Month: December 2021
Global Big Data Analytics in Telecom Market Size 2021-2028, By Types, Applications & Top …
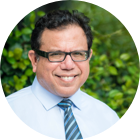
MMS • RSS
Posted on mongodb google news. Visit mongodb google news
The recent report on “Big Data Analytics in Telecom Market Report 2021 by Key Players, Types, Applications, Countries, Market Size, Forecast to 2028” offered by Credible Markets, comprises of a comprehensive investigation into the geographical landscape, industry size along with the revenue estimation of the business. Additionally, the report also highlights the challenges impeding market growth and expansion strategies employed by leading companies in the “Big Data Analytics in Telecom Market”.
Key Benefits for Stakeholders
- The report provides a quantitative analysis of the current Big Data Analytics in Telecom market trends, estimations, and dynamics of the market size from 2015 to 2028 to identify the prevailing opportunities.
- Porter’s five forces analysis highlights the potency of buyers and suppliers to enable stakeholders to make profit-oriented business decisions and strengthen their supplier–buyer network.
- In-depth analysis and the market size and segmentation assists to determine the prevailing Big Data Analytics in Telecom market opportunities.
- The major countries in each region are mapped according to their revenue contribution to the market.
- The market player positioning segment facilitates benchmarking and provides a clear understanding of the present position of the market players in the Big Data Analytics in Telecom industry.
Big Data Analytics in Telecom Market: Competition Landscape
The Big Data Analytics in Telecom market report includes information on the product launches, sustainability, and prospects of leading vendors including: (, Microsoft Corporation, MongoDB, United Technologies Corporation, JDA Software, Inc., Software AG, Sensewaves, Avant, SAP, IBM Corp, Splunk, Oracle Corp., Teradata Corp., Amazon Web Services, Cloudera)
Click the link to get a free Sample Copy of the Report @ https://crediblemarkets.com/sample-request/big-data-analytics-in-telecom-market-243501?utm_source=AkshayT&utm_medium=SatPR
Big Data Analytics in Telecom Market: Segmentation
Big Data Analytics in Telecom market is split by Type and by Application for the period 2021-2028, the growth among segments provides accurate artifices and forecasts for sales by Type and by Application in terms of volume and value. This analysis can help you expand your business by targeting qualified niche markets.
Market segment by Type, covers
Cloud-based
On-premise
Market segment by Application, can be divided into
Small and Medium-Sized Enterprises
Large Enterprises
Big Data Analytics in Telecom Market: Regional Analysis
All the regional segmentation has been studied based on recent and future trends, and the market is forecasted throughout the prediction period. The countries covered in the regional analysis of the Global Big Data Analytics in Telecom market report are U.S., Canada, and Mexico in North America, Germany, France, U.K., Russia, Italy, Spain, Turkey, Netherlands, Switzerland, Belgium, and Rest of Europe in Europe, Singapore, Malaysia, Australia, Thailand, Indonesia, Philippines, China, Japan, India, South Korea, Rest of Asia-Pacific (APAC) in the Asia-Pacific (APAC), Saudi Arabia, U.A.E, South Africa, Egypt, Israel, Rest of Middle East and Africa (MEA) as a part of Middle East and Africa (MEA), and Argentina, Brazil, and Rest of South America as part of South America.
Direct Purchase this Market Research Report Now @ https://crediblemarkets.com/reports/purchase/big-data-analytics-in-telecom-market-243501?license_type=single_user;utm_source=AkshayT&utm_medium=SatPR
Major Points Covered in TOC:
Market Overview: It incorporates six sections, research scope, significant makers covered, market fragments by type, Big Data Analytics in Telecom market portions by application, study goals, and years considered.
Market Landscape: Here, the opposition in the Worldwide Big Data Analytics in Telecom Market is dissected, by value, income, deals, and piece of the pie by organization, market rate, cutthroat circumstances Landscape, and most recent patterns, consolidation, development, obtaining, and portions of the overall industry of top organizations.
Profiles of Manufacturers: Here, driving players of the worldwide Big Data Analytics in Telecom market are considered dependent on deals region, key items, net edge, income, cost, and creation.
Market Status and Outlook by Region: In this segment, the report examines about net edge, deals, income, creation, portion of the overall industry, CAGR, and market size by locale. Here, the worldwide Big Data Analytics in Telecom Market is profoundly examined based on areas and nations like North America, Europe, China, India, Japan, and the MEA.
Application or End User: This segment of the exploration study shows how extraordinary end-client/application sections add to the worldwide Big Data Analytics in Telecom Market.
Market Forecast: Production Side: In this piece of the report, the creators have zeroed in on creation and creation esteem conjecture, key makers gauge, and creation and creation esteem estimate by type.
Research Findings and Conclusion: This is one of the last segments of the report where the discoveries of the investigators and the finish of the exploration study are given.
Do You Have Any Query Or Specific Requirement? Ask to Our Industry Expert @ https://crediblemarkets.com/enquire-request/big-data-analytics-in-telecom-market-243501?utm_source=AkshayT&utm_medium=SatPR
Key questions answered in the report:
- What will the market development pace of Big Data Analytics in Telecom market?
- What are the key factors driving the Global Big Data Analytics in Telecom market?
- Who are the key manufacturers in market space?
- What are the market openings, market hazard and market outline of the market?
- What are sales, revenue, and price analysis of top manufacturers of Big Data Analytics in Telecom market?
- Who are the distributors, traders, and dealers of Big Data Analytics in Telecom market?
- What are the Big Data Analytics in Telecom market opportunities and threats faced by the vendors in the Global Big Data Analytics in Telecom industries?
- What are deals, income, and value examination by types and utilizations of the market?
- What are deals, income, and value examination by areas of enterprises?
About US
Credible Markets is a new-age market research company with a firm grip on the pulse of global markets. Credible Markets has emerged as a dependable source for the market research needs of businesses within a quick time span. We have collaborated with leading publishers of market intelligence and the coverage of our reports reserve spans all the key industry verticals and thousands of micro markets. The massive repository allows our clients to pick from recently published reports from a range of publishers that also provide extensive regional and country-wise analysis. Moreover, pre-booked research reports are among our top offerings.
The collection of market intelligence reports is regularly updated to offer visitors ready access to the most recent market insights. We provide round-the-clock support to help you repurpose search parameters and thereby avail a complete range of reserved reports. After all, it is all about helping you reach an informed strategic decision about purchasing the right report that caters to all your market research demands.
