Month: January 2021
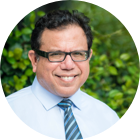
MMS • RSS
Article originally posted on Data Science Central. Visit Data Science Central
5G is critical to many business applications.
Below is a great free opensource book to get started with 5G.
But first, here are five reasons why you should study 5G
Private 5g
If you want to deploy broadband within the Enterprise – there are two main options: rely on WiFi or work with the public telecom networks.
These choices exclude many applications which need seamless, business critical connectivity and are deployed within a specific locality – for example factories, warehouses, schools, airports, hospitals, buildings and conference venues.
For such applications, we need both simplicity of WiFi networks (which do not scale up) and the reliability of public telecoms networks(which do not scale down).
Private 5G offers a new option by providing a network that provides the reliability and coverage of public cellular with the simplicity and affordability of WiFi
Private 5G is a component of 5G deployments that can deliver low latency and high bandwidth connectivity within enterprise campus. Because the network is effectively managed by the enterprise, the data is secure and private.
IoT local area and wide area networks
5G is the missing link for IoT and Edge applications. According to Frost & Sullivan, edge computing in wireless networks would grow from a $64.1 million business in 2019 to a $7.23 billion business in 2024. 90% of industrial enterprises will use edge computing by 2022. This trend can only be accelerated by the pandemic especially post lockdown.
Telemedicine – remote medicine
5G networks enable many telemedicine applications especially those that need video or high bandwidth. These include transferring large files, remote consultations through video, Augmented reality and virtual reality
5G and the Cloud
The Cloud enables the allocation of resources dynamically as per the needs of applications. Cloud native computing is a software development approach that utilizes cloud computing to create and deploy scalable applications. Cloud native applications are enable the creation of loosely coupled systems that are resilient. Telecoms Operators are deploying their own cloud platforms and at the same time, they are also partnering with cloud providers like AWS, Azure, GCP and IBM to provide on demand infrastructure resources such as compute, network and storage.
5G and the Future of work
5G will play a key part in the future of work post pandemic and will enable behaviours like remote work, online education and entertainment.
The book is 5G Mobile networks: A systems approach co-authored by Larry Peterson – Princeton University Computer Science professor
TABLE OF CONTENTS
Chapter 1: Introduction
1.1 Standardization Landscape
1.2 Access Networks
1.3 Edge Cloud
Chapter 2: Radio Transmission
2.1 Coding and Modulation
2.2 Scheduler
2.3 New Radio (NR)
Chapter 3: Basic Architecture
3.1 Main Components
3.2 Radio Access Network
3.3 Mobile Core
3.4 Security and Mobility
3.5 Deployment Options
Chapter 4: RAN Internals
4.1 Packet Processing Pipeline
4.2 Split RAN
4.3 Software-Defined RAN
4.4 Architect to Evolve
Chapter 5: Advanced Capabilities
5.1 Optimized Data Plane
5.2 Multi-Cloud
5.3 Network Slicing
Chapter 6: Exemplar Implementation
6.1 Framework
6.2 Platform Components
Chapter 7: Cloudification of Access
7.1 Multi-Cloud
7.2 EdgeCloud-as-a-Service
7.3 Research Opportunities
Image source 5G Mobile networks: A systems approach
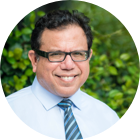
MMS • Uday Tatiraju
Article originally posted on InfoQ. Visit InfoQ
GraalVM has released major version 21.0 with a new component, Java on Truffle, that provides a Java Virtual Machine (JVM) implementation written in Java. GraalVM is itself a polyglot virtual machine that provides a shared runtime to execute applications written in multiple languages like Java, Python, and JavaScript.
Prior to this release, running Java applications in GraalVM was possible by using the Java HotSpot VM with the GraalVM Just-In-Time (JIT) compiler, or by compiling to a native executable using GraalVM Native Image. With this release, Java on Truffle, a JVM written in Java using the Truffle framework, provides an additional option to run Java applications.
Java on Truffle, codenamed Espresso, can be installed using the GraalVM Updater, gu
. gu
is a package manager that downloads and installs packages not included in the core distribution of GraalVM.
gu install espresso
To run a Java application using Java on Truffle, the -truffle
option should be passed to the java command.
java -truffle -jar awesomeApp.jar
Java on Truffle is a minified JVM that provides all the core components of a JVM like the bytecode interpreter, the Java Native Interface (JNI), and the Java Debug Wire Protocol (JDWP). It implements the Java Runtime Environment library (libjvm.so) APIs and reuses all the required JARs and native libraries from GraalVM. And just like with a traditional JVM, Java on Truffle supports running other JVM based languages like Kotlin.
Since it is implemented in Java and is able to run Java, Java on Truffle is essentially self-hosted Java or Java on Java. This opens up interesting opportunities to research and innovate the JVM as it allows Java developers to read and understand the JVM source code and improve it.
