Month: August 2019
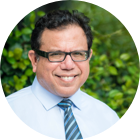
MMS • RSS
Article originally posted on InfoQ. Visit InfoQ
Working remotely is becoming routine: more than two-thirds of knowledge workers around the world work away from the office at least once every week. But in many organisations, more remote work has led to disconnection, isolation, and an erosion of cultures that supported creative collaboration and disruptive innovation.
Remote meetings are the most important way for teams to stay connected. They can be far more useful than text-based, asynchronous systems when it comes to challenging conversations such as troubleshooting, giving difficult feedback, or working together to develop great ideas.
But while remote meeting technology is steadily improving, outdated remote meeting practices aren’t keeping pace with changes in the way teams work. For example, rigid agendas and command-style chairmanship, near-obsolete when it comes to in-person meetings, are still common online.
In this series we’ll look at how teams worldwide are successfully facilitating complex conversations, remotely. And we’ll share practical steps that you can take, right now, to upgrade the remote conversations that fill your working days.
Free On-line Meeting
Supporting the series will be a free online meeting taking place on October 1st. This interactive learning session will help you learn proven practices for leading remote teams and running effective meetings.
Series Contents
2
Great Global Meetings: Navigating Cultural Differences
Navigating cross-cultural differences can be hard enough when team members are face-to-face, but when most communications are through some kind of technology—email, phone, IM, video, or online conferencing—it becomes infinitely more complex.
Future Articles
3
Can Your Meeting Kit Cut It?
Every team meets. Most run their meetings the same way their grandparents and their grandparents’ grandparents did. Meeting records predating the Romans describe a leader pontificating, brief back-and-forth discussion, then a conclusion with an inconclusive bit of mumbled agreement. Meetings haven’t changed much in thousands of years.
4
Remote Meetings Reflect Distributed Team Culture
Are you having problems connecting with people in your distributed meetings? Do you feel like you and your remote colleagues don’t meet goals in your meetings? The problem may not be with the meetings. It might be the culture in which you run your meetings.
5
Strengthen Distributed Teams with Social Conversations
Yes, online meetings should result in outcomes; they should follow agendas or meeting plans and should be run efficiently. But, there is an aspect of remote team meetings that often gets overlooked—the opportunity to strengthen relationships with our team members.
Series Manager
Judy Rees is a facilitator, consultant, and trainer, mostly working online. As a former journalist and editor she’s been working in—and leading—distributed teams since before there was an internet! For more than ten years she’s been facilitating and training online, over phone conference at first before video came along. Her current live online courses include Remote Agile Facilitator for Adventures With Agile, and Facilitating Exceptional Remote Learning for ICAgile. She’s the co-author of a recorded online mini-course Engaging Distant Participants. Her weekly linkletter connects more than 3,500 readers to resources around remote work, facilitation, Clean Language and more. Learn more at judyrees.co.uk
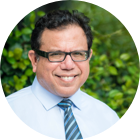
MMS • RSS
Article originally posted on Data Science Central. Visit Data Science Central
This article was written by Moses Olafenwa.
One of the important fields of Artificial Intelligence is Computer Vision. Computer Vision is the science of computers and software systems that can recognize and understand images and scenes. Computer Vision is also composed of various aspects such as image recognition, object detection, image generation, image super-resolution and more. Object detection is probably the most profound aspect of computer vision due the number practical use cases. In this tutorial, I will briefly introduce the concept of modern object detection, challenges faced by software developers, the solution my team has provided as well as code tutorials to perform high performance object detection.
Object detection refers to the capability of computer and software systems to locate objects in an image/scene and identify each object. Object detection has been widely used for face detection, vehicle detection, pedestrian counting, web images, security systems and driverless cars. There are many ways object detection can be used as well in many fields of practice. Like every other computer technology, a wide range of creative and amazing uses of object detection will definitely come from the efforts of computer programmers and software developers.
Getting to use modern object detection methods in applications and systems, as well as building new applications based on these methods is not a straight forward task. Early implementations of object detection involved the use of classical algorithms, like the ones supported in OpenCV, the popular computer vision library. However, these classical algorithms could not achieve enough performance to work under different conditions.
Source: here
The breakthrough and rapid adoption of deep learning in 2012 brought into existence modern and highly accurate object detection algorithms and methods such as R-CNN, Fast-RCNN, Faster-RCNN, RetinaNet and fast yet highly accurate ones like SSD and YOLO. Using these methods and algorithms, based on deep learning which is also based on machine learning require lots of mathematical and deep learning frameworks understanding. There are millions of expert computer programmers and software developers that want to integrate and create new products that uses object detection. But this technology is kept out of their reach due to the extra and complicated path to understanding and making practical use of it.
My team realized this problem months ago, which is why I and John Olafenwa built ImageAI, a python library that lets programmers and software developers easily integrate state-of-the-art computer vision technologies into their existing and new applications, using just few lines of code.
To read the rest of the article, click here.
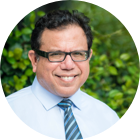
MMS • RSS
Article originally posted on InfoQ. Visit InfoQ
npm, Inc., the company behind the popular eponymous JavaScript package manager, will no longer allow packages which display ads. Developers will be able to silence terminal messages which push ads or calls for donations, and which stem from the regular use of the npm
command line interface.
Ahmad Nassri, CTO for npm, Inc, detailed in an email to ZDnet upcoming changes in npm policies:
[…] we’re making updates to our policies to be more explicit about the type of commercial content we do deem not acceptable.
ZDnet details in an article the packages which will no longer be allowed:
- Packages that display ads at runtime, on installation, or at other stages of the software development lifecycle, such as via npm scripts.
- Packages with code that can be used to display ads are fine. Packages that themselves display ads are not.
- Packages that themselves function primarily as ads, with only placeholder or negligible code, data, and other technical content.
The policy changes come after Standard, a JavaScript style guide, linter, and formatter, experimented with funding
, a npm package which installs open source software, and displays a message from a supporting company. Feross Aboukhadijeh, the maintainer of both Standard and Funding, together with 100+ packages on npm, shows an example of such messages:
Aboukhadijeh took pain to explain in detail the motivations behind the experiment and its self-imposed limitations:
The current model of sustaining open source is not working. We desperately need more experimentation. This is one such experiment.
[…] The goal is to make sure that packages are well-maintained now and for the foreseeable future, with regular releases, improved reliability, and timely security patches. Healthy open source packages benefit users and maintainers alike.
[…] There is no tracking or data collecting — and it will always stay this way. You can look at the code to verify – indeed, this is the beauty of open source!
[…] you can permanently silencefunding
by adding an environment variableOPEN_SOURCE_CONTRIBUTOR=true
to your terminal environment.
[…]funding
also respects npm’sloglevel
setting, so e.g.npm install --silent
andnpm install --quiet
will be respected.
the community of JavaScript developers received the experiment with mixed feelings. Some developers quickly published packages (like no-cli-ads
, or npm-adblock
) to block command line interface ads.
Other developers, among which open source maintainers and open source contributors, emphasize the right for package authors to ask users to support their projects, outline the fact that the free nature of such software does not entitle users to dictate policy, and point at a long list of packages displaying donation messages.
Developers generally seem to be in agreement about the necessity to find a path for sustainable funding for open-source projects, while disagreeing on the path towards such goal. As one developer mentions:
[ad funding] is not the perfect end solution, but that’s not the point – it is about moving the conversation about how to build healthy relationships between our commons and companies forward.
Aboukhadijeh decided to cancel the funding experiment and shared his thoughts on the lessons learnt and possible ways forward to allow open-source software to capture a larger part of the value they create.
Can an ethical and algorithmically transparent cloud kitchen prevent future Amazon fires?
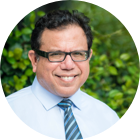
MMS • RSS
Article originally posted on Data Science Central. Visit Data Science Central
Can an ethical and algorithmically transparent cloud kitchen prevent future Amazon fires?
We often view AI with suspicion – but AI can be used to solve complex problems currently facing society where innovative approaches are needed
For many of us, the Amazon fires are disturbing and a serious problem because the Amazon cannot be recovered once its gone.
It seems that there is nothing we can do to mitigate this man-made (and economically driven) disaster.
However, I believe that in the very near future we can
And the solution maybe to create a spirit of activism through transparent algorithms to bring about social change
Two technologies could be key – and they are both currently viewed with some suspicion
Algorithmic transparency is viewed with concern i.e. regulators are forcing us to create transparent algorithms. However, transparent algorithms could lead to a competitive advantage as I explain below
The second technology is Cloud Kitchens, The VC Michael Moritz recently spoke of the disruptive nature of Cloud Kitchens and it’s negative connotations on the restaurant industry.
However, a combination of Cloud kitchens and algorithmic transparency (coupled with blockchain) could provide a complete end-to-end transparency across the entire food chain
All these components exist but we just need an ‘ethical and algorithmically transparent’ cloud kitchen to put it all together.
