our instrumentation within the kernel, then we don’t need to change the pod definition. We’re automatically getting that visibility from the kernel’s perspective, because the kernel can see everything that’s happening on that host. As long as we add eBPF programs onto every host, we will get that comprehensive visibility. That also means that we can be resilient to attacks. If somehow our host gets compromised, if someone manages to escape a container and get on to the host, or even if they run a separate pod somehow, your attacker is probably not going to bother instrumenting their processes and their pods with your observability tools. If your observability tools are running in the kernel, they will be seen regardless. You can’t hide from tooling that’s running in the kernel. This ability to run instrumentation without sidecars is creating some really powerful observability tools.
Resilient, Observable, Secure Deployments – Sidecarless Service Mesh
It also takes us to the idea of a sidecarless service mesh. Service mesh is there to be resilient and observable and secure. Now with eBPF, we can implement service mesh without the use of sidecars. I showed before the diagram showing how we can bypass the networking stack on the host using eBPF. We can take that another step further for service mesh. In the traditional sidecar model, we run a proxy, perhaps it’s Envoy, inside every pod that we want to be part of the service mesh. Every instance of that proxy has routing information, and every packet has to pass through that proxy. You can see on the left-hand side of this diagram, the path for network packets is pretty torturous. It’s going through essentially five instances of the networking stack. We can dramatically shortcut that with eBPF. We can’t always avoid a proxy. If we are doing something at layer-7, we need that proxy, but we can avoid having a proxy instance inside every pod. We can be much more scalable by having far fewer copies of routing information and configuration information. We can bypass so many of those networking steps through eBPF connections at the XDP layer within the networking stack, or at the socket layer. eBPF will give us service mesh that’s far less resource hungry, that’s much more efficient. I hope that’s given a flavor of some of the things that I think eBPF is enabling around networking, observability, and security, that’s going to give us far more resilient and scalable deployments.
Summary
I’ve pretty much been talking about Linux so far. It is also coming to Windows. Microsoft have been working on eBPF on Windows. They’ve been part, alongside Isovalent and a number of other companies that are interested in massively scalable networks. We’ve come together to form the eBPF Foundation, which is a foundation under the Linux Foundation, really to take care of eBPF technology across different operating systems. I hope that gives a sense of why eBPF is so important, and it’s so revolutionary for resilient deployments of software, particularly in the cloud native space, but not necessarily limited to. Regardless of whether you’re running Linux or Windows, there are eBPF tools to help you optimize those deployments and make them more resilient.
Resources
You can find more information about eBPF at the ebpf.io site, and Cilium is at cilium.io. There’s also a Slack channel that you’ll find from both of those sites, where you’ll find experts in Cilium and in eBPF. Of course, if you want to find out more about what we do at Isovalent, please visit isovalent.com.
Questions and Answers
Watt: Which companies are using Cilium in production at the moment that you’re seeing and know about?
Rice: We’ve actually got a list in the Cilium GitHub repo of users who have added themselves to the list of adopters. There are certainly dozens of them. There’s companies using it at significant scale. Bell Canada, for example, using it in telco, Adobe, Datadog, these are just a few examples of companies that I know I can speak about publicly. It’s pretty widely adopted.
Watt: It’s certainly one of the technologies on the up and coming road. I think the fact that there are already some big players in the market that are already using this, is testament I think to where it’s going.
Rice: The other two integrations to really mention, the Dataplane V2 in GKE is actually based on Cilium. Amazon chose Cilium as the networking CNI for their EKS Anywhere distribution. I feel like that’s a very strong vote of confidence in Cilium as a project and eBPF as a technology.
Watt: One of the areas we’re looking at on the track is around chaos engineering, and that side of things. How do you see eBPF potentially helping out or providing ways to do different things from a chaos engineering perspective?
Rice: I think this is something that we just touched on, about having eBPF programs running in the kernel and potentially changing events, that could be a really great way of triggering chaos tests. For example, if you wanted to drop some percentage of packets and see how your system behaved, or insert errors, all manner of disruptive things that you might want to do in chaos testing, I think eBPF could be a really interesting technology for building that on.
See more presentations with transcripts
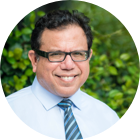
MMS • Simon Maple
Article originally posted on InfoQ. Visit InfoQ
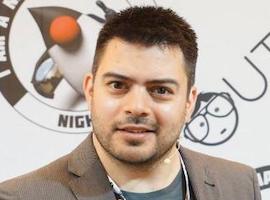
Transcript
Maple: My name is Simon Maple. I’m the Field CTO at Snyk. We’re going to be looking at the impact that Log4Shell had on us as an ecosystem, and how we can better prepare ourselves for a future Log4Shell. I’ve been in Java for 20 years plus commercially, as a developer, as dev advocacy, as community, and now as a Field CTO at Snyk, which is a developer security company creating a platform to help developers add security into their developer workflows.
Outline
We’re going to be first of all looking at the Log4Shell incident, the vulnerability that existed in the Log4j library. We’re going to extract from that the bigger problems that can impact us in future in a similar way to Log4Shell. In fact, as I am recording this, we’re literally a day into another potential Log4Shell type incident, which is potentially being called Spring4Shell, or Spring Shell, which looks like another remote code execution incident that could potentially be in the Spring ecosystem. These are the types of incidents that we are looking to better prepare ourselves for in future. Once we talk about the steps we can take to really help ourselves to mitigate that, we’re going to look at what is actually beyond the Log4Shell risk, what is beyond that open source risk that we as Java developers and Java organizations, steps we can take to first of all understand where that risk is, and what steps we can actually take to better prepare ourselves and mitigate those risks as we go.
What Is Log4Shell?
Let’s jump in, first of all with what the Log4Shell incident was, and some of the bigger problems that we really can understand and take out for future learnings. This blog post is one of the blog posts that we wrote from Brian Vermeer, on December 10th, the day that the vulnerability came out. Of course, it had to be a Friday: Friday afternoon, Friday morning, where the Java ecosystem was alerted en masse at a new critical Log4j vulnerability. This was a remote code execution vulnerability. At the time, the suggested upgrade was to version 2.15. This was the version that at the time was thought to contain the entire fix for this incident. CVSS score, which is the score that is essentially almost built from a scorecard of a number of security questions to determine what the risk is, how easy it is to break into, that was given a 10. This is out of 10, so the highest possible score for that.
Java Naming and Directory Interface (JNDI)
Let’s dig a little bit deeper into what is actually happening under the covers and how this vulnerability came about. It really starts off with a couple of things, first of all, the JNDI, and secondly, Java serialization. The JNDI, the Java Naming and Directory Interface is essentially a service that is there by default in the JDK. It allows our applications that are deployed into a JDK to access potentially locally, like we’ve done here, a number of objects that can be registered into that JNDI. I’m sure there are many Java devs that are very familiar with this already. It’s been a very core part of the JDK for many years. Now, examples here, you might make a request with a particular string that is effectively the key of an object that has been registered in the JNDI. For example, env/myDS, my data source. You might want to qualify that with a java:comp, which is similar to a namespace/env/myDS. What we would get back is the myDS Java object, which we can then use to get data from a database.
We don’t always have to look to the local JNDI to register or get these objects. What we can also do is make a request out to a remote JNDI. In this case, here’s an example of what might happen if I was to create a remote evil JNDI, which I was to stand up on one of my evil servers. My application that I’ve deployed into the JDK can make a request out specifying, in this case, the JNDI LDAP server, parsing in an evil.server URL with a port here of 11, and requesting a bad object. What I would get back is a serialized object, bad, that I could reconstruct, and I could potentially execute there. Obviously, my application isn’t going to go out and request this bad object from my evil server. What an attacker would try and do to any attack vector here for this type of attack, is to parse in something to the application, so that the application will use that input that I give it to request something of my evil JNDI server?
That’s all very well and good, but what does this have to do with Log4j? We know Log4j is a logging environment, it’s a logging library and function. Why does that yield? What’s that got to do with the JNDI? Many years ago, I think it was around 2013, a feature was added into Log4j to be able to look up certain strings, certain properties for variables and things like that, configurations from the JNDI. Very often though, if a logger sees a string, which is a JNDI like lookup string, it will automatically try and perform that lookup as part of that request while logging. As a result, there is a potential to exploit something like this by trying to log a user input, which is a JNDI string, which contains my URL with an evil input, which will pull my evil object and potentially run that. Typically, login very often happens on exception parts and error parts. What we’re going to see here is an attempt for attackers to try and drive down an exception path with a payload of the JNDI string. That JNDI string will relate to my evil object, which in this case here it’s going to perform an exec parsing and maybe some sensitive data back to my URL, and I can extract credentials and other things. This is one example of what could happen.
One of the big problems with this specific vulnerability and what made this rock the Java ecosystem so much is the prevalence of Log4j, not just in the applications that we write, but in the third party libraries that we pull in, because of course, everyone needs logging, everyone uses some logging framework. The question is, how do we know that if we’re not using it, that someone we are relying on isn’t using it as well? That’s one of the biggest problems.
What Is the Industry Exposure?
At Snyk, we noticed the number of things from the customers that use us and are scanning with us, we noticed over a third of our customers are using Log4j. We scan a number of different ecosystems. The vast majority of our Java applications had Log4j in them, but 35% of overall customers had Log4j. Interestingly, 60%, almost two-thirds of those are using it as a transitive dependency. They’re not using it directly in their applications, but the libraries, the open source third-party packages that they are using are making use of Log4j. That makes it extremely hard to work out whether you have Log4j in your application or not. Because if you ask any developer, are you using Log4j? They’ll know if they’re interacting directly most likely with Log4j. However, do they know that three levels deep there is a library that they probably don’t even know they’re using that uses Log4j? Possibly not. The industry exposure as a result is very broad, because Log4j gets pulled in, in so many different places.
The Fix
What was the fix? If we look back at what the original fix or suggested fixes were, it’s important to note that this changed very rapidly as more information came in, and that is because this was a zero-day vulnerability. The exploit was effectively widely known before the vulnerability was even disclosed. As a result, everyone was chasing their tails in terms of trying to understand the severity, the risk, how things could be attacked. As a result, there was changing mitigation strategies and changing advice, depending on literally the hour of the day that it was going through. Here’s a cheat sheet that I wrote back in December, to really suggest a number of different ways that it could be fixed.
