Month: May 2019
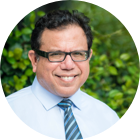
MMS • RSS
Article originally posted on InfoQ. Visit InfoQ

Transcript
Jarmul: This talk is based partially on a talk that I gave at Strange Loop last year, which was called “Privacy: The Last Stand for Fair Algorithms.” so now we will revisit it together. When I decided that we should revisit this, or when I was invited to revisit this topic, especially at an audience here, in the Bay Area, in Silicon Valley, sometimes called the Belly of the Beast, I thought to myself, “Ok, I might change a few things and talk about privacy with an emphasis on the larger tech companies here and what they’re doing and/or not doing in some cases around privacy.
To begin, we’ll pick on Google, because why not? Is anybody here from Google? No, perfect, even better. Here is Mr. Peter Fleischer, he is Google’s Global Counsel for Privacy. This means that he represents Google on a global level when it comes to privacy and he’s also their longest serving privacy officer. Privacy officer is similar to security officers, they tend to have a short term, let’s just say. As soon as something happens, they’re gone and they get replaced with somebody else, but Peter Fleischer, he’s been in service for more than a decade.
Here he is, this is from his own blogspot, this is an image of him. He lives in France, so I’m assuming this is by the Alps, gorgeous, this is how he sees himself. I can’t really see his face, so I said, “I’ve got to find a picture of this guy’s face. How can we talk about him without showing his face?” so I went to his employer, Google, and I searched, and this is the top result on Google images. I said, “Ok, this is him speaking at a conference, it’s a European conference on privacy, he’s obviously dressed in his suit. He has a little bit of a funny look on his face. It’s a little bit of a weird expression.”
I don’t know how he feels, because how he sees himself is on the left, I also don’t see any grey hairs on the left. On the right, he looks a little bit older, so how he sees himself and how he presents himself is the left image, but the top Google search result is, of course, the right image. How he sees himself and how Google, usually supported by algorithms and/or at least minimally computer programs, how they’ve generated an image because this is a YouTube screen grab, so how they’ve generated an image to represent him, this is quite different.
I think that this starts to touch upon consent, and this starts to touch upon how we define ourselves on the internet, and how other people define us on the internet, we’re going to go a little bit deeper in that. Mr. Fleischer had some opinions on this. He, of course, has his own blogspot, and he’s written quite a lot about the right to be forgotten. What he has written on the right to be forgotten is, “All of my empathy for wanting to let people edit out some of the bad things of their past doesn’t change my conviction that history should be remembered, not forgotten, even if it’s painful. Culture is memory.” This is quite eloquent.
I’m curious, how many people here share this idea about Google and the internet, that we should never delete things? They’re the cultural history, a cultural memory. He has gone on to say that Google will be the history of the future, this is how we can keep track of things. How many people here believe that the right to be forgotten simply erases history, that this is not something that we should allow? Keep your hands up. I want you to continue to keep your hands up if you think that Mr. Fleischer or if you yourself have been a victim of revenge porn. What about unlawful arrest? Do you think Mr. Fleischer was illegally or unlawfully arrested by police? Was he targeted or discriminated? What about employment? Do you think Mr. Fleischer has had some problems with employment based on the search history, based on things that people can find about him on the internet? Do you think he suffered that? Do you think the people that believe there shouldn’t be a right to be forgotten have suffered that?
I want to come to Mr. Fleischer with some facts and these facts were actually leaked by Google themselves. Ironically, in their transparency report, they published a bunch of data in the source code of the page, and they accidentally leaked their own data, which is something that Google might do. The point is that 95% of over 200,000 requests for the right to be forgotten were not related at all to a convicted criminal, to a public official, or to some other public figure.
These were people that wanted to remove their private information, often, from the internet. “I didn’t know that my address was posted there, I would like it removed. I didn’t know that this photo was posted there, I would like it removed.” These are private citizens, these are not public figures, and they would like things removed from the internet that they just don’t want in a search when their employer goes to search for them or when something wrongful happened to them. Mr. Fleischer here actually, ironically enough, he’s a convicted criminal, but do you think it’s affected his life? Did he get fired from Google? He was convicted for Google’s crimes against privacy in Italy towards child images, but he still has a nice life in France hiking Alps.
Privacy as Privilege, Power & Security
This touches on a deeper topic here, which is within our society today, privacy acts as a form of privilege. Those of us that have privacy, we can choose to opt in or out. We choose and we say, “Ok, I would like to give up some of my privacy to get this thing. I would like to give you some of my data to get this thing.” but we don’t need it as a core part of our life, we don’t need it to survive, we don’t need it to get food stamps, we don’t need it to get coupons so that we can afford the things in our society.
How many people here, when you see the free Wi-Fi, and you have to fill out a bunch of your personal data, how many people put their actual personal date there? We laugh because we know how that data is used and resold, and we would never give our personal data there, but do you think that everybody knows that? Do you think that the average person knows all that information, and do you think that this trade, essentially, of some service or some reward, for data, do you think that this is fair for people that don’t have other ways to access these rewards? Is this a fair way to treat people? Is privacy equally distributed, do you think?
Privacy also is a form of power, so privacy is privilege and privilege is usually some form of power. In privacy is power, we can think of this as information asymmetry. When you enter a negotiation or any type of back-and-forth business interaction, what you want is equal footing, what you want is you want to know at least as much information as the other side knows. This is essentially game theory, also business theory, and so forth.
What happens when we have erosion of our privacy and massive data collection, which we may or may not be aware of, is we have created now an information asymmetry that is so great, that our ability to negotiate with these people that hold our data is almost irrelevant, because they already have all of the information they need from us. We don’t have any leverage to say, “Oh, well can I trade this extra piece of information?” “No, sorry, already got that. I bought it from somebody. It’s fine.” This comes into play a lot online when these larger tech companies, some of which may be your employers, use this to make decisions about what people see, about what people buy, about what prices people pay for things based on this information asymmetry.
In as such, it also affects their personal and their data security, it also possibly affects our societal security. When people’s private data are breached, or sold, or given, like the news from Mark Zuckerberg today that he used private data as leverage for business deals, that he said, “Hey, man, you want to do business with us, I’ll give you some data. Fine. It’s just my users, no big deal.” We saw it again, of course, with Cambridge Analytica. The idea that you can feel secure, both that your data is protected, that it’s secure, stored in an encrypted way, hopefully, that somebody’s looking out for you, that the data that you’ve given over to somebody via a trust mechanism, that this is respected in some way, shape, or form, also technologically, and then to have a breach, like Cambridge Analytica, classified as a breach, we’ll call it a breach, and to have that data then used against you to manipulate yourself and people like you and to potentially influence elections around the globe, so privacy also operates as security.
I was thinking about it when I was walking around San Francisco two days ago, I was thinking about what is the ultimate lack of privacy? It’s homelessness, right? The ultimate lack of privacy is you don’t even have a private place to sleep, or eat, or do anything. As I walked around San Francisco and I see big, massive bank or tech building, and then homeless people outside, I think I saw quite a gulf there. To me, it really illustrated this idea of privacy as privilege, power and security, and the fact that we see such an example directly in front of a place that controls quite a lot of data, and therefore, people’s privacy.
Privacy & Fairness
How does this relate to fairness? I hope some of the connections are somewhat clear to you already, because if it’s privilege, power, and security, then also, of course, this might be unequally distributed, and therefore, this might correlate with fairness. I want to give you an even more illustrative example, I think that most people that work in applied AI, also myself, within the machine learning space, I’ve done lots of years of natural language processing, we see AI as something that can help us. We want to help people, we want to build good products, we want to do good work, we want to work on challenging problems.
Very rarely have we been hurt by this. In our experience, a lot of times, this automation, this AI driven change within our society, this has really benefited us, it has added convenience for us, it has added easier access to things, it has added quite a lot of positivity in our life. Usually speeding up processes, limiting paperwork, getting things done faster, or better, something like this.
Probably rarely have we been affected by the negative consequences of these automations, or the negative consequences of unfair treatment, or unequal access to privacy. Usually, we’re not the one getting the loan denied message with no explanation, usually, that’s not us. It might not even be anybody we know, so we’re essentially separated both in our knowledge and use of privacy in other people’s data, and also our understanding of fairness and fairness criteria within our space.
Fairness through Awareness
This was further advanced by Cynthia Dwork. If you’re not familiar with Cynthia Dwork, she’s a leading researcher, and I would say even activist, on privacy and machine learning. What Cynthia Dwork did, her initial research that really contributed to this space, was about a process called differential privacy. What happened was first she disproved the notion, there used to be this notion that you could do something called statistical disclosure. This notion was the idea that you could release something and not expose private information about an individual.
She actually disproved that theory mathematically, and also with a series of logic proofs. What she was then tasked with, really, is when you destroy the way that people have done anonymized releases forever, then you probably should rebuild something, what she rebuilt was differential privacy mechanisms. This essentially is what we consider, in a lot of ways, the state of the art way to measure privacy loss over time, so we can use differential privacy to quantify privacy loss and to measure that over a longer period of time with something that we often refer to as a privacy budget, this is some of her background work.
After her work on differential privacy, she decided, “Can I apply differential privacy principles to something outside of a query-based approach?” which is the original approach to differential privacy and she came up with this paper, “Fairness through Awareness.” Her goal of “Fairness through Awareness” was to mathematically prove that privacy and fairness were correlated, that when we increased privacy for the individual, specifically for certain sensitive or private attributes, such as, let’s say, gender, age, race, and so forth, that we could then improve the fairness of their treatment. The goal was “Can we treat similar people similarly?”
If it’s between myself and yourself, and our only difference is gender, our only difference is race, and everything, all else aside, we have very comparative backgrounds, why should you get the job rather than me? Or why should I get the job rather than you, based on my skin color? Her idea, essentially, is that we can create a mapping space that essentially can remove the impact of these private or sensitive attributes, so we create a representation that can remove this variable or the influence of these variables.
Learning Fair Representations
Mathematically, it worked but mathematically working and working in practice are two different things. The research was actually expanded upon by several researchers at the University of Toronto, and they actually implemented this mapping. I can highly recommend this paper, it’s called “Learning Fair Representations.” What they did was they compared it with several practices that were very popular at the time, including a fair bayesian approach and a regularized logistic regression approach. With any time that you use fairness or other criteria alongside accuracy, you need to find some sort of optimization, you have to determine some optimization equation.
Here we see two wants. The one on the left is minimum discrimination, so let’s minimize the discrimination of the groups, and the one on the right is the maximum delta between accuracy and discrimination, so trying to essentially push the gulf between the highest accuracy with some error, essentially, for the discrimination. What they were able to show, it’s the dark blue line here to the far right, is that the learned fair representations performed quite well, especially compared to some of the other fairness approaches, especially in terms of retaining a level of accuracy that was higher.
Essentially, what we can see here and what we can take away here is that not only is it important for us to choose optimizations that allow us to evaluate things perhaps, like fairness and privacy, but also that these fair representations can actually be reused for other similar tasks, so tasks with similar objectives. This is something that you can actually take and utilize for more than one problem, this was really the goal of that paper.
Implement Private and Fair Machine Learning
When Wes asked me to come give the keynote, said, “Can you make it a little practical because I know that this is a practical engineering conference, and we want something that we can take home, take to work, implement, and so forth?” For the next rest of the talk, we’re going to shift from this theory and these ideas of privacy into how we can actually implement them into systems that we’re building and into machine learning or AI systems that we want to create.
Another piece of research that was recently released was by a team called GoDataDriven. They’re based in the Netherlands, and they’re actively working on fairness problems, as well as other machine learning problems. What we see here is an iteration across similar datasets, this is a dataset that predicts whether you earn more than 50,000 or less than 50,000, and they try to essentially remove the sensitive attribute. Here we have a GAN, so if anybody’s used GANs before, we have essentially two machine learning systems that are interacting with one another. And the first one is just working on the classification problem, so does this row earn more or less? The second one is a discriminator that’s trying to guess the gender or the race based on that classification.
