Month: October 2022
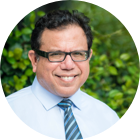
MMS • RSS
Posted on mongodb google news. Visit mongodb google news
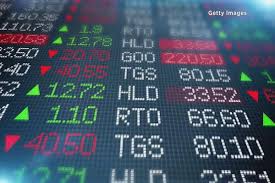
MongoDB Inc. (NASDAQ:MDB)’s traded shares stood at 1.34 million during the last session, with the company’s beta value hitting 1.14. At the close of trading, the stock’s price was $191.00, to imply an increase of 3.37% or $6.23 in intraday trading. The MDB share’s 52-week high remains $590.00, putting it -208.9% down since that peak but still an impressive 12.77% since price per share fell to its 52-week low of $166.61. The company has a valuation of $13.78B, with an average of 1.63 million shares in intraday trading volume over the past 10 days and average of 1.73 million shares over the past 3 months.
After registering a 3.37% upside in the last session, MongoDB Inc. (MDB) has traded red over the past five days. The stock hit a weekly high of 197.97 this Thursday, 10/20/22, jumping 3.37% in its intraday price action. The 5-day price performance for the stock is 5.66%, and -8.81% over 30 days. With these gigs, the year-to-date price performance is -63.92%. Short interest in MongoDB Inc. (NASDAQ:MDB) saw shorts transact 3.87 million shares and set a 2.34 days time to cover.
MongoDB Inc. (MDB) estimates and forecasts
Looking at statistics comparing MongoDB Inc. share performance against respective industry, we note that the company has outperformed competitors. MongoDB Inc. (MDB) shares are -49.45% down over the last 6 months, with its year-to-date growth rate higher than industry average at 45.76% against 2.20%. Revenue is forecast to shrink -16.70% this quarter before falling -27.30% for the next one. The rating firms project that company’s revenue will grow 36.40% compared to the previous financial year.
Revenue forecast for the current quarter as set by 16 analysts is $282.4 million. Meanwhile, for the quarter ending Oct 2022, a total of 16 analyst(s) estimate revenue growth to $294.85 million.
MDB Dividends
MongoDB Inc. has its next earnings report out between December 05 and December 09. However, it is important to take into account that this dividend yield ratio is just an indicator to only serve the purpose of guidance. Investors interested to invest in the stock should ponder company’s other fundamental and operations related aspects too. MongoDB Inc. has a forward dividend ratio of 0, with the share yield ticking at 0.00% to continue the rising pattern observed over the past year. The company’s average dividend yield trailing the past 5-year period is 0.00%.
MongoDB Inc. (NASDAQ:MDB)’s Major holders
MongoDB Inc. insiders hold 3.68% of total outstanding shares, with institutional holders owning 90.86% of the shares at 94.33% float percentage. In total, 90.86% institutions holds shares in the company, led by Price (T.Rowe) Associates Inc. As of Mar 30, 2022, the company held over 8.23 million shares (or 12.09% of shares), all amounting to roughly $3.65 billion.
The next major institution holding the largest number of shares is Capital World Investors with 6.45 million shares, or about 9.46% of shares outstanding. As of the market price on Mar 30, 2022, these shares were worth $2.86 billion.
We also have Growth Fund Of America Inc and Vanguard Total Stock Market Index Fund as the top two Mutual Funds with the largest holdings of the MongoDB Inc. (MDB) shares. Going by data provided on Jun 29, 2022, Growth Fund Of America Inc holds roughly 4.62 million shares. This is just over 6.78% of the total shares, with a market valuation of $1.2 billion. Data from the same date shows that the other fund manager holds a little less at 1.83 million, or 2.69% of the shares, all valued at about 812.81 million.
Article originally posted on mongodb google news. Visit mongodb google news
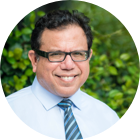
MMS • RSS
Posted on nosqlgooglealerts. Visit nosqlgooglealerts
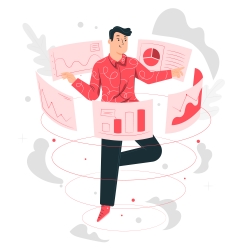
TigerGraph, provider of the leading advanced analytics and ML platform for connected data, today announced its commitment to support openCypher, a popular query language for building graph database applications. Developers can now access a limited preview translation tool to learn how openCypher support will appear in TigerGraph’s
flagship graph query language, GSQL. Support for openCypher will give developers more choice to build or migrate graph applications to TigerGraph’s scalable, secure, and fully managed graph database platform.
“Our mission at TigerGraph has always been to bring the power of graph to all, and our support for openCypher helps us do just that. We are empowering enterprises to accelerate their graph technology adoption via our advanced graph analytics and ML platform,” said Yu Xu, CEO and founder of TigerGraph. “With openCypher support, we are pushing the bounds of graph innovation and arming developers with another way to adopt and expand their use of graph to
find competitive insights in their data.”
Developers who are familiar with openCypher can now learn how to harness the power of TigerGraph to achieve superior performance and scalability for in-database computation and analysis of relationships within their data. With access to TigerGraph’s sophisticated GSQL language, developers will experience several benefits, including:
-
More expressive: Advanced, powerful queries covering advanced graph algorithms, including 55+ algorithms available in TigerGraph’s Data Science Library coded in GSQL
-
More performant: With easy optimization, queries run at a faster speed to take advantage of the built-in parallel processing power of the underlying engine
For example, PageRank is a popular graph algorithm used to calculate the relative importance of a web page based on how different pages reference each other. The PageRank algorithm can be coded with only 10 lines of GSQL code across all sizes of data, while openCypher can only code a portion of the algorithm.
The openCypher-to-GSQL translation tool is now available to the openCypher developer community to access a side-by-side comparison of openCypher queries and the equivalent GSQL queries with proposed openCypher syntax support. With this limited preview, developers are encouraged to learn how openCypher will appear in GSQL and provide valuable feedback to guide the incremental buildout of full support features. Those who want to learn advanced GSQL can now access TigerGraph’s industry-leading offerings, including its distributed computing database, in-database machine learning workbench, and graph data science library, which consists of more than 55 graph algorithms.
In addition, TigerGraph’s openCypher support aligns with support for industry standard GQL. TigerGraph serves on the ISO steering committee that is developin g GQL, the new international standard query language that will be available in early 2024.
Oracle Brings Database Innovations to Simplify Development and Enhance Protection of …
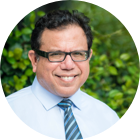
MMS • RSS
Posted on mongodb google news. Visit mongodb google news
Oracle Database 23c Beta includes a new approach for addressing the object-relational mismatch for application developers
Enhancements to APEX low-code application development provide a better native mobile user experience
Oracle today announced Oracle Database 23c Beta, the latest version of the world’s leading converged database, supporting all data types, workloads, and development styles. Oracle Database 23c, code named “App Simple,” focuses on simplifying applications and development. Many additional innovations across Oracle’s database services and products portfolio extend Oracle’s leadership in performance, security, and availability for mission-critical workloads.
Oracle Database 23c “App Simple” delivers advanced new capabilities that enable breakthrough developer productivity for applications that are written using JSON, Graph, or microservices, while also enhancing SQL to make it even easier to use and adding JavaScript as a stored procedure language. For example, Oracle Database 23c introduces a groundbreaking new approach called JSON Relational Duality for addressing the mismatch between how applications represent data versus how relational databases store data. JSON Relational Duality simplifies application development by allowing data to be simultaneously used as application-friendly JSON documents and as database-friendly relational tables.
“At Oracle, our primary goal remains to help the developers achieve maximum innovation and ROI along with limited time investment. With the rising importance of developers, especially in India, we understand that leveraging single purpose database engines are not the most efficient way to build modern apps and developers need a more effective platform to ensure innovation. To resolve that, our latest announcement will help developers create modern application of any scale and criticality along with simplifying data driven app development with more flexibility, efficiency and performance.” said P Saravanan, Vice-President, of Cloud Engineering, Oracle India
He added “We are also making it easier for existing MongoDB users to benefit from Oracle Database’s performance, scale and availability by offering on-premises support for Oracle Database API for Mongo DB and Oracle Golden Gate’s ability to provide zero downtime migration and real time replication from MongoDB.”
“Modern applications are built using new types of data such as JSON and Graph, new types of analytics such as machine learning, and new development styles such as microservices. The breadth and depth of data technologies used by modern applications can make developing and running apps increasingly complex,” said Juan Loaiza, executive vice president, mission-critical database technologies, Oracle. “Oracle Database 23c ‘App Simple’ introduces game changing new technologies that make it dramatically easier to develop and run these modern apps.”
To enhance data protection for mission-critical Oracle Database services on Oracle Cloud Infrastructure (OCI), Oracle also announced Oracle Database Zero Data Loss Autonomous Recovery Service, enabling organizations to address the challenges of ransomware, outages, and human errors more effectively. In addition, Oracle introduced Oracle Full Stack Disaster Recovery Service which allows customers to configure, monitor, and manage the disaster recovery process for the full stack of technologies used to build applications—including middleware, databases, networks, storage, and compute from the OCI console.
Industry analyst commentary
“JSON Relational Duality in Oracle Database 23c brings substantial simplicity and flexibility to modern app dev,” said Carl Olofson, research vice president, Data Management Software, IDC. “It addresses the age-old object—relational mismatch problem, offering an option for developers to pick the best storage and access formats needed for each use case without having to worry about data structure, data mapping, data consistency, or performance tuning. No other specialized document databases offer such a revolutionary solution.”
“With over 300 new features and enhancements, including JSON Relational Duality, Operational Graphs, Microservices support, real-time machine learning and support for new data types, the next generation Oracle Database 23c is poised to gain app developer mindshare and make it extremely simple to develop and run data-driven mission-critical apps,” said Holger Mueller, vice president and principal analyst, Constellation Research. “Clearly Oracle has delivered on the ‘App Simple’ code name of its latest Database 23c, and it’ll undoubtedly be a ‘must see’ debut at CloudWorld.”
“Oracle Database 23c more than lives up to its code name—App Simple—by taking application development to unprecedented levels of task reduction, simplification, and automation,” said Marc Staimer, senior analyst, Wikibon. “Oracle Database 23c definitively ends the long running ‘relational vs. document’ debate with JSON Relational Duality delivering the best of both worlds. Data is stored as rows in relational format, while data can be accessed as JSON formats. Developers can operate on the same data without having to worry about data structure, data mapping, data consistency, or performance tuning.”
Developer productivity improvements
- Oracle Database 23c brings new functionality to assist developers in building the next generation of mission-critical, high-performance database applications. As the only complete and simple converged database for developers, data engineers, and DBAs, Oracle Database 23c includes JSON Relational Duality, JavaScript stored procedures, property graph analysis of operational data, automated handling of distributed microservices transactions (known as sagas), enhanced Automatic Materialized Views, real-time SQL Plan Management, True Cache, ML-enhanced prediction of data statistics for optimizing SQL execution, and native replication of database shards. Additional functionality includes the ability to enable Kafka applications to run directly against Oracle Database and protection from unauthorized SQL via any execution path using the new SQL Firewall embedded in the Oracle Database. It is now available in beta globally for Oracle customers who complete the beta sign-up process.
