Month: May 2018
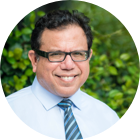
MMS • RSS
Article originally posted on Database Journal – Daily Database Management & Administration News and Tutorials. Visit Database Journal – Daily Database Management & Administration News and Tutorials
Oracle has decided that Solaris needs to retire, giving those who are running Oracle on Solaris roughly two years to move to another operating system. A possible choice involves commodity hardware (x86) and Linux. Since OEL is a ‘ready to use’ version of Red Hat Linux it would be a reasonable presumption that many companies will be choosing it as a Solaris replacement. Available as a full release for a bare-metal system and as a pre-configured VM it’s positioned to be a ‘go-to’ option for migration.
Solaris, on SPARC, is a big-endian operating system, where the most-significant byte is stored last. Linux, on the other hand, is a little-endian system where the bytes are stored in the order of significance (most-significant is stored first). This can make moving to Linux from Solaris a challenge, not for the software installation but for the database migration as the existing datafiles, logfiles and controlfiles can’t be used directly; they must be converted to the proper endian format. Time and space could be important issues with such conversions/migrations as there may be insufficient disk space to contain two copies of the database files, on big-endian and on little-endian. The actual conversion shouldn’t be difficult as RMAN can convert the files from a recent backup. Other considerations include access to the new servers (since they may not be on the production LAN while the build and conversions are taking place) and replicating the current Oracle environment, including scripts, NFS mounts, other shared file systems and utilities, from Solaris to Linux. RMAN isn’t the only method of migration as expdp/impdp can be used to transfer users, tables and data from the source database to its new home; using datapump means the destination database can’t be rolled forward after the import has completed, which can cause a longer outage than the enterprise may want to endure since all user access to the application data must be stopped prior to the export.
Looking at the ‘worst-case scenario’ let’s proceed with the migration using Oracle Enterprise Linux and the datapump transfer of data and tables. (Installing the software should be a fairly simple task so that portion of the migration will not be covered here.) Once the server is running and Oracle is installed the first step in this migration is to create an “empty” Oracle database as the destination. This takes care of the endian issue since the new datafiles are created with the proper endian format. If the Linux server is created with the same file system structure as the source then it’s simply a matter of performing the import and checking the results to verify nothing went awry. Any tnsnames.ora files that applications use for connectivity need to be modified to point to the replacement database server and remote connections need to be tested to verify they do, indeed, work as expected. After successful application testing has completed the database and its new ‘home’ should be ready to replace the original Solaris machines.
It’s possible that the new Linux server will use a different storage configuration; it’s also possible that the DBA team, in an effort to simplify tasks, decides to use Oracle Managed Files. In either case the import may not succeed since datapump can’t create the datafiles. This is when the tablespaces need to be created ahead of the datapump import; using the SQLFILE parameter to datapump import will place all of the DDL into the specified file. Once this file is created it can be edited to change the file locations or to use OMF when creating tablespaces. It’s critical that file sizes are sufficient to contain the data and allow for growth. Datapump will create users if the necessary tablespaces exist so the only DDL that should be necessary to run prior to the import will be the CREATE TABLESPACE statements; all other DDL should be removed prior to running the script. It’s also possible to create a DBCA template to create the destination database by modifying an existing template. Creating the database manually or with a template is a DBA team decision; in the absence of database creation scripts that can be modified it might be a good decision to modify an existing template to minimize errors.
Presuming the storage configuration for the x86 system is different from that on Solaris, and that the file system structure doesn’t match the source server, the tablespace DDL has been extracted, modified and is ready to run. After the tablespaces are in place the import can be run; it is always good practice to make a test run into the new database before the final import is executed, to verify that the process runs smoothly. The final import should put the new database in ‘proper working order’ for the applications that use it so all insert/update/delete activity must be stopped prior to the export. This will ensure data consistency across the tables.
One area that may be an issue is application account passwords in the new database. It’s a good idea to verify that the passwords from the source database work in the new database. If not, they can be reset to the current values; end-users and batch jobs will be happier if logins are successful.
Connectivity to remote systems is also critical, so any tnsnames.ora files that are in use on the source system need to be copied to the destination system and database links need to be tested. This may involve the security and system administration teams to open ports, set firewall rules and ensure that any software packages not included with the operating system are installed. There should be no surprises once the new server and database are brought online.