In a way, this is exactly what Cynthia Dwork was proving, that if we can remove our ability to guess the gender, then we are treating similar people similarly, if we can remove the influence of race, then we are treating similar people more similarly. They have open sourced their code on this, feel free to check it out. What they showed is that, yes, there is this tension between accuracy and fairness, and accuracy and privacy, but they were able to optimize for both using a GAN model, so it’s a fairly novel approach.
Collect Data with Privacy Guarantees: Group Fairness, Individual Privacy
Another approach that you can use is collecting data with privacy guarantees. There are many ways that we collect data and more often than not, we don’t actually need all the data that we collect. I was at a conference recently and somebody said, “We just collect all the data so that we save it for a rainy day.” I would say a rainy day is just a data breach waiting to happen, but that’s just my opinion. Can we figure out how to collect data with privacy guarantees? Can we then utilize this data in order to show things like group fairness?
This is a nice use case, this is also from Google, it was reported on by The New York Times. A group of Googlers got together and said, “We’re going to create a Google Sheet. Everybody there is going to put their gender and their rank, so to speak, their level of engineering title, and then they’re going to put salary.”
This isn’t necessarily anonymized, because if I were to watch you and I knew that you just entered your data, then there’s a lot of ways that one could attack this and re-identify the data, but we’re not going to go into that. What we’re going to go into is, the idea that each row would not be attributable to any one person. In aggregate, this showed a trend and this showed a trend that they actually were able to use in a class action lawsuit against Google that showed discriminatory pay for the women engineers that were working there.
If you’re concerned about fairness, one way that you can enable people to share more information on this is to allow for individual privacy, because it means that I don’t have to go up, as a female Google engineer and say, “Well, I heard my coworker is making $10,000 more than me. Can you please give me a raise?” The onus becomes not on the individual, but on the data that we can share as a group, and therefore, together we are actually stronger in this case.
Privacy by Design: Protect User Data in Software Design
Another thing that you can work on and do is implementing privacy by design. Privacy by Design principles allow us to create software where privacy is at the core. This means end-to-end encryption, this means very clear and transparent use of data, this means ensuring that the user can enable or opt in or out of privacy controls quite easily.
This is all a part of privacy by design, and it should sound a little bit familiar. Does it sound a little bit familiar in terms of topics? If you had to implement GDPR, you’re probably very clear on the fact that Privacy by Design was a core piece of GDPR. In fact, there’s an entire section or article within the GDPR that simply focuses on Privacy by Design, but this was a theory created by a working group in Canada before, I highly recommend you take a look at both.
GDPR
Let’s dive a little bit into GDPR because it’s really funny for me when I come here, to the U.S. I’m originally a U.S. citizen. I live in Europe now, Germany for five years and it’s pretty funny for me the way people talk about GDPR here. It’s a good point of view into the American psyche. This is because I think people see GDPR as punitive, which is surprising to me because when I think about GDPR as a European resident, I think that we’ve created first-class data citizens and other. I think we’ve created a group that has control and ownership and ability, agency over their data, and we’ve created everyone else.
It’s funny to me that even though I live in a place of GDPR and so forth, and yes, maybe I can’t read the LA Times- I’m not really very concerned about the LA Times, I live in Berlin- but it’s amazing to me, that the blocking of sites is somehow punitive towards me, when really, if I were you, and I lived here, I’d be pretty concerned about what the LA Times is doing with my data. That’s how I would look at that, “Wait a second, what are they doing?” This is because, really, the LA Times has decided that it’s easier to just block an entire region of the world than to implement Privacy by Design principles, so, have fun on the LA Times, let me know how that goes for you.
What I ask is maybe we should treat privacy as a right for everyone, maybe we shouldn’t just let the nice European residents, like myself, have all the fun. If you’ve actually implemented GDPR at your company, it would be pretty easy to not offer this only to a geographic zone, it would be pretty easy to offer this to other users on your site. And you know what? This amount of transparency and openness and agency that you’re giving the users, this is actually going to be seen as a nice, goodwill effort. I would say probably your trust in safety teams and your customer success teams will give you a thumbs up on allowing people to do some basic rights with regard to their data and how you’re using their data.
Choose Fairness and Privacy Metrics Early and Often
Some final thoughts on fairness and privacy within machine learning. First and foremost is there was a talk yesterday from Google about a few different fairness metrics. I’ve spoken about a few privacy metrics. And what I suggest, if you’re actually on an applied machine learning team, is that you choose and designate types of fairness and privacy metrics early and often, that before you go about training and modeling and so forth, you actually incorporate those into your choices. This is because you don’t want to get to the end stage and then say, “Oh yeah, did anybody check the fairness thing?” and then you have bad and worse.
You need to choose this often, but this diagram is from an algorithmic fairness group. This is a group of two different research departments. One is the University of Utah and the other, I believe, is Haverford University, and they have groups working on algorithmic fairness. The problem really is that there is at least 21 documented definitions of algorithmic fairness. This was released in a paper, I believe either at FAT ML or NIPS last year. What they saw is that some of them- these are correlation charts- some of them are in direct adversary relationships with one another, so they’re negatively correlated. If I improve this fairness metric, I’m actually removing this fairness metric, this is really essential that you get this right because if you optimize for the wrong one, you’re actually increasing inequality with the way that you’re analyzing your fairness.
There’s lots of research on this, you can dive in deep, but the point is, for the task at hand and for the population that your model will be touching or affecting or training on, then you need to think and define the fairness metric, and maybe reevaluate over time. I warn that you please avoid the fallacy of metrics. What is the fallacy of metrics? The fallacy of metrics is the idea that I’ve optimized the metrics, and so this means I’ve fixed it. I fixed data science, I fixed ethical machine learning, we can all go home, it’s all done because I’ve created one model that wasn’t racist, it means I totally fixed everything.
I hate to pick on Google- maybe I don’t actually hate it- but the idea is that if you create a fair or an equal opportunity bomber, you still created a bomber. You can optimize fairness for quite a lot of things, but it doesn’t actually make the work ethical, you have to think about the greater picture. Just because you improved equal treatment of users by one model doesn’t mean that that model does the right thing from a moral standpoint. It doesn’t mean that we fixed the problem in society, it doesn’t mean that we changed anything by just looking at one fairness implementation.
I don’t know how many people here are familiar with Danah Boyd and her work, she’s been working in privacy, particularly in social media. I noticed that there are lots of social media companies and/or other companies where the users are really your data, and the users are really your product. If you work at a social network and nobody’s there, then you have no job, if you work at a place like Uber or Lyft, then you have no drivers and also no riders, so you have no job. When the users are the core part of your product, then you really have to think about them, you have to think about their perspective, because they can change their mind and go somewhere else, even if you think you have a monopoly now.
Danah Boyd has studied quite a lot of this, of social media context and what does privacy mean in social media context. There was a recent debate on a mailing list that I’m part of where somebody said, “Ok, fairness and transparency are at odds.” This is Mr. Fleischer’s thing, too, we can’t have transparency and privacy, we can’t have these, these are not available. She responded, and I want to read her response or a section of her response, “Privacy also has a long and complex history, in both legal and technical contexts. This often gets boiled down to who has access to the data. From my vantage point, that’s a dreadful way to define privacy. Alice Marwick and I spent years interviewing teenagers about what they understood to be privacy violations, and what became clear to us is that privacy, in the colloquial sense, means control over a social situation. In other words, it’s not about access, but about expected use. And this is where we realized that one line of legal thinking actually got this historically correct, a reasonable expectation of privacy.”
What she’s saying is not, of course, that privacy means security for the data, although I would argue that that’s important as well as part of trust, maybe. Privacy is actually that the data is being used in a way that the user anticipates and expects, and that transparency actually joins that quite well, because if we’re transparent with users about how we’re using their data, if we’re transparent with them about how we allow them controls for that data, then this means that privacy and transparency are actually working together in this case.
As Danah Boyd would say, and I would say, please give users some agency in this process. It’s not that hard to give them some agency and some real transparency of how their data is being used, and the amount of trust and happiness you’ll get from people by actually respecting them and asking them, and not just doing something and waiting for somebody to report about it in the newspaper, this is a bond that will not break, you will get lifetime users.
Privacy & Privilege Revisited
I want to bring back this concept of privacy and privilege, I want to do it with this idea of the right to be forgotten. I don’t know how many people here saw this Tweet, or heard about it. It was in Oakland and there was this black man who was barbecuing by the lake and there was this white woman who called the police. She said that he was barbecuing illegally, even though this was a common area where people barbecued. This photo was posted on social media and got quite a lot of attention and reporting and so forth. Let’s go back to the right to be forgotten, let’s say that either both individuals in this photo or one or the other were tagged or indexed, that in the search results now for their name, there’s this image. Let’s say that one or both of them wants this to be removed, do either of them or both of them have the right to be forgotten?
We may not like the idea that the woman has the right to be forgotten because we may say, “Oh, well, her behavior was abhorrent. Everybody should know that she did that.”, but let’s think about the stakes for each of them, because we can’t just apply right to be forgotten whenever we feel like. That’s currently how Google does it, but we should probably try to be fair about it, so let’s say the stakes for her, what’s going to happen to her if her name and then this, comes up? Maybe she might get in trouble with her employer, maybe she gets even fired, although this really depends on the employer, maybe she’ll get sensitivity training, maybe she’ll lose that one black friend that she had.
What are the stakes for him? Because there’s quite a lot of police call and arrest data that’s being used for predictive policing. There are numerous jurisdictions that are actually using arrests without double checking that any conviction was ever made, there are many departments doing that. They’re feeding it into automated and machine learning systems, and they’re saying, “Yes, no, it’s totally fine.” but what I recommend there is that these systems are proven to be feedback loops, feedback mechanisms, mathematically that if you have an overabundance, let’s say an over representation of, let’s say, black men in incarceration and/or in arrests, whether they’re lawful or not, and then you feed it into a model, and then you send more police to the neighborhoods where black men tend to live, and then you continue the cycle. It’s mathematically proven, it makes a lot of sense if you just think about it.
The stakes for him, if this data then goes somewhere, if this right to be forgotten is not implemented and his data is then used as another arrest statistic, is that him and/or people like him may be negatively affected, continually negatively affected by this data being available. We can see here, perhaps, a little bit of how privacy, fairness, and privilege operate together when we think about machine learning and automation in our country.
I want to close here with blind justice. This is Lady Justice, also sometimes referred to as Blind Justice, this is an icon for the times that really says she is just. She doesn’t see things like your skin color, she doesn’t see things like your gender, she doesn’t see things like where you live or what type of phone you have, this is not a way that she judges you. What I want to challenge us to do is when we’re thinking of applied machine learning, when we’re thinking of how we treat user data, when we’re thinking of all of this, that we think about whether we’re treating data like Lady Justice would.
Are we implementing data collection that allows users to control their privacy or are we just telling them, “Don’t worry about it, we got it. We’re totally doing all the security stuff, don’t worry about it.” Are we being open and transparent about the scales that we’re using, or are we just saying, “No, we’re doing the fairness research, it’s totally fine. I can’t really answer questions about that because my employer doesn’t let me answer that question.” or are we pushing ourselves to a new plateau, a new ethical grade where we say privacy is actually important, it’s connected with fairness, and users deserve minimally that.
I don’t want us to be on the wrong side of history. I don’t want us to be those people when we walk outside of the building with a homeless person and we’re not concerned about privacy, or fairness, or implementing anything. We’re instead concerned about implementing whatever our employer tells us to do. What I hope is that we can be this push forward, we can be the advocates for privacy, and that we can essentially be that last stand, and I believe this is the last stand for implementing truly fair and private algorithms.
See more presentations with transcripts
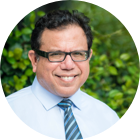
MMS • RSS
Article originally posted on InfoQ. Visit InfoQ

Low-code provider Appian recently released a survey conducted among IT developers to gauge their satisfaction at work. According to the survey, the three worst aspects of IT jobs are time spent troubleshooting application issues, pressure due to time constraints and deadlines, and time wasted on repetitive coding task.
Appian survey also gives a rough idea of the kind of applications IT developers are increasingly asked for, namely those aiming to integrate emerging technologies with legacy systems. This effort is often aimed to improve customer experience and engagements, to optimize internal processes, and to enable innovation.