- Oracle Database API for MongoDB now provides MongoDB compatibility to Oracle
Database for on-premises environments. The API enables MongoDB developers to build and run new MongoDB applications on Oracle Database using the MongoDB tools, drivers, and frameworks that they are familiar with, as well as the ability to migrate existing MongoDB workloads to Oracle Database without modifying their applications. - GoldenGate 23c is certified with Oracle Database 23c and previous versions, and introduces new features that improve usability, performance, diagnostics, and security. Highlights include faster JSON replication performance, new replication support for blockchain and immutable tables, and application patching without downtime using Edition-Based Redefinition. In addition, OCI GoldenGate now supports 40+ new data connections from Oracle and non-Oracle sources across multicloud environments, including AWS and Azure, and new Stream Analytics for continuous data integration and data-in-motion analytics.
- GoldenGate Free enables prospects, customers, developers, and students to use GoldenGate and its new user experience and entirely automated replication lifecycle for free. It is designed for development, devops, test, and production source or target databases of 20GB or less on OCI, on other clouds, or on-premises.
- Autonomous Data Warehouse introduces new capabilities enabling organizations to improve collaboration across teams by sharing data with the open-source Delta Sharing protocol and business models using in-database Analytic Views. Along with existing built-in support for Oracle Analytics and tools such as Tableau, a new Microsoft Excel add-in and a complete and embedded data integration tool with Transforms will be available. In addition, new Oracle Application Accelerators for Oracle E-Business Suite provide ready-to-use data models, KPIs, and data integration.
- Oracle APEX 22.2 (preview) strengthens its position in low-code application development by introducing enhancements to progressive web apps to provide a virtually native mobile user experience. Also available is a new workflow approval component for integrating task management into APEX apps. In addition, developers now have access to out-of-the-box integrations with 3rd-party apps and data, providing a richer application development platform. Oracle APEX is a fully supported, no-additional-cost feature of Oracle Database and Oracle Autonomous Database, as well as a developer service on OCI.
- Transaction Manager for Microservices Free enables use of distributed transactions in microservices-based applications deployed in Kubernetes. With Transaction Manager for Microservices, customers can create a global transaction that includes multiple microservices developed in various programming languages and on different application platforms. Transaction Manager for Microservices is now free for use by prospects, customers, developers, and students.
- Tuxedo 22c provides enhancements for deploying Tuxedo applications (written in C/C++ or COBOL) in Kubernetes and cloud environments, as well as XA transaction interoperability with microservices deployed in Kubernetes using Oracle Transaction Manager for Microservices. It includes ready-to-use container images, sample Helm charts for various Kubernetes distributions, integration with native Kubernetes tools and environments, new HA enhancements, and stronger security. Used together, the combination of Tuxedo 22c and Transaction Manager for Microservices accelerates the mainframe modernization initiatives underway at many large enterprises.
Continuous protection of mission-critical databases
- Oracle Database Zero Data Loss Autonomous Recovery Service provides secure backup and fast, predictable recovery for Oracle Database services and Autonomous Database running on OCI. It uses database-aware intelligence and automation to help protect transactions as they occur, reduces overhead on operational database services, validates database recoverability, and automates both backup and recovery processes. With this new service, organizations can help mitigate the impact of ransomware, outages, and human errors by restoring databases to the point-in-time just before the attack, outage, or error occurred.
- OCI Full Stack Disaster Recovery Service allows customers to monitor and manage the entire disaster recovery process of their entire technology stack from the OCI console. With a single click, it manages disaster recovery for applications, middleware, networks, storage, and compute for a variety of disaster recovery topologies. It offers intelligent features to quickly create cross-regional relationships, DR plans, and performs comprehensive checks before a disaster recovery plan is executed to ensure success in the standby region.
New Oracle Autonomous Database support for applications
- Oracle E-Business Suite is certified to run on the Oracle Autonomous Database service on OCI, enabling organizations to further reduce database administration, optimize resource utilization, and lower costs.
Additional Resources
Article originally posted on mongodb google news. Visit mongodb google news
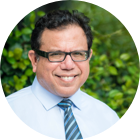
MMS • Liz Rice
Article originally posted on InfoQ. Visit InfoQ
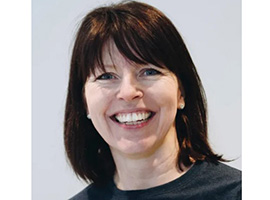
Transcript
Rice: My name is Liz Rice. I’m the Chief Open Source Officer at Isovalent. I’m also an ambassador and board member for OpenUK. Until recently, I was chair of the Technical Oversight Committee at the Cloud Native Computing Foundation. I joined Isovalent just over a year ago, because that team has so much expertise in eBPF, which is the technology that I’m talking about. I’ve been excited about eBPF for a few years now. From my CNCF work, I’ve seen some of the really incredible range of things that eBPF can enable. I want to share some of the reasons why I’m so excited about it, and specifically talk about the ways that eBPF can help us build more resilient deployments.
What is eBPF?
Before we get to that, let’s talk about what eBPF is. The acronym stands for Extended Berkeley Packet Filter. I don’t think that’s terribly helpful. What you really need to know is that eBPF allows you to run custom code in the kernel. It makes the kernel programmable. Let’s just pause for a moment and make sure we’re all on the same page about what the kernel is. Kernel is a core part of your operating system, which is divided into user space and the kernel. We typically write applications that run in user space. Whenever those applications want to interface with hardware in any way, whether they want to read or write to a file, send or receive network packets, accessing memory, all these things require privileged access that only the kernel has. User space applications have to make requests of the kernel whenever they want to do any of those things. The kernel is also looking after things like scheduling those different applications, making sure that multiple processes can run at once.
Normally, we’re writing applications that run in user space. eBPF is allowing us to write kernels that run within the kernel. We load the eBPF program into the kernel, and we attach it to an event. Whenever that event happens, it’s going to trigger the eBPF program to run. Events can be all sorts of different things. It could be the arrival of a network packet. It could be a function call being made in the kernel or in user space. It could be a trace point. It could be a perf event. There are lots of different places where we can attach eBPF programs.
eBPF Hello World
To make this a bit more concrete, I’m going to show an example here. This is going to be the Hello World of eBPF. Here is my eBPF program. The actual eBPF program are these few lines here. They’re written in C, the rest of my program is in Python. My Python code here is going to actually compile my C program into BPF format. All my eBPF program is going to do is write out some tracing here, it’s going to say hello QCon. I’m going to attach that to the event of the execve system call being made. Execve is used to run a new executable. Whenever a new executable runs, execve is what causes it to run. Every time a new executable started on my virtual machine, that’s going to cause my tracing to be printed out.
If I run this program, first of all, we should see we’re not allowed to load BPF unless we have a privilege call CAP BPF which is typically only reserved for root. We need super-user privileges to run. Let’s try that with sudo. We start seeing a lot of these trace events being written out. I’m using a cloud VM. I’m using VS Code remote to access it. That it turns out is running quite a lot of executables. In a different shell, let’s run something, let’s run ps. We can see the process ID, 1063059. Here is the trace line that was triggered by me running that ps executable. We can see in the trace output, we don’t just get the text, we’re also getting some contextual information about the event that triggered that program to run. I think that’s an important part of what eBPF is giving us. We get this contextual information that could be used to generate observability data about the events that we’re attached to.
eBPF Code Has to be Safe
When we load an eBPF program into the kernel, it is crucial that it’s safe to run. If it crashes, that would bring down the whole machine. In order to make sure that it is safe, there’s a process called verification. As we load the program into the kernel, the eBPF verifier checks that the program will run to completion. That it never dereferences a null pointer. That all the memory accessing that it will do is safe and correct. That ensures that the eBPF programs we’re running won’t bring down our machine and that they’re accessing memory correctly. Because of this verification process, sometimes eBPF is described as being a sandbox. I do want to be clear that this is a different kind of sandboxing from containerization, for example.
Dynamically Change Kernel Behavior
What eBPF is allowing us to do is run custom programs inside the kernel. By doing so, we’re changing the way that the kernel behaves. This is a real game changer. In the past, if you wanted to change the Linux kernel, it takes a long time. It requires expertise in kernel programming. If you make a change to the kernel, it then typically takes several years to get from the kernel into the different Linux distributions that we all use in production. It can be quite often five years between a new feature in the kernel arriving in your production deployments. This is why eBPF has suddenly become such a prevalent technology. As of the last year or so, almost all production environments are running Linux kernels that are new enough to have eBPF capabilities in them. That means pretty much everyone can now take advantage of eBPF and that’s why you’ve suddenly seen so many more tools using it. Of course, with eBPF, we don’t have to wait for the Linux kernel to be rolled out. If we can create a new kernel capability in an eBPF program, we can just load it into the machine. We don’t have to reboot the machine. We can just dynamically change the way that that machine behaves. We don’t even have to stop and restart the applications that are running, the changes affect the kernel immediately.
Resilience to Exploits – Dynamic Vulnerability Patching
We can use this for a number of different purposes, one of which is for dynamically patching vulnerabilities. We can use eBPF to make ourselves more resilient to exploits. One example that I like of this dynamic vulnerability patching is being resilient to packets of death. A packet of death is a packet that takes advantage of a kernel vulnerability. There have been a few of these over time where the kernel doesn’t handle a packet correctly. For example, if you put a length field into that network packet that’s incorrect, maybe the tunnel doesn’t handle it correctly and perhaps it crashes, or bad things happen. This is pretty easy to mitigate with eBPF, because we can attach an eBPF program to the event that is the arrival of a network packet. We can look at the packet, see if it is formed in the way that would exploit this vulnerability, the packet of death. Is it the packet of death? If it is, we can just discard that packet.
Example – eBPF Packet Drop
As an example of how easy this is, I’m just going to show another example of a program that will drop network packets of a particular form. In this example, I’m going to look for ping packets. That’s the protocol ICMP. I can drop them. Here’s my program. Don’t worry too much about the details here, I’m essentially just looking at the structure of the network packets. I’m identifying that I’ve found a ping packet. For now, I’m just going to allow them to carry on. XDP_PASS means just carry on doing whatever you would have done with this packet. That should emit whatever tracing you get. This is actually a container called pingbox. I’m going to start sending pings to that address and they’re being responded to. We can see the sequence number here ticking up nicely. At the moment, my eBPF program is not loaded. I’m going to run a makefile that will compile my program, clean up any previous programs attached to this network interface, and then load my program. There’s make running the compile, and then attaching to the network interface eth0 here. You see immediately it’s started tracing out, Got ICMP packet. That hasn’t affected the behavior, and my sequence numbers are still just ticking up as before.
Let’s change this to say, drop. We’ll just make that. What we should see is the tracing here is still being generated. It’s continuing to receive those ping packets. Those packets are being dropped, so they never get responded to. On this side here, the sequence numbers have stopped going up, because we’re not getting the response back. Let’s just change it back to PASS, and make it again. We should see, there’s my sequence numbers, there were 40 or so packets that were missed out, but now it’s working again. What I hope that illustrates is, first of all, how we can attach to a network interface and do things with network packets. Also, that we can dynamically change that behavior. We didn’t have to stop and start ping. We didn’t have to stop and start anything. All we were doing was changing the behavior of the kernel live. I was illustrating that as an illustration of how handling packet of death scenarios would work.
