Month: September 2018
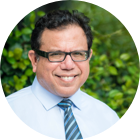
MMS • RSS
Article originally posted on Data Science Central. Visit Data Science Central
AI has snuck into our daily lives and there is nothing to stop it. Not only does AI power autonomous vehicles, but AI already decides what products you should buy, what movies you should watch, what music you should listen to, and whom you should date. If you talk to your iPhone, Google Home, and Amazon Echo, you are talking to an AI engine that powers these personal virtual assistants. AI decides whether you are approved for a loan, determines the outcome of a job applications, identifies threats to national security, and recommends medical treatment. AI is even helping sports teams minimize injuries to their most important assets (see “Leveraging Agent-based Models (ABM) and Digital Twins to Prevent Injuries”).
AI is already everywhere and will soon become an indispensable component in nearly every aspect of your life. Yes, Moneyball is coming to every aspect of your life, Millennials. But now is not the time for Millennials to panic. As I stated in the blog “How State Governments Can Protect and Win with Big Data, AI and Privacy:”
“We can’t operate in a world of fear; jump to conclusions based upon little or no information, or worse yet, misinformation or purposeful lies.”
AI poses either a threat or an opportunity…depending upon your mindset…that will guide your career and life. The article “Millennials, This Is How Artificial Intelligence Will Impact Your Job For Better And Worse” states that:
- AI will create 500,000 more jobs than it will displace over the next three years
- Millennials are the most vulnerable generation to the threats AI poses
So what does AI really mean to our future business and society leaders – the Millennials? And what should they be doing today to not only prepare for a world dominated by AI, but flourish?
AI and Millennials
I asked my daughter, Amelia, a Millennial who is a sophomore at California State in San Marcos, for her impressions on AI and the potential impact AI might have on her blooming career.
What are your first impressions when you hear the phrase Artificial Intelligence or AI?
My first impression when I hear the phrase “AI” is a visual image of technology and brains integrating into one. I first saw it as computers knowing our thoughts and answers before we even know them ourselves. Initially, it caught me off-guard. The thought of having our intelligence converted into technology, is slightly off-putting… yet insanely interesting.
What are your initial impressions from the propositions in the article, that Millennials are the most vulnerable generation to the threats AI poses? How does that statement make you feel?
This statement makes me nervous. The idea that technology could consume the jobs we are training for in college is scary. However, I am excited about the potential to take advantage of AI to compliment my career. I am adopting a “How can I keep up?” mindset; that is, how can I stay relevant in a world of rapid technology innovation.
What do you think Millennials need to do to prepare themselves for a world dominated by AI?
I think Millennials need to prepare themselves for lots of different technologies, not just AI. The most important piece of advice given to me by my brother Max, is to develop the discipline to read something new every day. You have to keep up with the most recent topics and developments. For example, computer science is a huge topic right now. To master and fully comprehend the fundamentals of coding and breaking down those pieces, will help me keep up with the advancements of technology in ways I may not even realize today.
What are you doing to prepare yourself for a world dominated by AI?
I am seeking to understand first-hand from more experienced folks. For example, I recently sat down with a woman who works at Google to discuss her views on AI and how I can prepare myself for such a drastic innovation. As a result of that discussion, I was able to get a solid understanding of what future employers will look for. In addition to that, I have been reading and listening to podcasts that not only my mother’s friend recommended, but also podcasts and books that a co-worker of my father’s suggested. Millennials need to “learn to learn” and constantly seek out new and different perspectives if we want to stay relevant for the future.
Educating the Next Generation of Business and World Leaders
As fortune would have it, the University of San Francisco School of Management Advisory Board had our first meeting this week. We started the meeting with an inspirational video from David Jones, Executive Producer of Envisioning at Microsoft, about the Future of Workin a connected and “intelligent world”.
Our Advisory Board was then asked to contemplate what the university must do to be relevant in 2025. Here’s what we came up with:
Goal: Create fearless, well-rounded students
Create a results-centric, contextual curriculum which includes:
- Outcomes (results) focused, not process-focused, which teaches students how to identify, validate, value and prioritize the solution requirements.
- Logical reasoning – deductive, inductive, and abductive – that trains students how to triage and decompose a problem into smaller, more manageable problem sets.
- Accepting of critical feedback as the foundation for improving. Learn to get feedback from both hard (performance) data as well as soft (behavioral) data.
- Mastering the “Art of Failure” as the foundation for learning. Be willing to try new things, approaches, and techniques in order to come up with ideas that “might” provide better results.
- Critical thinking skills in order to challenge or question the initial ideas and results in order to find better.