Being Appian itself a provider of low-code development solutions, it’s no surprise that, among respondents to the survey, 80% stated that low-code can help improve their satisfaction at work by automating repetitive tasks and freeing up time to work on higher-level projects. InfoQ has spoken with Malcolm Ross, vice president of product at Appian, to learn more about how low-code can help accomplish that.
According to your survey, a large portion of surveyed IT developers think that a low-code platform can positively impact their work. Would you please summarize what are the benefits of low-code and how it can be used by IT developers?
Appian’s recent survey shows that nearly 80% of IT developers say low-code can improve key aspects of their job satisfaction and it’s with good reason. Often times, the development of applications required in today’s competitive business climate calls for extensive coding knowledge and effort, meaning organizations must invest far more time and resources than they can manage. That’s why low-code platforms are becoming so important in the developer world, low-code is essential for removing the barriers that stand in the way.
By lowering the requirement of extensive coding and replacing it with visual, drag-and-drop tools, low-code simplifies the development process and strengthens the connection between IT and meeting business needs. With traditional, code-intensive processes gone, developers are freed up to focus on more innovative and forward-thinking projects. In fact, Appian’s survey found that nearly 80% of developers believe that using low-code can free up time to work on higher-level projects. And 80% agree that low-code is useful for automation of repetitive development tasks, such as coding forms and business rules. Ultimately, this means faster development, more powerful and intelligent applications, and a lower cost.
The promise behind low-code platforms seems high-valued. Yet, some people think low-code cannot scale within large organizations and others even go so far as to say low-code platforms promise cannot be actually fulfilled. How would you frame those claims? Have you got any significant use case to disprove them?
While it may be the opposite of what many think when they consider low-code, enterprise-grade low-code is what empowers an IT organization to fully meet the growing and complex needs of a business at scale while fostering widespread innovation. That may not be the case for every platform, but today leading enterprise-grade low-code platforms can manage complex processes and large data sets with high security, scalability and reliability. In fact, what we’ve seen recently with the rapid rise of widespread access to new applications, software and services in the digital marketplace is evidence of the scalability and impact that low-code is having on the industry.
Thanks to enterprise low-code offering faster and simplified development at a lower cost, organizations can scale applications for any project, program, or business, no matter how large, without sacrificing security, quality and reliability.
The IT world has been recently revolutionized by DevOps and containerization. How do you see the two trends coexisting? Are there any synergies or leverage between them?
Containerization is the modern way to deploy applications while low-code is the modern way to create applications. The two co-exist perfectly. In fact, Appian makes it easy to run its platform in Docker containers, allowing Appian servers to be easily scaled to accommodate higher load. Pairing low-code with containers amplifies the benefits and allows for streamlined and cost-effective development processes, which ultimately equates to more powerful applications that meet growing business needs. Low-code is a technology that helps promote a DevOps approach to development. Once the “building blocks” are in place, developers can create apps rapidly and also make changes across all platforms simultaneously much more easily than with custom coded applications.
Conducted by IDG, Appian “Impact of Low-code on IT Satisfaction” survey can be downloaded from Appian website.
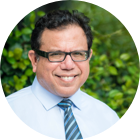
MMS • RSS
Article originally posted on InfoQ. Visit InfoQ

Transcript
Hi, my name is Hannah [Howard]. Before we start, I’m going do that real quick self intro. If you like this talk and you want to find me on the internet, I usually go by the handle @techgirlwonder, I use she/her pronouns, that’s my logo. I’ve only done one good drawing in my entire life, and I intend to milk that for at least a decade.
I work for a company called Carbon Five. We are a product development agency, we work with all kinds of companies. We take their products basically from conception to completion, and from startups to enterprise. We are both hiring and hireable, so talk to me afterwards if you’re into it. And finally, here is my dog, and she’s pretty cute, obviously, and she can vouch for me so you know that I’m a nice person or something.
What is a Computer Program?
So this is a talk about reactive programming, and why I believe it is a better way to write frontend applications. It’s in five parts. Here we go. Hello to all of the legendary children, and welcome to the functional reactive programming ball. Tonight the category is, “What is a computer program?” So there’s a very important computer science textbook that I refer to frequently, and that textbook defines a computer program as “a sequence of instructions for performing a task designed to solve specific problems.” The part of this that stands out to me is this phrase, “sequence of instructions.” And the reason it stands out is, whenever I think about it, I think of like a to-do list, right? You imagine programming in a very sequential order.
If we were to take this into a different context, like a classroom, you might think of it as a lesson plan for the lesson. “First I’m going to do this, then I’m going to do this, and then I’m going to do this.” But is that really an accurate metaphor? So if you are programming, maybe a command line utility, it’s probably pretty accurate, because you’re just executing things sequentially. But the context of this talk is frontend programming. And so if you’re doing frontend programming, I have a different metaphor that I think applies better.
And that’s the unruly classroom, because in frontend programs, they don’t run sequentially. There is no sequence. In fact, the flow of execution is constantly interrupted by user input, right? Whether you’re talking about user input or you’re talking to a server that may or may not be responsive, there’s a million other things. And I actually would argue that the central challenge of writing frontend programs is dealing with interruptions.
A Brief History of Interruptions
So to talk about that, let’s look at a brief history of how we’ve dealt with interruptions in the context of frontend programming. So I started programming in the early ’90s-ish, and at the time, the very first type of programming I ever did was DOS game programming. You all are going have to bear with me while I do one thing, because I just realized that I did not do one small piece of setup here. Just give me one moment. You know that you’re writing your presentations with code when you have to clear local storage in order for your presentation to work. Let’s refresh. Thank you all for bearing with, I appreciate it. I was so close to a flawless presentation, but not today. And that’s still not there, but whatevs.
So I started programming in C. Now, I don’t know how many of you all have ever programmed in C. You don’t have to have programmed in C to get this example. Basically, the way it would work is, if you wanted to accept user input, you had this facility whereby you could override something called the BIOS. And the BIOS was this super-duper low level, almost hardware piece of like code, and you could basically jump in the middle and say, every time there’s a key tapped on the keyboard, I want to handle it, right? And then your new keyboard, this would be like your new keyboard code, and it would probably do something like collect key presses in some kind of a buffer.
And then your whole program would look like a loop. And the loop, the contents of it, would be, basically you would on each iteration, you would check some kind of input, whether it’s keyboard, mouse, joystick, or whatever, and then you would process it and do something with it. So you can imagine a bus of events. And you’d basically do that until you were done. Now, there’s one problem with this model, which is, if you wrote a bad piece of code to handle the keyboard handler, like in this case an infinite loop, you’ve actually taken over the keyboard. So there is no control-C. Your program is running forever, and you’re going to have to restart your computer.
Briefly, I did some programming in Windows. And Windows was basically the same model, except it did one innovation, which was they decided to not give regular programmers direct access to the hardware, which was probably a good idea. But if you look at the actual code, it looks pretty similar. You’re again just doing this sort of infinite loop, where you take each message, you do something, you try to read it, figure out what it is, and then process it. And then the main code that you would write as a Windows programmer is a program to look at each message, basically decide which type of message it is, and update the state of your application.
So that takes us up to about 1999, when we realized that the global event bus was a limited pattern, and we left it in the dust for better things. Or did we? Because if you look at this code, right, and you look at the core logic of your program being a switch statement that takes a message and then updates the state based on that type of message … How many people here are programming in React, or have programmed in React? All right, so if any of you all are using Redux, this might look a little bit similar, because it’s basically a reducer, right? And, in fact, Redux uses a lot of the patterns of the global event bus in its design.
So, while programming in Redux may be programming like it’s 1999, there are some good reasons that they went that way, and I’ll talk about that in a bit. But, let’s move on to what I consider sort of the core pattern of doing UI programming that we’ve used as, effectively since we moved off of the global event bus, and that’s this thing called the observer pattern. And I’m going to explain it in a little bit of detail, because it’s really important to understanding the things we’re going to talk about in the rest of the talk.
I’m going to do a short digression to explain the observer pattern, and it’s into the world of influencers. So, let’s say you’re an influencer on the internet, and you’re a content creator, and you’re wondering that very important question, how are people going to see my awesome content, right? So there was an old way of doing it in the pre-internet age, which is you tracked people down and you were like, “You’ve got to check out my thing and watch this.” This is when producers would stand out on the street and be like, “Check out my album.” But, in the modern age, we have this better model for doing this. If you are a content creator, you essentially say, “I’m going to publish content.” And then me as your adoring fan, I go on to one of our wonderful, scary surveillance social media platforms, and I subscribe to your content. And once I’ve subscribed, when you make content, I will get a notification that you’ve made new content. And that’ll give me the opportunity to watch it.
And this model is effectively what the observer pattern is. You have these two concepts, the subject and the observer. The subject is analogous to the content creator, and the observer is analogous to the fan. And the subject essentially says, “I’m going to produce some kind of event.” The observer says, “I want to subscribe to that event.” And then whenever that event happens, the observer gets notified and can choose what to do with it. And if you’re programming in JavaScript, you’ve actually probably done this a few times. I’m having a little trouble with this but, yes. So, if you’ve programmed in JavaScript at almost any point in the web, you’ve actually implemented this pattern and you may not have known it.
So, in this scenario, we’ve got two elements on the page. We have a switch, or a button you can click, to toggle the content of another content box. So imagine it’s like a light switch, and when you click on it, the goal is to change the text in the other box from on to off, right? So how do you implement this? This piece of code is how we would normally look up a DOM element. In this case, we’re looking up the switch, right? And then we’ve written a function here that when called, essentially flips the text in the other element, the toggle from on to off. So how are we going to connect these two things?
So we have this function that you can call, and this is part of the DOM API, where you can add an event listener to a user event on a DOM element. In this case, we’re adding an event listener to the click event on the toggle switch. And by doing that, and then we pass to it a handler, and by doing that, we convert our modifyText function into an observer. So now, essentially, every time I click, the modifyText function is going to get called, and that will trigger, that will cause the switch to change. So this is effectively the observer pattern, and you’re using it every day.
So how does this compare to the global event bus? Well, one thing that’s great about it is that it’s way simpler. You’ve probably used this pattern a million times without even thinking about it. We wanted to process, we wanted to set up one user interaction, so we set up one observer and one function, and then we were done. It’s basically much more localized. You don’t have to deal with all the events you don’t care about.
The one downside to this is you do have to set up subscriptions. So you do write a little bit of code to subscribe to the events, so that you can subscribe to these different events. And that works really well, but there are certain cases where it gets a little complicated. I don’t know if anyone here has ever attempted to implement drag and drop, not with the current browser APIs, but with the old fashioned, mouse down, mouse move, and mouse up. It’s pretty complicated, because what you end up doing is you have one handler listening for the mouse down. Once you get the mouse down, you set up another handler to handle the mouse moving, and then meanwhile you have to also have a handler and the mouse up that removes the mouse move handler when it’s done. It’s like quite a bit of stuff.
The issue here is you’re mixing two concerns in your code. On the one hand, you’re handling events, but then in complicated user interactions, your event handlers themselves, end up having to handle the task of setting up and tearing down subscriptions. And it gets pretty complicated. So the observer pattern is great, and it’s reliable, but it has some downsides. And although this is not an official story, this is my notion of how Redux might have gotten created, because they might have been looking at the observer pattern and being like, “This is actually getting kind of cumbersome. Maybe we should give this global event bus a try.” But, maybe there’s another way to do this that offers the convenience of the observer pattern, but with the flexibility of the global event bus. And that is what the rest of this talk is about.
Functional Reactive Programming
I want to talk about functional reactive programming, and how you can use it to write frontends like a boss. So I want to go back to our classroom analogy. Once upon a time, I don’t know if this was in my bio, but I actually was a middle school teacher. But I was really bad at it. So don’t learn from me. I want to look at how good teachers handle that situation, this unruly classroom. So I don’t know if you’ve ever watched a good teacher in action, but they’re almost like Jedi. They have this way where like a student is interrupting, and they just sort of like Obi-wan them back into, you want to be, this is the lesson you’re looking for. So how do they do that.