Resilience to Exploits – BPF Linux Security Module
We can be resilient to a number of other different exploits using BPF Linux security modules. You may have come across Linux security modules, such as AppArmor, or SELinux. There’s a Linux security module API in the kernel which gives us a number of different events that something like AppArmor can look at and decide whether or not that event is in or out of policy and either allow or disallow that particular behavior to go ahead. For example, allowing or disallowing file access. We can write BPF programs that attach to that same LSM API. That gives us a lot more flexibility, a lot more dynamic security policies. As an example of that, there’s an application called Tracee, that’s written by my former colleagues at Aqua, which will attach to LSM events and decide whether they are in or out of policy.
Resilience to Failure – Superfast Load Balancing
We can use eBPF to help us be resilient to exploits. What other kinds of resiliency can we enable with eBPF? One other example is load balancing. Load balancing can be used to scale requests across a number of different backend instances. We often do it not just for scaling, but also to allow for resilience to failure, high availability. We might have multiple instances so that if one of those instances fails in some way, we still have enough other instances to carry on handling that traffic. In that previous example, I showed you an eBPF program attached to a network interface, or rather, it’s attached to something called the eXpress Data Path of a network interface. eXpress Data Path is very cool, in my opinion. You may or may not have a network card that allows you to actually run the XDP program, so run the eBPF program on the hardware of your network interface card. XDP is run as close as possible to that physical arrival of a network packet. If your network interface card supports it, it can run directly on the network interface card. In that case, the kernel’s network stack would never even see that packet. It’s blazingly fast handling.
If the network card doesn’t have support for it, the kernel can run your eBPF program, again, as early as possible on receipt of that network packet. Still super-fast, because there’s no need for the packet to traverse the network stack, certainly never gets anywhere near being copied into user space memory. We can process our packets very quickly using XDP. We can make decisions like, should we redirect that packet. We can do layer-3, layer-4 load balancing in the kernel incredibly quickly, possibly not even in the kernel, possibly on a network card to decide whether or not we should pass this packet on up to the network stack and through to user space on this machine. Or perhaps we should be load balancing off to a different physical machine altogether. We can redirect packets. We can do that very fast. We can use that for load balancing.
The Kube-proxy
Let’s just briefly turn our thoughts to Kubernetes. In Kubernetes, we have a load balancer called the kube-proxy. The kube-proxy balances or allows load balancing or tells pod traffic how to reach other pods. How can a message from one pod get to another pod? It acts as a proxy service. What is a proxy if not essentially a load balancer? With eBPF we have the option not just to attach to the XDP interface close to the physical interface as possible. We also have the opportunity to attach to the socket interface, so as close to the application as possible. Applications talk to networks through the socket interface. We can attach to a message arriving from a pod and perhaps bypass the network stack because we know we want to send it to a pod on a different machine, or we can bypass the network stack and loop straight back to an application running on the same physical machine or the same virtual machine. By intercepting packets as early as possible, we can make these load balancing decisions. We can avoid having to go through the whole kernel’s network stack, and it gives us some incredible performance improvements. Kube-proxy replacement performance compared to an iptables based Kube-proxy can be dramatically quicker.
eBPF Enables Efficient Kubernetes-Aware Networking
I want to now dive a little bit more into why eBPF can enable this really efficient networking particularly in Kubernetes. In that previous diagram, I just showed the kernel network stack as one box. Networking stack is pretty complicated. Typically, a packet going through the kernel’s network stack goes through a whole bunch of different steps and stages, as the kernel decides what to do with it. In Kubernetes, we have not just the networking stack on the host, but we typically run a network namespace for every pod. Each pod, by having its own network namespace has to run its own networking stack. Imagine a packet that arrives on the physical eth0 interface. It traverses the whole kernel’s networking stack to reach the virtual Ethernet connection to the pod where it’s destined to go. Then it goes through the pod’s networking stack to reach the application via a socket. If we use eBPF, and particularly if we know about Kubernetes identities and addresses, we can bypass that stack on the host. When we receive a packet on that eth0 interface, if we already know whether that IP address is associated with a particular pod, we can essentially do a lookup and just pass that packet straight to the pod where it then goes through the pod’s networking stack, but doesn’t have to go through all the complication of everything happening on the host’s networking stack.
Using an eBPF enabled networking interface for Kubernetes like Cilium, we can enable this network stack shortcutting because we’re aware of Kubernetes identities. We know what IP addresses are associated with which pods but also which pods are associated with which services, with namespaces. With that knowledge, we can build up these service maps showing how traffic is flowing between different components within our cluster. eBPF is giving us visibility into the packet. We can see, not just the destination IP address and port, we can route through a proxy to find out what HTTP type of request it is. We can associate that flow data with Kubernetes identities.
In a Kubernetes network, IP addresses change all the time, pods come and go. An IP address one minute may mean one thing, and two minutes later, it means something completely different. IP addresses are not terribly helpful for understanding the flows within a Kubernetes cluster. Cilium can map those IP addresses to the correct pod, the correct service at any given point in time and give you much more readable information. It is measurably faster. Whether you’re using Cilium or other implementations of eBPF networking, that ability to get the networking stack on the host gives us measurable performance improvements. We can see here that the blue line on the left is the request-response rate for number of requests per second that we can achieve without any containers at all, just directly sending and receiving traffic between nodes. We can get performance that’s nearly as fast using eBPF. Those yellow and green lower bars in the middle show us what happens if we don’t use eBPF, and we use the legacy host routing approach through the host network stack, it’s measurably slower.
eBPF Network Policy Decisions
We can also take advantage of having that knowledge of Kubernetes identities and the ability to drop packets to build very efficient network policy implementations. You saw how easy it was to drop packets. Rather than just inspecting the packet and deciding that it was a ping packet, can compare the packet to policy rules and decide whether or not they should be forwarded or not. This is quite a nice tool that we have. You can find this at networkpolicy.io to visualize Kubernetes network policies. We talked about load balancing, and how we can use load balancing within a Kubernetes cluster in the form of kube-proxy. After all, Kubernetes gives us a huge amount of resiliency. If an application pod crashes, it can be recreated dynamically without any operator intervention. We can scale automatically without operator intervention.
Resilience to Failure – ClusterMesh
What about the resiliency of the cluster as a whole, if your cluster is running in a particular data center and you lose connectivity to that data center? Typically, we can use multiple clusters. I want to show how eBPF can make the connectivity between multiple clusters really very straightforward. In Cilium, we do this using a feature called ClusterMesh. With ClusterMesh, we have two Kubernetes clusters. The Cilium agent running in each cluster will read a certain amount of information about the state of other clusters in that ClusterMesh. Each cluster has its own database of configuration and state stored in etcd. We run some etcd proxy components that allow us to just find out about the multi-cluster specific information that we need, so that the Cilium agents on all the clusters can share that multi-cluster state.
What do I mean by multi-cluster state? Typically, this is going to be about creating highly available services. We might run multiple instances of a service on multiple clusters to make them highly available. With ClusterMesh, we simply mark a service as global, and that connects them together such that a pod accessing that global service can access it on its own cluster, or on a different cluster, should that be necessary. I think this is a really nice feature of Cilium, and remarkably easy to set up. If the backend pod on one cluster is destroyed for some reason, or indeed if the whole cluster goes down, we still have the ability to route requests from other pods on that cluster to backend pods on a different cluster. They can be treated as a global service.
I think I have an example of this. I have two clusters. My first cluster is up, we can see cm-1, standing for ClusterMesh 1, and a second cluster, cm-2. They are both running some pods. We quite often in Cilium do some demos with a Star Wars theme. In this case, we have some X-wing fighters that want to be able to communicate with the Rebel base. We also have some similar X-wings and Rebel bases on the second cluster. Let’s just take a look at the services. In fact, let’s describe that Rebel base, service rebel-base. You can see it’s annotated by Cilium as a global service. It’s been annotated by me as part of the configuration to say I want this to be a global service. The same is true if I look on the second cluster there. They’re both described as global. What that means is, I can issue requests from an X-wing on either cluster, and it will receive responses from a load balanced across those two different clusters, across backends on those two different clusters. Let’s try that. Let’s run it in a loop. Let’s exec into an X-wing. It doesn’t really matter which X-wing. We want to send a message to the Rebel base. Hopefully, what we should see is, we’re getting responses from sometimes it’s cluster 1, sometimes it’s cluster 2, at random.
What if something bad were to happen to the Rebel base pods on one of those clusters? Let’s see which nodes are on the code. Let’s delete the pods on cluster 2. In fact, I’ll delete the whole deployment of Rebel base on the second cluster. What we should see is that all the requests are now handled by cluster 1. Indeed, you can see, it’s been cluster 1 now for quite some time. That resiliency where we literally just have to mark our services as global, it’s an incredibly powerful way of enabling that multi-cluster high availability.
Visibility into Failures – eBPF Observability Instrumentation
Lest I give you the impression that eBPF is just about networking, and advantages in networking, let me also talk a bit about how we can use eBPF for observability. Which is, after all, incredibly important, if something does go wrong. We need observability so that we can understand what happened. In a Kubernetes cluster, we have a number of hosts, and each host has only one kernel. However many user space applications we’re running, however many containers we’re running, they’re all sharing that one kernel per host. If they’re in pods, still only one kernel however many pods there are. Whenever those applications in pods want to do anything interesting, like read or write to a file, or send or receive network traffic, whenever Kubernetes wants to create a container. Anything complicated involves the kernel. The kernel has visibility and awareness of everything interesting that’s happening across the entire host. That means if we use eBPF programs to instrument the kernel, we can be aware of everything happening on that whole host. Because we can instrument pretty much anything that’s happening in the kernel, we can use it for a wide variety of different metrics and observability tools, different kinds of tracing, they can all be built using eBPF.
As an example, this is a tool called Pixie, which is a CNCF sandbox project. It’s giving us with this flamegraph, information about what’s running across the entire cluster. It’s aggregating information from eBPF programs running on every node in the cluster to produce this overview of how CPU time is being used across the whole cluster with detail into specific functions that those applications are calling. The really fun thing about this is that you didn’t have to make any changes to your application, you don’t have to change the configuration even to get this instrumentation. Because as we saw, when you make a change in the kernel, it immediately affects whatever happens to be running on that kernel. We don’t have to restart those processes or anything.
This also has an interesting implication for what we call the sidecar model. In a lot of ways, eBPF gives us a lot more simplicity compared to the sidecar model. In the sidecar model, we have to inject a container into every pod that we want to instrument. It has to be inside the pod because that’s how one user space application can get visibility over other things that are happening in that pod. It has to share namespaces with that pod. We have to inject that sidecar into every pod. To do that, that requires some YAML be introduced into the definition of that pod. You probably don’t write that YAML by hand to inject the sidecar. It’s probably done perhaps in admission control or as part of a CI/CD process, something will likely automate the process of injecting that sidecar. Nevertheless, it has to be injected. If something goes wrong with that process, or perhaps you didn’t mark a particular pod as being something you want to instrument, if it doesn’t happen, then your instrumentation has no visibility into that pod.