These are the basic skills that tomorrow’s leaders – the Millennials – are going to need in order to stay relevant a world of constant technology change that can unleash new work and life opportunities. In fact, these are the basic skills that everyone is going to need to master to stay relevant in a world of constant technology change.
As Amelia said:
I am constantly challenging myself. Continuous learning is the most crucial preparation skill a Millennial can master. The opportunity to learn from the more experienced experts is an underrated opportunity. The more people within the industry I speak to, the more I am preparing myself for the uncertain world of AI.
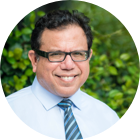
MMS • RSS
Article originally posted on InfoQ. Visit InfoQ
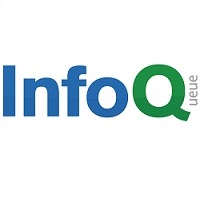
At the recent Event-Driven Microservices Conference in Amsterdam, Russ Miles claimed that the biggest challenge for an architect is that you get ignored. You have great ideas like event-driven microservices, but the reaction too often is that it sounds good, but that it’s overly complicated for the needs at hand. Miles commonly get this reaction when he suggests that companies should consider looking at asynchronous event-driven systems as a way of introducing scaling, redundancy and fault tolerance. The words often make sense to the company, but just as often they get ignored.
The main goal for Miles in his work is having reliable systems. Reliability for him is a measure of what the customer wants; a system that is feature-rich and always running. This means we have two opposing forces which don’t coexist easily, especially notable in complicated systems – continuous innovation and change, versus a system that is always working.
According to Miles, the hardest thing for an architect is to get everyone to understand that you are building resilient systems, and Miles emphasizes that he is not just talking about technology, he is referring to the whole system which includes the people, the practices and the processes that surrounds it. Considering all this, he regards it a minor miracle that systems in production ever work.
Miles refers to John Allspaw for defining resilience. If you build systems with a lot of redundancy, replication, distribution and so on, you may be building robust systems. For Allspaw resilience is when you also involve people. In the same way, chaos engineering is beyond the tools – it’s about how people think and approach a system.
For Miles, chaos engineering is a technique for finding failures before they happen, but also a mindset:
- Never let an outage go to waste. Learn from failures when they happen.
- Have a pre-mortem attitude. You learn from outages but it’s better to explore weaknesses before they occur.
- It’s collaborative; you don’t run experiments against other’s systems. Everyone should know beforehand and agree on what you want to learn.
- Start tiny with one small experiment. If the system survives, then you can choose to increase the scope.
- Start working manually using your brain. After that you can start automating using the tools available.
The single most important thing about chaos engineering for Miles is that you must be part of the team working on the system. You cannot be someone that hurts a system and then wait for others to fix the problem. You must be part of the effect of what you have done and work with everyone else to fix it. Miles has seen companies that have a group of people that hurt systems for a living, but in his experience this doesn’t work.
Miles points out that in his mind, chaos engineering is simple. There are only two main key practices to learn, and he emphasizes that there is no need for any certification program:
- Game days, where you gather all the teams and change some condition in production that you all agreed can happen, and see how you deal with it. He notes that game days can be expensive since they take a lot of the team’s time.
- Automatic chaos experiments are when you automate the experiments to be able to continuously explore and look for weaknesses.
If you are ready to start working with chaos engineering at your company, Miles’ first advice is to not use the term at all. Don’t talk about breaking things; instead talk about incidents that have happened and what you can learn from them and improve. He notes that you are in a learning loop trying to get a system that gradually gets more and more resilient.
In a summary of his points, Miles noted some rules from the “Chaos Club” that you must follow:
- Don’t talk about chaos. The concepts are becoming more mainstream, but the term may still set people off. Start using it when people are more comfortable.
- Learn without breaking things. You are trying to improve across the whole socio-technical system by finding and dealing with the weaknesses before the users.
- Chaos should not be a surprise.
- If you know the system will break, don’t do the experiment. Try to fix the weaknesses you already know about before you try to find new weaknesses.
When working with event-driven microservices based system, one of the hardest things is to get developers to understand how to become a good citizen in production. This includes having the right endpoint exposures to declare your health and the right touchpoints to say if you are OK or not. Good logging is an important aspect and a way to improve on this is to have developers read their own logs, for example during a game day when they must understand what the system did through their own logs.
When doing chaos engineering, one advantage with event-sourced systems is the observability it brings. For Miles, observability means the ability to debug the system in production without changing it. If you are doing some form of chaos experiment, the first thing you want to do is debugging the system to figure out what went wrong, and with an event-sourced system you have a system of record, you know exactly what happened and when.