A cloud kitchen is a restaurant with no dine-in facility i.e. serving customers through Cloud providers like Deliveroo, Just-Eat etc. In that sense, it has a business model based on disrupting existing restaurants.
But a cloud kitchen driven by values could be extremely disruptive
And those values are likely to be those of urban, younger, socially and environmentally conscious customers
Informed by transparent algorithms, they will take value-based decisions – favourable to the environment
With a single swipe of an app – they can include / remove a specific component in the food value chain (for example a farm responsible for clearing forests in the amazon) – making the food value chain transparent, accountable and values-based.
This idea could extend to other things that customers value – for example – the ethical treatment of animals
We thus remove the commercial incentive to damage the environment and encourage a values-based culture
The idea is not far-fetched
Millennials are dramatically changing food logistics driven by the environment – such as rejecting canned food and excessive packaging
Vegan food has become the UK’s fastest growing takeaway
Through ethical and algorithmically transparent cloud kitchen, we are just making explicit – what is already a trend
The views expressed in this article are personal and not affiliated to any organization I am associated with.
Image source: BBC
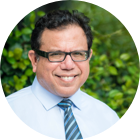
MMS • RSS
Article originally posted on InfoQ. Visit InfoQ
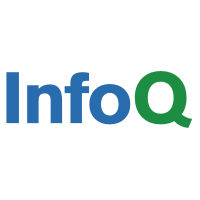
Etsy recently published a blog post detailing how they store and manage structured data. Etsy is a marketplace allowing sellers to post one-of-a-kind items. Their landing page slogan “If it’s handcrafted, vintage, custom or unique, it’s on Etsy.” reveals that uniqueness is a selling point for Etsy. As long as an item falls within the three broad categories of craft supplies, handmade, and vintage, it can be listed in the marketplace.
Etsy’s organisation of structured data, defines a taxonomy comprised of categories, attributes, values and scales. For example, an item in the taxonomy can have category “boots”, attribute “women’s shoe size”, with value “7” and scale “United Kingdom”. Etsy’s taxonomy contains over 6,000 categories, 400 attributes, 3,500 values and 90 scales. All these hierarchies combine together to form over 3,500 filters. JSON files are used to represent the taxonomy. With one json file per category, the file contains information about the relative placing of the category in the hierarchical tree and attributes, values and scales for items in this category.
The taxonomy is used in two cases. For the seller, it is used to categorise the listing at the time of creation. When a seller is creating a new listing, they can choose its category from within the taxonomy. Using a smart auto-complete suggestion textfield, they can select the most appropriate category. Based on the category selection, json provides information about attributes, value ranges and scales (e.g. “women’s shoe size”, “2…10”, “United Kingdom”). This reduces the need for overloaded product descriptions, simplifies the process for sellers and guarantees that Etsy collects just the relevant attributes for each category.
On the other hand, the taxonomy is used by the buyer to allow users to search by category & subcategory. Every category has its own distinct filters that are defined by the taxonomy. Any search query gets classified to a taxonomy category via a big data job which then gets displayed to the user along with the category’s filters.
The next steps for Etsy revolve around structuring unstructured data like listing titles and descriptions. Also, Etsy can use Machine Learning to discover more information about a listing. An example could be inferring the colour of a listing by the submitted image rather than asking the seller. The goal for Etsy is to use structured data to power deeper category-specific experiences, thus creating a better connection between buyers and sellers.
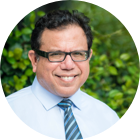
MMS • RSS
Article originally posted on InfoQ. Visit InfoQ
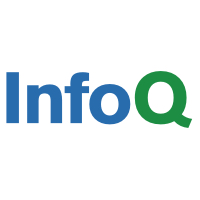
Recently, Microsoft released an update on the current adoption of .NET Standard by the development community.
The .NET Standard is a formal specification of the APIs that are common across the existing .NET implementations for different platforms (thus allowing cross-platform development). The current specification (version 2.0) was released two years ago, supported in .NET Core 2.0 and .NET Framework 4.6.1 platforms (among others). According to Immo Landwerth, a program manager on the .NET team at Microsoft and contributor to the Standard:
.NET Standard is for sharing code. .NET Standard is a set of APIs that all .NET implementations must provide to conform to the standard. This unifies the .NET implementations and prevents future fragmentation. It replaces Portable Class Libraries (PCLs) as the tool for building .NET libraries that work everywhere.
Using the Standard allows a developer to create .NET libraries that can be consumed across the different .NET implementations. These libraries can also be distributed and consumed as NuGet packages. Cross-platform development with .NET Standards 2.0 is supported by Visual Studio since version 15.3.
The current adoption to .NET Standard was measured by data collected from nuget.org, considering (i) the top one thousand packages (ranked by the number of downloads) and (ii) all packages available at nuget.org. As of July 2019, .NET Standard was supported by 47% of the top one thousand packages and by 30% of all packages. The adoption rate has been measured since the release of the 2.0 specification of .NET Standard. A linear extrapolation of the adoption rate growth since them provides an estimate of 100% adoption by around 2022 – with a strong remark about the fact that this is a trendline prediction.
As part of its .NET library guidance, Microsoft recommends that all developers target .NET Standard:
With few exceptions, all libraries should be targeting .NET Standard. Exceptions include UI-only libraries (e.g. a WinForms control) or libraries that are just as building blocks inside of a single application.
.NET Standard is currently being updated to version 2.1, which will include support to newer versions of .NET implementations (including .NET Core 3.0, scheduled to be released next month). However, Microsoft states that all libraries should keep targeting the 2.0 specification, especially considering that .NET Framework 4.8 will not implement the 2.1 specification.
More information about .NET Standard can be found here. Also, Immo Landwerth keeps a curated list of short videos on YouTube showing how .NET Standard works, and how it can be used by developers.
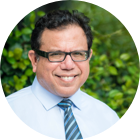
MMS • RSS
Article originally posted on InfoQ. Visit InfoQ
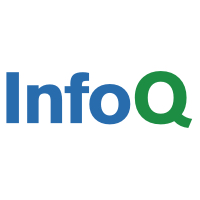
During a period in which Steven Lemon and his team had less features to implement, the technical leadership at the company he was working for decided to move their existing monolith into a microservices architecture. After a month of preparation, they realized that microservices would be hurting their development process without bringing any benefits. This surprised them but they decided to stay with the monolith, and Lemon recently wrote a case study of their findings.
Their application was working on top of an external third-party product with a set of back end services in between handling transformations between the two parts. An important reason for their difficulties in moving to microservices was that there was a mismatch between the domains in their own application and the domains in the external product. This mismatch had an impact on how their domain could be split into microservices. Letting their microservices follow the domains in the external product would cause duplication of logic across the microservices. With the microservices following their own domains, they would have to communicate with two or more domains in the third-party product. Both ways seemed for the team as a violation of microservices guidelines and leading to an increased coupling.
When they started looking into ways of splitting the monolith, they couldn’t find any obvious microservice candidates. They therefore started to arbitrarily split their domain models, which created a list of potential microservices but also a lot of shared business logic and coupling. They tried to mitigate this by dividing their services into even smaller parts, but that just increased the problems.
Lemon notes though that the main reason for failing to divide the monolith was that it only dealt with one single business concern. It was from the beginning designed to bring together disparate concepts from the external product, something they had been working on for years. They had underestimated the importance of finding the right boundaries, with the result that each feature in the application would be touching more than one microservice. In the end, this prevented any microservice from being owned by one team.
Another problem was that they didn’t have a platform ready for running microservices, and this complicated the communication between services. Their solution was to duplicate shared logic and common reads from storage, which removed the need for communication but created a significant amount of duplication.
They were also facing frequent requirement changes, making it hard to find the proper domain separations. What was fine one day could be overthrown a few months later, requiring another set of microservices. They also had a fairly short time frame, preventing them from reflecting over the design choices made, and the team lacked experience from previous work implementing a microservices architecture.
As the problems piled up, they started to realize that they didn’t know why they were trying to migrate to microservices. They lacked a list of pain points and didn’t understand how all the work would mitigate the pain points they did know about. Realizing this, they paused and looked into what benefits microservices commonly provide and which of these that would actually be beneficial to them.
With the architecture they already had and because of the compromises they would have to make in a move to microservices, they would end up with a pool of microservices shared by all teams and features spread over multiple microservices. This was a major disadvantage which prevented many of the common benefits with microservices.
Lemon also points out that there were a lot of infrastructure concerns already solved in their monolith that would have to be addressed again. When looking at their monolith, he notes that they had very few issues. It was straightforward to work with and had a CI/CD setup simplifying deploy and rollback. Their branching and testing strategies ensured that very few issues made it into production.
In the end, they realized that a monolith isn’t automatically something bad and microservices always something good. The move to microservices was cancelled, and they instead started to look at ways they could improve the monolith. They refactored the structure which made the domain models clearer and indicated areas where coupling and duplication existed. In the end, this also meant in the future they could more easily find candidates for microservices if the need arised.
