Month: January 2023
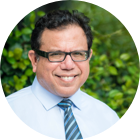
MMS • RSS
Posted on nosqlgooglealerts. Visit nosqlgooglealerts
Aerospike, Inc., the real-time data platform leader, announced the release of Aerospike Connect for Elasticsearch. The new connector enables developers and data architects to leverage Elasticsearch, a popular open-source search and analytics technology, to perform fast full-text-based searches on real-time data stored in Aerospike Database 6.
Aerospike Connect for Elasticsearch furthers the company’s commitment in 2023 to deliver comprehensive search and analytics capabilities for the Aerospike Database. The new connector enables extremely fast, full-text searches on data in Aerospike Database 6 using Elasticsearch. Further, it complements the recently announced Aerospike SQL powered by Starburst product, which allows users to perform large-scale SQL analytics queries on data stored in Aerospike Database 6. With a comprehensive list of capabilities, including the recently announced JSONPath Query support on Aerospike Database 6, Aerospike customers now have a wide variety of options to choose from to power their search and analytics use cases.
“We found the combination of Aerospike and Elasticsearch the best fit for our data platform stack,” says Eviatar Tenne, Data Platform Director, Cybereason. “We leveraged the Aerospike Real-time Data Platform with the Elasticsearch search engine and found these two technologies complement each other while incorporating a wide range of opportunities and capabilities for our business.”
“With enterprises around the world rapidly adopting the Aerospike Real-time Data Platform, there is a growing demand for high-speed and highly reliable full-text search capabilities on data stored in Aerospike Database 6,” said Subbu Iyer, CEO of Aerospike. “Aerospike Connect for Elasticsearch unlocks new frontiers of fast, predictably performant full-text search, which is critical to meet our customers’ needs.”
Full-Text-Search for Real-time Data Use Cases
Using Aerospike Connect for Elasticsearch, architects and developers can seamlessly integrate Aerospike’s high-performance, scalable, NoSQL database with Elasticsearch to enable a wide range of full-text search-based use cases such as:
- E-commerce: enriched customer experience that increases shopping cart size.
- Customer Support: enhanced self-service and reduced service delivery costs.
- Workplace Applications: unified search across multiple productivity tools.
- Website Experience: faster access to resources and increased site conversions.
- Federal: Smart cities will experience better results in maintaining mission-critical real-time applications.
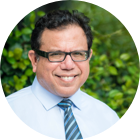
MMS • Michael Redlich
Article originally posted on InfoQ. Visit InfoQ
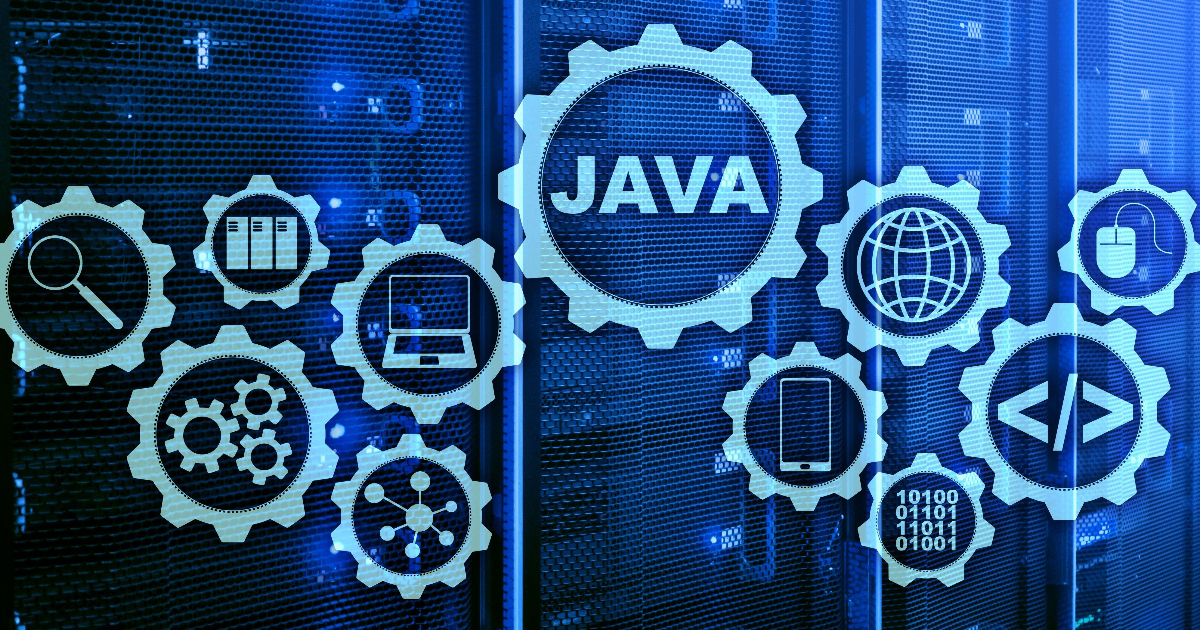
This week’s Java roundup for January 16th, 2023, features news from JDK 20, JDK 21, Spring Cloud Gateway 4.0, Spring Boot 3.0.2 and 2.7.8, Spring Modulith 0.3, Liberica JDK versions 19.0.2, 17.0.6, 11.0.18 and 8u362, Payara Platform, Micronaut 3.8.2, WildFly 26.1.3, TomEE 8.0.14, first three milestone releases of Job Runr 6.0 and Gradle 8.0-RC2.
JDK 20
Build 32 of the JDK 20 early-access builds was made available this past week, featuring updates from Build 31 that include fixes to various issues. More details on this build may be found in the release notes.
JDK 21
Build 6 of the JDK 21 early-access builds was also made available this past week featuring updates from Build 5 that include fixes to various issues. More details on this build may be found in the release notes.
For JDK 20 and JDK 21, developers are encouraged to report bugs via the Java Bug Database.
Spring Framework
Spring Cloud Gateway 4.0 has been released featuring new filters that enhance caching, request headers and JSON processing.
The release of Spring Boot 3.0.2 delivers bug fixes, improvements in documentation and dependency upgrades such as: Spring Framework 6.0.4, Spring Data 2022.0.1, Apache Tomcat 10.1.5 and Micrometer 1.10.3. More details on this release may be found in the release notes.
Similarly, the release of Spring Boot 2.7.8 delivers bug fixes, improvements in documentation and dependency upgrades such as: Spring Framework 5.3.25, Spring Data 2021.2.7, Apache Tomcat 9.0.71 and Micrometer 1.9.7. It is also important to note that the coordinates of the MySQL JDBC driver has changed from mysql:mysql-connector-java
to com.mysql:mysql-connector-j
. More details on this release may be found in the release notes.
Spring Modulith 0.3 has been released with new features such as: instances of the PublishedEvents
interface may now see events published from asynchronous event listeners; a new dedicated interface, ApplicationModuleInitializer
, to be executed on application startup to demarcate components in modules-specific order; and allow information exposed by the JSON actuator to be statically rendered. More details on this release may be found in the release notes.
BellSoft
BellSoft has released a Critical Patch Update (CPU) for versions 17.0.5.0.1, 11.0.17.0.1 and 8u361 of Liberica JDK, their downstream distribution of OpenJDK. CPU releases include patches for Common Vulnerabilities and Exposures (CVE). In addition, a Patch Set Update (PSU) for versions 19.0.2, 17.0.6, 11.0.18 and 8u362 of Liberica JDK, containing non-critical fixes and general improvements, was also made available. Overall, this release features 778 bug fixes and backports of which 24 issues were addressed by BellSoft.
Payara
Payara has released their January 2023 edition of the Payara Platform that includes Community Edition 6.2023.1 and Enterprise Edition 5.47.0.
The Community Edition delivers bug fixes, component upgrades, and the ability to use an environment variable when using the create-connector-connection-pool
command with the asadmin
utility. More details on this release may be found in the release notes.
Similarly, the Enterprise Edition delivers bug fixes, component upgrades and improvements such as: the ability to use an environment variable when using the create-connector-connection-pool
command with the asadmin
utility; Java Native Access (JNA) is now compatible on Apple Silicon chips; and the Start-Up, Post-Boot, Deployment and Post-Start-Up phases have been streamlined for consistent behavior. More details on this release may be found in the release notes.
For both editions, an upgrade to OpenSSL 1.1.1q provides a security fix in Payara Docker images.
Micronaut
The Micronaut Foundation has released Micronaut 3.8.2 featuring bug fixes and updates to modules: Micronaut Security 3.9.2, Micronaut Views 3.8.1, Micronaut Micrometer 4.7.1, and Micronaut Servlet 3.3.5. More details on this release may be found in the release notes.
WildFly
WildFly 26.1.3 is a maintenance release that addresses CVE-2022-46364, a vulnerability in which a Server-Side Request Forgery (SSRF) attack is possible from parsing the href
attribute of XOP:Include
in Message Transmission Optimization Mechanism (MTOM) requests. Dependency upgrades include: Jackson Databind 2.12.7.1, Apache CXF 3.4.10 and Eclipse Implementation of Jakarta XML Binding 2.3.3. More details on this release may be found in the release notes.
TomEE
TomEE 8.014 has been released featuring bug fixes and dependency upgrades such as: Hibernate 5.6.14, Tomcat 9.0.71, Apache CXF 3.4.10 and HSQLDB 2.7.1. More details on this release may be found in the release notes.