Here is a list of noteworthy features and benefits provided by Java on Truffle:
- Leverage the enhanced HotSwap capabilities to change methods, lambdas, and access modifiers of classes at runtime during a debugging session.
- Ability to run a version of Java bytecode that is different from the host JVM. For example, a Java 8 only library can be invoked from within a Java 11 application via the Polyglot API.
- Isolate the host JVM and the Java application running on Truffle to sandbox and run less trusted guest code. In this context terms host and guest are used to differentiate the different layers where Java is executed. Java on Truffle refers to the guest layer.
- Allow Java applications to directly interoperate with non JVM languages like JavaScript and Python, and pass data back and forth in the same memory space
- Leverage the standard tools provided by the Truffle framework. For example, the CPU sampler profiling tool can be used to see which part of a Java application takes more CPU time.
- Build an Ahead-Of-Time (AOT) compiled Java native image and dynamically load Java code to run and use Espresso’s JIT compiler. For example, a CI/CD application like Jenkins can be built as a native image while arbitrary plugins can be dynamically loaded.
A collection of demo applications have been provided by GraalVM to showcase these features and benefits.
Note that Java on Truffle is an experimental component and is not ready for production use. And while it is expected that the warmup and peak performance of Java on Truffle will be improved in future releases, the peak performance in this new release of GraalVM is several times slower compared to the regular JVM. Here is a list of noteworthy Java on Truffle limitations that may be addressed in future releases of GraalVM:
- Does not implement the JVM Tool Interface (JVMTI). The corresponding VM options
-agentlib
and-agentpath
are not supported. - Does not implement the
java.lang.instrument
interface. The corresponding VM option-javaagent
is not supported. - Java Management Extensions (JMX) has been partially implemented. Some methods may return partial data.
In addition to Java on Truffle, this release comes with other enhancements. For example, the use of Java serialization in native image binaries is now allowed. The serialization usage configuration can be auto generated with the javaagent
prior to building the native image.
This release of GraalVM also comes with some compatibility and runtime improvements across its Ruby, Python, and LLVM distributions. GraalVM’s implementation of the WebAssembly language, GraalWasm, has also been optimized to improve warmup time and overall peak performance of interpreting WebAssembly.
The Java versions in this release of GraalVM community edition are updated and based on OpenJDK 1.8.0_282 and OpenJDK version 11.0.10. The Node.js distribution of GraalVM has been updated to 12.20.1.
On the tools front, GraalVM 21.0 comes with an improved gu
tool that allows easy version updates. A GraalVM Extension Pack for Visual Studio Code has also been released to assist in the development and debugging of GraalVM based applications.
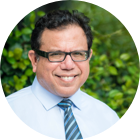
MMS • Aditya Kulkarni
Article originally posted on InfoQ. Visit InfoQ
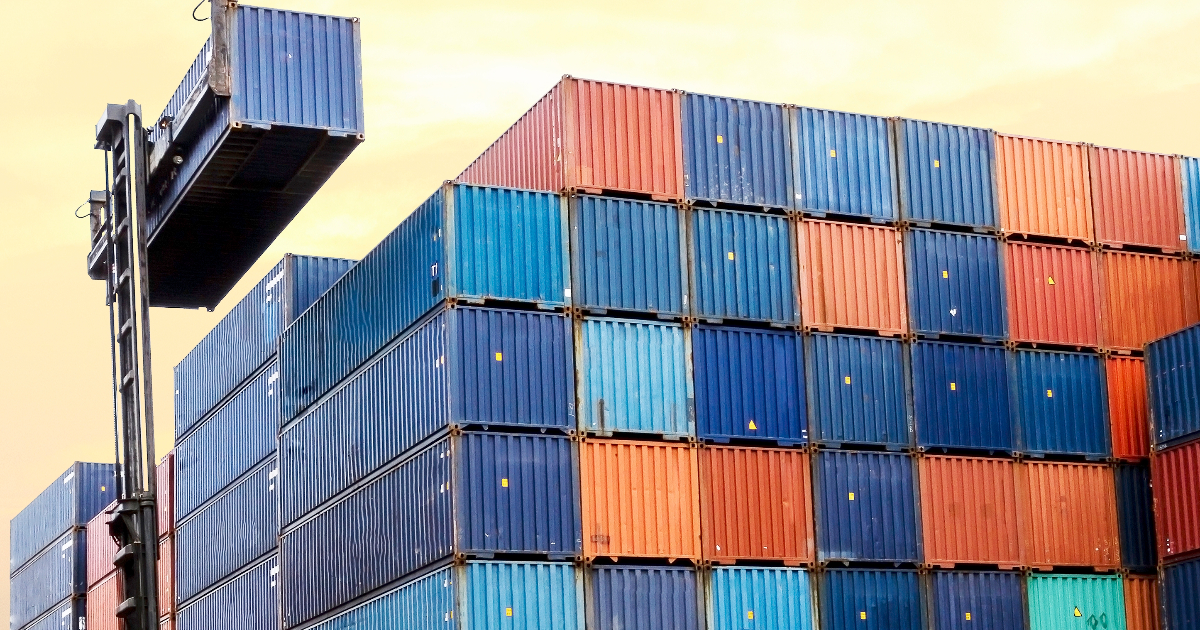
Docker announced the next release of Docker Engine 20.10, adding support for cgroups v2 with improvements in the command line interface (CLI) and support for dual logging. This is the first major release after Docker Engine 19.03, released on 22nd July 2019.