Well, the first thing is that teachers don’t start with a strict lesson plan. They have a lesson plan, but it’s kind of like a guide. Because if you start with a strict plan, and you try to stick to it rigidly, you’re going to get interrupted. Instead, you can think of teaching as kind of like a series of semi-planned reactions to different types of interruptions. And really good teachers, if a student does something, they know how to not just respond and bring that student back to the lesson, but to actually take that interruption and kind of fold it into the flow of the lesson in a way that just sort of inevitably flows toward the final objectives that they’re trying to get to for the overall lesson. So it is kind of like Jedi mind control. And that’s why teachers are actually way more talented workers than most of us. And I think we ought to pay them like we pay us, but, sidebar.
How could we write programs like this? Could we write programs as a series of planned responses to interruptions? What would it look like? Well, so I want you to consider a statement, “Y equals X plus 3.” You’ve probably written something like this somewhere along the line in your history of being a computer programmer, right? And in the imperative context, this means something very specific. It means take the value of X, add three to it, and assign it once to Y. If X later changes, it’s kind of like Y still has the value you assigned it.
But let’s think about that statement in a different context. In math, Y equals X plus 3 actually describes an entire line on a graph, with the whole slope-intercept function, whatever. So it doesn’t just describe one assignment of like Y to X plus 3; it describes an entire possible line you could plot on the graph. And in reactive programming, this statement would express a relationship between Y and X. So X is a value that can change over time, and Y is a value, and essentially this says that Y is a value that will change every time X changes, and it will always have the value X plus 3.
So you can imagine X, let’s imagine it as like a stream of values over time. And then, if you imagine it as a stream of values over time, if you were to express the Y stream, it would essentially be another stream of values, where each value emitted was the same, was essentially the value emitted by X, plus 3. And if you think of this concept of a stream of data, it doesn’t necessarily have to be actual numbers. It could be anything. It could be a stream of mouse clicks. We could just track every time the user clicks the mouse over time.
So how would you program like this? Well, that seems really cool in theory, but most of us know that at least somewhere under the hood, there’s a computer sequentially executing instructions, like the original definition said. So how would we program like this? What we would need is some kind of pattern so that as each value changes over time, we could subscribe to those changes and get notified and then be able to respond. So that pattern is the observer pattern. We just need to add a few things and extend it a little bit. So the observer pattern kind of assumes that we’re dealing with some kind of user- sorry, I’m going to go right to the next slide. So, we’re going to rewind. What I’m getting at is we’re going to introduce this concept called an Observable. An Observable is like a subject, except that it could be, you could observe anything. It’s just effectively a stream of any kind of value that changes over time. So how could we program with this? Let’s look at how this could work.
So imagine we have an X, and we’ve said it’s a stream of data values, a stream of numbers, that changes over time. If we wanted to print out the values of X, we could just subscribe to it. This would just be the standard observer pattern. And then, we might say, let’s say we want to print out the values of Y. Well, we could subscribe to X, and we could just print out whatever was emitted plus 3. And this would give us an output of the values of Y. But what if we wanted to actually listen to Y itself? How would we do that?
So let’s step back for a moment and just rewind to something a little more familiar, and imagine X is just an array of numbers, right? In most computer programming languages, if we wanted to calculate Y as the array of numbers that are in Y, based on the array in X, we could use an operation like map. That’s usually what most languages call it, but if they don’t, they often have some kind of operation like this. So in the Observable case, it basically functions the same, and we’re going to use an operator that looks exactly like the operator we would use in the context of an array. The difference here is that this is not all happening at once. This is a map of values over time. So the map happens continuously as an X, the new X value appears, its mapped, and then you get a new Y value from that.
So let’s look at this in a UI context. In this case, we have two buttons on the screen and a ball. Just bear with me with the abstractness of this. And you can say that, essentially design this so when you click on each button, the ball goes from one end to the other. So how could we implement this UI interaction with Observables? So let’s say that we have- just for the moment, let’s imagine we can write an Observable that emits the left button clicks and the right button clicks. So essentially as I click on each side, those Observables emit. Now if we have that map operation, we could transform each of these clicks into an actual semantic event. So I could effectively translate the click into a desired position I want the ball to be in. So you can see it’s clicking and then it’s being transformed into a right as a desired position. And if we merge these two streams of positions, we actually get the current position of the ball. So that’s how we could assemble this UI interaction using Observables.
So let’s look at what that looks like in code. Sorry for this little situation. So for the rest of the talk, I’m going to use a really great Observable library called RxJS. And our remaining examples are going to be all in JavaScript, but there are great Rx libraries in actually almost every major programming language, which means you can apply these patterns to UI programming in a native Android context, a native iPhone context, or almost any UI platform. So the first step is to look up the two DOM elements, so this is pretty standard. (Ignore that, cancel. Preview of the rest of the talk.) Now, we need to create two Observable streams of the click events on each button. So the simple thing about this is that RxJS just provides us a function to do this. It’s essentially a function called fromEvent that takes a DOM element and an event and produces an Observable stream of those events on that DOM element.
Now, we would use the map operation to convert the fact that those things are occurring to an actual position. Now, this syntax might look a little different, and this is partly because RxJS has specific syntax around this, where it wraps all these operations in this pipe function, but it’s essentially the same thing. We’re mapping a fact that a click occurred to a desired position. And then finally, RxJS provides a function to merge two streams. So we’d just use this merged stream. We’re merging it and then we’re adding one more thing, which is we’re inserting an initial position. And this produces the desired position of the ball. So that’s basically it.
How Do I Actually Use This?
We’re probably about 25 minutes in, and you’re already on board, you’re ready to write every single program that you work on this way. But maybe you need a little bit of guidance on how to do this in the real world. So before I get into this, I am going to return to the slide from Laurie’s talk, if any of you all were here before lunch. He showed this graph of all these different technologies that are being used in the React ecosystem. And I saw his talk at a couple conferences ago, and when I saw it, I was like, “Wait a second, what is the green line?” And then I was like, “Wait a second, is that, oh my god, it’s RxJS and it is the most popular library in the world. It is more popular than React.” So, yes, how did that happen? He said I would explain it. Well, the short answer is that people who write some pretty important libraries are using RxJS. RxJS is in the tool chain of libraries for ESLint, and it’s also heavily depended on by the Angular framework. So you combine those two together, you get some massive usage.
Now, this isn’t saying that’s how you use RxJS, it’s just saying that the folks who write a lot of the libraries that you depend on are actually using this as a tool to write their own code. But let’s say you want to put Rx in your programs in your own user space code. There’s a couple questions you need to answer. One is, how do I actually architect real applications using RxJS? And then the second is how do I integrate this with my current application stack?
So, for this scenario, to explain how you would build a program with RxJS, I want to look at a more real world scenario. And we’re going to look at a scenario that you’ve probably all dealt with, which is a login form. So I have like a really silly login form demo here where I can enter a username and password, I’m probably going to want to provide some feedback if the wrong thing is entered. I may want to disable the Submit button while a login is in progress, because it’s probably going to need to go out to a server, and I hope not to have double logins. So, it’s a little more complicated functionality here. So how would we implement this with RxJS?
Well, let’s start from the thing we’ve like already seen, which is that we can make UI inputs- I don’t know if you all can see that, so that says username, password, and Submit button- and convert them to streams. So I can imagine this username, password just emits every time that I type in a new username and the password, same, this is a super secure password form where you can see what I’m typing. And then whenever I click Submit, I get a submit. So if I have all those, we can convert this into effectively a stream of login attempts. Because what is a login attempt? A login attempt is basically combining the last typed username and password every time that a Submit button is clicked, and sending it off to a server. So I could produce a stream of effectively login attempts.
And so then, from login attempts, I can start build, deriving other things. When the response comes back from the server, I could create a stream of responses. And then once I’ve got that, I can do a bunch of other stuff. I could derive whether there’s a login in progress from the time between when a login attempt occurs and when a login response occurs. Once I have a response, I might need to filter it out into successful logins and error logins. And then once I have those, I can convert them to stuff. The login failure, I might be able to extract a stream of failure messages out of it, and the login success I might extract a user token from. And then we could use that user token to kind of feed into another stream of things that could occur. I might use that user token to actually go get a protected resource. And so I can build this entire pretty complicated logic tree from different combinations of Observables.
So if I submit a bad username and password, you can see that it essentially filters all the way down to a failure message. And if I submit a good username and password, and I’m never sure if I’ve set this up correctly. QCon and awesome, let’s try that, see if that works. So I’m submitting, it was a success. So that becomes a user token, and then I go make another API call to a protected resource, and I get my protected stuff. So what would this actually look like in the codes? You’re probably like, “well, that was really cool watching little things move around the screen, but doesn’t sound like actual computer code.”
First of all, let’s imagine I’ve just made a fake abstraction of an API here. It has a login endpoint and it has a protected resource endpoint, and we can assume that both of these return promises. Okay. So here are our three user inputs, so in this case, I’m using something slightly different than I was before. I’m not going to get too into this, but there’s this concept of a subject, which you would use to assemble a stream from user input. So we have a username, a password, and a Submit button. And we could produce our login attempts by essentially, whenever the Submit button occurs, we attach to it the latest username and password emitted. And there’s an operator for this called withLatestFrom.
Once we have that, we could convert that attempt into an API call. If you look at this login attempt code, what I’m doing is on each event, sorry each emission, I’m doing a call to our API and submitting the username and password. Now, an API call, in this case, we’ve said our API returns a promise. So we don’t want a stream of promises because that’s not very useful data to us. We want a stream of what actually it resolves to. So there’s an operator for this, and that operator, it’s little bit oddly named, it’s called mergeMap. And what that’ll do is it’ll take this map function, which produces a promise, and it will convert it all to a stream of the actual unwrapped values when the promise returns.
You can again derive the login in progress by just merging the attempts and the responses mapped to essentially the true or false state. And you obviously want to start with it not being in progress. Getting the login successes is really easy. There’s just another operator called filter, which effectively takes a stream and produces a stream where the only values that are emitted are the ones that satisfy some condition. In this case, we’re filtering the responses that have status of success, and the failures are basically the same thing.
And then, once we have those, we convert to semantic data. In this case, we’re mapping our login failures and pulling out the error message from within the payload returned from the server, and producing a message that we then display on the screen as feedback to the user. You can imagine the user token operates the same way. And then once we have the user token, we can effectively start this process over again. We can take that user token and map it to a new set of API responses. And I’ve got the order a little bit different, but you’re effectively doing the same thing with the mergeAll, where you’re converting a stream of promises to a stream of actual data coming back. And that’s basically how you can build a pretty complex piece of user interaction functionality and state logic with Observables.
So there is a concept, you’ve seen me draw these diagrams a couple times with these like emissions from Observables, and it’s a way to conceptualize how data propagates through a program that’s built with Observables. It’s called a signal graph. And in this case, in the case of a signal graph, the signals are emissions from the different Observables, and the graph is how they’re all tied together. And that’s basically the fundamental architecture pattern for building programs using Observables. Our programs become a series of, effectively, reactive data streams, starting with the first user inputs, which we call primary signals, and then propagating to all of these other derived streams of data, and taken together, those form the signal graph. And where I work, at Carbon Five, we actually build a number of programs with RxJS, and we’ve developed a practice of maintaining the signal graph as an independent drawing that we kind of keep alongside our code base, that tracks how the logic of our program is developing. And this is taken from a real world application.
Now, if you actually do this, and we’ve been doing this for a couple years, and we’ve noticed that there are certain challenges that do come up, you’re probably wondering how do I actually test all this? You probably want to make sure your graph doesn’t end up having cycles in it, because that could produce a lot of unpredictable results. Although I’ve glossed over it, there are a few idiosyncrasies in RxJS. If you Google hot and cold Observables, you will learn a lot about that. And as your program grows, one thing that we’ve found is the graph gets really big, and that drawing gets real complicated. So one thing that we’ve been struggling with is do we maintain just one, conceive of just one graph, or do we have, imagine there’s multiple graphs to handle different features, areas of the program, and if so, how do you connect them?
And finally, the graph diagrams tend to take a lot of time to maintain, which doesn’t feel very agile. We’re supposed to be valuing, working software over documentation, I don’t know if I’m getting my words right there. Anyway, so, but we’ve been working on it for a couple years, and we’ve developed a lot of good practices for it. And so recently, I was like, “What if we take all these practices and combine them all into like a library that other people could use?”
