Month: March 2018
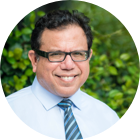
MMS • RSS
Article originally posted on Data Science Central. Visit Data Science Central
Summary: GDPR carries many new data and privacy requirements including a “right to explanation”. On the surface this appears to be similar to US rules for regulated industries. We examine why this is actually a penalty and not a benefit for the individual and offer some insight into the actual wording of the GDPR regulation which also offers some relief.
GDPR is now just about 60 days away and there’s plenty to pay attention to especially in getting and maintaining permission to use a subscriber’s data. It’s a tough problem.
If you’re an existing social media platform like Facebook that’s a huge change to your basic architecture. If you’re just starting out in the EU there are some new third party offerings that promise to keep track of things for you (Integris, Kogni, and Waterline all emphasized this feature at the Strata Data San Jose conference this month).
The item that we keep coming back to however is the “right to explanation”.
In the US we’re not strangers to this requirement if you’re in one of the regulated industries like lending or insurance where you already bear this burden. US regulators have been pretty specific that this means restricting machine learning to techniques to the simplest regression and decision trees that have the characteristic of being easy to understand and are therefore judged to be ‘interpretable’.
Recently University of Washington professor and leading AI researcher Pedro Domingos created more than a little controversy when he tweeted “Starting May 25, the European Union will require algorithms to explain their output, making deep learning illegal”. Can this be true?
Trading Accuracy for Interpretability Adds Cost
The most significant issue is that restricting ourselves to basic GLM and decision trees directly trades accuracy for interpretability. As we all know, very small changes in model accuracy can leverage into much larger increases in the success of different types of campaigns and decision criteria. Intentionally forgoing the benefit of that incremental accuracy imposes a cost on all of society.
We just barely ducked the bullet of ‘disparate impact’ that was to have been a cornerstone of new regulation proposed by the last administration. Fortunately those proposed regs were abandoned as profoundly unscientific.
Still the costs of dumbing down analytics keep coming. Basel II and Dodd-Frank place a great deal of emphasis on financial institutions constantly evaluating and adjusting their capital requirements for risk of all sorts.
This has become so important that larger institutions have had to establish independent Model Validation Groups (MVGs) separate from their operational predictive analytics operation whose sole role is to constantly challenge whether the models in use are consistent with regulations. That’s a significant cost of compliance.
The Paradox: Increasing Interpretability Can Reduce Individual Opportunity
Here’s the real paradox. As we use less accurate techniques to model, that inaccuracy actually excludes some individuals who would have been eligible for credit, insurance, a loan, or other regulated item, and includes some other individuals whose risk should have invalidated them for selection. This last increases the rate of bad debt or other costs of bad decisions that gets reflected in everyone’s rates.
At the beginning of 2017, Equifax, the credit rating company quantified this opportunity/cost imbalance. Comparing the mandated simple models to modern deep learning techniques they reexamined the last 72 months of their data and decisions.
Peter Maynard, Senior Vice President of Global Analytics at Equifax says the experiment improved model accuracy 15% and reduced manual data science time by 20%.
The ‘Right to Explanation’ is Really No Benefit to the Individual
Regulators apparently think that rejected consumers should be consoled by this proof that the decision was fair and objective.
However, if you think through to the next step, what is the individual’s recourse? The factors in any model are objective, not subjective. It’s your credit rating, your income, your driving history, all facts that you cannot change immediately in order to qualify.
So the individual who has exercised this right gained nothing in terms of immediate access to the product they desired, and quite possibly lost out on qualifying had a more accurate modeling technique been used.
Peter Maynard of Equifax goes on to say that after reviewing the last two years of data in light of the new model they found many declined loans that could have been made safely.
Are We Stuck With the Simplest Models?
Data scientists in regulated industries have been working this issue hard. There are some specialized regression techniques like Penalized Regression, Generalized Additive Models, and Quantile Regression all of which yield somewhat better and still interpretable results.
This last summer, a relatively new technique called RuleFit Ensemble Models was gaining prominence and also promised improvement.
Those same data scientists have also been clever about using black box deep neural nets first to model the data, achieving the most accurate models, and then using those scores and insights to refine and train simpler techniques.
Finally, that same Equifax study quoted above also resulted in a proprietary technique to make deep neural nets explainable. Apparently Equifax has persuaded some of their regulators to accept this new technique but are so far keeping it to themselves. Perhaps they’ll share.
The GDPR “Right to Explanation” Loophole
Thanks to an excellent blog by Sandra Wachter who is an EU Lawyer and Research Fellow at Oxford we discover that the “right to explanation” may not be all that it seems.
It seems that a legal interpretation of “right to explanation” is not the same as in the US. In fact, per Wachter’s blog, “the GDPR is likely to only grant individuals information about the existence of automated decision-making and about “system functionality”, but no explanation about the rationale of a decision.”
Wachter goes on to point out that “right to explanation” was written into a section called Recital 71 which is important because that section is meant as guidance but carries no legal basis to establish stand-alone rights. Wachter observes that this placement appears intentional indicating that legislators did not want to make this a right on the same level as other elements of the GDPR.
Are We Off the Hook for Individual Level Explanations?
At least in the EU, the legal reading of “right to explanation” seems to give us a clear pass. Will that hold? That’s completely up to the discretion of the EU legislators, but at least for now, as written, “right to explanation” should not be a major barrier to operations in the post GDPR world.
