Month: May 2020
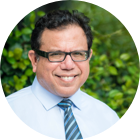
MMS • Adelina Turcu
Article originally posted on InfoQ. Visit InfoQ
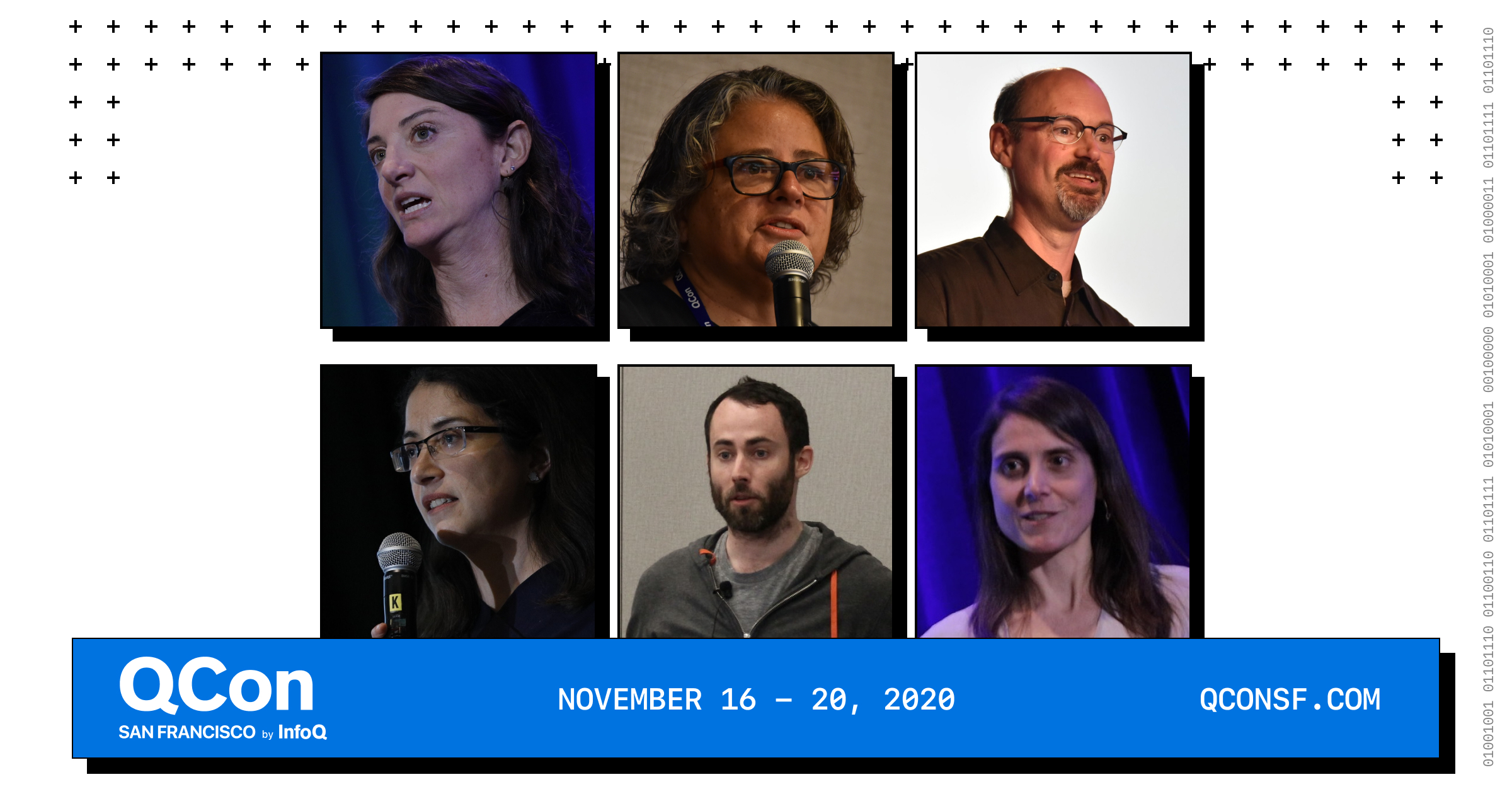
The QCon team has finalized the Program Committee for QCon San Francisco 2020 (Nov 16-18), and the lineup of software leaders that are shaping the conference agenda is confirmed as:
The program committee works on all aspects of software development. At QCon topics, track hosts and speakers are handpicked to guarantee relevant and timely content.