Miles concluded by stating that for the first time in his career, there is a best practice. For the complex and maybe chaotic systems we build today, chaos engineering is a technique for which he wants to say “just do it”. Do a small amount of it, manually, as a game day or whatever works for you. If you care about the reliability or resilience of your systems, he believes it’s a tool for you.
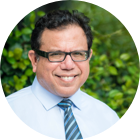
MMS • RSS
Article originally posted on Data Science Central. Visit Data Science Central
Monday newsletter published by Data Science Central. Previous editions can be found here. The contribution flagged with a + is our selection for the picture of the week.
Featured Resources and Technical Contributions
- Free Book: The Definitive Guide to Pandas
- K-Nearest Neighbors (KNN): Solving Classification Problems
- Sequence Modeling with Neural Networks – Part I
- R, Python, Julia – and Polyglot
- Convolutional Neural Network?(CNN) From Scratch
- Automated Machine Learning Hyperparameter Tuning in Python
- Who cares if unsupervised ML is supervised learning in disguise?
- More Free eBooks from Packt
- Setting up your first Kafka development environment in Google Cloud
- K Means Clustering Algorithm & its Application
- Popularity of software programs for data science using recent reviews
- Question: The Future of Data Science
Featured Articles
- Full Stack Data Scientist: The Elusive Unicorn and Data Hacker
- MBA vs. Data Science qualifications – Does AI and DataScience explain the fall in MBA applications?
- What’s New in Data Prep
- Can Artificial Intelligence replace Data Scientists?
- Data Engineers: Nobody Puts Baby in a Corner! +
- Using Confusion Matrices to Quantify the Cost of Being Wrong
- My Learning Sabbatical
- How to pay your data scientists to increase retention
- Difference Between Data Science and Web Development
- Using Blockchain Technology to Secure the Internet of Things (IoT)
- 7 Fundamental Tips for Building a Successful AI
- North America Behavior Analytics Market to cross $2bn by 2024
- The Importance of Data Security in Healthcare Communications
- Minimizing Errors & Authenticating Transactions with Blockchain
Picture of the Week
Source for picture: contribution marked with a +
To make sure you keep getting these emails, please add mail@newsletter.datasciencecentral.com to your address book or whitelist us.
Hire a Data Scientist | Search DSC | Classifieds | Find a Job | Post a Blog | Ask a Question
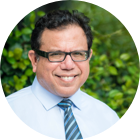
MMS • RSS
Article originally posted on Data Science Central. Visit Data Science Central
Pandas is a Python package providing fast, flexible, and expressive data structures designed to make working with “relational” or “labeled” data both easy and intuitive. It aims to be the fundamental high-level building block for doing practical, real world data analysis in Python. Additionally, it has the broader goal of becoming the most powerful and flexible open source data analysis / manipulation tool available in any language. It is already well on its way toward this goal.
pandas is well suited for many different kinds of data:
- Tabular data with heterogeneously-typed columns, as in an SQL table or Excel spreadsheet
- Ordered and unordered (not necessarily fixed-frequency) time series data.
- Arbitrary matrix data (homogeneously typed or heterogeneous) with row and column labels
- Any other form of observational / statistical data sets. The data actually need not be labeled at all to be placed into a pandas data structure
The two primary data structures of pandas, Series(1-dimensional) and DataFrame (2-dimensional), handle the vast majority of typical use cases in finance, statistics, social science, and many areas of engineering. For R users, DataFrame provides everything that R’s data.frame
provides and much more. pandas is built on top of NumPy and is intended to integrate well within a scientific computing environment with many other 3rd party libraries.
Here are just a few of the things that pandas does well:
- Easy handling of missing data (represented as NaN) in floating point as well as non-floating point data
- Size mutability: columns can be inserted and deleted from DataFrame and higher dimensional objects
- Automatic and explicit data alignment: objects can be explicitly aligned to a set of labels, or the user can simply ignore the labels and let Series, DataFrame, etc. automatically align the data for you in computations
- Powerful, flexible group by functionality to perform split-apply-combine operations on data sets, for both aggregating and transforming data
- Make it easy to convert ragged, differently-indexed data in other Python and NumPy data structures into DataFrame objects
- Intelligent label-based slicing, fancy indexing, and subsetting of large data sets
- Intuitive merging and joining data sets
- Flexible reshaping and pivoting of data sets
- Hierarchical labeling of axes (possible to have multiple labels per tick)
- Robust IO tools for loading data from flat files (CSV and delimited), Excel files, databases, and saving / loading data from the ultrafast HDF5 format
- Time series-specific functionality: date range generation and frequency conversion, moving window statistics, moving window linear regressions, date shifting and lagging, etc.