The important thing to note is the fix was made available very soon. The strongest mitigation case here was to upgrade Log4j at the time to version 2.15. Of course, in some cases, that wasn’t possible. There are certain times where we needed to say, what are the next steps then? The vast majority of people actually had a bigger problem before saying, let me just upgrade my Log4j. The biggest problem people had here was visibility, gaining visibility of all the libraries that they are using in production, all the libraries that their application is pulling in. There are a couple of ways of doing that. Of course, there are tools that can do a lot of that on your behalf. One of the things that you could do if you’re using something like Maven or Gradle, there were certain ways of pulling that data from your builds. However, it’s hard to be able to do that from a build process up, because essentially, you need to make sure that this is being used everywhere. It’s sometimes easier to look at it from the top-down, and actually be able to scan large repositories of your application so that you can get a good understanding from the top-down of what is in your environments, what is in your Git repositories, for example.
Obviously, the upgrade path here is heavily to upgrade. I believe we’re over in 2.17 these days in terms of what the suggested fixes are. However, for those who perhaps you’re using binaries, rather than actually pulling in, in your source. I think GitHub Enterprise, for example, was using Log4j. What do you do in that case where you can’t actually have access to the source to actually perform an upgrade? In some cases, there were certain classes that you could just remove from the JDK before restarting it. When you remove those classes, the vulnerable methods, the vulnerable functions had effectively been removed. It’s impossible to get to go down those paths. However, there are, of course, operational problems with that, because if you were to go through those paths, you might get unexpected behavior. Luckily, in this case, because people were either doing JNDI lookups on purpose or not, it was a little bit more predictable. It wasn’t something that was very core functionality.
There were some other things that could be done. Some of these were later on discovered that they weren’t as effective as others. Upgrading JDK is a good example whereby a lot of people said yes, that’s what you need to do straight away. However, after a little bit of time, it was discovered that that wasn’t as effective because attackers were mutating the way that they were approaching the attack, and circumventing some of the ways in which we’re trying to fix.That really goes and points to the way, which if we were to look at it from the runtime point of view, and look at things like egress traffic, look at things like WAFs, these are very short-lived fixes that we can put out. Because the ability for an attacker to change the way that they attack your environments changes literally, by the minute, by the day. Because as you block something in your WAF, your Web Application Firewall, which essentially is trying to block traffic inbound which has certain characteristics about the way it looks, an attacker would just say, “You’ve blocked that, I’ll find another way around it.” There’s always an edge case that attackers will find that can circumvent those kinds of attacks.
The last thing was really monitoring projects, and monitoring your environments. Because with these kinds of things, all the eyes go to these projects and try and understand whether the fix was correct, whether there are other ways which you can actually achieve the remote code execution in those projects. There were a number of future fixes that had to be rolled out as a result of this Log4Shell incident. As a result, it was very important at varying risks at different times. It was very important to monitor the upgrade so that as new vulnerabilities and CVEs were released, you were getting notified. Of course, there’s an amount of tooling which Snyk and others can provide to do this, but this was typically the remediation that was available. Of course, if you’re looking to still do these remediations, be sure to check online for the latest and greatest, to make sure that the version changes are including the latest movements from these libraries.
Log4j Timeline
Looking at the timeline, what can we learn from this? Obviously, we know that it was a zero-day. If you look at the timeline of when the code that introduced the vulnerability first came in, as I mentioned, 2013, almost nine years before. It wasn’t till 2021, late November, an Alibaba security researcher approached Apache with this disclosure, and it was being done with Apache. The problem is, when you actually roll out a fix, when you actually put a fix into an open source project, people can look at that and say, why are you making these code changes? They can see what you’re essentially defending against. What this can do then is actually almost partly disclose this type of vulnerability to the ecosystem, because all of a sudden, before others can actually start adopting that latest fix, you’re essentially showing where the attack vector can be breached through or exploited. This happened on December 9th, and straightaway a PoC was published on GitHub. It was leaked on Twitter as well. We know how this goes. It snowballs. December 10th was the officially disclosed CVE. Although this was leaked on Twitter and GitHub the day before, the CVE hadn’t even been published. At this stage you look here day by day, and the poor Log4j maintainers were working day and night on understanding where future issues and things like that could be found and fixed. That’s an interesting timeline there.
December 10th, on the Friday afternoon, I’m sure everyone was probably in the incident room getting a team together. The first question, which is very often the hardest question is, are we using Log4j? Where are we using it? How close are they to my critical systems? Are we exploitable? The most common questions. Can you answer that? Were you able to answer that straightaway? Those people who could, were very often in ownership of an SBOM, a software bill of materials. Software bill of materials is inventory. It’s like a library, essentially, that itemizes all the components, all the ingredients, as it were, that you are using to construct your applications and put them into your production environments. This will list all the third party libraries, all the third party components that you’re building in. What this will allow you to do is identify, in this case, are we using the Log4j-core, for example, and its particular version? Are we using a vulnerable version of Log4j? Are we using Log4j at all anywhere? What projects are we using it in? Where in the dependency graph are we using it? Is it a direct dependency? Is it somewhere down the line? Are they fixable? These were the questions that if we had this software bill of materials, we can answer extremely quickly.
Competing Standards
An SBOM, a software bill of materials, there are actually standards for this. There’s two competing standards right now which we’re likely to keep both in our industry. One is CycloneDX, one is SPDX. Essentially, they’re just different formats. One is under OWASP, the other the Linux Foundation. CycloneDX is one which is a little bit more developer focused, in the sense of what you’ll see is tooling and things that are being created more for the open source world where they can actually start testing and really getting hands-on quicker. The SPDX project is more standards based, and so a lot of the folks from standards backgrounds will tend to resonate more along this angle. Both are reasonable standards, and we’re going to likely see various tools that are probably going to support both. These are the formats that you can expect your SBOMs to exist. How can you create an SBOM? Of course, there are many tools out there, Snyk and others. There are plenty of tools out there whereby you can scan all of your repositories. It’ll take a look at your POM XML files, your Gradle build files, create dependency graphs. It will effectively give you this software bill of materials where you can identify and list, catalog the open source libraries that you’re using.
How to Prepare Better for the Next Log4Shell Style Incident
How can we prepare ourselves then better for the next Log4Shell style incident, whether that’s Spring4Shell right now? What can we do ourselves? There’s three things, and if you take DevSecOps as a good movement here, the three core pieces of DevSecOps are people, process, and tooling. These three are the core things which we need to look at in order to improve our posture around security. People here is the most important, so let’s start with people.
The Goal: Ownership Change
Within our organizations, DevOps has changed the way that we are delivering software. I remember 20 years ago when I was working with two year release cycles, people weren’t pushing to production anywhere near as fast as they are today, which tends to be daily. As a result, the more periodic security traditional audit style was more appropriate back then, compared to today. When we’re delivering and deploying to production multiple times a day in more of a CI/CD DevOps way, we need to recognize that every change that goes to production needs to be scanned in the same way as we would for quality, unit tests or integration tests and things like that. There’s two things that need to happen there. First of all, you tend to see 100 devs, 10 DevOps folks, and 1 security person. That one security person can’t be there to audit every change, to provide information about what to fix on every single change. As a result, we need an ownership change here. Shift left is good, maybe for a waterfall style, but this is very much more an efficiency play. This is doing something earlier. What we need here is an ownership change, where we go away from this dictatorial security providing for the developer, and more to empowering developers. We want to go from top to bottom. Rather than it’s a dictatorial model, you’re really empowering everyone who’s making those changes. There are a number of things that need to alter not just our outlook here, but our processes, the tools we choose. The core thing here is the responsibility that we as developers have in this new type of model.
Developer vs. Auditor Context
While we go into responsibility, let’s take a look at the context of difference in terms of how we look at things. A developer cares about the app, that’s their context. They want to get the app working. They want to get the app shipped. They care about various aspects of the app, not just security, whereas an auditor purely cares about risk. They care about what vulnerabilities exist here. What is the risk? What is my exposure in my environment? You zoom out, and the developer, they care about availability, they care about resilience. A huge number of things way beyond security. Whereas the auditor, they then care about, where’s my data? What other services are we depending on? How are they secure? They look at the overall risk that exists beyond the app. We have very different perspectives and points of view. As a result, we need to think about things differently. Auditors or security folks audit. What they care about is doing scans, running tests, creating tickets. That’s their resolution, the creating tickets. The developers, when we want to provide and empower developers in security, their end goal is to fix. They need to resolve issues. They need solutions that they can actually push through rather than just creating tickets. As a result, our mentality and the changes we need to make need to reflect this model.
Where Could Log4shell Have Been Identified?
Let’s take a look at Log4j now, and think about where we identified this issue. Where could have this exposure been identified? We couldn’t really do anything in our pipeline here, because we’re not introducing Log4Shell here. We were already in production. This is a zero-day. This is something whereby it is affecting us today and we need to react to that. This is a zero-day where we need to react as fast as we can. Of course, there are other ways, which we will cover. At its core, we need it to be on this right-hand side.
Assess Libraries You Adopt
Other things that we can do in order to address other issues. For example, what if we were on the left-hand side, and we’re introducing a library which potentially has a known vulnerability, or we just want to assess the risk? How much can we trust that library? There are things that we can do when we’re introducing libraries to avoid that potential Log4j style zero-day going forward. This is a guide. There are obviously certain anomalies that can exist, for example, Spring being one of them, which here today potentially has this Spring4Shell issue. When you’re using a library, it’s important for your developers to ask these questions as they introduce them. Assess these libraries when you’re using them. Don’t just pull them in because they have the functionality because they’re allowing you to do what you need to do in order to push this use case through.
Check maintenance. How active is the maintenance here? Look at the commits over the last year, is it an abandoned project? Is it something whereby if an issue was found, it would be resolved pretty quickly? How many issues are there? How many pull requests are currently open? What is the speed at which they’re being resolved? How long ago was the last release? Potentially, if there’s something that it depends on, and there is a new release of a version of a transitive dependency, how long will this library take in order to perform a release consuming that latest version? The maintenance is very important to consider.