Wes Reisz, QCon San Francisco Lead Chair, Co-host of the InfoQ Podcast |
QCon San Francisco 2020 is scheduled to take place at the Hyatt Regency, located on the Embarcadero waterfront. The QCon team is working on implementing additional measures to ensure the safety of all participants.
The goal with the 14th edition of the conference is to cover a wide variety of technical and non-technical topics to give as much support as possible during these challenging times. Attend QCon San Francisco to explore how industry leaders deal with adversity and learn techniques for effectively debating and making decisions in relation to technical and non-technical decisions. Join your peers to get inspired and take the next best steps in your career.
Registration is $1870 ($995 off) for the 3-day conference if you register before May 30th
QCon is brought to you by InfoQ and is the software conference dedicated to providing a platform for innovator and early adopter companies to tell their stories and share their successes and their failures. It is the place where senior software engineers, architects, and team leads meet to share knowledge, network, and make an impact on their teams and businesses.
Article: Adoption of Cloud Native Architecture, Part 2: Stabilization Gaps and Anti-patterns
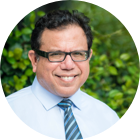
MMS • Srini Penchikala Marcio Esteves
Article originally posted on InfoQ. Visit InfoQ
In this second part of cloud native adoption article series, authors discuss the anti-patterns to watch out for when using microservices architecture in your applications. They also discuss how to balance between architecture and technology stability by ensuring to not reinvent the wheel in every new application and at the same time, avoiding arbitrary reuse of technologies.
By Srini Penchikala, Marcio Esteves
AWS Releases its Machine Learning Powered Enterprise Search Service Kendra into General Availability
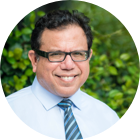
MMS • Steef-Jan Wiggers
Article originally posted on InfoQ. Visit InfoQ
Recently Amazon announced the general availability of its enterprise search service Kendra on AWS. With the GA release of Amazon Kendra, the public cloud provider added a few new specialized features and improved service accuracy.
A preview version of Amazon Kendra was launched during re:Invent in December last year, providing customers with an enterprise search service that heavily uses machine learning. During the preview phase, Amazon gathered feedback from customers using the service and added a couple of new features and optimization based on that feedback with the GA release. The service now has:
- New connectors for Salesforce, ServiceNow and Microsoft’s OneDrive cloud storage service.
- Improved vocabulary with additional domain-specific terms across eight more fields: Automotive, Health, HR, Legal, Media and Entertainment, News, Telecom, Travel and Leisure.
- Faster indexing, and improved accuracy.
- New scaling options for the Enterprise Edition, as well as a newly introduced Developer Edition.
With Amazon Kendra, customers can index structured and unstructured data stored in different backends, such as file systems, applications, Intranet, and relational databases. The service is optimized to understand complex languages from domains such as healthcare, IT, and many others. Moreover, the multi-domain expertise of the service provides better search results. Furthermore, developers can explicitly tune the relevance of results, using criteria such as authoritative data sources or document freshness.
Julien Simon, artificial intelligence and machine learning evangelist for EMEA at AWS, wrote in a blog post on the GA release:
Kendra search can be quickly deployed to any application (search page, chat apps, chatbots, etc.) via the code samples available in the AWS console, or via APIs. Customers can be up and running with state the art semantic search from Kendra in minutes.
Customers can set up Amazon Kendra easily through the AWS Console, create a new index, wait approximately 30 minutes or so, add and configure the data source, subsequently synchronize, and then test by running queries. Note that customers can also leverage Kendra via the AWS SDKs, and the AWS CLI.
For enterprises, it makes sense to use a search service as they have massive amounts of data – mostly underused as a Forrester survey indicates that between 60% and 73% of all data within corporations is never analyzed for insights. And, cloud providers can change that by bringing optimizing search with machine learning. Currently, Amazon is not the only one that offers a managed search service on its platform. Microsoft provides customers with Azure Cognitive Search as part of its Azure platform, and Google offers Cloud Search, available on its cloud platform. Furthermore, there is an alternative, according to a respondent on a Reddit thread:
Haystack is an OSS alternative to implement a scalable semantic search pipeline for enterprises using the latest NLP transformer models like BERT and RoBERTa.