About the author: Bill Vorhies is Editorial Director for Data Science Central and has practiced as a data scientist since 2001. He can be reached at:
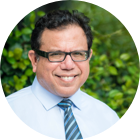
MMS • RSS
Article originally posted on Data Science Central. Visit Data Science Central
Summary: We’re stuck. There hasn’t been a major breakthrough in algorithms in the last year. Here’s a survey of the leading contenders for that next major advancement.
We’re stuck. Or at least we’re plateaued. Can anyone remember the last time a year went by without a major notable advance in algorithms, chips, or data handling? It was so unusual to go to the Strata San Jose conference a few weeks ago and see no new eye catching developments.
As I reported earlier, it seems we’ve hit maturity and now our major efforts are aimed at either making sure all our powerful new techniques work well together (converged platforms) or making a buck from those massive VC investments in same.
I’m not the only one who noticed. Several attendees and exhibitors said very similar things to me. And just the other day I had a note from a team of well-regarded researchers who had been evaluating the relative merits of different advanced analytic platforms, and concluding there weren’t any differences worth reporting.
Why and Where are We Stuck?
Where we are right now is actually not such a bad place. Our advances over the last two or three years have all been in the realm of deep learning and reinforcement learning. Deep learning has brought us terrific capabilities in processing speech, text, image, and video. Add reinforcement learning and we get big advances in game play, autonomous vehicles, robotics and the like.
We’re in the earliest stages of a commercial explosion based on these like the huge savings from customer interactions through chatbots; new personal convenience apps like personal assistants and Alexa, and level 2 automation in our personal cars like adaptive cruise control, accident avoidance braking, and lane maintenance.
Tensorflow, Keras, and the other deep learning platforms are more accessible than ever, and thanks to GPUs, more efficient than ever.
However, the known list of deficiencies hasn’t moved at all.
- The need for too much labeled training data.
- Models that take either too long or too many expensive resources to train and that still may fail to train at all.
- Hyperparameters especially around nodes and layers that are still mysterious. Automation or even well accepted rules of thumb are still out of reach.
- Transfer learning that means only going from the complex to the simple, not from one logical system to another.
I’m sure we could make a longer list. It’s in solving these major shortcomings where we’ve become stuck.
What’s Stopping Us
In the case of deep neural nets the conventional wisdom right now is that if we just keep pushing, just keep investing, then these shortfalls will be overcome. For example, from the 80’s through the 00’s we knew how to make DNNs work, we just didn’t have the hardware. Once that caught up then DNNs combined with the new open source ethos broke open this new field.
All types of research have their own momentum. Especially once you’ve invested huge amounts of time and money in a particular direction you keep heading in that direction. If you’ve invested years in developing expertise in these skills you’re not inclined to jump ship.
Change Direction Even If You’re Not Entirely Sure What Direction that Should Be
Sometimes we need to change direction, even if we don’t know exactly what that new direction might be. Recently leading Canadian and US AI researchers did just that. They decided they were misdirected and needed to essentially start over.
This insight was verbalized last fall by Geoffrey Hinton who gets much of the credit for starting the DNN thrust in the late 80s. Hinton, who is now a professor emeritus at the University of Toronto and a Google researcher, said he is now “deeply suspicious“ of back propagation, the core method that underlies DNNs. Observing that the human brain doesn’t need all that labeled data to reach a conclusion, Hinton says “My view is throw it all away and start again”.
So with this in mind, here’s a short survey of new directions that fall somewhere between solid probabilities and moon shots, but are not incremental improvements to deep neural nets as we know them.
These descriptions are intentionally short and will undoubtedly lead you to further reading to fully understand them.
Things that Look Like DNNs but are Not
There is a line of research closely hewing to Hinton’s shot at back propagation that believes that the fundamental structure of nodes and layers is useful but the methods of connection and calculation need to be dramatically revised.
Capsule Networks (CapsNet)
It’s only fitting that we start with Hinton’s own current new direction in research, CapsNet. This relates to image classification with CNNs and the problem, simply stated, is that CNNs are insensitive to the pose of the object. That is, if the same object is to be recognized with differences in position, size, orientation, deformation, velocity, albedo, hue, texture etc. then training data must be added for each of these cases.
In CNNs this is handled with massive increases in training data and/or increases in max pooling layers that can generalize, but only by losing actual information.
The following description comes from one of many good technical descriptions of CapsNets, this one from Hackernoon.
Capsule is a nested set of neural layers. So in a regular neural network you keep on adding more layers. In CapsNet you would add more layers inside a single layer. Or in other words nest a neural layer inside another. The state of the neurons inside a capsule capture the above properties of one entity inside an image. A capsule outputs a vector to represent the existence of the entity. The orientation of the vector represents the properties of the entity. The vector is sent to all possible parents in the neural network. Prediction vector is calculated based on multiplying its own weight and a weight matrix. Whichever parent has the largest scalar prediction vector product, increases the capsule bond. Rest of the parents decrease their bond. This routing by agreement method is superior to the current mechanism like max-pooling.