Then, of course, next, the popularity. There’s a number of reasons why this is very useful. Popularity is a very important trend, so make sure you’re not the only person using this library, but this is in fact well trusted by the ecosystem. It’s something which lots of people rely on, and you’re not by yourself in a space whereby no one else is using these kinds of libraries. This reliance on a library will very often push things like the demand for maintenance and so forth.
Thirdly, security, in terms of looking at the most popular version that this library has released that people are using, and across the version ranges, where are security issues being added? How fast are they being resolved, both in your direct dependency for that library, and the transitives as well? If a transitive has a vulnerability, how soon is that being removed? Then, finally, the community. How active is the community? How many contributors are there in the community? How likely is it that if there’s obviously, just one or two contributors, does that give an amount of risk for this being a library that could potentially be abandoned, and so forth? With all of these metrics, what we want to pull together is essentially a health score. In this case, this is an npm package, for example, called Passport. This is a free advisor service that’s on the Snyk website, but we provide a score out of 100 to give you almost like a trust metric, or at least a health metric in terms of how reliable this library might be in your environment. You can run this thing across your dependency graph almost and identify where the weak points are for your dependency graph.
Rethink Process
When we think about other places, so for example, the Log4Shell thing happened when we were in production already. Could we have taken steps to identify potential libraries that are more prone to these kinds of things? We could have done that while we were coding before we add these in. Of course, anomalies are going to happen. Log4j is one of those very popular libraries that if you go through those kinds of processes, sometimes that thing is just going to happen. Sometimes you might think it’s less likely, potentially, for there to maybe be a malicious attack on Log4j, but potentially more likely for a risk to actually be a greater magnitude on the impact it can have on the ecosystem.
Of course, the other area where we can look at is known vulnerabilities. Known vulnerabilities are essentially a vulnerability which has potentially a CVE or other vulnerability databases. They have an entry there, which basically says, this is the vulnerability, this is how severe it is. This is the library it’s in with this version range, between ultimately where it was introduced, and just before it was fixed. This is where it exists in your environment. It’s very important to be able to automate those kind of checks to see when you create your dependency graph, if the libraries that have vulnerabilities in are being introduced into your applications through your builds. This could be done at various stages. You can automate this into your Git repository, so that as you create pull requests, you can automatically test whether the delta change is introducing new issues. That might be license issues or security vulnerabilities. This is a great way of being able to, rather than look at potentially a big backlog, make sure you improve your secure development, just by looking at your future development first.
You can of course test in your CI/CD as well. Run tests in your Jenkins builds. There’s the opportunity here to block and make sure that if we get a very critical high severity vulnerability, we just don’t want to push this through. Very often, that can cause issues with a nicely, very slick, fast moving build process. You want to judge where you want to be more aggressive, where you want to be less aggressive, and more, be there for visibility, and be there to raise tickets potentially with an SLA to fix within a certain number of days. The core thing is run automation at these various points and have that awareness and that feedback to your developers as early as their IDE, with integrations and things like that.
Rethinking Tooling
One of the core things that we mentioned previously was about developer tooling and giving your development teams tools that will address what their needs for security are. That is to fix, not just to be an auditor, and to find. Here are some of the things that you need to think about when trying to work out what tooling your development teams should have. Make sure there’s a self-service model to use those tools. Make sure there’s plenty of documentation that your teams, as well as the vendor is creating for that. The Rich API, and the command line interface, and the number of integrations is core as well, as well as having a big open source community behind it. From a platform scope, there are many security acronyms, DAST, SAST, IAST, which tend to look more to your code, but think about the wider, more broader application that you’re delivering as a cloud native application security concern. Finally, the one piece that I want to stress here is this governance approach. When you’re looking at a tool, ask the question, is this tool empowering me as a developer or my developers, or is it dictating to my developers? That will help you determine whether this is a tool that should fit in your DevOps process, or whether it doesn’t fit the process or the model that you’re striving for?
Cloud Moves IT Stack into App
Finally, we’re going to talk about what’s beyond the Log4Shell risk in our applications. This is beyond open source libraries. When we think about how we as developers used to write code many years ago, certainly when I started 20 years ago, pre-cloud, we thought about the open source libraries that we used to develop. We thought about the application code that we used to develop. We used to consume open source libraries as well as developing them ourselves. This constituted the application which we then threw over the wall to an operations team that looked after the platform, looked after the operations piece, the production environment. Whereas today in a cloud environment, so much of that is now more under the control, more under the governance, the development of a regular application. The Docker files, the infrastructure as code, whether it’s Terraform scripts, Kubernetes config, these are the more typical things that we as developers are touching on a day-to-day basis. As a result, we need more of an AppSec solution to make sure that things that we change, things that we touch are being done in a secure manner. A lot of the time all of these things are existing in our Git repositories. As a result, they’re going through the development workflows. What we need to be able to do is make sure we have solutions in place, which test these in that development workflow, in our IDEs, in our GitHub repositories, and so forth.
Rethinking Priorities
When we think about traditionally, as developers potentially looking at what we are securing, we absolutely go straight to our own code. While this is an important thing to statically test, as well as dynamically test, it’s important to look at what’s beneath the water almost as that iceberg. Open source code, you can tone as your infrastructure as code, your misconfigurations there, think about where you are most likely to be breached. Is it an open source library, is it your own code, or is it an S3 bucket where there’s a misconfiguration? Is there a container that contains vulnerabilities in the open source packages? Look at this as one, and trying to identify where your most critical issues are based on the stack that you are using.
Supply Chain Security: A Novel Application Risk
One of the last things here I want to cover really is something called the supply chain. Maybe you’ve heard a lot about supply chain security, supply chain risk more recently. The problem is essentially when I started in my development days, we had very much internal build systems, internal build. We had build engineers that were running builds literally on our own data centers. Much more of that is now done by third party software, done by SaaS software, potentially, as well. It’s a much more complex pipeline that we’ve built up over the last couple of decades. There’s a lot of trust that we need to put on many of these different components in this pipeline. Additionally, we need to understand what’s in that pipeline, but also where the weak links are in our chain, where the weak links are in our supply chain as well as that pipeline to identify where we are most vulnerable.
Let’s take a look at where the security risks are potentially, as part of our pipeline. First of all, we have the pipeline that we deliver. We as developers checking code into Git, pushing to a build pipeline, storing perhaps in an artifact repository before pushing to a consumer, maybe into our production environment, or to another supply chain, potentially as well. The thing that we’ve mentioned mostly here is these third party libraries. There’s two pieces here, one is the risk that we add into our application from our supply chain. The second one is a potential supply chain attack. The two are quite different. The second one there is about a compromise of our dependency.
Do you think Log4j, Log4Shell, was a supply chain attack? Is it a supply chain attack? Think about who the attacker is. Who is the attacker that is potentially trying to perform the attack? What are they trying to attack? What is it that they are trying to break, trying to attack, trying to compromise? They’re typically some attacker out there who is trying to break your application that contains Log4j. They’re attacking your application, they’re not attacking your supply chain. Log4j is providing you with supply chain risk, but it’s not a supply chain attack. An attack on your supply chain is where the attacker is trying to intentionally break, maybe a library, maybe trying to compromise your library, your container, for example, and other things that we’ll talk about. The attacker is breaking your supply chain. They’re not trying to attack your application. They’re not trying to attack your endpoints. They’re trying to break your supply chain. The actual attack vector will get introduced in your supply chain. They’re not trying to attack an endpoint or attack something that you’ve put into production.
Obviously, containers, very similar there with vulnerabilities or compromises that can come in through your container images as well, public container images. A second one is a compromise of the pipeline, compromise of developer code. Someone trying to attack your Git repository. Someone trying to break into your build through misconfigurations in your build environments, potentially. Unauthorized attacks into your pipeline. Then the third piece of a supply chain attack is this compromise of a pipeline dependency. For example, Codecov was one. SolarWinds was another one here with the build tools and the Codecov plugin that was added here. They were compromised and they were added as a CI plugin, Codecov as a CI plugin. The compromised malicious version of that plugin was added into other people’s pipelines, attacking their pipelines, taking credentials, taking environment variables and sending it off to evil servers. This is what a supply chain attack really looks like.
These are potentially very lucrative to exploit because if you look at Codecov, that was a cascading supply chain attack. The actual attack happened on the Codecov supply chain that was then used in other supply chain, so it cascades to huge numbers of pipelines, giving out huge numbers of credentials. This is where we’re thinking beyond the open source libraries. Cascading effects are some of the biggest ones that can be attacked.
Conclusion
Hopefully, that gives you a good insight here into actionable tips that you can actually take away, and also areas of risk that you can look at, because while today it’s Log4Shell or Spring4Shell, tomorrow there could be another attack vector. We need to think very holistically about the overall application that we’re deploying, and where the greatest risk in our processes, our teams, and where our tooling can really help us out there.
