Month: August 2018
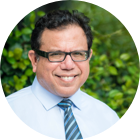
MMS • RSS
Article originally posted on InfoQ. Visit InfoQ
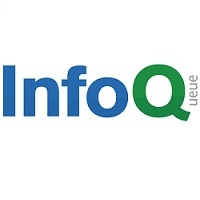
Earlier this year, Perpetual Guardian, a New Zealand company that manages trust, wills and estates, invited its two hundred and forty employees to join an eight-week experiment during which they would work four days a week and enjoy an extra leisure day, although getting the same amount of money.
The trial, led by the company’s CEO and co-founder Andrew Barnes, sought to challenge the entire company in joining a discussion about the future of work and how increased flexibility and free time may impact employees’ productivity and company outcomes.
To ensure robustness of the process, they hired two academic researchers to prepare, conduct and analyse the results of the research.
Some of the key results shared by the research are:
- Leaders reported that job performance remained at the same level
- Staff stress levels were lowered from 45% to 38%
- Work-life balance improved significantly, from 54% to 78%
- Team engagement levels increased from an interval of 64%-68% to 82%-88%
According to Barnes,
Our analysis of the results shows the objectives of the trial were successfully met. The key areas we sought to measure, including work-life balance, engagement, organisational commitment and work stimulation, all showed positive increases – that is a powerful combination that leads to job satisfaction
Christine Brotherton, head of people and capability of the company, mentioned that the research was also an opportunity for some unexpected findings. For instance, she observed a clear relationship between the empowerment given by managers to embrace the change and the staff motivation levels to succeed.
We have been able to identify deficiencies in leadership that perhaps we may not have had the opportunity to see if not for the trial,
she mentioned.
From the researchers’ perspective, both quantitative and qualitative analyses have shown positive signs. Jarrod Haar, professor of human resource management at Auckland University of Technology, has looked into numbers related to job satisfaction, engagement and retention, and stated that:
Already high pre-trial, these significantly increased post-trial and the scores are easily the highest I have seen in my New Zealand data. In summary, employees reported enhanced job attitudes reflecting positive effects from the trial.
In correlation, Dr Helen Delaney, senior lecturer at the University of Auckland Business School, mentioned that due to the fact that employees were involved in the preparation and planning of the trials, they have expressed a sense of greater empowerment and as a result of that,
Employees designed a number of innovations and initiatives to work in a more productive and efficient manner, from automating manual processes to reducing or eliminating non-work-related internet usage.
As the subject gained attention from the business world, having been mentioned in places like The Guardian, CNBC or The New York Times, the company is inviting anyone who’s interested in participating in this discussion to join them on the four-day week website.
InfoQ spoke to Barnes in order to get more insights into how the idea was born, how it can be applied in other companies for instance in the professional software development industry, and what the plans and expectations for the near future are.
InfoQ: How did you come up with the idea of a four-day work week? Were there any specific triggers for that?
Andrew Barnes: I read a couple of articles in the Economist which indicated that true productive hours were less than 2.5 hours a day. This got me thinking as to the reasons for this. 1. Social… people spend time each day having coffee, talking to coworkers on social topics and also using social media. 2. Work interruptions, especially in open plan offices; the evidence suggests that if one is interrupted whilst working it can take 20+ minutes to get back to the same level of productivity. 3. Home and family, issues which crop up, e.g building work etc., which need to be dealt with during the working week. My thesis was if one gifted a day a week to staff, would this change these behaviours…and if so, we didn’t need a significant productivity gain each day to make up for the lost output.
InfoQ: In your opinion, what was the most unexpected result of the trial?
Barnes: People’s perception of their workload was less under the trial. I expected stress levels to drop and work-life balance to go up, but I didn’t expect that the staff would then view their workload to be more manageable over four days, rather than five.
InfoQ: InfoQ is a knowledge sharing and innovation community on professional software development. Do you see approaches like this working in this industry?
Barnes: I see no reason why not. The key is to ask team members how they want the approach to be adopted, and to give them ownership of the methods by which it is introduced and monitored.
InfoQ: If someone wants to try it out, does it need to be applied to the entire company or do you see it as feasible considering only some areas of the company, or even only a couple of teams?
Barnes: My suggestion is to try it out whichever way works for you. At least one of our teams opted out of the trial. The key thing is to demonstrate that it works and to apply the learnings to the whole business.
InfoQ: You’ve been noticed on several news websites. Do you have any concrete plans for the near future?
Barnes: This is now a bit of a movement, and given the international and domestic reaction to our trial, I am keen to ensure that the conversation regarding more flexible working is continued. But the first thing is to implement the four-day week permanently in our company; we are just working through the legal terms now.
InfoQ: When we hear you speaking about your vision, it resonates as much bigger than changing the way your company and employees behave. May I ask what would make you happy in three years?
Barnes: To see this, or variants of this adopted in New Zealand and globally. There has to be a better way.
Besides the work being done in his company, Barnes is challenging other leaders to think differently too. The following are examples of recent quotes of his:
If you can have parents spending more time with their children, how is that a bad thing? Are you likely to get better educational outputs as a consequence?
Are you likely to get fewer mental health issues when you have more time to take care of yourself and your personal interests -probably. If you can take 20 percent of people off the roads every day, what does that mean?
I am therefore challenging all other CEOs out there – start your own trial.
Your worst-case scenario is that you will gain a more engaged, committed and energized workforce.
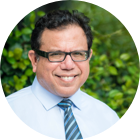
MMS • RSS
Article originally posted on InfoQ. Visit InfoQ
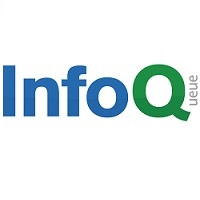
California has enacted the California Consumer Privacy Act (CCPA) of 2018 which, starting on January 1, 2020, would grant consumers several rights with respect to information about them that businesses collect, store, sell, and share. Consumers are “natural persons” who are residents of California. This is the first legislation of its kind in the United States.
Overview
Consumers would have the right to request that a business disclose the information it has about them, the categories of sources from where it was collected, the business purposes for collecting or selling, and the categories of third parties with which it is shared. Business would have to delete personal information based on a verified request by a consumer. Consumers also have the right to know what information is collected ahead of time.
Generally, a consumer must opt-out to the collection of data.
The law prohibits discrimination against a consumer that makes such a request. The definition of discrimination includes a different quality of good or service except if it is related to the value of the consumer’s data. How this applies to a business that offers free services in exchange for information collection is unclear.
Businesses must state their privacy policy and their policy regarding the use and sale of consumer information. Businesses are allowed to keep information for internal purposes. The law does not define what that means.
Definition of Personal Information
The CCPA defines personal information broadly, going beyond what is usually considered sensitive or financial information. “”Personal information” means information that identifies, relates to, describes, is capable of being associated with, or could reasonably be linked, directly or indirectly, with a particular consumer or household.” These include, but are not limited to:
- “Identifiers such as a real name, alias, postal address, unique personal identifier, online identifier Internet Protocol address, email address, account name, social security number, driver’s license number, passport number, or other similar identifiers.”
- Commercial information, including records of personal property, products or services purchased, obtained, or considered, or other purchasing or consuming histories or tendencies.
- Biometric information.
- “Internet or other electronic network activity information, including, but not limited to, browsing history, search history, and information regarding a consumer’s interaction with an Internet Web site, application, or advertisement.”
- Geolocation data.
- “Audio, electronic, visual, thermal, olfactory, or similar information.”
- “Professional or employment-related information.”
- “Educational information”
- “Inferences drawn from any of the information identified in this subdivision to create a profile about a consumer reflecting the consumer’s preferences, characteristics, psychological trends, preferences, predispositions, behavior, attitudes, intelligence, abilities, and aptitudes.”
It does not include publically available information.
Allowable Exceptions
Small businesses and non-profit organizations appear to be generally exempt, but could be covered if they engage in certain information gathering activities. Information must be supplied to comply with federal, state, or local laws, as well as, legal and regulatory subpoenas, summons or reasonable law enforcement investigations.