CapsNet dramatically reduces the required training set and shows superior performance in image classification in early tests.
gcForest
In February we featured research by Zhi-Hua Zhou and Ji Feng of the National Key Lab for Novel Software Technology, Nanjing University, displaying a technique they call gcForest. Their research paper shows that gcForest regularly beats CNNs and RNNs at both text and image classification. The benefits are quite significant.
- Requires only a fraction of the training data.
- Runs on your desktop CPU device without need for GPUs.
- Trains just as rapidly and in many cases even more rapidly and lends itself to distributed processing.
- Has far fewer hyperparameters and performs well on the default settings.
- Relies on easily understood random forests instead of completely opaque deep neural nets.
In brief, gcForest (multi-Grained Cascade Forest) is a decision tree ensemble approach in which the cascade structure of deep nets is retained but where the opaque edges and node neurons are replaced by groups of random forests paired with completely-random tree forests. Read more about gcForest in our original article.
Pyro and Edward
Pyro and Edward are two new programming languages that merge deep learning frameworks with probabilistic programming. Pyro is the work of Uber and Google, while Edward comes out of Columbia University with funding from DARPA. The result is a framework that allows deep learning systems to measure their confidence in a prediction or decision.
In classic predictive analytics we might approach this by using log loss as the fitness function, penalizing confident but wrong predictions (false positives). So far there’s been no corollary for deep learning.
Where this promises to be of use for example is in self-driving cars or aircraft allowing the control to have some sense of confidence or doubt before making a critical or fatal catastrophic decision. That’s certainly something you’d like your autonomous Uber to know before you get on board.
Both Pyro and Edward are in the early stages of development.
Approaches that Don’t Look Like Deep Nets
I regularly run across small companies who have very unusual algorithms at the core of their platforms. In most of the cases that I’ve pursued they’ve been unwilling to provide sufficient detail to allow me to even describe for you what’s going on in there. This secrecy doesn’t invalidate their utility but until they provide some benchmarking and some detail, I can’t really tell you what’s going on inside. Think of these as our bench for the future when they do finally lift the veil.
For now, the most advanced non-DNN algorithm and platform I’ve investigated is this:
Hierarchical Temporal Memory (HTM)
Hierarchical Temporal Memory (HTM) uses Sparse Distributed Representation (SDR) to model the neurons in the brain and to perform calculations that outperforms CNNs and RNNs at scalar predictions (future values of things like commodity, energy, or stock prices) and at anomaly detection.
This is the devotional work of Jeff Hawkins of Palm Pilot fame in his company Numenta. Hawkins has pursued a strong AI model based on fundamental research into brain function that is not structured with layers and nodes as in DNNs.
HTM has the characteristic that it discovers patterns very rapidly, with as few as on the order of 1,000 observations. This compares with the hundreds of thousands or millions of observations necessary to train CNNs or RNNs.
Also the pattern recognition is unsupervised and can recognize and generalize about changes in the pattern based on changing inputs as soon as they occur. This results in a system that not only trains remarkably quickly but also is self-learning, adaptive, and not confused by changes in the data or by noise.
We featured HTM and Numenta in our February article and we recommend you read more about it there.
Some Incremental Improvements of Note
We set out to focus on true game changers but there are at least two examples of incremental improvement that are worthy of mention. These are clearly still classical CNNs and RNNs with elements of back prop but they work better.
Network Pruning with Google Cloud AutoML
Google and Nvidia researchers use a process called network pruning to make a neural network smaller and more efficient to run by removing the neurons that do not contribute directly to output. This advancement was rolled out recently as a major improvement in the performance of Google’s new AutoML platform.
Transformer
Transformer is a novel approach useful initially in language processing such as language-to-language translations which has been the domain of CNNs, RNNs and LSTMs. Released late last summer by researchers at Google Brain and the University of Toronto, it has demonstrated significant accuracy improvements in a variety of test including this English/German translation test.
The sequential nature of RNNs makes it more difficult to fully take advantage of modern fast computing devices such as GPUs, which excel at parallel and not sequential processing. CNNs are much less sequential than RNNs, but in CNN architectures the number of steps required to combine information from distant parts of the input still grows with increasing distance.
The accuracy breakthrough comes from the development of a ‘self-attention function’ that significantly reduces steps to a small, constant number of steps. In each step, it applies a self-attention mechanism which directly models relationships between all words in a sentence, regardless of their respective position.
Read the original research paper here.
A Closing Thought
If you haven’t thought about it, you should be concerned at the massive investment China is making in AI and its stated goal to overtake the US as the AI leader within a very few years.
In an article by Steve LeVine who is Future Editor at Axios and teaches at Georgetown University he makes the case that China may be a fast follower but will probably never catch up. The reason, because US and Canadian researchers are free to pivot and start over anytime they wish. The institutionally guided Chinese could never do that. This quote from LeVine’s article:
“In China, that would be unthinkable,” said Manny Medina, CEO at Outreach.io in Seattle. AI stars like Facebook’s Yann LeCun and the Vector Institute’s Geoff Hinton in Toronto, he said, “don’t have to ask permission. They can start research and move the ball forward.”
As the VCs say, maybe it’s time to pivot.
About the author: Bill Vorhies is Editorial Director for Data Science Central and has practiced as a data scientist since 2001. He can be reached at:
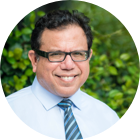
MMS • RSS
Article originally posted on MongoDB. Visit MongoDB
NEW YORK, March 13, 2018 /PRNewswire/ — MongoDB, Inc. (NASDAQ: MDB), the leading modern, general purpose database platform, today announced its financial results for the fourth quarter and fiscal year ended January 31, 2018.