Many of these principles are here to address the shortcomings frequently experienced using other languages / scientific research environments. For data scientists, working with data is typically divided into multiple stages: munging and cleaning data, analyzing / modeling it, then organizing the results of the analysis into a form suitable for plotting or tabular display. pandas is the ideal tool for all of these tasks.
Some other notes
- pandas is fast. Many of the low-level algorithmic bits have been extensively tweaked in Cython code. However, as with anything else generalization usually sacrifices performance. So if you focus on one feature for your application you may be able to create a faster specialized tool.
- pandas is a dependency of statsmodels, making it an important part of the statistical computing ecosystem in Python.
- pandas has been used extensively in production in financial applications.
Content of the Guide
What’s New
Installation
Contributing to pandas
Package overview
10 Minutes to pandas
Tutorials
Cookbook
Intro to Data Structures
Essential Basic Functionality
Working with Text Data
Options and Settings
Indexing and Selecting Data
MultiIndex / Advanced Indexing
Computational tools
Working with missing data
Group By: split-apply-combine
Merge, join, and concatenate
Reshaping and Pivot Tables
Time Series / Date functionality
Time Deltas
Categorical Data
Visualization
Styling
IO Tools (Text, CSV, HDF5, …)
Enhancing Performance
Sparse data structures
Frequently Asked Questions (FAQ)
rpy2 / R interface
pandas Ecosystem
Comparison with R / R libraries
Comparison with SQL
Comparison with SAS
Comparison with Stata
API Reference
Developer
Internals
Extending Pandas
Release Notes
Download the guide, or read it online, here.
DSC Resources
- Invitation to Join Data Science Central
- Free Book: Applied Stochastic Processes
- Comprehensive Repository of Data Science and ML Resources
- Advanced Machine Learning with Basic Excel
- Difference between ML, Data Science, AI, Deep Learning, and Statistics
- Selected Business Analytics, Data Science and ML articles
- Hire a Data Scientist | Search DSC | Classifieds | Find a Job
- Post a Blog | Forum Questions
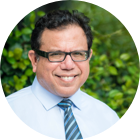
MMS • RSS
Article originally posted on InfoQ. Visit InfoQ
Key Takeaways
- Autonomy has to be learned and earned.
- Autonomy doesn’t work without a corresponding accountability model.
- Structure organizations with a goal to simplify alignment & prioritization.
- Optimize organizations around end-to-end ownership and a single customer group.
- Leader autonomy is as essential as team autonomy.
Autonomy isn’t something you can just give to a team, it’s something that teams learn and earn over time. It has to come with accountability to amplify working towards a purpose. At Zalando, creating the right architecture and organizational structure reduced the amount of alignment needed and freed up the energy to be more thorough where alignment is needed.
Eric Bowman, VP Digital Foundation at Zalando SE, gave a talk titled “Organized Autonomy: Cracking the Paradox” at Agile Summit Greece 2018. InfoQ is covering this event with summaries, articles, and Q&As.
InfoQ interviewed Bowman about how they scaled autonomy at Zalando.
InfoQ: What makes team autonomy so important?
Eric Bowman: Autonomy is essential for getting the best out of great people. Dan Pink is right that autonomy is a crucial ingredient for sustained intrinsic motivation. When we can create a context where autonomy works, it is a powerful force for engagement and creativity. Autonomy is also essential for scaling a large organization that continues to innovate. Centralized decision-making doesn’t work beyond a particular scale, and so for a modern company to continue to grow, it has to figure out how to distribute decision-making to get stronger over time through the right feedback loops. We want autonomous teams that can move in parallel since that is the most efficient and powerful application of all the resources we have to bear. Once parallelism is unlocked with the right feedback loops in place, an organization can move fast and adapt quickly and feels like the most enjoyably efficient way to provide massive value to both customers and the business.
In the InfoQ article Don’t Copy the Spotify Model, Marcin Floryan mentioned that “Autonomy is futile without alignment”. Achieving autonomy is very hard, there are lots of challenges. He gave examples of things Spotify does, like giving trust to people, providing them the right information, and giving purpose: “You have to have a purpose in your autonomy to make people do the right thing”.
InfoQ: What challenges did you face when working to create a culture that provides autonomy to teams?
Bowman: Our biggest challenge was that we treated autonomy like an input to teams: we needed their help to move fast, so overnight we increased the autonomy of all our software teams, and we expected good things to happen as a result. What we learned is that autonomy is more like an output of the team — it is something that teams learn and earn over time. We see autonomy as an aspiration and a reward, not a gift, which is a change from where we started in 2015. I agree with Marcin’s point — the analogy I use is that unaligned teams are like the air molecules in a balloon: moving fast but not running together as a group. Where I disagree is around alignment — obviously, autonomous teams and leaders have to align, but solving the alignment problem for us was more like a side-effect of solving what we see as the core challenge of enabling and scaling autonomous teams. The solution, for us, is an effective accountability mechanism to amplify our work toward a purpose, not just purpose alone.