Other choices, such as Unified Auditing or Database Vault, that require an Oracle kernel relink need to be discussed and decided upon before the destination database is created. Re-linking the Oracle kernel before any database exists reduces overall downtime for the migration.
No document can cover every conceivable issue or problem that may arise so it’s possible the first pass at moving from Solaris to x86 may reveal issues that weren’t obvious at the beginning of this process. It may also take more than one pass to “iron out” all of the “kinks” to get a smoothly running process. The time and effort expended to ensure a smoothly running migration will pay off handsomely when the day finally arrives.
Moving from Solaris to x86 (or any other operating system) may not be at the top of your wish list, but it will become a necessity when Solaris is no longer supported. Getting a start on this now will provide the needed time to fix any issues the migration may suffer so that when the fateful weekend arrives the DBA team is ready to provide a (mostly) painless move.
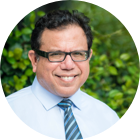
MMS • RSS
Article originally posted on BigML. Visit BigML
Unless you’ve been hiding under a rock, you’ve probably heard of the Cambridge Analytica Scandal and Mark Zuckerberg’s statements about the worldwide changes Facebook is making in response to European Union’s General Data Protection Regulation (GDPR). If your business is not yet in Europe, you may be taken aback by the statement from U.S. Senator […]
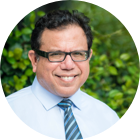
MMS • RSS
Article originally posted on MongoDB. Visit MongoDB
NEW YORK, May 23, 2018 /PRNewswire/ — MongoDB, Inc. (NASDAQ: MDB), the leading modern, general purpose database platform, today announced it will report its first quarter fiscal year 2019 financial results for the three months ended April 30, 2018, after the U.S. financial markets close on Wednesday, June 6, 2018.
In conjunction with this announcement, MongoDB will host a conference call on Wednesday, June 6, 2018, at 5:00 p.m. (Eastern Time) to discuss the Company’s financial results and business outlook. A live webcast of the call will be available on the “Investor Relations” page of the Company’s website at http://investors.mongodb.com. To access the call by phone, dial 800-239-9838 (domestic) or 323-794-2551 (international). A replay of this conference call will be available for a limited time at 844-512-2921 (domestic) or 412-317-6671 (international) using conference ID 8152278. A replay of the webcast will also be available for a limited time at http://investors.mongodb.com.
About MongoDB
MongoDB is the leading modern, general purpose database platform, designed to unleash the power of software and data for developers and the applications they build. Headquartered in New York, MongoDB has more than 5,700 customers in over 90 countries. The MongoDB database platform has been downloaded over 35 million times and there have been more than 850,000 MongoDB University registrations.
Investor Relations
Brian Denyeau
ICR for MongoDB
646-277-1251
ir@mongodb.com
Media Relations
MongoDB, North America
866-237-8815 x7186
communications@mongodb.com
View original content with multimedia:http://www.prnewswire.com/news-releases/mongodb-inc-announces-date-of-first-quarter-fiscal-2019-earnings-call-300653632.html
SOURCE MongoDB
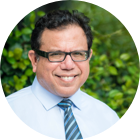
MMS • RSS
Article originally posted on Data Science Central. Visit Data Science Central
Summary: Researchers in Synthetic Neuro Biology are proposing to solve the AGI problem by building a brain in the laboratory. This is not science fiction. They are virtually at the door of this capability. Increasingly these researchers are presenting at major AGI conferences. Their argument is compelling.
If you step outside of all the noise around AI and the hundreds or even thousands of startups trying to add AI to your car, house, city, toaster, or dog you can start trying to figure out where all this is going.
Here’s what I think we know:
- The narrow and pragmatic DNN approaches to speech, text, image, and video are getting better all the time. Transfer learning is making this a little easier.
- Reinforcement learning is coming along as are GANNs and those will certainly help. Commercialization is a few years away.
- The next generation of neuromorphic (spiking) chips are just entering commercial production (BrainChip, EtaCompute) and those will result in dramatic reductions in training datasets, training time, size, and energy consumption. In addition, we hope they can learn from one system and apply it to another.
But what about artificial general intelligence (AGI) that we all believe will be the end state of these efforts? When will we get AGI that brings fully human capabilities, learns like a human, and can adapt knowledge like a human?
How Far Away is AGI?
When I looked into this two years ago the range of estimates was around 2025 to 2040.
With a few years more experience under our belt, here are the estimates given by 7 leading thinkers and investors in AI (including Ben Goertzel and Steve Jurvetson) at a 2017 conference on Machine Learning at the University of Toronto when asked ‘How far away is AGI’.