“MongoDB’s fourth quarter results capped a milestone year for the company and were highlighted by strong customer additions and 50% revenue growth,” said Dev Ittycheria, President and Chief Executive Officer of MongoDB. “A rapidly growing number of customers recognize that MongoDB offers a superior way to work with data, the ability to intelligently put data where it is needed and the freedom to run anywhere. Moreover, we are very pleased with the continued rapid growth of MongoDB Atlas, which reflects increasing demand for a flexible, high performance and cost-effective database-as-a-service offering.”
Ittycheria added, “The recent announcement that MongoDB 4.0 will support multi-document ACID transactions is a major product breakthrough. With this new capability, we believe that our modern general purpose database platform can address any possible use case far better than a traditional database. In fiscal 2019, we intend to build on our market and product momentum and are well positioned to drive continued strong revenue growth.”
Fourth Quarter Fiscal 2018 Financial Highlights
- Revenue: Total revenue was $45.0 million in the fourth quarter fiscal 2018, an increase of 50% year-over-year. Subscription revenue was $41.9 million, an increase of 54% year-over-year, and services revenue was $3.2 million, an increase of 16% year-over-year.
- Gross Profit: Gross profit was $32.6 million in the fourth quarter fiscal 2018, representing a 72% gross margin, consistent with the year-ago period. Non-GAAP gross profit was $33.0 million, representing a 73% non-GAAP gross margin.
- Loss from Operations: Loss from operations was $27.3 million in the fourth quarter fiscal 2018, compared to $21.3 million in the year-ago period. Non-GAAP loss from operations was $21.1 million, compared to $16.8 million in the year-ago period.
- Net Loss: Net loss was $26.4 million, or $0.52 per share based on 50.3 million weighted-average shares outstanding, in the fourth quarter fiscal 2018. This compares to $21.8 million, or $1.69 per share based on 12.9 million weighted-average shares outstanding, in the year-ago period. Non-GAAP net loss was $20.2 million, or $0.40 per share based on 50.3 million weighted-average shares outstanding, which we refer to as non-GAAP net loss per share. This compares to $17.2 million, or $0.44 per share based on 38.8 million non-GAAP weighted-average shares outstanding, in the year-ago period.
- Cash Flow: As of January 31, 2018, MongoDB had $279.5 million in cash, cash equivalents, short-term investments and restricted cash. During the three months ended January 31, 2018, MongoDB used $7.7 million of cash in operations and $0.4 million in capital expenditures, leading to negative free cash flow of $8.1 million, compared to negative free cash flow of $10.3 million in the year-ago period.
Full Year Fiscal 2018 Financial Highlights
- Revenue: Total revenue was $154.5 million for the full year fiscal 2018, an increase of 52% year-over-year. Subscription revenue was $141.5 million, an increase of 55% year-over-year, and services revenue was $13.0 million, an increase of 29% year-over-year.
- Gross Profit: Gross profit was $111.7 million for the full year fiscal 2018, representing a 72% gross margin, an improvement compared to 71% in the prior year. Non-GAAP gross profit was $112.9 million, representing a 73% non-GAAP gross margin.
- Loss from Operations: Loss from operations was $97.3 million for the full year fiscal 2018, compared to $85.9 million in the prior year. Non-GAAP loss from operations was $76.0 million, compared to $64.9 million in the prior year.
- Net Loss: Net loss was $96.4 million, or $4.06 per share based on 23.7 million weighted-average shares outstanding, for the full year fiscal 2018. This compares to $86.7 million, or $7.10 per share based on 12.2 million weighted-average shares outstanding, in the prior year. Non-GAAP net loss was $75.2 million, or $1.74 per share based on 43.2 million non-GAAP weighted-average shares outstanding. This compares to $65.7 million, or $1.73 per share based on 38.1 million non-GAAP weighted-average shares outstanding, in the prior year.
- Cash Flow: During the twelve months ended January 31, 2018, MongoDB used $44.9 million of cash in operations and $2.1 million in capital expenditures, leading to negative free cash flow of $47.0 million, compared to negative free cash flow of $39.8 million in the prior year.
A reconciliation of each Non-GAAP measure to the most directly comparable GAAP measure has been provided in the financial statement tables included at the end of this press release. An explanation of these measures is also included below under the heading “Non-GAAP Financial Measures.”
Fourth Quarter Fiscal 2018 and Recent Business Highlights
- Announced that MongoDB 4.0, scheduled for release in the summer of 2018, will extend ACID transaction support currently available in a single document to multiple documents. Transactions provide a set of guarantees for data integrity when making changes to the database. While single-document transactions are sufficient for most use cases, the addition of multi-document transactions gives companies the peace of mind to build any application on MongoDB. With the addition of transactions, we believe MongoDB will be the best choice for any use case, making it easier than ever for customers to choose MongoDB as their default database platform. The beta program for multi-document ACID transactions is currently underway with customers.
