Category: InfoQ
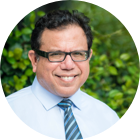
MMS • RSS
Article originally posted on InfoQ. Visit InfoQ
Christoph Erdmann recently detailed an interesting technique for image lazy loading using Progressive JPEG and HTTP range requests. Websites using the technique may quickly display a minimal, recognisable, embedded image preview (EIP) through a first HTTP range request, with a delayed, second range request fetching the rest. Unlike other image placeholder and lazy loading techniques, using range requests do not result in downloading extra image data.
Addy Osmani, Engineering Manager working on Google Chrome, quoted a study from the HTTP Archive revealing that images may account for more than half of the content loaded for the average site. Given the positive effect of images on conversion rates, that percentage is expected to remain significant.
In a context of devices with a large spectrum of hardware and network capabilities, and with an ever growing user base on low-end devices, web developers thus seek to optimize user experience with lazy image loading and other image optimization techniques. The importance of lazy loading is illustrated by the fact that it is now natively present in the Chrome browser since Chrome 74.
José M. Pérez, Solutions Engineer at Facebook, provides an infographics summarizing the existing image loading techniques:
The last strategy may present solid advantages vs. the alternatives in terms of user experience, and is often termed as LQIP (Low Quality Image Placeholder). A variant using SVG-based previews (SQIP) may also be leveraged to generate small, low-quality image previews.
However, common LQIP strategies often involve downloading two images, the preview and the actual image, resulting in extra data being fetched. Erdmann presents a strategy based on two HTTP range requests, which does not incur in data overhead.
Erdmann recommends leveraging progressive JPEG, a JPEG compression format in which an image is encoded in several passes, each successively leading to a more accurate display of the encoded image:
Erdmann mentions that the regular progressive JPEG approach comes with some caveats:
If your website consists of many JPEGs, you will notice that even progressive JPEGs load one after the other. This is because modern browsers only allow six simultaneous connections to a domain. Progressive JPEGs alone are therefore not the solution to give the user the fastest possible impression of the page. In the worst case, the browser will load an image completely before it starts loading the next one.
Erdmann’s remedy to these caveats is to use HTTP range requests. An initial HTTP request asks for the range of data which contains the first encoded pass of the Progressive JPEG. A second range request, scheduled by developers at a convenient time, may fetch the rest of the data.
As no preview image needs be created, no superfluous data needs be downloaded. Furthermore, images encoded with Progressive JPEG are generally not heavier than their baseline JPEG counterpart. On the downside, Cloudinary, a popular image and video platform, reports that decoding Progressive JPEG may result in higher CPU usage than with baseline JPEG encoding, and provides mitigating techniques which adjust the number and content of passes in the Progressive JPEG encoding.
Erdmann mentions three steps to implement his recommended image loading technique.
First, the Progressive JPEG must be created. This can be achieved for instance with jpegtran
:
$ jpegtran input.jpg > progressive.jpg
Second, the byte offset to determine the range of the two HTTP requests must be computed. Third, a JavaScript script must implement the lazy loading and perform and schedule the HTTP requests. The three steps may be performed manually by the developer or integrated in an automated workflow.
Erdmann provides a prototype for developers to experiment with his lazy loading technique. The prototype source code is available in a GitHub repository. Comments on the prototype are welcome and may be posted in the related blog post.
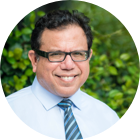
MMS • RSS
Article originally posted on InfoQ. Visit InfoQ
About the conference
Many thanks for attending Aginext 2019, it has been amazing! We are now processing all your feedback and preparing the 2020 edition of Aginext the 19/20 March 2020. We will have a new website in a few Month but for now we have made Blind Tickets available on Eventbrite, so you can book today for Aginext 2020.
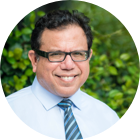
MMS • RSS
Article originally posted on InfoQ. Visit InfoQ
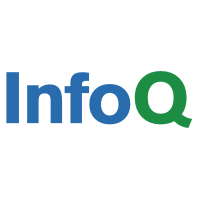
Microsoft is making the official specification for exFAT freely available in a move meant to make it possible to include an exFAT driver directly into the Linux kernel. Additionally, when an exFAT-enabled Linux kernel will be published, Microsoft will support its inclusion in the Open Invention Network’s Linux System Definition.
It’s important to us that the Linux community can make use of exFAT included in the Linux kernel with confidence. To this end, we will be making Microsoft’s technical specification for exFAT publicly available to facilitate development of conformant, interoperable implementations.
What Microsoft decision entails is the possibility of making an exFAT driver part of the Linux kernel, which was previously impossible due to Microsoft aggressive stance against Linux during the Ballmer era, and more recently to the non-inclusion of any exFAT-related patents into the patent non-aggression pact Microsoft signed when joining the Open Invention Network (OIN).
Instead, exFAT drivers have been available under Linux as FUSE modules, which means they run in userspace, or else they were implemented inside of the kernel but outside of the Linux mainline. This was the case for Android kernels, for example, but OEM were then obliged to license exFAT from Microsoft and pay the corresponding royalties.
Among the benefits of having exFAT available as a Linux kernel driver are its availability out-of-the-box, and better performance. According to Greg Kroah-Hartman, who submitted a patch to the Linux kernel adding support for exFAT, a kernel exFAT driver is much faster than its FUSE equivalent module. Specifically, FUSE modules have higher latency and worse IOPs, which is usually not a big issue on desktop-systems that have plenty of computing power and energy available but it is on embedded and IoT systems.
OIN is a mutual-defense organization that acquires patents and licenses them to its members for free. OIN members, in exchange, agree not to sue other members for their use of OIN patents in Linux-related systems. Presently, OIN has over 3,000 members, including major Linux distributions such as RedHat, Ubuntu, and SUSE. It is worth noting that neither Debian nor ArchLinux are listed among OIN members. Also, being OIN exclusively Linux-oriented, the inclusion of exFAT in the OIN Linux System Definition will not foreseeably bring any benefits to FreeBSD and other *BSD OSes.