Business can use, retain, sell, or disclose de-identified or aggregated information.
Business can collect or sell personal information if every aspect of the transactions takes place wholly outside of California. Business cannot, however, store personal information on a device while a consumer is in California, and use it to collect data outside of California.
Penalties for Failure to Comply
The bill provides for enforcement by the Attorney General of California, as well as allowing private consumers to sue after a data breach. A data breach is defined as unauthorized access, theft, or disclosure of unencrypted or non-redacted information as a result of a business failing to maintain a reasonable security procedure. There is also a liability for loss of paper data. The Attorney General can enforce all provisions of the legislation.
The bill defines a method for distribution of proceeds of Attorney General actions. The bill would create the Consumer Privacy Fund in the General Fund with the moneys in the fund, upon appropriation by the Legislature, to be applied to support the purposes of the bill and its enforcement. The bill would provide for the deposit of penalty money into the fund.
The act does not define what a reasonable security procedure is. The California Attorney General has previously cited the twenty controls in the Center for Internet Security’s Critical Security Controls as defining the minimum level of information security for organizations that use personal information.
The legislation “would void a waiver of a consumer’s rights under its provisions.”
The bill would require the Attorney General to solicit public participation for the purpose of adopting regulations, as specified. The bill would authorize a business, service provider, or 3rd party to seek the Attorney General’s opinion on how to comply with its provisions.
Legislative History
The bill was reintroduced, passed, and signed by the Governor in one week.
The reason for this speed was to pre-empt a ballot initiative that would appear in the November 2018 election. The sponsors of the ballot stated they would withdraw the proposal from the ballot if the California Consumer Privacy Act was passed and signed by the Governor by June 29.
Dating from the progressive era, citizens of California can put on ballot a proposed law. If it passes, it becomes state law that cannot be amended by the state legislature. Amendments must be made by other initiatives.
Impact
While the legal protections are only available to citizens of California, in practice they will affect a large fraction of the United States. The state is the most populous in the United States, and its economy, if it were a separate country would be the 5th largest in the world, bigger than the UK or France. Hence, many businesses outside of the state would find it cumbersome to treat California and non-California residents differently with respect to privacy policies or opt-out provisions. Theoretically, even a non-California IP address could be used inside California, and a California resident could transact business temporarily outside of California.
Comparison with European Union’s General Data Protection Regulation
Despite being superficially similar they are very different. The California legislation’s scope is much more restricted. Nonetheless, compliance with the General Data Protection Regulation (GDPR) will not make you automatically compliant with the CCPA.
The California legislation is restricted to consumer privacy rights and consumer disclosures. The GDPR not only regulates consumer disclosures, but it has procedures for data breaches, notifications to individuals and regulators, how to implement data security, as well as rules concerning cross-border data transfers. The GDPR grants rights such as rectification, not to be subject to a decision based on automated decision making, and possibly a right to be forgotten. (link to my article). The GDPR requires users to actively opt-in for consent. The CCPA generally requires only the ability to opt-out from the use of their personal data. The CCPA requires a toll-free number to be available, the GDPR does not. How companies comply with these conflicting requirements is unclear.
The GDPR does not disallow different pricing mechanisms depending on the degree of data consent. The CCPA is not completely clear on what allowable financial incentives business can offer their customers to encourage data collection. The definition of consumer data is much broader under the CCPA than the GDPR. The allowable exceptions to disclosure and deletion are different.
Implications
The legislation does not take effect for another year and a half. That would give plenty of time for the law to be amended, or for national legislation to be passed that would override the California law. The latter is a distinct possibility if multiple, conflicting state laws are passed. Industry may feel that it is able to get weaker, national legislation passed, especially with respect to eliminating consumer lawsuits. Industry groups and national legislators are also caught between what their customers now expect, and what they think their businesses need. As is usual with such legislation, the national government would either create a new agency, or have the Federal Trade Commission, write new regulations.
The other interesting aspect is that the large technology companies may want complicated, detailed regulations which only they have the time, money, and resources to implement. This would create a barrier to entry for competitors. There have been reports of small companies in Europe shutting down because the GDPR is too onerous.
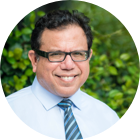
MMS • RSS
Article originally posted on InfoQ. Visit InfoQ
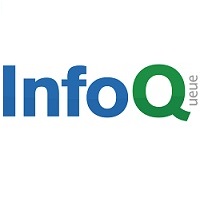
The International Consortium for Agile (ICAgile) hosted a panel discussion at the Agile2018 conference in San Diego on Aug 7th, 2018 about the Agile Coaching profession.
The panel discussed what an agile coach is, the coaching competencies, where the career has been and the future direction of coaching. ICAgile is an international accreditation and certification body that provides knowledge and competence-based learning programs for various agile disciplines. ICAgile’s Agile Coaching Track is one of its most popular learning tracks, with thousands certified from various learning partners around the globe.
What Does a Coach Do?
- Does a coach give advice?
- Ask powerful questions?
- Teach the team plays?
- Lead Change?
- Show by Example?
All of the above!
The panel discussed the various “coaching stances” that Agile Coaches use throughout their day as agile coaches. These stances highly relate to the critical skills that agile coaches need to be effective. Many who grow into the Agile Coaching role, typically from a Tech Lead, Scrum Master, or Project Management role, may understand one of these stances (Teaching, Facilitating, Mentoring, Coaching). Learning how to act effectively in all of them, and even harder, choosing the right one at the right time is where the coaching skill is truly in practice.
“Coaching” by definition is often defined as asking powerful questions to lead an individual or group to action. While this is a critical skill for Agile Coaches, knowing when to be a mentor, facilitator, teacher, or coach is also part of the secret sauce.
The Agile Coaching discipline has come along way. In 2011 there wasn’t much definition or a learning path to grow in the career. The agile industry needed a common definition and alignment on what agile coaching means. ICAgile got a group together to address this challenge, and the result was the initial Agile Coaching Track, Learning Outcomes, and Competencies.
The panel discussed some key skills and attributes of Agile Coaches:
- In control of themselves
- Devoted to the outcome, and hold the team and organization to that outcome
- Able to intervene
- Hard facilitation
- Give advice
- Raise awareness
- Be in service of a bigger outcome
- Empathy to meet a team where they are at
- Patience
Agile coaching is a role and career that can be very rewarding, requires a lot of skill, and may not be a natural role for everyone. With learning, practice, and awareness of oneself and others, many can learn and grow amazing careers in coaching.
As the discipline is constantly evolving, the learning objectives, content, and competencies are evolving as well. The latest learning objectives can be found here
Speaker Info
The Panel, moderated by Shannon Ewan, Managing Director of ICAgile:
Panel:
Podcast: Mike Lee Williams on Probabilistic Programming, Bayesian Inference, and Languages Like PyMC3
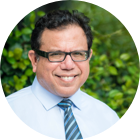
MMS • RSS
Probabilistic Programming has been discussed as a programming paradigm that uses statistical approaches to dealing with uncertainty in data as a first class construct. On today’s podcast, Wes talks with Mike Lee Williams of Cloudera’s Fast Forward Labs about Probabilistic Programming. The two discusses how Bayesian Inference works, how it’s used in Probabilistic Programming, production-level languages in the space, and some of the implementations/libraries that we’re seeing.
Key Takeaways
- Federated machine learning is an approach of developing models at an edge device and returning just the model to a centralized location. By taking the averages of the edge models, you can protect privacy and distribute processing of building models.
- Probabilistic Programming is a family of programming languages that make statistical problems easier to describe and solve.
- It is heavily influenced by Bayesian Inference or an approach to experimentation that turns what you know before the experiment and the results of the experiment into concrete answers on what you should do next.
- The Bayesian approach to unsupervised learning comes with the ability to measure uncertainty (or the ability to quantify risk).
- Most of the tooling used for Probabilistic Programming today is highly declarative. “You simply describe the world and press go.”
- If you have a practical, real-world problem today for Probabilistic Programming, Stan and PyMC3 are two languages to consider. Both are relatively mature languages with great documentation.