Contact Us
Credible Markets Analytics
99 Wall Street 2124 New York, NY 10005
Email: [email protected]
Article originally posted on mongodb google news. Visit mongodb google news
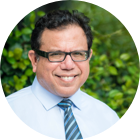
MMS • RSS
Posted on nosqlgooglealerts. Visit nosqlgooglealerts

This is a contributed article by Patrick McFadin, Vice President Developer Relations, DataStax.
Whatever metaphor you prefer to use – from oil, gold, nuclear waste, or even bacon – the role for data in business is not going down. Companies want to use data that they have, and they want it to power the applications and services that make them money. However, data is not simple. The services used for long term analytics are different to those that power a business.
While a lot of attention and funding has gone into that analytics side of the market, the fast data side will be where the biggest changes take place in 2022.
Prediction #1 – 2022 will be the year we hear the words “Legacy NoSQL”
After being the next big thing for years, NoSQL has now been around long enough to see long-term installations. With anything in IT, there’s always going to be a discussion about migrating to the latest technology. In this case, seeing the world of cloud and cloud native data, IT teams will be debating what the right choices are for them to run on for the next ten years.
In those meetings taking place in 2022, there will be some interesting new language when somebody says “Legacy NoSQL” when talking about existing systems that don’t run natively in Kubernetes. Mainstream NoSQL will be tighter aligned with cloud native applications, and there will be more development work taking place around how NoSQL databases run as part of those cloud deployments.
Prediction #2 – Cloud providers will blink on egress pricing
For as long as cloud providers have been in business, there has been lopsided pricing around data coming in and going out. Data coming in is usually free, which was a great way to drive adoption into the clouds. Data leaving cloud providers are where the real costs started racking up. Again, to drive not only adoption but incentivise users to keep data inside the cloud.
Cracks have started to form on this business plan. Cloudflare boldly announced no egress fees on up to one terabyte of data, while a handful of smaller providers charge a flat fee on bandwidth. AWS responded to this by upping its free tier for data egress from a measly one gigabyte to 100 gigabytes.
Just like how storage became the weapon in a price war, we’ll see egress and ingress fees becoming much lower and normalised on a race to the bottom in 2022. Will this make more companies consider alternative cloud providers, or will it make AWS more popular with cost-conscious customers? This will remain to be seen.
Prediction #3 – Kubernetes will spread beyond applications and single cluster deployments
The Kubernetes cluster is a popular destination for cloud native applications. By design, that cluster can be an island with very tight security and controls for what comes in and out. Use cases now require more than one cluster to be involved in the total application.
This has created a lot of discussion and movement in the Kubernetes project around how to bridge multiple clusters. Meanwhile, more Kubernetes clusters are getting pushed to the edge. This is all pointing to one thing. 2022 is when Kubernetes breaks free of the single cluster norm.
In practice, during 2022, more applications will get deployed with that distributed model in mind from centre to edge. This will also force more companies to look at how they adopt distributed data approaches that do the same thing – put the data next to the application, whether this is one cloud or multiple ones, or in the centre out to the edge.
Prediction #4 – A newly renamed Facebook will enter the cloud infrastructure market
If you missed it, Facebook has renamed to Meta to serve as an umbrella for many sub-brands. This new company is also going to be heavily focused on VR/AR which they feel is a huge growth area of the future. When it comes to being an infrastructure company, Facebook has one of the largest footprints in the world. It wouldn’t be a stretch to see them offer cloud services to developers building VR applications.
In a real 2022 surprise, Meta will announce their cloud platform and quickly become the number four horse in the race with a huge head start.
Prediction #5 – Data and privacy regulations will explode
After years of people being angry at social media platforms and technology in general, government regulation around the world will finally catch up with public opinion. The US state of California has adopted data privacy rules based on the European Union’s General Data Protection Regulation, while the EU has extended its work to areas like open data and artificial intelligence.
All this work is necessary for privacy, but the result will be a very complicated regulatory maze for anyone building data driven applications. Which means to say, everyone. Data regulation has not kept up with the sophistication of privacy issues that constantly come up. Just this year, we saw private industry try to self-regulate with a battle between Apple and Facebook. At the speed of government, this was too little too late.
2022 will be the beginning of a multi-year journey through new regulations and deadlines. To keep up with this, companies will want to keep control over where their data gets deployed, how this data is managed, and how they use it for their business. This fast data approach will rely on an open-source stack to work.
Patrick McFadin is the Vice President of Developer Relations at DataStax, where he leads a team devoted to making users of DataStax products successful. He has also worked as Chief Evangelist for Apache Cassandra and consultant for DataStax, where he helped build some of the largest deployments in production. Previous to DataStax, he was Chief Architect at Hobsons and an Oracle DBA/developer for over 15 years.
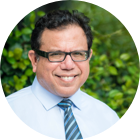
MMS • Johan Janssen
Article originally posted on InfoQ. Visit InfoQ

Neo4j Labs has released Neo4j Migrations, a database migration and refactoring tool that offers version control for relational databases. Inspired by FlywayDB, Neo4j Migrations depends on the Neo4j Java Driver and ClassGraph which is used to detect migrations on the classpath.
This tool may be used as a CLI via the neo4j-migrations
command, after downloading the CLI binary from the GitHub releases page. Binaries are available for both Windows and Unix-like operating systems. Homebrew offers an installation for macOS:
brew install michael-simons/homebrew-neo4j-migrations/neo4j-migrations
A JVM-based version is also available for download and it’s the only one supporting Java-based migrations with the --package
argument.