See more presentations with transcripts
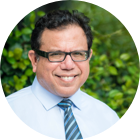
MMS • Sergio De Simone
Article originally posted on InfoQ. Visit InfoQ
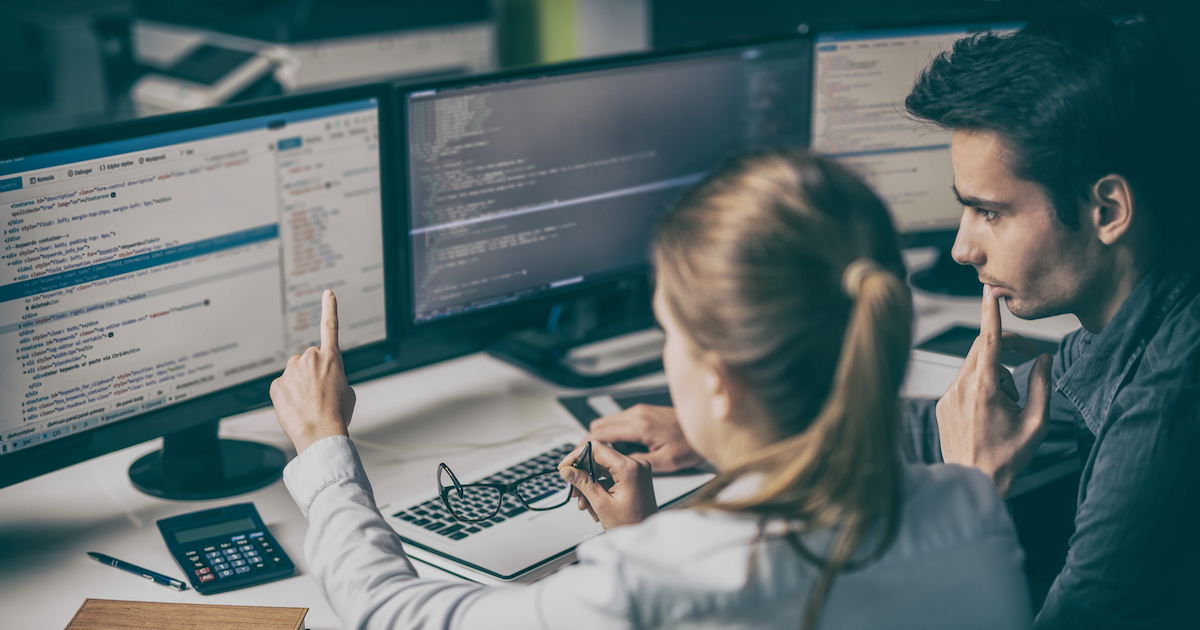
Two Git vulnerabilities affecting local clones and git shell
interactive mode in version 2.38 and older have been recently patched.
Classified as a high severity vulnerability, the git shell
vulnerability may allow an attacker to gain remote code execution by triggering a bug in the function that splits command arguments into an array. Since this function return an int
to represent the number of entries, the attacker can easily overflow it. This value is then used as the index count for the argv
array passed into execv()
, leading to arbitrary heap writes. This vulnerability can only be exploited when git shell
is enabled a login shell.
To fix this vulnerability
git shell
is taught to refuse interactive commands that are longer than 4MiB in size.split_cmdline()
is hardened to reject inputs larger than 2GiB.
The second, medium-severity vulnerability exploits symbolic links when doing a local clone to expose sensitive information to an attacker. A local clone is a clone operation where both the source and the target reside on the same volume.
Git copies the contents of the source’s
$GIT_DIR/objects
directory into the destination by either creating hardlinks to the source contents, or copying them (if hardlinks are disabled via--no-hardlinks
). A malicious actor could convince a victim to clone a repository with a symbolic link pointing at sensitive information on the victim’s machine.
This vulnerability can also be triggered when copying a malicious repository embedded via a submodule from any source when using the --recurse-submodules
option.
To fix to this vulnerability, Git will no longer dereference symbolic links and will refuse to clone repositories having symbolic links in the $GIT_DIR/objects
directory.
Both vulnerabilities have been patched in versions 2.30.6, 2.31.5, 2.32.4, 2.33.5, 2.34.5, 2.35.5, 2.36.3, and 2.37.4. If upgrading is not an option, there are workarounds that can help in the short-term.
The local clone vulnerability can be avoided by disabling cloning untrusted repositories using the --local
flag. Alternatively, you can explicit pass the no-local
flag to git clone
. Additionally, you should not clone untrusted repositories with the --recurse-submodules
.
The git shell
vulnerability can be avoided by disabling access via remote logins altogether or just disabling interactive mode by removing the git-shell-commands
directory.
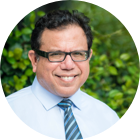
MMS • Matt Campbell
Article originally posted on InfoQ. Visit InfoQ
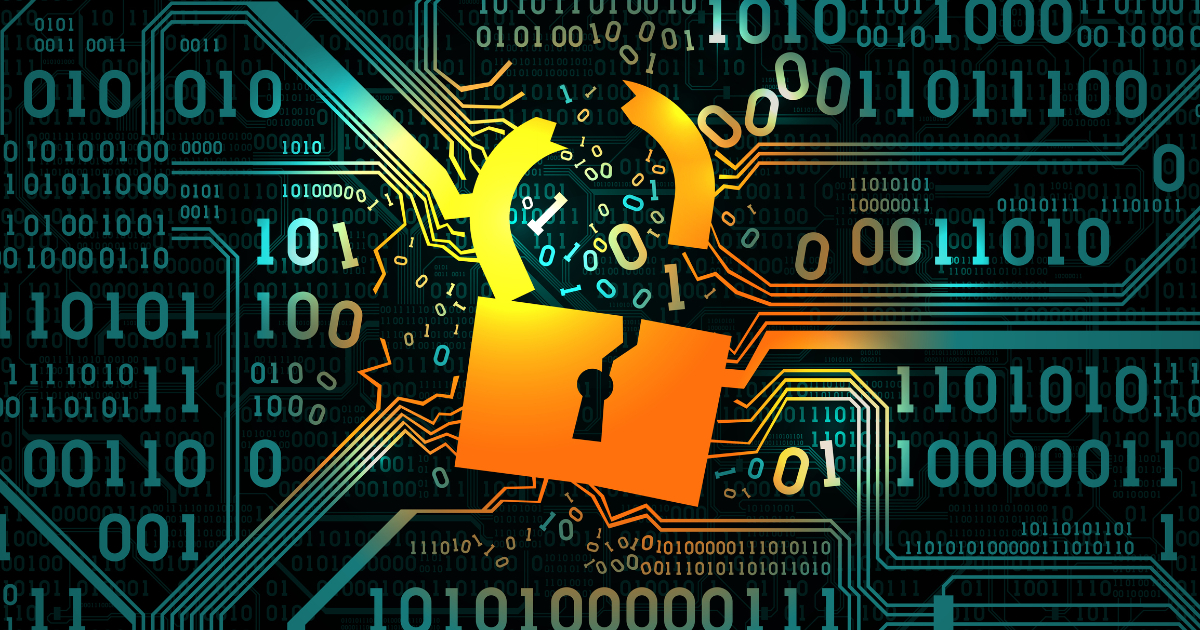
A recent report from Orca Security found several security gaps within the assessed cloud environments. These vulnerabilities include unencrypted sensitive data, S3 buckets with public READ access set, root accounts without multi-factor authentication enabled, and publically accessible Kubernetes API servers. In addition, they found that the average attack path only requires three steps to reach business-critical data or assets.
The report shared that 78% of identified attack paths will use a known exploit (CVE) as the initial access point. This works as the first of three steps needed, on average, to reach what the report authors call “the crown jewels”. This could be personally identifiable information (PII), corporate financials, intellectual property, or production servers.
Once initial access is obtained, typically through the known CVE, the report found that 75% of organizations have at least one asset that enables lateral movement to another asset within their environment. They found that 36% of organizations have unencrypted sensitive data and that 72% have at least one S3 bucket that permits public read access. They note that the top-end goal of most attack paths is data exposure.
Just within the past year, there have been numerous cases of sensitive data being exposed due to misconfigured public cloud storage. John Leyden shared that unencrypted data from Ghana’s National Service Secretariate (NSS) was discovered by researchers at vpnMentor. According to Leyden much of the data was publically accessible and “the AWS S3 bucket itself was neither encrypted nor password protected”. A similar incident occurred in July of this year when a misconfigured S3 bucket resulted in over 3TB of airport data, including photos of airline employees and national ID cards, becoming publicly accessible.
Of note within the report is that 10% of organizations still have vulnerabilities present that were disclosed over ten years ago. In addition, most organizations have at least 11% of their assets in a neglected security state where they are running an unsupported operating system or the asset has been unpatched for over 180 days.
The authors conclude that more effort needs to be applied to fixing known vulnerabilities. They concede that “many lack the staff to patch these vulnerabilities, which in more complex, mission critical systems is often not a simple matter of just running an update.” They continue by recommending a focused approach:
It is close to impossible for teams to fix all vulnerabilities. Therefore, it is essential to remediate strategically by understanding which vulnerabilities pose the greatest danger to the company’s crown jewels and need to be fixed first.
The report lists several best practices that they feel are not being followed correctly. This includes 99% of organizations using at least one default AWS KMS key. Orca Security recommends the use of customer-managed keys (CMK) instead of AWS managed. They also recommend enabling automatic key rotation, which only 20% of organizations had.
The recommendation to use CMKs comes from the additional level of control that they grant. This includes being able to create key policies, IAM policies, grants, tagging, and aliasing. User semanticist noted another potential use case on Reddit “if you want to be able to segment your keys (for instance, having one more-locked down and heavily-audited CMK that you use for the most sensitive S3 objects), then you would need to use a customer managed CMK.” There are additional costs incurred in using CMKs over the AWS managed KMS keys.
A recent misconfiguration by AWS to their AWSSupportServiceRolePolicy
granted the S3:GetObject
permission to AWS support staff. While this was quickly reverted, Victor Grenu, independent cloud architect, shared some key takeaways from the event:
- IAM is HARD, even AWS is failing
- Changes made to IAM should always be peer-reviewed, manually, and using linting
- Encrypt using your own customer-managed keys
In line with the first two points, the report found that 44% of environments have at least one privileged IAM role and that 71% are using the default service account in Google Cloud. They note that this account provides editor permissions by default and therefore violates the principle of least privilege. A similar finding violating least privilege was found in 42% of scanned accounts having more than 50% of the organization’s users having administrative permissions.
For more details on the report’s findings, readers are directed to the Orca Security 2022 State of the Public Cloud Security report.