- Prophet, a time-series forecasting library built at Facebook as a wrapper around Stan, is a particularly approachable place to use Bayesian Inference for forecasting use cases general purpose.
- 01:55 I did my PhD in astrophysics too many years ago; it turns out that astrophysics is a great preparation for a career in data science.
- 02:05 Firstly, there are a lot of stars out there – it’s a big data problem.
- 02:15 Secondly, there are problems with the data all the time.
- 02:25 This mirrors what we see in tech – big data, with various problems, trying to draw conclusions from it that allow you to take actions – publish or perish in the academic world.
- 02:40 It turns out the preparation is appropriate.
- 02:45 The downside of astronomy is that many of us don’t have a CS background, we’re self-taught coders, we maybe don’t know what Big O notation is.
- 02:55 It’s something I’ve personally worked on with my own skillset – one of the challenges in migrating from astrophysics.
Many CS developers feel that there is gaps they are missing.
- 03:20 My PhD was measuring how much matter is missing in galaxies.
- 03:30 I used to say what I was doing was to saying how big the hole is accurately.
- 03:35 Particle physicists and theoretical physicists tried to fill in the hole.
What is federated learning?
- 04:00 At Fast Forward Labs, we publish a report four times a year that we think will be of interest to our subscribing clients.
- 04:10 Federated learning is what we’re working on right now.
- 04:15 Imagine you are training your learning model on an edge device, like a mobile phone, which has the training data.
- 04:20 The traditional way of handling this is to ship the training data to a central computer somewhere from all the mobile devices, in order to train the model.
- 04:30 The problem with that is that most people are uncomfortable with that idea.
- 04:45 In certain legal areas like healthcare it’s not even possible.
- 04:50 We’ve all got data plans with finite amounts of data so we don’t want to ship a lot of data to a central place.
- 05:00 Federated learnings comes in to train little local models, that aren’t very big, on a variety of devices.
- 05:10 Instead of shipping back the training data, you ship back the model, which is a much smaller piece of data.
- 05:20 Additionally, it’s not the data, so it’s not the most privacy sensitive data.
- 05:30 There are ways, given a model, of finding out what went into it, and it’s a much harder job – and you can add in differential privacy to give guarantees.
- 05:45 The central authority’s job is to average the models, and that becomes the global model over all the data, but in a privacy preserving way.
- 05:50 We preserve data locality and privacy, which has a lot of implications for consumer tech and heatlhcare.
- 06:00 Also, in industrial situations where there is a lot of data, and potentially in remote locations or the data connection isn’t stable, the reduction in data can be a big win.
What is probabilistic programming?
- 06:40 Probabilistic programming is a programming paradigm in the same style as functional or object-oriented programming.
- 06:45 It is a family of programming languages that make a kind of problem easier to describe and solve.
- 06:55 The problem that probabilistic programming solves is statistical problems – analysing data and drawing conclusions from data.
- 07:00 The reason that it is difficult in general is that all data is flawed in some way.
- 07:15 The conclusions that you draw are uncertain because the data might be flawed.
- 07:20 You may not have all the data – an A/B test isn’t unlimited but rather run over a week, for example.
- 07:25 You might have data that is dubious in some other way – measuring a physical system with variability, such as the speed of a car may not be accurate to a millimetre per second.
- 07:40 That uncertainty manifests itself as uncertain conclusions, and we’d like to know the bounds of that uncertainty.
- 07:45 At a high level, what probabilistic programming does is provide a more user-friendly way to answer some of those questions.
How is the A/B testing affected?
- 08:20 If you do an A/B test, and users convert for layout A 4% of the time, and for layout B 5% of the time, you might conclude that layout B was better.
- 08:30 But if it turns out that the A/B test was very small, then 5% of the users converted but there are only 100 visitors, then you’re less certain that layout B is better.
- 08:45 What probabilistic programming gives you is a number that can tell you how uncertain these results are.
- 09:10 It’s important to note that probabilistic programming is a family of languages, but what we are doing is Bayesian inference, which is a 250/300 year old algorithm.
What is Bayesian inference?
- 09:30 The Reverend Bayes, hundreds of years ago, came up with Bayes’ rule, which is a relatively simple one.
- 09:40 It relates the thing yo know before the experiment, and the results of the experiment, and tells you what you know after the experiment.
- 09:50 In the case of the A/B test, if you have a prior belief that the conversion rate is 5% give or take a couple of percent.
- 10:05 The data may be at a high level consistent with the beliefs, but the beliefs will necessarily change after that.
- 10:15 Bayesian inference is a set of machinery about the prior beliefs and turning them into beliefs after the experiments (the posterior beliefs).
- 10:35 It’s a tool for turning what you know before the experiment, and the results of the experiment, into answers about what to do next.
- 10:45 The equation is really simple – but as a practical matter, its implementation in code can be quite tricky.
- 11:00 What those imply is that for you and I it was quite a difficult approach to solve real problems.
- 11:15 Reverend Bayes used it to solve simple problems hundreds of years ago, but internet scale problems with large data and large numbers of parameters are difficult computationally to solve.
- 11:30 Probabilistic programming is exciting, because it abstracts a huge amount of that complexity away and provides a gold standard technique accessible to less sophisticated statisticians.
- 11:45 If the last time you took stats was high school – this approach is accessible for non-professional statisticians.
The process can be iterative to improve the beliefs?
- 12:20 It’s a virtuous circle – if you’re in a streaming environment where the data is arriving every day, you don’t have to wait until you have gathered a week’s data to see results.
- 12:35 You can use the stream of data to update the beliefs as new data comes in.
- 12:40 As each piece of data arrives, your prior is updated to a posterior distribution.
- 12:45 That posterior distribution becomes the next prior for the next piece of data coming in.
- 12:50 The posterior at the end of this sequence of data is available to you throughout the stream as a light picture of your knowledge of the world.
- 13:05 In the layout test, you know whether A or B is doing, and you can end that test early if the results are obvious.
- 13:10 Perhaps most famously this analysis is done during drug trials – is the drug working, or is it killing people?
- 13:20 You don’t want to wait until the end of the trial to find out that three people have died, and that is statistically more than you’d expect.
- 13:30 You want to be able to end the trial as soon as you know the drug is effective (or it is dangerous).
So you can bring forward the prediction models to be almost live with the data coming in?
- 14:05 It’s a coherent way of looking at things – it works in the batch setting as well.
- 14:10 You can give me a million data points, and I can run the batch analysis telling you whether layout A or layout B is better.
- 14:20 You can also give me a thousand data points at a time, or a day’s worth of data, and you can get the data with a day’s worth of latency.
- 14:35 Alternatively, you can go with streaming data and keep this posterior data updated as each visitor arrives.
- 14:40 Obviously there are engineering practicalities with that approach, but all three of these approaches are using Bayes’ rule.
- 15:00 It’s a difference of degrees, rather than a new system, but you can migrate from batch analysis to streaming analysis.
- 15:10 As you say, the really exciting possibility is being able to forecast about the future.
- 15:15 Bayesian inference or probabilistic programming aren’t the only ways to be able to do this; supervised machine learning in general is capable of doing that.
- 15:30 The thing I like about it is that Bayesian predictions come with measures of uncertainty.
- 15:40 If we are doing the layout test, we can imagine predicting about a number of how many dollars we are going to get in the future.
- 15:50 What is at least as useful is a measure of how certain I am about that.
- 16:00 Do I think I am going to make a million dollars, plus or minus a dollar, or plus or minus a hundred thousand dollars?
- 16:10 Those have different implications about how the CFO should plan or how much money needed in the bank.
- 16:15 You can quantify the risk – in financial situations, that’s very relevant.
- 16:20 In situations where you are planning for the future, you need resources in proportion to how often something happens.
- 16:30 If you’re able to predict when something is going to happen, and how uncertain you are, makes your ability to prepare that much richer and more rigorous.
What did your QCon New York talk about?
- 17:40 The approach I took in my talk was trying to build a probabilistic programming system from scratch.
- 17:55 One of the things you get when you build a probabilistic programming system from scratch is how slow it can be without being very clever.