So I want to talk briefly about a library that I’ve written called Signal. And it’s basically a library for doing state management in your frontend JavaScript apps using signal graphs. So let’s take a look at how it works. Awesome, cool. So the first thing you do when you use Signal is you define a signal graph. And there’s a really simple DSL for adding different signals for your graph. You can define primary signals and then you can define derived signals. And I’m going to not get too deep into this DSL, but essentially, you have primary signals, and everything after that is derived signals. And the nice thing about this, I always think of it as a routes file for your frontend application, where you can see all the different things that are going to go into this big piece of logic.
And in terms of actual operations of the derived signals, you define a name, you can see that addDerived, you give a name to the derived signal and then you define a factory function for actually making that signal. And the reason you do this is it’s really easy to test. So you can see that I’m making a factory function for login attempts that takes as parameters the Submit button, the username, and password, and then produces the resulting signal. So the nice thing is, this is a pure function. I can open up a test and dependency inject it with mock versions of these signals, and they’re pretty easy to test then.
And then once you define your graph, you can use a function called initializeWith to hydrate your graph with state. One of the challenges that we’ve come up against, is how do you set up the initial state of the program. And then finally, you build your graph, and there’s just a function to build the graph. And the nice thing about this is Signal is going to take the definition you’ve provided, along with all the definitions for how you’re going to build your Observables, and it’s going to handle all the work of setting all that up for you. It’s also going to handle stuff like avoiding cyclic dependencies.
And then once you’ve defined one graph, if you need to define another graph and connect it, it provides an API for connecting basically two graphs together by connecting a, in this case, it’s a primary signal for the top graph with a derived signal from the other graph. Now, you might be wondering what are all those strings, this looks very Angular 1-ish. Well, yes, it is string dependency injection, however, if you are using TypeScript, this is all super-duper locked down. And so you can’t type in the wrong thing there. It will complain if your types don’t match. So it’s actually a really specific system for doing dependency injection, if you’re using TypeScript, which is a really awesome language. And stay tuned, because we’re talking about that next.
So it is available now. I didn’t want to crimp on my favorite messaging app’s name, so I put it under a namespace. You can install it at npm install @rxreact/signal. Caveat is, I put out like two weeks ago. So, use at your own risk. Or in production. What could go wrong? One thing I haven’t been able to get yet, and this is one of the things I really want to get, is an actual thing that will build automatic graph visualizations. So those visualizations you saw earlier, they are really code, they really do operate based off the user input you give to the form. But I would like to be able to just generate them, though right now, they’re hand coded. And I believe it’s possible, I just haven’t been able to get it there.
All right, finally, integration. So now you want to, you’ve got an idea of how to write applications, but you’re working in a context where you are integrating most likely with a JavaScript framework. So how do I tie all this work into traditional JavaScript framework coding? Well, the good news is if you’re using Angular, step one is there is no step one. Angular authors integrated RxJS from the beginning, and there’s some interesting tooling around it as well, but it’s basically part of the framework. But, as we saw earlier in the last talk, Angular, not the most popular framework.
You’re probably wondering about the more popular framework, AKA React. And this is sort of the origin of where I got started. I really want to use my React with my reactive programming, and there hasn’t been that much tooling out there for it. So, I work at a consultancy. I can’t just basically be like, “I’m going to throw in a bunch of obscure reactive programming tools, and then hand it off to your client dev team.” That would be somewhat irresponsible. So I’ve been wanting to build tooling such that that maybe takes off and then maybe we can get a movement going to do reactive programming with React.
So, the good news is, I have something else for this, and that’s where the namespace comes from. All of these tools are under the heading “rxreact” because they are tools to integrate React with RxJS. There are actually a few things out there. This is just one example that I happen to be showing because I’m the person giving the talk and I wrote it, but there are several tools out there and many of them are quite good. So the first tool that I’ve written is a library called signal-connect. And this is basically, if you are using Signal and you want to connect the outputs of the graph to React components, you can use this function called connect. It looks a lot like Redux.
In this case, you can imagine we have a box; we have the box that’s moving around, this is from the previous example, and we want to connect that to the output of the position stream. So we just use this connect function, and we essentially provide it the signal graph we’re using, the properties we want to set on the React component, and then the name of the stream we want to use to feed that React component.
We can do the same thing in reverse with our primary signals, where in this case, we’re taking our left button, and we’re taking the component, which has an onClick handler, and we are passing as a property onClick and using the leftClick primary signal. And this will essentially connect that event handler, so when I call the onClick function, it emits a value on the leftClick stream. And that’s basically all there is. And one thing that is really cool about this, it kind of goes off the bottom of the screen, but that entire login form component that you saw is about 12 lines of code to connect that entire, the form, to the signal graph.
Used Car Sales Portion
So this is the last portion of the talk, and it’s what I call the used car salesman portion, because I’m going to just cover a few more things that I’ve written very quickly. And this is also the part where my notes get all sparse. I’m obviously a little bit tired today, because I was just over canvassing in Nevada yesterday. So there’s another library. If you’re like, “I don’t really get the signal graph thing, I’m not really into it. I just want to connect React components to Observables.” The original library I wrote was called rxreact/core. And it’s a simpler version, and the one nice thing is I’ve been developing this one for a while, and it’s basically just to directly connect; if you have an Observable, in this case, here’s our leftClick Observable, and we’re directly connecting it to an onClick property on the left button. And it’s essentially the same thing but without all of this structure of the signal graph.
Obviously, some of you guys might be into TypeScript. You should totally hang around because the next talk is about TypeScript, continuing the JavaScript track theme of cross-marketing our talks. So stay tuned for Lauren’s talk about TypeScript. And the nice thing is, while I’ve kept all my examples in JavaScript, all of this code is written in TypeScript, because I love TypeScript. It’s kind of how I like to write my code.
So there is that. There is also- if you are like, “I really like reducers, because I’ve been programming in Redux,” I’ve been working on a version of this that uses a reducer model combined with Observables to provide an API for backing your components with state. That’s very similar to the ReasonML spec. Anyway, that’s another thing. That’s going through various revisions. That’s not out yet, but it will be. I’ll have it out soon. Yes, that’s the name of that one, and that’s all I have. Vote “Yes” on Prop 10 tomorrow, I’m just going to say that. And thank you all for bearing with me.
See more presentations with transcripts
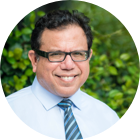
MMS • RSS
Article originally posted on Data Science Central. Visit Data Science Central
By Avrim Blum, John Hopcroft, and Ravindran Kannan (2018).
Computer science as an academic discipline began in the 1960s. Emphasis was on programming languages, compilers, operating systems, and the mathematical theory that supported these areas. Courses in theoretical computer science covered finite automata, regular expressions, context-free languages, and computability. In the 1970s, the study of algorithms was added as an important component of theory. The emphasis was on making computers useful. Today, a fundamental change is taking place and the focus is more on applications. There are many reasons for this change. The merging of computing and communications has played an important role. The enhanced ability to observe, collect, and store data in the natural sciences, in commerce, and in other fields calls for a change in our understanding of data and how to handle it in the modern setting. The emergence of the web and social networks as central aspects of daily life presents both opportunities and challenges for theory.
The book is available and freely downloadable here. For more information, visit this Microsoft webpage. More free books are available here.
Contents
1 Introduction 9
2 High-Dimensional Space 12
2.1 Introduction . . . . . . . . . . . . . . . . . . . . . . . . . . . . . . . . . . . 12
2.2 The Law of Large Numbers . . . . . . . . . . . . . . . . . . . . . . . . . . 12
2.3 The Geometry of High Dimensions . . . . . . . . . . . . . . . . . . . . . . 15
2.4 Properties of the Unit Ball . . . . . . . . . . . . . . . . . . . . . . . . . . . 17
- 2.4.1 Volume of the Unit Ball . . . . . . . . . . . . . . . . . . . . . . . . 17
- 2.4.2 Volume Near the Equator . . . . . . . . . . . . . . . . . . . . . . . 19
2.5 Generating Points Uniformly at Random from a Ball . . . . . . . . . . . . 22
2.6 Gaussians in High Dimension . . . . . . . . . . . . . . . . . . . . . . . . . 23
2.7 Random Projection and Johnson-Lindenstrauss Lemma . . . . . . . . . . . 25
2.8 Separating Gaussians . . . . . . . . . . . . . . . . . . . . . . . . . . . . . . 27
2.9 Fitting a Spherical Gaussian to Data . . . . . . . . . . . . . . . . . . . . . 29
2.10 Bibliographic Notes . . . . . . . . . . . . . . . . . . . . . . . . . . . . . . . 31
2.11 Exercises . . . . . . . . . . . . . . . . . . . . . . . . . . . . . . . . . . . . . 32
3 Best-Fit Subspaces and Singular Value Decomposition (SVD) 40
3.1 Introduction . . . . . . . . . . . . . . . . . . . . . . . . . . . . . . . . . . . 40
3.2 Preliminaries . . . . . . . . . . . . . . . . . . . . . . . . . . . . . . . . . . 41
3.3 Singular Vectors . . . . . . . . . . . . . . . . . . . . . . . . . . . . . . . . . 42
3.4 Singular Value Decomposition (SVD) . . . . . . . . . . . . . . . . . . . . . 45
3.5 Best Rank-k Approximations . . . . . . . . . . . . . . . . . . . . . . . . . 47