Benjamin De St Paer-Gotch, Principal Product Manager at Docker Inc, provided details of this release in a blog post. Docker Engine comprises a client-server application, with a server dockerd
, APIs specifying interfaces to be used by programs, and a CLI client, docker
.
Building on the foundational Linux Kernel, Docker Engine has introduced cgroups v2
to isolate the running processes and files associated with them. Docker uses namespaces
with cgroups
, to achieve the said isolation. Adding this feature has enabled Docker to graduate “rootless” to a fully supported feature. Rootless mode facilitates running the entire Docker environment without root system privileges.
Supporting dual logging for the first time, various third party logging drivers can now read docker logs
. This will provide a seamless approach when attempting to collect automated container log. Continuing support for CentOS8, the 20.10 release has added support for Ubuntu 20.10 and Fedora 33.
Focussing on the effectiveness of the CLI tool, Docker Engine 20.10 has new options such as:
docker push
now works similar todocker pull
, so that if the image name is pushed without a tag, only the:latest
tag will be pushed, rather than all tags- Environment variables can now be stored in a file and passed when running docker exec. The new
-env-file
allows for parsing environment variables from the specified file - Flags for
--pull=missing|always|never
options withcreate
andrun
commands provide more control over when to pull the images - Support for swarm jobs, to run batch jobs
Thanking the contributions by community, Paer-Gotch said, “When I say ‘we’ throughout this (blog) article I don’t just mean the (awesome) engineers at Docker, I mean the (awesome) engineers outside of Docker and the wider community that have helped shape this release.”
As the year 2020 concluded, Docker has repeatedly been in the news, as they introduced subscription tiers, and Kubernetes deprecated Docker Engine support with v1.20.0.
For getting started, our readers can install the packages available via the Docker website. To learn more about this release, check out the release notes. As of this writing, Docker has released two minor versions.
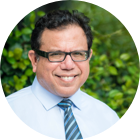
MMS • Dan Woods
Article originally posted on InfoQ. Visit InfoQ
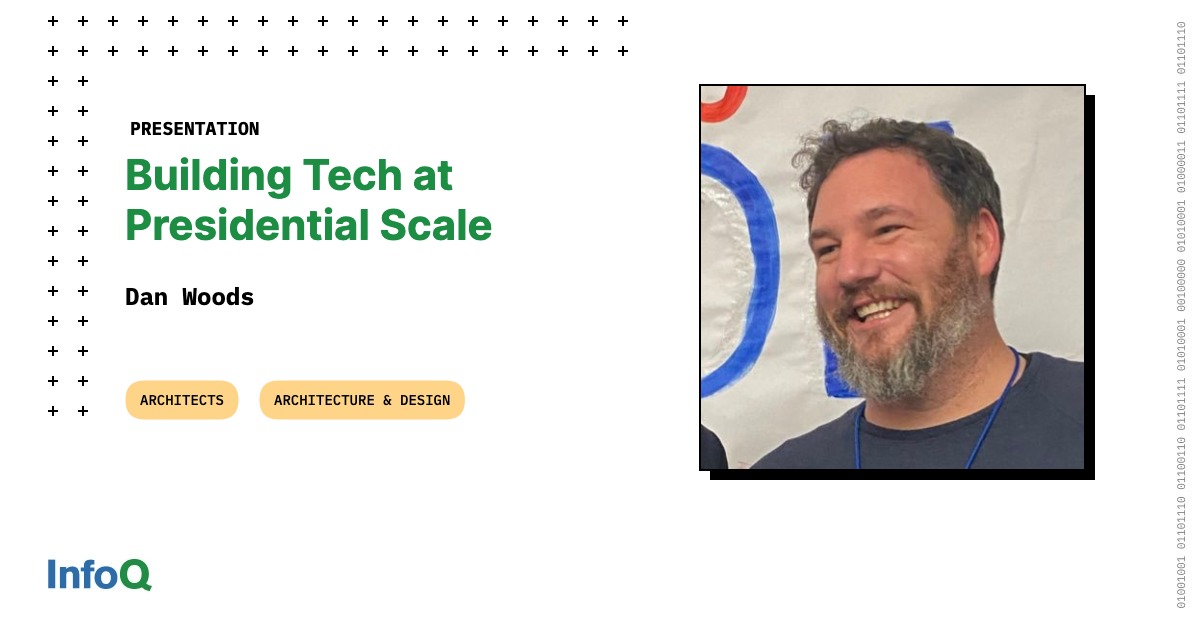
Transcript
Woods: I’m Dan Woods. I’ll be covering some of the more elaborate architectures built by the Biden for President 2020 campaign tech team. Overall, this is going to be a fairly technical conversation with deep dives into specific tools we built to solve a variety of problems.