One of the AWS customers using Kendra is 3M, and their technical director of the Corporate Research Systems Lab David Frazee said in an AWS press release of the service:
Finding the right information is often exhausting, time-consuming, and sometimes incomplete. With Amazon Kendra, our scientists find the information they need quickly and accurately using natural language queries. With Kendra, our engineers and researchers are enthusiastic about the ability to quickly find information which will enable them to innovate faster, collaborate more effectively, and accelerate the ongoing stream of unique products for our customers.
Amazon Kendra is currently available in the Northern Virginia, Oregon, and Ireland AWS regions. Customers can choose between enterprise or developer edition, which has similar features; however, the developer edition is limited in the number of queries per day, has no scaling options, and is available only in a single availability zone. And finally, the pricing details of the service are available on the pricing page.
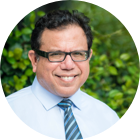
MMS • Arthur Casals
Article originally posted on InfoQ. Visit InfoQ
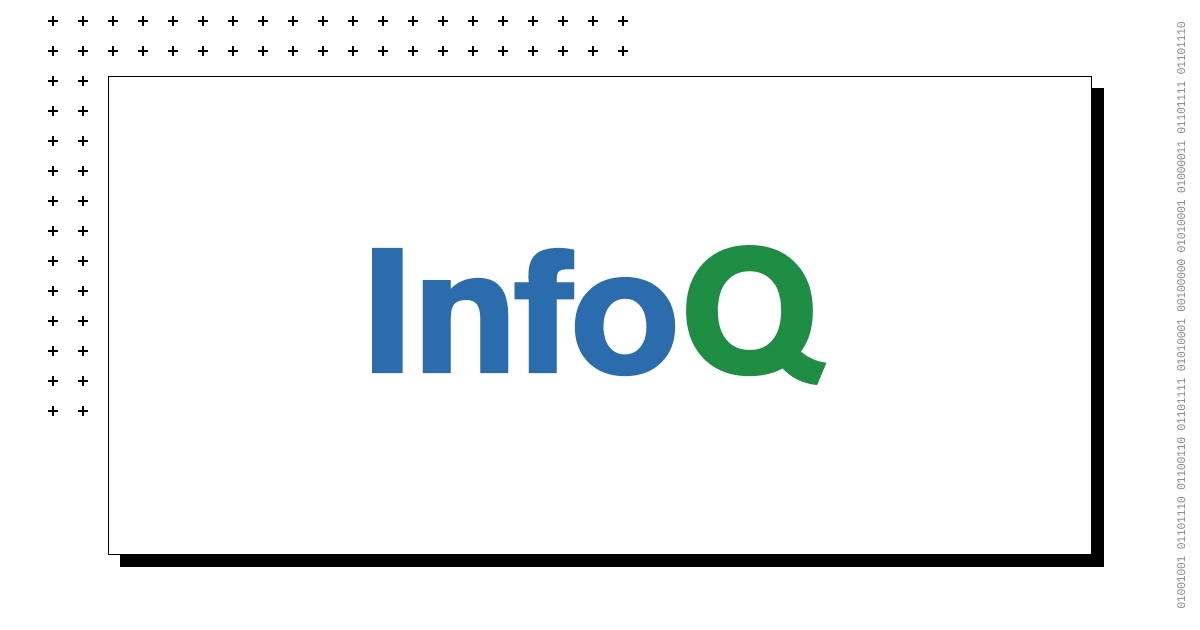
Last week Microsoft held the 10th edition of Build, its annual conference aimed at developers using Microsoft technologies. The online event included multiple important announcements and releases, such as the general availability of Blazor WebAssembly, updates on the upcoming .NET 5, Azure Static Web Apps, and new projects related to IoT and Artificial Intelligence.