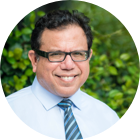
MMS • RSS
Article originally posted on InfoQ. Visit InfoQ
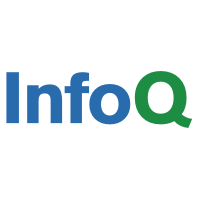
Spring Cloud has introduced a new framework called Spring Cloud App Broker which eases the development of Spring Boot applications that implement the Open Service Broker API, and the provisioning of those applications as managed services.
The Open Service Broker API enables developers to provide backing services to applications running on cloud native platforms like Kubernetes and Cloud Foundry. The key component of this API is the Service Broker. A Service Broker manages the lifecycle of services. The broker provides commands to fetch the catalog of services that it offers, provision and deprovision instances of the services, and bind and unbind applications to the service instances. Cloud native platforms can interact with the Service Broker to provision and gain access to the managed services offered by a Service Broker. For those familiar with OSGI, the Open Service Broker API is similar in spirit to the OSGI services, service references and service registry.
Spring Cloud App Broker is an abstraction layer on top of Spring Cloud Open Service Broker and provides opinionated implementations of the Spring Cloud Open Service Broker interfaces. The Spring Cloud Open Service Broker is itself a framework used to create Service Brokers and manage services on platforms that support the Open Service Broker API.
Spring Cloud App Broker can be used to quickly create Service Brokers using externalized configurations specified through YAML or Java Properties files. To get started, create a Spring Boot application and include the App Broker dependency to the project’s build file. For projects that use Gradle as the build tool, the following dependency should be added:
dependencies {
api 'org.springframework.cloud:spring-cloud-starter-app-broker-cloudfoundry:1.0.1.RELEASE'
}
For illustration, say we want to define and advertise a service that provides Natural Language Understanding (NLU) features like intent classification and entity extraction, include the Spring Cloud Open Service Broker configuration using properties under spring.cloud.openservicebroker as shown in this snippet:
spring:
cloud:
openservicebroker:
catalog:
services:
- name: mynlu
id: abcdef-12345
description: A NLU service
bindable: true
tags:
- nlu
plans:
- name: standard
id: ghijk-678910
description: A standard plan
free: true
Include the Spring Cloud App Broker configuration using properties under spring.cloud.appbroker as shown in this snippet:
spring:
cloud:
appbroker:
services:
- service-name: mynlu
plan-name: standard
apps:
- name: nlu-service
path: classpath:mynlu.jar
deployer:
cloudfoundry:
api-host: api.mynlu.com
api-port: 443
default-org: test
default-space: development
Utilizing these configurations, Spring Cloud App Broker will take care of advertising the NLU service, and it will manage the provisioning and binding of the service.
The project’s documentation describes the various service configuration options, customizations and deployment options. Note that as of now, Spring Cloud App Broker supports only Cloud Foundry as a deployment platform.
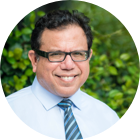
MMS • RSS
Article originally posted on InfoQ. Visit InfoQ
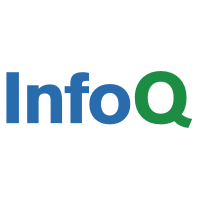
While leaving the language mostly unchanged, Go 1.13 brings a number of improvements to the toolchain, runtime, and libraries, including a relatively controversial default Go module proxy and Checksum database which requires developers to accept a specific Google privacy policy.
At the language level, Go 1.13 supports a more uniform set of prefixes for numeric literals, such as 0b
for binary numbers, 0x
for hexadecimal numbers, 0o
for octals, and so on; enables the use of signed shift counts with operators <<
and >>
, thus removing the need for uint
type casting when using them. Both changes do not break Go promise of source compatibility with previous versions of the language.
More extensive are the improvements to the toolchain, including some polish for go get
so it works more consistently in both module-aware and GOPATH mode; support for version suffix @patch
when getting a package; additional validation on the requested version string; improved analysis of which variables and expressions could be allocated on the stack instead of heap; and more.
In particular, the Go module system now supports out-of-the-box the module mirror and checksum database Google recently launched. The module mirror is aimed to improve go get
performance by implementing a more efficient fetching mechanism than what sheer version management systems such as Git provide, acting as a proxy to the package sources. For example, the module mirror does not require downloading the full commit history of a repository and provides an API more suited to package management, which includes operations to get a list of versions of a package, information about a package version, a mode
and zip file for a given version, and so on. The checksum database aims to ensure your packages are trusted. Prior to its introduction, the Go package manager used to ensure authenticity of a package using its go.sum
file, which basically contains a list of SHA-256 hashes. With this approach, the authenticity of a package is established on its first download, then checked against for each subsequent download. The checksum database is a single source of truth which can be used to ensure a package is legit even the first time it is downloaded by comparing its go.sum
with the information stored in the database.
This checksum database allows the go command to safely use an otherwise untrusted proxy. Because there is an auditable security layer sitting on top of it, a proxy or origin server can’t intentionally, arbitrarily, or accidentally start giving you the wrong code without getting caught.
The announcement of the new module mirror and checksum database sparked a reaction from developers worried about Google being able to track what packages developers use if they do not modify go get
default configuration:
This is the first language in my 23 years programming that reports my usage back to the language authors. It’s unprecedented.
Another complaint concerns the requirement to accept a specific Goggle privacy policy which could change at any time in future. It must be said that developers can disable go get
default behaviour by setting the following environment variables:
go env -w GOPROXY=direct
go env -w GOSUMDB=off
This is considered not robust enough, though, due to the possibility of those variables not being set by mistake, e.g., on a new machine, or being inadvertently cleared, e.g. when using su -
.