- 18:05 To get it to be fast, you need to have a graduate degree in statistics.
- 18:10 A number of deep mathematical problems were solved in the last couple of decades, and they are robust.
- 18:20 That means the user of the algorithm doesn’t need to tune it for each problem – it’s idiot proof in a lot of ways.
- 18:35 The most robust algorithm – already not the most cutting edge, as it’s a fast moving field – is Hamiltonian Monte-Carlo with automatic differentiation and a no u-turn sampler.
- 18:50 It’s harder to code than it is to say, and it’s pretty difficult to say.
- 18:55 It turns out my background in astrophysics is surprisingly relevant to that algorithm – shares a lot in common with orbital mechanics of the Solar system.
- 19:15 All this is building up to saying is that probabilistic programming languages to an extent become one line.
- 19:20 It’s up to you to describe your data and your problem, but you essentially push the Hamiltonian Monte-Carlo button, and out pops your posterior.
- 19:30 In practice, it can be harder or easier for some kinds of problems.
- 19:35 It is, in terms of code, a one-liner.
- 19:40 Probabilistic programming languages have industrial strength very fast implementations of algorithms that would otherwise be tremendously difficult for most people to implement.
- 19:50 The other thing they do is define a primitive data type for the objects we thing about when doing probabilistic programming.
- 20:00 If you have a GUI toolkit library, you’re going to define a window and a close button.
- 20:05 Probabilistic programming languages do the same thing – they provide a library of primitives relevant to these kind of problems.
- 20:10 In particular, random variables and probability distributions are off-the-shelf, well designed, fast implementations that you can compose together to describe your problem.
- 20:25 The problem description you and up writing in these languages ends up being very declarative.
- 20:30 You don’t find yourself writing a lot of for loops, or the order in which things need to happen: you simply describe the world and press go, and the probabilistic programming language figures out the implications of that.
- 20:40 That at least is the goal; the devil is in the detail.
What are some of the languages being used in real world settings?
- 20:55 If you have a practical, real-world problem today, there are two languages: Stan and PyMC3.
- 21:00 Both of these are appropriate and fast enough to solve big problems – they are relatively mature languages with great documentation.
- 21:10 PyMC3 as you may have guessed from the name is like a super-set of Python – and in that sense PyMC3 is probably the more user friendly for most people listening to this podcast.
- 21:20 On the back end it is using a library called ‘Torch’, which if you have done any deep learning you may have come across before – it’s an end-of-life 1.0 deep learning library.
- 21:30 What PyMC3 uses it for is to do automatic differentiation – they didn’t implement it themselves, they used a deep learning library.
- 21:40 It’s not deep learning we are talking about but it has strong overlaps at a high level and low level.
- 21:50 PyMC3 is going to do all of these things – it has got a fast implementation of algorithms like Hamiltonian Monte-Carlo, and other algorithms that may be more appropriate in other situations.
- 22:00 PyMC3 is pretty smart about figuring out which of those algorithms to use, in addition to doing a good job of implementing them.
- 22:05 It’s got random distribution and random variable primitives.
- 22:10 You write Python syntax, which looks a little unusual, but fundamentally you are describing a problem and then press go.
- 22:20 Because you’re using Python, that raises the possibility of wiring the application into a web server, and then you’ve got an API that serves off samples from the posterior distribution or the answers to statistical questions over a web API.
- 22:40 Your front-end can talk to your back-end, which is essentially a dialect of a general purpose programming language.
What are Stan’s strengths?
- 22:55 Stan has historically had the fastest implementations of all of these libraries, perhaps a little less true than it used to be.
- 23:00 Stan is academic code that is used in production.
- 23:05 It is used in the pharmaceutical industry in drug trials and environmental sciences in industrial applications.
- 23:15 In terms of the way most production software engineers think, it’s a slightly unusual language.
- 23:20 The documentation is voluminous as well as good.
- 23:25 The community around Stan is incredible; the mailing list is amazing.
- 23:30 If you have a PyMC3 question, and you can translate it to Stan, then ask it on the Stan mailing list and you’ll get a great response.
- 23:35 They are both worth checking out.
Is Stan used by Facebook?
- 23:45 Stan is a general purpose programming language; in a CS sense, it is Turing complete.
- 23:50 You wouldn’t use it to solve general problems.
- 24:00 It is very flexible; that flexibility is for some use-cases a disadvantage.
- 24:05 If you have a very specific problem you want to solve, and you want to make it accessible to data analysts or data scientists, you might encapsulate a Stan program with some plumbing to allow it to be used in languages like Python and R.
- 24:25 That’s what the Facebook data science team did when they created Prophet.
- 24:30 Prophet is a general time-series forecasting library.
- 24:35 You feed in a time series, and it predicts the future.
- 24:40 It captures any seasonality in your time-series; if you get more visitors to your website Monday through Friday than at the weekend, Stan is going to capture that.
- 24:45 It also captures seasonality at lower and higher levels, not just days of the week.
- 24:50 Crucially, its forecast incorporates that seasonality and incorporates uncertainty bounds on what can happen in the future that are implied by your data.
- 25:00 The more data that you have in your archive of data in general, the more confident your prediction about the future will be.
- 25:05 If you have a very short archive of data, Prophet will still make a prediction and won’t give up, but the prediction will be correspondingly uncertain.
- 25:20 Because of very good UX decisions about how the model talks to Python and R, this is a particularly accessible place for most people to start.
- 25:30 You can be up and running in four or five lines of code, and looking at real output of Bayesian inference; namely the posterior distribution, without necessarily opening up the (frankly messy) black box.
- 25:55 It’s a generic problem, and most of us have time-series data.
- 26:00 I’m a machine learning person with a background in time-series, and I find working with time-series pretty tricky.
- 26:05 There’s a reason why the ‘hello world’ of machine learning are classifying orchids and deaths on the Titanic – they are simple tabular data-sets.
- 26:20 Time-series are not like that – they can be arbitrarily long, have all sorts of long-range dependencies (periodic on different time-scales).
- 26:30 They can be a nightmare to analyse – but they are really common.
How do the data structures around Bayesian and probabilistic programming languages affect core programming languages? Where do you see them going?
- 27:30 The first is probabilistic data structures – like Bloom filters – being incorporated in the language.
- 27:45 If you’re interested in Bloom filters, check out Cuckoo filters – they are the new hotness.
- 27:50 You can delete things from Cuckoo filters, which is something that famously you can’t do in Bloom filters.
- 28:00 Analysis at the programming language level is a very useful affordance in the world we live in, where data is coming in at such a rate that you can’t store it to do batch analysis on it.
- 28:20 At the language level, you can imagine that becoming more and more useful.
- 28:25 The one place that I’ve seen Bayesian inference incorporated at the language level is an MIT project called BayesDb.
- 28:45 BayesDb is essentially a super-set of SQL which allows you to do selects and filters and all those sorts of SQL things.
- 28:50 The really nice thing is it allows you to impute missing data and select on a criteria which is missing, and the gaps are filled in in a probabilistic way.
- 29:00 It adds to SQL’s set of primitives an ‘infer’ statement which allows you to infer missing values, and under the hood it’s using Bayesian inference.
- 29:10 That code is public, and you could use it on the database side with missing data, and think how you could make potentially quite complicated algorithms to users; BayesDb is very interesting.
What is PyMC4 and how does it relate to Tensorflow?
- 30:00 PyMC3 uses torch, which isn’t going to see any further developments.
- 30:15 They have decided to switch their back-end over to a new back-end, Tensorflow probability, a Google project.
- 30:30 Tensorflow probability I don’t recommend you go out and install immediately; it’s a low-level set of tools that are geared towards probabilistic programming languages or very innovative research and analysis.
- 30:50 You can think of PyMC4 as a user-level implementation of probabilistic programming that uses Tensorflow at the back-end.
- 31:00 This is kind of similar when people ask me where to start with deep learning; I don’t recommend Tensorflow, because it is a relatively low-level library.
- 31:10 There are higher level libraries that abstract away some of the complexity and provide building blocks of neural networks that are perhaps more user-friendly.