This year’s Build was significantly different from its past editions. Due to the current pandemic situation, Microsoft decided to transform it into an online event that was entirely free for all attendees. Instead of a three-day gathering, the online conference comprised multiple digital sessions streamed in parallel for 48 hours. Another difference from its recent editions was the nature of its sessions: rather than being balanced with product announcements, it was much more focused on developer-oriented content.
The conference started with a keynote presented by Microsoft CEO Satya Nadella, who addressed the COVID-19 situation and applauded the response of tech companies to the crisis. It was followed by eight parallel sessions with announcements related to Microsoft Azure, .NET, Windows, Office 365, and the recently acquired GitHub. The program also featured multiple development tutorials, expert Q&A sessions with different development teams from Microsoft, focus groups on different aspects of Microsoft products, and “Community Connections” – sessions aimed at connecting developers geographically close to each other.
Two of the most relevant announcements related to Microsoft .NET development were: the official release of ASP.NET Blazor WebAssembly and the introduction of .NET MAUI. Blazor is a cross-platform, open-source component-based web UI framework for building single-page apps using .NET and C# instead of JavaScript. Blazor WebAssembly allows Blazor components to be hosted client-side in the browser using a WebAssembly-based .NET runtime. Combined with the already existing Blazor Server, the release represents Microsoft’s production-ready framework for full-stack .NET web development. However, it is important to notice that this is not an LTS release – an upgrade is necessary once .NET 5 is released (later this year).
.NET MAUI (short for Multi-platform App UI) is an evolution of the Xamarin.Forms toolkit. Its purpose is to provide a single mobile development stack supporting Android, iOS, macOS, and Windows, promoting the “Single Project Developer Experience”: a single project targeting multiple platforms. The general availability of .NET MAUI is targeted to November 2021 (with .NET 6), but the preview releases start later this year.
There were also different releases related to Visual Studio: ML.NET Model Builder is now part of Microsoft’s IDE, along with a new Windows Forms designer for .NET Core (both available as preview features in Visual Studio 2019 version 16.6). Another relevant related release was the support for connecting the IDE with Visual Studio Codespaces (formerly Visual Studio Online), Microsoft’s cloud-hosted development environment based on Visual Studio Code. The new feature is currently available in private preview.
Other releases related to Microsoft .NET include previews of Entity Framework Core 5 and .NET 5, insights on the upcoming C# 9.0, and Project Tye – an experimental tool aimed at Kubernetes-based microservices development. Interestingly enough, there were no releases related to F# 5 (although F# 5 Preview 4 was released in the same period).
In the Microsoft Azure sphere, there were several announcements on different fronts. Azure CosmosDB gained multiple new features and capabilities, and Azure Cosmos DB serverless is going to be available in preview in the coming months. There was also a particularly interesting session on enhancing Azure Cognitive Search with Azure Machine Learning.
Other exciting Azure-related releases were the preview of Azure Quantum (for quantum computing development) and a service called Azure Static Web Apps, which allows full-stack web apps to be automatically built and deployed to Azure from a GitHub repository.
In the IoT domain, Microsoft announced projects Bonsai and Moab: Bonsai is a machine learning-based component used to build, operate, and manage autonomous systems. Moab is an open-source, 3D-printable balancing robot that can be used with Bonsai to teach engineers how to build real-world autonomous control systems.
One of the most important announcements for the Windows platform was Project Reunion – the official name for the ongoing effort to unify Windows desktop and UWP apps. The idea is to allow developers to build “universal” apps that can run across multiple Windows devices. The announcement of Project Reunion included the preview of the Windows SDK .NET package, a .NET interop for all Windows WinRT APIs. Windows Terminal 1.0 and a preview of Windows Package Manager were also released, and multiple new features for the next generation of Windows Subsystem for Linux (WSL2) were announced – including GPU support and a real built-in Linux kernel.
Finally, Project Cortex – a Microsoft 365 service that uses Artificial Intelligence and Microsoft Graph to create a knowledge network from different data sources – will be generally available in “early summer.” A productivity application called Microsoft Lists is also going to be added to Microsoft 365, and Visual Studio Code now has an extension that allows the development of third-party tools for Microsoft Teams.
The conference program also included multiple tutorials and discussions related to GitHub products (from Azure and Visual Studio integrations to DevOps practices and focus groups), Rust, Java, and JavaScript. Most of the GitHub-related sessions followed the recent GitHub Satellite 2020 conference.