While the new module mirror and checksum database undoubtely improve Go module system performance and security, there is thus a chance their acceptance might not be very high among privacy-conscious developers and Google Go team review its approach by making their usage `off` by default or providing a more robust way to disable them, especially in environments such as enterprise where accepting Google privacy policy could entail some complication. InfoQ will keep reporting on this as new information becomes available.
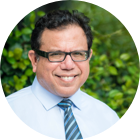
MMS • RSS
Article originally posted on InfoQ. Visit InfoQ
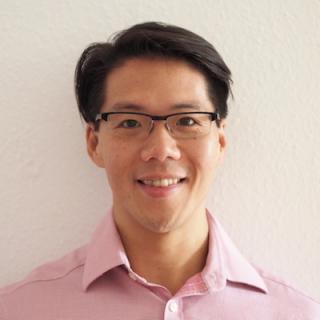
Cultivating organisational culture is much like gardening: you can’t force things, but you can set the right conditions for growth. Often, the most effective strategy is to clearly communicate the overall vision and goals, lead the people, and manage the systems and organisational structure.
Many software delivery organisations have technical and leadership career tracks, and employees can often switch between these as their personal goals change. However, this switch can be challenging, particularly for engineers who may have previously been incentivised to simply solve problems as quickly and cleanly as possible, and an organisation must support any transition with effective training.
Much like the values provided by Netflix’s Freedom and Responsibility model, there is a need for all organisations to balance autonomy and alignment. Managers can help their team by clearly defining boundaries for autonomy and responsibility. It is also valuable to define organisation values upfront. However, these can differ from actual culture, which more about what behaviours you allow, encourage, and stop.
In this podcast we discuss a holistic approach to technical leadership, and Pat provides guidance on everything from defining target operating models, cultivating culture, and supporting people in developing the career they would like. There are a bunch of great stories, several book recommendations, and additional resources to follow up on.
Mozilla Will Continue to Support Existing Ad Blockers Despite Google's Draft Extension Manifest V3
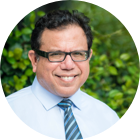
MMS • RSS
Article originally posted on InfoQ. Visit InfoQ
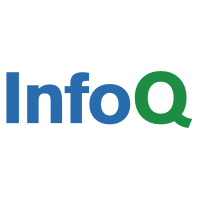
Mozilla will continue to support existing extensions which prevent ads from being displayed, unlike Google, which, in its draft Extensions Manifest v3 proposes changes to the browser extensions mechanism which may break ad-blockers.
Google proposed in early 2019 an update to its Extensions Manifest v2, with a view to alleviate security and privacy issues related to its Chrome browser extensions . The manifest defines the scope of capabilities of Chrome extensions.
Google points at the fact that, with Extensions Manifest V2’s Web Request API, any extension can silently have access to all of a user’s Chrome traffic data. 42% of malicious extensions reportedly do exploit the API for ill-intended purposes.
A notable change, thus, in the draft Extensions Manifest v3, is that extensions would no longer be allowed to leverage the blocking capabilities of the Web Request API, and would instead use a stricter Declarative Net Request API. With the new manifest, extensions can only monitor browser connections, but are no longer able to modify the content of the data before it is displayed.
Popular extensions like uBlock Origin and Ghostery make extensive use of the Web Request API to intercept ads and trackers before they are downloaded on the host computer. Other extensions, like Tampermonkey, are likely to be affected by the API changes.
In response to the initial comments from extension developers and its own testing, Google adjusted some of the parameters of the V3 API but the overall change proposal remains mostly stable. Google states:
[The new manifest] has been a controversial change since the Web Request API is used by many popular extensions, including ad blockers. We are not preventing the development of ad blockers or stopping users from blocking ads. Instead, we want to help developers, including content blockers, write extensions in a way that protects users’ privacy.
Mozilla’s Caitlin Neiman, community manager for Mozilla Add-ons (extensions), announced that Firefox will continue to support the blocking webRequest
API:
We have no immediate plans to remove
blocking webRequest
and are working with add-on developers to gain a better understanding of how they use the APIs in question to help determine how to best support them.
[…] Once Google has finalized their v3 changes and Firefox has implemented the parts that make sense for our developers and users, we will provide ample time and documentation for extension developers to adapt.
Neiman emphasized that, while maintaining compatibility with Chrome is important for their extension developers and Firefox users, Mozilla is under no obligation to implement all the changes brought by Google’s proposal. As a matter of fact, Mozilla’s equivalent WebExtensions API already departs in several areas from the Extensions Manifest v2.
Mozilla however acknowledges the need for increased extension security. As a matter of fact, a week ago, Mozilla removed Ad-Blocker, an extension guilty of violating security rules by running remote code.
Some users have reacted positively to the news, while others noted with interest that Mozilla’s decision to not discontinue the blocking API was framed as temporary.
While Chromium-based browser makers seemingly have less leeway, some of them have expressed their intent to continue to facilitate ad-blocking capabilities.
Brave and Opera, two Chromium-based browsers, include ad-blocking functionality by default and the functionality will continue to operate as usual, regardless of the new Manifest file for extensions.
Vivaldi, another Chromium-based browser, explained that it will seek a work-around:
For the time being, Google’s implementation [of the Extension Manifest V3] is not final and will likely change. It’s also possible the alternative API will eventually cover the use cases of the webRequest API.
The good news is that whatever restrictions Google adds, at the end we can remove them. Our mission will always be to ensure that you have the choice.
Extension Manifest V3 may ship in a stable version of Chrome in early 2020. The Chrome team currently strives to finalize a Developer Preview of Manifest v3.
Mozilla will provide ongoing updates about occurring breaking changes on the add-ons blog.
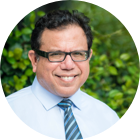
MMS • RSS
Article originally posted on InfoQ. Visit InfoQ
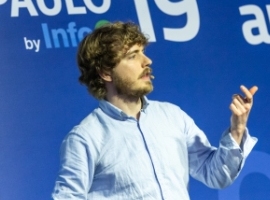
Transcript
Wayman: Hi, my name is Eric Wayman. I’m a member of the Einstein Platform team at Salesforce. Today I’m going to talk a little bit about the work we do, some of the challenges we face, and how we go about solving some of these problems. In particular, I’ll focus on how we use various metrics to help us use your time efficiently and do things at scale.