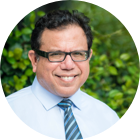
MMS • Ben Linders
Article originally posted on InfoQ. Visit InfoQ
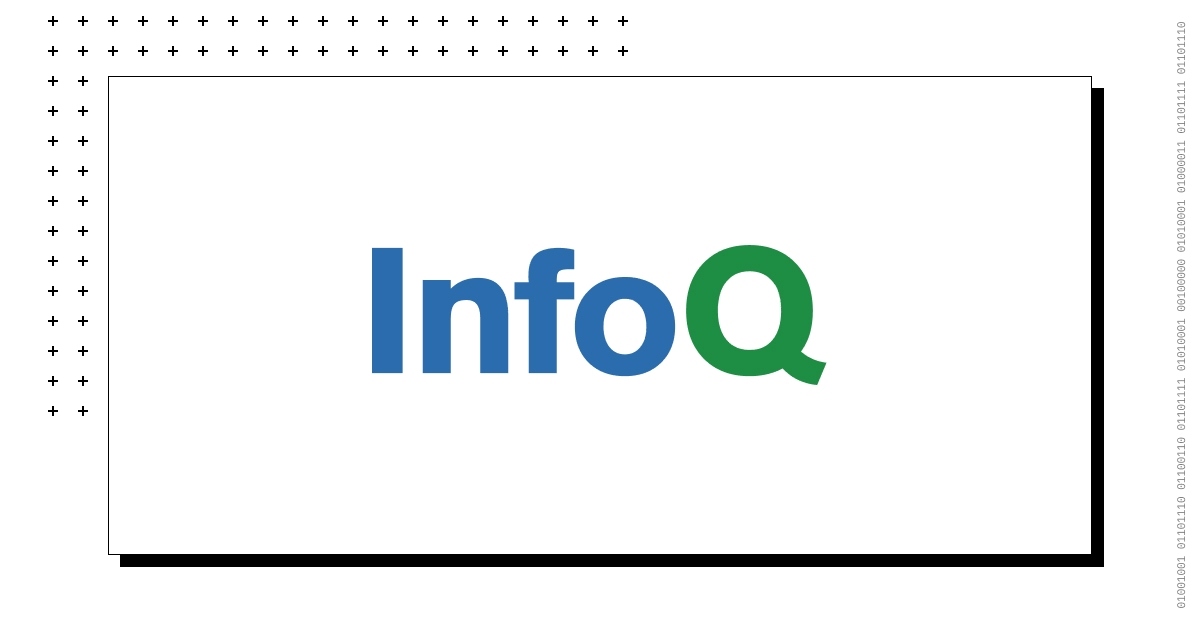
Progressive workplaces focus on purpose and value, having networks of teams supported by leaders with distributed decision-making. Employees get freedom and trust, and access to information through radical transparency that enables them to experiment and adapt the organization. In such workplaces, people can develop their talents and work on tasks they like to do, and have more fun.
Pim de Morree from Corporate Rebels spoke about unleashing the workplace revolution at Better Ways 2022.
The way we work is broken, De Morree stated. According to the State of the American Workplace Report 2017, only 15% of the employees are engaged in their work. When it comes to burnout symptoms, 44% of the employees sometimes have them whereas 23% always or often have them (based on Arbobalans 2018). The report Socially Useless Jobs states that 40% of the employees feel that their work is not useful and that they are not contributing to society.
De Morree stated that it’s beneficial for companies to create a workplace where people want to be, as this leads to higher productivity and profit, lower absenteeism, and fewer accidents and defects.
To figure out ways to motivate employees to the highest possible state, De Morree and Joost Minnaar are visiting pioneering organizations around the globe. Based on this they have identified eight trends in progressive workplaces:
- Focus on values and put purpose first in decision-making.
- Reinvent the entire structure, breaking down the hierarchy into a network of autonomous teams.
- Leaders become coaches and coordinators who support the success of their teams.
- Experiment and adapt; try out new ways of working.
- Creating a work environment where people have trust and freedom.
- Get rid of rules and distribute authority throughout the company.
- Grant company-wide access to data, documents, financials—in real-time, radical transparency.
- Finding the individual main talents and finding ways for people to develop those.
De Morree mentioned that there are different approaches to transform and propagate change in a company:
- Start small with one team and then add other teams.
- Involve all teams into change at once (big bang)
- Start with one department to remove the hierarchy into a network of teams.
- Radically change the whole company at once. This usually only works when there’s an urgent and important need.
He provided ideas for improvements that progressive teams can start with:
- Redesign your meetings: have fewer and better meetings. There should be a clear structure for meetings where De Morree referred to sociocracy: no agenda upfront, but created on the spot- people can choose how to be involved. First inform everyone and then make a decision based on consent.
- Distribute decisions using consent-based decision-making. This can lead to quicker and better decisions.
- Give better feedback. One technique that can be used is the “stop, start, continue” technique.
- Create a list of all activities, cluster them into roles, and let people choose what role fits them.
- Resolve conflict better by turning conflicts into opportunities in a graceful way.
- Adopt result-based working; assess team and individuals on outcomes and give freedom for people to boost performance.
The world needs more rebels to make work more fun, De Morree concluded.
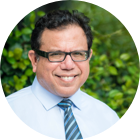
MMS • Johan Janssen
Article originally posted on InfoQ. Visit InfoQ
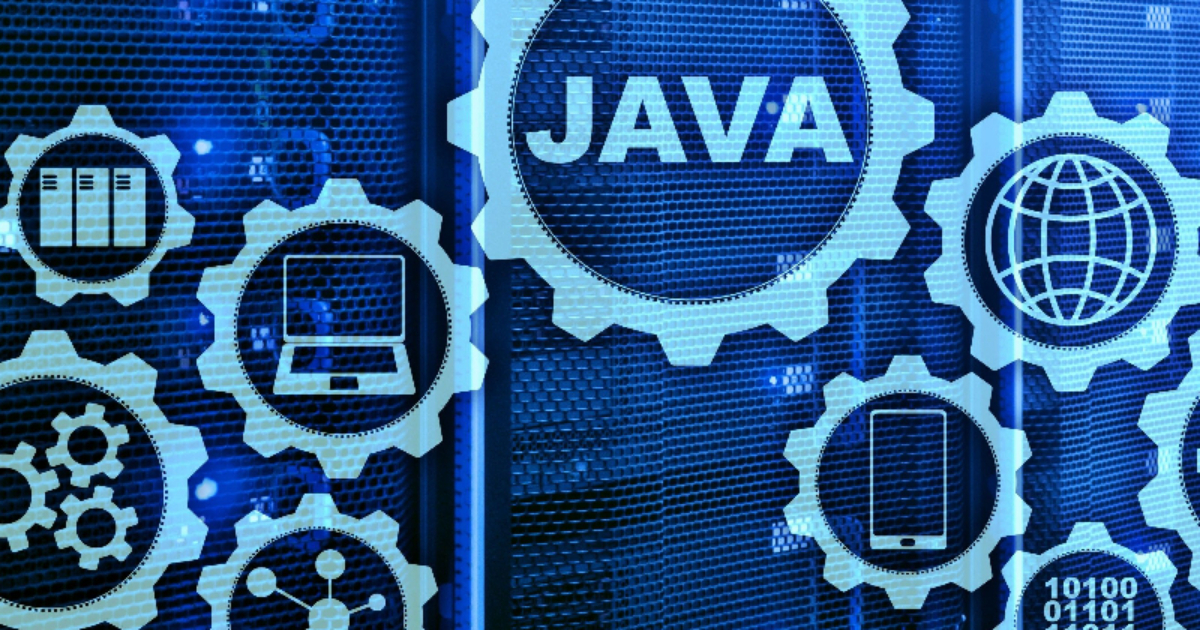
BellSoft has released Alpaquita Linux, an operating system based upon Alpine Linux, optimized for containerized Java applications. A plain Docker image is available, as well as Docker images with Liberica JDK or JRE or a Native Image Kit based upon GraalVM.
Alternatively, Alpaquita Linux can be installed via Windows Subsystem for Linux (WSL), Linux repositories or an ISO file.
Alpaquita Linux offers some additional benefits such as LTS releases, support for the musl and glibc C standard libraries and modern security features with fast security patches for both the operating system and the Java runtime. Security options include the kernel lockdown feature which makes it impossible to directly or indirectly access a running kernel image. The kernel module signing is another option and works with SHA-512 to disallow loading modules with invalid keys and unsigned modules. The small number of operating system components reduces the attack surface. Hardening is supported with userspace compilation options such as the -Wformat-security
argument which issues a warning for format functions which may cause security issues.
The operating system supports kernel module compression, and contains the malloc implementations mimalloc, jemalloc and rpmalloc.
This is the only Linux operating system optimized for Java applications with a TCK-verified runtime and optimized memory consumption.
BellSoft provides four years support for Long Term Support (LTS) releases, two years more than Alpine Linux. The current LTS is released this year and the next one will be released in 2024. BellSoft offers various commercial support plans.
The Alpaquita Linux Docker image based on musl is 3.22 MB and the image based on glibc is 7.8 MB. That’s just a bit bigger than the Alpine Docker images which start at less than 2.5 MB. Additional Docker images with Python or GCC are available as well.
Alternatively, the Liberica Runtime Container, based on Alpaquita Linux, may be used for Java applications. Docker images are available for Java 8, 11 and 17 where the smallest JRE and JDK images for Java 17 are less than 75MB. The images are based upon Liberica Lite which is optimized for size, performance and cloud deployments.
The last alternative based on Alpaquita Linux is the Liberica Native Image Kit (NIK) which uses GraalVM. Docker images are available for Java 11 and 17, where the Java 17 images start at a bit more than 308 MB. The kit may be used to compile JVM applications into ahead of time compiled native executables that offer faster startup times and lower memory consumption compared to traditional applications. The executable binary file contains the application, dependencies and runtime components including the Java Virtual Machine (JVM). Applications written in JVM languages such as Java, Kotlin, Scala and Groovy may be converted into executables. The Native Image Kit works on Windows, Linux and macOS on machines with x86, x64 or ARM processors (Linux only).
Alpaquita Linux is part of the Alpaquita Cloud Native Platform together with Liberica JDK Lite and Liberica Native Image Kit (NIK).
More information can be found in the introduction blog.
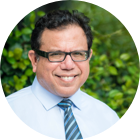
MMS • Emeni Oghenevwede
Article originally posted on InfoQ. Visit InfoQ

Key Takeaways
- Separation of concerns is a Node.js architecture that ensures code readability, easy refactoring, and good code collaboration.