- 5 years to subhuman capability
- 7 years
- 13 years maybe (By 2025 we’ll know if we can have it by 2030)
- 23 years (2040)
- 30 years (2047)
- 30 years
- 30 to 70 years
There’s significant disagreement but the median is 23 years (2040) with half the group thinking longer. Sounds like we’re learning that this may be harder than we think.
What’s the Most Likely Path to AGI?
Folks who think about this say that everything we’ve got today in DNNs and reinforcement learning is by definition ‘weak’ AI. That is it mimics some elements of human cognition but doesn’t achieve it in the same way humans do.
This may or may not also be true of next gen Spiking neural nets that have adapted some new elements from neuroscience research. They look like they’re a step in the right direction but we really don’t know yet.
There is general agreement that weak AI, while commercially valuable will never give us AGI. Only if we create broad and strong AI systems that mimic human reasoning can we ever achieve AGI.
Is this all on the Same Incremental Pathway?
So far there have been two primary schools of thought.
The Top Down school is an extension of our current incremental engineering approach. Basically it says that once the sum of all these engineering problems is resolved the resulting capabilities will in fact be AGI.
Those who disagree however say that truly human-like intelligence can never be the result of simply adding up a group of specific algorithms. Human intelligence could never be reduced to the sum of mathematical parts and neither can AGI.
The Bottom Up school is the realm of researchers who propose to build a silicon analogue of the entire human brain. They propose to build an all-purpose generalized platform based on an exact simulation of human brain function. Once it’s available it will immediately be able to do everything our current piecemeal approach has accomplished and much more.
Personally, my bet is on the Bottom Up school though I think we are learning valuable hardware and software lessons along the way from our pragmatic DNN approach.
How About a Radical New Path
What I discovered in revisiting all this is that my own thinking has been too constrained. For example, in writing about 3rd gen spiking neural nets or the neuromorphic modeling approach of Jeff Hawkins at Numenta I assumed that hardware and software modeling of individual neurons interacting was the agreed approach.
Not only is this not true (I’ll write more about this later), but our fundamental assumption about working in silicon is not the only approach being explored.
Folks like neuroscientist George Church at Harvard are proposing that we simply build a brain in the biology lab and train it to do what we want it to.
Computational Synthetic Biology (CSB) – Wetware
Computational Synthetic Biology (aka synthetic neuro biology) is much further along than you think, and in terms of a 25 year forward timeline might just be the first horse to the finish line of AGI.
As far back as 10 years ago, the field of Systems Biology sought to reduce molecular and atomic level cellular activities to ‘bio-bricks’ that could be strung together with different ‘operators’ to achieve an understanding of how these processes worked.
If Systems Biology is about understanding nature as it is, Computational Synthetic Biology takes the next step to understand nature as it could be.
Here’s a graphic of the Infobiotics Workbench from 2010. Anyone familiar with predictive modeling will immediately recognize the similarities with a data analytics dashboard, including setting hyperparameters, loss functions, and comparing champion models. Source: 2010 GECCO Conference Tutorial on Synthetic Biology by Natalio Krasnogor, University of Nottingham.
Fast forward to the 2018 O’Reilly Artificial Intelligence Conference in New York where Harvard researcher George Church tells us just how much further we’ve come. (See his original presentation here. Graphics that follow are from that presentation.)
Synthetic neuro biology (SNB) is already ahead of silicon simulations in both energy (much lower) and computational efficiency. Capabilities are increasing exponentially faster than Moore’s Law, in some years by a factor of 10X.
In the upper right, SNB is already operating close to the biological limit of compute efficiency. Church says silicon could catch up in 3 decades at the current growth rate, except that silicon is already plateauing.
Church says “We are well on our way to reproducing every kind of structure in the brain”.
In the lab, he can already create all types of neurons to order including being able to build significant human cerebral cortex structures complete with supporting vasculature. This includes myelin wrapping of the axons so that signals can be sent over long distances at high speeds allowing action potentials to jump from node to node without signal dissipation.
In short, the position of SNB researchers is it’s easier to copy an unknown (brain function, human cognition, AGI) in a made-to-order biological brain than it is to translate that simulation into silicon. In the silicon simulation you’ll never really know if it’s actually right.
To add one more level to these futuristic projections, what is the possibility that we can modify or augment our current physiology to create super intelligence? The field of biological augmentation is already well underway principally in the field of curing disease. Why not extend it?