Before I started at Salesforce, I studied math for grad school, and then I moved on to data science consulting and then I really wanted the opportunity to start productionizing models. That’s what interested me in joining Einstein.
Just a brief introduction about what we do here at Einstein. We have thousands of different customers with many different use cases. We can’t possibly handle all these ourselves, so we really need to empower admins who are using Salesforce, who are using our CRM platform, to be able to do a lot of machine learning themselves. In particular, my team – the Einstein Builder team – focus on helping admins make custom predictions from their data. Any kind of object, we want to walk them through the process of being able to do machine learning on their data and handle the various custom use cases that come up. We can’t possibly send a data scientist to help each customer, so that’s where the Builder app we developed comes in to help empower them to do their own machine learning.
Here’s a quick introduction to Builder. Essentially, it’s a web app that guides admins through building machine learning models with a few clicks and without having to write any code. As I mentioned, it allows them to make any different objects. We have this machine learning pipeline, an automated machine learning pipeline, in the back end, that trains all the models once we receive the info on the front end. We need to serve many different use cases and we don’t have an intimate look ourselves at the data; just some of the example use cases of use, some common ones, binary classification. A lot of customers have subscription-based models, and they might have records of all the customers who have left in the past year or so. This might be something they want to do to predict, “Which of my customers are at risk of churning? Which ones are at risk of leaving?” This would be a binary classification that predicts which customers are at risk of losing, so then they might be able to take up some measures to target these customers and try to improve the retention rates.
Another use case is regression, for revenue management. If one of their own customers might be late to pay their bill, they could help manage revenue and see when they can expect things to come in. Then lastly, our case classification. This isn’t a prediction builder, but it’s the same back end. It’s using the same auto ML pipeline and they’re doing multi-class classification, so getting a bunch of different support tickets and trying to classify which type of ticket it is.
A Data Scientist’s View of the Journey to Building Models
Throughout most of my career as a data scientist, my process for building models looked something roughly like this. First, it starts with data exploration, really get to know all the different fields, the domain, everything I was working with, get some understanding of the data. After that, of course, move on to feature engineering, try to build some good predictive features. Then moving on to trying different types of models and after that, examine the results. If we’re happy, we’d go on, but if not, maybe go back and iterate on some of the previous steps. Do some more data exploration, try different types of feature engineering, and new model types if necessary. Then as a final step, just push them out alive and just forget about it. As a consultant, this is the workflow we’d usually have, just prototyping things, rinsing your hand clean, built another model.
On an ML pipeline, this doesn’t really work, we can’t really do this. This is just the beginning of our journey. Now that we have a model in production, and let’s say it’s doing great on our holdout data, some holdout data set from training time and everything’s looking great. A lot can change once we go into production. Let’s say all of a sudden our productions start looking terrible. What do we do now? Machine learning is notoriously a black box and this can be quite difficult to debug and figure out when something’s really gone wrong. What do we do in this case?
The Metrics-Driven Approach to Model Development
Here on the Einstein Platform team, we really developed what we like to call up a metrics-driven approach to machine learning. What do we mean by this? The first thing we do is to break into this black box a little bit, we will create a lot of different metrics to identify opportunities to improve and fix some of these errors. For example, on our new evaluation data, the new live data that’s coming in, we start tracking R squared if we’re doing our regression, or maybe auPR or auROC if we’re doing your accuracy, if we have a binary classification.
Then we want to formulate a hypothesis and implement some ideas. Great performance during training, things start to drop off, and maybe we notice that, say we’re doing a regression and we noticed a lot of our coefficients are near-zero and we have hundreds of non-zero coefficients, so we think, “Maybe we’re over-fitting to our data a little bit.” Maybe we want to start doing regularization, try to reduce the number of parameters in our models, so we developed this theory, for example. The next thing, we have a few theories, let’s run some experiments. Let’s try comparing what the model was like before, now we add L2, L1 regularization, different combinations, different parameters, and run a bunch of experiments. Just start rerunning of a lot of models or a bunch of different use cases because we’re developing one pipeline serving thousands of different models. Any changes we make, we need to make changes in an automated fashion to be able to work for all customers.
We carefully run and track these. Then from this, we’d identify the most promising solution. Once we have that, before we push it to production, we want to do some regression tests on it; regression in the sense of we want to make sure that across a suite of models, that the performance doesn’t degrade too much and that it doesn’t fall off. If everything looks good, if it passes all the benchmarks, no warning signs, then we can push it to production and start thinking of new metrics to collect, and getting more info and continuing this development cycle.
Why is it so critical that we systematically correct, collect, and report our modeling metrics? By modeling metrics, I mean any kind of quantitative piece of data telling us something about our whole model training pipeline. I can share some examples in a bit. As a data scientist, this is kind of our main tool for figuring out what’s going on with the model, if we’re trying to debug it and get a real understanding of it. If we’re not collecting it in an automated fashion, then we’re going to have to do it somewhat in an ad hoc fashion. The problem is that this doesn’t scale so well. Most likely, you may not be at Salesforce scale with tens of thousands and thousands of different models, but you’re probably going to have more than one. Data scientists are hard to find and expensive, so one data scientist per model is not something that scales very well. This will quickly become a needle in the haystack problem, and it can quickly become quite overwhelming. How do we find one problem going wrong with all these thousands of models?