Neo4j Migrations offers two options to apply migrations or any other changes to Neo4j. Cypher-based migrations are written down as Cypher statements, where a ‘;
’ separates each statement. The .cypher
files in classpath:neo4j/migrations
are automatically detected and each file is executed in a single transaction. Other locations on the classpath or filesystem may be specified as follows:
MigrationsConfig configLookingAtDifferentPlaces = MigrationsConfig.builder()
.withLocationsToScan(
"classpath:[custompath]",
"file:/[custompath]"
).build();
Alternatively, Java-based migrations may be used, which offer more flexibility as Java logic can be used to change anything in the database by implementing the JavaBasedMigration
interface:
import ac.simons.neo4j.migrations.core.JavaBasedMigration;
import ac.simons.neo4j.migrations.core.MigrationContext;
import org.neo4j.driver.Driver;
import org.neo4j.driver.Session;
public class CustomMigration implements JavaBasedMigration {
@Override
public void apply(MigrationContext context) {
try (Session session = context.getSession()) {
…
}
}
}
A Maven plugin is available to trigger the database migration via the build, instead of using the CLI, by supplying the following configuration:
<plugin>
<groupId>eu.michael-simons.neo4j</groupId>
<artifactId>neo4j-migrations-maven-plugin</artifactId>
<version>[version]</version>
<executions>
<execution>
<id>migrate</id>
<goals>
<goal>migrate</goal>
</goals>
<configuration>
<user>[username]</user>
<password>[password]</password>
<address>[address]</address>
<verbose>true</verbose>
</configuration>
</execution>
</executions>
</plugin>
The plugin automatically detects the migrations within neo4j/migrations
. Other directories may be specified with:
<locationsToScan>
<locationToScan>file://${project.build.outputDirectory}/[custom-path]</locationToScan>
</locationsToScan>
Alternatively, the Spring Boot starter may be used to trigger the migration by configuring the dependency:
<dependency>
<groupId>eu.michael-simons.neo4j</groupId>
<artifactId>neo4j-migrations-spring-boot-starter</artifactId>
<version>[version]</version>
</dependency>
The starter uses the Neo4j Java Driver which can be configured directly when using Spring Boot 2.4 or newer. The starter doesn’t scan by default for Java-based migrations and uses classpath:neo4j/migrations
as the default directory for Cypher statements. Various properties are available to configure the starter, such as:
spring.neo4j.authentication.username=[username]
spring.neo4j.authentication.password=[location]
spring.neo4j.uri=[uri]
# To configure additional locations with migrations
org.neo4j.migrations.packages-to-scan=location1, location2
Spring Boot supports Neo4j tests with the @DataNeo4jTest
annotation and can be used together with the migrations by supplying the following configuration:
@Testcontainers(disabledWithoutDocker = true)
@DataNeo4jTest
@ImportAutoConfiguration(MigrationsAutoConfiguration.class)
public class CustomTest {
@Container
private static Neo4jContainer<?> neo4j = new Neo4jContainer<>("neo4j:4.2")
.withReuse(TestcontainersConfiguration.getInstance().environmentSupportsReuse());
@DynamicPropertySource
static void neo4jProperties(DynamicPropertyRegistry registry) {
registry.add("spring.neo4j.uri", neo4j::getBoltUrl);
registry.add("spring.neo4j.authentication.username", () -> "neo4j");
registry.add("spring.neo4j.authentication.password", neo4j::getAdminPassword);
}
@Test
void yourTest(@Autowired Driver driver) {
…
}
}
Sebastian Daschner explains in his blog and the accompanying video how the CLI may be used to migrate Neo4j graph schemas in Kubernetes by using init containers.
The complete documentation including all the configuration options is available on GitHub and also contains several example projects.
Java News Roundup: More Log4Shell Statements, Spring and Quarkus Updates, New Value Objects JEP
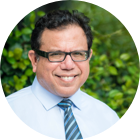
MMS • Michael Redlich
Article originally posted on InfoQ. Visit InfoQ

This week’s Java roundup for December 20th, 2021, features news from OpenJDK with a new draft on value objects, JDK 18, JDK 19, Project Loom, additional statements from vendors on Log4Shell, numerous Spring and Quarkus updates, Hibernate ORM 6.0.0-M3, point releases from Apache Camel and Camel Quarkus, Apache Tika 2.2.1 and GraalVM Native Build Tools 0.9.9.
OpenJDK
A new JEP draft, Value Objects (Preview), was added to the list this past week. This new preview language and VM feature proposes to enhance the Java object model with value types defined as identity-free value classes and specifying the behavior of their instances. These classes contain only final instance fields and have no object identity.
JDK 18
Build 29 of the JDK 18 early access builds was made available this past week, featuring updates from Build 28 that include fixes to various issues. More details may be found in the release notes.
JDK 19
Build 3 of the JDK 19 early-access builds was also made available this past week, featuring updates from Build 2 that include fixes to various issues.
For JDK 18 and JDK 19, developers are encouraged to report bugs via the Java Bug Database.
Project Loom
Build 19-loom+1-11 of the Project Loom early-access builds was made available to the Java community and is based on Build 2 of the JDK 19 early access builds.
Additional Vendor Statements to Log4Shell Vulnerability
Oracle statement on Helidon:
By default Log4j is not used by Helidon based applications and does not appear on the classpath. However Helidon provides an optional Log4j integration module (
helidon-logging-log4
) and Helidon manages the version of Log4j.If your application uses
helidon-logging-log4
, or if your application uses Log4j directly then your application will have declared an explicit dependency on Log4j. But the version of this dependency might be managed by Helidon.
JetBrains statement on Third-party Plugins within the JetBrains Marketplace
Because of how many IntelliJ-based plugins there are, we initially used API Watcher to check what plugins and which of their exact versions used anything from log4j. We have temporarily hidden all plugin versions in which we detected any use of log4j.
We understand that such a check can produce some false positives. But we’d rather play it extra safe and draw the attention of many plugin authors to the potential risks, rather than miss some plugins that have repackaged log4j.
Spring Framework
It was a very busy week over at Spring as there were a number of point releases with various Spring projects.
There were two point releases on the Spring Boot 2.6 and 2.5 release trains:
Version 2.6.2 features 55 bug fixes and documentation improvements along with many dependency upgrades such as Log4j 2.17.0, Kotlin 1.6.10, Hibernate 5.6.3.Final and multiple Spring-related projects.
Version 2.5.8 features 46 bug fixes and documentation improvements along with many dependency upgrades such as Log4j 2.17.0, Kotlin 1.5.32, Hibernate 5.4.33 and multiple Spring-related projects.
Spring Cloud Square 0.4.0 has been released featuring: support for providing adjustments on non-load-balanced use cases; the ability to configure different WebClient.Builder
beans for each instance of a Retrofit client annotated with @RetrofitClient
; and integration of Spring Cloud Sleuth for an instance of the OkHttpClient
class.
Spring Native 0.11.1 was made available to include 13 bug fixes and documentation improvements along with a dependency upgrade to Spring Boot 2.6.2.
Spring Integration 5.5.7 has been released featuring a number of bug fixes. More details may be found in the migration guide (for potential breaking changes) and the changelog.
Point releases for Spring Security versions 5.2.15, 5.3.13, 5.4.10, 5.5.4 and 5.6.1 were made available this past week featuring bug fixes and minor improvements.
Quarkus
Quarkus 2.5.4.Final, a maintenance release, features a dependency upgrade to Jackson 2.12.6 to fix a possible denial-of-service attack in Jackson Databind. Further details may be found in the changelog.