- Saw significant momentum with MongoDB Atlas, our fully managed database-as-a-service offering. A year-and-a-half since its launch, Atlas now comprises 11% of total revenue for the fourth quarter fiscal 2018, representing over 500% year-over-year growth and over 40% quarter-over-quarter growth. Atlas has rapidly grown to more than 3,400 customers due to its strong product-market fit and customers’ embracing MongoDB’s “run anywhere” strategy.
- Results from Stack Overflow’s Annual Developer Survey, which included more than 100,000 global respondents, were announced today. MongoDB was named the database developers most want to work with for the second year in a row. This report from the largest online community for developers is further demonstration of MongoDB’s clear leadership as the most popular next-generation database platform.
- Increased momentum in MongoDB’s partner ecosystem. In December 2017, MongoDB announced the availability of MongoDB Atlas on AWS Marketplace, making it easier for the more than 160,000 existing AWS customers to buy and consume MongoDB Atlas. MongoDB also joined the AWS SaaS Sales Alignment Program, enabling the AWS sales force to drive MongoDB Atlas revenue through co-selling. MongoDB and Microsoft also launched a new co-sell program for MongoDB Atlas on Microsoft Azure. Furthermore, Tata Consulting Services developed a mainframe modernization practice built around MongoDB and elevated MongoDB as a Top 20 global strategic partner.
Business Outlook
Based on information as of today, March 13, 2018, MongoDB is issuing the following financial guidance for the first quarter and full year fiscal 2019:
First Quarter Fiscal 2019 |
Full Year Fiscal 2019 |
|
Revenue |
$45.5 million to $46.5 million |
$211.0 million to $215.0 million |
Non-GAAP Loss from Operations |
$(22.0) million to $(21.5) million |
$(84.0) million to $(82.0) million |
Non-GAAP Net Loss per Share |
$(0.44) to $(0.43) |
$(1.66) to $(1.62) |
Reconciliation of non-GAAP loss from operations and non-GAAP net loss per share guidance to the most directly comparable GAAP measures is not available without unreasonable efforts on a forward-looking basis due to the high variability, complexity and low visibility with respect to the charges excluded from these non-GAAP measures; in particular, the measures and effects of stock-based compensation expense specific to equity compensation awards that are directly impacted by unpredictable fluctuations in our stock price. We expect the variability of the above charges to have a significant, and potentially unpredictable, impact on our future GAAP financial results.
Conference Call Information
MongoDB will host a conference call today, March 13, 2018, at 5:00 p.m. (Eastern Time) to discuss its financial results and business outlook. A live webcast of the call will be available on the “Investor Relations” page of MongoDB’s website at http://investors.mongodb.com. To access the call by phone, dial 800-239-9838 (domestic) or 323-794-2551 (international). A replay of this conference call will be available for a limited time at 844-512-2921 (domestic) or 412-317-6671 (international). The replay conference ID is 5850950. A replay of the webcast will also be available for a limited time at http://investors.mongodb.com.
About MongoDB
MongoDB is the leading modern, general purpose database platform, designed to unleash the power of software and data for developers and the applications they build. Headquartered in New York, MongoDB has more than 5,700 customers in over 90 countries. The MongoDB database platform has been downloaded over 35 million times and there have been more than 800,000 MongoDB University registrations.
Forward-Looking Statements
This press release includes certain “forward-looking statements” within the meaning of Section 27A of the Securities Act of 1933, as amended, or the Securities Act, and Section 21E of the Securities Exchange Act of 1934, as amended, including statements concerning our financial guidance for the first quarter and full year fiscal 2019, our position to execute on our go-to-market strategy, our introduction of future product enhancements and the potential advantages of those enhancements, and our ability to expand our leadership position and drive revenue growth. These forward-looking statements include, but are not limited to, plans, objectives, expectations and intentions and other statements contained in this press release that are not historical facts and statements identified by words such as “anticipate,” “believe,” “continue,” “could,” “estimate,” “expect,” “intend,” “may,” “plan,” “project,” “will,” “would” or the negative or plural of these words or similar expressions or variations. These forward-looking statements reflect our current views about our plans, intentions, expectations, strategies and prospects, which are based on the information currently available to us and on assumptions we have made. Although we believe that our plans, intentions, expectations, strategies and prospects as reflected in or suggested by those forward-looking statements are reasonable, we can give no assurance that the plans, intentions, expectations or strategies will be attained or achieved. Furthermore, actual results may differ materially from those described in the forward-looking statements and are subject to a variety of assumptions, uncertainties, risks and factors that are beyond our control including, without limitation: our limited operating history; our history of losses; failure of our database platform to satisfy customer demands; our investments in new products and our ability to introduce new features, services or enhancements; the effects of increased competition; our ability to effectively expand our sales and marketing organization; our ability to continue to build and maintain credibility with the developer community; our ability to add new customers or increase sales to our existing customers; our ability to maintain, protect, enforce and enhance our intellectual property; the growth and expansion of the market for database products and our ability to penetrate that market; our ability to maintain the security of our software and adequately address privacy concerns; our ability to manage our growth effectively and successfully recruit additional highly-qualified personnel; the price volatility of our common stock; and those risks detailed from time-to-time under the caption “Risk Factors” and elsewhere in our Securities and Exchange Commission (“SEC”) filings and reports, including our Quarterly Report on Form 10-Q filed on December 15, 2017, as well as future filings and reports by us. Except as required by law, we undertake no duty or obligation to update any forward-looking statements contained in this release as a result of new information, future events, changes in expectations or otherwise.