The key is that we use metrics to guide us to the right problems and then through dashboards and alerting, we can figure out these issues. I’ll discuss this a little more. I like to think of metrics as our main tool for answering these three fundamental questions. How do we know we’re focusing on the right problems? Without metrics to point us to the right problems, we’re just making blind guesses. It doesn’t make sense to try some exotic deep learning model if a real issue is data quality issues. This makes us use our time in a much more informed and efficient manner. The second question helps us answer “How do we know what the right solution is?” This is where we run a bunch of experiments and a regression test to record how these different changes do before we merge into our pipeline. Then the last thing it helps us answer is, how do we achieve scale? Through dashboards and alerts, we can get a real-time global view of our model and draw our attention to things in production that we’re having issues with.
How Metrics Help Us Focus on the Right Problems
Let’s dig into how metrics help us focus on the right problems. From different modeling architectures to new hyper-parameters to different types of feature engineering, there are endless approaches we can take to make changes to our pipeline to try to improve it. How do we figure out where to focus our time? All different sorts of things we can do. The key is we need metrics to focus on the right problems. I like to say that modeling without metrics is like coding without testing. Just as unit tests and error messages can help draw your attention to pieces of code that are going wrong and give you insight for how to fix it, modeling metrics can do the same for your models. Imagine having a complicated piece of code and trying to figure out what’s wrong when you have some unexpected behavior with no error messages or no code, no testing. I would argue that doing machine learning, especially at scale, is pretty much the same unless we’re tracking metrics.
How do we know which modeling metrics to track? I like to think of pressing questions I like to know about my models if possible, and then just try to see if I can formulate some metrics around these questions. Some things we might want to know are what do our label and residual distributions look like? Which features are the most important? Do I have any outliers and how can I deal with them? Which features were dropped? Then as we saw in that example before, how does the model performances on my training data and holdout data and also evaluation data, all compare?
Here are a few examples of some high-level metrics that we track. One of the things we track is a number of scores we ship back every day across all the platforms and all our predictions we’re making over all of our models. This is a great high-level metric, because one, it’s easy to compute, and two, it gives us an overall sense of the volume of everything we’re doing; then, of course, as I was mentioning the comparison of how we did during training on the training data itself, on some holdout set at training time and the live data. This helps us track for things like over-fitting and label leakage, which I’ll talk about in more detail. Something that’s also a little more subtle, which I’ll dig into later in this talk, are changes in the distribution of my prediction. I’ll look at the total distribution of all the predictions I made over a week or a day or some time period, and compare it to see how that changes over time. That can indicate some things.
Let’s go back to this example of digging into this metric a little more, our comparison on training versus a holdout set of training time and versus the evaluation data. That’s that new data coming in. Often what you’ll see is this model three-type performance. On the training data, your accuracy or whatever metric looks really good, drops off a bit during holdout, and then when you’re doing live data, things change a little bit and the model tends to drop off. Your performance isn’t quite as good. Hopefully, it’s not something catastrophic like in the model one and two examples. If you have near-perfect performance at training time, then it drops off. The most common situation like we talked about is you’re probably over-fit.
Another example, something that comes up a lot is, in our use cases – and I’ll discuss this in greater detail – is label leakage. We built our model and some data and some of the features we used are very predictive, but the problem is these features are only known after we have the label, so some information from the label leaked in.
Just to make this a little clearer, let’s take a look at this example. Here, we’re trying to picture ourselves to be a salesperson. We’re trying to predict which of our customers in our sales pipeline will close, which ones are going to sign a deal. You could picture our sales pipeline as a big funnel. At the first step, it’s maybe everyone who we sent out some marketing email, but in that marketing email, there’s a link. Some percentage of those will click on that link and they’ll be on our web page.
Anyone who’s visited the web page is a little closer to going, and maybe they’ve signed up for a white paper and so on, or maybe we’ve had a phone call with them. Each step they’re funneling down the pipeline and getting smaller and smaller and I want to know which customers should we really focus our time on in the sales pipeline? Which ones are most likely to close? You can see this example record here and we have a bunch of different information about our theoretical customer. This is all the information we’re going to use to predict whether or not this customer closes or not. The problem is, if you noticed this deal value here, this is one of the fields I might blindly use to make my prediction, but the problem is I only know the deal value if the deal is closed already. I can’t make my predictions using this because the fact that I have a deal value of $1,000 says already this customer accepted. You might be saying to yourself, “Yes, ok. This is a simple problem.” Just don’t use the fields that are filled out after the label. Only use the fields that you know beforehand.
The problem is in our use case, this often isn’t so simple. We’re suffering from a bit of a cold start problem. Typically, to do our first model, we’re starting with having a snapshot of the data, looking at something like that T0, that first-time step. We have records A, B, and C. Those have positive and negative labels already. Then we have D, E, and F. Those are records that haven’t closed yet but are still open. We can take some subset of the A, B, and C ones, the ones that are closed, train our model, leave out some portion for holdout to validate, and so on.
But with these A, B, and C records, since we’re just dealing with a snapshot, we have no sense of history of when these things were filled out. We could have gotten these records after they’ve closed and so I’m putting the deal value after the fact. So the real test for how well our model performs is, for examples D and E, when we have records that now get a label, or G, H, and I, those records that at later times T1 and T2 came in, and then that T2 and T3 they got labels. It’s really overcoming this cold start problem. The main issue is waiting long enough that we get enough new records coming in and something like depending on what you’re selling, it could take a lot of time.
We’ve established that label leakage is a potential problem. We want to be able to identify, “We have this model. It’s looking great during training, it’s looking great on my holdout data set, but it falls off during performance. I need to know why.” For one, why is it so important that we know why? If the problem is something like over-fitting, then I might have one solution. I might be looking at things like regularization, I want to know how to do regularization. But if it’s label leakage, then my solution is going to be slightly different. I need to find all my fields that are leaking, all my label leaking fields, and I need to throw them out presumably. This is where getting the right metrics to identify the problems really comes in handy.
Just kind of high-level – we’ll go into a few of these in a little more detail, some examples later – one thing we can look at to try to identify this is start tracking my feature completion rates during training, and then live during evaluation. The example of the deal value, that’s something for all my new records, my records I haven’t closed. It’s going to be null, because I don’t have a deal value if there’s no deal yet. That’ll only be filled in after the fact when I have a deal. One thing that you might pick up on are the differences in the percentage of nulls I have from training versus my new records.