Quarkus 2.6.0.Final has been released featuring: dependency upgrades to SmallRye Reactive Messaging 3.13, Kafka 3.0, Kotlin 1.6 and Camel 3.14; extensions, such as Neo4J, Amazon Alexa and Reactive Messaging HTTP having been moved to the Quarkiverse Hub; a new programmatic API for caching; and a smaller Docker image, based on UBI Micro, for native executables. This release did not include the fixes from Quarkus 2.5.4.Final, but were included in Quarkus 2.6.1.Final.
Quarkus 2.6.1.Final, the last maintenance release of 2021, includes all the fixes from Quarkus 2.5.4.Final and some dependency upgrades. More details may be found in the changelog.
Hibernate
The third beta release of Hibernate ORM 6.0 was made available this past week featuring new annotations: @IdGeneratorType
allows configuration of the IdentifierGenerator
interface in a type-safe way; @TenantId
uses column-based multi-tenancy to mark an attribute that defines the tenant; and @AttributeBinderType
allows customization of how mapping of an attribute is defined. Further details may be found in the Hibernate 6.0 user guide and migration guide.
Apache Camel
The Apache Software Foundation has provided the last point release in the Camel 3.7 release train. Version 3.7.7 features seven bug fixes and improvements, and dependency upgrades to Logback 1.2.8, Log4j 2.16.0, and the camel-nsq
and camel-corda
components. More details may be found in the release notes.
Maintaining alignment with Quarkus, Apache also released Camel Quarkus 2.6.0 containing Camel 3.14.0, Quarkus 2.6.0.Final and JFR native support.
Apache Tika
Apache Tika has released version 2.2.1 of their metadata extraction toolkit. Formerly a subproject of Apache Lucene, this latest version includes an upgrade to Log4j 2.17.0 and a critical fix for an Office Open XML (docx
/pptx
/xlsx
) regression that was introduced in version 2.2.0. Further details may be found in the release notes.
GraalVM Native Build Tools
On the road to version 1.0, Oracle Labs has released version 0.9.9 of Native Build Tools, a GraalVM project consisting of plugins for interoperability with GraalVM Native Image. This latest release provides fixes for the Gradle plug-in, namely: leniency with the resource detection mechanism if a classpath entry is missing; a proper native inference task associated with custom binaries, i.e., binaries outside of the main
and test
binaries; and a rework on the operation of disabling toolchain detection.
The Zacks Analyst Blog Highlights: Microsoft, Workiva, MongoDB and Datadog – Yahoo Finance
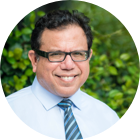
MMS • RSS
Posted on mongodb google news. Visit mongodb google news
For Immediate Release
Chicago, IL – December 27, 2021 – Zacks.com announces the list of stocks featured in the Analyst Blog. Every day the Zacks Equity Research analysts discuss the latest news and events impacting stocks and the financial markets. Stocks recently featured in the blog include: Microsoft Corporation MSFT, Workiva Inc. WK, MongoDB, Inc. MDB and Datadog, Inc. DDOG.
Here are highlights from Thursday’s Analyst Blog:
4 Big Data Stocks that Could Explode in 2022
Rapid digitization and technological advancement have led to the creation of an enormous volume of data from diverse sources. It is becoming difficult for traditional data processing solutions to harness, process and provide insights in real-time.
This has paved the way for big data concepts as an effective way for decision-making in today’s business world. The adoption of big data analytics is likely to witness tremendous growth owing to accelerated digitization amid the ongoing coronavirus-led crisis, thereby leading to increased investments in the space. Thus, industry players like Microsoft, Workiva, MongoDB and Datadog should gain in 2022 and beyond.
Big Data Analytics Transforming Businesses
Big data along with other analytical tools helps companies in handling large volumes of data and use them to achieve valuable insights in many areas, including in-depth customer research, cost management, product development and competitive analysis.
Big data analytics also provides operational intelligence, making troubleshooting far more convenient for organizations. The technology enhances the quick decision-making capabilities of companies, thereby helping them increase their business performances and generate better revenues.
The applications of big data analytical tools are useful across every industry. Retailers use big data analytics in understanding customer behavior, buying patterns, improving customer experience and making more personalized recommendations. This helps companies in improving purchase rates in stores as well as on online platforms.
Big data analytics helps media companies, especially video and music streaming services providers, make their platforms more user-friendly so that consumers conveniently find the content that suits their liking.
The banking industry uses the big data concept in minimizing risks and fraud. In the manufacturing sector, faster problem-solving boosts productivity.
Impressive Prospects for Big Data Market
The adoption of big data analytics is growing rapidly owing to vast volumes of data being created and consumed worldwide. The pandemic has led to an accumulation of data in bulk over the past year as the majority of the global population is working, learning, and entertaining themselves from home.
According to an Expert Market Research report, the global big data market was worth $208 billion in 2020 and is expected to reach $450 billion by 2026 at a compounded annual growth rate (CAGR) of 10% during the 2021-2026 forecast period.
Another market research firm, Allied Market Research, expects the global big data market to witness a CAGR of 13.5% during 2021-2030 and reach $684.12 billion in 2030 from $198.08 billion in 2020.
Investments in big data analytics software, hardware and services are only expected to grow ahead due to rapid digitization across industries and the rise in the number of smartphones and apps. Advancements in artificial intelligence and machine learning are major catalysts as well. Additionally, growth in 5G and Internet of Things technologies has accelerated the amount of everyday data, thereby fueling the demand for big data analytics.
Therefore, investors who seek to make the most of this rising trend should consider investing in big data companies.
Investors can tap this burgeoning market by keeping track of the following stocks.
Microsoft: The company’s Azure HDInsight platform is a managed, full-spectrum, open-source analytics service in the cloud for enterprises. The platform is designed to simplify analyzing large volumes of data spread across several computer structures. The Azure HDInsight cloud platform can run on several popular open-source frameworks including Apache Spark, Apache Hive, Apache Kafka, Apache Storm and Hadoop.
Currently, Microsoft carries a Zacks Rank #3 (Hold). The Zack Consensus Estimate for its fiscal 2022 earnings has moved up by a penny over the past seven days. Shares of MSFT have surged 50.8% year to date (YTD). You can see the complete list of today’s Zacks #1 Rank (Strong Buy) stocks here.
Workiva: It offers a cloud-based and mobile-enabled platform for enterprises to collect, manage, report and analyze critical business data in real-time. Workiva’s software helps organizations streamline complex business and reporting processes by linking teams, documents and data from initial sources to final reports.