JobRunr
Three milestone releases of JobRunr 6.0 were made available this past week.
The first milestone release features new functionality and improvements such as: Job Builders that provide a single API to configure all the aspects of a Job
class via a builder instead of using the @Job
annotation; Job Labels such that jobs can be assigned labels that will be visible in the dashboard; support for Spring Boot 3.0; and improvements in stability.
The second milestone release allows for multiple instances of the JobScheduler
class with different table prefixes inside one application and an update of all transitive dependencies.
The third milestone release provides a bug fix related to Amazon DocumentDB.
Gradle
The second release candidate of Gradle 8.0.0 features improvements to the Kotlin DSL and buildSrc
, the latter of which will now behave more like included builds, such as running buildSrc
tasks directly, skipping tests, having init tasks and including other builds with buildSrc
. There were also performance improvements with enhancements to the configuration cache such as loading tasks from the cache entry and running tasks as isolated and in parallel. More details on this release may be found in the release notes.
Chromium to Allow the Use of Third-Party Rust Libraries to Improve Safety and Security
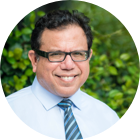
MMS • Sergio De Simone
Article originally posted on InfoQ. Visit InfoQ
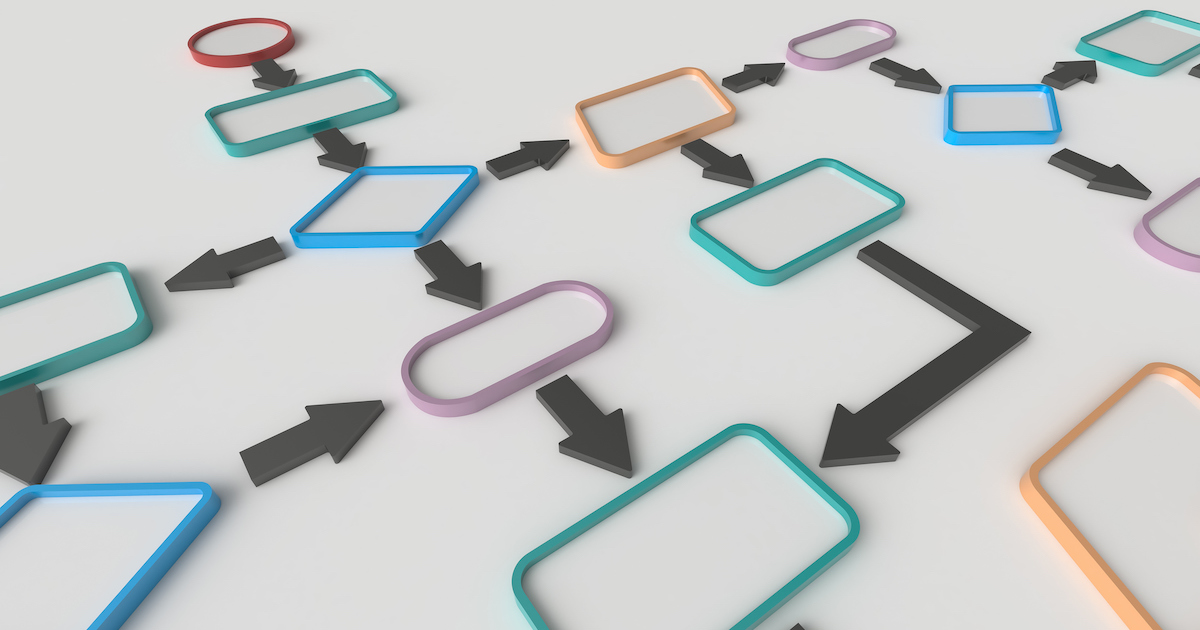
The Chromium Project is going to add a Rust toolchain to its build system to enable the integration of third-party libraries written in Rust with the aim to improve security, safety, and speed up development.
Rust was developed by Mozilla specifically for use in writing a browser, so it’s very fitting that Chromium would finally begin to rely on this technology too. Thank you Mozilla for your huge contribution to the systems software industry. Rust has been an incredible proof that we should be able to expect a language to provide safety while also being performant.
The promise that Rust brings to the Chromium Project, explains Dana Jansens, member of the Chromium Security Team, is providing a simpler and safer way to satisfy Chromium’s “rule of two“, which governs how to write code to parse, evaluate, or handle untrustworthy inputs from the Internet in a safe way.
The rule states that only two of the following conditions may hold at the same time: untrusted inputs, unsafe language use, and high execution privilege. For example, a C/C++ program, being inherently unsafe, can only process untrustworthy inputs in a process with very low privilege, i.e. in a sandbox. If C/C++ code is only used instead to process trustworthy inputs, then the sandbox is not required.
There are a number of benefits that the team aims to gain thanks to the introduction of Rust, including the ability to use a simpler mechanism than IPC, less complexity at the language level, less code to write and review, and reducing the bug density of the code. Those should contribute to Chromium overall safety, security, and development velocity.
It is important to observe that the Chromium Project is only considering the integration of Rust libraries, and not the broader adoption of Rust as a development language.
We will only support third-party libraries for now. Third-party libraries are written as standalone components, they don’t hold implicit knowledge about the implementation of Chromium. This means they have APIs that are simpler and focused on their single task. Or, put another way, they typically have a narrow interface, without complex pointer graphs and shared ownership.
Besides, there are only a fixed number of cases in which a Rust library will be considered for integration. In particular, the Rust implementation should be the best in terms of speed, memory, and bugs; it should allow moving the task to a higher privileged process to reduce the cost of IPC or C++ memory-safety mitigation; or, it should bring an advantage in terms of bug risks in comparison to alternatives.
In symmetry with their stepwise approach to the coexistence of Rust and C++ code, the Chromium team is limiting interop to only be allowed from C++ to Rust. Jansens provides a reasoned overview of the complexities inherent to allowing full interoperability including the possibility that safe Rust code land in intrinsically unsafe C++ code if a call from Rust to C++ were allowed, or the need for C++ developers to understand Rust rules to avoid violating them.
Full interop is not ruled out for the future, anyway, but it will require a significant investment in and evolution of interop tooling to ensure everything works smoothly. Meanwhile, the Chromium Team decision aims to gain access to the wealth of crates provided by the Rust ecosystem without incurring big penalties.
Article: Software Testing, Artificial Intelligence and Machine Learning Trends in 2023
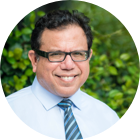
MMS • Adam Sandman
Article originally posted on InfoQ. Visit InfoQ
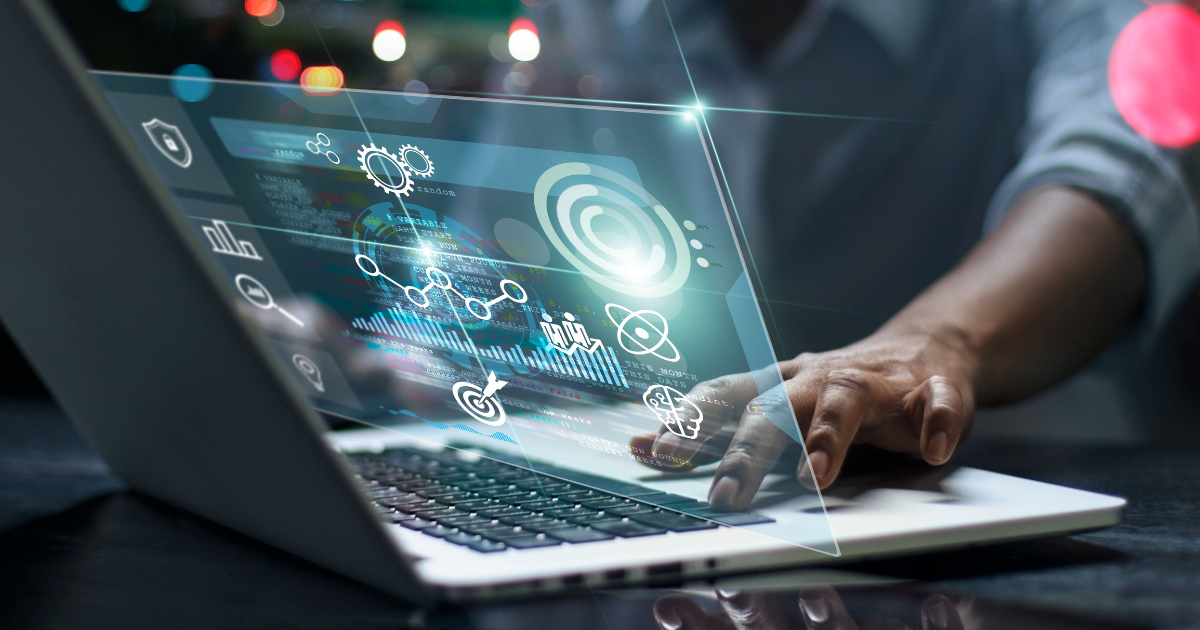
Key Takeaways
- Significant changes are coming in 2023 and the years ahead that will affect the software testing industry in big and small ways and as a result, you should start investigating how AI and ML can be used to improve your testing processes, leverage AI-based security tools, and implement risk-based methods such as risk-based testing that can leverage big-data insights.