- Using the separation of concerns principle, you can ensure that the final system is stable and maintainable.
- The principle of separation of concerns ensures that components are not duplicated, making the system easier to maintain and refactor.
- The separation of concerns principle holds that business logic should be kept separate from controllers. This simplifies the development of thin controllers and the writing of tests.
- Separation of Concerns principle aids in code reusability. This reduces maintenance costs and time by making it simple to determine where a fault is coming from and how to isolate it from the entire system.
Best Practices for Creating a Good Node.js Project Architecture
Most of the time, we work in large groups with different people handling different parts of the system, which can get messy if everything isn’t properly arranged and just jumbled all together. More teams are working remotely as a result of the pandemic, and having clear and well-defined code structures has never been more crucial.
Essentially, project structuring is an important topic because how you bootstrap your application can determine the overall development experience throughout the project’s life cycle.
The amazing and somewhat frustrating aspect of Node.js is that you can structure your code however you want. There is no “correct way”. You have the option of writing all of your code in a single app.js file or creating multiple files and placing them in different folders.
Most developers, however, would recommend structuring your projects by grouping related data together rather than having it all together. It would be preferable to know that you can make changes to your models by browsing through the model folders rather than having to navigate through a single file containing models, controllers, loaders, and services.
Why is good project architecture so important?
As previously stated, good project architecture is critical, and messy architecture can be problematic. Here are a few benefits of good architecture:
- makes code more readable and tidy.
- simpler to avoid repetition.
- makes scaling and changes easier.
- simplifies test authoring
Separation of concerns
Separation of concerns is a design principle that divides a software program into segments. Each subsection attempts to address a distinct issue, a group of details that have an impact on the program’s code.
This concept essentially refers to an architecture pattern in which program logic is separated from program content and presentation. This makes the project easier to maintain, and less prone to repetitions. It also streamlines changes and team collaboration.
A Node.js project can be organized in a variety of ways. Every organizational method has advantages and disadvantages. At the end of the day, every developer’s goal is to create scalable and clean codes. Projects which follow this architecture pattern are often structured this way:
└───app.js # Our Application's entry point
└───api # Contains controllers, routes, middlewares
└───config # Application configs for development and production
└───loaders # Contains the startup processes
└───models # Database models
└───services # Contains our business logic
└───jobs # Jobs definitions(if you have cron jobs in your program, we don't)
└───subscribers # Event handlers for async task
└───test # Our Program test files here
In order to explain folder structuring and the concept of separation of concerns, we will create a simple authentication REST API. We will be constructing a scalable structure that facilitates team collaboration. We will be utilizing Node.js, Express.JS, and MongoDB. To get started, make sure you have Node.js and MongoDB installed.
Our example application is a simple REST API for authentication. When a user registers, their information is saved in our MongoDB database. When a user attempts to login, we verify their information and return a token if they are verified. While building this, we will implement a scalable project structure and see what is required to achieve it.
Setting up our project folders
Our application will be structured in the following manner:
- All files and logic will be kept in a single folder called src.
- The app entry and startup are taken care of by our server.js and app.js scripts.
- The api folder comprises subfolders for controllers, middlewares, routes, and repositories, which are mostly used to handle tasks like data transmission, request processing, and validation.
- Our configuration folder, config, contains information about how our development and production environments are managed.
- The loaders folder contains the actions that the program performs when it first launches. This comprises our database loader, which tells our database to start, and our express loaders, which execute our express app.
- The models folder contains files that describe the type of data transferring to or receiving from the database.
- The services folder contains reusable business logic that handles tasks such as data processing, implementing unique business logic, calling the database, etc.
- The utils folder includes documents such as helpers, validators, error handlers, constants, etc. Other files in the application may call these files in order to help with an operation.
└───src
└───app.js
└───server.js
└───api
└───controllers
└───middlewares
└───routes
└───config
└───loaders
└───models
└───services
└───utils
Utils Folder – Helper files
These files support other portions of the application. They get utilized by several files or modules to possibly validate or change a request or piece of data because they have a reusable structure. For instance, developing a helper function that verifies that emails are written in an appropriate format. This feature can be used to verify that the email being input follows the proper format in places like sign up or login.
Our utils folder contains four files:
- validator.js
- helpers.js
- error_handler.js
- error_response.js
validator.js
A method in this file called signupValidator verifies that the required arguments are being supplied and that they are being passed correctly. For instance, we verify that the name and email are supplied and the password is in the format that we desire (at least 8 characters and a mix of Alphanumeric and special characters).
import { celebrate, Joi, Segments } from 'celebrate';
export default class Validator {
static signupValidator = celebrate({
[Segments.BODY]: Joi.object().keys({
name: Joi.string().required(),
email: Joi.string().email().required().trim().lowercase(),
password: Joi.string().regex(/^(?=.*[a-z])(?=.*[A-Z])(?=.*d)[a-zA-ZdwW]{8,}$/).required().label('Password').messages({
"string.min": "{#label} Must have at least 8 characters",
"string.pattern.base": "{#label} must include at least eight characters, one uppercase and lowercase letter and one number"
})
}),
});
}
helpers.js
This file contains functions that manage the format of our JSON responses, the hashing of our passwords, the generation of random strings, and more. The helpers file simply contains many functions that are utilized by many services; instead of building these functions within your services, import them as needed to keep code clean and accelerate development.
import bcrypt from 'bcryptjs';
import crypto from 'crypto';
const ENCRYPTION_KEY = "(some_r**n_5_str_$$8276_-yuiuj6]"; // Must be 256 bits (32 characters)
const IV_LENGTH = 16; // For AES, this is always 16
export class JsonResponse {
constructor(statusCode = 200) {
this.statusCode = statusCode;
}
error = (res, message, data) => {
return res.status(this.statusCode).json({
status: false,
message,
data
})
}
success = (res, message, data) => {
return res.status(this.statusCode).json({
status: true,
message,
data
})
}
}
export const randomString = (length) => {
let numbers = "0123456789";
let chars = "acdefhiklmnoqrstuvwxyzABCDEFGHIJKLMNOPQRSTUVWXY";
let randomstring = '';
let randomstring2 = '';
for (let x = 0; x < Math.floor(length / 2); x++) {
let rnum = Math.floor(Math.random() * chars.length);
randomstring += chars.substring(rnum, rnum + 1);
}
for (let y = 0; y bcrypt.compare(string, hash);
export const hashString = async function (string) {
const salt = await bcrypt.genSalt(10);
return await bcrypt.hash(string, salt);
}
export const encryptData = data => {
let iv = crypto.randomBytes(IV_LENGTH);
let cipher = crypto.createCipheriv('aes-256-cbc', Buffer.from(ENCRYPTION_KEY), iv);
let encrypted = cipher.update(data);
encrypted = Buffer.concat([encrypted, cipher.final()]);
return iv.toString('hex') + ':' + encrypted.toString('hex');
}
export const decryptData = data => {
let textParts = data.split(':');
let iv = Buffer.from(textParts.shift(), 'hex');
let encryptedText = Buffer.from(textParts.join(':'), 'hex');
let decipher = crypto.createDecipheriv('aes-256-cbc', Buffer.from(ENCRYPTION_KEY), iv);
let decrypted = decipher.update(encryptedText);
decrypted = Buffer.concat([decrypted, decipher.final()]);
return decrypted.toString();
}
error_handler.js
This defines the error response structure. For instance, you can call this in the catch section whenever you try to build a try-catch event and provide the necessary parameters, such as the status, data, and message. Instead of having to declare an error structure everywhere, you can reuse this approach. It is crucial to display errors accurately since it helps the user and developer consuming the API to comprehend the issue at hand.
export default class ErrorResponse extends Error {
constructor(message, status) {
super(message);
this.status = status;
}
}
error_response.js
We can infer from the file’s name that this contains functions that handle different error conditions. For instance, it has functions for dealing with 404 problems, duplicate fields in our database, and server issues.
import ErrorResponse from './error_response';
import { isCelebrateError } from 'celebrate';
const errorHandler = (err, req, res, next) => {
console.log(err)
let error = { ...err };
error.message = err.message;
//celebrate error
if (isCelebrateError(err)) {
if (!err) {
error = new ErrorResponse("Unable to process request, try again", 400);
} else {
const errorBody = err.details.get('body');
if (errorBody) {
const { details: [errorDetails] } = errorBody;
console.log(errorDetails)
const message = errorDetails.message;
error = new ErrorResponse(message, 400);
} else {
error = new ErrorResponse("Invalid payload sent, review and try again", 400);
}
}
}
// mongoose duplicate error
if (err.code == 11000) {
const message = "Field already exists or duplicate value encountered";
error = new ErrorResponse(message, 400);
}
// mongoose validation error
if (err.name == "CastError") {
const message = "Invalid parameter passed";
error = new ErrorResponse(message, 400);
}
// mongoose validation error
if (err.name == "ValidationError") {
const message = Object.values(err.errors).map(val => val.message);
error = new ErrorResponse(message, 400);
}
res.status(error.status || 500).json({
status: false,
message: error.message || "Server error! request not completed",
data: {}
});
}
export default errorHandler;
Config Folder – Environment Management
Most of the time, we have varying environmental variables. For instance, if we are working locally in our development environment, our MongoDB URI will most likely begin with localhost, whereas a link leading to an atlas database will be present in our production environment. Therefore, it is wise to handle these differences with care. Our config folder will contain three files: dev.js (for the development environment), prod.js (for the production environment), and an index.js file, where they get imported. Additionally, the index.js file has a switch case that determines which file should get utilized depending on the environment.