Workiva carries a Zacks Rank #3 at present. The Zacks Consensus Estimate for its current-year earnings has been revised upward to 30 cents per share from a loss of 7 cents per share estimated 60 days ago. Shares of WK have gained 44.3% YTD.
MongoDB: The company operates as a general-purpose database platform that helps companies gather insight with ease, thus creating organizational value. MongoDB’s NoSQL database can support many big data systems, both in real-time operations as well as in offline capacities.
Currently, MongoDB carries a Zacks Rank #3. For fiscal 2022, the Zacks Consensus Estimate has improved to a loss of 73 cents per share from $1.17 per share projected 30 days ago. Shares of MDB have increased 46.6% YTD.
Datadog: It offers a monitoring and analytics platform for developers, IT operations teams and business users in the cloud age. The company’s business runs around its portfolio of over 400 out-of-the-box integrations including public cloud, private cloud, on-premise hardware, databases and third-party software.
Datadog carries a Zacks Rank #3 at present. The Zacks Consensus Estimate for its 2021 earnings has been revised upward by 48% to 40 cents per share over the past 60 days. Shares of DDOG have gained 67.3% YTD.
Media Contact
Zacks Investment Research
800-767-3771 ext. 9339
support@zacks.com
https://www.zacks.com
Past performance is no guarantee of future results. Inherent in any investment is the potential for loss. This material is being provided for informational purposes only and nothing herein constitutes investment, legal, accounting or tax advice, or a recommendation to buy, sell or hold a security. No recommendation or advice is being given as to whether any investment is suitable for a particular investor. It should not be assumed that any investments in securities, companies, sectors or markets identified and described were or will be profitable. All information is current as of the date of herein and is subject to change without notice. Any views or opinions expressed may not reflect those of the firm as a whole. Zacks Investment Research does not engage in investment banking, market making or asset management activities of any securities. These returns are from hypothetical portfolios consisting of stocks with Zacks Rank = 1 that were rebalanced monthly with zero transaction costs. These are not the returns of actual portfolios of stocks. The S&P 500 is an unmanaged index. Visit https://www.zacks.com/performance for information about the performance numbers displayed in this press release.
Want the latest recommendations from Zacks Investment Research? Today, you can download 7 Best Stocks for the Next 30 Days. Click to get this free report
Microsoft Corporation (MSFT) : Free Stock Analysis Report
Workiva Inc. (WK) : Free Stock Analysis Report
MongoDB, Inc. (MDB) : Free Stock Analysis Report
Datadog, Inc. (DDOG) : Free Stock Analysis Report
To read this article on Zacks.com click here.
Zacks Investment Research
Article originally posted on mongodb google news. Visit mongodb google news
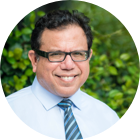
MMS • RSS
Article originally posted on Data Science Central. Visit Data Science Central
Okay, so I am still confused by the concept of a Data Mesh (I’m a slow learner).
Recently, I wrote a blog that posed the question: Are Data Meshes the Enabler of the Marginal Propensity to Reuse? The ability to engineer data and analytic assets that can be shared, reused, and continuously refined is the heart of what makes data (and analytics) the most valuable resource in the world. And if Data Meshes can make this happen, then it’s a winner in my eyes.
A Data Mesh is composed of three separate components: data sources, data infrastructure, and domain-oriented data pipelines managed by business function owners. Underlying the data mesh architecture is a layer of universal interoperability, reflecting domain-agnostic standards, as well as observability and governance (Figure 1).
Figure 1: What is a Data Mesh?
A data mesh supports a distributed, domain-specific data structure that views “data-as-a-product,” with each domain handling their own data pipelines. The tissue connecting these domains and their associated data assets is a universal interoperability layer that applies the same syntax and data standards[1].
Wait. This sounds very familiar:
- Is a distributed, domain-specific data structure the same as business process-specific data marts?
- Is the universal interoperability layer the same as the Enterprise Data Warehouse Bus Architecture?
Sounds like a Data Mesh is a modern-day version of Business Process-centric Data Marts connected with Conformed Dimensions and the Enterprise Data Warehouse Bus Architecture. Heck, that’s something that Ralph Kimball and Margy Ross developed and have been teaching for over 30 years. They literally wrote the book(s) on this – starting with the “The Data Warehouse Toolkit: The Definitive Guide to Dimensional Modeling” – in the 1990’s.
What can we learn from the work that Ralph and Margy pioneered, and the countless organizations that have successfully implemented these business process-centric data marts connected with conformed dimensions and the enterprise data warehouse bus architecture?
Lesson #1: Incrementally Build Business Process-centric Data Marts
A Data Mart is a collection of related fact and dimension tables that is typically derived from a single business process data source. A data mart is a business process-oriented data repository that is focused on a single business process such as orders, shipments, and payments (Figure 2).
Figure 2: Business Process-centric Data Mart Architecture
The Data Mart approach tackles one business process at a time, so it’s an incremental approach rather than trying to build the Data Warehouse all at once. And any organization that wants to analyze those business process’s data should be involved in the design (e.g., both Marketing and Sales would be involved in the design of the orders data mart).
The Data Mart approach overcomes the concerns with the big bang enterprise data warehouse approach where the enterprise data warehouse needs to first be built before organizations can start deriving value from their data.
Important Note: data marts are tied to business processes, not departments or business units. A business process is a set of activities (e.g., orders, sales, payments, returns, procurement, logistics, repairs) that accomplish a specific organizational goal and from which operational data is generated and business process performance is monitored and reported. And it is at the business process level that data quality and data governance can be managed.
Lesson #2: Connect Business Process-centric Data Mart with Conformed Dimensions and the Bus Architecture
The key to linking or federating the business process-oriented data marts are the conformed dimensions. Conformed Dimensions are common, standardized, master (file) dimensions (e.g., Customers, Products, Suppliers, Stores, Employees, Campaigns) that are managed once in the ETL or ELT processes (data pipelines) and then reused or federated across multiple business process fact tables. The benefit of conformed dimensions is that they enforce consistent descriptive attributes across the fact tables; provides a common enterprise glossary to avoid terminology inconsistencies and organizational confusion.
Conformed Dimensions enable cross enterprise analysis with ability to drill across and integrate data from different business processes. Also, reusing conformed dimensions shortens time-to-market by eliminating redundant design and development efforts.
Note: One key aspect of the conformed dimensions approach is that the enterprise bus architecture is based on “business processes,” not business departments or business units. Business processes are the organization’s business measurement events, like orders, shipments, payments, etc. (Figure 3).