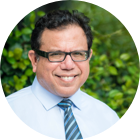
MMS • RSS
Article originally posted on InfoQ. Visit InfoQ
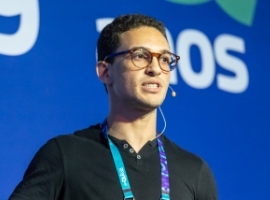
Transcript
Mourafiq: This talk is going to be about how to automate machine learning and deep learning workflows and processes. Before I start, I will talk a bit about myself. My name is Mourad [Mourafiq], I have a background in computer science and applied mathematics. I’ve been involved and working in the tech industry and the banking industry for the last eight years, and I’ve been involved in different roles involving mathematical modeling, software engineering, data analytics, data science. For the last two years, I’ve been working on a platform to automate and I manage the whole life cycle of machine learning and the model management, called Polyaxon.
Since I will be talking about a lot of processes and best practices and ideas to basically streamline your model managements at work, I’ll be referring a lot to Polyaxon as an example of a tool for doing these data science workflows. Several approaches and solutions are based on my own experience developing this tool, and talking with customers and the community users since the platform is open source.
What is Polyaxon?
What is Polyaxon? Polyaxon is a platform that tries to solve the machine learning life cycle. Basically, it tries to automate as much as possible so that you can iterate as fast as possible on your model production and model deployments. It has a no lock-in feature. Basically, you can deploy it on premise or any cloud platform. It’s open source. I believe that the future of machine learning will be based on open source initiatives. We already saw how the Python scientific libraries had huge impacts on the tech industry and also other industries. It’s agnostic to the type of languages, frameworks, libraries that you are using for creating models, so it works pretty much with all and major deep learning and machine learning frameworks.
It can be used by solo researchers and it scales to different large teams and large organizations. Basically, it has built-in features for compliance, auditing, and security. Obviously, when we talk about new platforms for machine learning, a lot of people are still skeptical about, “Why do we need new tools to manage machine learning operations?” A lot of people would ask, “Why can’t we use the tools that we already know and already love to automate data science workflows?”
Although I don’t agree, I think I understand pretty much why people ask these kinds of questions. I think the software industry has matured a lot in the last couple of decades. We’ve produced a lot of tools and software to improve the quality of software engineers’ work and make them a lot of tools for reviewing, sharing processes and also sharing knowledge, but I don’t think that these tools can be used for machine learning. In fact, I think the software engineer has matured a lot, so that when we use, for example, words from other engineering disciplines or civil engineering in infrastructures or platforms, we feel that it makes sense. For machine learning, I think it’s quite different, and to understand that, we need to ask ourselves two questions. What is the difference between traditional software development and machine learning development? The second aspect or the second question that we need to ask as well is, what is the difference between software deployments and machine learning deployments?
The Difference between Software Development and ML Development
The first big aspect or the first big question is, what is the difference between software developments and machine learning developments? There are three key aspects to this difference. The first one is, what do we need to develop when we’re doing the traditional software? And what are the objectives? In general, we have some specifications, so a manager comes with some specification, engineers try to write code to answer all the aspects of that specification. If you are developing a form or an API, you have already an idea of where you want to get to.
In machine learning, however, it’s quite different because we don’t have specifications. We just try to optimize some metrics, whether you want to increase the conversion rates or improve the CTR, or the engagements in your app, or at the time people are consuming your feeds; that’s the most important thing that you want to do and you don’t have a very specific way to describe this. You need to optimize as much as possible your current metrics to have an impact on your business.
The second aspect is how do we vet and assess the quality of software or machine learning models? I think it’s also very different. In software engineering, we developed a lot of metrics; we developed a lot of tools to do reviewing. We have metrics about complexity, lines of code, number of functions in a file or in a class, how many flows we need so that we can understand a piece of software in an easy way, and then we can have the green light to deploy it.
In machine learning, you might try the best piece of code based on TensorFlow or Scikit-learn or PyTorch, but the outcome can still be valid because we have another aspect to that, which is data. The way you look at the data is not objective; it’s very subjective. It depends on the person who’s looking at the data and doing all these kinds of developing and intuition on the data. You need some industry insights. You need to think about the distribution, if there’s some bias and you need to remove it. That way you also make sure that the quality of your model is good and is also different.
Finally, the tools that we use for doing traditional software developments and machine learning developments are different. In traditional software development in general, when you think about companies, you can’t even say that “this company is a Java shop, or C++ shop, or Python shop.” We think about companies by thinking about the most used language they have, the framework. A lot of people ask, “What are the companies using Rail?” Other people would say, “It’s GitHub, GitLab.” “Who is using Django?” They would say, for example, “Pinterest” or “Instagram.” Recently, there was a news post on Hacker News about how Netflix is using Python for data science, and one of the people who made a comment was really surprised that they are using Python because he thought that it was a Java shop.
For data science, you don’t think about frameworks. Data scientists probably will use different types of framework libraries. They just want to get the job done. If you can derive insights using Excel, you should use Excel. It doesn’t matter what type of tools you use to have an impact on your business. Even this aspect is also different.
Deployments – I don’t think that there is a big difference between deployments in terms of the traditional software engineer and machine learning deployments, but I think machine learning deployments are much more complex, because they have another aspect, which is the feedback loop. When you develop software and you deploy it, you can even leave it on an auto-complete process. If you have some sprints and you want to do some refinements, you might develop, for example, a form, and then if you miss validation, in the next sprint you can add this validation and deploy it, and everything should be fine. The people who are involved are the software engineer, maybe some QA, and then the DevOps.
Machine learning is different, because first of all, you cannot deploy in autopilot mode. The model will get stale, the performance would start decreasing and you will have some new data that you need to feed to the model to increase the performance of this machine learning model. Iteration is also different. Say you also have sprints and you did some kind of experimentation; you read some good results and you want to deploy them, but you still have a lot of ideas and a lot of configuration that you want to explore. The people who are involved in these refinements are completely different, because maybe you will ask some data engineer to be involved in doing some kind of cleaning or augmentation, or feature engineering, before you can even start doing the experimentation process. Then there’s, again, the DevOps to deploy.
I think that there are major differences between normal, standard software development and machine learning development, which means that we need to think about new tooling to help data scientists and many other types of employees who are involved in the machine learning life cycle to be more productive.
What a ML Platform Should Answer
This is how I think about it. If you are thinking about building something in-house or adopting a tool, whether it’s open source or paid, you need to think about how this tool can be flexible in order to provide and support open source initiatives. We all agree that every two weeks, there’s a new tool about time to ease, anomaly detection, some kind of new classifier that your data scientist should be able to use in an easy way. If you are developing or thinking about developing a tool, it should allow this kind of support for new initiatives. It should also provide different types of deployments. When you think about deployments, you also think about how you are distributing your models, whether for internal usage or for consumers who are going to use an API call. So, you need to think about different deployments for the model.
Ideally, I think it should be open source, I believe that open source is the future of machine learning. We all need to think about giving back to the open source community and try to immerse specifications or some standard so that we can mature this space as fast as possible. You should allow your data scientists and other machine learning engineers or data analytics who are going to interact with the platform to integrate and use their tools. You just need to provide them with some augmentation on the tooling that they are using right now. It should scale with users and by that, it’s not only the human factor, but also the computational factor, providing access to, for example, a larger cluster to do distributed learning or hyperparameter tuning, for instance. Finally, you need to always have an idea about how you can incorporate compliance, auditing, and security, and we’ll talk about that in a bit. To summarize, these are all the questions that a machine learning platform should answer.
ML Development Lifecycle
This is how, at least from the feedback that I got from a lot of people, the developments or the model management for a whole life cycle should look like. You first need to start by accessing the data; this is the first step. If you don’t have data, you just have a traditional software, so you need to get some data to start doing prediction and getting insights. Once we have access to the data, you need to provide different type of user access to create features, to do exploration, to refine the data, to do augmentation, to do cleaning, and many other kinds of things on top of data.
Once you have now the access to the data and the features, you can start the iterative process of experimentation. When we talk about the experimentation process, you need to think also about how we can go from one environment to another, how we can onboard new users or new data scientists in your company, how you can do risk management if someone is leaving. The best way is doing packaging. You need to think about the packaging format so that you can have reusability, portability and reproducibility of the experimentation process. Doing experimentation process by hand could be easy, but then you start thinking about scaling. As I said, you can scale the number of employees working on a specific project, but you can also just scale what one user can do by providing robust scheduling, robust orchestration, and hyperparameter tuning, and optimization.
When you scale the experimentation process, you will generate a lot of recall, a lot of experiments, and you need to start thinking about how you can get the best experiments, how you can go from these experiments to models to deploy, and it’s very hard because one user can generate thousands or hundreds of thousands of experiments. Now, you need to think about how you can track those experiments generating in terms of metrics, artifacts, parameters, configurations, what data went into this experiment, and how we can easily get to the best performance in experiments.