For example, the average human brain weighs 1.3 kg and consumes 20W of power (compared to the 85,000W required by Watson to win Jeopardy). What if we could enlarge it to 25kg, about 3X the size of a whale’s brain, but still within the physical limit of what our skeletons could support? It seems the only penalty would be to have to provide about 100W of power, equivalent to eating about 8,000 calories per day. Donuts – yumm.
Could Silicon Based AGI Ever Be Completely Human-Like?
While silicon AGI modelers still struggle to understand brain function sufficiently to build their simulations there are legitimate questions about whether their best result will ever be enough. Will they ever have the capabilities that make us philosophically human? Our science fiction robots have some or all of these qualities:
Consciousness: To have subjective experience and thought.
Self-awareness: To be aware of oneself as a separate individual, especially to be aware of one’s own thoughts and uniqueness.
Sentience: The ability to feel perceptions or emotions subjectively.
Sapience: The capacity for wisdom.
If we construct an intelligent ‘brain’ in the lab from the same human biological components – is it still just a simulation? Will it be a ‘mind’?
So far computational synthetic biological research remains in the lab or where it has matured, is being applied to cure disease. But increasingly researchers like George Church are presenting at major AGI conferences. They are already able to skip the entire step of creating a silicon analog of the brain where most silicon AGI researchers are currently stuck. If there’s a 15 or 25 year runway to develop AGI, I wouldn’t bet against this wetware approach.
Other articles on AGI:
In Search of Artificial General Intelligence (AGI) (2017)
Artificial General Intelligence – The Holy Grail of AI (2016)
Other articles by Bill Vorhies.
About the author: Bill Vorhies is Editorial Director for Data Science Central and has practiced as a data scientist since 2001. He can be reached at:
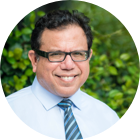
MMS • RSS
Article originally posted on Database Journal – Daily Database Management & Administration News and Tutorials. Visit Database Journal – Daily Database Management & Administration News and Tutorials
Introduction
Adaptive query processing is the latest, improved query processing feature introduced in the SQL Server database engine. This method is available in SQL Server (starting with SQL Server 2017 (14.x)) and Azure SQL Database.
Query performance is always a subject of research and innovation. Every so often, Microsoft includes new feature and functionality in SQL Server to improve query performance to help their users. In SQL Server 2016, Microsoft introduced Query Store. Query Store monitors query performance and provides methods to choose better query plans for execution. Query store is a feature to persist query execution plans and analyze the history of query plans to identify the queries that can cause performance bottlenecks. Any point in time these plans can be reviewed, and the user can use plan forcing to enforce the query processor to select and use a specific query plan for execution. You can read more about Query Store in Monitor Query Performance Using Query Store in SQL Server.
In SQL Server 2017, Microsoft went one step further and worked on improving the query plan quality. This improved query plan quality is Adaptive Query Processing.
Query Execution Plan
Prior to SQL Server 2017, query processing was a uniform process with a certain set of steps. The SQL Server query optimizer first generates a set of feasible query plans for a query or batch of T-SQL code submitted by the database engine to query optimizer. Based on the cost of the query plan, optimizer selects the lowest-cost query plan.
Once the query execution plan is ready and available to execute, SQL Server storage engines get the query plan and execute it based on the actual query plan and return the data set.
This end to end process (query plan generation, and submitting the plan for processing) once started, has to run to completion. Please see Understanding a SQL Server Query Execution Plan for more information.
In this process, query performance depends on the overall quality of the query plan, join order, operation order and selection of physical algorithm like nested loop join or hash join, etc. Also, cardinality estimates play a vital role to decide the quality of the query plan. Cardinality represents the estimated number of rows returned on execution of a query.
The accuracy of cardinality drives a better quality of query execution plan and more accurate allocation of memory, CPU to the query. This improvement leads to a better and improved execution of query, known as adaptive query processing. We will discuss exactly how SQL Server achieves this in rest of the section,.
Adaptive Query Processing
Prior to SQL Server 2017, the behavior of Query Optimizer was not optimal; it bound to select the lowest cost query plan for execution, despite poor initial assumptions in estimating inaccurate cardinality, which led to bad query performance.
In Adaptive Query Processing, SQL Server optimized the query optimizer to generate more accurate and quality query plans with more accurate cardinality. Adapting Query Processing gets a better-informed query plan. There are three new techniques for adapting to application workload characteristics.