On the other hand, if we use eBPF, we’re running our instrumentation within the kernel, then we don’t need to change the pod definition. We’re automatically getting that visibility from the kernel’s perspective, because the kernel can see everything that’s happening on that host. As long as we add eBPF programs onto every host, we will get that comprehensive visibility. That also means that we can be resilient to attacks. If somehow our host gets compromised, if someone manages to escape a container and get on to the host, or even if they run a separate pod somehow, your attacker is probably not going to bother instrumenting their processes and their pods with your observability tools. If your observability tools are running in the kernel, they will be seen regardless. You can’t hide from tooling that’s running in the kernel. This ability to run instrumentation without sidecars is creating some really powerful observability tools.
Resilient, Observable, Secure Deployments – Sidecarless Service Mesh
It also takes us to the idea of a sidecarless service mesh. Service mesh is there to be resilient and observable and secure. Now with eBPF, we can implement service mesh without the use of sidecars. I showed before the diagram showing how we can bypass the networking stack on the host using eBPF. We can take that another step further for service mesh. In the traditional sidecar model, we run a proxy, perhaps it’s Envoy, inside every pod that we want to be part of the service mesh. Every instance of that proxy has routing information, and every packet has to pass through that proxy. You can see on the left-hand side of this diagram, the path for network packets is pretty torturous. It’s going through essentially five instances of the networking stack. We can dramatically shortcut that with eBPF. We can’t always avoid a proxy. If we are doing something at layer-7, we need that proxy, but we can avoid having a proxy instance inside every pod. We can be much more scalable by having far fewer copies of routing information and configuration information. We can bypass so many of those networking steps through eBPF connections at the XDP layer within the networking stack, or at the socket layer. eBPF will give us service mesh that’s far less resource hungry, that’s much more efficient. I hope that’s given a flavor of some of the things that I think eBPF is enabling around networking, observability, and security, that’s going to give us far more resilient and scalable deployments.
Summary
I’ve pretty much been talking about Linux so far. It is also coming to Windows. Microsoft have been working on eBPF on Windows. They’ve been part, alongside Isovalent and a number of other companies that are interested in massively scalable networks. We’ve come together to form the eBPF Foundation, which is a foundation under the Linux Foundation, really to take care of eBPF technology across different operating systems. I hope that gives a sense of why eBPF is so important, and it’s so revolutionary for resilient deployments of software, particularly in the cloud native space, but not necessarily limited to. Regardless of whether you’re running Linux or Windows, there are eBPF tools to help you optimize those deployments and make them more resilient.
Resources
You can find more information about eBPF at the ebpf.io site, and Cilium is at cilium.io. There’s also a Slack channel that you’ll find from both of those sites, where you’ll find experts in Cilium and in eBPF. Of course, if you want to find out more about what we do at Isovalent, please visit isovalent.com.
Questions and Answers
Watt: Which companies are using Cilium in production at the moment that you’re seeing and know about?
Rice: We’ve actually got a list in the Cilium GitHub repo of users who have added themselves to the list of adopters. There are certainly dozens of them. There’s companies using it at significant scale. Bell Canada, for example, using it in telco, Adobe, Datadog, these are just a few examples of companies that I know I can speak about publicly. It’s pretty widely adopted.
Watt: It’s certainly one of the technologies on the up and coming road. I think the fact that there are already some big players in the market that are already using this, is testament I think to where it’s going.
Rice: The other two integrations to really mention, the Dataplane V2 in GKE is actually based on Cilium. Amazon chose Cilium as the networking CNI for their EKS Anywhere distribution. I feel like that’s a very strong vote of confidence in Cilium as a project and eBPF as a technology.
Watt: One of the areas we’re looking at on the track is around chaos engineering, and that side of things. How do you see eBPF potentially helping out or providing ways to do different things from a chaos engineering perspective?
Rice: I think this is something that we just touched on, about having eBPF programs running in the kernel and potentially changing events, that could be a really great way of triggering chaos tests. For example, if you wanted to drop some percentage of packets and see how your system behaved, or insert errors, all manner of disruptive things that you might want to do in chaos testing, I think eBPF could be a really interesting technology for building that on.
See more presentations with transcripts
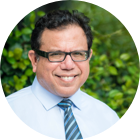
MMS • Simon Maple
Article originally posted on InfoQ. Visit InfoQ
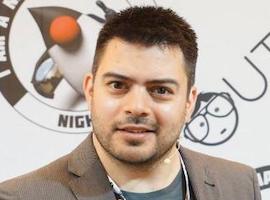
Transcript
Maple: My name is Simon Maple. I’m the Field CTO at Snyk. We’re going to be looking at the impact that Log4Shell had on us as an ecosystem, and how we can better prepare ourselves for a future Log4Shell. I’ve been in Java for 20 years plus commercially, as a developer, as dev advocacy, as community, and now as a Field CTO at Snyk, which is a developer security company creating a platform to help developers add security into their developer workflows.
Outline
We’re going to be first of all looking at the Log4Shell incident, the vulnerability that existed in the Log4j library. We’re going to extract from that the bigger problems that can impact us in future in a similar way to Log4Shell. In fact, as I am recording this, we’re literally a day into another potential Log4Shell type incident, which is potentially being called Spring4Shell, or Spring Shell, which looks like another remote code execution incident that could potentially be in the Spring ecosystem. These are the types of incidents that we are looking to better prepare ourselves for in future. Once we talk about the steps we can take to really help ourselves to mitigate that, we’re going to look at what is actually beyond the Log4Shell risk, what is beyond that open source risk that we as Java developers and Java organizations, steps we can take to first of all understand where that risk is, and what steps we can actually take to better prepare ourselves and mitigate those risks as we go.
What Is Log4Shell?
Let’s jump in, first of all with what the Log4Shell incident was, and some of the bigger problems that we really can understand and take out for future learnings. This blog post is one of the blog posts that we wrote from Brian Vermeer, on December 10th, the day that the vulnerability came out. Of course, it had to be a Friday: Friday afternoon, Friday morning, where the Java ecosystem was alerted en masse at a new critical Log4j vulnerability. This was a remote code execution vulnerability. At the time, the suggested upgrade was to version 2.15. This was the version that at the time was thought to contain the entire fix for this incident. CVSS score, which is the score that is essentially almost built from a scorecard of a number of security questions to determine what the risk is, how easy it is to break into, that was given a 10. This is out of 10, so the highest possible score for that.
Java Naming and Directory Interface (JNDI)
Let’s dig a little bit deeper into what is actually happening under the covers and how this vulnerability came about. It really starts off with a couple of things, first of all, the JNDI, and secondly, Java serialization. The JNDI, the Java Naming and Directory Interface is essentially a service that is there by default in the JDK. It allows our applications that are deployed into a JDK to access potentially locally, like we’ve done here, a number of objects that can be registered into that JNDI. I’m sure there are many Java devs that are very familiar with this already. It’s been a very core part of the JDK for many years. Now, examples here, you might make a request with a particular string that is effectively the key of an object that has been registered in the JNDI. For example, env/myDS, my data source. You might want to qualify that with a java:comp, which is similar to a namespace/env/myDS. What we would get back is the myDS Java object, which we can then use to get data from a database.
We don’t always have to look to the local JNDI to register or get these objects. What we can also do is make a request out to a remote JNDI. In this case, here’s an example of what might happen if I was to create a remote evil JNDI, which I was to stand up on one of my evil servers. My application that I’ve deployed into the JDK can make a request out specifying, in this case, the JNDI LDAP server, parsing in an evil.server URL with a port here of 11, and requesting a bad object. What I would get back is a serialized object, bad, that I could reconstruct, and I could potentially execute there. Obviously, my application isn’t going to go out and request this bad object from my evil server. What an attacker would try and do to any attack vector here for this type of attack, is to parse in something to the application, so that the application will use that input that I give it to request something of my evil JNDI server?
That’s all very well and good, but what does this have to do with Log4j? We know Log4j is a logging environment, it’s a logging library and function. Why does that yield? What’s that got to do with the JNDI? Many years ago, I think it was around 2013, a feature was added into Log4j to be able to look up certain strings, certain properties for variables and things like that, configurations from the JNDI. Very often though, if a logger sees a string, which is a JNDI like lookup string, it will automatically try and perform that lookup as part of that request while logging. As a result, there is a potential to exploit something like this by trying to log a user input, which is a JNDI string, which contains my URL with an evil input, which will pull my evil object and potentially run that. Typically, login very often happens on exception parts and error parts. What we’re going to see here is an attempt for attackers to try and drive down an exception path with a payload of the JNDI string. That JNDI string will relate to my evil object, which in this case here it’s going to perform an exec parsing and maybe some sensitive data back to my URL, and I can extract credentials and other things. This is one example of what could happen.
One of the big problems with this specific vulnerability and what made this rock the Java ecosystem so much is the prevalence of Log4j, not just in the applications that we write, but in the third party libraries that we pull in, because of course, everyone needs logging, everyone uses some logging framework. The question is, how do we know that if we’re not using it, that someone we are relying on isn’t using it as well? That’s one of the biggest problems.
What Is the Industry Exposure?
At Snyk, we noticed the number of things from the customers that use us and are scanning with us, we noticed over a third of our customers are using Log4j. We scan a number of different ecosystems. The vast majority of our Java applications had Log4j in them, but 35% of overall customers had Log4j. Interestingly, 60%, almost two-thirds of those are using it as a transitive dependency. They’re not using it directly in their applications, but the libraries, the open source third-party packages that they are using are making use of Log4j. That makes it extremely hard to work out whether you have Log4j in your application or not. Because if you ask any developer, are you using Log4j? They’ll know if they’re interacting directly most likely with Log4j. However, do they know that three levels deep there is a library that they probably don’t even know they’re using that uses Log4j? Possibly not. The industry exposure as a result is very broad, because Log4j gets pulled in, in so many different places.
The Fix
What was the fix? If we look back at what the original fix or suggested fixes were, it’s important to note that this changed very rapidly as more information came in, and that is because this was a zero-day vulnerability. The exploit was effectively widely known before the vulnerability was even disclosed. As a result, everyone was chasing their tails in terms of trying to understand the severity, the risk, how things could be attacked. As a result, there was changing mitigation strategies and changing advice, depending on literally the hour of the day that it was going through. Here’s a cheat sheet that I wrote back in December, to really suggest a number of different ways that it could be fixed.
The important thing to note is the fix was made available very soon. The strongest mitigation case here was to upgrade Log4j at the time to version 2.15. Of course, in some cases, that wasn’t possible. There are certain times where we needed to say, what are the next steps then? The vast majority of people actually had a bigger problem before saying, let me just upgrade my Log4j. The biggest problem people had here was visibility, gaining visibility of all the libraries that they are using in production, all the libraries that their application is pulling in. There are a couple of ways of doing that. Of course, there are tools that can do a lot of that on your behalf. One of the things that you could do if you’re using something like Maven or Gradle, there were certain ways of pulling that data from your builds. However, it’s hard to be able to do that from a build process up, because essentially, you need to make sure that this is being used everywhere. It’s sometimes easier to look at it from the top-down, and actually be able to scan large repositories of your application so that you can get a good understanding from the top-down of what is in your environments, what is in your Git repositories, for example.