Another thing is, sometimes you have a label leakage field that’s almost the same as a label. It’s one of these too-good-to-be-true type fields, so this could be too highly correlated. That’s another thing you might want to check. None of these things in themselves tell you that you have label leakage, but they can be indications, things that can point you to it. Then there’s association rule learning. There are different ways to find relationships between variables. I’ll talk a little about this more in the future.
How Metrics Help Us Find the Right Solutions
We’ve identified some problems. Now, let’s take a look at how metrics can help us find the right solutions. The main way we find lots of solutions is by running lots and lots of experiments. The key to doing this, we really want to ask ourselves two questions. One, what’s the data we need to track and evaluate our solutions? To do this as part of our pipeline, we have something that’s called the metrics collector. We have an API so we can write metrics and compute them at various points in our pipeline. Then when we run this pipeline on some data for those models, it’ll track all those metrics and put it in a table so that we can query them later and really get good at evaluating these. The second question is how do we evaluate our solutions? That’s where the experimentation framework comes in. This is just a way for us to take a bunch of different models that we want to investigate and run different experimental branches on them.
These two things combined gives me the ability to try these different options, like A/B testing type stuff. I can do a bunch of different development branches, run them across the same data, record the metrics for each. Then once I have it in the database, then I can really start querying these and comparing the metrics and see if things are going the right way.
I like to say that if we don’t track it, we won’t improve it. You might say, “This is just a matter of I just need a great auPR or auROC or really high accuracy. That’s what I care about.” But as I showed in some of the examples, we want to really figure out these things at training time. We want to get our models as good as possible upfront and we have this cold start problem. At training time, the best we can do is evaluate our model on some holdout data. As we talked about with over-fitting and with label leakage especially, these can be misleading. We can sometimes get a high holdout metric and then when things go live, things don’t work as well. It’s more than just maximizing holdout performance. We need to start looking at some other metrics that we can track to evaluate the different solutions we’re doing. This is a little more subtle.
Let’s dig into an example here dealing with date fields. As part of our feature engineering pipeline, we deal with different features by types. We found that date fields can be incredibly useful. One of the things they help us do is detect seasonality in trends. Maybe you notice in November and December you get a spike in sales or Sunday is a slow day, these sort of things. The way we like to pick up on these seasonality trends is we extract different pipe time periods, so you have a timestamp. We’ll pull out the month of the year, the day of the week, the day of the month, things like this, and then we’ll map it onto the unit circle, and then return the coordinate. If you have April 1st, 2019, then the April part, that gets mapped to one zero on the unit circle. We have that numeric feature for it and so on for different time periods. That’s the good.
The bad is we had this phenomenon we observed of bulk uploads. A bunch of records getting uploaded and as a result, all having the same dates. The problem we found is that this can kind of skew the data distribution and lead to spurious results. We really need to get some metrics to try to detect this, because we want to use these date fields in our pipelines, but because of bulk uploads, we have some problems.
This example illustrates one potential thing that happens with bulk uploads. Think of the same example – I have my sales pipeline, I’m trying to predict which of my leads are likely to convert. Let’s say we are migrating our data to some new system. Often what you’ll see happen is this thing sort of thing where I’m not going to migrate all my records. Maybe I’m only going to do the good ones. You can see from April 1st that all these records have the label one, all the deals signed. I’m only moving in my good records in the new system. Then in the later dates after that, that’s when my new records are coming in and they’re going to have the real distribution. Some will close, some won’t.
The problem is – you can see all these created dates are April 1st – this can create some spurious relationships on the data bulk upload. The model might learn something like, “April 1st is a great sales day. If you have a lead in April 1st, for sure it’s going to close.” These are the things we need to pick up on.
How can this affect us in production? Aside from degrading our model performance, this spurious correlation, this might not be something that we see right during training time. It might be something that appears right away, so we really need metrics that will alert us to the problem as soon as possible. One thing that could happen is, because of different distributions in scores, we’re having a lot of good records during our training time and a much smaller percent as they’re trickling in. We might see some drift in a prediction, some scores. You could see here that the training ones are going to be overly optimistic. Our prediction, the probability that these things will close, is going to be much higher because we just moved in our good results. Then during evaluation, when we have the new data coming in, there will be much more ground there, a much smaller percentage of a record actually scores.
How can we detect this? One thing we want to do then is come up with different metrics for detecting drifts. We can do something simple, just so you can compare the means of your prediction values, a probability you think they’ll convert during training and compare that each week. That’s it with the new records coming in. Maybe you could also check the standard deviations, some other things, different metrics for how close two distributions are to each other. One we use is Jensen-Shannon Divergence.
Here’s just another example. Label leakage was a problem we’ve identified, something we try to pick up on. Yes. What metrics can we use to track it? Getting back to this example of label leakage from deal value, the same use case, I’m trying to predict which of my leads are likely to convert. Here you can see this contingency matrix, you can see a non-null deal value is perfectly predictive of a lead converting. If I have a deal value, that means the deal is closed. If it says the deal is worth $1,000, that in itself means that the deal is closed. This isn’t one of those too-good-to-be-true features because you can see in the bottom row here, I have a bunch of examples of each type deal closing and deal not closing that don’t have that field filled out where that deal value is null. That could be maybe, deal value is a new record. Maybe it had a different name before, so a bunch of the records where they’re closed, they don’t have that filled out or maybe it’s just missing data, stuff that isn’t in there.
How can I try to detect this? One thing we use is, we can compute the confidence matrix. This tells us the fraction of each type that is associated with the label. This tells us 100% of the time when the deal value is not null, I have a true label and 0% of the time when it’s not null, I have a false label. There’s no deal that didn’t close that has a deal value, and then it’s a little murkier on the bottom row, some fraction associated with this label. This is something we could do. It’s not as if most of the time this feature is null, so it’s not going to be one of these too-good-to-be-true features.