- Software Testing Trends – The need to rapidly reinvent business models or add new capabilities to handle remote working/living during the pandemic, developers were in high demand and in short supply, resulting in the paradoxical need for more programming expertise to do testing and more competition for those programming skills.
- Machine Learning Changing Software Testing – Software applications are constantly changing as users want additional features or business processes to be updated; however, these changes often cause automated tests to no longer work correctly. One of the first ways we’ve seen ML being used in testing is to make the current automated tests more resilient and less brittle.
- How AI Is Changing Security Testing – AI is poised to transform the cybersecurity industry in multiple ways and we are now seeing AI being used for the first time to target and probe systems to find weaknesses and vulnerabilities actively.
- New Roles and Careers – As AI becomes more mainstream, there are likely to be entirely new career fields that have not yet been invented.
In many ways, 2022 has been a watershed year for software; with the worst ravages of the pandemic behind us, we can see the temporal changes and which ones have become structural. As a result, companies that used software to build a sustainable long-term business that disrupted the pre-pandemic status quo have thrived. Yet, at the same time, those that were simply techno-fads will be consigned to the dustbin of history.
The software testing industry has similarly been transformed by the changes in working practices and the criticality of software and IT to the world’s existence, with the move to quality engineering practices and increased automation. At the same time, we’re seeing significant advances in machine learning, artificial intelligence, and the large neural networks that make them possible. These new technologies will change how software is developed and tested like never before. In this article, I discuss trends we’re likely to see in the next few years.
Software Testing Trends
Even before the pandemic, software testing was being transformed by increased automation at all levels of the testing process. However, with the need to rapidly reinvent business models or add new capabilities to handle remote working/living during the pandemic, developers were in high demand and in short supply. This resulted in the paradoxical need for more programming expertise to do testing and more competition for those programming skills.
One of the outcomes was moving to ‘low-code’ or ‘no-code’ tools, platforms, and frameworks for building and testing applications. On the testing side, this has meant that code-heavy testing frameworks such as Selenium or Cypress have competition from lower-code alternatives that business users can do. In addition, for some ERP and CRM platforms such as Salesforce, Dynamics, Oracle, and SAP, this has meant that the testing tools themselves need to have more intelligence and understanding of the applications being tested themselves.
Machine Learning Changing Software Testing
One of the first ways we’ve seen machine learning (ML) being used in testing is to make the current automated tests more resilient and brittle. One of the Achilles heels of software testing, mainly when you are testing entire applications and user interfaces rather than discrete modules (called unit testing), is maintenance. Software applications are constantly changing as users want additional features or business processes to be updated; however, these changes will cause automated tests to no longer work correctly.
For example, if that login button changes its position, shape, or location, it may break a previously recorded test. Even simple changes like the speed of page loading could fail an automated test. Ironically, humans are much more intuitive and better at testing than computers since we can look at an application and immediately see what button is in the wrong place and that something is not displayed correctly. This is, of course, because most applications are built for humans to use. The parts of software systems built for other computers to use (called APIs) are much easier to test using automation!
To get around these limitations, newer low-code software testing tools are using ML to have the tools scan the applications being tested in multiple ways and over multiple iterations so that they can learn what range of results is “correct” and what range of outcomes is “incorrect.” That means when a change to a system deviates slightly from what was initially recorded, it will be able to automatically determine if that deviation was expected (and the test passed) or unexpected (and the test failed). Of course, we are still in the early stages of these tools, and there has been more hype than substance. Still, as we enter 2023, we’re seeing actual use cases for ML in software testing, particularly for complex business applications and fast-changing cloud-native applications.
One other extensive application for ML techniques will be on the analytics and reporting side of quality engineering. For example, a longstanding challenge in software testing is knowing where to focus testing resources and effort. The emerging discipline of “risk-based testing” aims to focus software testing activities on the areas of the system that contain the most risk. If you can use testing to reduce the overall aggregate risk exposure, you will have a quantitative way to allocate resources. One of the ways to measure risk is to look at the probability and impact of specific events and then use prior data to understand how significant these values are for each part of the system. Then you can target your testing to these areas. This is a near-perfect use case for ML. The models can analyze previous development, testing, and release activities to learn where defects have been found, code has been changed, and problems have historically occurred.
How AI Is Changing Security Testing
If ML is changing the software testing industry, then AI is poised to transform the cybersecurity industry in multiple ways. For example, it is already touted that many antivirus and intrusion detection systems are using AI to look for anomalous patterns and behaviors that could be indicative of a cyber-attack. However, we are now seeing AI being used for the first time to target and probe systems to find weaknesses and vulnerabilities actively.
For example, the popular OpenAI ChatGPT chatbot was asked to create software code for accessing a system and generating fake but realistic phishing text to send to users using that system. With one of the most common methods for spear phishing using some kind of social engineering and impersonation, this is a new frontier for cyber security. The ability for a chatbot to simultaneously create working code and realistic natural language based on responses it receives in real-time from the victim allows AI to create dynamic real-time offensive capabilities.
If you don’t believe that you would be fooled, here’s a test – one of the paragraphs in this article has been written by ChatGPT and pasted unaltered into the text. Can you guess which one?
How Do We Test or Check AI or ML Systems?
The other challenge as we deploy AI and ML-based systems and applications is: how do we test them? With traditional software systems, humans write requirements, develop the system, and then have other humans (aided by computers) test them to ensure the results match. With AI/ML-developed systems, there often are no discrete requirements. Instead, there are large data sets, models, and feedback mechanisms.
In many cases, we don’t know how the system got to a specific answer, just that the answer matched the evidence in the provided data sets. That lets AI/ML systems create new methods not previously known to humans and find unique correlations and breakthroughs. However, these new insights are unproven and maybe only as good as the limited dataset they were based on. The risk is that you start using these models for production systems, and they behave in unexpected and unpredictable ways.
Therefore, testers and system owners must ensure they have a clear grasp of the business requirements, use cases, and boundary conditions (or constraints). For example, defining the limits of the data sets employed and the specific use cases that the model was trained on will ensure that the model is only used to support activities that its original data set was representative of. In addition, having humans independently check the results predicted by the models is critical.
How Is AI Changing Computer Hardware?
One of the physical challenges facing AI developers is the limits of the current generation of hardware. Some of the datasets being used are on the scale of Petabytes, which is challenging for data centers that simply don’t have sufficient RAM capacity to run these models. Instead, they must use over 500 General Processing Units (GPUs), each with hundreds of gigabytes of RAM, to process the entire dataset. On the processing side, the problem is similar, where the current electrical CPUs and GPUs generate large amounts of heat, consuming vast quantities of electricity, and the speed of parallel processing is limited by electrical resistance. One possible solution to these limitations is to use optical computing.
Optical computing is a type of computing that uses light-based technologies, such as lasers and photodetectors, to perform calculations and process information. While there has been research on using optical computing for artificial intelligence (AI) applications, it has yet to be widely used for this purpose. There are several challenges to using optical computing for AI, including the fact that many AI algorithms require high-precision numerical computations, which are difficult to perform using optical technologies.
That being said, there are some potential advantages to using optical computing for AI. For example, optical computing systems can potentially operate at very high speeds, which could be useful for certain AI applications that require real-time processing of large amounts of data. Some researchers are also exploring the use of photonics, a subfield of optics, for implementing artificial neural networks, which are a key component of many AI systems.
What New Roles and Careers Will We Have?
As AI becomes more mainstream, there are likely to be entirely new career fields that we have not yet been invented. For example, if you ever try using chatbots like ChatGBT, you will find out that it can write large amounts of plausible, if completely inaccurate, information. Beyond simply employing teams of human fact-checkers and human software testers, there is likely to be a new role for ethics in software testing.
Some well-known technologies have learned biases or developed discriminative algorithms from the datasets fed in. For example, the Compass court-sentencing system would give longer prison sentences to persons of color or facial recognition technology that works better on certain races than others. The role of software testers will include understanding the biases in such models and being able to evaluate them before the system is put into production.
Another fascinating career field would be the reverse of this, trying to influence what AI learns. For example, in the field of digital marketing, it is possible that chatbots could partially replace the use of search engines. Why click through pages of links to find the answer when a chatbot can give you the (potentially) correct answer in a single paragraph or read it out to you? In this case, the field of Search Engine Optimization (SEO) might be replaced by a new field of Chat Bot Optimization (CBO). Owners of websites and other information resources would look to make their content more easily digestible by the chatbots, in the same way that web developers try to make websites more indexable by search engines today.
Which paragraph did ChatGBT write?
Did you guess? It was the last paragraph in the section “How Is AI Changing Computer Hardware?”
Summary
In conclusion, significant changes are coming in 2023 and the years ahead that will affect the software testing industry in big and small ways. As a result, you should start investigating how AI and ML can be used to improve your testing processes, leverage AI-based security tools, and implement risk-based methods such as risk-based testing that can leverage big-data insights.