Obviously, the upgrade path here is heavily to upgrade. I believe we’re over in 2.17 these days in terms of what the suggested fixes are. However, for those who perhaps you’re using binaries, rather than actually pulling in, in your source. I think GitHub Enterprise, for example, was using Log4j. What do you do in that case where you can’t actually have access to the source to actually perform an upgrade? In some cases, there were certain classes that you could just remove from the JDK before restarting it. When you remove those classes, the vulnerable methods, the vulnerable functions had effectively been removed. It’s impossible to get to go down those paths. However, there are, of course, operational problems with that, because if you were to go through those paths, you might get unexpected behavior. Luckily, in this case, because people were either doing JNDI lookups on purpose or not, it was a little bit more predictable. It wasn’t something that was very core functionality.
There were some other things that could be done. Some of these were later on discovered that they weren’t as effective as others. Upgrading JDK is a good example whereby a lot of people said yes, that’s what you need to do straight away. However, after a little bit of time, it was discovered that that wasn’t as effective because attackers were mutating the way that they were approaching the attack, and circumventing some of the ways in which we’re trying to fix.That really goes and points to the way, which if we were to look at it from the runtime point of view, and look at things like egress traffic, look at things like WAFs, these are very short-lived fixes that we can put out. Because the ability for an attacker to change the way that they attack your environments changes literally, by the minute, by the day. Because as you block something in your WAF, your Web Application Firewall, which essentially is trying to block traffic inbound which has certain characteristics about the way it looks, an attacker would just say, “You’ve blocked that, I’ll find another way around it.” There’s always an edge case that attackers will find that can circumvent those kinds of attacks.
The last thing was really monitoring projects, and monitoring your environments. Because with these kinds of things, all the eyes go to these projects and try and understand whether the fix was correct, whether there are other ways which you can actually achieve the remote code execution in those projects. There were a number of future fixes that had to be rolled out as a result of this Log4Shell incident. As a result, it was very important at varying risks at different times. It was very important to monitor the upgrade so that as new vulnerabilities and CVEs were released, you were getting notified. Of course, there’s an amount of tooling which Snyk and others can provide to do this, but this was typically the remediation that was available. Of course, if you’re looking to still do these remediations, be sure to check online for the latest and greatest, to make sure that the version changes are including the latest movements from these libraries.
Log4j Timeline
Looking at the timeline, what can we learn from this? Obviously, we know that it was a zero-day. If you look at the timeline of when the code that introduced the vulnerability first came in, as I mentioned, 2013, almost nine years before. It wasn’t till 2021, late November, an Alibaba security researcher approached Apache with this disclosure, and it was being done with Apache. The problem is, when you actually roll out a fix, when you actually put a fix into an open source project, people can look at that and say, why are you making these code changes? They can see what you’re essentially defending against. What this can do then is actually almost partly disclose this type of vulnerability to the ecosystem, because all of a sudden, before others can actually start adopting that latest fix, you’re essentially showing where the attack vector can be breached through or exploited. This happened on December 9th, and straightaway a PoC was published on GitHub. It was leaked on Twitter as well. We know how this goes. It snowballs. December 10th was the officially disclosed CVE. Although this was leaked on Twitter and GitHub the day before, the CVE hadn’t even been published. At this stage you look here day by day, and the poor Log4j maintainers were working day and night on understanding where future issues and things like that could be found and fixed. That’s an interesting timeline there.
December 10th, on the Friday afternoon, I’m sure everyone was probably in the incident room getting a team together. The first question, which is very often the hardest question is, are we using Log4j? Where are we using it? How close are they to my critical systems? Are we exploitable? The most common questions. Can you answer that? Were you able to answer that straightaway? Those people who could, were very often in ownership of an SBOM, a software bill of materials. Software bill of materials is inventory. It’s like a library, essentially, that itemizes all the components, all the ingredients, as it were, that you are using to construct your applications and put them into your production environments. This will list all the third party libraries, all the third party components that you’re building in. What this will allow you to do is identify, in this case, are we using the Log4j-core, for example, and its particular version? Are we using a vulnerable version of Log4j? Are we using Log4j at all anywhere? What projects are we using it in? Where in the dependency graph are we using it? Is it a direct dependency? Is it somewhere down the line? Are they fixable? These were the questions that if we had this software bill of materials, we can answer extremely quickly.
Competing Standards
An SBOM, a software bill of materials, there are actually standards for this. There’s two competing standards right now which we’re likely to keep both in our industry. One is CycloneDX, one is SPDX. Essentially, they’re just different formats. One is under OWASP, the other the Linux Foundation. CycloneDX is one which is a little bit more developer focused, in the sense of what you’ll see is tooling and things that are being created more for the open source world where they can actually start testing and really getting hands-on quicker. The SPDX project is more standards based, and so a lot of the folks from standards backgrounds will tend to resonate more along this angle. Both are reasonable standards, and we’re going to likely see various tools that are probably going to support both. These are the formats that you can expect your SBOMs to exist. How can you create an SBOM? Of course, there are many tools out there, Snyk and others. There are plenty of tools out there whereby you can scan all of your repositories. It’ll take a look at your POM XML files, your Gradle build files, create dependency graphs. It will effectively give you this software bill of materials where you can identify and list, catalog the open source libraries that you’re using.
How to Prepare Better for the Next Log4Shell Style Incident
How can we prepare ourselves then better for the next Log4Shell style incident, whether that’s Spring4Shell right now? What can we do ourselves? There’s three things, and if you take DevSecOps as a good movement here, the three core pieces of DevSecOps are people, process, and tooling. These three are the core things which we need to look at in order to improve our posture around security. People here is the most important, so let’s start with people.
The Goal: Ownership Change
Within our organizations, DevOps has changed the way that we are delivering software. I remember 20 years ago when I was working with two year release cycles, people weren’t pushing to production anywhere near as fast as they are today, which tends to be daily. As a result, the more periodic security traditional audit style was more appropriate back then, compared to today. When we’re delivering and deploying to production multiple times a day in more of a CI/CD DevOps way, we need to recognize that every change that goes to production needs to be scanned in the same way as we would for quality, unit tests or integration tests and things like that. There’s two things that need to happen there. First of all, you tend to see 100 devs, 10 DevOps folks, and 1 security person. That one security person can’t be there to audit every change, to provide information about what to fix on every single change. As a result, we need an ownership change here. Shift left is good, maybe for a waterfall style, but this is very much more an efficiency play. This is doing something earlier. What we need here is an ownership change, where we go away from this dictatorial security providing for the developer, and more to empowering developers. We want to go from top to bottom. Rather than it’s a dictatorial model, you’re really empowering everyone who’s making those changes. There are a number of things that need to alter not just our outlook here, but our processes, the tools we choose. The core thing here is the responsibility that we as developers have in this new type of model.
Developer vs. Auditor Context
While we go into responsibility, let’s take a look at the context of difference in terms of how we look at things. A developer cares about the app, that’s their context. They want to get the app working. They want to get the app shipped. They care about various aspects of the app, not just security, whereas an auditor purely cares about risk. They care about what vulnerabilities exist here. What is the risk? What is my exposure in my environment? You zoom out, and the developer, they care about availability, they care about resilience. A huge number of things way beyond security. Whereas the auditor, they then care about, where’s my data? What other services are we depending on? How are they secure? They look at the overall risk that exists beyond the app. We have very different perspectives and points of view. As a result, we need to think about things differently. Auditors or security folks audit. What they care about is doing scans, running tests, creating tickets. That’s their resolution, the creating tickets. The developers, when we want to provide and empower developers in security, their end goal is to fix. They need to resolve issues. They need solutions that they can actually push through rather than just creating tickets. As a result, our mentality and the changes we need to make need to reflect this model.
Where Could Log4shell Have Been Identified?
Let’s take a look at Log4j now, and think about where we identified this issue. Where could have this exposure been identified? We couldn’t really do anything in our pipeline here, because we’re not introducing Log4Shell here. We were already in production. This is a zero-day. This is something whereby it is affecting us today and we need to react to that. This is a zero-day where we need to react as fast as we can. Of course, there are other ways, which we will cover. At its core, we need it to be on this right-hand side.
Assess Libraries You Adopt
Other things that we can do in order to address other issues. For example, what if we were on the left-hand side, and we’re introducing a library which potentially has a known vulnerability, or we just want to assess the risk? How much can we trust that library? There are things that we can do when we’re introducing libraries to avoid that potential Log4j style zero-day going forward. This is a guide. There are obviously certain anomalies that can exist, for example, Spring being one of them, which here today potentially has this Spring4Shell issue. When you’re using a library, it’s important for your developers to ask these questions as they introduce them. Assess these libraries when you’re using them. Don’t just pull them in because they have the functionality because they’re allowing you to do what you need to do in order to push this use case through.
Check maintenance. How active is the maintenance here? Look at the commits over the last year, is it an abandoned project? Is it something whereby if an issue was found, it would be resolved pretty quickly? How many issues are there? How many pull requests are currently open? What is the speed at which they’re being resolved? How long ago was the last release? Potentially, if there’s something that it depends on, and there is a new release of a version of a transitive dependency, how long will this library take in order to perform a release consuming that latest version? The maintenance is very important to consider.
Then, of course, next, the popularity. There’s a number of reasons why this is very useful. Popularity is a very important trend, so make sure you’re not the only person using this library, but this is in fact well trusted by the ecosystem. It’s something which lots of people rely on, and you’re not by yourself in a space whereby no one else is using these kinds of libraries. This reliance on a library will very often push things like the demand for maintenance and so forth.
Thirdly, security, in terms of looking at the most popular version that this library has released that people are using, and across the version ranges, where are security issues being added? How fast are they being resolved, both in your direct dependency for that library, and the transitives as well? If a transitive has a vulnerability, how soon is that being removed? Then, finally, the community. How active is the community? How many contributors are there in the community? How likely is it that if there’s obviously, just one or two contributors, does that give an amount of risk for this being a library that could potentially be abandoned, and so forth? With all of these metrics, what we want to pull together is essentially a health score. In this case, this is an npm package, for example, called Passport. This is a free advisor service that’s on the Snyk website, but we provide a score out of 100 to give you almost like a trust metric, or at least a health metric in terms of how reliable this library might be in your environment. You can run this thing across your dependency graph almost and identify where the weak points are for your dependency graph.
Rethink Process
When we think about other places, so for example, the Log4Shell thing happened when we were in production already. Could we have taken steps to identify potential libraries that are more prone to these kinds of things? We could have done that while we were coding before we add these in. Of course, anomalies are going to happen. Log4j is one of those very popular libraries that if you go through those kinds of processes, sometimes that thing is just going to happen. Sometimes you might think it’s less likely, potentially, for there to maybe be a malicious attack on Log4j, but potentially more likely for a risk to actually be a greater magnitude on the impact it can have on the ecosystem.
Of course, the other area where we can look at is known vulnerabilities. Known vulnerabilities are essentially a vulnerability which has potentially a CVE or other vulnerability databases. They have an entry there, which basically says, this is the vulnerability, this is how severe it is. This is the library it’s in with this version range, between ultimately where it was introduced, and just before it was fixed. This is where it exists in your environment. It’s very important to be able to automate those kind of checks to see when you create your dependency graph, if the libraries that have vulnerabilities in are being introduced into your applications through your builds. This could be done at various stages. You can automate this into your Git repository, so that as you create pull requests, you can automatically test whether the delta change is introducing new issues. That might be license issues or security vulnerabilities. This is a great way of being able to, rather than look at potentially a big backlog, make sure you improve your secure development, just by looking at your future development first.