The development, release, and timing of any features or functionality described for our products remains at our sole discretion. This information is merely intended to outline our general product direction and it should not be relied on in making a purchasing decision nor is this a commitment, promise or legal obligation to deliver any material, code, or functionality.
Non-GAAP Financial Measures
This press release includes the following financial measures defined as non-GAAP financial measures by the SEC: non-GAAP gross profit, non-GAAP gross margin, non-GAAP loss from operations, non-GAAP net loss, non-GAAP net loss per share and free cash flow. Non-GAAP gross profit, non-GAAP gross margin, non-GAAP loss from operations and non-GAAP net loss exclude stock-based compensation expense and, in the case of non-GAAP net loss, change in fair value of warrant liability. Non-GAAP net loss per share is calculated by dividing non-GAAP net loss by the weighted-average shares used to compute net loss per share attributable to common stockholders, basic and diluted, and for periods prior to and including the period in which we completed our initial public offering, giving effect to the conversion of preferred stock at the beginning of the period. MongoDB uses these non-GAAP financial measures internally in analyzing its financial results and believes they are useful to investors, as a supplement to GAAP measures, in evaluating MongoDB’s ongoing operational performance. MongoDB believes that the use of these non-GAAP financial measures provides an additional tool for investors to use in evaluating ongoing operating results and trends and in comparing its financial results with other companies in MongoDB’s industry, many of which present similar non-GAAP financial measures to investors.
Free cash flow represents net cash used in operating activities less capital expenditures and capitalized software development costs, if any. MongoDB uses free cash flow to understand and evaluate its liquidity and to generate future operating plans. The exclusion of capital expenditures and amounts capitalized for software development facilitates comparisons of MongoDB’s liquidity on a period-to-period basis and excludes items that it does not consider to be indicative of its liquidity. MongoDB believes that free cash flow is a measure of liquidity that provides useful information to investors in understanding and evaluating the strength of its liquidity and future ability to generate cash that can be used for strategic opportunities or investing in its business in the same manner as MongoDB’s management and board of directors.
Non-GAAP financial measures have limitations as an analytical tool and should not be considered in isolation from, or as a substitute for, financial information prepared in accordance with GAAP. In particular, other companies may report non-GAAP gross profit, non-GAAP gross margin, non-GAAP loss from operations, non-GAAP net loss, non-GAAP net loss per share, free cash flow or similarly titled measures but calculate them differently, which reduces their usefulness as comparative measures. Investors are encouraged to review the reconciliation of these non-GAAP measures to their most directly comparable GAAP financial measures, as presented below. This earnings press release and any future releases containing such non-GAAP reconciliations can also be found on the Investor Relations page of MongoDB’s website at http://investors.mongodb.com.
Investor Relations
Brian Denyeau
ICR for MongoDB
646-277-1251
ir@mongodb.com
Media Relations
MongoDB
866-237-8815 x7186
communications@mongodb.com
MONGODB, INC. |
|||
CONDENSED CONSOLIDATED BALANCE SHEETS |
|||
(in thousands, except share and per share data) |
|||
(unaudited) |
|||
As of January 31, |
|||
2018 |
2017 |
||
Assets |
|||
Current assets: |
|||
Cash and cash equivalents |
$ 61,902 |
$ 69,305 |
|
Short-term investments |
217,072 |
47,195 |
|
Accounts receivable, net of allowance for doubtful accounts of $1,238 and $958 as of January |
46,872 |
31,340 |
|
Deferred commissions |
11,820 |
7,481 |
|
Prepaid expenses and other current assets |
5,884 |
3,131 |
|
Total current assets |
343,550 |
158,452 |
|
Property and equipment, net |
59,557 |
4,877 |
|
Goodwill |
1,700 |
1,700 |
|
Acquired intangible assets, net |
1,627 |
2,511 |
|
Deferred tax assets |
326 |
114 |
|
Other assets |
8,436 |
6,778 |
|
Total assets |
$ 415,196 |
$ 174,432 |
|
Liabilities, Redeemable Convertible Preferred Stock and Stockholders’ Equity (Deficit) |
|||
Current liabilities: |
|||
Accounts payable |
$ 2,261 |
$ 2,841 |
|