This is also very important when you provide an easy way to do tracking; you will have auto documentation. You will not rely on other people creating documentation for your experiments, because you will have a knowledge center; you will have an easy way to also distribute the knowledge between your employees. Managers can also have a very good idea about when, for example, a model is good enough that you can expect it in two weeks, and then communicate that with other teams, for example, marketing or business, so that we can start a campaign about the new feature.
Finally, when you have all these aspects solved – you have a lot of experiments, you have decent experiments that you want to try out in production – you need to start thinking about a new type of packaging which is a packaging for the model, and it’s different than the packing for the experiments. We will go back to all these aspects, but this is just to give you an overall idea. Then you can deploy and distribute the models to your users.
There’s not only one data scientist behind the computer creating models; there are a lot of type of employees involved in this whole process. Some of them are DevOps, some of them are managers, and they need to have an idea; for example, if it is a new regulation and your data has some problems with this regulation, you need to know which experiments use which data, which models are deployed right now using this data, and you need to take it down or upgrade it, or change it. You might also, in your packaging, have some requirements or dependency on some packages that have security issues, and you need to also know exactly how you can upgrade or take down models.
Finally, if you went through all these steps, you already have models and they’re probably having a good impact on your business; you need to refine and automate all these processes, going from one step to another. This is done by listening to events, for example, new data coming in on buckets, or probably because there are some automatic ways for just upgrading the minor of a package that you are using for deploying the models. You need to think about a workflow that can create different types of pipelines, to go from cashing all the features that you created in the second step, creating a hyperparameter tuning group, and take, for example, the top five experiments, deploy them, have an AV testing on these experiments, and keep two and do some in-sampling over these two experiments.
We will be talking about all these aspects one by one, and we’ll start with the data access. As a data scientist, I believe that you need access to a lot of data coming from a variety of backends. Basically, you need to allow your data scientists and data engineers to access data coming from Hadoop, from SQL, from other cloud storages.
When you provide data to users, you also need to have some kind of governance. You need to know who can access this data. In a team that can access credit card data, probably not everyone in the company can have access to credit card data, but some users can access the data. You need to also think about the processes of doing some encryption or obfuscation of that data, so that other people who are going to intervene later on can access this data in a very simple way.
When you do have access to the data, you can start thinking about how you can refine this data and develop some kind of intuition about it, how can you develop features. When you want to create these features and all this institution about the data, you need to have plug-ins, you need to have some scheduling so that you can allow the users, data analysts or data engineers to use subnotebooks, some internal dashboards, and also create jobs that can just run for days, to create all these features. You need to have some kind of catalog. In doing that, you need to think about caching all these steps, because if you have multiple employees who need to have access to some features, they don’t need to run the job on the same type of data twice, because it will just be a waste of computation and time. We need to think about cataloging of the data and also for the features.
Now that we have the data and the features already prepared, we can start the experimentation process which is an iterative process. You might probably start with working on some local version of your experiments to develop an intuition or a benchmark, but you might also want to use some computational resources, like TPUs or GPUs. We all know that when someone starts doing this experimentation, they start installing packages, pip install this, pip install that, and then after a couple of days you’re asking someone else to run the experiments and they find themselves unable to even get the environment running. I think this is a big risk management. You need to think about the packaging of formats of the experiments so that you can have this portability and reusability of these artifacts.
When you start the experimentation, whether it’s on a local environment or cluster users in general, they have different kinds of tooling, and you need to allow them to use all this tooling. I think one of the easiest way to do that is basically taking advantage of containers, and even for the most organized people who might have, for example, a Docker file, it’s always very hard for other people to use those Docker files, or even requirements files, or conda environments. It’s always super hard.
For example, in Polyaxon, we have these very simple packaging formats. It gives you an idea about how you can, for example, use a base image, what type of things that you want to install in this image. Thinking about user experience is super important when developing this, although in ad hoc teams. For the packaging, it should be super simple and super intuitive; what can you install and what you want to run, and this is enough for people to run it either locally or another environment. If you hired someone the next week or one of your employees is sick, the next person doesn’t need to start reading documentation to recreate the environment. They can just run one command and they already have an experiment running, and they start having really empirical impressions about how the experiment is going.
The specification should also allow more than just simple use cases, it should allow more advanced use cases, for example, using local files or using conda environments or cloned environments. Once you start doing experimentation locally or even on the cluster, you might start thinking about how we can scale this experimentation process. The first one is you can hire some more people, and when hiring more people, they probably don’t have the same gear or you want to centralize all the experimentation process in one place in a cluster, and now you need to start thinking about scheduling and orchestration – for example, using Kubernetes to take advantage of all the orchestration that it has and then building on top of that a scheduler that’s allowed to schedule to different type of nodes depending on who can access those nodes. By doing that, you give access to the cluster, to multiple users, and multiple users then start collaborating on this experimentation process.
You might also just give a couple of users more power, giving them the possibility to start distributed training, so using multiple machines. You don’t ask them to become DevOps engineers, they don’t need to create the deployment’s process manually. They don’t need to create a topology of machines manually and start training their experiments. The platform needs to know, for TensorFlow, PyTorch, these types of environments and these types of machines, and how they need to communicate. You also need to think about how you can do hyperparameters tuning, so that you can run hundreds of thousands of experiments in parallel.
Once you get to the point where you’re running thousands of experiments, you need to start thinking about how you can track them so that you can create a knowledge center, and the source should take care of getting all the metrics, all the parameters, all the logs, artifacts, anything that these experiments are generating. User experience here is very important, from the data access and data processing to the experimentation, there different type of users, and different types of users are expecting different types of complexity or simplicity of what they’re using, in terms of APIs and SDKs.
At Polyaxon this is the tracking API. It provides a very simple interface for tracking pretty much everything that a data scientist needs to report all the results to the central platform on. Once you have all this information, you can start deriving insights, creating, reporting, having a knowledge distribution among your team, having a knowledge center, basically. Now you have different types of employees who are also accessing the platform to also know how your progress is going. If you don’t have this platform, managers will ask in an ad hoc way, who’s doing what, what is the current state of the progress. But if you have tracking and you have a knowledge center where you can generate reports and knowledge, people can, from different types of departments, access the platform and get a quick idea of how the whole team is progressing. You need some insights, you need comparison between the experiments, you need visualizations, and you need to also provide a very simple way of adding other plug-ins, for example, using Tensor World or Notebooks.
At this point we already have a lot of experiments. We know how to get to the top performing experiments, and we need to start thinking about how we can deploy them. Deployment is very broad work because it could be for internal use, for some batch operation, it could also be deployments on a Lambda function, it could be an API or GRPC server, and you need to think about all these kinds of deployments that you need to provide inside the company. Lineage and the problems of the model are very important. When you deploy, you need to know how to get to this model, how can we easily track who creates this model using what, and if we should do some operation on top.
The packaging is also different than the experimentation, because the packaging for the model should know, “Given these experiments, I have some artifacts and it was using this framework. How can we package it as a container, and deploy it to the right destination?” When you already have a couple of deployments, you’re seeing performance improving and managers are happy. There’s probably a regulation that changed recently and you need to act as fast as possible, so you need the lineage and improvements of this model. You need an auditable workflow to have a rigorous workflow to know exactly how the model was created, and how we can reproduce it from scratch. Thinking about this, user experience is very important because if you have ad hoc teams working on different components, you need to provide them different type of interfaces to derive as many insights as possible.
We get to the state where we went through the experimentation, we created a lot of experiments, we generated reports, and we allowed a lot of users to access the platform. We are thinking now about how we can do refinements. With refinements, you should think about how you can automate as much as possible jumping from one aspect to another, because if you don’t have an easy way to automate this jumping from one aspect to another, you will involve the same people going from a data analyst or a data engineer, machine learning practitioner, or data scientists, QA, and then DevOps, and then everyone need to do the same work again and again, and you need to think about how you can cache all the steps so that these people can only intervene if they need to intervene. Again, the user experience is important, so if you are doing events, action or pipeline engine, you need to think about what your users are doing right now. If they are using Jenkins or Airflow, we should not just push a new platform and ask them to change everything. You need to think about how you can incorporate and integrate this already-used tooling inside the company and justify augmenting their usage.
Finally, you need to think about what kind of event you are expecting so that you can trigger these pipelines. By event, this could come from different types of sources. If you are doing CICD for software engineering, you need to think about also CICD for machine learning. It’s quite different because in here, not only do you have databases on code, if you have new code, you need to trigger some process or pipeline. You might also have that because you are polling for some data and there is new data, and you need to trigger this workflow. You might also trigger the workflow for different types of reasons. For example, you have a model that’s already deployed or a couple of models that already deployed, and some metrics stop dropping. You need to know exactly what happens when a metric starts dropping. Are you going to run this pipeline or the other pipeline? Are you on multiple pipelines? What happens when the pipeline starts? Do you need some employees to intervene at that point with some manual work or is it just an automatic pipeline where it just starts training by itself, it looks at the data and deploys it?