You can of course test in your CI/CD as well. Run tests in your Jenkins builds. There’s the opportunity here to block and make sure that if we get a very critical high severity vulnerability, we just don’t want to push this through. Very often, that can cause issues with a nicely, very slick, fast moving build process. You want to judge where you want to be more aggressive, where you want to be less aggressive, and more, be there for visibility, and be there to raise tickets potentially with an SLA to fix within a certain number of days. The core thing is run automation at these various points and have that awareness and that feedback to your developers as early as their IDE, with integrations and things like that.
Rethinking Tooling
One of the core things that we mentioned previously was about developer tooling and giving your development teams tools that will address what their needs for security are. That is to fix, not just to be an auditor, and to find. Here are some of the things that you need to think about when trying to work out what tooling your development teams should have. Make sure there’s a self-service model to use those tools. Make sure there’s plenty of documentation that your teams, as well as the vendor is creating for that. The Rich API, and the command line interface, and the number of integrations is core as well, as well as having a big open source community behind it. From a platform scope, there are many security acronyms, DAST, SAST, IAST, which tend to look more to your code, but think about the wider, more broader application that you’re delivering as a cloud native application security concern. Finally, the one piece that I want to stress here is this governance approach. When you’re looking at a tool, ask the question, is this tool empowering me as a developer or my developers, or is it dictating to my developers? That will help you determine whether this is a tool that should fit in your DevOps process, or whether it doesn’t fit the process or the model that you’re striving for?
Cloud Moves IT Stack into App
Finally, we’re going to talk about what’s beyond the Log4Shell risk in our applications. This is beyond open source libraries. When we think about how we as developers used to write code many years ago, certainly when I started 20 years ago, pre-cloud, we thought about the open source libraries that we used to develop. We thought about the application code that we used to develop. We used to consume open source libraries as well as developing them ourselves. This constituted the application which we then threw over the wall to an operations team that looked after the platform, looked after the operations piece, the production environment. Whereas today in a cloud environment, so much of that is now more under the control, more under the governance, the development of a regular application. The Docker files, the infrastructure as code, whether it’s Terraform scripts, Kubernetes config, these are the more typical things that we as developers are touching on a day-to-day basis. As a result, we need more of an AppSec solution to make sure that things that we change, things that we touch are being done in a secure manner. A lot of the time all of these things are existing in our Git repositories. As a result, they’re going through the development workflows. What we need to be able to do is make sure we have solutions in place, which test these in that development workflow, in our IDEs, in our GitHub repositories, and so forth.
Rethinking Priorities
When we think about traditionally, as developers potentially looking at what we are securing, we absolutely go straight to our own code. While this is an important thing to statically test, as well as dynamically test, it’s important to look at what’s beneath the water almost as that iceberg. Open source code, you can tone as your infrastructure as code, your misconfigurations there, think about where you are most likely to be breached. Is it an open source library, is it your own code, or is it an S3 bucket where there’s a misconfiguration? Is there a container that contains vulnerabilities in the open source packages? Look at this as one, and trying to identify where your most critical issues are based on the stack that you are using.
Supply Chain Security: A Novel Application Risk
One of the last things here I want to cover really is something called the supply chain. Maybe you’ve heard a lot about supply chain security, supply chain risk more recently. The problem is essentially when I started in my development days, we had very much internal build systems, internal build. We had build engineers that were running builds literally on our own data centers. Much more of that is now done by third party software, done by SaaS software, potentially, as well. It’s a much more complex pipeline that we’ve built up over the last couple of decades. There’s a lot of trust that we need to put on many of these different components in this pipeline. Additionally, we need to understand what’s in that pipeline, but also where the weak links are in our chain, where the weak links are in our supply chain as well as that pipeline to identify where we are most vulnerable.
Let’s take a look at where the security risks are potentially, as part of our pipeline. First of all, we have the pipeline that we deliver. We as developers checking code into Git, pushing to a build pipeline, storing perhaps in an artifact repository before pushing to a consumer, maybe into our production environment, or to another supply chain, potentially as well. The thing that we’ve mentioned mostly here is these third party libraries. There’s two pieces here, one is the risk that we add into our application from our supply chain. The second one is a potential supply chain attack. The two are quite different. The second one there is about a compromise of our dependency.
Do you think Log4j, Log4Shell, was a supply chain attack? Is it a supply chain attack? Think about who the attacker is. Who is the attacker that is potentially trying to perform the attack? What are they trying to attack? What is it that they are trying to break, trying to attack, trying to compromise? They’re typically some attacker out there who is trying to break your application that contains Log4j. They’re attacking your application, they’re not attacking your supply chain. Log4j is providing you with supply chain risk, but it’s not a supply chain attack. An attack on your supply chain is where the attacker is trying to intentionally break, maybe a library, maybe trying to compromise your library, your container, for example, and other things that we’ll talk about. The attacker is breaking your supply chain. They’re not trying to attack your application. They’re not trying to attack your endpoints. They’re trying to break your supply chain. The actual attack vector will get introduced in your supply chain. They’re not trying to attack an endpoint or attack something that you’ve put into production.
Obviously, containers, very similar there with vulnerabilities or compromises that can come in through your container images as well, public container images. A second one is a compromise of the pipeline, compromise of developer code. Someone trying to attack your Git repository. Someone trying to break into your build through misconfigurations in your build environments, potentially. Unauthorized attacks into your pipeline. Then the third piece of a supply chain attack is this compromise of a pipeline dependency. For example, Codecov was one. SolarWinds was another one here with the build tools and the Codecov plugin that was added here. They were compromised and they were added as a CI plugin, Codecov as a CI plugin. The compromised malicious version of that plugin was added into other people’s pipelines, attacking their pipelines, taking credentials, taking environment variables and sending it off to evil servers. This is what a supply chain attack really looks like.
These are potentially very lucrative to exploit because if you look at Codecov, that was a cascading supply chain attack. The actual attack happened on the Codecov supply chain that was then used in other supply chain, so it cascades to huge numbers of pipelines, giving out huge numbers of credentials. This is where we’re thinking beyond the open source libraries. Cascading effects are some of the biggest ones that can be attacked.
Conclusion
Hopefully, that gives you a good insight here into actionable tips that you can actually take away, and also areas of risk that you can look at, because while today it’s Log4Shell or Spring4Shell, tomorrow there could be another attack vector. We need to think very holistically about the overall application that we’re deploying, and where the greatest risk in our processes, our teams, and where our tooling can really help us out there.
See more presentations with transcripts
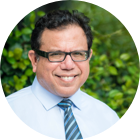
MMS • Sergio De Simone
Article originally posted on InfoQ. Visit InfoQ
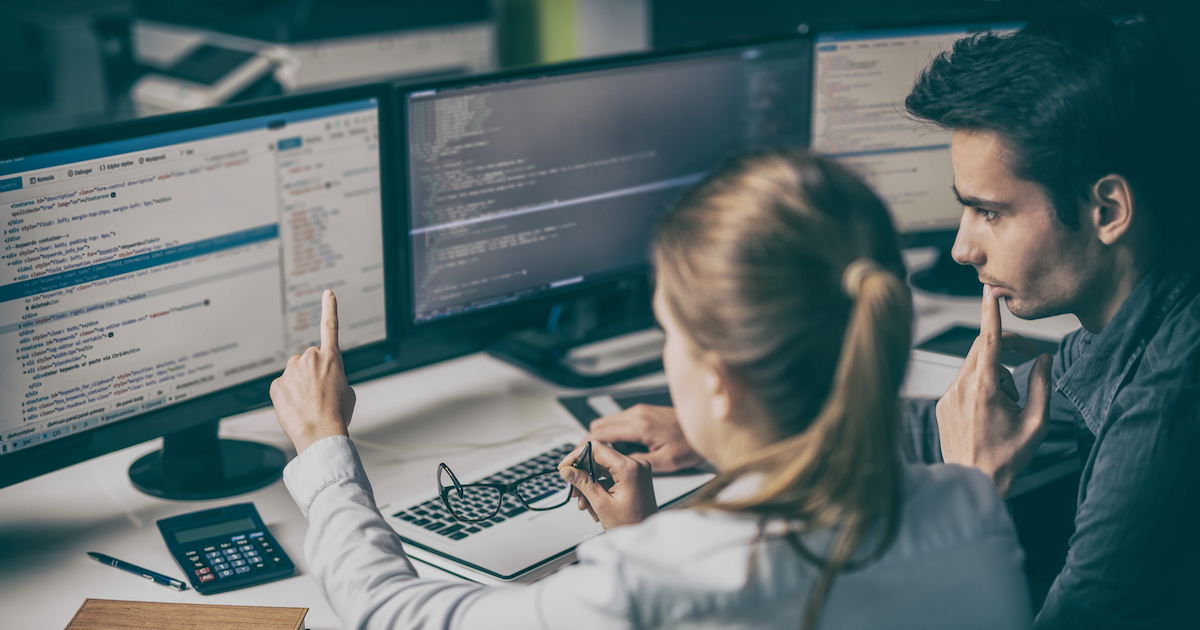
Two Git vulnerabilities affecting local clones and git shell
interactive mode in version 2.38 and older have been recently patched.
Classified as a high severity vulnerability, the git shell
vulnerability may allow an attacker to gain remote code execution by triggering a bug in the function that splits command arguments into an array. Since this function return an int
to represent the number of entries, the attacker can easily overflow it. This value is then used as the index count for the argv
array passed into execv()
, leading to arbitrary heap writes. This vulnerability can only be exploited when git shell
is enabled a login shell.
To fix this vulnerability
git shell
is taught to refuse interactive commands that are longer than 4MiB in size.split_cmdline()
is hardened to reject inputs larger than 2GiB.
The second, medium-severity vulnerability exploits symbolic links when doing a local clone to expose sensitive information to an attacker. A local clone is a clone operation where both the source and the target reside on the same volume.
Git copies the contents of the source’s
$GIT_DIR/objects
directory into the destination by either creating hardlinks to the source contents, or copying them (if hardlinks are disabled via--no-hardlinks
). A malicious actor could convince a victim to clone a repository with a symbolic link pointing at sensitive information on the victim’s machine.
This vulnerability can also be triggered when copying a malicious repository embedded via a submodule from any source when using the --recurse-submodules
option.
To fix to this vulnerability, Git will no longer dereference symbolic links and will refuse to clone repositories having symbolic links in the $GIT_DIR/objects
directory.
Both vulnerabilities have been patched in versions 2.30.6, 2.31.5, 2.32.4, 2.33.5, 2.34.5, 2.35.5, 2.36.3, and 2.37.4. If upgrading is not an option, there are workarounds that can help in the short-term.
The local clone vulnerability can be avoided by disabling cloning untrusted repositories using the --local
flag. Alternatively, you can explicit pass the no-local
flag to git clone
. Additionally, you should not clone untrusted repositories with the --recurse-submodules
.
The git shell
vulnerability can be avoided by disabling access via remote logins altogether or just disabling interactive mode by removing the git-shell-commands
directory.