Don’t forget to make an .env file with all the variables you require.
dev.js
import '../.env'
import dotenv from 'dotenv';
dotenv.config()
export const config = {
secrets: {
jwt: process.env.JWT_SECRET_DEV,
jwtExp: '100d'
},
dbUrl: process.env.MONGO_URI_DEV,
}
prod.js
import '../.env'
import dotenv from 'dotenv';
dotenv.config()
export const config = {
secrets: {
jwt: process.env.JWT_SECRET,
jwtExp: '7d'
},
dbUrl: process.env.MONGO_URI,
index.js
import { merge } from 'lodash';
const env = process.env.NODE_ENV || 'development';
const port = process.env.PORT || 4002;
const baseConfig = {
env,
isDev: env === 'development',
port,
}
let envConfig = {}
switch (env) {
case 'dev':
case 'development':
envConfig = require('./dev').config
break
case 'prod':
case 'production':
envConfig = require('./prod').config
break
default:
envConfig = require('./dev').config
}
export default merge(baseConfig, envConfig)
Loaders Folder
The loaders folder contains files required for the initialization of specific functions. For example, we have an express and a database loader that start the express app and database, respectively.
The idea is to divide the application’s startup process into testable components. The various loaders are imported into an index.js file in the loaders folder, which makes them available to other files.
db-loader.js
import mongoose from 'mongoose';
import dotenv from 'dotenv';
import options from '../config';
require('dotenv').config({path: __dirname + '/.env' })
export default (url = options.dbUrl, opts = {}) => {
let dbOptions = { ...opts, useNewUrlParser: true, useUnifiedTopology: true };
mongoose.connect(url, dbOptions);
const conn = mongoose.connection;
return conn;
}
express-loader.js
import * as fs from 'fs';
import morgan from 'morgan';
import mongoSanitize from 'express-mongo-sanitize';
import rateLimit from 'express-rate-limit';
import helmet from 'helmet';
import xss from 'xss-clean';
import cors from 'cors';
import ErrorResponse from '../utils/error_response';
import errorHandler from '../utils/error_handler';
// import routes
import apiRoutes from '../api/routes';
const apiLimiter = rateLimit({
windowMs: 20 * 60 * 1000, // 20 minutes
max: 100, // Limit each IP to 100 requests per `window` (here, per 20 minutes)
standardHeaders: true, // Return rate limit info in the `RateLimit-*` headers
legacyHeaders: false, // Disable the `X-RateLimit-*` headers
handler: (_request, res, _next) => res.status(429).json({
status: false,
message: "Too many requests, please try again later."
}),
})
export default ({ app, express }) => {
app.disable('x-powered-by');
app.use(express.json())
app.use(express.urlencoded({ extended: true }))
// Dev logging middleware
if (process.env.NODE_ENV === 'development') {
app.use(morgan('dev'));
}
app.enable('trust proxy');
app.use(cors())
app.use(mongoSanitize());
// add secure headers
app.use(helmet());
app.use(xss());
app.get('/ip', (request, response) => response.send(request.ip))
app.use('/api/v1', apiLimiter, apiRoutes);
app.use(errorHandler);
app.use((_req, _res, next) => next(new ErrorResponse('Route not found', 404)));
app.use(errorHandler);
return app;
};
index.js
import dbConnect from './db-loader';
import expressLoader from './express-loader';
export default async ({ app, express }) => {
const connection = dbConnect();
console.log('MongoDB has been Initialized');
expressLoader({ app, express });
console.log('Express package has been Initialized');
}
Entry Files
Our app’s entry point is app.js. It is common practice to put significant amounts of code here, but the separation of concerns ensures that all logic gets separated. We will create two entry points, namely server.js and app.js. In our server.js file, we will import our loaders and configuration files, as well as begin listening to our PORT. Our app.js file simply imports our server.js file. So, technically, when our server attempts to run our application, it reaches the app.js file and attempts to start the various functions specified in our server.js file.
server.js
import express from 'express';
import dotenv from 'dotenv';
import appLoader from './loaders';
import appConfig from './config';
export const app = express();
require('dotenv').config({path: __dirname + '/.env' })
export const start = async () => {
try {
await appLoader({ app, express });
app.listen(appConfig.port, () => {
console.log(`REST API on http://localhost:${appConfig.port}/api/v1`);
console.log(process.env.MONGO_URI_DEV);
console.log(appConfig.dbUrl);
});
} catch (e) {
console.error(e)
}
}
app.js
import { start } from './server'
start()
process.on('unhandledRejection', (err, _) => {
console.log(`Server error: ${err}`)
})
So far, when we run our application, we get a message saying that our app is running on our preferred port, that the express server has started, and that our database has been successfully connected.
Models
Then there are the models which are simply interfaces between our application and the database. They structure the data that we want to pass around our application. As a result, we’ll make two files in our models folder: user models file and an index.js file into which we’ll import every other model.
user.model.js
import mongoose from 'mongoose';
import bcrypt from 'bcryptjs';
import { sign } from 'jsonwebtoken';
import config from '../config';
const UserSchema = new mongoose.Schema({
name: {
type: String,
trim: true,
required: [true, "Name is required"]
},
email: {
type: String,
trim: true,
unique: true,
match: [/^(([^()[].,;:s@"]+(.[^()[].,;:s@"]+)*)|(".+"))@(([[0-9]{1,3}.[0-9]{1,3}.[0-9]{1,3}.[0-9]{1,3}])|(([a-zA-Z-0-9]+.)+[a-zA-Z]{2,}))$/, "Please enter a valid email"],
required: [true, "Email is required"]
},
password: {
type: String,
select: false,
},
created_at: {
type: Date,
default: Date.now
}
})
Index.js
import User from './user.model';
export { User};
Services
Services handle things like data manipulation, database calls, and other business logic. Separating app services from controllers is a separation of concerns technique. The service layer contains business-related logic and nothing related to the HTTP layer. This technique allows for easier test writing, refactoring, and thinner controllers. Services implement our application’s logic and communicate with the database via the data access layer before returning a necessary response to the controller. We made a simple auth service file that contains our signin and signup logic.
auth.js
import { User } from '../models';
import ErrorResponse from '../utils/error_response';
import { randomToken } from '../utils/helpers';
export default class AuthService {
//User register
async signup(data) {
try {
const { email, password, name } = data;
// find user by email
let query = { $or: [{ email: { $regex: email, $options: 'i' } }] };
const hasEmail = await User.find(query);
console.log(hasEmail);
// throw error if user not found
if (hasEmail.length > 0) { throw new ErrorResponse('Email already exists', 400); }
const user = await User.create({ email, password, name });
console.log(user)
return user;
} catch (e) {
throw e;
}
}
async signin(data) {
try {
let { email, password } = data;
let query = {
$or: [
{ email: { $regex: email, $options: 'i' } }
]
};
// find user by email
const user = await User.findOne(query).select('+password');
// throw error if user not found
if (!user) { throw new ErrorResponse('Invalid credentials', 401); }
// check user password
const isMatch = await user.comparePassword(password);
if (!isMatch) { throw new ErrorResponse('Invalid credentials', 401); }
return {
user: user.toMap(),
token: user.getJwtToken(),
};
} catch (e) {
throw e;
}
}
}
APIs
Finally, we have our api folder, which contains three other important folders: controllers, routes, and middleware, which we will go over individually.
Middleware
Middlewares are in charge of handling various validation or other general checks in an application. We’ll make two files, async_handler.js and auth_handler.js, to handle res (response) and req (request) objects, as well as user authorization.
async_handler..js
export const asyncHandler = fn => (req, res, next) => Promise.resolve(fn(req, res, next)).catch(next);
auth_handler.js
import { verify } from 'jsonwebtoken';
import ErrorResponse from '../../utils/error_response';
import { asyncHandler } from './async_handler';
import config from '../../config';
export const userAuth = asyncHandler(
async (req, res, next) => {
let authHeader = req.headers.authorization;
let token = authHeader && authHeader.startsWith('Bearer') && authHeader.split(' ')[1];
if (!token) {
return next(new ErrorResponse('Unauthorized access', 401));
}
try {
const decoded = verify(token, config.secrets.jwt);
req.user = decoded.result;
next();
} catch (e) {
return next(new ErrorResponse('Unauthorized access', 401));
}
});
Controllers
Controllers receive requests, make a call to the required service, and then communicate with the database via the data access layer, then sending the results back to the service, which then sends the results back to the controller, and the controller delivers the result to the client. We will create a file called index.js in our controllers folder that will contain our signin and signup controllers. These controllers use the res and req objects in the async handler.js file to send requests to various services.
index.js
import { asyncHandler } from '../middlewares/async_handler';
import { JsonResponse } from '../../utils/helpers';
import AuthService from '../../services/auth';
export default class IndexController {
constructor() {
this.authService = new AuthService();
}
index = asyncHandler(
async (req, res, _) => {
res.json({ status: true, message: "Base API Endpoint." })
});
loginUser = asyncHandler(
async (req, res, _) => {
const { user, token } = await this.authService.signin(req.body);
return new JsonResponse().success(res, "User logged in successfully", { user, token });
});
registerUser = asyncHandler(
async (req, res, _) => {
await this.authService.signup(req.body);
return new JsonResponse(201).success(res, "User account created successfully", {});
});
}
Routes
Routes simply define how our application should respond to HTTP requests from clients. It is the portion of our program’s code related to HTTP verbs. Middleware may or may not protect these routes. Routes’ primary function is to handle requests as they arrive.
For example, a POST request makes the route and expects data to be posted or passed.
In our routes folder, we’ve created an index.js file that contains all of the routes required to access the platform’s various services. Routes receive a request, forward it to the controller, which then forwards it to the database and returns a report to the controller.
index.js
import { Router } from 'express';
import Validator from '../../utils/validator';
import IndexController from '../controllers';
const router = Router();
// import all controllers
let indexController = new IndexController();
// register all routes
router.get('/', indexController.index);
router.post('/login', indexController.loginUser);
router.post('/register', Validator.signupValidator, indexController.registerUser);
//export the base router
export default router;
Conclusion
Every developer should strive for clean, readable, and reusable code, which makes it easier to refactor, collaborate with others, test, and make fewer mistakes. There are various approaches to designing API architecture, and there are many right ways; At all costs, make sure that scalability and readability are your top considerations when choosing an architecture.
We do, however, recommend using the separation of technique architecture because, as you can see, it has numerous advantages. This technique has proven to be useful in building projects regardless of project complexity or team size. You don’t want anything to go wrong during production!
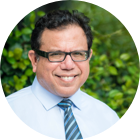
MMS • Jitesh Gosai
Article originally posted on InfoQ. Visit InfoQ

Key Takeaways
- Testability enables teams to confidently make changes to their systems via tests that indicate if the system’s behaviour has been altered.