- 31:20 Tensorflow probability is kind of the same deal; identify a library that sits on top of it rather than it directly, if this is the first time you are hearing about probabilistic programming.
- 31:40 If you already knew about everything here, go and check out Tensorflow probability – it is a very cool work.
Tensorflow is more of the brand name?
- 31:50 Yeah, if you go onto GitHub and go to the Tensorflow organisation, there are lots of packages (including the Tensorflow deep learning library).
- 32:00 Not all of these are immediately directly related to one another.
- 32:05 I think Tensorflow probability falls under an umbrella.
Mentioned
Fast Forward labs
PyMC3
Stan
PyTorch
BayesDB
TensorFlow
More about our podcasts
You can keep up-to-date with the podcasts via our RSS feed, and they are available via SoundCloud and iTunes. From this page you also have access to our recorded show notes. They all have clickable links that will take you directly to that part of the audio.
Previous podcasts
Google Releases App Engine Second Generation Runtime Supporting Python 3.7 and PHP 7.2 Support
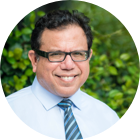
MMS • RSS
Article originally posted on InfoQ. Visit InfoQ
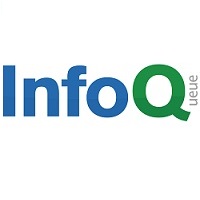
Google Cloud recently announced the release of its Second Generation App Engine standard runtimes, an upgrade to the web framework and cloud computing platform for building apps. This update allows users to run their web apps with up-to-date versions of popular languages, frameworks and libraries. Including the Python 3.7 and PHP 7.2 libraries.
During Cloud Next, Google announced that Python 3.7 and PHP 7.2 would be the ones of the new second generation runtimes it will support. Moreover, according to the announcement, the second generation runtimes including Python will remove many of the previous App Engine restrictions. Developers can now write portable web apps and microservices that take advantage of App Engine’s auto-scaling, built-in security and pay-per-use billing model.
Python 3.7 and PHP 7.2 are available in beta for the App Engine standard environment and also support the Google Cloud client libraries. Thus, developers can integrate GCP services into their app, and run it on App Engine. Note that Google is currently upgrading the App Engine APIs to make them accessible across all GCP platforms, and are not yet available in Second Generation runtimes, including Python 3.7 and PHP 7.2.
With the new runtimes in App, Engine developers can leverage Python 3 support for arbitrary third-party libraries, including those that rely on C code and native extensions. By adding libraries like Django 2.0, NumPy, scikit-learn or another library of choice to requirements.txt file, the App Engine will install them once the developer deploys the app. To deploy an app supporting PHP 7.2 developers need to download and install the Google Cloud SDK. Next, they can create app.yaml and index.php.
# app.yaml
runtime: php72
<?php
// index.php
echo 'Hello, World!';
Finally, they can deploy to the App Engine using the following command:
gcloud app deploy
Some Google App Engine customers are now using Python 3.7 on the App Engine standard. According to the announcement LumApps, a Paris-based provider of enterprise intranet software, has chosen App Engine to optimize for scale and developer productivity. Their CTO & co-founder, Elie Mélois, said:
With the new Python 3.7 runtime on App Engine standard, we were able to deploy our apps very quickly, using libraries that we wanted such as scikit. App Engine helped us scale our platform from zero to over 2.5M users, from three developers to 40—all this with only one DevOps person!
Developers can now write apps using Python 3.7 or PHP 7.2 on the App Engine standard environment and more details are available in the Google App Engine Python 3 Standard Environment documentation and Google App Engine PHP 7.2 Standard Environment documentation. Furthermore, Google will announce more App Engine releases soon.
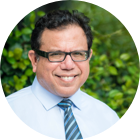
MMS • RSS
Article originally posted on Data Science Central. Visit Data Science Central
Hope you all enjoying all the banking services available at your fingertips. Imagine living in the same world without banks and their functions. What would be the best alternative for banking and financial systems?
Many business giants around the world proved Trust-based financial systems results in the gaining active user base. Later it helps your business regarding sales and trade.
If you have ever exchanged with your books with a friend in return for of their books, you have bartered. In the old civilization, people are exchanged goods and services without cash, there are no money Transactions involved.
Eleven thousand years ago people used to trade with other people within their Community who they knew and trusted. But the rapid growth of population its turn to become tough to trust everyone.
Now we can use technology for national and international financial transactions. At the same time, we are facing problems with the hackers, who are using advanced techniques to steal the money and information about people. So because of this kind of issues people feeling insecure about their transactions. To get rid of such kind of fraudulent acts Bitcoin was introduced which is used to Encrypt the currency into the digital format and which is highly secured. But it failed to gain the public trust and confidence. Recently a software was developed for financial and informational storage transactions which are advanced and secure, especially the information in it can not be altered or hacked by anyone. It is nothing but the “Blockchain”.
What Is A Blockchain?
To make a long story short, Blockchain is an evaluating software platform for digital assets. In simple terms, blockchain is a chain of blocks where each block is an incorruptible digital ledger of economic transactions. Blockchain technology implementation has no intermediate entities like a government or the bank controlling it.
In this technology systems are distributed with a collection of networks, which means all computers in the network stores the same information.
How Big Is Blockchain?
1> $ 430 Millions invested in startups in 2015.
2> $ 1.1 billions of Total investments in cryptocurrency related Technologies.
3> 42 systemically Essential for financial institutions and the part of R3-CEV fellowship to do research and development on blockchain for fintech.
4> $140 Million Increased in 9 blockchain investment rounds by investors like AXA strategic Ventures, such as a J.P Morgan Santander and Goldman into ventures.
5> 24% of infotech ventures are innovating in marketplace platforms, and 17% are learning backend innovation.
6>311k Tweets per 1 hr on the blockchain.
7>16 insurance start-ups are looking exclusively at P2P insurance.
8>4X YOY raised the number of blockchain startups.
People mistake blockchain to be used as an alternative to financial transactions. But it is not limited to payments, but the applications of Blockchain Spread every industry, like health, education, transportation, insurance industries and many more.
So, how exactly do blockchains figure into the different fields? Below are a few use cases:
Education
In fact, the most important factor in the educational sector is academic credentials must be universally identified and acknowledge. By nature in both the secondary and primary university and schooling environments, Tracking academic credentials by the manual is the big challenge for management. (burdened by cumbersome paperwork and manual processes).
Using blockchain technology in the education sector, we can reduce the unauthorized claims and unearned educational credits.
Banking Sector
We are following the traditional banking system for our financial transactions. In the conventional banking system, it takes a longer time to do a commercial operation and the cost is also high. Even though it takes extra time and cost, we cannot wholly rely on the banking system because of hackers. Banks are facing the cybersecurity issues. Due to cyber attacks, the SWIFT network of the banking sector has lost $ 100 million in recent years. With the usage of blockchain system in the financial industry can results in increased efficiency, more transparency, and decreased cost. The blockchain is highly secured when compared with traditional banking. Some of the prominent banks which have already joined the blockchain are Bank of America, Royal Bank of Canada, American Merrill Lynch, Banco Santander, Standard Chartered, UniCredit, and Westpac.
Insurance
The blockchain is ready to disrupt the insurance industry with its unique capabilities. In the traditional way of the insurance process, it has a long process, and it will take time to settle the matured claims.
Inefficiency and lack of trust in insurance industry lead to underinsurance. For instance, the likelihood of experiencing the earthquake is very high in California, but the percentage of insured is only 17%. Implementation of blockchain in the insurance industry can lead to drastic changes in the sharing of data, process claims and preventing fraud. It can create an innovative insurance industry. Blockchain can detect the fraudulent acts, and it can process the matured claims instantly. Blockchain implementation in insurance sector leads to gaining the people trust and thereby we can increase the sales.
Transportation Industry
Transportation industry plays a crucial role in supplying the goods and services from the area they are manufactured to the customer point. Due to an increased requirement for transportation, it is difficult to meet the needs of the customers. Now due to technological advancements, customers are given an option to get their ordered product in one hour or same day delivery. To meet such kind of customer requirements and sustain in the competitive industry, it is difficult with the traditional transportation system.