Background
I was the primary CTO for Biden for President 2020, which means I built and led the technology organization within the campaign, and was overall responsible for the technology operations as a whole. I had a brilliant team along with me that built the best tech stack in presidential politics. We covered everything from software engineering, to IT operations, to cybersecurity, and all pieces in between. I’m currently a Distinguished Engineer at Target, which is also what I did before the campaign. I focused on infrastructure and operations with an emphasis on building high scale, reliable distributed systems. Prior to joining Target, I was a member of the team that worked on the Hillary for America 2016 technology platform by way of The Groundwork, which was a campaign adjacent technology company. Before my foray into presidential politics, I worked as a senior software engineer at Netflix, where I worked in the operations engineering organization, and helped create Netflix’s open source continuous delivery platform, Spinnaker. I’m also a member of the Ratpack web frameworks open source team, though haven’t been as involved lately as I’ve been a little bit busy. I wrote a book a couple years ago on Ratpack, and it’s published under O’Reilly. Be sure to go and check that out.
On Campaign Structure
Before we dive right into the technology, I want to spend a couple of minutes to discuss the mechanics of the organization of a campaign. Hopefully, this will show the intricacies of the moving parts of the campaign and help to underscore some of the specific avenues we had to follow when considering tech in this environment. I’ve laid out the different departments within the campaign to give you a sense of the varying degrees of responsibility across the organization. There were a number of teams across the campaign, each with their own specific concentration on a day-to-day basis. Although campaign tech gets a lot of attention in the press, it is suffice to say that tech is not the most important thing in politics. Obviously, reaching voters, driving the candidate’s message, and connecting as many people as possible to the democratic process is the North Star for everyone on the team.
The Role of Tech on a Campaign
What does tech do on a campaign? Quite literally, whatever needs to be done. Nearly every vertical of the campaign needs tech in one form or another. In practical terms, my team was responsible for building and managing our cloud footprint, all IT operations, vendor onboarding, and so forth. Our approach to building technology during the presidential cycle is simply to build the glue that ties vendors and systems together. This is not the right environment to delve into full-blown product development. As you’ll soon see, the needs of the campaign very much drive toward a similar outcome.
When a vendor or open source tool didn’t exist, or quite frankly, when we didn’t have the money to buy something, we would build tools and solutions. Overall, a huge portion of the work that needs to be done by a campaign tech team is simply getting the data from point A to point B. Strictly speaking, this involves creating a lot of S3 buckets. In addition to all that, we ended up developing quite a wide range of technology over the year and a half of the campaign cycle. We built dozens of websites, some big and some small. We built a mobile app for our field organizing efforts. We built Chrome extensions to help simplify the workloads for dozens of campaign team members. Likewise, though unglamorous to build, Word and Excel macros were developed to drive better efficiency. On a presidential campaign, time is everything, and anything that we could do to simplify a process to save a minute here or there, it was well worth the investment. Much of what we’ve done distills down to automating the tasks needed to reduce the load on the team any way that we could. It’s desirable at times to try to distill campaign tech down to one specific focus, data, or digital, or IT, or cybersecurity. In reality, the technology of the campaign is all of those things, and more, and a critical component of everything the campaign does.
What We Did
What did we do? To spell it out, plainly, we were a small and scrappy team of highly motivated technologists. Whatever the ask, we’d take it on and do our best where we could. Over the course of the campaign, we built and delivered over 100 production services. We built more than 50 Lambda functions that delivered a variety of functionality. We had more than 10,000 deployments in the short time we were in operation, all with zero downtime and a focus on stability and reliability. We built a best-in-class relational organizing mobile app for the primary cycle, the Team Joe App, which gave the campaign the leverage to connect thousands of eager voters and volunteers directly with the people they knew.
When we won the primary, we wanted a new, fresh branding on the website in time for the general election. We dug in and designed and delivered a brand new web experience for joebiden.com. In addition to all that, we implemented a bespoke research platform with robust automation that built on cloud based machine learning that ended up saving us tens of thousands of hours of real-world human time, and gave us incredible depth of insight. We made a commitment early on as a campaign, that we would hold ourselves to a higher standard, and make sure that we were not accepting donations from organizations that harm our planet, or from individuals who may have ulterior motives. To hold us true to that promise, we built an automation framework that vetted our donors in near real-time for FEC compliance and campaign commitments.