Overall, it is safe to say that the sessions revolved around a unified, platform-oriented development strategy (which is in line with Microsoft’s recent efforts in the .NET ecosystem). In this context, one of the sessions re-broadcasted during the entire event was a summary of a 90-minute pre-recorded video in which Scott Hanselman and Scott Hunter (both at Microsoft) talk about the current state and future of .NET 5.
All recorded sessions from Build (including other general announcements from Microsoft) can be found on Channel9 and Microsoft’s YouTube channel.
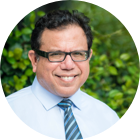
MMS • Dr. Michelle OSullivan Douglas Talbot
Article originally posted on InfoQ. Visit InfoQ

In this podcast Shane Hastie, Lead Editor for Culture & Methods spoke to Dr Michelle O’Sullivan and Douglas Talbot about how managers and team leads can support the mental wellness of their teams through turbulent times
Key Takeaways
- Good work is one of the best things for our mental health. It gives us a sense of purpose and it provides us with a community
- Mental wellness is about how to speak with people, having open conversations, giving people practical support when they need it, and also just creating a safe space and a good culture within your team. Most of it is just about good line management in general.
- Checking in in about how people are sleeping can be a safe conversation and can be good indicator of potential deeper issues
- So if somebody is quite stressed by the pandemic or what’s happening with their loved ones, or someone’s sick, these are very real fears, and we don’t want to pathologize what is a very normal reaction to an abnormal situation
- As a manager it is important for you to model the behaviour you want to see in your team, be vulnerable and open about your own fears and concerns, which gives others permission to be vulnerable too
Subscribe on:
Show Notes
- 00:00 Shane: Good day, folks. This is Shane Hastie for the InfoQ Engineering Culture podcast. We’re sitting in lockdown and I’m chatting with Doug Talbot and Michelle O’Sullivan. Doug is an InfoQ editor but has been working deeply in the people space and Michelle, you and I met for the first time today, so welcome.
- 00:25 And we’re isolating 12,000 miles apart, in that I’m in New Zealand and the two of you are in London.
- 00:33 Michelle, could we start with just a quick introduction? Who are you and why are you here?
- 00:38 Michelle: I’m a clinical psychologist by trade. Traditionally, clinical psychology is about providing support to people when they’re distressed, generally through therapy.
- 00:48 However, I’m particularly interested in how we can prevent distress and keep people healthy to begin with. So, although I do work therapeutically, my main work is really about trying to keep people safe and healthy in the workplace. I work solely for the rail industry full time thinking about how we can change the way organizations think about and manage health and wellbeing in the workplace.
- 01:13 Ultimately, I think. When we talk about mental health and work, people often start talking about stress and how things can go wrong. But the thing that I think people often forget is that good work is actually one of the best things for our mental health. It gives us a sense of purpose, something to get out of bed for in the morning. It provides us with a community. So I think if we can create good working conditions for people, essentially we’re protecting people’s mental health. So that’s one of my big passions.
- 01:41 Shane: Thank you. Doug, you and I know each other well, but why are we talking today with Michelle and about mental health? What brings you into that space?
- 01:52 Douglas: Obviously we got Michelle to speak recently at QCon London, where her talk on mental wellbeing had great reviews and we wanted to dig a little bit deeper with Michelle today. And particularly my focus has been on how should we lead and manage teams in the tech industry and particularly connecting to agile thinking, and as we grow these ideas of tribes and squads, and we’re getting much more heavily into the sociology and the psychology of how we run our operations and our organizations.
- 02:23 I felt that mental health was getting a bit of a whitewashing or a very, very thin layer of investigation and where we wanted to go much deeper. So the bit that I really wanted to discuss with Michelle was how can she provide advice and some more in depth tone and dialogue and specifics for how managers interact with their teams and especially agile teams where they’re kind of collaborative and in each other’s laps all day, every day and trying to interact like that.
- 02:52 I think that advice more than the classic individual, how do I look after my personal health and safety is what I really wanted to dig into with Michelle.
- 03:02 Michelle: I’ve been heavily involved in a large research project in the last two years that’s been investigating mental health training for line managers.