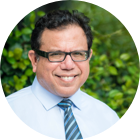
MMS • Matt Campbell
Article originally posted on InfoQ. Visit InfoQ
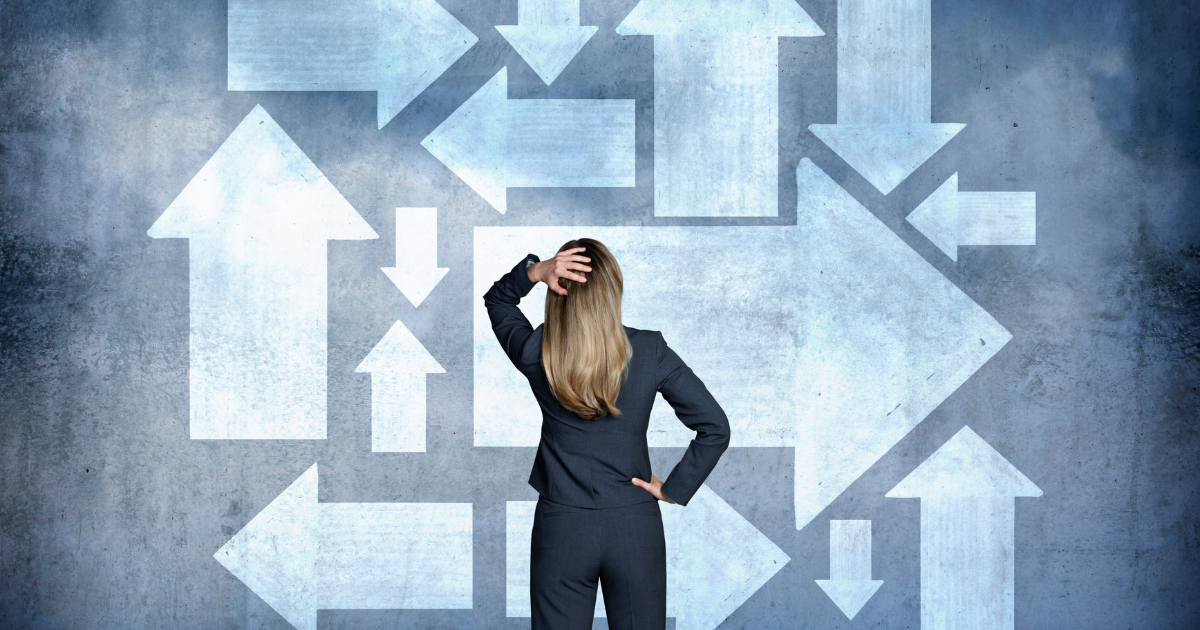
Fairwinds, a provider of Kubernetes software, has released their Kubernetes Benchmark Report 2023. The report shows an overall trend of worsening configuration issues across the surveyed organizations. This includes increases in organizations running workloads allowing root access, workloads without memory limits set, and workloads impacted by image vulnerabilities.
Last year, the report found that, in general, less than 10% of workloads were impacted by poor or improper configurations. This year, they found the spread to be more varied across the domains of reliability, security, and cost governance. The report provided some hypotheses as to why the overall trend year-over-year is towards more poorly configured workloads:
It’s clear DevOps teams are outnumbered and we need to do better as a community to support them. As Kubernetes usage expands, it’s harder for DevOps to manage configuration risk introduced by new teams.
The report surveyed over 150,000 workloads across hundreds of organizations. They found a 22 percentage point increase from last year in workloads that allow root access. They also saw an increase in workloads potentially impacted by image vulnerabilities with 25% of organizations having 90% of their workloads at risk. This is an increase of over 200% from last year’s report.
This is worrisome as a recent report from Orca Security found that the average attack path only requires three steps to reach business-critical data or assets. Combining root privileges with a potential image vulnerability, such as log4j, provides that initial entry point. The Orca Security report found that 78% of attack paths use a known exploit (CVE) as their initial access point.
The Fairwinds report did find that organizations that implemented Kubernetes guardrails in either shift-left approaches or at deployment time were able to correct 36% more issues where CPU and memory configurations were missing over those organizations without guardrails. In addition, organizations employing guardrails corrected 15% more image vulnerabilities than those not using them.
Danielle Cook, VP of Marketing at Fairwinds, explains that “[a]s Kubernetes usage expands, it’s harder for DevOps teams to manage configuration risk introduced by new teams.” Compounding this issue is identifying which team is responsible for building these guardrails and ensuring the configuration is correct. A survey by Armo found that 58% of respondents believe that the DevSecOps teams should own these solutions. The report also found that only 10% of respondents believed their teams were experts in handling the security of their Kubernetes environments.
Many agree that it is becoming harder for teams to understand and manage all the risks associated with their development activities, infrastructure, and tooling. Paula Kennedy, Chief Operating Officer at Syntasso, shares the impact this heavy cognitive load can have:
This is an on-going struggle for anyone trying to navigate a complex technical landscape. New tools are released every day and keeping up with new features, evaluating tools, selecting the right ones for the job, let alone understanding how these tools interact with each other and how they might fit into your tech stack is an overwhelming activity.
Fairwinds reports that guardrails or paved paths can help teams with following best practices. As Kennedy notes, these approaches help “to streamline the number of tools offered, reduce the cognitive load of too many options, as well as reduce technical bloat of the platform.”
For more results from the Fairwinds Kubernetes Benchmark Report 2023, users are directed to the Fairwinds site.
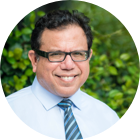
MMS • RSS
Posted on nosqlgooglealerts. Visit nosqlgooglealerts
Some time ago I was covering what’s new in Dart. Today I’d like to spark your imagination with five awesome, open source projects written in this programming language. You can browse the code and learn how to code, just use these projects or even contribute to them!
Let’s dive into what I have prepared for you!
BlackHole — Dart Music…
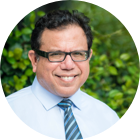
MMS • Matt Campbell
Article originally posted on InfoQ. Visit InfoQ
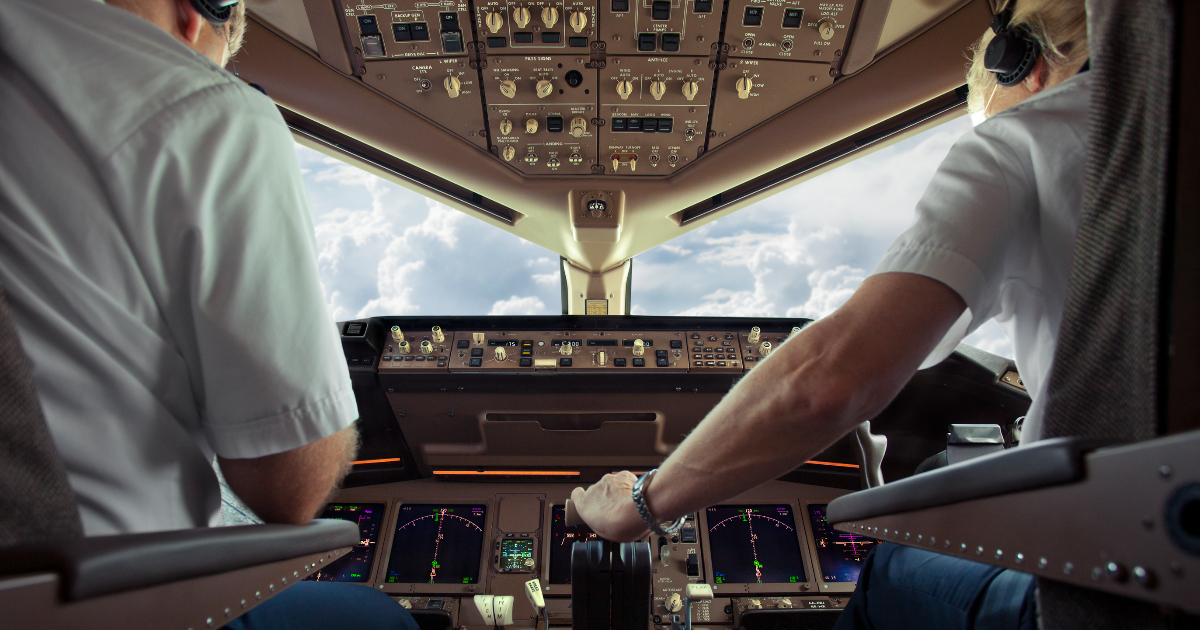
GitHub has announced Copilot for Business, a business plan for their OpenAI-powered coding assistant Copilot. The release follows a recent class action lawsuit against Microsoft, GitHub, and OpenAI for violating open-source licenses.
Copilot was made generally available back in July of 2022. The tool is powered by the artificial intelligence model OpenAI Codex which was trained on tens of millions of public repositories. Copilot is a cloud-based tool that analyzes existing code and comments and provides suggestions for developers.
Copilot for Business provides the same feature set as the single license tier. It also adds license management and organization-wide management capabilities. With license management, administrators can decide which organizations, teams, and developers receive licenses. GitHub has also stated that with Copilot for Business they “won’t retain code snippets, store or share your code regardless if the data is from public repositories, private repositories, non-GitHub repositories, or local files.”
According to GitHub, the organization-wide management capabilities will include being able to block Copilot from suggesting codes matching or nearly matching public code found on GitHub. This feature introduced back in June, blocks suggestions of 150+ characters that match public code. GitHub does warn that around 1% of the time a suggestion may contain code snippets longer than 150 characters.
However, Tim Davis, Professor of Computer Science at Texas A&M, has reported that GitHub Copilot has produced “large chunks of my copyrighted code, with no attribution, no LGPL license” even when the block public code flag is enabled. This is not the only controversy surrounding the tool.