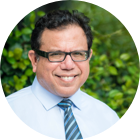
MMS • Matt Campbell
Article originally posted on InfoQ. Visit InfoQ
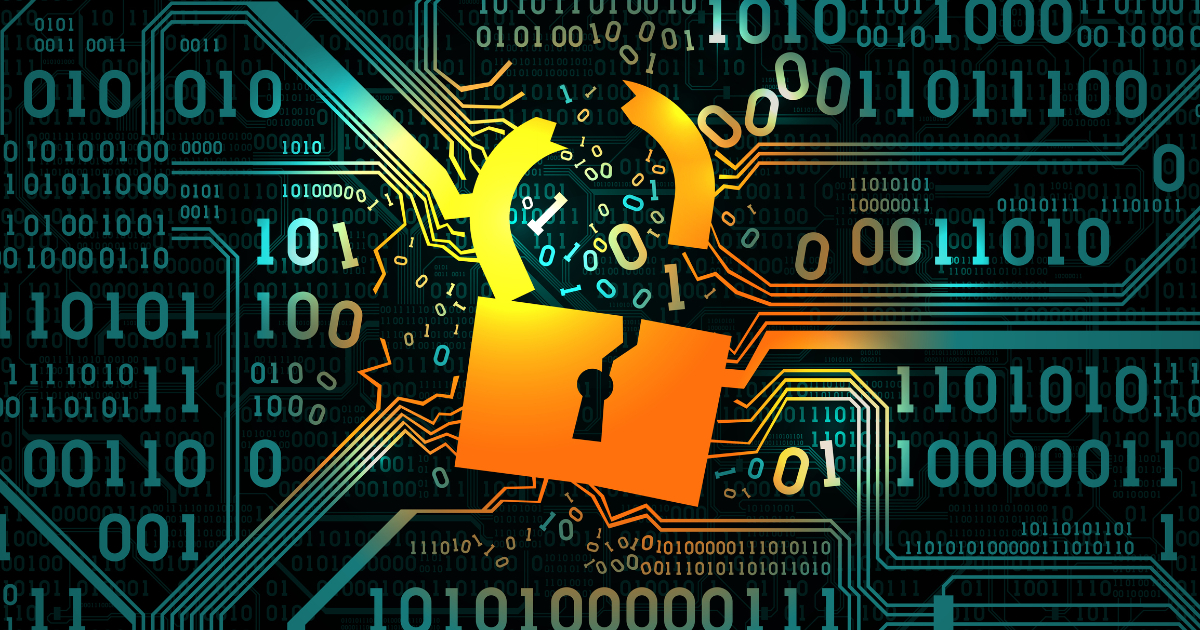
A recent report from Orca Security found several security gaps within the assessed cloud environments. These vulnerabilities include unencrypted sensitive data, S3 buckets with public READ access set, root accounts without multi-factor authentication enabled, and publically accessible Kubernetes API servers. In addition, they found that the average attack path only requires three steps to reach business-critical data or assets.
The report shared that 78% of identified attack paths will use a known exploit (CVE) as the initial access point. This works as the first of three steps needed, on average, to reach what the report authors call “the crown jewels”. This could be personally identifiable information (PII), corporate financials, intellectual property, or production servers.
Once initial access is obtained, typically through the known CVE, the report found that 75% of organizations have at least one asset that enables lateral movement to another asset within their environment. They found that 36% of organizations have unencrypted sensitive data and that 72% have at least one S3 bucket that permits public read access. They note that the top-end goal of most attack paths is data exposure.
Just within the past year, there have been numerous cases of sensitive data being exposed due to misconfigured public cloud storage. John Leyden shared that unencrypted data from Ghana’s National Service Secretariate (NSS) was discovered by researchers at vpnMentor. According to Leyden much of the data was publically accessible and “the AWS S3 bucket itself was neither encrypted nor password protected”. A similar incident occurred in July of this year when a misconfigured S3 bucket resulted in over 3TB of airport data, including photos of airline employees and national ID cards, becoming publicly accessible.
Of note within the report is that 10% of organizations still have vulnerabilities present that were disclosed over ten years ago. In addition, most organizations have at least 11% of their assets in a neglected security state where they are running an unsupported operating system or the asset has been unpatched for over 180 days.
The authors conclude that more effort needs to be applied to fixing known vulnerabilities. They concede that “many lack the staff to patch these vulnerabilities, which in more complex, mission critical systems is often not a simple matter of just running an update.” They continue by recommending a focused approach:
It is close to impossible for teams to fix all vulnerabilities. Therefore, it is essential to remediate strategically by understanding which vulnerabilities pose the greatest danger to the company’s crown jewels and need to be fixed first.
The report lists several best practices that they feel are not being followed correctly. This includes 99% of organizations using at least one default AWS KMS key. Orca Security recommends the use of customer-managed keys (CMK) instead of AWS managed. They also recommend enabling automatic key rotation, which only 20% of organizations had.
The recommendation to use CMKs comes from the additional level of control that they grant. This includes being able to create key policies, IAM policies, grants, tagging, and aliasing. User semanticist noted another potential use case on Reddit “if you want to be able to segment your keys (for instance, having one more-locked down and heavily-audited CMK that you use for the most sensitive S3 objects), then you would need to use a customer managed CMK.” There are additional costs incurred in using CMKs over the AWS managed KMS keys.
A recent misconfiguration by AWS to their AWSSupportServiceRolePolicy
granted the S3:GetObject
permission to AWS support staff. While this was quickly reverted, Victor Grenu, independent cloud architect, shared some key takeaways from the event:
- IAM is HARD, even AWS is failing
- Changes made to IAM should always be peer-reviewed, manually, and using linting
- Encrypt using your own customer-managed keys
In line with the first two points, the report found that 44% of environments have at least one privileged IAM role and that 71% are using the default service account in Google Cloud. They note that this account provides editor permissions by default and therefore violates the principle of least privilege. A similar finding violating least privilege was found in 42% of scanned accounts having more than 50% of the organization’s users having administrative permissions.
For more details on the report’s findings, readers are directed to the Orca Security 2022 State of the Public Cloud Security report.
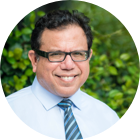
MMS • Ben Linders
Article originally posted on InfoQ. Visit InfoQ
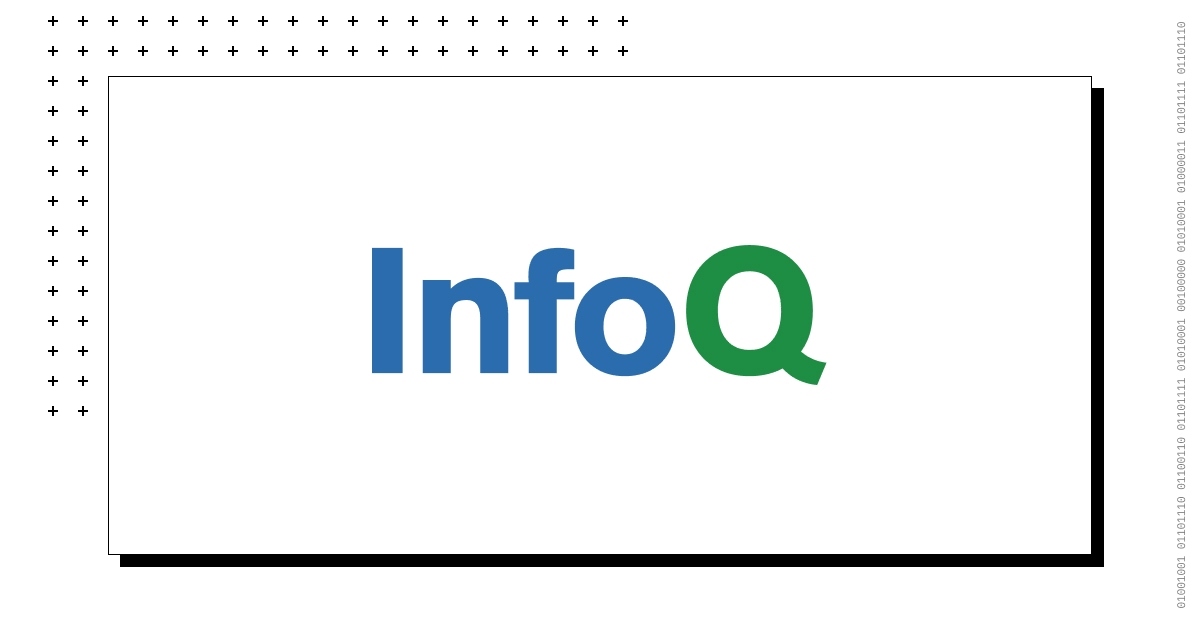
Progressive workplaces focus on purpose and value, having networks of teams supported by leaders with distributed decision-making. Employees get freedom and trust, and access to information through radical transparency that enables them to experiment and adapt the organization. In such workplaces, people can develop their talents and work on tasks they like to do, and have more fun.
Pim de Morree from Corporate Rebels spoke about unleashing the workplace revolution at Better Ways 2022.
The way we work is broken, De Morree stated. According to the State of the American Workplace Report 2017, only 15% of the employees are engaged in their work. When it comes to burnout symptoms, 44% of the employees sometimes have them whereas 23% always or often have them (based on Arbobalans 2018). The report Socially Useless Jobs states that 40% of the employees feel that their work is not useful and that they are not contributing to society.
De Morree stated that it’s beneficial for companies to create a workplace where people want to be, as this leads to higher productivity and profit, lower absenteeism, and fewer accidents and defects.
To figure out ways to motivate employees to the highest possible state, De Morree and Joost Minnaar are visiting pioneering organizations around the globe. Based on this they have identified eight trends in progressive workplaces:
- Focus on values and put purpose first in decision-making.
- Reinvent the entire structure, breaking down the hierarchy into a network of autonomous teams.
- Leaders become coaches and coordinators who support the success of their teams.
- Experiment and adapt; try out new ways of working.
- Creating a work environment where people have trust and freedom.
- Get rid of rules and distribute authority throughout the company.
- Grant company-wide access to data, documents, financials—in real-time, radical transparency.
- Finding the individual main talents and finding ways for people to develop those.
De Morree mentioned that there are different approaches to transform and propagate change in a company:
- Start small with one team and then add other teams.
- Involve all teams into change at once (big bang)
- Start with one department to remove the hierarchy into a network of teams.
- Radically change the whole company at once. This usually only works when there’s an urgent and important need.
He provided ideas for improvements that progressive teams can start with:
- Redesign your meetings: have fewer and better meetings. There should be a clear structure for meetings where De Morree referred to sociocracy: no agenda upfront, but created on the spot- people can choose how to be involved. First inform everyone and then make a decision based on consent.
- Distribute decisions using consent-based decision-making. This can lead to quicker and better decisions.
- Give better feedback. One technique that can be used is the “stop, start, continue” technique.
- Create a list of all activities, cluster them into roles, and let people choose what role fits them.
- Resolve conflict better by turning conflicts into opportunities in a graceful way.
- Adopt result-based working; assess team and individuals on outcomes and give freedom for people to boost performance.
The world needs more rebels to make work more fun, De Morree concluded.