Accrued compensation and benefits |
17,433 |
11,402 |
|
Other accrued liabilities |
8,423 |
5,269 |
|
Deferred revenue |
114,500 |
78,278 |
|
Total current liabilities |
142,617 |
97,790 |
|
Redeemable convertible preferred stock warrant liability |
— |
1,272 |
|
Deferred rent, non-current |
925 |
1,058 |
|
Deferred tax liability, non-current |
18 |
108 |
|
Deferred revenue, non-current |
22,930 |
15,461 |
|
Other liabilities, non-current |
55,213 |
— |
|
Total liabilities |
221,703 |
115,689 |
|
Redeemable convertible preferred stock, par value $0.001 per share; no shares authorized, |
— |
345,257 |
|
Stockholders’ equity (deficit): |
|||
Class A common stock, par value of $0.001 per share; 1,000,000,000 and 162,500,000 shares |
13 |
— |
|
Class B common stock, par value of $0.001 per share; 100,000,000 and 113,000,000 shares |
38 |
13 |
|
Additional paid-in capital |
638,680 |
62,557 |
|
Treasury stock, 99,371 shares as of January 31, 2018 and 2017 |
(1,319) |
(1,319) |
|
Accumulated other comprehensive loss |
(159) |
(364) |
|
Accumulated deficit |
(443,760) |
(347,401) |
|
Total stockholders’ (deficit) equity |
193,493 |
(286,514) |
|
Total liabilities, redeemable convertible preferred stock and stockholders’ equity (deficit) |
$ 415,196 |
$ 174,432 |
MONGODB, INC. |
|||||||
CONDENSED CONSOLIDATED STATEMENTS OF OPERATIONS |
|||||||
(in thousands, except share and per share data) |
|||||||
(unaudited) |
|||||||
Three Months Ended January 31, |
Year Ended January 31, |
||||||
2018 |
2017 |
2018 |
2017 |
||||
Revenue: |
|||||||
Subscription |
$ 41,887 |
$ 27,217 |
$ 141,490 |
$ 91,235 |
|||
Services |
3,154 |
2,717 |
13,029 |
10,123 |
|||
Total revenue |
45,041 |
29,934 |
154,519 |
101,358 |
|||
Cost of revenue(1): |
|||||||
Subscription |
9,097 |
5,696 |
30,766 |
19,352 |
|||
Services |
3,304 |
2,649 |
12,093 |
10,515 |
|||
Total cost of revenue |
12,401 |
8,345 |
42,859 |
29,867 |
|||
Gross profit |
32,640 |
21,589 |
111,660 |
71,491 |
|||
Operating expenses: |
|||||||
Sales and marketing(1) |
32,863 |
22,474 |
109,950 |
78,584 |
|||
Research and development(1) |
16,788 |
13,232 |
62,202 |
51,772 |
|||
General and administrative(1) |
10,242 |
7,166 |
36,775 |
27,082 |
|||
Total operating expenses |
59,893 |
42,872 |
208,927 |
157,438 |
|||
Loss from operations |
(27,253) |
(21,283) |
(97,267) |
(85,947) |
|||
Other income (expense), net |
1,349 |
(71) |
2,195 |
(15) |
|||
Loss before provision for income taxes |
(25,904) |
(21,354) |
(95,072) |
(85,962) |
|||
Provision for income taxes |
470 |
466 |
1,287 |
719 |
|||
Net loss |
$ (26,374) |
$ (21,820) |
$ (96,359) |
$ (86,681) |
|||
Net loss per share attributable to common stockholders, basic and diluted |
$ (0.52) |
$ (1.69) |
$ (4.06) |
$ (7.10) |
|||
Weighted-average shares used to compute net loss per share attributable to common |
50,287,162 |
12,891,905 |
23,718,391 |
12,211,711 |
|||
(1)Includes stock-based compensation expense as follows: |
|||||||
Three Months Ended January 31, |
Year Ended January 31, |
||||||
2018 |
2017 |
2018 |
2017 |
||||
Cost of revenue—subscription |
$ 227 |
$ 145 |
$ 730 |
$ 570 |
|||
Cost of revenue—services |
170 |
85 |
462 |
482 |
|||
Sales and marketing |
1,964 |
1,168 |
6,364 |
5,514 |
|||
Research and development |
1,680 |
1,237 |
5,752 |
5,755 |
|||
General and administrative |
2,128 |
1,852 |
7,927 |
8,683 |
|||
Total stock‑based compensation expense |
$ 6,169 |
$ 4,487 |
$ 21,235 |
$ 21,004 |
MONGODB, INC. |
|||
CONDENSED CONSOLIDATED STATEMENTS OF CASH FLOWS |
|||
(in thousands) |
|||
(unaudited) |
|||
Three Months Ended January 31, |
|||
2018 |
2017 |
||
Cash flows from operating activities |
|||
Net loss |
$ (26,374) |
$ (21,820) |
|
Adjustments to reconcile net loss to net cash used in operating activities: |
|||
Depreciation and amortization |
914 |
970 |
|
Stock-based compensation |
6,169 |
4,487 |
|
Deferred income taxes |
(465) |
(41) |
|
Change in fair value of warrant liability |
— |
106 |
|
Change in operating assets and liabilities: |
|||
Accounts receivable |
(11,248) |
(13,863) |
|
Prepaid expenses and other current assets |
(475) |
985 |
|
Deferred commissions |
(3,328) |
(3,675) |
|
Other long-term assets |
(17) |
(581) |
|
Accounts payable |
(1,058) |
1,568 |
|
Deferred rent |
(48) |
(179) |
|
Accrued liabilities |
5,952 |
1,891 |
|
Deferred revenue |
22,266 |
20,066 |
|
Net cash used in operating activities |
(7,712) |
(10,086) |
|
Cash flows from investing activities |
|||
Purchases of property and equipment |
(421) |
(261) |
|
Proceeds from maturities of marketable securities |
8,000 |
(17) |
|
Purchases of marketable securities |