Adapting Query Processing Techniques
- Batch Mode Memory Grant Feedback. This technique helps in allocating the required memory to fit in all returning rows. The right allocation of memory helps in, 1) reducing excessive memory grants that avoid concurrency issues and, 2) fixing underestimated memory grants that avoid expensive spills to disk.
Query performance suffers when memory allocation sizes are not sized correctly. In Batch Mode Memory Grant Feedback, optimizer repeats the workload and recalculates the actual memory required for a query and then updates the grant value for the cached plan. When an identical query statement is executed, the query uses the revised memory grant size and improves the query performance.
- Batch Mode Adaptive Join. This technique provides the choice of algorithm selection and the query plan dynamically switches to a better join strategy during plan execution. This defers either hash join or nested loop join selection until after first input has been scanned.
The new operator in the family, Adaptive Join operator helps in defining a threshold. This threshold is used to compare with the row count of the build join input; if the row count of the build join input is less than the threshold, a nested loop join would be better than a hash join and query plan switches to nested loop join. Otherwise, without any switches query plan continues with a hash join.
This technique mainly helps the workload with frequent fluctuations between small and large join input scans.
- Interleaved Execution. Interleaved execution is applicable to multi-statement table valued functions (MSTVFs) in SQL Server 2017 because MSTVFs have a fixed cardinality guess of “100” in SQL Server 2014 and SQL Server 2016, and an estimate of “1” for earlier versions, which leads to passing through inaccurate cardinality estimates to the query plan. In Interleaved execution technique, whenever SQL Server identifies MSTVFs during the optimization process, it pauses the optimization, executes the applicable subtree first, gets accurate cardinality estimates and then resumes optimization for rest of the operations. This technique helps in getting actual row counts from MSTVFs instead of fixed cardinality estimates to make plan optimizations downstream from MSTFs references; this results in improvement in workload performance. Also, Interleaved execution enables plans to adapt based on the revised cardinality estimates as MSTVFs changes the unidirectional boundary of single-query execution between the optimization and execution phase.
Additional facts for Adaptive Query Processing Techniques
There are certain facts associated with each Adaptive Query Processing technique while optimizer improves the query performance using these techniques.
Batch Mode Memory Grant Feedback. Feedback is not persisted once a plan is evicted from cache. You will not be able to get the history of the query plan in Query Store, as Memory Grant Feedback will change only the cached plan and changes are currently not captured in the Query Store. Also, in case of failover, feedback will be lost.
Batch Mode Adaptive Join. SQL Server can dynamically switch between the two types of joins and always choose between nested loop and hash join; merge join is not currently part of the adaptive join. Also, adaptive joins introduce a higher memory requirement than an indexed nested loop Join equivalent plan.
Interleaved Execution. There are some restrictions; 1) the MSTVFs must be read-only, 2) the MSTVFs cannot be used inside of a CROSS APPLY operation, 3) the MSTVFs are not eligible for interleaved execution if they do not use runtime constants.
Configure Adapting Query Processing
There are multiple options to configure Adaptive Query Processing. These options are as follows:
Option-1: SQL Server by default enables Adaptive Query Processing and executes the queries under compatibility level 140.
Option-2: T-SQL is available to enable and disable all three Adaptive Query Processing techniques:
T-SQL to enable Adaptive Query Processing techniques
-
ALTER DATABASE SCOPED CONFIGURATION SET DISABLE_BATCH_MODE_MEMORY_GRANT_FEEDBACK = ON;
-
ALTER DATABASE SCOPED CONFIGURATION SET DISABLE_BATCH_MODE_ADAPTIVE_JOINS = ON;
-
ALTER DATABASE SCOPED CONFIGURATION SET DISABLE_INTERLEAVED_EXECUTION_TVF = ON;
T-SQL to disable Adaptive Query Processing techniques
-
ALTER DATABASE SCOPED CONFIGURATION SET DISABLE_BATCH_MODE_MEMORY_GRANT_FEEDBACK = OFF;
-
ALTER DATABASE SCOPED CONFIGURATION SET DISABLE_BATCH_MODE_ADAPTIVE_JOINS = OFF;
-
ALTER DATABASE SCOPED CONFIGURATION SET DISABLE_INTERLEAVED_EXECUTION_TVF = OFF;
Option-3:Enforce Adaptive Query Processing technique using query hint. Query hint takes precedence over a database scoped configuration and other settings.