The over dependency on paperwork by the transportation industry resulted in a 20% increment in cost of shipment. Regularly transportation industry faces $140 billion tides up disputes for payments.
To overcome this issue, there is only one solution which is blockchain. Blockchain technology can help the transportation industry with the decentralized distributed system. The blockchain is a digitized shared network.
Through blockchain technology helps in reducing the paperwork and eliminating the middleman for faster delivery of goods and services. With the implementation of blockchain technology helps in speedier delivery, reduced time & cost and causes insufficient loss.
Final Thoughts
No one can have the right or ability to stop the technological advancements in every industry. Technological changes are inevitable. When it comes to blockchain technology, it is growing incredible. It has been entering every sector and showing its impact. We can say that every industry is going to have a brilliant and near experience with blockchain technology. Near future blockchain is going to have its footprint in every segment.
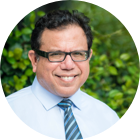
MMS • RSS
Article originally posted on InfoQ. Visit InfoQ
Key Takeaways
- Agile and Scrum frameworks are designed to help teams work together more efficiently. Make sure that everyone understands their roles and that strong leadership is in place to guide everyone through the process.
- Time spent together as a team is precious. Building rapport is crucial for a highly collaborative team environment. A good team needs a bit of down time to build those social bonds.
- Stick to a schedule, streamline the standup, and cover all of the most important topics in a timely manner.
- Identify your team’s strengths and weaknesses and find ways to use them as an advantage to make the team even stronger.
- Encourage communication by sharing knowledge and experience through localized databases.
- Trust your team and encourage self-management; don’t get caught up in controlling every aspect of the project.
Agile and Scrum have been buzzwords in the project management and development world for years now. These approaches have proven time and time again to be a key factor in not just how projects are completed, but how development teams become smarter and more efficient. The truth is that many teams have implemented Agile and Scrum frameworks because they work wonders and can help teams accomplish goals in an organized fashion.
If you are reading this post, you probably know or have an idea of what Agile and Scrum processes are. To reiterate, Agile refers to a group of development methodologies in which outcomes are the result of collaboration between self-organization and cross-functional teamwork.
Scrum is a process that falls under the umbrella of Agile. It is a set of practices that must be followed in a particular formula with certain roles of the team defined. There are three key roles in a Scrum team:
- Product Owner – The head stakeholder who conveys the overall vision and objectives of the project. The Product Owner is the one accountable for managing the backlog and accepting the completed increments of work.
- The ScrumMaster – Works directly with the Product Owner but holds no hierarchy over the rest of the team. The ScrumMaster is responsible for making sure the principles of the Scrum theory are carried out and the team is working at the highest efficiency.
- The Development Team – The self-organizing, cross-functional group producing the deliverables. This team includes anyone participating in the creation process of the product. There are no titles in the Development Team.
The series of 5 Scrum events involves:
- Sprint Planning
- Daily Scrum
- Sprint Review
- Sprint Retrospect
- The Sprint
According to last year’s Pulse of the Profession survey, conducted by PMI, nearly 71% of organizations report using Agile tactics within their organization. This can likely be attributed to the notion that Agile methods generally produce a disciplined process that thrives on in-depth examination, collaborative thinking, and constant refinement. While the concepts in the famous Agile Manifesto seem relatively straightforward, being at the helm and carrying out this leadership philosophy from A to Z is no simple task.
No matter what your Agile team looks like or how you plan to integrate these principles into your strategy, there is one factor that must remain constant: strong leadership. Taking the reigns as an Agile or Scrum leader is an important task. As you prepare to take charge, there are some key principles to keep in mind that will help to ensure everything goes as planned.
Let’s discuss.
1. Streamline the Meetings
When implementing the Scrum strategy to a team, one of the most important steps is hosting the daily Scrum event. According to the State of Agile report, 90% of Scrum teams hold these meetings every day, making it the most commonly implemented Agile framework.
These tend to be quick get-togethers (The Scrum Organization recommends a 15-minute limit) typically held first thing in the morning with the entire team present in order to talk about the day’s goals, next steps, and any issues that need to be addressed. Many teams call this the Daily Stand-up because they have found teams have a better focus and pay attention when they remain standing.
This meeting is a time to synchronize and draw up a battle plan for the next twenty-four hours. The Development team leads these meetings, so they are in charge of going over the goals for the day ahead and ensuring that everyone knows their roles, tasks, and objectives. Team members are encouraged to ask questions during this part of the meeting to make sure that they are all on the same page.
Now, that sounds like a fairly short list to accomplish, but these meetings can certainly run the risk of dragging out longer than necessary. As a leader in this regard, it is up to you to keep everyone focused on the task at hand. In order to do this, everyone must understand why each part of the meeting is important.
Apart from Daily Stand-ups, your team will also hold meetings once a Sprint is completed. This Sprint Review Meeting is important to keep your team on track and focused on the vision of the entire project. There are three key parts of the Spring Review Meeting which include:
- Retrospective: This is the time that teams should reflect on what worked well during the last Sprint and what did not.
- Battle Plan: Teams should decide what needs to happen next during the upcoming Sprint and delegate tasks appropriately.
- Solution Stage: This is the time for teams to share their ideas for solving recurring issues that happened during the last Sprint. Teams are encouraged to talk about any problems they ran into and share their ideas for resolution.
Now, that’s a lot to accomplish, which is why these Sprint Review Meetings can certainly run long if leaders don’t keep the team in check and on task. By understanding why each part of the meeting is held and what needs to happen during those times can help.
First of all, the retrospective period is essential. Unfortunately, many teams skip over this step or spend far too little time reflecting on the past Sprint, which weakens the influence it can have on a team’s success. Even a mediocre retrospective can have an adverse effect and easily turn into a time of complaining and making excuses for lazy behavior. Instead, this time should be an open but professional conversation regarding struggles or obstacles. This is not a time to point blame; instead the goal should be to get to the root of the problem. Ask the team if there were any communication issues, misunderstandings, or unexpected changes. By shifting the perspective and looking at the core issues, the retrospective aspect can be strengthened and become far more effective.
Secondly, the battle plan stage must be straightforward and to the point. As a general rule, teams should have a Scrum board that organizes projects into small steps that need to be accomplished in a certain order. Whether this is a physical board or an online one is up to the team’s preferences, but some visual representation is certainly necessary. Be sure that everyone is looking at the board to see which tasks are moved to the next phase.
Finally, be sure to focus on the solution stage, but don’t drag it out. Since you’ve already determined the core of the issues during the retrospective, the solutions should hopefully be clear and easy to find. Moreover, there should be actionable ways to implement them. Rather than say “we need to communicate better”, an actionable solution would be, “meet up with your team leader once a day to make sure you’re on track and any questions are answered’.
While it is certainly tempting to make the daily meeting a time to chat around the water cooler, it is important to stick to a plan and make the most of your team’s time. Try to keep small talk to a minimum, cover these three important steps, and streamline the process as much as possible.
2. Be Upfront about the Strengths and Weaknesses of the Team
One of the key principles of the Agile framework is to work as a team and find systems that make everyone more successful. Chances are, a great team won’t come together by coincidence; teams are made up of individuals who have their own strengths, weaknesses, and personalities. The key to creating a strong Scrum team is to be honest and upfront about these qualities rather than skirting around the issue(s).
Creating an environment of psychological safety is imperative for a high-performing team. Google conducted a two-year long study on team collaboration and found that when individuals felt that they could share their honest opinions without fear of backlash, they performed far better. When employees feel that their opinions matter, their engagement levels peak and, according to Gallup’s research, their productivity increases by an average of 12%.
But unfortunately, this doesn’t always happen, especially when teams are new to Agile and Scrum. The Agile Report found that one of the top challenges reported while adopting an Agile approach was its alignment to cultural philosophy, along with lack of leadership support and troubles with collaboration. All of these issues are related to people’s personalities, including their strong points and areas of weakness. If a strong leader is put in place and a strategy is designed to work to each member’s strength, many of these problems can diminish.