- Successfully implementing testability needs developers and testers to collaborate effectively.
- Effective collaboration doesn’t just happen; it needs to be nurtured by team leaders by encouraging experimentation and reflection on what is and isn’t working.
- The key to effective collaboration is psychological safety so that team members can take interpersonal risks without fear of judgement or reprimand.
- Implementing an experimental approach to working allows team members to try things and fail in a risk-free environment.
Testability can enable teams to make changes to their code bases without requiring extensive regression testing. To build testability, team members must collaborate and leverage each other’s unique skills. Unfortunately, effective collaboration does not come naturally to people and therefore needs leadership to nurture people’s ability to speak up and share their knowledge.
Why testability
As a development team, we wanted to reduce the uncertainty introduced when we made changes to our media player- changes such as bug fixes or refactoring the code to make it more maintainable as the system evolved. Any modification, be it bug fixes or a new feature, would almost always need an extensive regression testing cycle. We couldn’t confidently say that a change to the system had not adversely affected its behaviour without our testers exploring the main features and confirming that it still behaved as we intended.
Testability for us meant reducing the uncertainty introduced when making changes to our system. This meant we could release the player to our product teams, who could incorporate it into their apps. Therefore we could say with much greater confidence that we did not need to go into long manual regression testing cycles.
Implementing testability
We knew if we continued working as we had, we would end up with the same result, so we needed to change our development process. Initially, developers would write the code and do whatever testing they needed to ensure that what they developed worked. Then developers would hand it over to the testers to carry out any additional testing they thought it required. If they found an issue, it would be raised with the developers, who would fix it and send it back to the testers. This cycle would continue until both parties were happy and the team could make the release.
Essentially developers and testers were handing work off to each other in their mini silos. It wasn’t as bad as throwing it over a wall, but more like a relay race, each sprinting to do their bit to help get the work to the finish line, hopefully making up for any lost time during their mini sprint. Everyone is working together but very focused on their part of the race. It was more cooperating to get the work done, rather than collaborating.
So to change how we worked, we went back to the drawing board and to prevent developers and testers from handing work off to each other, we took pair programming a step further and moved to a triplet. This triplet consisted of two developers and a tester. Their job was to write and test together until the feature was built and released. In this trio, the developers would test with the support of the tester, and the tester would write code supported by the developers. Each would slowly learn to understand what the other did but would crucially begin to see how they could do things differently.
At first, both parties were a little hesitant to work like this. The testers were unsure if they could keep up with the developers and understand what they were developing, let alone write code. Developers were worried that they would have to go too slowly and would get bogged down with exploratory testing. So to get them going, the dev lead worked with the trio, modelling how the group could work together. They would mob program with each person taking turns to code, but they would pair program with the tester to show how this could work. Once something was developed that could be exploratory tested, the dev lead would let the tester take over and lead the group in what needed to be tested.
This approach slowly showed the trio how they could work together, and with the dev lead being present, it made it safe. Safe to go slower than the developers would typically. Safe that the tester could ask questions and say if they didn’t understand something. Safe for the developers to push back if testing is beyond the scope of the changes.
The aim here was not to turn testers into developers, or developers in testers, but rather to show how they could better make use of the skills the other possessed. So, how could developers write code-level tests that gave the testers confidence that the code worked as intended? But also, how could testers carry out exploratory testing that showed developers the limitations of their code tests?
This trio eventually began to appreciate the skills the other brought to the table and developed a much deeper understanding of how their combined skills could produce a much higher quality product. This level of collaboration allowed the team to move more quickly than before. When the trio developed a feature, it would be fully exploratory tested and supported with automated tests written at the code level. The automated tests allowed developers to make changes to the code base with high certainty that they had not affected the system’s behaviour.
As the testers had been directly involved in creating the tests, they no longer felt they needed more regression testing before a release had to be made. In addition, these tests could be made available to the product teams to see what had and hadn’t been tested, slowly building their confidence in the team’s ability to deliver a stable and tested media player.
What we learned
At first, the trio took time to figure out how to work with each other, but also what they needed to do and how to do it, which meant the trio were considerably slower than how the team usually worked. The developers could have just pair programmed to get the feature built and then let the tester know what they had done. But working as a trio meant the developers had to go slower than they usually would as they would need to help the tester understand what they were doing and how it affected the system.
Other times the tester wanted to do some exploratory testing which the developer would need to get involved with so they could understand what different things they needed to test for that the code tests were unable to cover. By both disciplines doing the work of the other, they began to understand the intricacies that their roles necessitated that simply telling them would never have accomplished.
The problem is that people don’t just naturally work like this. They need to be encouraged and supported to do so. Leadership must enable them to take a more experimental approach to their work; to try things and figure out what works for them. They also need to encourage them to be more reflective of the results and share what they do and don’t understand.
Working this way takes time, so leaders need to give the team the space and support to figure out what works and doesn’t.
One of the best ways leadership can enable this is by getting involved with the process. It could sometimes be taking the driving seat and leading the way, while other times letting others take over and supporting them to do the work. Leading in this way allows the teams to see what is and isn’t acceptable, and lets the team question assumptions and other beliefs that may not be true, such as you cannot make mistakes, say that you don’t understand, or go slower while skilling up others.
Creating a learning environment
Simply throwing people together and expecting them to figure out how to work together like this will most likely result in failure, but that’s the point. You want them to fail, not to stop and go back to how things were, but to figure out why they failed. You want them to talk about what is and isn’t working and what they can do differently.
The problem leadership needs to help these people overcome is the assumption that high-performing people and teams don’t fail. So we will do our best to avoid failure at the first signs of it. Leadership must show that failure is a natural by-product of experimentation and that high performers produce and share their failures; not avoid, deny, ignore or distort them, but learn from them.
But for people to embrace failure like this, they need high levels of psychological safety, meaning team members can take interpersonal risks and be vulnerable by sharing what they don’t know, what they don’t understand or mistakes they have made without fear of judgement or that it will affect their prospects negatively.
Leadership must help people see that these invisible boundaries hold us back and that we are not trying to take people’s jobs away or replace them, but to understand them better. Also, people need to be encouraged to step over their disciplinary boundaries and be more willing to understand what their peers do and how and why they do it the way they do. And one of the best ways to learn is by doing.
Together we can be much more than just the sum of our disciplines. By enabling team members to show fallibility, learn from failure and work across disciplines boundaries, we can move people from coordinating their actions to true collaboration: collaboration which is high in psychological safety and steeped in trust and mutual respect, that we are all trying to do our best work and that failure is a natural part of the process.
Fostering psychological safety
When the dev team moved to a trio of two developers and a tester to implement testability, they found psychological safety by accident. Once the dev lead had modelled how the group could work together, the trio were pretty much left alone. Now this group, by chance, appeared just to be more open about what they did and didn’t know, asking for help and asking to be left alone too when they needed space to think and work through problems alone, always knowing that the other team members were on hand if they needed support.
One act that solidified safety in the trio was when the tester within the trio called a meeting between the two devs and the dev lead. They felt that the trio was going too slow and that they were the reason for the slowdown. They thought they couldn’t pick up the skills fast enough and should either revert to the old way of working or find another, more competent tester. The dev lead laughed and said he thought they were announcing they were leaving. He reassured them that the trio were going at the right pace and that they should keep going just as they were. This showed everyone in the group that it’s ok to go slow and take our time picking up the new skills and that leadership will support them.
While this group found psychological safety by accident, there are things that leadership can do to be more deliberate about creating an environment considered high in psychological safety, such as by starting with the basics and ensuring leadership knows what psychological safety means. Many assume they know it and typically associate it with high trust and creating safe spaces. But psychological safety is all about people being willing to take interpersonal risks, which will mean getting uncomfortable. A safe space, to my mind, is quite a bit different, which is all about staying in your comfort zone without fear of being pushed or challenged by questions or ideas.
Trust is another misconception about psychological safety. The logic is that if we all just trusted each other, people would be more willing to speak up and take interpersonal risks. Now while I agree trust does play a part, if we equate psychological safety to another form of trust, we risk missing the bigger picture. Psychological safety is a group phenomenon about how willing people are to take interpersonal risks at a particular moment. In contrast, trust is between individuals or groups, which is the firm belief in the reliability, truth or ability of the other person or group to carry out some future action. Psychological safety is about the present state, while trust is about the future.
From that foundation, leadership will be in a much better position to model behaviours to demonstrate how to take interpersonal risks and show that leaders will not punish people for doing so. Behaviours include showing fallibility, sharing their mistakes, and encouraging others to share theirs, but also to demonstrate that leadership doesn’t have all the answers and that it is imperative that people share what they do and don’t know.
Leadership must adopt three core mindsets to encourage interpersonal risk-taking. These are curiosity that there is always more to learn, humility that we don’t have all the answers, and empathy in that taking risks is complicated and needs to be nurtured.
Celebrating the success and failures of experimentation
Jeff Bezoes once said, “It’s not an experiment if you know it’s going to work”, and I think he was getting to a fascinating point.
We all assume that we know how to run experiments and that people will work this way. But this isn’t always the case. People naturally believe failure is bad and should be avoided at all costs, but failure is a natural part of learning and risk-taking. An organisation should actively encourage teams to experiment by providing them with structures they can adopt and adapt for their use cases.
In addition, experimentation should be celebrated, not just successes but failures too. People should be actively rewarded for experimenting, failing and sharing what they’ve learned if we want people to push into the unknown, take risks and create new ways of working.
Building in testability often sounds like a technical problem, and teams just need to figure out how to isolate their system into modules and build in testing around those modules. While this is partly true, those tests are almost useless if team members don’t trust them. Also, if leadership is absent, team members will default away from collaboration and towards self-protection, which can typically look like each person working in isolation on their part of the development lifecycle, with everyone hoping it will all come together at the end.
One way to build that trust is by developers and testers collaborating to develop those tests together. But to collaborate like this, leadership needs to model behaviours that show that team members can take interpersonal risks and experiment with their ways of working.