-
OPTION (USE HINT ('DISABLE_BATCH_MODE_MEMORY_GRANT_FEEDBACK'));
-
OPTION (USE HINT('DISABLE_BATCH_MODE_ADAPTIVE_JOINS'));
-
OPTION (USE HINT('DISABLE_INTERLEAVED_EXECUTION_TVF'));
Summary
The adaptive query processing improves the quality of a query plan. This helps in selecting the right join, right order of operation and more accurate memory allocation to fit all rows. The three techniques of adaptive query processing make SQL Server 2017 significantly faster at processing the workload. Also, adaptive query processing provides significant improvements without refactoring T-SQL code.
Overall, adaptive query processing is a great addition in SQL Server and enables SQL Server to generate well informed quality query plans to manage application workload processing.
OptiML Webinar Video is Here: Automatically Find the Optimal Machine Learning Model!
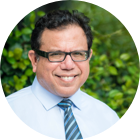
MMS • RSS
Article originally posted on BigML. Visit BigML
The latest BigML release has brought OptiML to our platform, and it is now available from the BigML Dashboard, API, and WhizzML. This new resource automates Machine Learning model optimization for all knowledge workers to lower even more the barriers for everyone to adopt Machine Learning. OptiML is an optimization process for model selection and parametrization that […]
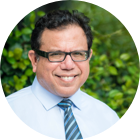
MMS • RSS
Article originally posted on BigML. Visit BigML
One click and you’re done, right? That’s the promise of OptiML and automated Machine Learning in general, and to some extent, the promise is kept. No longer do you have to worry about fiddly, opaque parameters of Machine Learning algorithms, or which algorithm works best. We’re going to do all of that for you, trying various things in […]
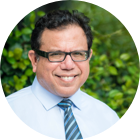
MMS • RSS
Article originally posted on BigML. Visit BigML
This blog post, the fifth of our series of posts about OptiML, focuses on how to programmatically use this resource with WhizzML, BigML’s Domain Specific Language for Machine Learning workflow automation. To refresh your memory, WhizzML allows you to execute complex tasks that are computed completely on the server side with built-in parallelization. BigML Resource Family Grows […]
2ML Underscores the Need to Adopt Machine Learning in all Businesses and Organizations
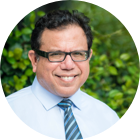
MMS • RSS
Article originally posted on BigML. Visit BigML
More than 300 attendees and 20 international speakers didn’t miss the second edition of 2ML: Madrid Machine Learning. #2ML18 gathered mostly decision makers and Machine Learning practitioners coming from six different countries: the US, Canada, China, Uruguay, Holland, Austria, and of course Spain, as the event was held in Madrid. The opening remarks were given […]
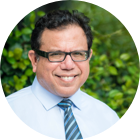
MMS • RSS
Article originally posted on Database Journal – Daily Database Management & Administration News and Tutorials. Visit Database Journal – Daily Database Management & Administration News and Tutorials
Sometimes it’s desired to move data from production to test or development environments, and if done with the original exp/imp utilities, issues can arise since these were written for database versions older than 9.x. Those utilities don’t support features found in newer database versions, which can create performance problems.
Tables with Top-n or hybrid histograms, when exported with exp, won’t get those histograms replicated to the destination database; both Top-n and hybrid histograms will be converted to Frequency histograms. Looking at a table in 12.1.0.2 (from an example by Jonathan Lewis) let’s see what histograms are present:
COLUMN_NAME Distinct HISTOGRAM Buckets
-------------------- ------------ --------------- ----------
FREQUENCY 100 FREQUENCY 100
TOP_N 100 TOP-FREQUENCY 95
HYBRID 100 HYBRID 50
Using legacy exp the table is exported. Importing this into another 12.1.0.2 database using legacy imp the histogram types have changed:
COLUMN_NAME Distinct HISTOGRAM Buckets
-------------------- ------------ --------------- ----------
FREQUENCY 100 FREQUENCY 100
TOP_N 100 FREQUENCY 95
HYBRID 100 FREQUENCY 50
Note that the Oracle release is the same in both databases; it’s the exp/imp utilities creating the problem. Using datapump to transfer the data would have preserved the histograms. If there are scripts in use that use these old utilities it’s probably time to rewrite them to take advantage of datapump export and import.
It’s sometimes easier to use what’s already written but in the case of conventional export and import it’s time to retire these scripts when using Oracle releases that support datapump.