At Polyaxon – it was supposed to be released last week, it’s open source – it’s a tool called Polyflow. It’s an event action framework which basically adds this event in mechanism to the main platform so that you can listen to Kafka streams or listen to new artifacts, get generated on some buckets or listen to hooks coming from GitHub to start experiments. This is where user experience is very important. I think not having a complete pipeline is important, but having just a couple of steps done correctly with user experience in mind is very important. When you are building something like these pipelining engines or this kind of framework, you need to think about what is the main objective that you are trying to solve, and I believe that is trying to have as much impact on your business as possible. To have this kind of natural impact, you need to make your employees very productive.
That’s it for me for today. I hope that you at least have some ideas if you are trying to build something in-house in your company, if you are trying to start incorporating all these deep learning, machine learning advances and technologies. I cannot emphasize enough that user experience is the most important; whether we are a large company or not, or whether we have different types of teams working on different types of aspects of this life cycle, we should always have this large picture and not just be creating APIs that communicate in a very weird or very complex way. You need to think about who is going to access the platform. By that time, I think that the data analysts, data engineers, machine learning practitioners, data scientists, and DevOps, and engineers as well who are doing the APIs and everything, every one of these employees, every one of these users should have the right way of accessing the platform, the right way of seeing how the progress is going, the right way of also adding value to the whole process.
All these workflows are based on my own experience developing Polyaxon. It’s an open source platform that you can pretty much use for doing a lot of things that I talked about right now. The future of machine learning will be based on all these kinds of open source initiatives, and I hope that in the future also as a community, we can develop some common specifications or common standards so that users can always integrate the new tools, can jump from one platform to another without having to feel like they’re locked in into some system that will just have negative impacts on their productivity.
Questions and Answers
Participant 1: How does your tool connect to well-known frameworks, like TensorFlow or Keras? How is the connection with the tool with these kinds of frameworks for deep learning?
Mourafiq: You are talking about Polyaxon, I assume. In Polyaxon, there are different kinds of integrations. There’s some kind of abstraction that is created and each framework has its own logic behind, but the end user does not know about this complexity. They just say, “This is Michael. This is the kind of data that I want access to. These are how I want to use the experiments. If it’s distributed learning, I need five workers and two parameter servers,” and the platform knows that this is for TensorFlow, not MXNet, so it creates all the topology and knows how to track everything and then communicate the results back to the user without them thinking about all these DevOps operations.
Participant 2: What kind of hyperparameter optimizations does Polyaxon support?
Mourafiq: At the moment, there are four types of algorithms that are built in the platform, Grid search and Random search, and there’s Hyperband and the Bayesian optimization, and the interface is the same as I showed in the packaging format. This packaging format changes so that you can expose more complexity for creating hyperparameter tuning. Once you communicate this packaging format, the platform knows that it needs to create a thousand or two thousand experiments running. You can also communicate what the concurrency is that the platform should do for this experiment; for example, running 100 experiments at the same time. If you also want to stop the whole process at some point if some metrics or some of the experiments reach, for example, a metric level, you don’t need to keep running all these experiments and consuming the resources of your cluster.
Participant 3: How do you keep version of the data? Because if you wanted to repeat some of these experiments later on and maybe you do not have the original data anymore or the original data source. How do you version the data?
Mourafiq: This is a very simple or minimalistic version that you provide, but you can also say what type of data you want to access, and the platform knows how to provide the type of credentials to access to the data. For tracking the versions, you can have this log; that’s our reference. Then, if you are splitting the data or doing some more things during the experiments, you can have these caches that represent the data.
See more presentations with transcripts
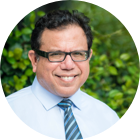
MMS • RSS
Article originally posted on InfoQ. Visit InfoQ
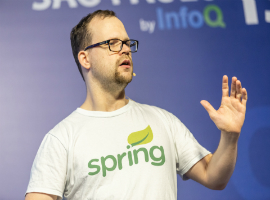
Transcript
Long: My name is Josh Long. I work on the Spring team as a developer advocate. If you’re in China, this is a great thing to know about. Here are some videos that I do on Safari. These are on the All You Can Eat side of technical marketplace, it’s a buffet for technical content. They are five, six, seven, eight hours each one. Lots of good content there.
I have a book called, “Cloud-native Java” that’s all about how to build applications that survive in production using Spring Boot and Spring Cloud, and Cloud Foundry. The book is something that I’m very proud of, it’s something that took a long time to get right. There’s a good reason that we were so delayed. We finished the book pretty quickly, but there was a long debate, a long back and forth, a long discussion that we had with our publisher, O’Reilly, that took a little bit longer. Not much longer, we took six months to write the book, and then it took another two years. It was not a big deal. The extra two years we spent debating the animal on the cover.
Anybody who knows anything about O’Reilly books, knows that it doesn’t matter what’s in the book itself, obviously, nobody cares. Have you ever read these books? No. Look at all the Amazon reviews, it’s just, “Great animal, great animal.” “Good choice.” “Great selection.” We eventually settled upon a blue-eared kingfisher. It’s a bird with blue ears that is only found in the Indonesian Java islands. It’s native to Java and birds fly through the clouds. It’s a Cloud-native Java bird. I have a podcast every Friday called “A Bootiful Podcast.” I do videos every Wednesday, that’s Spring Tips playlist. You find me doing half-hour, hour long introductions to all sorts of different topics: how do you Spring in Google cloud, Alibaba cloud, Microsoft Azure, how to use messaging, integration, security, everything, all sorts of topics, circuit breakers, whatever.
Reactive Programming
I have a new book called, “Reactive Spring.” This book is all about how to build applications using reactive programming, and that’s what we’re going to talk about today, my friends. It’s a very exciting thing to discuss. It’s exciting, but it is not new; or really actually, reactive programming is kind of new, but the problem that it solves is very old. The problem that we’re trying to solve is, how do I handle more users in my system? As I add more capacity to the system, how can I scale the number of users I can support?
If you have been following the patterns and the trends from the last 10 years, then you were already building microservices. You’re doing cloud-native applications, you’re doing 12-Factor App style services, stateless, small, singly focused microservices, and these services are cheap to scale out horizontally. You can just put them in a load balancer and then randomly load balance load across the different instances and you’re fine. That’s a very easy pattern to do. All cloud infrastructure today will do that for you. It’s a built-in thing, no problem at all.
This is a useful pattern because it allows us to handle more users. The reason we do this is because we can’t handle infinite numbers of users on one machine. We talk about Netflix, the cloud-native architecture from 10 years ago, right around the same time as Q-Con first came out. The first cloud-native architecture was, “Let’s get stateless microservices and Cassandra.” All the state is in the database and the database scales horizontally. The reason that’s such a useful pattern is because you can write applications that are just HTTP APIs, and even if the HTTP API is not super-efficient, who cares? You can just add more app instances.
I work for Pivotal, and at Pivotal we sell cloud computing technology. I love that answer, that’s a great answer. Every time you scale out, companies like mine make more money. It’s awesome, I love that, but there’s a question. Why can’t we do more with the same machine? How do we handle more users with the same hardware? The question is, how can I do more with the same computer? Not, how can I go faster per transaction? The question then is, how do I handle more users in the same computer? Obviously, there are some interesting answers here. You’ve probably heard about the C10k problem, the 10,000 concurrent users’ problem. This is a very simple problem posed 20 plus years ago. The question was, how do I handle more than 10,000 users at the same time on a single machine? The answer is, not with threads. Threads are actually a bit of a problem.
Think about how most traditionally approaches to input and output work. You have a web server and each time a user comes in, a new thread is created, and in that thread, a response is produced, and while you’re producing the response, what are you doing? You’re calling the database, waiting for the bytes to come back. You’re calling other APIs, other gRPC, or REST, or whatever services you’re calling. You’re making network calls across the wire. You might be calling things in the file system, might be sending messages over to message cues. All the while, as this is happening, you’re waiting and waiting, and bytes are coming back and forth. You’re sitting on that thread waiting for the bytes. The problem is that, while you’re doing this, you’re stuck on that thread, that thread is not usable for anybody else. It’s unavailable.
The question is, is that ok? Can I just scale out infinitely? Can I just add more threads forever? Of course, the answer is no. I’m sure you’ve all tried creating a thousand threads. That’s not going to be a great result. There are a few reasons for this. First of all, threading is an illusion. It doesn’t actually exist beyond the number of cores you have. Beyond the number of cores, you’re just scheduling, you’re doing work very quickly and moving it from one processor to another. After that, you’re just going to be thrashing; there’s a cost for context switching.
Now, even then, can you do the thrashing? Can you do the context switching very fast? That’s actually a slow operation, to go from user space to the kernel and then back again for code like that. That’s slow, so you’re not going to have a lot of good results if you create a large number of threads. Also, on the JVM, each thread takes about 1 megabyte of space RAM. If you have a thousand threads, you’ve got a gig of extra RAM. That’s before you’ve done anything. You haven’t solved any of your problems yet, and you’ve already wasted a gig. This is not a good solution.