Kent Beck tweeted in April 2017, “Autonomy without accountability is just vacation.” This tweet resonated because it reflected what we saw as the core learning from our initial approach to agility (which we called Radical Agility): that accountability is like the mathematical dual, or the other side of the coin, of autonomy. Almost like good vs. evil, each requires the other to have meaning. By not implementing an accountability model as the currency for earning autonomy and trust, we denied teams the chance to learn how to become more autonomous. We didn’t fully realize the opportunity to take innovation to the next level by unlocking all these amazingly smart people we hired to work toward a common purpose.
This is a departure from Dan Pink and Drive, where he wrote, “Motivation 3.0 begins with a different assumption. It presumes that people want to be accountable — and that making sure they have control over their task, their time, their technique, and their team is the most effective pathway to that destination.” We don’t agree. Our job as leaders is to make accountability something to which people aspire. Accountability has different implementations, some empowering and some horrible. We strive to implement a humanist form of accountability consistent with our values and a culture we wish to work in every day.
Accountability has somehow a lousy name in agile. People tend to associate it with counting coins. However, for us, accountability is more like, “to give an account of.” When we talk about accountability, we mean the following: energy, transparency, and commitment around a set of goals, their achievability, the plan to get there, and progress to date. Being accountable requires an openness to being evaluated on whether you achieved your goals. When you have all these components in place, alignment is a natural byproduct: you can’t state ambitious goals you honestly expect to complete, and report progress against them, without having aligned. Finally, you need experienced tech leaders who understand that software delivery is hard and rife with essential ambiguity, that you uncover many unknowns along the way, and that there’s a reason for agile methodologies, even if they are not the whole solution.
InfoQ: How do teams align at Zalando?
Bowman: We started using OKRs in 2015, and we introduced a couple of conventions around the OKR process to help alignment. First, we considered a 0.3 scoring as a form of commitment. Each team committed implicitly to complete the work to achieve a 0.3 score. Second, we held company-wide OKR alignment weeks as part of the OKR planning phase. And it went ok — something is a lot better than nothing.
As we’ve continued to scale, we’ve learned that it’s vital to structure (and restructure) an organization (of nearly any size) in a way that optimizes for end-to-end ownership of customer experiences and business outcomes, and to service the needs of a single customer group. We found teams with lots of different customer groups end up with impossibly hard alignment and prioritization challenges, unable to benefit from this profound simplification heuristic: there are many things we coulddo, but why don’t we start with what our customers want?
A simple case could be a team building software for, say, both our fashion customers and our fashion buyers. We see this as an anti-pattern — better to have two teams, in this case; one for each group, and two leaders as well. Probably they belong in entirely different parts of our organization, even if long ago these systems were created by a single team intent on creating an end-to-end solution.
The leverage achievable through using organizational structures to simplify and optimize alignment and prioritization ultimately drove us away from a single, monolithic Tech organization — it had too many customers for us to prioritize and align well. Instead, we embedded engineering teams across the company to form self-contained units capable of solving customer problems end-to-end. One symptom of an organization with too many customers to efficiently align and prioritize is an overly-internal focus as it tries to diagnose what’s going wrong, which further exacerbates the problem.
Applying these constraints at scale requires an accommodating architecture. We have an architecture for a microservice approach to front-end development — the open source Mosaic project. Mosaic enabled us to break apart a monolithic front-end and distribute it through the business to allow more end-to-end ownership for different parts of our customer-facing businesses. This architecture and the organizational structure it enables leads to teams with fewer business-related points of alignment; instead, the alignment happens more locally, and at a lower frequency around technical contracts. By reducing the amount of alignment needed, we freed up the energy to be more thorough where alignment isneeded. Many companies underestimate the extent to which some pernicious combination of Conway’s Law and legacy prevents them from building true end-to-end ownership into the organization. Not being able to do so increases complexity almost exponentially which massively slows down product development. The right architecture is essential if you want to see these principles work in a large organization.
We’ve also improved the mechanisms we use to prioritize at a company level, which helps reduce the amount of overall alignment needed by injecting prioritization rules simultaneously across the company. We do this by layering a prioritization for company-wide initiatives on top of the OKR process. This gives most teams the tools and context to make good decisions on behalf of the company, autonomously. For example, we used this mechanism to implement GDPR: we had one team do a lot of work to figure out what had to be done by all our teams, and once that work was complete, we activated a company-wide Global Project to get it done, requiring the attention of many teams for a short amount of time. This approach drove us toward an efficient plan: the standards to become a Global Project meant that a lot of legwork was completed early to understand the scope of the work, and once the project phase kicked in, it was crystal clear to everyone across the company that GDPR took priority over everything except customer-facing operational issues.