(179,503) |
— |
|
Net cash (used in) provided by investing activities |
(171,924) |
(278) |
|
Cash flows from financing activities |
|||
Proceeds from exercise of stock options, including early exercised stock options |
166 |
1,033 |
|
Repurchase of early exercised stock options |
(93) |
(26) |
|
Proceeds from issuance of Series F financing, net of issuance cost |
— |
34,942 |
|
Proceeds from the IPO, net of underwriting discounts and commissions |
— |
— |
|
Proceeds from exercise of redeemable convertible preferred stock warrants |
— |
— |
|
Payment of offering costs |
(1,384) |
— |
|
Net cash (used in) provided by financing activities |
(1,311) |
35,949 |
|
Effect of exchange rate changes on cash, cash equivalents, and restricted cash |
109 |
(35) |
|
Net (decrease) increase in cash, cash equivalents, and restricted cash |
(180,838) |
25,550 |
|
Cash, cash equivalents, and restricted cash, beginning of period |
243,265 |
43,862 |
|
Cash, cash equivalents, and restricted cash, end of period |
$ 62,427 |
$ 69,412 |
MONGODB, INC. |
|||||||
RECONCILIATION OF GAAP MEASURES TO NON-GAAP MEASURES |
|||||||
(in thousands, except share and per share data) |
|||||||
(unaudited) |
|||||||
Three Months Ended January 31, |
Year Ended January 31, |
||||||
2018 |
2017 |
2018 |
2017 |
||||
Reconciliation of GAAP gross profit to non-GAAP gross profit: |
|||||||
Gross profit on a GAAP basis |
$ 32,640 |
$ 21,589 |
$ 111,660 |
$ 71,491 |
|||
Gross margin (Gross profit/Total revenue) on a GAAP basis |
72.5 % |
72.1 % |
72.3 % |
70.5 % |
|||
Add back: |
|||||||
Stock-based compensation expense: Cost of Revenue—Subscription |
227 |
145 |
730 |
570 |
|||
Stock-based compensation expense: Cost of Revenue—Services |
170 |
85 |
462 |
482 |
|||
Non-GAAP gross profit |
$ 33,037 |
$ 21,819 |
$ 112,852 |
$ 72,543 |
|||
Non-GAAP gross margin (Non-GAAP gross profit/Total revenue) |
73.3 % |
72.9 % |
73.0 % |
71.6 % |
|||
Reconciliation of GAAP loss from operations to non-GAAP loss from operations: |
|||||||
Loss from operations on a GAAP basis |
$ (27,253) |
$ (21,283) |
$ (97,267) |
$ (85,947) |
|||
Add back: |
|||||||
Stock-based compensation expense |
6,169 |
4,487 |
21,235 |
21,004 |
|||
Non-GAAP loss from operations |
$ (21,084) |
$ (16,796) |
$ (76,032) |
$ (64,943) |
|||
Reconciliation of GAAP net loss to non-GAAP net loss: |
|||||||
Net loss on a GAAP basis |
$ (26,374) |
$ (21,820) |
$ (96,359) |
$ (86,681) |
|||
Add back: |
|||||||
Stock-based compensation expense |
6,169 |
4,487 |
21,235 |
21,004 |
|||
Change in fair value of warrant liability |
— |
106 |
(101) |
(38) |
|||
Non-GAAP net loss |
$ (20,205) |
$ (17,227) |
$ (75,225) |
$ (65,715) |
|||
Reconciliation of GAAP net loss per share attributable to common stockholders, basic and diluted, to |
|||||||
Net loss per share attributable to common stockholders, basic and diluted, on a GAAP basis |
$ (0.52) |
$ (1.69) |
$ (4.06) |
$ (7.10) |
|||
Add back: |
|||||||
Stock-based compensation expense |
0.12 |
0.35 |
0.90 |
1.72 |
|||
Change in fair value of warrant liability |
— |
0.01 |
— |
— |
|||
Impact of additional weighted-average shares giving effect to conversion of preferred |
— |
0.89 |
1.42 |
3.65 |
|||
Non-GAAP net loss per share attributable to common stockholders, basic and diluted |
$ (0.40) |
$ (0.44) |
$ (1.74) |
$ (1.73) |
|||
Reconciliation of GAAP weighted-average shares outstanding, basic and diluted, to non-GAAP weighted- |
|||||||
Weighted-average shares used to compute net loss per share attributable to common |
50,287,162 |
12,891,905 |
23,718,391 |
12,211,711 |
|||
Add back: |
|||||||
Additional weighted-average shares giving effect to conversion of preferred stock at the |
— |
25,864,824 |
19,494,691 |
25,856,309 |
|||
Non-GAAP weighted-average shares used to compute net loss per share, basic and diluted |
50,287,162 |
38,756,729 |
43,213,082 |
38,068,020 |
|||
The following table presents a reconciliation of free cash flow to net cash used in operating activities, the most directly comparable GAAP measure, for each of the periods indicated: |
Three Months Ended January 31, |
Year Ended January 31, |
|||||
2018 |
2017 |
2018 |
2017 |
||||
Net cash used in operating activities |
$ (7,712) |
$ (10,086) |
$ (44,881) |
$ (38,078) |
|||
Capital expenditures |
(421) |
(261) |
(2,135) |
(1,683) |
|||
Capitalized software |
— |
— |
— |
— |
|||
Free cash flow |
$ (8,133) |
$ (10,347) |
$ (47,016) |
$ (39,761) |
View original content with multimedia:http://www.prnewswire.com/news-releases/mongodb-inc-announces-fourth-quarter-and-full-year-fiscal-2018-financial-results-300613447.html
SOURCE MongoDB