The question is, what can we do to be more efficient with the threads that we’ve got? We can look back at that situation I just described, where we’re waiting for the bytes to come back. The reason we’re waiting for the bytes is because we’re using synchronous blocking input and output. The answer here is to use asynchronous I/O. Asynchronous I/O is not a new idea at all. In fact, it’s been in Java since 1.4. 1.4 came out in 2002, 17 years ago. It’s been around for a long time. You can use it to great effect today, it’s already been there for 17 years. You know what that means? If it’s in Java, every single operating system that supports Java has to already have good support for asynchronous I/O.
This means that every operating system that supports Java and has asynchronous I/O support has had it for at least another year or two more than Java. They don’t just throw that into Java immediately after its added to an operating system, they let it stabilize a little bit. This means that every operating system, for at least let’s say 20 years, has supported asynchronous I/O, even Windows. We have asynchronous I/O support, why don’t we all use this? Are you all writing code using java.nio? Is your everyday line of business all about that? I think not. I think most of us don’t write code that low level.
Most of us don’t write code that low level and we don’t use Java I/O either. We use higher order abstractions. We have collections and things like this. These collections, these abstractions map nicely to the underlying machine, to the mechanism that we use for input and output. Now, we need something that supports that same kind of mapping for asynchronous I/O and this is where the Reactive Stream specification comes in. The Reactive Stream specification was defined by at least four different companies, maybe a few more. You’ve got Pivotal, you’ve got Netflix, you’ve got the Eclipse Foundation, you’ve got Lightbend – they were called Typesafe at that time. All these different organizations got together, and we defined four very simple types. These are like asynchronous arrays, they’re the reactive streams types. They’re very useful, but they’re kind of like an array, they’re not high level enough. Most of us want operators so that we can deal with streams of data.
This is where we get project Reactor, and RxJava, and Akka Streams, and so on. These projects support higher order computations on streams from these reactive stream types. Is that enough? Can we go to production yet? The answer for most people is no, not really. What good are those types if they don’t support working with other kinds of infrastructure? If they cannot support us in our efforts to build business software to deliver into production, what good is that?
Imagine, just hypothetical, for whatever reason, that these technologies, these tools that we are familiar with, the ones that we have used for so long, imagine that these technologies did not understand or support java.util.Collection types, like java.util.List or java.util.ArrayList. Imagine, for whatever reason, that whenever you tried to use Hibernate to map a one-to-many relationship. Imagine that it didn’t just throw an exception. It really hates java.util.Collection. Obviously it does not, but imagine it did. It really hates java.util.List and set, it really hates it. It doesn’t just throw an exception, it actually renders an ASCII middle finger, and then kernel panics the machine.
Would you continue to use this if you knew that you can expect this kind of abuse? Of course not. You would use whatever Hibernate told you to use so that you could get software into production. Your job is to go to production and to deliver business value. It’s not to figure out how to reinvent Hibernate. You can do better – you will do better, so you’ll just use the path of least resistance. The same is true here. What good are these reactive streams types if the software that we use to deliver business value don’t support them? This is where, for Spring, a big day came in September of 2017 when we released Spring Framework 5, and then Spring Boot, and then Spring Data, and Spring Security, and Spring Cloud. All these releases, after that, supported Java 8 as a baseline and the reactive streams types and a reactive web runtime.
My friends, today, we’re going to take a very quick journey and look at all these different components, all these different pieces, and see how they help us build better software, faster. Of course, to do that, we’re going to go to my second favorite place on the internet. My first favorite place of course is production, I love production. You should love production, you should go as early and often as possible, but if you haven’t been to production, you can begin your journey here at start.spring.io.
start.spring.io – live coding
We’re going to build a new application called the reservation-service. We’re going to use Spring Boot 2.2 snapshot. We’re going to bring in RSocket, we’re going to bring in Lombok and the Reactive Web. We’ve got all the good stuff that we need there. I’m happy with my selections, RSocket, Lombok, Reactive Web, Reactive MongoDB. I’m going to hit generate and that’s going to give us a new zip file. I’m going to open up this zip file in my IDE. I’m going to go here, CD, downloads, UAO.
We have a new application, it’s started up. It’s a public static void main Spring Boot application. What I’m going to do is I’m going to build an application that writes data to the database and I have MongoDB running in the background on my local machine. It’s going to be an object that we’re going to map that’s going to have two fields, ID and name. We’re going to say “@ID” and “@” and then “name”. It’s going to be a document. In MongoDB, a document is a single row and you have a collection which is multiple rows. It’s like a table. This is the essence of what I want to express, but of course, it’s Java, so getters, and setters, and two string, and constructor, and equals, and hashCode, so modern, so good. No, probably not.
We get rid of that and we use Lombok. Lombok is a compile time code generator. It’s an annotation processor. With that, I have my getters and setters. Now, what I want to do is to write data into the database, and I’m going to create a repository which is a pattern of course from domain-driven design. It’s a thing that handles the boring lead, write, update, and delete logic. This interface, we don’t have to implement it, we just define it and Spring will provide an object that supports these methods, all these methods like save, save all, find by ID, check if it exists, find all, delete, count, etc – all these things that are very obvious. The names are obvious, but the types might be a little different for you.
First of all, here’s a publisher. A publisher comes from the reactive streams. It’s one of those four types that I was telling you about before. The publisher is a thing that publishes, it emits, it broadcasts items of type T to a subscriber. The subscriber listens for the item, and whenever the item arrives, onNext is called. When there is an error of some sort, throwable is given in the onError method. Now, keep in mind, in Reactive programming, it’s kind of functional programming, and so in this respect, errors are not special, they’re not exceptional. They don’t get their own control flow mechanism. You deal with them in the same way that you deal with any other kind of error. Then finally we have onComplete which gets called when they’re done processing the data.
I skipped over this because this is the most important part. When the subscriber subscribes to the publisher, it is given a subscription. The subscription is used by the subscriber to ask the publisher to slow down, it’s very important. When the subscriber is being overwhelmed, it can request just to five records, or 10, or a million, or whatever. It will not get more than this, this is called flow control. We ensure stability by slowing down the publisher. In flow control, in the world of reactive programming, we call flow control “back pressure.” If you have seen back pressure, if you have heard that word before, now you know what it means. If you want to cancel the production of data, you just call cancel.
That’s three of those four types. The fourth type is called a processor, and that’s this one right here, processor. Subscriber and publisher, that’s it. I forgot to do something very important on the Spring initializer. I’m going to use Java 11, that’s the only sane choice in 2019. I’ll go ahead and add that. Now, I’ve got my application, let’s write some data to the database. I’m going to say “sample data initializer,” and it’s just going to be a Spring bean that listens for an event. ApplicationReadyEvent.class, public void go. When the application starts up, this Spring bean is going to be given a call back. I’m going to inject a reservation repository like this into the constructor. I can do that like this, or I can use Lombok and say “@RequiredArgsConstructor.”
What I want to do is, I want to write some data to the database. I’ll say “flux.just” and we’ll have some names here. I’ve got Louise, I’ve got Josh, I’ve got Anna, Spencer, Cornelia, Veronica, Madura, and Olga. Just eight different names. I’ve got a reactive streams publisher. Now, you haven’t seen this type, Flux is from Reactor, it extends publisher. You can see it’s a publisher, but Flux also supports operations – flat map, map, filter, all these kinds of things above and beyond regular publishers. It publishes 0 to X values, where X could be unlimited.
I have a publisher of names, and now I want to map each one, each name like this, new reservation, null passing and that. That gives me a publisher of reservations, res. Now, I want to map each one of those, map r and I’m going to save this to the database using the repository, so saving that in.
What does map do? Map takes the return value from the Lambda and it collects it into a container type. What does save do? Well, save returns a mono. You can see it says “mono of reservation”. A mono is another publisher, it produces at most one value. I’ve got now a mono and I’ve got a publisher, this is not what I want. If you look at the results, I get publisher of publisher of reservation. I don’t want that, I don’t want both. Instead, I want to get just one publisher, so I say, “flat map” and I get rid of the intermediate type.
If I run this code, obviously there are some opportunities here to clean it up. I can say “saved”. Now, if I run this code, what happens? The answer is nothing. Nothing is going to happen because I have to activate the stream. It’s a cold stream, in order to make it hot, you say “saved.subscribe”. Now, if I run this code, we will see some problems. First of all, when I run this code multiple times, I’ll have eight records in the database, and then 16, and then 24, and then the same data over and over. I want to delete everything. Can I just do this? What about this? This .reservationRepository.deleteAll. Well, deleteAll returned to publisher, so I have to subscribe. The problem with this, of course, is that it’s asynchronous. This could execute on a different thread. I need to force this to happen before this. I could do this.