3.6 Left Singular Vectors . . . . . . . . . . . . . . . . . . . . . . . . . . . . . . 48
3.7 Power Method for Singular Value Decomposition . . . . . . . . . . . . . . . 51
- 3.7.1 A Faster Method . . . . . . . . . . . . . . . . . . . . . . . . . . . . 51
3.8 Singular Vectors and Eigenvectors . . . . . . . . . . . . . . . . . . . . . . . 54
3.9 Applications of Singular Value Decomposition . . . . . . . . . . . . . . . . 54
- 3.9.1 Centering Data . . . . . . . . . . . . . . . . . . . . . . . . . . . . . 54
- 3.9.2 Principal Component Analysis . . . . . . . . . . . . . . . . . . . . . 56
- 3.9.3 Clustering a Mixture of Spherical Gaussians . . . . . . . . . . . . . 56
- 3.9.4 Ranking Documents and Web Pages . . . . . . . . . . . . . . . . . 62
- 3.9.5 An Application of SVD to a Discrete Optimization Problem . . . . 63
3.10 Bibliographic Notes . . . . . . . . . . . . . . . . . . . . . . . . . . . . . . . 65
3.11 Exercises . . . . . . . . . . . . . . . . . . . . . . . . . . . . . . . . . . . . . 67
4 Random Walks and Markov Chains 76
4.1 Stationary Distribution . . . . . . . . . . . . . . . . . . . . . . . . . . . . . 80
4.2 Markov Chain Monte Carlo . . . . . . . . . . . . . . . . . . . . . . . . . . 81
- 4.2.1 Metropolis-Hasting Algorithm . . . . . . . . . . . . . . . . . . . . . 83
- 4.2.2 Gibbs Sampling . . . . . . . . . . . . . . . . . . . . . . . . . . . . . 84
4.3 Areas and Volumes . . . . . . . . . . . . . . . . . . . . . . . . . . . . . . . 86
4.4 Convergence of Random Walks on Undirected Graphs . . . . . . . . . . . . 88
- 4.4.1 Using Normalized Conductance to Prove Convergence . . . . . . . . 94
4.5 Electrical Networks and Random Walks . . . . . . . . . . . . . . . . . . . . 97
4.6 Random Walks on Undirected Graphs with Unit Edge Weights . . . . . . . 102
4.7 Random Walks in Euclidean Space . . . . . . . . . . . . . . . . . . . . . . 109
4.8 The Web as a Markov Chain . . . . . . . . . . . . . . . . . . . . . . . . . . 112
4.9 Bibliographic Notes . . . . . . . . . . . . . . . . . . . . . . . . . . . . . . . 116
4.10 Exercises . . . . . . . . . . . . . . . . . . . . . . . . . . . . . . . . . . . . . 118
5 Machine Learning 129
5.1 Introduction . . . . . . . . . . . . . . . . . . . . . . . . . . . . . . . . . . . 129
5.2 The Perceptron algorithm . . . . . . . . . . . . . . . . . . . . . . . . . . . 130
5.3 Kernel Functions . . . . . . . . . . . . . . . . . . . . . . . . . . . . . . . . 132
5.4 Generalizing to New Data . . . . . . . . . . . . . . . . . . . . . . . . . . . 134
5.5 Overfitting and Uniform Convergence . . . . . . . . . . . . . . . . . . . . . 135
5.6 Illustrative Examples and Occam’s Razor . . . . . . . . . . . . . . . . . . . 138
- 5.6.1 Learning Disjunctions . . . . . . . . . . . . . . . . . . . . . . . . . 138
- 5.6.2 Occam’s Razor . . . . . . . . . . . . . . . . . . . . . . . . . . . . . 139
- 5.6.3 Application: Learning Decision Trees . . . . . . . . . . . . . . . . . 140
5.7 Regularization: Penalizing Complexity . . . . . . . . . . . . . . . . . . . . 141
5.8 Online Learning . . . . . . . . . . . . . . . . . . . . . . . . . . . . . . . . . 141
- 5.8.1 An Example: Learning Disjunctions . . . . . . . . . . . . . . . . . . 142
- 5.8.2 The Halving Algorithm . . . . . . . . . . . . . . . . . . . . . . . . . 143
- 5.8.3 The Perceptron Algorithm . . . . . . . . . . . . . . . . . . . . . . . 143
- 5.8.4 Extensions: Inseparable Data and Hinge Loss . . . . . . . . . . . . 145
5.9 Online to Batch Conversion . . . . . . . . . . . . . . . . . . . . . . . . . . 146
5.10 Support-Vector Machines . . . . . . . . . . . . . . . . . . . . . . . . . . . . 147
5.11 VC-Dimension . . . . . . . . . . . . . . . . . . . . . . . . . . . . . . . . . . 148
- 5.11.1 Definitions and Key Theorems . . . . . . . . . . . . . . . . . . . . . 149
- 5.11.2 Examples: VC-Dimension and Growth Function . . . . . . . . . . . 151
- 5.11.3 Proof of Main Theorems . . . . . . . . . . . . . . . . . . . . . . . . 153
- 5.11.4 VC-Dimension of Combinations of Concepts . . . . . . . . . . . . . 156
- 5.11.5 Other Measures of Complexity . . . . . . . . . . . . . . . . . . . . . 156
5.12 Strong and Weak Learning – Boosting . . . . . . . . . . . . . . . . . . . . . 157
5.13 Stochastic Gradient Descent . . . . . . . . . . . . . . . . . . . . . . . . . . 160
5.14 Combining (Sleeping) Expert Advice . . . . . . . . . . . . . . . . . . . . . 162
5.15 Deep Learning . . . . . . . . . . . . . . . . . . . . . . . . . . . . . . . . . . 164
- 5.15.1 Generative Adversarial Networks (GANs) . . . . . . . . . . . . . . . 170
5.16 Further Current Directions . . . . . . . . . . . . . . . . . . . . . . . . . . . 171
- 5.16.1 Semi-Supervised Learning . . . . . . . . . . . . . . . . . . . . . . . 171
- 5.16.2 Active Learning . . . . . . . . . . . . . . . . . . . . . . . . . . . . . 174
- 5.16.3 Multi-Task Learning . . . . . . . . . . . . . . . . . . . . . . . . . . 174
5.17 Bibliographic Notes . . . . . . . . . . . . . . . . . . . . . . . . . . . . . . . 175
5.18 Exercises . . . . . . . . . . . . . . . . . . . . . . . . . . . . . . . . . . . . . 176
6 Algorithms for Massive Data Problems: Streaming, Sketching, and Sampling 181
6.1 Introduction . . . . . . . . . . . . . . . . . . . . . . . . . . . . . . . . . . . 181
6.2 Frequency Moments of Data Streams . . . . . . . . . . . . . . . . . . . . . 182
- 6.2.1 Number of Distinct Elements in a Data Stream . . . . . . . . . . . 183
- 6.2.2 Number of Occurrences of a Given Element. . . . . . . . . . . . . . 186
- 6.2.3 Frequent Elements . . . . . . . . . . . . . . . . . . . . . . . . . . . 187
- 6.2.4 The Second Moment . . . . . . . . . . . . . . . . . . . . . . . . . . 189
6.3 Matrix Algorithms using Sampling . . . . . . . . . . . . . . . . . . . . . . 192
- 6.3.1 Matrix Multiplication using Sampling . . . . . . . . . . . . . . . . . 193
- 6.3.2 Implementing Length Squared Sampling in Two Passes . . . . . . . 197
- 6.3.3 Sketch of a Large Matrix . . . . . . . . . . . . . . . . . . . . . . . . 197
6.4 Sketches of Documents . . . . . . . . . . . . . . . . . . . . . . . . . . . . . 201
6.5 Bibliographic Notes . . . . . . . . . . . . . . . . . . . . . . . . . . . . . . . 203
6.6 Exercises . . . . . . . . . . . . . . . . . . . . . . . . . . . . . . . . . . . . . 204
7 Clustering 208
7.1 Introduction . . . . . . . . . . . . . . . . . . . . . . . . . . . . . . . . . . . 208
- 7.1.1 Preliminaries . . . . . . . . . . . . . . . . . . . . . . . . . . . . . . 208
- 7.1.2 Two General Assumptions on the Form of Clusters . . . . . . . . . 209
- 7.1.3 Spectral Clustering . . . . . . . . . . . . . . . . . . . . . . . . . . . 211
- 7.2 k-Means Clustering . . . . . . . . . . . . . . . . . . . . . . . . . . . . . . . 211
- 7.2.1 A Maximum-Likelihood Motivation . . . . . . . . . . . . . . . . . . 211
- 7.2.2 Structural Properties of the k-Means Objective . . . . . . . . . . . 212
- 7.2.3 Lloyd’s Algorithm . . . . . . . . . . . . . . . . . . . . . . . . . . . . 213
- 7.2.4 Ward’s Algorithm . . . . . . . . . . . . . . . . . . . . . . . . . . . . 215
- 7.2.5 k-Means Clustering on the Line . . . . . . . . . . . . . . . . . . . . 215
7.3 k-Center Clustering . . . . . . . . . . . . . . . . . . . . . . . . . . . . . . . 215
7.4 Finding Low-Error Clusterings . . . . . . . . . . . . . . . . . . . . . . . . . 216
7.5 Spectral Clustering . . . . . . . . . . . . . . . . . . . . . . . . . . . . . . . 216
- 7.5.1 Why Project? . . . . . . . . . . . . . . . . . . . . . . . . . . . . . . 216
- 7.5.2 The Algorithm . . . . . . . . . . . . . . . . . . . . . . . . . . . . . 218
- 7.5.3 Means Separated by Ω(1) Standard Deviations . . . . . . . . . . . . 219
- 7.5.4 Laplacians . . . . . . . . . . . . . . . . . . . . . . . . . . . . . . . . 221
- 7.5.5 Local spectral clustering . . . . . . . . . . . . . . . . . . . . . . . . 221
7.6 Approximation Stability . . . . . . . . . . . . . . . . . . . . . . . . . . . . 224
- 7.6.1 The Conceptual Idea . . . . . . . . . . . . . . . . . . . . . . . . . . 224
- 7.6.2 Making this Formal . . . . . . . . . . . . . . . . . . . . . . . . . . . 224
- 7.6.3 Algorithm and Analysis . . . . . . . . . . . . . . . . . . . . . . . . 225
7.7 High-Density Clusters . . . . . . . . . . . . . . . . . . . . . . . . . . . . . 227
- 7.7.1 Single Linkage . . . . . . . . . . . . . . . . . . . . . . . . . . . . . . 227
- 7.7.2 Robust Linkage . . . . . . . . . . . . . . . . . . . . . . . . . . . . . 228
7.8 Kernel Methods . . . . . . . . . . . . . . . . . . . . . . . . . . . . . . . . . 228
7.9 Recursive Clustering based on Sparse Cuts . . . . . . . . . . . . . . . . . . 229
7.10 Dense Submatrices and Communities . . . . . . . . . . . . . . . . . . . . . 230
7.11 Community Finding and Graph Partitioning . . . . . . . . . . . . . . . . . 233
7.12 Spectral clustering applied to social networks . . . . . . . . . . . . . . . . . 236
7.13 Bibliographic Notes . . . . . . . . . . . . . . . . . . . . . . . . . . . . . . . 239
7.14 Exercises . . . . . . . . . . . . . . . . . . . . . . . . . . . . . . . . . . . . . 240
8 Random Graphs 245
8.1 The G(n, p) Model . . . . . . . . . . . . . . . . . . . . . . . . . . . . . . . 245
- 8.1.1 Degree Distribution . . . . . . . . . . . . . . . . . . . . . . . . . . . 246
- 8.1.2 Existence of Triangles in G(n, d/n) . . . . . . . . . . . . . . . . . . 250
8.2 Phase Transitions . . . . . . . . . . . . . . . . . . . . . . . . . . . . . . . . 252
8.3 Giant Component . . . . . . . . . . . . . . . . . . . . . . . . . . . . . . . . 261
- 8.3.1 Existence of a giant component . . . . . . . . . . . . . . . . . . . . 261
- 8.3.2 No other large components . . . . . . . . . . . . . . . . . . . . . . . 263
- 8.3.3 The case of p < 1/n . . . . . . . . . . . . . . . . . . . . . . . . . . . 264
8.4 Cycles and Full Connectivity . . . . . . . . . . . . . . . . . . . . . . . . . . 265
- 8.4.1 Emergence of Cycles . . . . . . . . . . . . . . . . . . . . . . . . . . 265
- 8.4.2 Full Connectivity . . . . . . . . . . . . . . . . . . . . . . . . . . . . 266
- 8.4.3 Threshold for O(ln n) Diameter . . . . . . . . . . . . . . . . . . . . 268
8.5 Phase Transitions for Increasing Properties . . . . . . . . . . . . . . . . . . 270
8.6 Branching Processes . . . . . . . . . . . . . . . . . . . . . . . . . . . . . . 272
8.7 CNF-SAT . . . . . . . . . . . . . . . . . . . . . . . . . . . . . . . . . . . . 277
- 8.7.1 SAT-solvers in practice . . . . . . . . . . . . . . . . . . . . . . . . . 278
- 8.7.2 Phase Transitions for CNF-SAT . . . . . . . . . . . . . . . . . . . . 279
8.8 Nonuniform Models of Random Graphs . . . . . . . . . . . . . . . . . . . . 284
- 8.8.1 Giant Component in Graphs with Given Degree Distribution . . . . 285
8.9 Growth Models . . . . . . . . . . . . . . . . . . . . . . . . . . . . . . . . . 286
- 8.9.1 Growth Model Without Preferential Attachment . . . . . . . . . . . 287
- 8.9.2 Growth Model With Preferential Attachment . . . . . . . . . . . . 293
8.10 Small World Graphs . . . . . . . . . . . . . . . . . . . . . . . . . . . . . . 294
8.11 Bibliographic Notes . . . . . . . . . . . . . . . . . . . . . . . . . . . . . . . 299
8.12 Exercises . . . . . . . . . . . . . . . . . . . . . . . . . . . . . . . . . . . . . 301
9 Topic Models, Nonnegative Matrix Factorization, Hidden Markov Models, and Graphical Models 310
9.1 Topic Models . . . . . . . . . . . . . . . . . . . . . . . . . . . . . . . . . . 310