- 03:08 We’ve learned a lot of lessons from that research. So hopefully I can share a few top tips with the tech industry that will make your workplaces a little bit healthier and happier.
- 03:17 Douglas: Tell us a little bit about that specific study, Michelle, what were you trying to study and what was the result?
- 03:23 Michelle: Well, I think one of the big things that we see with mental health is an organizations are often really trying to do the right thing, but there isn’t much research for what’s the right thing actually is.
- 03:31 The first thing that we did is we looked at what research was already out there and what the core topics are that line managers should be taught. Often when I’m speaking to line managers, they say to me, look, Michelle, I’m not a therapist. Why should I be talking to my team about mental health? And you don’t need to be a therapist.
- 03:48 You don’t need to know loads about depression and anxiety. You don’t really need to know anything about diagnoses. It’s really about how to speak with people and having open conversations and giving people practical support when they need it, but also just creating a safe space and a good culture within your team. Most of it is just about good line management in general.
- 04:09 What we compared face to face training for line managers and e-learning to no training at all, so it was a randomized controlled trial. And what we found was that this half-day training or the e-learning as well both improve line managers’ confidence around talking around mental health, their preparedness to take action.
- 04:27 Well, one of the things I found quite interesting was that without continuously practicing those skills, they do fade. So this isn’t about doing some training and expecting it to be a panacea for how you manage your team. It’s really about thinking about how you talk to people and you have to keep talking to people again and again and again.
- 04:46 And it sounds a bit obvious, but I think sometimes we go on training and we remember it for a couple of days and then we try really hard to put things into practice, but then we kind of slip back into our old way. So it’s really trying to embed mental health into business as usual within your teams.
- 05:01 Shane: We’re certainly not in a business as usual state with most organizations today. So, what does mental wellness look like in a suddenly distributed environment? Doug was talking about his focus on the agile environment where people typically have been co-located and that was one of the things that we spoke about was the need for cross-functional co-located teams, and these teams have now become cross-functional distributed teams, suddenly without a lot of preparation.
- 05:35 As a manager what do I do to help my team.
- 05:38 Michelle: That is one of the biggest challenges right now, trying to adapt to these changing circumstances. I do think the tech industry is actually probably better placed than a lot of other industries because people, you know, have the tech to adapt to begin with.
- 05:50 So it’s really about trying to adapt some of our human behaviors. If you would check in with someone at the water cooler X amount of times a day, you need to think, how can you compensate for those physical check-ins? So, for example, within my own team, we make sure that every morning we have a 15 minute call on Teams, which is not really about work, it’s just, it’s just a social call to touch base and see how people are.
- 06:17 And that works for us. And I think having that call in the morning can give people a routine for the day. People know they’ve got something at half nine, so it’s not too early, but it’s also a reason to get out of bed. So, it helps provide that little bit of structure. And I think one if the really helpful things about having a call in the morning is, although none of us are doing anything too exciting in lockdown, what you often end up talking about is how a person has slept, and I always think of sleep as being a little bit like the canary in the coal mine because for starters, when you’re not feeding too well, it can be one of the first things to go. So people might have trouble falling asleep or staying asleep or waking up a lot quite early in the morning, or some people might end up sleeping absolutely loads and sleep is much more socially acceptable to talk about then mental health generally. So, it’s really good for how a person is doing.
- 07:07 Someone might not be comfortable saying, you know what, I’m feeling really, really low today, but they might be able to say, you know what, I didn’t get a wink of sleep last night.
- 07:16 And that kind of gives you permission to start asking questions around how they’re doing and how that’s going.
- 07:22 Douglas: One of the things I asked around a few tech managers that I know, and one of the things that they’re really interested in is how do we spot mental health or wellbeing issues, particularly in this remote state?
- 07:34 And that’s a brilliant example. Do you have any other signs that you might give us advice as laypeople, how we might spot something going wrong in our teams, particularly now that we don’t have that body language and that maybe that day to day or that hour by hour interaction.
- 07:50 Michelle: I think the key to picking up when something is wrong is noticing what’s normal for an individual and what they’re not, there’s a change in their usual behavior.