Figure 3: Conformed Dimensions and Enterprise Data Warehouse Bus Architecture
Conformed Dimensions are underpinned with the Enterprise Data Warehouse Bus Architecture, which enables the decomposition of an organization’s data and analytics strategy into manageable pieces by focusing on the organization’s core business processes, along with the associated conformed dimensions.
The Conformed Dimensions approach enables the business process data to be stored once in an organization (with dimensions that are shared across measurement events) rather than Sales having their copy of orders data and Finance having their version of orders data and Logistics having their copy. If you store data by business department or business unit, then you’ll likely end up multiple departmental or business unit versions of the truth.
The Enterprise Data Warehouse Bus Matrix is a key design tool representing the organization’s core business processes and associated dimensionality. It’s the architectural blueprint that provides a top-down strategic perspective to ensure data from the different business processes can be integrated across the enterprise, while agile bottom-up delivery occurs by focusing on a single business process at a time (Figure 4).
Figure 4: Enterprise Data Warehouse Bus Matrix
So, upon closer inspection, it sure does seem that the Data Mesh is really the next generation of business process-specific data marts interconnected using conformed dimensions and the enterprise data warehouse bus architecture.
Lesson #3: Importance of Master Data Management (MDM)
In a world of conformed dimensions and data meshes, master data management becomes the key connecting tissue. Master data management is a business discipline in which business and information technology work together to ensure the uniformity, accuracy, stewardship, semantic consistency, and accountability of the enterprise’s official shared master data assets.
From the Master Data Management guru and author of the seminal book “Telling Your Data Story: Data Storytelling for Data Management”, Scott Taylor, we get the following about the critical role of master data management in a world of business process-centric data marts interconnected with conformed dimensions.
Whatever the socio-technological impacts of a mesh or interconnected architecture, the data still needs to be mastered somehow, governed always, and trusted everywhere across the enterprise. The need for master data, reference data, and metadata is macro-architecture agnostic.
Master data is tangible outcome. Mesh proponents might debate MDM as an approach but it’s hard to make a case against having master, reference, and meta data. The data mesh approach calls for unique identifiers on entities to link shared data across systems. That sure sounds like master data to me!
It is an absolute requirement for data management and data monetization success that there be tight coordination across the different business processes with respect to master data management and data governance.
Summary: Data Meshes equals Data Marts with Conformed Dimensions?
I feel a bit like Marty McFly and heading “Back to the Future” based upon the work that I was doing with Ralph, Margy, and Bob Becker 20+ years ago. But business process-centric data marts interconnected with enterprise governed conformed dimensions and master data management works. And now the concept of Data Meshes promises to take advantage of modern-day technology advances to refresh the advantages of a business process approach to data warehousing, data management, master data management, and data governance.
Let’s hear it, Huey Lewis…
[1] Source: “Data Mesh 101: Everything You Need To Know to Get Started”, Monte Carlo Data
Mini book: The InfoQ eMag: Paths to Production: Deployment Pipelines as a Competitive Advantage
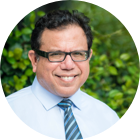
MMS • InfoQ
Article originally posted on InfoQ. Visit InfoQ

Enabling developers to push code to production at an ever-increasing velocity has become a competitive advantage. By rapidly deploying applications, companies can easily keep up with changes in the business and surrounding market and thus maintain competitiveness. Automating deployments allow developers to reduce errors, increase productivity, and deploy more frequently.
In the early days, deployments from development environments to production were predominantly a manual process or consisted of utilizing a chain of custom scripts. Both developers and operations teams had to spend a lot of time on laborious manual chores like code testing and release. With the introduction of continuous integrations and deployments capabilities through tooling, this process became more automated. Moreover, pipelines were introduced with the primary purpose of keeping the software development process organized and focused.
Over the years, pipelines became more sophisticated and more critical for companies’ IT departments that went through digital transformations. More software became available online as services, APIs, and products requiring a quick update and maintenance cycle. Furthermore, companies embraced the DevOps processes around pipelines and – once applied correctly – gained a competitive advantage, according to the research from the “Accelerate” book by Nicole Forsgren, Jez Humble, and Gene Kim.
In this eMag, you will be introduced to the paths to production and how several global companies supercharge developers and keep their competitiveness by balancing speed and safety. We’ve hand-picked three full-length articles to showcase that.
We would love to receive your feedback via editors@infoq.com or on Twitter about this eMag. I hope you have a great time reading it!
Free download
Big Data Analytics in Telecom Market Impressive Gains including key players Microsoft … – CyclicMint
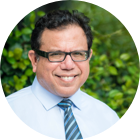
MMS • RSS
Posted on mongodb google news. Visit mongodb google news

A new research study from JCMR with title Global Big Data Analytics in Telecom Market Research Report 2021 provides an in-depth assessment of the Big Data Analytics in Telecom including key market trends, upcoming technologies, industry drivers, challenges, regulatory policies & strategies. The research study provides forecasts for Big Data Analytics in Telecom investments till 2029.
The report does include most recent post-pandemic market survey on Big Data Analytics in Telecom Market.
Competition Analysis : Microsoft Corporation, MongoDB, United Technologies Corporation, JDA Software, Inc., Software AG, Sensewaves, Avant, SAP, IBM Corp, Splunk, Oracle Corp., Teradata Corp., Amazon Web Services, Cloudera
Request Free PDF Sample Report @: jcmarketresearch.com/report-details/1492220/sample
Commonly Asked Questions:
- At what rate is the market projected to grow
The year-over-year growth for 2021 is estimated at XX% and the incremental growth of the Big Data Analytics in Telecom market is anticipated to be $xxx million.
Get Up to 40 % Discount on Enterprise Copy & Customization Available for Following Regions & Country: North America, South & Central America, Middle East & Africa, Europe, Asia-Pacific
- Who are the top players in the Big Data Analytics in Telecom market?
Microsoft Corporation, MongoDB, United Technologies Corporation, JDA Software, Inc., Software AG, Sensewaves, Avant, SAP, IBM Corp, Splunk, Oracle Corp., Teradata Corp., Amazon Web Services, Cloudera
- What are the key Big Data Analytics in Telecom market drivers and challenges?
The demand for strengthening ASW capabilities is one of the major factors driving the Big Data Analytics in Telecom market.
- How big is the North America Big Data Analytics in Telecom market?