This code makes me want to take a shower, this is not what we’re supposed to do. Instead, use the operators here. I’ve got a publisher. What I want to do is I want to turn this code into a nice single pipeline, a single intermediate-staged pipeline with multiple pipeline stages. Here we go, now I’ll say, delete everything, then write the data to the database, and then find all the data. Then finally when all the data comes back, let’s log it out. I’m going to use a logger, again, from Lombok. Now, run this, let’s see what happens. Is it going to work? Look at that, it worked. We’re all there in the database. You can see the unique IDs, the names, everything worked. Of course it worked, it was a demo. What were you expecting?
What I wanted to talk to you about is this. This is the Spring Boot ASCII artwork. This artwork took a long time to get right, but we on the Spring team have many people who are doctors, PhDs, and they worked very hard on this artwork. It makes me very sad and it makes me want to talk about this very problematic feature in IntelliJ, something that I consider a serious flaw in the product. Do you see this checkbox? If you click this checkbox, it suppresses the output of the ASCII artwork. What the hell? Why is this there? That’s a dumb feature. Nobody even asked you, IntelliJ, ok? I do what everybody would do. I went on the internet and I cried, I complained on the internet. I was sent a message of hope from my friend Yann Cebron who’s a software developer by passion at Jet Brains. He sent me this message which I want to share with you here today. He says, “Don’t worry, Josh. The next release will no doubt contain this feature. It will be fixed in the next release. Just relax, Josh.” He tells me all the time and we have been friends for a long time. I want to believe him, but you know what? I’m starting to think maybe he’s not being serious.
Anyway, we have a good ASCII artwork, we have data in the database. Now, we need to have an API. We can do this using the new functional reactive style in Spring Boot, in Spring Framework. I’m going to define a bean, like so, hit “build”. I’m going to create an end point that serves all the reservation data. In order to do this, I’m going to inject my repository, so I’ll say, “new HandlerFunction”. I’m going to say “return a ServerResponse.body” and I can say “rr.findAll(), Reservation.class.” There’s my handler function and my builder. It’s a nice Lambda, I can remove that. There you go, my brand-new HTTP M point. Not bad, I’ve got an HTTP service, not a big deal.
Moving on, I like HTTP. It’s awesome obviously, but a lot of people asked me, “Well, can I use asynchronous protocols? How do I do that?” Of course, in this case, remember, findAll just returns a publisher. Even though there are only eight names, only eight records, it’s still an asynchronous stream. Everything in the reactive world is a publisher, it makes life very simple. What if you want to do WebSockets? Well, you just use publishers. WebsocketConfig and you just create a bean like this, Simple Url Handler Mapping. All this is going to do is it’s going to tell the framework how to expose, how to mount a particular service, so I’ll say Map.of (“/ws/greeting”) and I’m going to inject a WebSocket handler. The WebSocket handler will have the actual business object and we’ll define that in a second.
Here’s my handler, and of course I’m going to set the order as well, so 10. Then the other thing I need to create is a WebSocket handler adapter. Then the next thing I need is a WebSocket handler and this is actually our business logic. Think about what I’m going to do here. I’m going to create a thing that is asynchronous. It doesn’t just return the reservation data, it’s going to return a stream of values that will never stop. Here, I’m going to create a simple bean that just produces new data all the time. “Flux greet,” and I’m going to create a greetings request. I haven’t defined these types, so let’s do that right now. Class GreetingResponse, and class GreetingRequest. I’m just defining two types and the response has a greeting in it, private String message and the request has the name of the person to be greeted, so @data, @AllArgsConstructor, @NoArgsConstructor, that’s beautiful. I want to take that and paste.
Now, I’ve got the simple service. It’s a simple thing that will produce data for me. It’s a never-ending stream. In order to do that, I’m going to use a publisher. I’m going to say, Stream.generate, a new stream from a new supplier and I’m going to create a new greeting response using the request.name, “Bom dia” Request.getName @instant.now. There’s my greeting. Now, the thing is, this is going to create a never-ending stream, it’s a stream that will go forever. What I want to do is I want to delay it, I want to stagger the results. What I will do is I will introduce some time. I can delay it by one second there. The reason I can do this is because there is a scheduler behind the scenes that controls threads.
Down here, when I wanted to write data to the database, remember, each line is on a different thread, it could be. It doesn’t have to be, but it could be, and that is controlled by the scheduler. By default, you have one thread per core. You can control that if you want, you can overwrite it by using a custom thread pool back to scheduler like this. I don’t need to, you shouldn’t need to, it’s considered a code smell if you do that, but if you need to, it’s there. Now I’ve got my greetings producer. Let’s actually produce a response here, so producer. What I’m going to do is I’m going to say, “Ok, when the session comes in, when I am connected to the client or when the client connects to my service, I will be given a brand-new WebSocket session.” I’m going to receive WebSocket messages and I’m going to map each WebSocket message into some text. I’m going to map each one of those into a greetings request, aren’t I? New greetings request passing in the text, that’s the name.
Now, what I want to do is I want to take each greetings request and call producer.greet like that. That’s going to give me a stream of greetings responses that I want to turn into text and then send back into the WebSocket as a text message, so session.textMessage. I’m creating a reactive stream, I’m responding to requests that come in and I’m turning them into strings that get set back out like that. It’s all very functional in Lambda E and all that stuff. The result of this all is a stream of WebSocket messages, a publisher of WebSocket message. I’m just going to send that session.send, like so. There’s my WebSocket handler. of course, this could be a Lambda.
In order to demonstrate this, I need to have something to use it, a client. I need to do something now that I’m not proud of, something that I only do because I think we’re friends. What I’m going to do is some JavaScript. File, WS HTML, body HTML, script, and I’m going to say “window.addEventListener, load, function E,” and then I’m going to create a WebSocket event, a WebSocket client, WS, Local host. I called it ws greetings, 8080/ws/greetings. I’m going to say addEventListener (“open”). When the WebSocket client is opened, I’m going to initiate a request. I’ll say, ws.send a name. Then I’ll say what name, “var nameToGreet will be window.prompt (“who should we greet?”), I’ll say nameToGreet.
In order to get the data that returns, I’ll say (“message”), let’s listen for the message, and log it out on the console, “new greeting” + msg.data. Local host, ws.html, 404 local host 8080. Friends, obviously, you need to tell Spring about your configuration otherwise it won’t care. That endpoint wasn’t registered because it didn’t know to look for the beans there. Who should we greet? Bob. There you go, every second, forever. The point of that is that we’re not actually blocking the thread on the server site. Each time it’s going to publish an event and then the thread is reused by something else.
You understand now that you can use publishers to create very simple stuff and it’s easy to do what we can already do. The real possibility comes when we take reactive programming and go further, so far we have done reactive MongoDB. We’re not blocking anywhere in MongoDB. MongoDB is a good example of a database that works naturally very nicely with reactive APIs. The driver is natively reactive. It’s asynchronous I/O from the bottom up. It’s not just a reactive facade on top of something that is blocking.
There are some nice things there. You can do transactions, you can do tailable queries, I like MongoDB. Let’s say that I want to go back and change my data layer, well, a question people ask is, can I use reactive SQL? There’s not been a great option here for a long time. There’s github.com, R2DBC from Pivotal, and this is I think a good choice, but keep in mind, this is not yet GA, it’s a simple SPI that supports natively reactive SQL data store integrations for PostgreSQL, Microsoft SQL server, and H2, and there’s even a third party one supporting MySQL. We can use this in our code, but right now, for the moment, there is no autoconfiguration of the box in Spring Boot, so we have to add it ourselves.
What I’m going to do is I’m going to is I’m going to go to my build, and add these dependencies here, supporting PostgreS, and Spring Data R2DBC and PostgreSQL. I’m going to comment out MongoDB, I don’t want MongoDB in this example for the moment. Because of that, I will have to change some things. First of all, that goes away, and second of all, I no longer have a primary key of a string; instead I have a monotonically incrementing ID. In order for this to work, I also need to connect my application to the database, extends AbstractR2dbcConfiguration, and I’m going to overwrite a connection factory. I’m doing a little bit of the work that you would normally not need to do in the future. In the future, this would be done for you automatically by Spring Boot.
This is a configuration class, and it’s going to have R2DBC repository support. In order for me to connect to my database, I need to tell it where to go, so I’m going to do something terrible. I’m just going to write the information here on the stage. Now, the application, psql -U orders, orders, select all from reservation. There we are, there are our names. We had it in MongoDB as well, so we can see now the IDs are primary keys, we’re using primary keys to do reactive SQL. I like this, I like R2DBC, it gives us a path forward for the existing applications using SQL data stores. Not all databases are supported, but more are coming all the time. If you have one of those four databases, we’d love to see you try it and let us know what we can do to make it better.
I’m going to say something pretty controversial here, this is kind of scandalous. I think that HTTP is going to be big. I think people are going to use it. They’re going to like it, HTTP has a future, I think it’s a great document retrieval protocol. There is a discussion to be made that it’s not a great application protocol, is that ok? Is that fair? What I mean by that is that there are certain kinds of message exchange patterns that aren’t well supported by HTTP. For example, HTTP does request/response. I make a request, I get a response, that’s very simple. But what about server-side push? How do I push the data down? Well, I could do Server-Sent events. I could do WebSockets, but in Server-sent events, it’s all text. How do I encode data that’s binary using Server-Sent events? I have to Base64 encode it, and then unencode it on the client.