Finally, we found that a set of principles and systems scales better than a set of prescriptions. While it is important to have practices like, “this is how we align”, our best practices necessarily change over time. Principles and systems have more staying power by allowing for evolving practices, and even encouraging them. Principles lead to higher-order mechanisms and feedback loops that lead to rapid “eventual alignment.” Alignment becomes an indirect consequence of the principle-driven decisions by leaders and teams striving to earn autonomy. An accountability model and growth mindset around “the account” and its evaluation can be structured into mechanisms, feedback loops, and especially review rituals that help unlock the value trapped in a growing organization.
InfoQ: What did you do to keep the strengths of a startup when the organization started to grow?
Bowman: I think we’ve kept the strengths of a startup through the uncompromising mindset of the founders and early employees, captured in what we call our founding mindset: customer focus, entrepreneurialism, speed, external focus, awareness of opportunities, learning fast, playing offense, acting decisively despite risks, simplifying for speed and focus, and making it happen. It sounds like a lot, but these ideas weave together into how our business thinks and acts, and is a core strength. Years ago, the idea that we could build our warehouses and the technology to operate them — and make it all profitable — was almost inconceivably bold. But we succeeded precisely due to this mindset. More recently, we’ve rolled out new countries (Ireland and the Czech Republic), a new category (Beauty), and entered whole new businesses that leverage our platform (e.g., Zalando Fulfillment Solutions) by fostering and growing this founding mindset in our people. And we’ve had tremendous success hiring great people across the company, who bring more strengths and diversity of approaches into the mix, and even as we reach ten years as a company, it still feels young and endlessly creative.
InfoQ: What’s your advice for leading autonomous teams?
Bowman: The most solid advice I have for leading autonomous teams is that autonomy is an aspiration, not an input. One can’t just give autonomy to a team and expect great things magically to happen without remarkable fortune and a remarkable team. Rather, leaders must help teams learn and earn trust by incorporating a simple accountability model and a gentle assessment feedback loop to establish a currency for acquiring autonomy.
When it works, it provides the mechanism for a team to get better continuously and to start playing from strength to strength through a sequence of accounts: the story of the team over time. For every setback or misstep, the leader has to help the team see it as an opportunity to learn and improve, help them seize that opportunity, and then help everyone learn from it. She needs to be comfortable being accountable for the output of her teams and to be a role model for accountability. As the team learns and earns autonomy, so too must the leader, and doing so at scale is a magic ingredient for how to grow a large organization that continues to deliver and innovate at a growing pace. Leaders play a foundational role in reducing complexity as connectors and simplifiers, and as a consequence of there being fewer of them. Alignment and communication, when done with skill and care, is more natural, more powerful, and more human than any leaderless self-organizing system. Don’t believe the hype!
Finally, what we see is a tendency in struggling tech organizations toward overly internal focus that must be deliberately avoided. This phenomenon can manifest as too much emphasis on tools and methodology, or culture, or organizational philosophy. Our founding mindset has been useful to keep us focused on our customers’ problems and how we can solve them. There is no known — let alone perfect — solution to the hardest challenges of a growing business. They still require energy, flexibility, creativity, and good situational instincts to solve, plus a little luck — precisely the core of what it means to be agile.
About the Interviewee
Eric Bowman is VP Digital Foundation at Zalando, Europe’s leading online fashion platform. Bowman leads a team of 500 focused on leveraging Zalando’s scale to help every part of the company’s digital business to innovate faster for less and disrupt how the world connects to fashion. As Zalando’s first VP Engineering, he led the engineering team through the architectural transformation to microservices, cloud, and SaaS, and was one of the founders of Radical Agility, Zalando’s unique tech culture. A 20-year industry veteran, Bowman has been a technical leader at multiple startups as well as global companies including Gilt Groupe, TomTom, Three, Electronic Arts, and Maxis, where a lifetime ago he was one of the original three amigos who built The Sims 1.0.
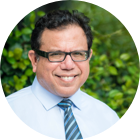
MMS • RSS
Article originally posted on InfoQ. Visit InfoQ
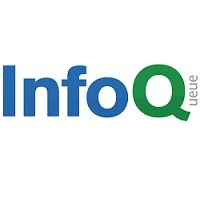
Facebook open sourced their internal distributed log storage project called LogDevice. It offers high write availability using replication, durable log storage and recovery from failure.