If you’re just looking at correlation, you’re not going to pick it up for various other metrics, but it does have this perfectly predictiveness that it won’t carry through when you’re running out in the wild. If you have a confidence entry at 1 or say above some threshold, and this is something you have to experiment with above 0.95, this is where the experiments come in to try to see how these things work in practice. Then you might want to say, “This is a leaky field, so let’s drop it.” The issue is it’s not quite that simple, because in some fields there are a bunch of different categories. Let’s say one category only appeared in five records and it just so happened that all those records are closed. It might seem like that category is perfectly predicted, but it’s really just the fact of only having a few of them. It’s just random chance there.
Then we might have to experiment. We want to look for a confidence that’s really high, but then at the same time we want to make sure that the support, the number of records in this category, isn’t too low. These are the problems we deal with and all these different experiments you have to run to try these different thresholds and see how it plays out in practice. Not quite an easy problem.
Here are some key learnings from all this work we’ve done for modeling experiments. Make metrics early and often, you can never have too much information. Developing models is like a black box, so we need as much information as possible. Then it’s a little hard at first maybe to know which metrics to track, but once you get a few, you get the ball rolling and alert you to other problems and giving you new ideas. Also, from your experiments, store these metrics in a database. It’s really great to be able to query and compare these efficiently, especially when you’re doing runs across thousands of different models. I need to look at it in the aggregate. Some models may get better, some may want to get worse, and how to balance all that is tricky. Just looking in logs isn’t scalable.
Next, you really want to make sure that your experiment process is reproducible. You really want to have some trust in it if the experiment I run should really do what I think it did, and plus for just believing your results. Sometimes you might want to run the experiments a few times to get an idea of signal to noise ratios and you might want to a run variance of it in the future. Then lastly, you minimize manual steps because this can lead to errors and the experiment might not do what you think it does, especially if there are a lot of manual steps running tons of these, so it can throw you off. Try to automate as much as possible.
How Metrics Help Us Attain Scale
We’ve seen now how we can use metrics to identify problems. Once we have these problems, we start experimenting, trying different things, and we’ve seen how the metrics can help us evaluate these solutions. The last step here is how metrics help us do this at scale.
A picture is worth 1,000 words and a dashboard 1,000 metrics. As we start collecting more and more metrics, it might seem like, “You actually can have too much of a good thing, getting all these metrics coming in and I just can’t keep track of it. I can’t know anymore.” This is really where dashboard and alerting come in to draw your attention to the most important metrics and most pressing models.
For those of you developing apps and software engineering, this is something that’s standard practice, first off in dashboard and alerting. Maybe you have a dashboard to monitor some key health of your apps, you get a good visualization of your overall app ecosystem. Similarly, I’d argue you do the same thing with all your machine learning models.
What metrics should we track? Just as you have key metrics that you might want to track for your web apps, like number of visits or average session, maybe some things like the number of models we’re training or something like with the number of scores we train, something that I give an example of. Also, a global view of our model health, maybe an average accuracy or the percentage of your models that are falling below a certain threshold and visualize all of those as red, anything that can help you pick up on anomalies. We have a data quality alerts dashboard, this lists out a bunch of different models according to metrics. For example, one of them will show our models that have the biggest gap in holdout, versus live training this week. We can really see which models are falling off in production and address our attention to those.
Another thing is models that haven’t scored anything, haven’t made a prediction in 30 days or more. Those could be dead, maybe there’s something wrong with the pipeline at some point. Then also ones that start showing drifts, ones where all of a sudden, our prediction distribution has started to change. As we saw, that could come up with the date fields where you have a bulk upload, or maybe what happened is some fields are no longer being used in the same way, that they’re no longer being filled out or now a field that used to be null isn’t and the model’s kind of stale. These really come in towards helping us find the right models to investigate and trigger our investigations to get at these problems in the first place.
Then we also have a global summary dashboard. This gives us a bird’s eye view of how well we’re doing. The total numbers of models we trained each day, the number of predictions, and then just different things like I mentioned, models, different codes for health and so on. All these things help us figure out where we should direct our time and that gets back to that first part I was telling you about. We look at these models, we can see what issues are really plaguing us and then spend our time appropriately.
Tying It All Together
Let me tie this all together, and talk through a recent case study that happens that illustrates how all these work together. We got an alert from our data quality dashboard. We had a model that had this massive amount of drift, something like the left graph there. During training, we had one score distribution. They were all pretty high, and then this week, for whatever reason, all the new records’ predicting was much different. What we did is a data deep dive, so looked deeper into this customer and then we had the dashboards. The things we look for are what are the highest features for feature importance. Maybe some of those are the too-good-to-be-true case. I should mention that as we were looking into it, we began to suspect that label leakage might’ve been an issue. We’re really trying to dig into the features we thought that could be a problem.
As in the deal value use case, you had a lot of non-nulls during training and then with your new records a lot of nulls, so compare distributions of how often is this record null during training time versus now. Things with a good gap, those are other features that could be reduced. Then we’d experiment and just drop all these, and we were able to reduce the Jenson-Shannon Divergence by over 90%. The key thing here why are we so focused on label leakage?
These new records that we’re scoring, keep in mind, they are live records. We don’t know the ground truth for these. We don’t have the labels for them. It could very well be that the labels were just different. This is something that we want to investigate, we want to find this as soon as possible. The fact that we had a problem was alerted to us not during live evaluation, because we don’t have the labels for these new records coming in, but just the fact that we have the scores. A follow-up to this, going back to this whole cycle, maybe we need to work on our label leakage detection a little bit, so a never-ending experiment.