What about security for both WebSockets and Server-sent events? There are no headers. There’s no message frame in those messages for headers. How do I propagate a security token for these kinds of things? These are basic things that all applications need, and yet aren’t addressed by these basic protocols. A lot of high scale organizations that want to do a better job that cannot afford to lose so much bandwidth and so much inefficiency on HTTP have used RPC or some sort of binary message encoding.
Google, very famously, created gRPC. Anybody here using gRPC? Some people are using it. It makes sense for certain use cases. It uses and requires Google protocol buffs, so that’s ok. Salesforce is a company that is in the Bay Area where I live, and they have a terrible ugly looking tower in the middle of the city. They created a pro talk gRPC compiler that uses our project Reactor. That’s ok, there’s another small website where I come from in San Francisco in the Bay Area called Facebook. They are apparently a very big website now and they needed to scale, so they created a binary protocol called RSocket.
RSocket is supported in Spring Framework 5.2. What I’m going to do is I’m going to create a RSocket controller here. RSocket is binary by default, you can use whatever message encoding you want. It supports four different message exchange patterns: request/response, single value in and single value out, single value in and stream out, stream in and stream out, and stream or single value in and no response, like fire and forget. You can create RSocket services, and they also have an interesting extra thing. It’s built by the team that came from Netflix that built RX Java. It’s natively reactive. The protocol is built to support reactive streams of data. It’s not just like RPC, it’s actually asynchronous reactive RPC on the network protocol. There are C++ bindings, JavaScript bindings and Java bindings. The Java binding from Facebook uses reactor and you can imagine what kind of scale that gets to use that.
We’re going to use RSocket here. It’s a service that we’re going to use. I’m going to create a GreetingsRsocketController. I’m going to create a publisher of greetings response, greet given a GreetingsRequest. Does this look familiar? In order to do that, I’m going to inject my GreetingsProducer and we’ll say @RequiredArgs and I’m going to say MessageMapping(“greeting”). This is the mapping. How does a client find this end point in the service? Normally if you use RSocket directly, you end up creating this switch statement, basically, but I’m just going to use RSocket like that. That’s my service, in order for me to start it up on the server, I need to specify which server I want to run on. I’m going to say port=8000.
On the client, I want to create a client to talk to this. I’m going to go back here, and I’ll just create a client, reservation client, and I’m going to bring in RSocket itself and I’ll bring in the Reactive Web, and Lombok, and there we go. I’m just going to bring in the basics here. My reactive RSocket service, I’ll open up the client here in my IDE. Keep in mind, RSocket also has an extra thing that’s very nice. It has the ability to tell you its up time. In the protocol itself, there is information about the service’s health, and you can change what shows up there, but it’s a good way for clients to do very smart load balancing. Imagine you connect to the service and the server says, “I’m too overwhelmed. I have too many users. Go to somebody else, please.” This is what actuator does. If you’re using Spring Boot, you have the actuator, this does that for you. Now it’s in the wire protocol itself.
I’m going to create an RSocket client, and in order to do this, I’m going to do something terrible, something that you should never ever do at home, I’m going to copy and paste some code. Here are my client types, and I’m going to run this application on port, let’s say, 9090. I’m going to create a simple API Adapter, not a Gateway. For more on Gateways, you should see Spencer Gibb’s talk on Gateways, API Gateways later today. That’s going to be good. I won’t focus on Gateways.
We’re going to create a simple API Adapter. That API Adapter is going to be an HTTP endpoint that just streams the data across the wire using RSocket. I’m going to create another functional reactive endpoint like this, and that’s going to be greetings. I’m going to stream the greetings here – actually, better yet, let’s just do a Spring MVC style controller, class GreetingshttpController, GetMapping (“/greetings/{name}”) flux of GreetingResponse, greet (@PathVariable String name). In order to make this network call, I’m going to use an RSocket client in order to get the data. I need to create an RSocket instance first of all, like this, return RSocketFactory, .connect(), .transport(TcpClientTransport on port 8000, so it will be .start(), .block().
Before I connect, I need to connect with a mime type. I’m going to use MimeType utils, application_json_utf8_value, and frame decoder will be this one. There’s my basic RSocket reference, and I can use that to create an RSocket requester. I’ll say, return RSocketRequester.create, passing in the RSocket reference. I’m going to change the mime type, I’m going to use a RSocket strategy, I’m going to inject RSocket strategy.
There’s my client, and in order for this to work, I’m going to make this an endpoint that serves server-sent events, so produces = MediaType.text_event_stream_value. In order to use this, I’ll use private final RSocketRequester, required args constructor, return this.requester.route, “greetings”, .data(new GreetingRequest) passing in the name. Retrieve a publisher of greetings response.class. I’m creating a server-sent event endpoint on the different port. This is my client running a port 9090. We know that if we go to 9090/greetings/name, it’s going to call my RSocket service on port 8000. 9090/greetings/Sao Paulo.
I hope you see the possibilities here. I’m a big fan, obviously, but you don’t have to take my word for it. Like I say, Netflix, Facebook, Alibaba, eBay, lots of other companies are building on Spring Boot, and Spring Cloud, and Reactive to build the better software faster, to get to production faster, and that, at the end of the day, is all that really matters.
See more presentations with transcripts
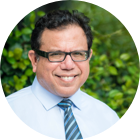
MMS • RSS
Article originally posted on Data Science Central. Visit Data Science Central
Introduction:
The art and science of discriminating between writing styles of authors by identifying the characteristics of the persona of the authors and examining articles authored by them is called Authorship Analysis. It aims to determine characteristics of an individual like age, gender, native language and personality traits based on “available information” pertaining to that individual.
In this article, “available information” refers to textual data only in the context of authorship analysis, however, information in this context could go beyond textual format as it might also involve usage of multi-modal observations. Multi-modal observations capture characteristic features such as voice, intonation, gestures, body posture and other physical behavioral aspects of an individual. A combination of all these characteristics reflects the persona of an individual and consequently helps in profiling that individual. In most cases, multi-modal data are sourced from videos which are then quantified to machine readable as well as processable format.
Application Areas:
Why authorship analysis is important? It plays a crucial role in forensic analysis and crime investigation. Besides, social media and the open web resources have invited a wide set of cyber crimes — fake profile creations, fake reviews by bots, plagiarism, dark web websites facilitating networked and organised terror, discerning terrorist proclamations, harassment and intimidation through social media messaging to name a few. [1]
Understanding consumer profiles and feedback analysis is paramount to Market Analysis and intends to examine the demographics of the author of anonymous feedback. The source of the raw texts could be blogs, online product reviews or social media forums. [3]
Other application areas include resolving disputes in authorship of novels, plagiarism detection, document dating, examining socio-economic factors and mental health examination.
Text Classification Tasks involved in Authorship Analysis:
Different objectives or tasks work towards a common goal of authorship analysis. The three major tasks are — Author Attribution, Author Verification and Author Profiling.
i) Author Attribution: Author Attribution is determining that, after investigating a collection text from multiple authors of unequivocal authorship, if an unforeseen text was written by a particular individual. This is ideally a closed-set multi-class text classification problem. [2]
ii) Author Verification: This task determines whether an individual has authored a piece of text or not by studying a corpora of the same author. This is a binary single-label text classification problem statement. Although, this task seems easy, author verification is a far more complicated process in real.
iii) Author Profiling: Author profiling could also be recognized as personality identification of an author by studying the authored texts. This involved predicting demographic features like gender, age, native language and personality traits of an author from examining their writing styles [1]. Author profiling can be viewed as a multi-class multi-label text classification and a clustering problem. This is a potential clustering problem because we aim to identify homogeneous writing styles and cluster them together for similarity analysis in the given corpus.
Each of these tasks are extensible depending on the kind of problem statement they are used for in the real world. Sometimes, these tasks overlap the objectives of each other.
These tasks are not limited to English as a language in automatic authorship analysis. Computerized applications are developed for other languages such as Greek, French, Dutch, Spanish and Italian.[2, 3]
References:
[1] Reddy, T. Raghunadha, B. Vishnu Vardhan, and P. Vijaypal Reddy. “A survey on authorship profiling techniques.” International Journal of Applied Engineering Research 11.5 (2016): 3092-3102.
[2] Stamatatos, Efstathios, et al. “Overview of the author identification task at PAN 2014.” CLEF 2014 Evaluation Labs and Workshop Working Notes Papers, Sheffield, UK, 2014. 2014.
[3] Stamatatos, Efstathios, et al. “Overview of the pan/clef 2015 evaluation lab.” International Conference of the Cross-Language Evaluation Forum for European Languages. Springer, Cham, 2015.
[4] Rangel, Francisco, et al. “Overview of the author profiling task at PAN 2013.” CLEF Conference on Multilingual and Multimodal Information Access Evaluation. CELCT, 2013.