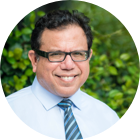
MMS • Johan Janssen
Article originally posted on InfoQ. Visit InfoQ
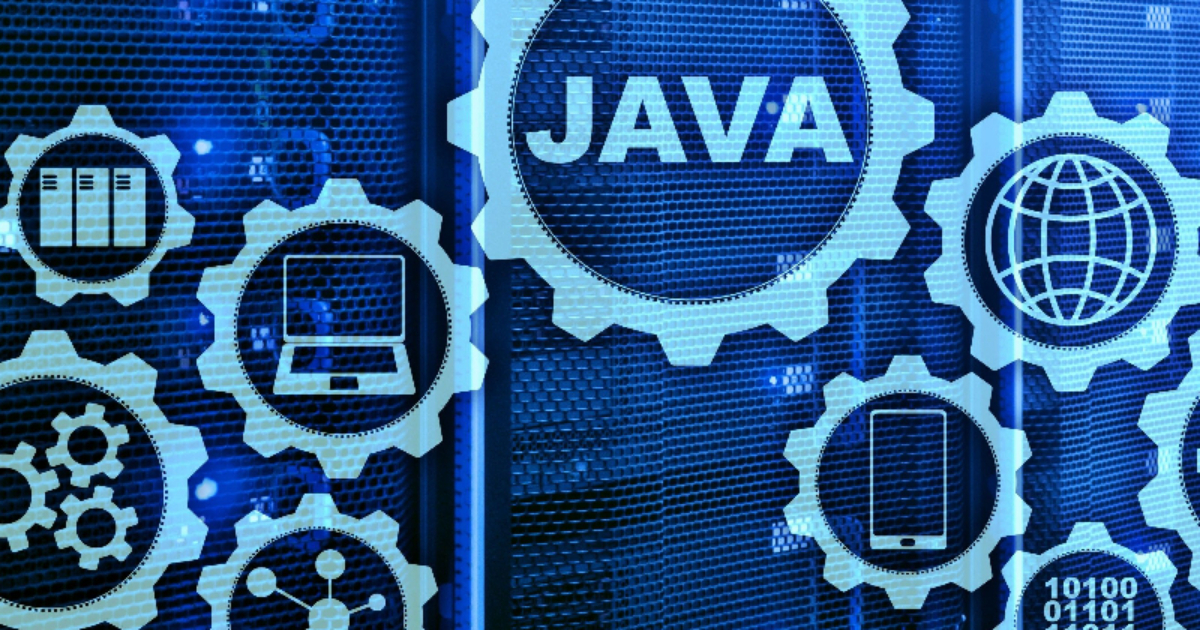
BellSoft has released Alpaquita Linux, an operating system based upon Alpine Linux, optimized for containerized Java applications. A plain Docker image is available, as well as Docker images with Liberica JDK or JRE or a Native Image Kit based upon GraalVM.
Alternatively, Alpaquita Linux can be installed via Windows Subsystem for Linux (WSL), Linux repositories or an ISO file.
Alpaquita Linux offers some additional benefits such as LTS releases, support for the musl and glibc C standard libraries and modern security features with fast security patches for both the operating system and the Java runtime. Security options include the kernel lockdown feature which makes it impossible to directly or indirectly access a running kernel image. The kernel module signing is another option and works with SHA-512 to disallow loading modules with invalid keys and unsigned modules. The small number of operating system components reduces the attack surface. Hardening is supported with userspace compilation options such as the -Wformat-security
argument which issues a warning for format functions which may cause security issues.
The operating system supports kernel module compression, and contains the malloc implementations mimalloc, jemalloc and rpmalloc.
This is the only Linux operating system optimized for Java applications with a TCK-verified runtime and optimized memory consumption.
BellSoft provides four years support for Long Term Support (LTS) releases, two years more than Alpine Linux. The current LTS is released this year and the next one will be released in 2024. BellSoft offers various commercial support plans.
The Alpaquita Linux Docker image based on musl is 3.22 MB and the image based on glibc is 7.8 MB. That’s just a bit bigger than the Alpine Docker images which start at less than 2.5 MB. Additional Docker images with Python or GCC are available as well.
Alternatively, the Liberica Runtime Container, based on Alpaquita Linux, may be used for Java applications. Docker images are available for Java 8, 11 and 17 where the smallest JRE and JDK images for Java 17 are less than 75MB. The images are based upon Liberica Lite which is optimized for size, performance and cloud deployments.
The last alternative based on Alpaquita Linux is the Liberica Native Image Kit (NIK) which uses GraalVM. Docker images are available for Java 11 and 17, where the Java 17 images start at a bit more than 308 MB. The kit may be used to compile JVM applications into ahead of time compiled native executables that offer faster startup times and lower memory consumption compared to traditional applications. The executable binary file contains the application, dependencies and runtime components including the Java Virtual Machine (JVM). Applications written in JVM languages such as Java, Kotlin, Scala and Groovy may be converted into executables. The Native Image Kit works on Windows, Linux and macOS on machines with x86, x64 or ARM processors (Linux only).
Alpaquita Linux is part of the Alpaquita Cloud Native Platform together with Liberica JDK Lite and Liberica Native Image Kit (NIK).
More information can be found in the introduction blog.
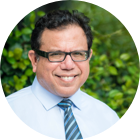
MMS • RSS
Posted on mongodb google news. Visit mongodb google news
Park Avenue Securities LLC lifted its position in MongoDB, Inc. (NASDAQ:MDB – Get Rating) by 157.9% during the second quarter, according to the company in its most recent filing with the Securities & Exchange Commission. The fund owned 1,346 shares of the company’s stock after buying an additional 824 shares during the period. Park Avenue Securities LLC’s holdings in MongoDB were worth $349,000 as of its most recent SEC filing.
A number of other institutional investors and hedge funds have also recently modified their holdings of the company. Vanguard Group Inc. grew its position in shares of MongoDB by 2.1% in the 1st quarter. Vanguard Group Inc. now owns 5,970,224 shares of the company’s stock worth $2,648,332,000 after buying an additional 121,201 shares during the last quarter. State Street Corp boosted its stake in MongoDB by 0.6% in the 1st quarter. State Street Corp now owns 1,343,657 shares of the company’s stock worth $596,033,000 after purchasing an additional 7,389 shares in the last quarter. 1832 Asset Management L.P. boosted its stake in MongoDB by 19.3% in the 1st quarter. 1832 Asset Management L.P. now owns 1,028,400 shares of the company’s stock worth $450,095,000 after purchasing an additional 166,400 shares in the last quarter. Wellington Management Group LLP boosted its stake in MongoDB by 26.4% in the 1st quarter. Wellington Management Group LLP now owns 672,545 shares of the company’s stock worth $298,334,000 after purchasing an additional 140,260 shares in the last quarter. Finally, TD Asset Management Inc. boosted its stake in MongoDB by 18.3% in the 1st quarter. TD Asset Management Inc. now owns 621,217 shares of the company’s stock worth $275,566,000 after purchasing an additional 96,217 shares in the last quarter. Hedge funds and other institutional investors own 89.85% of the company’s stock.
Analysts Set New Price Targets
A number of research firms recently commented on MDB. Needham & Company LLC lowered their target price on shares of MongoDB from $350.00 to $330.00 and set a “buy” rating for the company in a research note on Thursday, September 1st. Robert W. Baird lowered their target price on shares of MongoDB from $360.00 to $330.00 and set an “outperform” rating for the company in a research note on Thursday, September 1st. The Goldman Sachs Group lowered their target price on shares of MongoDB to $430.00 in a research note on Tuesday, September 6th. Mizuho lifted their target price on shares of MongoDB from $270.00 to $390.00 and gave the stock a “buy” rating in a research note on Wednesday, August 17th. Finally, Canaccord Genuity Group lifted their target price on shares of MongoDB from $300.00 to $360.00 and gave the stock a “buy” rating in a research note on Thursday, September 1st. One equities research analyst has rated the stock with a hold rating and nineteen have assigned a buy rating to the company’s stock. According to data from MarketBeat.com, the company has an average rating of “Moderate Buy” and a consensus target price of $379.11.
MongoDB Price Performance
NASDAQ:MDB opened at $189.97 on Wednesday. The company has a debt-to-equity ratio of 1.70, a current ratio of 4.02 and a quick ratio of 4.02. The firm has a market cap of $13.05 billion, a price-to-earnings ratio of -35.44 and a beta of 1.16. The company has a 50 day moving average price of $257.39 and a 200-day moving average price of $290.80. MongoDB, Inc. has a 1-year low of $166.61 and a 1-year high of $590.00.
MongoDB (NASDAQ:MDB – Get Rating) last posted its earnings results on Wednesday, August 31st. The company reported ($1.69) earnings per share for the quarter, missing the consensus estimate of ($1.52) by ($0.17). The firm had revenue of $303.66 million for the quarter, compared to analysts’ expectations of $282.31 million. MongoDB had a negative net margin of 33.43% and a negative return on equity of 52.05%. MongoDB’s revenue for the quarter was up 52.8% compared to the same quarter last year. During the same period last year, the firm earned ($1.15) earnings per share. Equities research analysts forecast that MongoDB, Inc. will post -5.37 EPS for the current year.
Insider Activity
In related news, Director Archana Agrawal sold 663 shares of the business’s stock in a transaction on Monday, August 29th. The shares were sold at an average price of $345.55, for a total value of $229,099.65. Following the completion of the sale, the director now owns 2,080 shares of the company’s stock, valued at approximately $718,744. The sale was disclosed in a legal filing with the SEC, which is available through this hyperlink. In related news, Director Archana Agrawal sold 663 shares of the business’s stock in a transaction on Monday, August 29th. The shares were sold at an average price of $345.55, for a total value of $229,099.65. Following the completion of the sale, the director now owns 2,080 shares of the company’s stock, valued at approximately $718,744. The sale was disclosed in a legal filing with the SEC, which is available through this hyperlink. Also, CRO Cedric Pech sold 288 shares of the business’s stock in a transaction on Monday, October 3rd. The stock was sold at an average price of $198.84, for a total transaction of $57,265.92. Following the completion of the sale, the executive now directly owns 34,157 shares of the company’s stock, valued at approximately $6,791,777.88. The disclosure for this sale can be found here. Insiders sold a total of 103,275 shares of company stock valued at $23,925,529 in the last three months. 5.70% of the stock is currently owned by corporate insiders.
About MongoDB
MongoDB, Inc provides general purpose database platform worldwide. The company offers MongoDB Enterprise Advanced, a commercial database server for enterprise customers to run in the cloud, on-premise, or in a hybrid environment; MongoDB Atlas, a hosted multi-cloud database-as-a-service solution; and Community Server, a free-to-download version of its database, which includes the functionality that developers need to get started with MongoDB.
Featured Stories
- Get a free copy of the StockNews.com research report on MongoDB (MDB)
- Does J&J Have Enough Alpha to Be a Solid Low Beta Stock?
- This Mid Cap Energy Stock is a 10-Bagger…And May Not Be Done
- Should You Make a Reservation in Marriott International Stock?
- The Outperforming Software Stock No One is Talking About
- Will Goldman Sachs’ Earnings & Revenue Beats Lift Sector Higher?
Want to see what other hedge funds are holding MDB? Visit HoldingsChannel.com to get the latest 13F filings and insider trades for MongoDB, Inc. (NASDAQ:MDB – Get Rating).
Receive News & Ratings for MongoDB Daily – Enter your email address below to receive a concise daily summary of the latest news and analysts’ ratings for MongoDB and related companies with MarketBeat.com’s FREE daily email newsletter.
Article originally posted on mongodb google news. Visit mongodb google news