The North America region will contribute XX% of the Big Data Analytics in Telecom market share
Enquiry for Big Data Analytics in Telecom segment [email protected] jcmarketresearch.com/report-details/1492220/enquiry
This customized Big Data Analytics in Telecom report will also help clients keep up with new product launches in direct & indirect COVID-19 related markets, upcoming vaccines and pipeline analysis, and significant developments in vendor operations and government regulations
Big Data Analytics in Telecom Geographical Analysis:
• Big Data Analytics in Telecom industry North America: United States, Canada, and Mexico.
• Big Data Analytics in Telecom industry South & Central America: Argentina, Chile, and Brazil.
• Big Data Analytics in Telecom industry Middle East & Africa: Saudi Arabia, UAE, Turkey, Egypt and South Africa.
• Big Data Analytics in Telecom industry Europe: UK, France, Italy, Germany, Spain, and Russia.
• Big Data Analytics in Telecom industry Asia-Pacific: India, China, Japan, South Korea, Indonesia, Singapore, and Australia.
Market Analysis by Types & Market Analysis by Applications are as followed:
Segment by Type – Cloud-based – On-premise Segment by Application – Small and Medium-Sized Enterprises – Large Enterprises
Some of the Points cover in Global Big Data Analytics in Telecom Market Research Report is:
Chapter 1: Overview of Global Big Data Analytics in Telecom Market (2013-2025)
• Big Data Analytics in Telecom Definition
• Big Data Analytics in Telecom Specifications
• Big Data Analytics in Telecom Classification
• Big Data Analytics in Telecom Applications
• Big Data Analytics in Telecom Regions
Chapter 2: Big Data Analytics in Telecom Market Competition by Players/Suppliers 2013 and 2018
• Big Data Analytics in Telecom Manufacturing Cost Structure
• Big Data Analytics in Telecom Raw Material and Suppliers
• Big Data Analytics in Telecom Manufacturing Process
• Big Data Analytics in Telecom Industry Chain Structure
Chapter 3: Big Data Analytics in Telecom Sales (Volume) and Revenue (Value) by Region (2013-2021)
• Big Data Analytics in Telecom Sales
• Big Data Analytics in Telecom Revenue and market share
Chapter 4, 5 and 6: Global Big Data Analytics in Telecom Market by Type, Application & Players/Suppliers Profiles (2013-2021)
• Big Data Analytics in Telecom Market Share by Type & Application
• Big Data Analytics in Telecom Growth Rate by Type & Application
• Big Data Analytics in Telecom Drivers and Opportunities
• Big Data Analytics in Telecom Company Basic Information
Continue……………
Note: Please Share Your Budget on Call/Mail We will try to Reach your Requirement @ Phone: +1 (925) 478-7203 / Email: [email protected]
Find more research reports on Big Data Analytics in Telecom Industry. By JC Market Research.
Thanks for reading this article; you can also get individual chapter wise section or region wise report version like North America, Europe or Asia.
About Author:
JCMR global research and market intelligence consulting organization is uniquely positioned to not only identify growth opportunities but to also empower and inspire you to create visionary growth strategies for futures, enabled by our extraordinary depth and breadth of thought leadership, research, tools, events and experience that assist you for making goals into a reality. Our understanding of the interplay between industry convergence, Mega Trends, technologies and market trends provides our clients with new business models and expansion opportunities. We are focused on identifying the “Accurate Forecast” in every industry we cover so our clients can reap the benefits of being early market entrants and can accomplish their “Goals & Objectives”.
Contact Us: https://jcmarketresearch.com/contact-us
JCMARKETRESEARCH
Mark Baxter (Head of Business Development)
Phone: +1 (925) 478-7203
Email: [email protected]
Connect with us at – LinkedIn
Article originally posted on mongodb google news. Visit mongodb google news
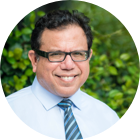
MMS • RSS
Posted on nosqlgooglealerts. Visit nosqlgooglealerts
The NoSQL Databases Software Market research report includes Market segmentation and overlays shadow upon the leading market players highlighting the favourable competitive landscape and trends prevailing over the years. This study provides information about the sales and revenue during the historic and forecasted period of 2021 to 2030. Understanding the segments helps in identifying the importance of different factors that aid the NoSQL Databases Software market growth.
Global NoSQL Databases Software Market: Regional Analysis
The NoSQL Databases Software report offers in-depth assessment of the growth and other aspects of the NoSQL Databases Software market in important regions. Key regions covered in the report are North America, Europe, Asia-Pacific and Latin America.
Download Sample Report on “NoSQL Databases Software Market” @ jcmarketresearch.com/report-details/1494638/sample
The NoSQL Databases Software report has been curated after observing and studying various factors that determine regional growth such as economic, environmental, social, technological, and political status of the particular region. Analysts have studied the data of revenue, production, and manufacturers of each region. This section analyses region-wise revenue and volume for the forecast period of 2020 to 2030 for NoSQL Databases Software market. These analyses will help the reader to understand the potential worth of investment in a particular region into NoSQL Databases Software market.
Global NoSQL Databases Software Market: Competitive Landscape
This section of the NoSQL Databases Software report identifies various key manufacturers of the market. It helps the reader understand the strategies and collaborations that players are focusing on combat competition in the NoSQL Databases Software market. The comprehensive NoSQL Databases Software report provides a significant microscopic look at the NoSQL Databases Software market. The reader can identify the footprints of the manufacturers by knowing about the global NoSQL Databases Software revenue of manufacturers, during the forecast period of 2020 to 2030.
Get Discount on NoSQL Databases Software full report @ jcmarketresearch.com/report-details/1494638/discount
Major Key Points of NoSQL Databases Software Market
- NoSQL Databases Software Market Overview
- NoSQL Databases Software Market Competition
- NoSQL Databases Software Market, Revenue and Price Trend
- NoSQL Databases Software Market Analysis by Application
- Company Profiles and Key Figures in NoSQL Databases Software Market
- Market Dynamics for NoSQL Databases Software market
- Methodology and Data Source for NoSQL Databases Software market
Companies Profiled in this NoSQL Databases Software report includes: MongoDB, Amazon, ArangoDB, Azure Cosmos DB, Couchbase, MarkLogic, RethinkDB, CouchDB, SQL-RD, OrientDB, RavenDB, Redis
Segment by Type
– Cloud Based
– Web Based
Segment by Application
– Large Enterprises
– SMEs
Leading market players and manufacturers are studied to help give a brief idea about them in the NoSQL Databases Software report. The challenges faced by them and the reasons they are on that position is explained to help make a well informed decision. Competitive landscape of NoSQL Databases Software market is given presenting detailed insights into the company profiles, developments, merges, acquisitions, economic status and best SWOT analysis.