[Click on the image to enlarge it]
First of all, you must choose your leadership wisely. For instance, the Scrum Master is often chosen because they are a person who understands the principles and Agile Manifesto so they are able to keep the team accountable. Due to Scrum teams being self-organized and self-managed, they should appoint the Scrum Master together. This person must be a good leader, but they also need to know their place. Scrum Masters have no more authority than anyone on the team, and they are often referred to as a servant leader who is there to help the rest of the team and provide support whenever needed. Therefore, the person who fills this role must be able to communicate the principles and keep everyone in check without overstepping. It is also interesting to note that the Scrum Master is not a concrete role; it is fulfilled by the person who is best equipped for the job during a specific point in time. As things change, the person who would be best for this important role may as well.
When appointing leadership among the team, it may be helpful to think of it as a job interview. Look at their past performance as a resume. Will their strengths support the team or will their weaknesses stand in the way of success?
Secondly, facilitate open conversations within the team about these areas. Compliment and comment on jobs well done; don’t berate people for their weaknesses, but help them find ways to improve and develop their skills.
There’s no need to keep people’s strengths and weaknesses a secret. Instead, by being honest and straightforward about them, it will be easier to identify ways to use them for the team’s advantage.
3. Leverage the Shared Pool of Knowledge
The goal of Agile is to create an environment where a team can work together seamlessly. There are four core values of the Agile Manifesto:
- To value individuals and interactions over processes and tools.
- To value working software over comprehensive documentation.
- To value customer collaboration over contract negotiation.
- To value responding to change over following a plan.
In other words, an Agile team must value each other and interactions with the entire stakeholder community over sticking to a strict schedule and getting so wrapped up in minute details that they lose sight of the bigger picture.
In order to stick to these values and ensure that the job is done correctly and efficiently, there must be systems in place to create a network of shared information. For some teams, an Excel spreadsheet may be enough; for others, a white board can work. And for others, a virtual dashboard might be necessary for organization.
Since 71% of all organizations use Agile approaches for team projects (at least occasionally), there are plenty of online resources available to help your team leverage their pool of knowledge. Using a project management system that is designed for Scrum teams specifically can be quite useful for keeping documents and information located in a single program for easy access. These tools often include virtual Scrum boards for task assignments to make sure everybody on the team is on track to hit their deadlines. In fact, one report found that teams who utilized project management software reported improvements in team communication, as well as the overall quality of their end product.
Of course, not all of this knowledge will be virtual. Each person on the team brings their own set of skills and experience to the table; be sure to utilize their gifts during team meetings by listening to their opinions and insight. Always encourage communication, specifically face-to-face (another Agile principle), to share this vast resource of personal knowledge.
4. Add a Buffer Zone (Avoid Micromanaging)
According to the Agile Manifesto, there are several key principles that teams must hold to in order for success. As the Manifesto puts it:
Business people and developers must work together daily throughout the project. Build projects around motivated individuals. Give them the environment and support they need and trust them to get the job done.
Clearly, this principle leaves no room for micromanagement. In fact, the point of a Scrum team is that it is self-sufficient and self-managing. While a team leader is appointed to ensure that deadlines are met and a Scrum Master is chosen to make sure that Agile principles are followed, teams should be fairly independent.
Of course, this may be quite difficult for a leader to accept, especially if there is little trust between team members.
The Agile approach offers a safe balance between micro-management and self-sufficiency. In some ways, Agile has some micro-management influence. For example, hosting daily meetings to go over what everyone did and what is next on the to-do list might seem like overkill for a usual team project. For Scrum teams, this is highly encouraged. However, the bulk of what defines Agile revolves around self-governing and holding team members accountable for what they do throughout a project.
The goal of Agile is to create self-organized teams who are able to manage themselves. Therefore, management should be far more focused on leadership and guidance than telling the team what to do.
If you find that it is difficult for you as a leader to find this balance, it may be helpful for you to look into Scrum certification. There are many online certification courses available that will help you to understand the practical application of Agile and Scrum principles – so you are able to integrate them more effectively. In fact, 81% of people who received Scrum certification reported that it significantly improved their practices.
Scrum teams must be built on a foundation of trust and understanding. A micromanager is not typically able to trust their team members, but a true leader must be able to. This trust may need to be built over time but increasing your knowledge and experience with Scrum and Agile can help you gain more confidence and help you better guide your team.
Conclusion
Taking charge as a leader in an Agile project with a Scrum team is an important undertaking. While the goal is to establish a self-managing, independent Scrum team, leadership is still necessary (especially at first) to ensure that the team is able to stick to Agile principles and get the project accomplished. By setting these goals and following these guidelines, you can ensure that your development team will be able to reach its fullest potential.
About the Author
Manish Dudharejia is the President and Founder of E2M Solutions Inc, a San Diego Based Digital Agency that specializes in Website Design & Development and eCommerce SEO. With over 10 years of experience in the Technology and Digital Marketing industry, Manish is passionate about helping online businesses to take their branding to the next level.
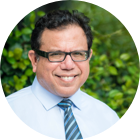
MMS • RSS
Article originally posted on InfoQ. Visit InfoQ
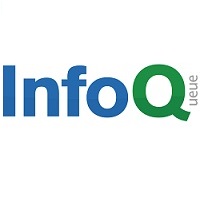
Hazelcast, makers of open-source developer-focused infrastructure components, including an in-memory data grid (IMDG) and a stream processing engine (Hazelcast Jet) have been through a management change.
The previous CEO, Greg Luck, moves to become Chief Technology Officer (a position he previously held) and member of the board. The incoming CEO is Kelly Herrell, a Silicon Valley veteran who was most recently Senior Vice President and General Manager of Brocade Communications, as a result of Brocade’s acquisition of Vyatta where Herrell was Chief Executive Officer.
InfoQ: Why the change in management right now?
Herrell: With Hazelcast doing very well with years of on-target growth performance and getting to 100 people, and Greg working partly from Australia, he decided that rather than migrate permanently to the US, it would be better personally to stay in Australia and replace himself with a Palo Alto based Growth CEO.
InfoQ: Is this purely a board level change, or are there other changes that will affect the rest of the organisation?
Herrell: Greg was a director by virtue of being CEO. With him stepping down, the board thought it was vital to keep his voice on the board for a smooth transition. So, an additional board seat was made available for him.
InfoQ: Vyatta produce software firewalls and appliances routers – the developer-led tools and infrastructure market is a little different. What are the main differences as you see them, and what from your background are you bringing to bear in this new market?
Herrell: Though the markets are different, there is strong similarity in how the products are adopted. Vyatta was unique in its origin as an open source networking offering, and adoption was initiated through frictionless access of a free download by a massive community of highly technical people worldwide, who also needed access to tools that helped them enable innovative solutions.
In addition, what’s incredibly common is, like Vyatta, Hazelcast is driving the front end of a powerful wave of innovation. New technologies and new solutions are coming together to solve new critical business challenges. I’ve built my career on highly innovative and disruptive forces that drive major industry changes; it’s a different skill-set compared to running a status-quo business. Being open-minded, sensing different patterns, and seeing around corners is key to staying on top of a rapidly-changing market.
InfoQ: Will this transition mark a change in technical direction? Has the strategy around open source changed at all? What is the expected impact on customers and users of Hazelcast’s products?
Herrell: There will be no change to our technical direction or open source commitment. Hazelcast IMDG is the market leading in-memory data grid. Hazelcast Jet is our new open source stream processing engine. Kelly has a lot of experience in scaling companies. At a high level, the only thing that will change is Hazelcast will move faster and become more strategically relevant to more customers worldwide.
Giving Greg the ability as CTO to focus on leading product development with Engineering and Product Management is a big part of what will continue to drive our product and technology leadership and keep differentiating ourselves from others. Customers and our open source community will both be very happy with the faster pace of innovation they see coming out of Hazelcast.
InfoQ: Let’s talk about the technology a bit. What’s the plan for IMDG?