In November of 2022, a class action lawsuit was launched against Microsoft, GitHub, and OpenAI. Submitted by Matthew Butterick and the law firm Joseph Saveri, the lawsuit claims that Copilot violates the rights of the developers whose open-source code the service is trained on. They claim that the training code consumed licensed materials without attribution, copyright notice, or adherence to the licensing terms.
Butterick writes that “The walled garden of Copilot is antithetical—and poisonous—to open source. It’s therefore also a betrayal of everything GitHub stood for before being acquired by Microsoft.”
Alex J. Champandard, Founder of creative.ai, agrees with Butterick that licensing should have been respected:
CoPilot is bold [and] innovative IMHO, but could have been equally transformative if they had obtained consent or respected the licenses — which would have been comparatively straightforward to achieve given their budget.
However, many users report how beneficial Copilot has been to their productivity. On Reddit, user ctrlshiftba shares that Copilot is “really good at [boilerplate]. When it’s working at it’s best it’s acting like an autocomplete with my code.” Alexcroox on Reddit agrees, “a lot of time it makes me faster just by autocompleting based on my current code base and code I’ve been writing that day.”.
GitHub does warn that “the training set for GitHub Copilot may contain insecure coding patterns, bugs, or references to outdated APIs or idioms.” They state that the end-user is responsible for ensuring the security and quality of their code, including the code generated and suggested by Copilot.
Some legal experts have argued that Copliot could put companies at risk if they unknowingly use copyrighted suggestions or code pulled from a repository with a copyleft license. GitHub has stated that they will introduce new features in 2023 that help developers have an understanding of code similar to the suggestion found in GitHub public repositories as well as the ability to sort that by license or commit date.
Copliot for Business is available now and is priced at $19 USD per user per month.
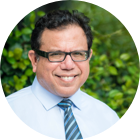
MMS • RSS
Posted on nosqlgooglealerts. Visit nosqlgooglealerts
There are many excellent options in the e-learning space, but Udacity’s database specialist certification is a must-have this year.
Data engineering involves designing and building pipelines that transport and transform data into a usable state for data workers. It often requires commercial software, specialized training, practitioner guidance, or professional services to accomplish. Data pipelines commonly take data from many disparate sources and collect them into data warehouses representing the data as a single source.
To do so, data engineers must manipulate and analyze data from each system as a pre-processing step. As data volumes and complexity continue to grow, new techniques, technologies, and theories are continually being developed to engineer data.
Our editors review all of the best data engineering courses and certifications each year to bring our audience the top picks for their use case. That’s why it’s in this spirit that Solutions Review editors bring you this recommendation to consider Udacity’s Azure data engineer expert certification if you’re looking to grow your skills this year.
Udacity is perfect for those who want the most in-depth experience possible through access to entire course libraries or learning paths.
TITLE: Learn SQL Nanodegree
OUR TAKE: This Udacity Nanodegree will help you learn big data’s core language for big data analysis, SQL. Students should come prepared with a basic understanding of data types and plan to spend 2 months on this training.
Description: Perform analysis on data stored in relational and non-relational database systems to power strategic decision-making. Learn to determine, create, and execute SQL and NoSQL queries that manipulate and dissect large-scale datasets. Begin by leveraging the power of SQL commands, functions, and data cleaning methodologies to join, aggregate, and clean tables, as well as complete performance tune analysis to provide strategic business recommendations.
Solutions Review participates in affiliate programs. We may make a small commission from products purchased through this resource.
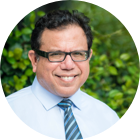
MMS • Sergio De Simone
Article originally posted on InfoQ. Visit InfoQ
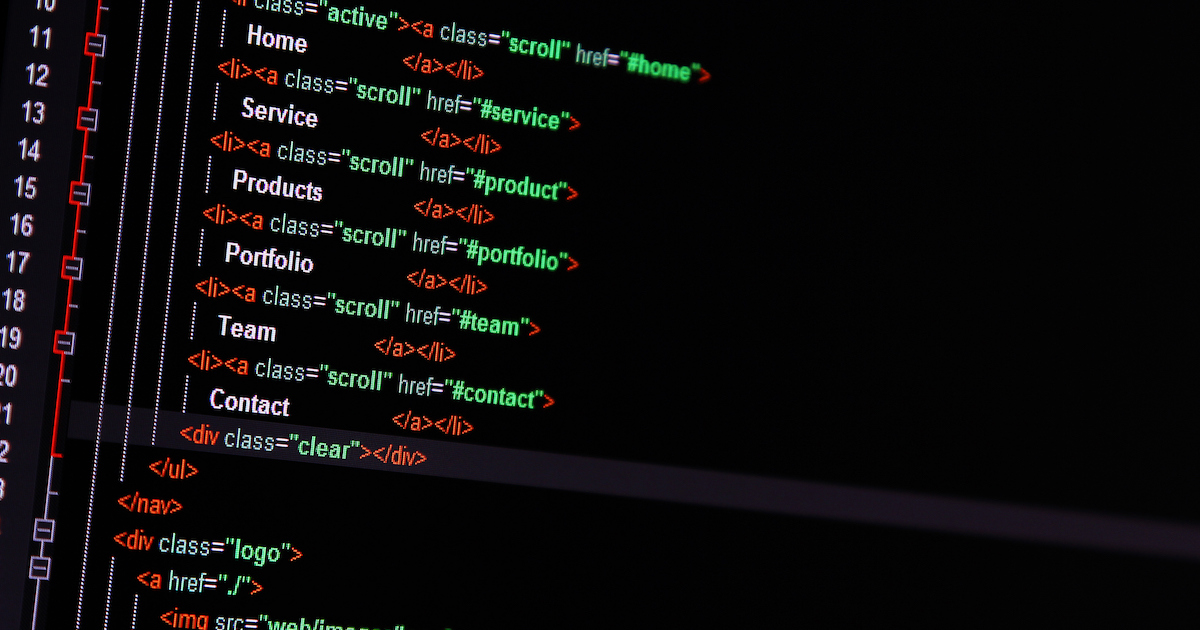
Two vulnerabilities affecting Git’s commit log formatting and .gitattributes
parsing in Git versions up to and including Git 2.39 have been recently patched. Both may lead to remote code execution, so users are required to upgrade immediately to Git 2.39.1.
One of the vulnerabilities, which was discovered by Joern Schneeweisz of GitLab, received the CVE-2022-41903 CVE identifier. It affects the git log
command when using the --format
option to customize the log format:
When processing the padding operators (e.g., %<(, %(, %>>(, or %><( ), an integer overflow can occur in
pretty.c::format_and_pad_commit()
where asize_t
is improperly stored as anint
, and then added as an offset to a subsequentmemcpy()
call.
The vulnerability can be triggered by running the git log --format=...
command supplying a malicious format specifiers. It can also be triggered indirectly by running the git archive
command using the export-subst
gitattribute, which expands format specifiers inside of files within the repository.
The other critical vulnerability, with identifier CVE-2022-23521, was discovered by Markus Vervier and Eric Sesterhenn of X41 D-Sec. It affects the gitattributes mechanism, which allows to assign specific attributes to paths matching certain attributes, as specified in a .gitattributes
file. Gitattributes can be used, for example, to specify which files should be treated as binary, what language to use for syntax highlighting, and so on.
When parsing gitattributes, multiple integer overflows can occur when there is a huge number of path patterns, a huge number of attributes for a single pattern, or when the declared attribute names are huge. These overflows can be triggered via a crafted
.gitattributes
file that may be part of the commit history.
This vulnerability specifically requires that the .gitattributes
file is parsed from the index, since git splits lines longer than 2KB when parsing gitattributes from a file.
Both vulnerabilities have mitigations, which consists in not using the affected features, but the suggested solution is upgrading to the latest Git version.
Additionally, the Git project has also disclosed a Windows-specific high severity vulnerability affecting Git GUI. CVE-2022-41953 can be triggered when cloning untrusted repositories on a Windows system due to how Tcl, the language used to implement Git GUI, deals with search paths on Windows:
Malicious repositories can ship with an
aspell.exe
in their top-level directory which is executed by Git GUI without giving the user a chance to inspect it first, i.e. running untrusted code
This issue has also been fixed in Git 2.39.1.
GitHub has taken action to ensure all of the repositories it hosts are not affected and to prevent any of those attacks. They also announced updates to all of its products integrating Git, including GitHub Desktop, GitHub Codespaces, GitHub Actions, and GitHub Enterprise Server.
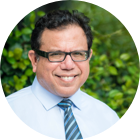
MMS • Jason Barto
Article originally posted on InfoQ. Visit InfoQ
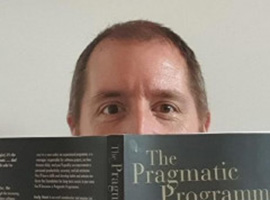
Transcript
Barto: Welcome to this session about fault isolation boundaries, and simple initial test that you can perform to test them. My name is Jason Barto. I work with a lot of teams and organizations to analyze their systems and develop ways to improve their system’s resilience. We’re going to introduce the concept of failure domains and reducing those failure domains using fault isolation boundaries. We will introduce some methods for creating fault isolation boundaries, and then try to apply this method to a sample system running on AWS. My hope is that by the end of this session, you will have a method that you can use to analyze your own systems and begin to test their implementation of fault isolation boundaries for reducing the impact of failures on your system.