A huge aspect of every campaign is reaching directly to voters to let them know when an event is coming to their area, or when it’s time to get out to vote. For that, we built an SMS outreach platform that facilitated massive nationwide scale, and saved us millions of dollars in operational expense along the way. Beyond that, we operationalized IT for HQ, states, and ultimately facilitated a rapid transition to being a fully remote organization at the onset of quarantine. Through all of this, we made sure we had a world class cyber security posture. Indeed, it was a core focus for everything we did. We built a number of services on top of robust machine learning infrastructure, and did we do a lot of that? Ultimately, though, no one job on the campaign is single responsibility, which means everyone needs to dig in and help where help is needed. Whether that’s calling voters and asking them to go and vote, sending text messages, or collecting signatures to get on the ballot. We did it all.
Infrastructure and Platform
A little bit more under the tech. We relied on a fully cloud based infrastructure for everything we did. It was paramount that we didn’t spend precious hours reinventing any wheels for the core foundations of tech. For the main website, joebiden.com, we made use of Pantheon for hosting. For non-website workloads, APIs, and services, we built entirely on top of AWS. In addition to that, we had a small Kubernetes deployment within AWS that helped us deliver faster and simple workloads, mostly for cronjobs. We still needed to deliver with massive scale and velocity overall. For a small team, it was critical that our build and deploy pipeline was reproducible. For continuous integration, we used Travis CI. For continuous delivery, we used Spinnaker.
After services were deployed, and once they were up and running in the cloud, there’s a core set of capabilities they all need, like being able to find other services and securely accessing config and secrets. For those, we made use of HashiCorp’s Consul and Vault. This helped us build fully immutable and differentiated environments between Dev and prod, with very few handcrafted servers along the way, not zero, but very few. A huge part of the technology footprint was dedicated to the work being done by the analytics team. To ensure they had best-in-class access to tools and services, the analytics data was built on top of AWS Redshift. This afforded a highly scalable environment with granular controls over resource utilization.
For all of the services that we built and deployed, we utilized Postgres via RDS as the back-end datastore. From an operational perspective, we desired a centralized view into the logging activity for every application, so that we could quickly troubleshoot and diagnose, and not spend unnecessary time on MTTR when there was a problem. For that, we deployed an ELK Stack and backed it by AWS Elasticsearch. The logs are very important to applications. Metrics were the primary insight into the operational state of our services, and critical when integrating with our on-call rotation. For service level metrics, we deployed Influx and Grafana, and wired it into PagerDuty to make sure we were never experiencing an unknown outage. Many of the automation workloads and tasks don’t fit the typical deployment model. For those, we’d favor Lambda where possible. Also, for any workload that needed to integrate with the rest of the AWS ecosystem, and fan-out jobs based on the presence of data. All in all, we ended up creating a truly polyglot environment. We had services and automation built in a wide variety of languages and frameworks, and we were able to do it with unparalleled resiliency and velocity.
We covered a lot of ground during the campaign cycle, and it would take a lifetime to dive deep on everything we did. I’m going to pick out a few of the more interesting architectures we ended up putting together on the software engineering side of the tech team. By no means should that take away from the impressive array of work that was done on the IT and cybersecurity side of things. We’ll dive deep on the architectures. Just know that for each one of these, there are at least a dozen more conversations, covering the full breadth of what we accomplished across the entire tech team.
Donor Vetting
Let’s get started by diving into the donor vetting pipeline. We made a commitment early on as a campaign that we were not going to accept donations from certain organizations and individuals. The only way really to do this at any measure of scale, is to have a group of people comb through the donations periodically, usually once a quarter, and flag people who might fit a filtering criteria. This process is difficult, time consuming, and is prone to error. When done by hand, this usually involves setting a threshold for the amount of money an individual has given, and research whether or not they fit into a category we flagged as problematic. Instead, what we were able to do was build a high scale automation process that would correlate details about a donation against a known set of criteria we wanted to filter on. For example, we committed to not accepting contributions from lobbyists and executives in the gas and oil industry. Every day, a process would kick off that would go to ngpvan, which is a source of truth for all contributions, export the contribution data to CSV, and dump that CSV into S3. The act of the CSV file appearing in S3 would trigger an SNS notification, which in turn would activate a sequence of Lambda functions. These Lambda functions would split the file into smaller chunks, re-export them to S3, and would kick off the donor vetting process. We could see a massive scale in Lambda while this workflow was executing, up to 1000 concurrent executions of the donor vetting code. It would only take a matter of minutes to go through the comprehensive list of donors and check them against lobbyists’ registries, foreign agent registries, and block lists for gas and oil executives.
Once the process completed, the flagged entries were collated into a single CSV file, which was then re-exported to S3 and made available for download. We then used SES to send an email to Danielle, the developer who built the system indicating that the flags were ready for validation. After validation, the results were forwarded to the campaign compliance team to review and to take the appropriate action, whether that be refunding a contribution or investigating it further. To say this process saved a material amount of time for the campaign would be a dramatic understatement. The donor vetting pipeline quickly became a core fixture in the technology platform and an important part of the campaign operations.