9.2 An Idealized Model . . . . . . . . . . . . . . . . . . . . . . . . . . . . . . . 313
9.3 Nonnegative Matrix Factorization – NMF . . . . . . . . . . . . . . . . . . . 315
9.4 NMF with Anchor Terms . . . . . . . . . . . . . . . . . . . . . . . . . . . . 317
9.5 Hard and Soft Clustering . . . . . . . . . . . . . . . . . . . . . . . . . . . . 318
9.6 The Latent Dirichlet Allocation Model for Topic Modeling . . . . . . . . . 320
9.7 The Dominant Admixture Model . . . . . . . . . . . . . . . . . . . . . . . 322
9.8 Formal Assumptions . . . . . . . . . . . . . . . . . . . . . . . . . . . . . . 324
9.9 Finding the Term-Topic Matrix . . . . . . . . . . . . . . . . . . . . . . . . 327
9.10 Hidden Markov Models . . . . . . . . . . . . . . . . . . . . . . . . . . . . . 332
9.11 Graphical Models and Belief Propagation . . . . . . . . . . . . . . . . . . . 337
9.12 Bayesian or Belief Networks . . . . . . . . . . . . . . . . . . . . . . . . . . 338
9.13 Markov Random Fields . . . . . . . . . . . . . . . . . . . . . . . . . . . . . 339
9.14 Factor Graphs . . . . . . . . . . . . . . . . . . . . . . . . . . . . . . . . . . 340
9.15 Tree Algorithms . . . . . . . . . . . . . . . . . . . . . . . . . . . . . . . . . 341
9.16 Message Passing in General Graphs . . . . . . . . . . . . . . . . . . . . . . 342
9.17 Graphs with a Single Cycle . . . . . . . . . . . . . . . . . . . . . . . . . . 344
9.18 Belief Update in Networks with a Single Loop . . . . . . . . . . . . . . . . 346
9.19 Maximum Weight Matching . . . . . . . . . . . . . . . . . . . . . . . . . . 347
9.20 Warning Propagation . . . . . . . . . . . . . . . . . . . . . . . . . . . . . . 351
9.21 Correlation Between Variables . . . . . . . . . . . . . . . . . . . . . . . . . 351
9.22 Bibliographic Notes . . . . . . . . . . . . . . . . . . . . . . . . . . . . . . . 355
9.23 Exercises . . . . . . . . . . . . . . . . . . . . . . . . . . . . . . . . . . . . . 357
10 Other Topics 360
10.1 Ranking and Social Choice . . . . . . . . . . . . . . . . . . . . . . . . . . . 360
- 10.1.1 Randomization . . . . . . . . . . . . . . . . . . . . . . . . . . . . . 362
- 10.1.2 Examples . . . . . . . . . . . . . . . . . . . . . . . . . . . . . . . . 363
10.2 Compressed Sensing and Sparse Vectors . . . . . . . . . . . . . . . . . . . 364
- 10.2.1 Unique Reconstruction of a Sparse Vector . . . . . . . . . . . . . . 365
- 10.2.2 Efficiently Finding the Unique Sparse Solution . . . . . . . . . . . . 366
10.3 Applications . . . . . . . . . . . . . . . . . . . . . . . . . . . . . . . . . . . 368
- 10.3.1 Biological . . . . . . . . . . . . . . . . . . . . . . . . . . . . . . . . 368
- 10.3.2 Low Rank Matrices . . . . . . . . . . . . . . . . . . . . . . . . . . . 369
10.4 An Uncertainty Principle . . . . . . . . . . . . . . . . . . . . . . . . . . . . 370
- 10.4.1 Sparse Vector in Some Coordinate Basis . . . . . . . . . . . . . . . 370
- 10.4.2 A Representation Cannot be Sparse in Both Time and Frequency Domains .. . . . 371
10.5 Gradient . . . . . . . . . . . . . . . . . . . . . . . . . . . . . . . . . . . . . 373
10.6 Linear Programming . . . . . . . . . . . . . . . . . . . . . . . . . . . . . . 375
- 10.6.1 The Ellipsoid Algorithm . . . . . . . . . . . . . . . . . . . . . . . . 375
10.7 Integer Optimization . . . . . . . . . . . . . . . . . . . . . . . . . . . . . . 377
10.8 Semi-Definite Programming . . . . . . . . . . . . . . . . . . . . . . . . . . 378
10.9 Bibliographic Notes . . . . . . . . . . . . . . . . . . . . . . . . . . . . . . . 380
10.10 Exercises . . . . . . . . . . . . . . . . . . . . . . . . . . . . . . . . . . . . . 381
11 Wavelets 385
11.1 Dilation . . . . . . . . . . . . . . . . . . . . . . . . . . . . . . . . . . . . . 385
11.2 The Haar Wavelet . . . . . . . . . . . . . . . . . . . . . . . . . . . . . . . . 386
11.3 Wavelet Systems . . . . . . . . . . . . . . . . . . . . . . . . . . . . . . . . 390
11.4 Solving the Dilation Equation . . . . . . . . . . . . . . . . . . . . . . . . . 390
11.5 Conditions on the Dilation Equation . . . . . . . . . . . . . . . . . . . . . 392
11.6 Derivation of the Wavelets from the Scaling Function . . . . . . . . . . . . 394
11.7 Sufficient Conditions for the Wavelets to be Orthogonal . . . . . . . . . . . 398
11.8 Expressing a Function in Terms of Wavelets . . . . . . . . . . . . . . . . . 401
11.9 Designing a Wavelet System . . . . . . . . . . . . . . . . . . . . . . . . . . 402
11.10Applications . . . . . . . . . . . . . . . . . . . . . . . . . . . . . . . . . . . 402
11.11 Bibliographic Notes . . . . . . . . . . . . . . . . . . . . . . . . . . . . . . 402
11.12 Exercises . . . . . . . . . . . . . . . . . . . . . . . . . . . . . . . . . . . . 403
12 Appendix 406
12.1 Definitions and Notation . . . . . . . . . . . . . . . . . . . . . . . . . . . . 406
12.2 Asymptotic Notation . . . . . . . . . . . . . . . . . . . . . . . . . . . . . . 406
12.3 Useful Relations . . . . . . . . . . . . . . . . . . . . . . . . . . . . . . . . . 408
12.4 Useful Inequalities . . . . . . . . . . . . . . . . . . . . . . . . . . . . . . . 413
12.5 Probability . . . . . . . . . . . . . . . . . . . . . . . . . . . . . . . . . . . 420
- 12.5.1 Sample Space, Events, and Independence . . . . . . . . . . . . . . . 420
- 12.5.2 Linearity of Expectation . . . . . . . . . . . . . . . . . . . . . . . . 421
- 12.5.3 Union Bound . . . . . . . . . . . . . . . . . . . . . . . . . . . . . . 422
- 12.5.4 Indicator Variables . . . . . . . . . . . . . . . . . . . . . . . . . . . 422
- 12.5.5 Variance . . . . . . . . . . . . . . . . . . . . . . . . . . . . . . . . . 422
- 12.5.6 Variance of the Sum of Independent Random Variables . . . . . . . 423
- 12.5.7 Median . . . . . . . . . . . . . . . . . . . . . . . . . . . . . . . . . 423
- 12.5.8 The Central Limit Theorem . . . . . . . . . . . . . . . . . . . . . . 423
- 12.5.9 Probability Distributions . . . . . . . . . . . . . . . . . . . . . . . . 424
- 12.5.10 Bayes Rule and Estimators . . . . . . . . . . . . . . . . . . . . . . . 428
12.6 Bounds on Tail Probability . . . . . . . . . . . . . . . . . . . . . . . . . . . 430
- 12.6.1 Chernoff Bounds . . . . . . . . . . . . . . . . . . . . . . . . . . . . 430
- 12.6.2 More General Tail Bounds . . . . . . . . . . . . . . . . . . . . . . . 433
12.7 Applications of the Tail Bound . . . . . . . . . . . . . . . . . . . . . . . . 436
12.8 Eigenvalues and Eigenvectors . . . . . . . . . . . . . . . . . . . . . . . . . 437
- 12.8.1 Symmetric Matrices . . . . . . . . . . . . . . . . . . . . . . . . . . 439
- 12.8.2 Relationship between SVD and Eigen Decomposition . . . . . . . . 441
- 12.8.3 Extremal Properties of Eigenvalues . . . . . . . . . . . . . . . . . . 441
- 12.8.4 Eigenvalues of the Sum of Two Symmetric Matrices . . . . . . . . . 443
- 12.8.5 Norms . . . . . . . . . . . . . . . . . . . . . . . . . . . . . . . . . . 445
- 12.8.6 Important Norms and Their Properties . . . . . . . . . . . . . . . . 446
- 12.8.7 Additional Linear Algebra . . . . . . . . . . . . . . . . . . . . . . . 448
- 12.8.8 Distance between subspaces . . . . . . . . . . . . . . . . . . . . . . 450
- 12.8.9 Positive semidefinite matrix . . . . . . . . . . . . . . . . . . . . . . 451
12.9 Generating Functions . . . . . . . . . . . . . . . . . . . . . . . . . . . . . 451
- 12.9.1 Generating Functions for Sequences Defined by Recurrence Relationships . . . . . . 452
- 12.9.2 The Exponential Generating Function and the Moment Generating Function . . . . . . . 454
12.10 Miscellaneous . . . . . . . . . . . . . . . . . . . . . . . . . . . . . . . . . . 456
- 12.10.1 Lagrange multipliers . . . . . . . . . . . . . . . . . . . . . . . . . . 456
- 12.10.2 Finite Fields . . . . . . . . . . . . . . . . . . . . . . . . . . . . . . . 457
- 12.10.3 Application of Mean Value Theorem . . . . . . . . . . . . . . . . . 457
- 12.10.4 Sperner’s Lemma . . . . . . . . . . . . . . . . . . . . . . . . . . . . 459
- 12.10.5 Prufer . . . . . . . . . . . . . . . . . . . . . . . . . . . . . . . . . . 459
12.11 Exercises . . . . . . . . . . . . . . . . . . . . . . . . . . . . . . . . . . . . . 460
Index
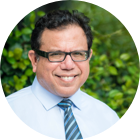
MMS • RSS
Article originally posted on Data Science Central. Visit Data Science Central
Not to be confused with this free Microsoft book same title.
Data science underlies Amazon’s product recommender, LinkedIn’s People You Know feature, Pandora’s personalized radio stations, Stripe’s fraud detectors, and the incredible insights arising from the world’s increasingly ubiquitous sensors. In the future, the world’s most interesting and impactful problems will be solved with data science. But right now, there’s a shortage of data scientists in every industry, traditional schools can’t teach students fast enough, and much of the knowledge data scientists need remains trapped in large tech companies.
This comprehensive, practical tutorial is the solution. Drawing on his experience building Zipfian Academy’s immersive 12-week data science training program, Jonathan Dinu brings together all you need to teach yourself data science, and successfully enter the profession.
First, Dinu helps you internalize the data science “mindset”: that virtually anything can be quantified, and once you have data, you can harvest amazing insights through statistical analysis and machine learning. He illuminates data science as it really is: a holistic, interdisciplinary process that encompasses the collection, processing, and communication of data: all that data scientists do, say, and believe.
With this foundation in place, he teaches core data science skills through hands-on Python and SQL-based exercises integrated with a full book-length case study. Step by step, you’ll learn how to leverage algorithmic thinking and the power of code, gain intuition about the power and limitations of current machine learning methods, and effectively apply them to real business problems. You’ll walk through:
-
Building basic and advanced models
-
Performing exploratory data analysis
-
Using data analysis to acquire and retain users or customers
-
Making predictions with regression
-
Using machine learning techniques
-
Working with unsupervised learning and NLP
-
Communicating with data
-
Performing social network analyses
-
Working with data at scale
-
Getting started with Hadoop, Spark and other advanced tools
-
Recognizing where common approaches break down, and how to overcome real world constraints
-
Taking your next steps in your study and career
Well-crafted appendices provide reference material on everything from the basics of Python and SQL to the essentials of probability, statistics, and linear algebra — even preparing for your data science job interview!
The book is available (pre-order) on Amazon, here.
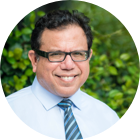
MMS • RSS
Article originally posted on Data Science Central. Visit Data Science Central
The following notes represent a complete, stand alone interpretation of Stanford’s machine learning course presented by Professor Andrew Ng and originally posted on the ml-class.org website during the fall 2011 semester. The topics covered are shown below, although for a more detailed summary see lecture 19. The only content not covered here is the Octave/MATLAB programming.
All diagrams are my own or are directly taken from the lectures, full credit to Professor Ng for a truly exceptional lecture course.