Most of Facebook’s applications that perform logging require high write availability, durable storage of logs, and workloads that vary in terms of performance and latency requirements. Another important requirement was to be able to survive hardware failures. An older Facebook project called Scribe was more focused on aggregating logs to central storage, and there were cases where data loss could occur. Scribe now uses LogDevice as a log storage backend.
Facebook uses LogDevice internally in its datacenters for stream processing pipelines, distribution of database index updates, machine learning pipelines, replication pipelines, and durable task queues where it ingests over 1TB/sec of data. Although Facebook has built a lot of open source tools to manage LogDevice clusters, they are yet to open source any of those except a basic toolset at this point. The LDShell tool allows cluster management from the command line, and the complementary LDQuery command can be used to view cluster statistics.
LogDevice uses the abstraction of a “log record” to demarcate individual log events, with each record assigned a unique id called a Log Sequence Number (LSN). The LSNs are generated based on an epoch number by a component called “Sequencer”, and the epoch numbers are stored in ZooKeeper. The log store is append only, i.e., once written a record cannot be modified. Like most log storage systems, LogDevice performs “trimming” – log rotation based on a time or a space based policy. It can also trim logs on demand. Apart from this, there are no restrictions on how long logs can be stored.
LogDevice achieves high availability, especially write availability, by storing multiple copies of each log record on different machines. Each record can be replicated across 20-30 storage nodes. However, a spike in the number of writes to a single log would limit the throughput if some of the machines that have copies of that log are slow or unavailable. LogDevice can automatically detect which nodes are down and exclude them from writes for new records. It attempts to minimize the impact of hardware failures as much as possible by replication, and by “rebuilding” the lost copies as fast as possible. During such a rebuilding, restoration can be done for “the replication factor of all records affected by the failure at a rate of 5-10GB per second.” The underlying storage is based on RocksDB, a key value store also open sourced by Facebook.
LogDevice’s team also had to deal with challenges in which they discovered that users of LogDevice would perform backfills, where older data hours or days old would be requested. This was triggered by downstream services that consume logs from LogDevice, and backfilling would happen when these services recovered from failures and had to reprocess the logs. These read spikes are handled by spreading the read load across members of a “node set”, which is the set of nodes that will store a given record.
LogDevice has been compared with other log storage systems like Apache BookKeeper and Apache Kafka. The primary difference with Kafka seems to be the decoupling of computation and storage that LogDevice does to be able to handle Facebook’s scale. LogDevice is written in C++ and hosted on GitHub.
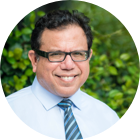
MMS • RSS
Article originally posted on InfoQ. Visit InfoQ
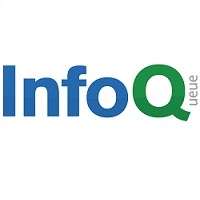
In a recent Hyperledger blog post, Daniel Hardman talks about Hyperledger Indy and its ‘Privacy by Design’ approach to address decentralized identity management. Unlike many systems that add privacy to their product or service after the fact, Hyperledger Indy has been built using a privacy first approach. As the world shifts to more regulation, including GDPR and ePrivacy requirements, Indy can minimize the amount of details a user shares when having their data validated by a third-party system.
Centralized identity providers, such as social media sites and consumer email services, provide convenience to users by offering the ability to sign into other online services using the same identity. However, this approach has recently received a lot of scrutiny as a result of privacy concerns and security breaches. Business Insider recently published a report citing a Facebook breach enabled attackers to use compromised Facebook credentials to access other services like Tinder, Spotify and Airbnb.
To avoid depending upon centralized identity providers, Hyperledger Indy, an open source blockchain project, is being built to address the current issues that exist in centralized identity providers including:
- A lack of transparency in how data is being used.
- Preventing the ‘over-sharing’ of data by providing only the minimal data attributes to a service provider.
- A single point of breach may have cascading consequences. If a user’s credentials have been used to sign up for other online services, those services can also be accessed using these credentials.
Avoiding data leakage is a key scenario that Hyperledger is trying to address. Hardman explains how they are able to do this:
Hyperledger Indy allows you to construct interactions where the degree of disclosure is explicit and minimal–much smaller than what was previously possible. Nothing about the mechanics of connecting, talking, or proving in Indy is leaky with respect to privacy; vulnerabilities that emerge must come from the broader context. No other technology takes this minimization as far as Indy does, and no other technology separates interactions from one another as carefully. If privacy problems are like a biohazard, Indy is the world’s most vocal champion of wearing gloves and using a sharps container for needles–and it provides the world’s best latex and disinfectants.