A Tale of Two Systems
I want to start by first sharing a story with you that caused me to take a very deep interest in the topic of resilience engineering. This is the story of a banking application that was running in the cloud. This system was critical to the bank as part of their regulated infrastructure. Because of this, downtime meant fines and customers who couldn’t make payments. The system was designed using a lot of recommended practices. The team used highly available components and built an application cluster with three nodes to reduce the impact of any one node going down. The application cluster used a quorum technology, which meant that if one of the nodes was down, the other two would continue to function, but in a reduced capacity. If the second node went down, the system would fall back to a read-only mode of operation. While the first system was built using highly available technologies, the bank wanted additional assurance that the system would remain available in the face of failure. They deployed a secondary instance of the application in the same AWS region, but in a separate VPC. They operated these systems in an active-passive fashion, and to fail over to the secondary system was a management decision that would redirect traffic by updating a DNS record. Firewalls and so forth had already been worked out so that communication with on-premise systems was possible from both the primary and the secondary deployment.
Unfortunately, one day, there was a disruption to an AWS availability zone, which impacted the infrastructure hosting one of the application cluster nodes, causing the system to operate in a reduced capacity mode. The bank decided that they did not want to run in a reduced capacity mode, so they made the decision to fail over to the secondary system. They modified the DNS records and began to route requests to the secondary system. However, the same availability zone disruption, which had impacted the primary system also affected the EC2 instances in the secondary system, because they both resided in the same availability zone and shared physical data centers. The bank then consigned itself to having to operate at a reduced capacity until the services had been restored, and the EC2 instances could be relaunched. Luckily, there was no downtime involved, and no customers were impacted. However, it highlighted for me that there is no such thing as a system which cannot fail, and that there are very real dependencies that even single points of failure, that as technologists, we either knowingly acknowledge and accept, or are completely unaware of.
How Do We Confidently Create Resilient Systems?
If we adopt best practices, and even deploy entirely redundant systems, only for undesirable behavior to still creep in, how can we say with confidence that the systems we have built are resilient? Every team I’ve ever worked on or with, always had an architecture diagram. However, those teams did not go through those diagrams and ask themselves, what happens when one of those lines breaks, or one of the boxes is slow to respond. We don’t often ask ourselves, how can the system fail? What can we do to prevent it? Then, also, how do we test it? We’re going to use this architecture diagram and the example system that it represents to identify the failure domains in the system, explore what fault isolation boundaries have been incorporated into the design. Then, also, test those fault isolation boundaries using some chaos experiments.
Failure Domains
What exactly is a failure domain? A failure domain is the set of resources which are negatively impacted by an individual incident or event. Failure domains are not mutually exclusive, and they can overlap with one another when one failure domain contains another failure domain as a subset. For example, the failure domain for a top of rack switch failure is all of the resources within the rack from a network computing perspective. The failure domain for a tornado is everything within the data center. The same concept can be applied to system software. Single points of failure in a system architecture will have a failure domain for all of the resources that rely on that single point of failure to complete their task.
Let’s look at a simple example to further illustrate our definition. Say there is an issue with the web server process running on a server. This is bad, but as requests to the system can flow through a secondary redundant web server, the failure does not have an impact on the system as a whole. The failure domain includes only the web server, which experienced the issue. However, if the database experiences an issue, maybe it loses its network connection or is somehow affected, it’ll have an impact on the system as a whole because both of the web servers rely on the database to service requests. The next logical question then is, how can we get a list of the failure domains within an architecture? This is a process that’s normally called hazard analysis. There are various techniques that can be used to create a solid understanding of the failure domains within a system. Adrian Cockcroft gave a talk at QCon San Francisco, which introduced many of these techniques, in 2019. Some examples of hazard analysis techniques include failure mode effect analysis, fault tree analysis, and system theoretic process analysis, which was described by Dr. Nancy Leveson of MIT in the STPA Handbook. All of these provide a formal method for diving into a system, identifying potential failures, and estimating their impact on a system.
In the interest of simplicity, we are going to forego these formalized methods and instead focus on any of the single points of failure in this architecture to identify the failure domains. By looking at the lines and boxes in the diagram, we can ask questions like, what happens if the database becomes unavailable, or when the system is unable to issue requests to the AWS Lambda service? We can then start to think about how to mitigate such occurrences. There are enough boxes and lines in this diagram to create a good list of what if scenarios. What if a database instance fails? What if the network connection to the database fails? What if a web server fails? For today, we’re going to focus on just two failure domains. The first is that of an availability zone. What happens when an availability zone is experiencing a failure? The second is, what happens when the system is unable to send a request to an AWS service, namely, AWS Lambda?
Fault Isolation Boundaries
We’ve identified some potential failure domains for our system, what can we then do to reduce the size of each failure domain? How can we mitigate the failures and their blast radius? Using conscious design decisions in the characteristics and design of our system, we can implement fault isolation boundaries to manage these failure domains. We can isolate the fault so that they have minimal impact on the system as a whole. A fault isolation boundary is going to limit the failure domain, reducing the number of resources that are impacted by any incident or event. Let’s talk about a couple of the patterns that you can use in your application code to create fault isolation boundaries. We see on the right, three upstream systems, client, and their downstream dependency, a service. The lines between these systems are where failures can happen. These lines could be disrupted by failures with servers, networks, load balancers, software, operating systems, or even mistakes from system operators. By anticipating these potential failures, we can design systems that are capable of reducing the probability of failure. It is of course impossible to build systems that never fail, but there are a few things that if they would be systematically done would really help to reduce the probability and impact of failures.
First, we can start by setting timeouts in any of the code which establishes connections to, or makes requests of that downstream dependency. Many of the libraries and frameworks that we use in our application code will often have default timeout values for things like database connections. Those defaults will be set to an infinite value, or, if not infinite, often to tens of minutes. This can leave upstream resources waiting for connections to establish or requests to complete that just never will. The next pattern that we can employ is to use retries with backoff. Many software systems use retries today, but not all of them employ exponential backoff in order to prevent or reduce retry storms, which could cause a cascading failure. If you’re looking for a library to provide backoff logic, ensure that it includes a jitter element so that not all upstream systems are retrying at exactly the same time. Also, limit the number of retries that you allow your code to make. You can use a static retry limit of three attempts or some other number. In most cases, a single retry is going to be enough to be resilient to intermittent errors. Often, more retries end up doing more harm than good. You can also make your retry strategy more adaptive by using a token bucket so that if other threads have had to retry and failed, that subsequent initial calls are not also then retrying. This is similar to a circuit breaker, which will trip open if too many errors are encountered in order to give the downstream dependency time to recover.
Just as we protect our upstream clients from a failing service, we should also protect the downstream service from a misbehaving client. To do this, we can use things like rate limiting to limit the number of requests that any one client can send at a time. This creates a bulkhead which prevents any one of the clients from consuming all of a service’s resources. Also, when the service does begin to slow down or get overloaded, don’t be afraid to simply reject new requests in order to reduce the load on your service. There are many libraries out there today that will help you to incorporate these patterns into your code. Two examples are the chi library for Golang, which implements things like load shedding and rate limiting out of the box. The Spring Framework for Java can also leverage Resilience4j which has implementations of the circuit breaker, bulkhead, and rate limiting patterns, amongst others.
At the infrastructure level, similar to redundancy, you can limit the blast radius of a failure through a cell based architecture. Cells are multiple instantiations of a service that are isolated from each other. These service structures are invisible to customers, and each customer gets assigned to a cell or to a set of cells. This is also called sharding customers. In a cell based architecture, resources and requests are partitioned into cells, which are then capped in size. The design minimizes the chance that a disruption in one cell, for example, one subset of customers, would disrupt other cells. By reducing the blast radius of a given failure within a service based on cells, overall availability increases and continuity of service remains. For a typical multi-tenant architecture, you can scale down the size of the resources to support only a handful of those tenants. This scaled down solution then becomes your cell. You then take that cell and duplicate it, and then apply a thin reading layer on top of it. Each cell can then be addressed via the routing layer using something like domain sharding. Using cells has numerous advantages among which, workload isolation, failure containment, and the capability to scale out instead of up. Most importantly, because the size of a cell is known once it’s tested and understood, it becomes easier to manage and operate. Limits are known and replicated across all of the cells. The challenge then is knowing what cell size to set up since smaller cells are easier to test and operate. While larger cells are more cost efficient and make the overall system easier to understand. A general rule of thumb is to start with larger cells, and then as you grow, slowly reduce the size of your cells. Automation is going to be key, of course, in order to operate these cells at scale.
Beyond the infrastructure level, we move to the data center level. We have to consider the fact that our sample application is running in the cloud, so it has a dependency on cloud services and resources. AWS hosts its infrastructure in regions, which are independent of one another, giving us isolated services in each region. If we have mission critical systems, there may be a need to consider how to use multiple regions so that our system can continue to operate when a regional service is impaired, creating a fault isolation boundary for our system around any regional failure. Each region is made up of availability zones. These availability zones are independent fault isolated clusters of data centers. The availability zones are far enough apart, separated by a meaningful distance, so that they don’t share a fate. They’re close enough together to be useful as a single logical data center. By using multiple availability zones, we can protect ourselves against the disruption to any one availability zone in a region. Our system has a dependency on these availability zones, and the data centers that operate them. Our system also has a dependency on the AWS services like AWS Lambda and Amazon DynamoDB, which are operated and deployed within our selected region.