Content
- 01 and 02: Introduction, Regression Analysis and Gradient Descent
- 03: Linear Algebra – review
- 04: Linear Regression with Multiple Variables
- 05: Octave[incomplete]
- 06: Logistic Regression
- 07: Regularization
- 08: Neural Networks – Representation
- 09: Neural Networks – Learning
- 10: Advice for applying machine learning techniques
- 11: Machine Learning System Design
- 12: Support Vector Machines
- 13: Clustering
- 14: Dimensionality Reduction
- 15: Anomaly Detection
- 16: Recommender Systems
- 17: Large Scale Machine Learning
- 18: Application Example – Photo OCR
- 19: Course Summary
To access this material, follow this link.
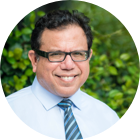
MMS • RSS
Article originally posted on Data Science Central. Visit Data Science Central
I stumbled upon this book by chance, when searching for material about time series (probably the most interesting chapter in this collection.) The various chapters are accessible from the top tabs, on this web page. It is mostly about R, but it has a few interesting chapters on statistical science too. Below is a summary.
Time series decomposition (in chapter 23)
This website was created with 6 major sections: Programming, Plotting, Regression, ANOVA, Advanced topics,and R-Apps.The tutorials build on each other, but can also be utilized independently from one another, and refer back to other chapters that cover related topics in greater depth.
- R-programming: includes 9 chapters which covers the basics of how install R, review of the important basic functions, and some advanced concepts such data manipulation and transformations to prepare your data for analysis.
- Plotting: included 2 chapters on how to make pretty plots for the most common uses in psychology.
- Regression: included 8 chapters spanning how to conduct different types of regressions (linear, multiple, moderation/mediation,moderated mediation, logistic, Poisson, and multilevel and Mixed). Chapters focus on how to be able to run models and check assumptions. Some have short theoretical reviews.
- ANOVA: included 2 chapters on how to run between-, within-, and mixed-subjects ANOVAs with simple set of follow-up tests.
- Advanced topics: included 4 chapters on selecting correlation types, AIRMA, decision trees and signal detection.
- R Apps: includes a chapter which shows how to make a Shiny application, a living online document which is reactive to user input and a chapter which shows how an ANOVA parses variance.
Some the chapters simulate datasets and others have links for you to download csv files. Each chapter might use different packages (i.e., library of functions), please install.packages("name of package")
indicated at the start of each chapter for doing the tutorial. For more information on installing packages see https://www.r-bloggers.com/installing-r-packages/.
- The Basics
- Indexing
- Logicals and Loops
- apply Functions
- plyr
- Sampling and Replication
- Melting & Casting
- Reshaping Data Using Tidyr
- GGplot for Scatterplots & Density Plots
- Boxplots and Bar Graphs
- Regression: Basics, Assumptions, & Diagnostics
- Plotting Regression Interactions
- Mediation and Moderation
- Moderated Mediation
- Multilevel Modeling
- Mixed Effects Modeling
- Testing the Assumptions of Multilevel Models
- Logistic and Poisson Regression
- Between-Subjects ANOVA in R
- ANOVA (afex): Within Subjects and Mixed Designs
- Correlation Types and When to Use Them
- Using ARIMA for Time Series Analysis
- Decision Trees
- Shiny Apps in RStudio
The authors of the tutorials were all graduate students in the department of psychology at the University of Illinois at Chicago.
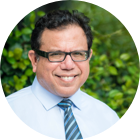
MMS • RSS
Article originally posted on Data Science Central. Visit Data Science Central
There are plenty of resources on the Internet to learn linear algebra or to get a refresher, including our own tutorial (here). Below are three interesting books found on Amazon.
Linear Algebra: Step by Step
The strength of the text is in the large number of examples and the step by step explanation of each topic as it is introduced. It is compiled in a way that allows distance learning, with explicit solutions to set problems freely available online. The miscellaneous exercises at the end of each chapter comprise questions from past exam papers from various universities, helping to reinforce the reader’s confidence. Also included, generally at the beginning of sections, are short historical biographies of the leading players in the field of linear algebra to provide context for the topics covered.
The dynamic and engaging style of the book includes frequent question and answer sections to test the reader’s understanding of the methods introduced, rather than requiring rote learning. When first encountered, the subject can appear abstract and students will sometimes struggle to see its relevance; to counter this, the book also contains interviews with key people who use linear algebra in practice, in both professional and academic life. It will appeal to undergraduate students in mathematics, the physical sciences and engineering.
This book is available here.
Introduction to Linear Algebra
This new fifth edition has become more than a textbook for the basic linear algebra course. That is its first purpose and always will be. The new chapters about applications of the SVD, probability and statistics, and Principal Component Analysis in finance and genetics, make it also a textbook for a second course, plus a resource at work. Linear algebra has become central in modern applied mathematics. This book supports the value of understanding linear algebra.
Introduction to Linear Algebra, Fifth Edition includes challenge problems to complement the review problems that have been highly praised in previous editions. The basic course is followed by eight applications: differential equations in engineering, graphs and networks, statistics, Fourier methods and the FFT, linear programming, computer graphics, cryptography, Principal Component Analysis, and singular values.
The book is available here.
Linear Algebra and Learning from Data
This is a textbook to help readers understand the steps that lead to deep learning. Linear algebra comes first especially singular values, least squares, and matrix factorizations. Often the goal is a low rank approximation A = CR (column-row) to a large matrix of data to see its most important part. This uses the full array of applied linear algebra, including randomization for very large matrices. Then deep learning creates a large-scale optimization problem for the weights solved by gradient descent or better stochastic gradient descent. Finally, the book develops the architectures of fully connected neural nets and of Convolutional Neural Nets (CNNs) to find patterns in data. Audience: This book is for anyone who wants to learn how data is reduced and interpreted by and understand matrix methods. Based on the second linear algebra course taught by Professor Strang, whose lectures on the training data are widely known, it starts from scratch (the four fundamental subspaces) and is fully accessible without the first text.
The book is available here.
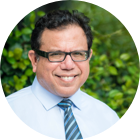
MMS • RSS
Article originally posted on Data Science Central. Visit Data Science Central
A free online version of the second edition of the book based on Stat 110, Introduction to Probability by Joe Blitzstein and Jessica Hwang, is now available here. Print copies are available via CRC Press, Amazon, and elsewhere. Stat110x is also available as an free edX course, here.
The edX course focuses on animations, interactive features, readings, and problem-solving, and is complementary to the Stat 110 lecture videos on YouTube, which are available here. The Stat110x animations are available within the course and here. For more information, visit Stat110 at Harvard. A 10-page cheat sheet summarizing the content, is available here. For more free books, visit this page.
Table of Contents
1. Probability and Counting
- Why study probability?
- Sample spaces and Pebble World
- Naive definition of probability
- How to count
- Story proofs
- Non-naive definition of probability
- Recap
- R
- Exercises
2. Conditional Probability
- The importance of thinking conditionally
- Definition and intuition
- Bayes’ rule and the law of total probability
- Conditional probabilities are probabilities
- Independence of events
- Coherency of Bayes’ rule
- Conditioning as a problem-solving tool
- Pitfalls and paradoxes
- Recap
- R
- Exercises
3. Random Variables and Their Distributions
- Random variables
- Distributions and probability mass functions
- Bernoulli and Binomial
- Hypergeometric
- Discrete Uniform
- Cumulative distribution functions
- Functions of random variables
- Independence of rvs
- Connections between Binomial and Hypergeometric
- Recap
- R
- Exercises
4. Expectation
- Definition of expectation
- Linearity of expectation
- Geometric and Negative Binomial
- Indicator rvs and the fundamental bridge
- Law of the unconscious statistician (LOTUS)
- Variance
- Poisson
- Connections between Poisson and Binomial
- *Using probability and expectation to prove existence
- Recap
- R
- Exercises
5. Continuous Random Variables
- Probability density functions
- Uniform
- Universality of the Uniform
- Normal
- Exponential
- Poisson processes
- Symmetry of iid continuous rvs
- Recap
- R
- Exercises
6. Moments
- Summaries of a distribution
- Interpreting moments
- Sample moments
- Moment generating functions
- Generating moments with MGFs
- Sums of independent rvs via MGFs
- *Probability generating functions
- Recap
- R
- Exercises
7. Joint Distributions
- Joint, marginal, and conditional
- D LOTUS
- Covariance and correlation
- Multinomial
- Multivariate Normal
- Recap
- R
- Exercises
8. Transformations
- Change of variables
- Convolutions
- Beta
- Gamma
- Beta-Gamma connections
- Order statistics
- Recap
- R
- Exercises
9. Conditional Expectation
- Conditional expectation given an event
- Conditional expectation given an rv
- Properties of conditional expectation
- *Geometric interpretation of conditional expectation
- Conditional variance
- Adam and Eve examples
- Recap
- R
- Exercises
10. Inequalities and Limit Theorems
Inequalities
Law of large numbers
Central limit theorem
Chi-Square and Student-t
Recap
R
Exercises
11. Markov Chains
- Markov property and transition matrix
- Classification of states
- Stationary distribution
- Reversibility
- Recap
- R
- Exercises
12. Markov Chain Monte Carlo
- Metropolis-Hastings
- Recap
- R
- Exercises
13. Poisson Processes
- Poisson processes in one dimension
- Conditioning, superposition, thinning
- Poisson processes in multiple dimensions
- Recap
- R
- Exercises
A Math
- Sets
- Functions
- Matrices
- Difference equations
- Differential equations
- Partial derivatives
- Multiple integrals
- Sums
- Pattern recognition
- Common sense and checking answers
B R Programming
- Vectors
- Matrices
- Math
- Sampling and simulation
- Plotting
- Programming
- Summary statistics
- Distributions
C Table of distributions
Bibliography
Index
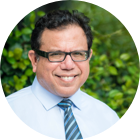
MMS • RSS
Article originally posted on Data Science Central. Visit Data Science Central
This glossary defines general machine learning terms as well as terms specific to TensorFlow. Below is a small selection of the most popular entries. You can access this glossary here. For other related glossaries, follow this link.
- A/B testing
- activation function
- agglomerative clustering
- artificial general intelligence
- AUC (Area under the ROC Curve)
- backpropagation
- bag of words
- Bayesian neural network
- Bellman equation
- binning
- boosting
- centroid-based clustering
- class-imbalanced dataset
- clustering
- collaborative filtering
- confusion matrix
- convenience sampling
- convex optimization
- convolutional filter
- convolutional neural network
- cross-entropy
- cross-validation
- data augmentation
- Dataset API (tf.data)
- decision boundary
- decision tree
- deep neural network
- Deep Q-Network (DQN)
- dimension reduction
- discriminative model
- ensemble
- false positive (FP)
- feature engineering
- feature extraction
- federated learning
- feedforward neural network (FFN)
- generalization curve
- generalized linear model
- generative adversarial network (GAN)
- gradient descent
- hashing
- hidden layer
- hierarchical clustering
- hinge loss
- hyperparameter
- input layer
- Keras
- Kernel Support Vector Machines (KSVMs)
- k-means
- k-median
- L1 loss
- L1 regularization
- learning rate
- least squares regression
- linear model
- linear regression
- logistic regression
- loss curve
- Markov decision process (MDP)
- Markov property
- matrix factorization
- Mean Squared Error (MSE)
- minimax loss
- model training
- multinomial classification
- natural language understanding
- neural network
- N-gram
- noise
- normalization
- NumPy
- objective function
- outliers
- overfitting
- pandas
- perceptron
- prediction bias
- pre-trained model
- prior belief
- proxy labels
- Q-function
- quantile
- quantile bucketing
- random forest
- recommendation system
- recurrent neural network
- regression model
- regularization
- reinforcement learning (RL)
- ridge regularization
- sampling bias
- scaling
- scikit-learn
- scoring
- selection bias
- semi-supervised learning
- sentiment analysis
- sigmoid function
- similarity measure
- size invariance
- sparse feature
- stationarity
- stochastic gradient descent (SGD)
- structural risk minimization (SRM)
- subsampling
- supervised machine learning
- synthetic feature
- TensorFlow
- time series analysis
- training set
- transfer learning
- true positive (TP)
- underfitting
- unsupervised machine learning
- validation
- vanishing gradient problem
- Wasserstein loss
- Weighted Alternating Least Squares (WALS)