As an alternative solution to centralized identity providers, and relying upon them to guard your data sufficiently, Indy uses Decentralized Identities (DIDs). DIDs are under the control of the user that owns it and is independent from a centralized provider or authority. By using DIDs, Self-Sovereign Identity (SSI) solutions can be developed so that a person or business can store their identity and provide relevant data to service providers who can validate it using claims at the time of using the service.
DIDs are pairwise unique and pseudonymous by default to prevent correlation. Phillip J. Windley, chair of Sovrin Foundation, explains why this is important:
Indy is all about giving identity owners independent control of their personal data and relationships. Indy is built so that the owner of the identity is structurally part of transactions made about that identity. Pairwise identifiers not only prevent correlation, but they stop third parties from transacting without the identity owner taking part since the identity owner is the only place pairwise identifiers can be correlated.
While DIDs seems like a step in the right direction for end-user privacy, there are ways in which organizations may try to defeat the protection that Indy is providing. Hardman explains:
If we use pairwise DIDs and zero-knowledge proofs, the message is clearly “don’t try to correlate me”, even if you could find a way to do it if you try hard enough. An HTTP Do-Not-Track header says “do not track me”, but it doesn’t offer any actual protection from tracking. The VRM community has been talking about user-defined terms for a long time. In a relationship, you can express “don’t use my data for advertising” or “delete my data after 14 days” or “use my data for research, but not commercially”.
Hardman feels that expressing these intentions in code and architecture does have value by itself and is optimistic about its effectiveness moving forward:
Over time, we expect that through regulation, trust frameworks, reputation, and similar mechanisms, not honoring such intentions will be discouraged. Of course we must always communicate clearly the limits of intentions and guarantees, lest we create a false sense of security that can lead to severe consequences.
Hyperledger does provide incentives for transforming privacy. Currently, storing Personal Identifiable Information (PII) creates risk for both consumers and corporations. However, by storing an opaque identifier for a customer and then making requests to a customer’s agent to obtain more information on-demand and discard after its use is a step in the right direction for consumers and de-risks broadscale data breaches.
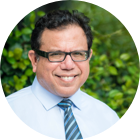
MMS • RSS
Article originally posted on InfoQ. Visit InfoQ
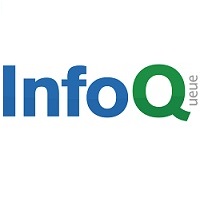
The Next.js team have announced version 7 of their open-source React framework. This release of Next.js focuses on improving the overall developer experience with 57% faster boot times and 40% faster builds in development, improved error reporting and WebAssembly support.
Next.js is a React framework whose primary goal is to provide great performance in production along with a great developer experience. In order to provide this great developer experience, Next.js supports server-side rendering, code splitting and client-side routing out the box.
Next.js is built on many industry standard tools within the JavaScript ecosystem such as Babel and Webpack and with version 7 comes the latest versions of these tools. These upgrades along with a new incremental compilation cache means that Next.js compilations are now 40% faster, a basic application will see compilation reduce from 304ms to 178ms.
With the Webpack upgrade Next.js 7 benefits from the new .mjs support allowing for all the common JavaScript modules such as CommonJS, AMD and ESM to be bundled but also support for EcmaScript, JSON and WebAssembly modules too.
Next.js 7 also bundles the latest version of Babel which brings support for TypeScript, fragment syntax, and experimental auto-polyfilling.
Initial payload sizes in Next.js 7 have been decreased by as much as 7.4%, taking a document size in previous versions of Next.js from 1.62kB to 1.50kB. These improvements have come from the Next.js team removing certain HTML elements and minifying some inline scripts.
Another major improvement with Next.js 7 is its support for the React Context API. The Context API is a way to share data across React components without having to explicitly share it every time. In Next.js this reduces memory usage by 16% due to Next.js’s ability to share code between pages.
Next.js 7 supports dynamic importing of modules; previously this was not possible due to Next.js rolling its own import functionality. They’ve now removed that and support default import functionality that comes out the box with Webpack, allowing dynamic imports, naming and bundling of files.
Next.js has received lots of praise within the community. reacttricks on Reddit says “I’ve been using Next.js exclusively for all projects in the last year and a half, I’d recommend everyone to try it.”. Other feedback includes confusion on what Next.js is; theineffablebob asks “Next just a framework that includes everything you need to get a site up and running? Is it sorta like one of those boilerplates?” Nextjs.org considers itself the PHP of the JavaScript and React world in this quote from nextjs.org: “Think about how webapps are created with PHP. You create some files, write PHP code, then simply deploy it. We don’t have to worry about routing much, and the app is rendered on the server by default.”.
You can get the latest version of Next.js from the Next.js website.