Tattletale
Let’s talk about cybersecurity. Tattletale was one of the most important pieces of technology we built on the campaign, especially very early on when we were small and very scrappy. Essentially, because we had so many vendors and so many cloud services and not nearly enough time or people to constantly go and check the security posture on each of them, we needed a way that we could easily develop rules against critical user facing systems to ensure we were always following cybersecurity best practices. Tattletale was developed as a framework for doing exactly that. We developed a set of tasks that would leverage vendor system APIs to check to ensure things like two factor authentication were turned on, or to notify us if a user account was active on the system but that user hadn’t logged in in a while. Dormant accounts present a major security risk, so we wanted to be sure everything was configured toward least privilege spec.
Furthermore, the rules within Tattletale could check that load balancers weren’t inadvertently exposed to the internet, or that IAM permissions were not scoped too widely, and so forth. At the end of the audit ruleset, if Tattletale recorded a violation, it would drop a notification into a Slack channel for someone to go and investigate further. It also had the capability to email a user of a violation so they could take their own corrective action. If the violation breached a certain threshold, a metric would be recorded in Grafana that would trigger a PagerDuty escalation policy, notifying the on-call tech person immediately. Tattletale became our cybersecurity eyes when we didn’t have the time or resources to look for ourselves. It also made sure we were standardizing on a common set of cybersecurity practices, and holding ourselves to the highest possible standard.
Conductor and Turbotots
I want to take a moment and remind everybody that the hardest problem in computer science is naming things. To be frank, we didn’t spend a lot of time getting creative on names. Joe Biden is known well for his affinity toward Amtrak trains, and aviator sunglasses, but we exhausted all those metaphors pretty quickly. That leads me to a service that we built early on called CouchPotato, which was one of the tools where we heavily leveraged machine learning. Once we got the potato theme down, that was the end of it. We ended up mostly calling everything some derivative of potato. Conductor affectionately named for the trains theme, and Turbotots, a very fast cooked potato, represented a very important part of the platform we built. When we reached a certain point of complexity and span of internal tools, we knew we would need to better organize the API footprint and consolidate the many UIs we had built to manage those systems. We also needed to standardize on a unified security model, so we wouldn’t have bespoke authentication and authorization all over the place. Conductor and Turbotots were born. Enter our internal tools UI and platform API.
This is a dramatically simplified diagram of a very complex architecture. The broad strokes are good enough to give you an idea of what Conductor and Turbotots did and represented. Conductor became the single pane of glass for all internal tools we were developing for use by people on the campaign. In other words, this was the one place anybody on the campaign needed to go to access services we’d made. Conductor was a React web app that was deployed via S3 and distributed with CloudFront. Turbotots was a unified API that Conductor talked to and provided a common authentication and authorization model for everything we did. We built the AuthN and AuthZ portions of Turbotots, on top of AWS Cognito, which saved loads of work and provided a very simple SSO through G Suite, which we were able to configure for only our internal domains. Authentication was achieved by parsing JWT tokens through the workflow. For managing this on the front-end, we were able to make use of the React bindings for AWS Amplify, which integrated seamlessly into the app.
To the right is where things get a little bit trickier to understand, but I’ll do my best to simplify. The forward facing API was an API Gateway deployment with a full proxy resource. API Gateway has a very easy to use integration with Cognito, which gave us full API security at request time. The Cognito authorizer that integrates with API Gateway also performs JWT validation prior to a request making it through the proxy. We could rely on that system to know that a request was fully validated before it made it to the back-end. When developing an API Gateway proxy resource, you can connect code running in your VPC, by way of a VPC link network load balancer. This is all very complicated, but as I understand it, this effectively creates an ENI that spans the internal zone in AWS between API Gateway and your private VPC. The NLB, in turn is attached to an autoscaling group that has a set of NGINX instances that served as our unified API. This is the actual portion that we called Turbotots, and is, essentially, a reverse proxy to all of our internal services that were running inside the VPC. Following this model, we never needed to expose any of our VPC resources to the public internet. We were able to rely on the security fixtures that were baked into AWS, and that made us all feel a lot more comfortable overall.
Once the requests have made it through to Turbotots, within Turbotots, a lightweight Lua script extracted the user portion of the JWT token and passed that data downstream to services as part of a modified request payload. Once the request reached the destination service, it could then introspect the user payload, and determine whether or not the user was validated for the request. Essentially, users could be added to authorization groups within Cognito, which would then give them different levels of access in the downstream services. The principle of least privilege still applies here, and there are no default permissions. At the end of the day, Conductor and Turbotots represent a single user interface for the breadth of internal tools that was fully secured with seamless SSO to the user’s campaign G Suite account. We use this exact same architecture in the next section to open the door on exposing a limited subset of the API to non-internal users as well.