Key Takeaway
The key takeaways are three things. Use metrics to verify that you’re working on the right problems. You don’t want to spend your time working on your favorite modeling architecture if that’s not your issue. If your issue is label leakage, then you need to spend your time with that. Modeling metrics are the only way you’re going to know what your problems are. Then running tons of experiments. You really want to validate things, data science is about data, so you want to make your changes in a data-driven manner. The metrics for each of your experiments, these are the data. If you’re collecting that and having a database, now all of a sudden you have data back your data, and you can start doing data analysis on that.
Then once you get big enough, the metrics come in handy as we saw in the previous problem, to really direct your attention to the right things, the pressing issues. That’s how you’re able to manage these tens of thousands of models when you’re pointing to the ones that are having issues.
Questions & Answers
Participant 1: For this time series, do you use a remodel also, or just a plain regression and classification models?
Wayman: Which time series do you mean?
Participant 1: To track seasonal data, seasonal events.
Wayman: We have this pipeline, so we’ll know it’s a regression, for example, but you may not necessarily know upfront is this a time series type thing, or is this kind of more static. So getting different templates to handle those differently is something that we’re working on developing, being able to make it more flexible if we know this type. A remodel is not something we’re doing now, but it could be something in the future that if we know you’re in a specific time series setting, you have those time-series features that might be a way to handle it. Yes, those are already things we’re looking for.
Participant 2: What is the technology that you use in prediction? Is this still a psyche to learn things?
Wayman: We use Spark. Everything is in Scala and we use Transmogrify, just last year we open-sourced it. It’s our auto ML machine learning library, which is open source so you can check it out on GitHub, Transmogrify, and then AI as an artificial intelligence. That’s based on Spark ML and that will do the things I’ve talked about. It will have free automated feature engineering, model selection, sanity checking. We look at features based on thresholds, drop it based on some of these metrics if you think they might be leaky filters and so on, so we use that library to build the app and install that on Scala and Spark.
Participant 3: I don’t know if you’re already facing something like this, but let’s suppose that there’s data where you see a possible leakage, there are some privacy concerns and you can’t access the user data or you have some difficulty in finding contracts, agreements or you can’t go directly to the data. How do you proceed to take the matrix and then work with that?
Wayman: Let me know when you figure it out. It’s not easy, because we have different customers and data privacy is a huge issue. We can’t combine different data, look at all these different things. Yes, sometimes you don’t have access to the data itself, so you have to go by this metrics approach. It’s really just getting enough data, getting enough of that quality data down the road, that evaluation data. That’s your golden ticket to see if it’s performing well in that.
I don’t know if I mentioned explicitly, but the cold start problem – you have no way of knowing if it’s changed after the fact. As the data starts trickling and we have the timestamps, so when we’re training on that new data, which is what we’re looking at, once we get enough of that to build a model, then I can say, “Let me build my features on a vector what it looked like before the label came in, some time period before. Maybe I can split the difference.” I use the old feature vector and then the new label after the fact. It’s really about getting the right data. That’s the only way to do automated without actually knowing the specific data, which as you mentioned, you can’t always have access to.
Participant 4: One of the main benefits that you raised from metrics was trying to scale out the machine learning problem and trying to reduce the need for data scientists all the time looking at the models. Do you have some kind of metric about how many data scientists per model or problem that you see at Salesforce?
Wayman: How many data scientists we can handle per model?
Participant 4: Yes, something like that. You’re trying to reduce number of people per problems.
Wayman: I don’t think that’s anything we track. Our team of data scientists is like 10 to 20 and we have tens of thousands of different models. It’s a new thing. We just went GA, just released the product in March so things are always changing and developing. Typically, what we do is, we’ll spend some time doing deep dives, digging in and trying to figure out the issues. That’s how we figured out in the first place that label leakage is a thing. Then when you think you satisfy it, you move on to the next thing and have it running. Whatever the size our team is now, that’s the size of the team we have to handle all the models coming in. We don’t always do a perfect job, but it just is what it is.
Participant 5: We have a very different problem from what you have. Most of us work in smaller organizations or we don’t have a lot of customers with a lot of different data sets, so we are not in the business of automating data science, but I think that a lot of the ideas make a lot of sense. Think about, for example, a small startup company doing something like project analysis of something like that, for financial tax. Do you think that this kind of automation is something that is obtainable for a small four, five persons team? Is it something you could see some gains from?
Wayman: Maybe, yes. Just for running variations end-to-end, there’s been a lot of talks about different pipelines and things. Maybe the automation parts are something that takes a while to fill in. Maybe the reason why your models are so good is because you have that domain knowledge, where you can really dig in and figure out what’s going on in a way that we can’t because we have to do at scale. I think the metrics – knowing what your models are doing, if you’re doing one model, that’s just as useful. Imagine having a library where you’re getting all this information out of it. If you’re debugging your model trying to figure out why it’s bad, the label distribution, feature importances, looking at your residuals, outliers, tracking these different feature correlations, and the confidence. Maybe you didn’t think you have label leakage, but maybe it comes up. I think at any level, the metrics come in handy, and the automation probably more down the road, but who knows?
Participant 6: Have you ever had the problem of mixing together the data of different distributions? For example, the company that I work for has many different customers; small customers, big customers, very big customers in different markets. Sometimes you suppose the data is predictable, but you see that it mixes many kinds of behaviors. How can you detect these things with metrics?
Wayman: You’re saying you might have a situation where one model only works good for my small customers, and then I have the big corporate customers and that has to be a whole separate model. Maybe the first thing you’d want to do is have a classifier. Does this fall into the big model category, or the small one? Then have different models customed to each of those. Yes, not an easy problem to solve and we have to do it. Maybe we don’t have to do the same thing for every customer, but we have to do it in the data-driven automated way. It’s hard because we’d have to figure out automatically how to do some tests to figure that out. I think just looking at your residuals and your distribution would be a place to start. Just see the things I’m predicting, do some have really small view field of values, other big? Are there different feature importance for each? Break it up and see. Then once you start digging in, you get more ideas based on what happens. Off the top of my head, that might be something you can think of.
See more presentations with transcripts