Looking back at our sample application, let’s review some of the mitigations that we have in place to create boundaries around the failure domains in our system. First, we’ve used redundancy to limit the failure of the database server. If the database fails, the system will switch over to the secondary database, which was kept in sync with the primary. We also have redundancy across the application servers, which operate independently across availability zones, and the application code will timeout and reconnect to the database if and/or when that database failure does occur. Also, where the application communicates with AWS Lambda services, we have used timeouts and retries with exponential backoff to send requests to the Lambda service. Then, finally, the Lambda service and the DynamoDB service operate across multiple availability zones, with rate limiting in place to improve their availability.
Initial Tests for Chaos Engineering
To test these fault isolation boundaries in our system, we’re going to use chaos engineering to simulate some of the failures that we’ve discussed earlier, and ensure that our system behaves as it was designed. We’ll introduce an initial set of tests that we can use to begin testing our fault isolation boundaries. This is not a chaos engineering talk. However, it’s worth a recap of chaos engineering theory and process just briefly. This talk is intended for teams who are new to chaos engineering, and these tests assume that the system they are testing on exist in a single AWS account by itself. As such, please do not inject these faults into a production environment until after the system has demonstrated a capacity to survive these tests in a non-production environment.
Earlier, we identified some failure domains for our system, and now, using the chaos engineering process, we will perform some initial tests to observe how our system performs when there is a failure with an availability zone, or with an AWS regional service. An initial test has two characteristics. The first is that the test is broadly applicable to most systems. The second is that it can be easily simulated or performed, having minimal complexity. For our sample system, there are a few initial tests that we can perform, which meet this criteria, and will allow us to begin to test our system and to develop our chaos engineering expertise. The set of initial tests includes whether the system can continue to function if it loses any one of the zonal resources such as a virtual machine or a single database instance. Also, whether the system can continue to function if an entire availability zone is disrupted, potentially losing roughly one-third of the compute resources required to run the system. Also, whether the system can continue to function if an entire service dependency is disrupted.
Let’s talk a little bit more about each of these initial tests. The first initial test to be applied to a singular resource will test whether a system can withstand a compute instance being stopped or a database falling over. These can easily be tested using AWS APIs. The command line examples that you see here use the AWS CLI tool to terminate an EC2 instance, or to cause an RDS database to failover. Both of these simulate some disruption to the resource software, virtual resource, or physical compute resource which is hosting the targeted capability. Going beyond the zonal resource, we can now move on to the shared dependency of the availability zone. We see another set of CLI commands here that can be used to simulate disruption of the network within the availability zone. These AWS CLI commands create a stateless firewall in the form of a network access control list, which drops all incoming and outgoing network traffic. The resulting network access control list can then be applied to all of the subnets in an availability zone in order to simulate a loss of network connectivity to the resources in that availability zone. This is the first initial test that we will be demonstrating in a moment. The other initial tests that we want to use today, was a disruption to a regional service. We can do this at the networking layer as in the previous slide, but for variety, these CLI commands that you see here will show the creation of a permissions policy, which prevents access to the AWS Lambda service. This will cause the service to respond with an Access Denied response, which we can use to simulate the service responding with a non-200 response to our requests. We will demonstrate this disruption as a part of the second test.
Demonstration
Let’s get into the demonstration. As part of that, I’ll briefly introduce the two chaos testing tools that we will use. As was mentioned earlier, there is a process to follow when performing an experiment, verifying the steady state, introducing a failure, observing the system, possibly even canceling the experiment if production is impacted. There are numerous tools available for performing chaos experiments. You can do things manually to get started using the CLI commands we showed earlier, for example, or you can go a step beyond manual and rely on shell scripts of some sort. For today’s test, though, I will rely on two tools, one per experiment, which provides some assistance in terms of the scripting and execution of our experiments. The first, AWS Fault Injection Simulator, is a prescriptive framework that allows us to define reusable experiment templates, and to execute them serverlessly. The execution history of each experiment is kept by the service for record keeping purposes, and can integrate with other AWS services such as AWS Systems Manager to give you the ability to extend its failure simulation capabilities. AWS FIS is also the only chaos testing tool that I know of, which can cause the AWS EC2 control plane to respond with API level errors.
The second tool that we’ll use is the Chaos Toolkit, which is an open source command line tool that executes experiments that are defined as JSON or YAML files. It supports extensions written in Python for interacting with numerous cloud providers, and container orchestration engines. Like the Fault Injection Simulator, the Chaos Toolkit keeps a log of the actions it takes and the results of each. Both of these tools enforce a structure much like we described earlier in terms of defining the steady state, the methods for injecting failures, and then observing the steady state to determine if the experiment was successful. For the system we have today, there’s also a dashboard that has been created. This dashboard has both the low level metrics and the high level KPIs that we will use to define our steady state. Along the bottom of this dashboard, we have counters and metrics for datum such as CPU usage, and the number of API calls to the AWS Lambda service. Up towards the top, we’re also tracking the client experience for those hitting the web application. Our key measure is going to be the percentage of successful requests that have been returned by the system. In addition to the metrics, we also have an alarm that is set to trigger if the percentage of HTTP 200 responses drops below 90%.
Demo: Simulate Availability Zone Failure
For this first demonstration, let’s use the AWS Fault Injection Simulator to simulate a network disruption across an availability zone using a network access control list. This system has been deployed into a test environment not into production. As such, we have a load generator running that is issuing requests to this system. You can see here in this dashboard a reflection of those requests as they hit the different API endpoints that are hosted by our application server. You can also see that the system is currently in its steady state as 100% of the requests that it’s receiving, are being returned successfully. If we look a little bit under the covers, we’ll see that there are currently three application servers running, all of them are healthy and handling traffic that are distributed by the load balancer.
If we take a look now at the AWS FIS console, we can see here a copy of the template that we’ve defined for today’s experiment. This template is defined in order to create and orchestrate the failure of an AWS availability zone, or rather to simulate that failure in order to allow us to test our application. The experiment first starts by confirming that the system is in its steady state. It does this by checking that alarm that we’ve defined earlier. That alarm again looks at the percentage of successful requests and ensures that it is above 90%. After confirming the steady state, step two is to execute an automation document that is hosted by Systems Manager. AWS Systems Manager will then execute that document using the input parameters that are provided to it by the Fault Injection Simulator. You can see those input parameters being highlighted here. Some of the key parameters that are going into that document are the VPC that contains the resources that is running our application, as well as the availability zone that we want to have failed by the document.
If we take a look at the automation document itself, we see that here in the Systems Manager console. There’s a number of bits of metadata associated with this document, such as any security risks that are associated with executing it, the input parameters that the document expects to receive, notably the AWS availability zone. Also, details about the support that this document has for things like cancellation and rollbacks. It’s important that if there is something that goes wrong, or when the experiment is concluded, that this document undoes or removes those network access control lists that it creates. We can see here the steps that the document follows to create the network access control list, attach it to the subnets, wait a period, and then remove them. Here we see the source code of this document, which is a combination of YAML and Python code. The YAML defines all of the metadata for the document, the input parameters, for example, that we’ve just discussed. As well as wraps around the Python code that defines how this document will execute and how it will interact with the different AWS APIs in order to create the network access control lists and attach them to the appropriate subnets.
Let’s go ahead and get the experiment started. We go back to the Fault Injection Simulator, and from actions, we click Start. As a part of this, we’re creating an experiment based on the experiment template. As a part of this, we’re going to add some metadata to this experiment so that we can go back later on and compare this experiment and its results with other experiments that are being run in the environment. After we’ve associated a couple of tags, we will then click Start experiment. The Fault Injection Simulator will confirm that we want to run this experiment. We type start and click the button, and away we go. After a few minutes, you’ll see that the Fault Injection Simulator has confirmed and is now running. We can see that reflected here in the dashboard as well for the system. You’ll notice that the alarm in the upper left-hand side of the dashboard has a small dip in it. We’ll come back to that in a moment. We can also see that the infrastructure is starting to fail. It’s reporting unhealthy nodes. Some of the application servers are not responding properly to their health checks. The autoscaling group is trying to refresh and replace those in order to achieve a healthy set of nodes.
Going back to the Fault Injection Simulator, we can see that it’s now executed the automation document and has now completed the experiment. We’re able to review the results or the details about that document. We can also see that the experiment itself is still making some final storing metadata and recording the logs. We’re just going to click refresh a couple of times while we wait for that experiment to conclude. The experiment is now completed, it’s now logged in the Fault Injection Simulator. We can go back and dive deeper into the logs and the actions that were taken, and compare the behavior of that against other experiments that were performed. If we go back to the dashboard for our system, we should see that it has now resumed at steady state. Actually, technically, it never left its steady state, so, actually, our hypothesis for this experiment holds. If we take a look at that small dip, I think we can see that the percent correct or the percent of percentage of requests that were served, never dropped below 98% or 97%. Our hypothesis is holding true, the chaos experiment is successful. We can now move on to our second chaos experiment. If we take a quick look at the infrastructure, we can also see that we now do have three healthy nodes as well. We are fully back online and having recovered from the disruption to the network.