Pencil and Turbotots
Allow me to introduce you to Pencil, the campaign peer-to-peer SMS platform. Pencil grew to be a bit of a beast of an architecture from a very simple subset of early on requirements. It’s suffice to say that Pencil ended up being an area where we dedicated a significant amount of time. Originally, the idea with building our own P2P SMS platform was a cost savings play. I knew that obviously, we could send text messages through Twilio for cheaper than any vendor would be able to resell us the same capabilities. Since, originally, we didn’t need the broad set of features that vendors were offering, it was fairly easy to put together a simple texting system that met the needs of the moment.
Eventually, the scope of the project grew dramatically as popularity increased and the value was seen at scale. In the end, Pencil was used by thousands of volunteers to reach millions of voters and became a critical component in our voter outreach workflow. If you received a text message from a volunteer on the campaign, it was likely sent through Pencil. The external architecture for how we pulled this off is going to look very familiar because we were able to reuse everything that we did with Conductor and just change a few settings without having to change any code.
The user facing component to Pencil is a React web app deployed to S3 and distributed via CloudFront. The React app in turn talks to an API Gateway resource which is wired to a Cognito authorizer. Pencil users got into the platform, they were invited via email, which was a separate process that registered their accounts in Cognito. At first login, Pencil would automatically add them to the appropriate user group in Cognito, which would give them access to the user portion of the Pencil API. That’s admittedly a little confusing to reason about. I think it’s enough to say that from a user perspective, they clicked a link to sign up to send text messages. After a short onboarding process with the campaign digital field organizing team, they were all set up and able to get to work without any additional steps. It was very easy to do and worked great because it was built on top of the same Turbotots infrastructure we’d already developed.
Tots Platform
Tots represents a very different slice of the architecture than Turbotots, but with something of a proceeding reference implementation for what Turbotots eventually became. Tots existed first, and therefore was able to keep its name. Tots is actually a direct downstream path from Turbotots. On those prior slides where I’d listed the services beneath Turbotots, a number of them were parsed by way of tots and are a part of this architecture. Tots is a very important platform, because as we start to build more tools that leverage machine learning, we quickly realized that we needed to consolidate logic and dry up the architecture. In effect, our biggest use case for machine learning in various projects was extracting entity embeddings from blocks of text. In turn, we were able to organize that data in an index in Elastic and make entire subjects of data available for rapid retrieval later on. We use AWS Comprehend to extract entity embeddings from text. It’s a great service. Just give it a block of text, and it’ll tell you about people, places, subjects, and so forth, about what’s being discussed in that text.
The blocks of text came into the platform in a variety of forms, be it multimedia, news articles, or document format. Figuring out what to do before something went off to Comprehend is much of the mechanics in the Tots platform that you’re looking at. The process codified in this platform saved the campaign, quite literally, tens of thousands of hours of equivalent human time on organizing and understanding these various documents. This includes time spent transcribing live events like debates, and getting them into a text format that could be read later on by various people on the campaign.
CouchPotato
Last we’ll cover today but certainly not least, is CouchPotato. CouchPotato became very important to us because of the sheer amount of time savings it gave us on audio, video material. It’s also one of the most technically robust platforms, and required us to solve the actual hardest problem in computer science, making audio work on Linux. In spite of the lines connecting independent services here, CouchPotato is the main actor in this architecture, but it needed a lot of supporting characters along the way. From the bottom up, CouchPotato’s main operating function was to take a URL or media file as input, open that media in an isolated X11 Virtual Frame Buffer, and listen to the playback on a pulseaudio loopback device, FFmpeg, then recorded the contents from the X11 session. Produced an mp3 file, which then was later able to send over to Transcribe to perform an automated speech recognition.
Once the ASR process was completed, it would correct the transcript for some common mistakes. For example, it could rarely get Mayor Pete’s name correct. We had a RegEx in place for some common replacements. Then once all that was done, it would ship it over to Comprehend to extract the entity embeddings. Finally, the text would be indexed in Elasticsearch. That was that. The real key to CouchPotato is that it was able to make use of FFmpeg’s segmentation feature to produce smaller chunks of audio that could be reasoned about. Then it would run the whole process for each segment, and make sure they all remained in uniform alignment as they were being indexed in Elasticsearch. The segmentation was an initial feature in CouchPotato, because we used it to transcribe debates in real time.
Normally, you’d have an army of interns sitting there watching the debate and typing out what was being said throughout. We didn’t have an army of interns to do that, and so CouchPotato was born. However, the segmentation also created a real-world serialization problem. Sometimes Transcribe would finish subsequent segments, ASR, before the prior segment had completed, meaning all the work needed to be done asynchronously, and the order recompiled after the fact. It sounds like a reactive programming problem to me. We invested a lot of time in solving the problem of serializing asynchronous events across stateless workers. We made it work. That’s also where docsweb came in handy for the debate. Docsweb connected the transcription output from the segments produced by CouchPotato to a Google Doc, which allowed us to share the transcribed text in near real-time, except for the lag from Transcribe to Elastic, which wasn’t too bad. We were able to share the output of that with the rest of the campaign. We transcribed every single debate and loads of other media that would have taken a fleet of interns a literal human lifetime to finish.