Herrell: Hazelcast IMDG has been going very well. It is the fastest and simplest IMDG. In recent years we have added native Nodes.js and Go clients. Cloud wise we support all the clouds. So, the plan is to continue to invest. One exciting development is that in the next release we will be adding a CP Subsystem. That is CP as in CAP. We will add new services which need this contract such as Directory (think Zookeeper) as well as migrating our Atomic structures to CP. The CP subsystem will use RAFT which we have already implemented.
We are also planning on introducing new data structures, enhancing query with SQL Select and JDBC, and adding native JSON support for document-oriented systems. So more than ever to do and busy as ever.
InfoQ: The other major Hazelcast product is Jet. How satisfied are you with the traction that you’re seeing for Jet? The 0.6 release was a fairly big step forward in terms of the maturity of the product, so what is the roadmap from here?
Herrell: 0.6 was like throwing a light switch. With 0.6, we added our Pipelines API (think an enhanced java.util.streams). Suddenly our solution architects got it. We are seeing huge interest from within our Hazelcast community, which is our logical starting point with Jet.
0.7 adds a new Management and Monitoring capability, more connectors and finishes off the Pipelines API. We expect the next release after 0.7, due at the end of the year, to be 1.0 and at that point we will have stable APIs.
InfoQ: Hazelcast have helped to grow the market for open source IMDG in the face of some excellent competitors. Do you see the stream processing market as similar? For developers, what differentiates Jet from the rest of the market?
Herrell: IMDG had great success for two mains reasons: it was the first open source IMDG and it had a really intuitive and simple API, so much so that developers could get going in just 15 minutes.
Jet is a more complex domain. We have tried and discarded three APIs at this point before finally developing the Pipeline API which we think resonates very well with Java 8 developers. We have made it work very well and very fast with IMDG as a source and sink.
So, if you are a Java developer, and you have your data in IMDG or want to put it there, Jet will be your easiest and fastest solution.
InfoQ: Any final comments for our readers?
Herrell: The digitization of everything is creating immense amounts of data quickly, and there is huge business value in leveraging that. We really encourage developers to think strategically about the right infrastructure for their needs – not just the immediate need they’re solving for, but also for the scale and performance they will need going forward.
The book Future Shock describes “the dizzying disorientation caused by the premature arrival of the future.” The world is changing fast; we all need to make decisions that simultaneously solve for the immediate need but anticipate the growing requirements around the corner.
Current production releases of Hazelcast IMDG and Hazelcast Jet are available from https://hazelcast.org/
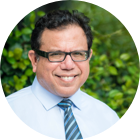
MMS • RSS
Article originally posted on Data Science Central. Visit Data Science Central
Yes, I know, this has been tried a few times and no one listens…. At least not yet. Despite several studies showing otherwise, teams still punt more than they should. Admittedly, some of these studies have been less than rigorous, and often times, assumptions are made that warrant scrutiny (assuming a 50% success rate on all 4th down attempts for example). But I don’t think it is the lack of scientific rigor that keeps change at bay. I think the failure to adopt a novel strategy has a lot more to do with the comfort that accompanies doing whatever is generally accepted as “the way to do it.”
If a coach fails doing it the same way everyone else does it, he has cover. If a coach adopts a novel strategy, even if he fails less, he will be judged harshly for those failures (more so than for failing the “right way”). It’s not until somebody comes along who has the courage to implement a novel approach AND the patience and support to allow that approach time to succeed, can the “way to do it” change.
Having said all of that, I am giving this punting thing another go. Maybe the right person is listening, but probably not. Personally, I don’t care. I love analytics in part as a way of changing minds, but mostly just to satisfy my own curiosity about things.
You can find my work here. I outline why my approach differs from others in the article, but probably the biggest difference is the way I expanded my data-set to incorporate “do-or-die” third downs as an analog for 4th down. If you are an NFL coach, please read on. If you are just curious like me, I hope you find my work interesting.
How the incorporation of prior information can accelerate the speed at which neural networks learn while simultaneously increasing accuracy
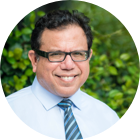
MMS • RSS
Article originally posted on Data Science Central. Visit Data Science Central
Deep neural nets typically operate on “raw data” of some kind, such as images, text, time series, etc., without the benefit of “derived” features. The idea is that because of their flexibility, neural networks can learn the features relevant to the problem at hand, be it a classification problem or an estimation problem. Whether derived or learned, features are important. The challenge is in determining how one might use what one learned from the features in future work (staying inside the same typology, e.g. images, text, etc.).
By eliminating the need to relearn these features one can increase the performance (speed and accuracy) of a neural networks.
In this post, we describe how these ideas are used to speed up the learning process in image datasets while simultaneously improving accuracy. This work is a continuation of our collaboration with Rickard Brüel Gabrielsson
As we recall from our first and second installments we can, discover how convolutional neural nets learn and how to use that information to improve performance while allowing for the generalization of those techniques between data sets of the same type.
These findings drew from a previous paper where we analyzed a high density set of 3×3 image patches in a large database of images. That paper identified the patches visible in Figure 1 below and allowed them to be described algebraically. This permits the creation of features (for more detail, see the technical note at the end). What was found in the paper was that at a given density threshold (e.g. the top 10% densest patches) those patches were organized around a circle. The organization is summarized by the following image.
Figure 1: Patches of Features Organized Around a Circle
While the initial finding was via the study of a data set of patches, we have confirmed the finding through the study of a space of weight vectors in a neural network trained on data sets of images of hand-drawn digits. Each point on the circle is specified by an angle θ, and there is a corresponding patch Pθ.
What this allows us to do is to create a feature that is very simple to compute using trigonometric functions, that for each angle θ that measures the similarity of the given patch to Pθ. See the technical note at the end for a precise definition. By adjoining these features to two distinct data sets, and treating them as separate data channels, we have obtained improved training results for the two distinct data sets, one MNIST and the other SVHN. We’ll call this approach the boosted method. We are using a simple two layer convolutional neural net in both cases. The results are as follows.
MNIST
Validation Accuracy |
# Batch iterations Boosted |
# Batch iterations standard |
.8 |
187 |
293 |
.9 |
378 |
731 |
.95 |
1046 |
2052 |
.96 |
1499 |
2974 |
.97 |
2398 |
4528 |
.98 |
5516 |
8802 |
.985 |
9584 |
16722 |
SVHN
Validation Accuracy |
# Batch iterations Boosted |
# Batch iterations standard |
.25 |
303 |
1148 |
.5 |
745 |
2464 |
.75 |
1655 |
5866 |
.8 |
2534 |
8727 |
.83 |
4782 |
13067 |
.84 |
6312 |
15624 |
.85 |
8426 |
21009 |
The application to MNIST gives a rough speedup of a little under 2X. For the more complicated SVHN, we obtain improvement by a rough factor of 3.5X until hitting the .8 threshold, and lowering to 2.5-3X thereafter. An examination of the graphs of validation accuracy vs. number of batch iterations suggests, plotted to 30,000 iterations, that at the higher accuracy ranges, the standard method may never attain the results of the boosted method.
These findings suggest that one should always use these features for image data sets in order to speed up learning. The results in our earlier post also suggest that they contribute to improved generalization capability from one data set to another.
These results are only a beginning. There are more complex models of spaces of high density patches in natural images, which can generate richer sets of features, and which one would expect to enable improvement, particularly when used in higher layers.
In our next post, we will describe a methodology for constructing neural net architectures especially adapted to data sets of fixed structures, or adapted to individual data sets based on information concerning their sets of features. By using a set of features equipped with a geometry or distance function, we will show how that information can be used to inform the development of architectures for various applications of deep neural nets.
Technical Note
As stated above, each point on the circle is specified by an angle θ, and there is a corresponding patch Pθ, which is in turn a discretization of a real valued function ƒθ on the unit square of the form
(x,y) —–> xcos (θ ) + ysin (θ )
By forming the scalar product of a patch (which is a 9-vector of values, one for each pixel) with the 9-vector of values obtained by evaluating ƒθ on the 9 grid points, we obtain a number, which is the feature assigned to the angle θ. By selecting down to a finite discrete set of angles, equally spaced, we get a finite set of features we can work with.