NOTE: Our team is studying Covid-19 and its impact on various industry verticals and wherever required we will be considering Covid-19 analysis of NoSQL Databases Software markets and industries. Cordially get in touch for more details.
Purchase a Copy of this full NoSQL Databases Software research @ jcmarketresearch.com/checkout/1494638
About Us
jcmarketresearch.com is a global market research and consulting service provider specialized in offering wide range of business solutions to their clients including market research reports, primary and secondary research, demand forecasting services, focus group analysis and other services. We understand that how data is important in today’s competitive environment and thus, we have collaborated with industry’s leading research providers who works continuously to meet the ever-growing demand for market research reports throughout the year.
Contact Us:
Mark Baxter (Head of Business Development)
2985, cedar Brook drive, Decatur GA 30033
JC Market Research
phone: +1(925)478-7203
Email: [email protected]
Website: www.jcmarketresearch.com
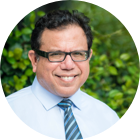
MMS • RSS
Article originally posted on Data Science Central. Visit Data Science Central
Web scraping deals with HTML almost exclusively. In nearly all cases, what is required is a small sample from a very large file (e.g. pricing information from an ecommerce page). Therefore, an essential part of scraping is searching through an HTML document and finding the correct information.
How that should be done is the matter of some debate, preferences, experience, and types of data. While all scraping and parsing methods are “correct”, some of them have benefits that may be vital when more optimization is required. Some methods may be easier for specific types of data. However, outside of a lone scientific study, succinct information on the differences and practicality of CSS Selectors, XPath, and RegEx is scarce.
Note: While most of the article is intended to be programming-language-agnostic, my foundation is in Python. Some assumptions or syntax might be lifted from it.
HTML through the eyes of scraping
Since in web scraping we’re mostly dealing with HTMLs documents that are made by someone else, we don’t need to go through the absolute basics to understand the following chapters. We can get away with just understanding HTML as an object.
When a web page is loaded in a browser, a Document Object Model (or DOM) is created. It is a tree-like object structure that contains all objects in the HTML. The Domain Object Model is a great way to visualize the website structure without worrying too much about any particular content.
Sample DOM. Source.
Since we’re using web scraping to wrestle out data (or content) from the website, we need to “tell” the application where the content is supposed to be. All three methods are, grossly simplified, different search functions used to cut through the structure.
CSS Selectors
Nearly every HTML will be served with CSS. It’s used for many ways of styling (e.g. text or image alignment, fonts, etc.) the content of an HTML file. CSS selectors are the way elements are, well, selected.
In web scraping, CSS Selectors is essentially a way to move from the root document to any particular element. However, the movement can only happen in that direction. Other methods, such as XPath, allow users to move bidirectionally.
Element selection happens based on CSS reference. For example, searching for “.text” would output all elements that contain “class=text” if any exist. Such a way to search HTML DOM is immensely useful for web scraping.
From our experience, CSS selectors are one of the fastest web parsing methods out of the three. At Oxylabs, we’ve used them quite a bit previously, however, with hardware and software capabilities quickly increasing, the speed benefits are getting smaller over time.
Additionally, CSS selectors are easy to maintain and manage. They are also supported by nearly every Python web scraping library, making it easier to transfer code and overall understanding if needed.
However, CSS selectors only allow one-directional flow, which greatly limits its capabilities. It cannot, for example, select a parent of an element. Every search has to be built from the top down to the particular element.
XPath
At Oxylabs, we primarily now use XPath. While it is theoretically slower, the differences between XPath and CSS are so minute that it won’t really have much of an impact. However, XPath provides much greater flexibility, especially for complex data structures.
XPath functions in a rather similar manner while being a bit bulkier in execution. For example, to find all elements that have “class=nice” instead of using “.nice”, XPath would use “//*[contains(@class, “nice”)]”. While XPath syntax is nearly always a bit more complex, it provides quite a few more features.
One of these beneficial features has been shown in the command “contains()”. It allows users to find partial matches within the HTML DOM. CSS selectors used to have “contains:”, however, that is no longer in use.
Additionally, XPath has “..” which allows the function to move towards ancestors. Thus, XPath avoids the limitation of CSS selectors, allowing users to move through the HTML DOM object with greater ease and freedom.
As mentioned previously, the primary and often cited drawback is the slower scraping speed. Yet, the differences usually are in several tens of milliseconds. It’s hard to think of a practical business case where such speed would make a difference.
RegEx
RegEx, or regular expression, is the final method. However, it’s not as practical for HTML as the other two as it’s used to search through strings. Yet, it’s still a great method for specific cases.
These cases are usually whenever the HTML files or elements are content heavy. Regular expressions work by being provided a pattern. Content is then matched to the pattern and an output is provided.
An example of a RegEx pattern in Python would be re.compile(r’^([A-Z]{1}.+?)(?:,)’) which would match all strings that start with one uppercase letter and are followed by any amount of characters until it runs into a comma. Essentially, it would find all items that are formulated into a list where elements start with an uppercase letter and end with a comma:
- John,
- Marty,
- Ellen,
The expression above should have hinted at the first weakness of RegEx. Whoever made the syntax for matching regular expressions seemed not to care about clarity. My example is actually one of the simpler searches as there are no special characters. Once ‘/’ and other special characters come into play, the syntax gets even messier. In turn, that makes it difficult to debug, especially in larger scripts.
Additionally, since in web scraping we’re extracting things from HTML files, it itself is one big string. RegEx doesn’t do as well once the language becomes structural and data from particular elements is required. Using CSS selectors or XPath is significantly easier in those cases.
At Oxylabs, we always use RegEx to find string matches as it’s universal. For that purpose, the method is excellent. However, we cover nearly everything else with XPath.
Conclusion
If you’re planning to embark on a web scraping journey or to optimize your current solution, I’d highly recommend learning XPath and RegEx as you would be able to gather data from nearly any source. CSS Selectors, on the other hand, are easy to use but limited in applicability.
Editorial Note: The debate between using CSS selectors vs. using XPath is one that has been going on for a long time. I made the argument, unsuccessfully, to incorporate XPath selectors into SVG behavior bindings specification all the way back in 2004. Unfortunately, like so much in the HTML world, the decision to turn away from XPath ended up putting a far weaker tool for node selection (CSS Selectors) in its place. I’m glad to see an intelligent use of XPath being recommended here, and gives me hope that XML isn’t quite as dead as some would like it to be – Kurt Cagle.