Part of solving the segmentation serialization problem is figuring out a way we could swap a set of active CouchPotato instance for a new one, say, during a deploy or when a live Transcribe event was already running. There’s a lot more we can go into on that subject itself even alone, like how we made audio frame stitching work, so we could phase out an old instance and phase in a new one. It’s too much to go into for just this presentation. Just know, there was a lot of work in HotPotato to make it so we could do hot deployments of CouchPotato with zero deployments and zero state loss.
KatoPotato is the entry point into this whole thing. It’s an orchestration engine that coordinated the movement of data between CouchPotato, docsweb, and Elastic, as well as acting as the main API for kicking off CouchPotato workloads. Furthermore, it was responsible for monitoring the state of CouchPotato, and deciding if HotPotato needed to step in with a new instance. The best thing I can say about that is sometimes audio on Linux can be finicky, and CouchPotato was able to report back to KatoPotato, whether it was actually capturing audio or not. If it was not capturing audio, KatoPotato could scale up a new working instance of CouchPotato and swap it out via HotPotato. At the end of the day, the marketing line for CouchPotato is it’s a platform that built on Cloud Machine Learning that gave us rapid insight into the content of multimedia without having to spend valuable human time on such a task. It’s a great piece of engineering, and I’m glad it was able to deliver the value it did.
Conclusion
We covered a lot of ground in this presentation. There’s so much more we can talk about like the unmatched relational organizing app we built, or the data pipelines we created to connect S3 and RDS to Redshift, or the dozens of microsites we built along the way, or even simpler things like how we manage work collaboratively in an extremely fast-paced and dynamic environment.
See more presentations with transcripts
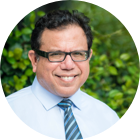
MMS • Steef-Jan Wiggers
Article originally posted on InfoQ. Visit InfoQ
Eventarc is an eventing functionality available on the Google Cloud Platform (GCP), allowing customers to send events to Cloud Run from more than 60 Google Cloud sources. In a recent blog post, the company announced the general availability (GA) of Eventarc.
In October last year, Google released the preview of Eventarc to provide customers with a service to connect Cloud Run services with events from various sources, adhering to the CloudEvents standard. With the GA release, the company made updates to the gcloud commands, allowing users to create triggers from existing Pub/Sub topics, and increase availability through additional regions.
The updated Eventarc gcloud commands do not need the beta flag anymore, and the –matching-criteria flag is renamed to –event-filters. Furthermore, the –destination-run-region is now optional, and to use an existing Pub/Sub topic, Eventarc now allows that with an optional –transport-topic gcloud flag. Additionally, users can leverage a new command to list available regions for triggers.
gcloud eventarc triggers create trigger-pubsub
--destination-run-service=${SERVICE_NAME}
--event-filters="type=google.cloud.pubsub.topic.v1.messagePublished"
--location=europe-west1
These updates are also incorporated into the Google Cloud SDK.
With Eventarc, Google joins other public cloud vendors in providing a generally available service on their respective cloud platform to manage events centrally. Microsoft released Event Grid, a service that enables developers to manage events in a unified way in Azure, over two years ago into GA.
Furthermore, AWS followed in 2019 with Amazon Eventbridge – a serverless event bus that allows AWS services, Software-as-a-Service (SaaS), and custom applications to communicate with each other using events. And lastly, other vendors are also providing an event bus service similar to Event Grid and EventBridge, such as TriggerMesh offering EveryBridge. All of them support CloudEvents.
In another Google blog post, Mete Atamel, developer advocate at Google, wrote:
The long term vision of Eventarc is to be the hub of events from more sources and sinks, enabling a unified eventing story in Google Cloud and beyond.
And:
In the future, you can expect to read events directly (without having to go through Audit Logs) from more Google Cloud sources (eg. Firestore, BigQuery, Storage), Google sources (eg. Gmail, Hangouts, Chat), 3rd party sources (eg. Datadog, PagerDuty) and send these events to more Google Cloud sinks (eg. Cloud Functions, Compute Engine, Pub/Sub) and custom sinks (any HTTP target).
Further details of Eventarc are available on the documentation landing page.
Article: AI Applied in Enterprises: Information Architecture, Decision Optimization, and Operationalization
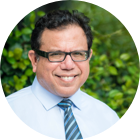
MMS • Ben Linders Eberhard Hechler Martin Oberhofer Thomas Schaeck
Article originally posted on InfoQ. Visit InfoQ
The book Deploying AI in the Enterprise by Eberhard Hechler, Martin Oberhofer, and Thomas Schaeck gives insight into the current state of AI related to themes like change management, DevOps, risk management, blockchain, and information governance. It discusses the possibilities, limitations, and challenges of AI and provides cases that show how AI is being applied.
By Ben Linders, Eberhard Hechler, Martin Oberhofer, Thomas Schaeck
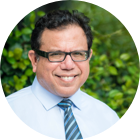