Demo: Simulate Region Failure
For the second demonstration, we’re going to hypothesize that the system will be able to continue functioning in the event that there is a disruption to the AWS Lambda service. As you saw earlier, we have included some fault isolation patterns such as retries and timeouts. What will be the effect on this system then if it’s unable to invoke the AWS Lambda service? Again, we’ve got our dashboard here, we’re going to first start by confirming the steady state of the system. We can see that there are a number of requests that are being sent to this by our load generator. Our alarm is currently in a healthy state. Our percent request complete is above 90%, so we’re good to go for the experiment.
Let’s have a quick look at the underlying infrastructure. Again, just confirming, we’ve got three healthy application servers. We haven’t looked at the console for it, but the database is also up and running and healthy, so we’re good to go. As we said, the Chaos Toolkit is a command line tool that relies on experiments that are defined in YAML, or JSON documents. This is our experiment for today. It’s a YAML document, obviously. Some of the key sections that I want to call out include the configuration section. This experiment is going to take an IAM policy and attach it to an IAM role that is associated with our application server. This will prevent the system from communicating with the AWS Lambda service. Using those variables that are defined in the configuration, we’ll then use those later on as part of the method. The next section that we are focusing in on is the steady state hypothesis. This is a standard part of the Chaos Toolkit experiment. It’ll use the AWS plugin, Forecast toolkit, to check that CloudWatch alarm and ensure that it is in an ok state.
If the system passes that test, the Chaos Toolkit will then move on to executing the method. Here, again, it’s going to use the AWS plugin for Chaos Toolkit to attach that IAM policy to the role, which is associated with our application servers. After it carries out that action, it’ll then pause for about 900 seconds or 15 minutes in order to give the system a chance to respond. After it’s done waiting, it’ll then check that alarm again, to ensure that it’s still in an ok state. Once it’s done, it’ll then execute its rollback procedure, which will detach that IAM policy from the AWS IAM role. Just a quick look at the IAM policy that we’re talking about here. You can see it’s a very simple IAM policy designed to deny access to the Invoke function and InvokeAsync APIs for any Lambda functions that are in the Ireland region.
Going to our command line, we’re going to execute the Chaos Toolkit command line tool. We’re going to give it our experiment.yaml file so it knows what experiment to execute. We’re going to tell it to roll back when it’s done, and to log everything to an exp-fail-lambda-run-003.log. We can see here in its output that it’s checked the steady state of the system, found that it’s in a good state, and proceeds by executing the action so that it attaches the policy to the IAM role. It’s now pausing for that 900 seconds as we saw in the experiment definition. Roll forward a few minutes, we can check our dashboard for the system and see that there is indeed quite an impact to the system. It looks like the percent correct is sitting around 60% at the moment, so it’s dropping drastically beyond that 90% threshold that we try to keep as part of our SLA. We can see over on the right-hand side that we’ve got 500 or so odd requests that are successfully being serviced, while 900 in total are being issued to the system. The reason for this discrepancy is that there’s actually two APIs that the system makes available. One of them relies on AWS Lambda as part of its critical path, one of them does not. We can also see that the infrastructure is starting to thrash as different application servers get replaced, as the system tries to replace them with healthy instances. Going back to the dashboard, we’re now at about 9% of successful requests being serviced. This is roughly in line with the distribution of traffic that our load generator is producing, where 10% of the requests are being issued to the path that doesn’t rely on AWS Lambda, 90% of the traffic does rely on AWS Lambda. This is about as I think we might expect.
Looking back again, the infrastructure is thrashing as instances are brought online, they’re failing their health checks, so they’re getting replaced. At this point, the experiment has concluded, Chaos Toolkit has waited 15 minutes, and rechecked that CloudWatch alarm. It’s found that it is not in an ok state, so it rolls back to detach that IAM policy and marks the experiment as deviated or essentially as a failure. We can then go through the logs, go through the dashboards, and start to perform our learning exercise in order to understand what happened. If we go back to the dashboard, again, we want to ensure that the system has resumed its steady state. The experiment isn’t over until the system has resumed its steady state. We can see that actually it has, with that IAM policy detached, it has resumed its steady state. Now that effectively the AWS Lambda service is back available, we’re able to issue requests to it. We are now successfully processing all of the requests that are flowing through the system, so we have effectively recovered. Equally, if we check the infrastructure, we’ll start to see that we do have some healthy instances that are now coming online. I’d imagine we’ll have one in each availability zone again shortly.
Key Takeaways
What are some key takeaways, and what have we shown today? From our first initial test, we demonstrated that the system was unaffected by the loss of an availability zone. As you saw, this system continued with a load balancer routing requests around the affected resources. The second initial test which prevented access to the AWS Lambda service demonstrated that, in fact, our system was hugely affected by not being able to successfully invoke its Lambda function. No amount of retries or timeouts were going to help if the service becomes completely unavailable. What we’ll need to do now is to understand and estimate the likelihood of this event, AWS Lambda not being reachable. If it’s a high enough priority, devise a mitigation for this failure mode, perhaps caching the data locally or posting the data to a queue where it can be processed later on by a Lambda function. Or perhaps we can call a Lambda function located in a different AWS region, understanding that there will be additional network latency as a result of this. After implementing a fix, we can perform the experiment again, to observe whether the system is more resilient.
Wrap-Up
First, the initial tests presented today trade realism for simplicity. The failures simulated availability zone network failure and regional service failure, were very binary in their nature and unrealistic as a result. With the failure simulation in place, all network traffic was dropped, and all API requests were immediately denied. This provided us a way to quickly assess the ability of the system to withstand such failures. However, in reality, it’s far more likely that only some of the network traffic will be dropped, and some of the API requests will receive no response, a delayed response, or a non-200 response. In this way, partial failures or gray failures are far more likely but are also harder to simulate. You can simulate them using tools like traffic control for Linux or a transparent network proxy. However, for initial testing purposes, a binary simulation is sufficient. Also, after the system is able to continue operating in the face of binary failure simulation, then you can consider taking on the additional complexity of simulating partial failures.
It can seem at first like a very large problem space to have to search when you first look to apply chaos engineering to a system. Other engineering disciplines for mission critical systems have devised formalized processes for creating an exhaustive inventory of all of the failures, which could affect the system. These are good processes to use and to be aware of. To get started, you could also simply look at your architecture and ask yourself, what happens when a line goes away, or when a box is disrupted? Where are the single points of failure in the architecture? This will be a good starting point to identify your failure domains and to begin to catalog your fault isolation boundaries. With that catalog to hand, you can then come up with ways to simulate these failures, and to ensure that your fault isolation boundaries are effective, and operating as designed.
Questions and Answers
Watt: You mentioned about some formal hazard analysis methods like the failure mode effect analysis. What are the differences between these methods? How do you actually choose which one to use?
Barto: It’s worth pointing out that what we’re trying to do with these hazard analysis methods is identify all those things that could cause our application to have a bad day. Initially, that can be quite a large space. If we are as developers just throwing darts at the wall and trying to figure out what breaks the system, it could be a very long time before we have a complete set of those hazards. Regardless of whether we’re talking about fault tree analysis, or failure mode effects analysis, or any of the other methods, that’s the goal at the end of the day, is to have that definitive list of this is what can cause us problems. There’s been some research done to understand where they overlap. As you said, are all of these at the end of the day equivalent? If we come up and we perform a system theoretic process analysis, are we going to come up with the same set of hazards that we would do if we did a failure mode effect analysis?
The research has shown that there’s roughly about a 60% or 70% overlap, but each one of those analysis methods does also find outliers that other analysis methods don’t find. What I would suggest is that, as engineers, a lot of us think bottom up. We think about the lower order architectural components in our system, and less about the higher order controls and processes that we use to govern that system. In that way, something like a failure mode effects analysis is fairly recognizable to us as engineers, and I see a lot of adoption of that within different teams. All of them are perfectly valid. It’s worth doing a little bit of reading, not extensive, but getting more familiarity with fault tree analysis, lineage driven fault analysis, and any of the other ones that you can come across, and just getting a feel for which one you think is going to work best for you and for your team.
Watt: What would happen like say, now you’ve got your architecture diagram, and you’ve got loads of possibilities, so many things can go wrong. How do you work out which ones will give you best bang for your buck in terms of testing them? How do you work out which ones to focus on and which ones maybe you should maybe leave for later?
Barto: Again, as engineers, we focus a lot on the architecture, we focus a lot on the systems. It’s important to recall that the reason we’ve got these IT systems running in our environment is to deliver different business services to our clients and to our customers. I always encourage people to start with those, with the services rather than the system itself. The reason for that is, is that any given system will probably host multiple services. In the demonstration today we saw a single system that had two APIs, a very simplistic example, but it illustrates the point. In that, depending on which API you were talking with, which service you were consuming from the system, your request either succeeded or failed based on the subsystems that that request went through in order to get serviced.
I would encourage customers, or I would encourage teams to look at the services that their business systems provide, and to then identify the components that support that service. This allows us to make the problem space a little bit smaller in terms of not considering all of the different permutations, but also allows us to identify deep criticality or importance of any one of those. Again, a single system delivers multiple services, what are the really important services? Because at the end of the day, once you do identify these different failure modes or hazards, it’s going to cost engineering time and effort. You’re going to be not implementing features while you’re implementing those mitigations. You want to make sure that we’re going to get return on the investment that we’re going to spend putting in.
See more presentations with transcripts