- 07:58 There’s no one perfect list of signs and symptoms that you should be looking out for, for any individual. Even when we look at diagnostic categories like depression and anxiety, although you do have questionnaires; as a clinical psychologist, if I’m assessing somebody, I’m going to be taking an incredibly detailed clinical history, potentially speaking with their loved ones as well as using psychometric tools.
- 08:21 So there’s no tick box exercise that you can go through in terms of determining whether somebody might be experiencing mental ill-health.
- 08:28 So the main thing to do is look at and consider what is normal for the people that you work with and whether or not there’s a change in that behavior for them.
- 08:38 if people in your team, if they’re normally, incredibly introverted, but all of a sudden they’re messaging loads and you’re having way more interaction with them, that’s a little bit unusual for them. So maybe just kind of touch base ask how they’re doing. Equally, if you have somebody who’s quite extroverted and very chatty and then they become withdrawn, that would kind of be an alarm bell to check in with them.
- 08:58 Generally, you might expect to see, changes in people’s moods. But again, right now in lockdown, it’s really hard to regulate our emotions in the current environment. So I would say we have to give ourselves some allowance for these extraordinary circumstances that we find ourselves in.
- 09:15 The other thing that you might often see is changes in people’s eating behaviors, so some people might lose their appetite or other people might be eating a lot more. Again, holding in mind that in lockdown, I’m sure a lot of us have been grazing more than we normally would.
- 09:31 Some people, when they’re incredibly stressed out, feel like they can’t cope, and so they might withdraw into themselves and they might not do as much work as they normally would. Other people go to the other extreme where they end up trying to do everything. So it’s really, really very personal.
- 09:46 And when people are talking about their worries, I suppose one of the key things that you should ask yourself is, is this a realistic worry?
- 09:54 So if somebody is quite stressed by the pandemic or what’s happening with their loved ones, or someone’s sick. That is a very real, very, we don’t want to pathologize what is a very normal reaction to an abnormal situation.
- 10:07 Douglas: You mentioned in the article that we’re going to publish, that it’s normal to have these emotional reactions, and like you’ve just said, it’s normal for things to change in the pandemic and for us to be naturally focused on real danger.
- 10:21 How would we spot when the trend has gone from healthy to unhealthy. Is there a barrier we should be considering or how would we know to take more action?
- 10:31 Michelle: I think that the two things that you should really keep an eye out for is number one, how long the distress is lasting for.
- 10:38 For things like anxiety and depression, normally you would have to be experiencing distress for over two weeks before a GP would be considered diagnosing you with anxiety or depression, but again, under these circumstances, I think a lot of people wouldn’t necessarily give anyone a diagnosis that easily under these really extreme circumstances. I think the only thing that’s useful to hold in mind as a comparison point is if you go through a bereavement, so if you go through bereavement and you lose a loved one, you’re not going to be diagnosed with depression immediately. It’s completely normal to feel incredibly sad and you have to let yourself feel that grief and work through it. It’s generally after about six months to a year where we would expect people to start feeding like they’re recovering, you might start thinking, well, maybe they might need a little bit more support.
- 11:27 I think if you notice that somebody is excessively distressed for a prolonged period of time, and again, during a pandemic, no one has written a book and said, what’s the norms of time to be stressed during a pandemic. So there is no magic two weeks or three weeks, but it’s how long is the stress going on for would be one thing.
- 11:45 And the other thing would be how much it’s impacting the day to day functioning of a person. For example, if they’re able to get up and go to work and engage in some socializing online and manage to keep some structure and routine around their life, that’s a really, really good sign.
- 12:04 Often I would say things around taking care of yourself. Shaving right now I think is a little bit optional. As is wearing makeup. I personally would never go into the office not wearing makeup, whereas I’ve been very rarely wearing makeup since lock down, and I’m sure lots of people can relate to that with shaving and whatnot.
- 12:22 So I guess it’s thinking about what is normal for the circumstances that we’re in. But if you notice somebody isn’t logging on, or if somebody is missing out on maybe online social events. Not able to take care of themselves quite so well. I think that is when you might want to think about what should you be stepping in and having a conversation with somebody, but ideally you should be having conversations about how people are doing all the time, really routinely.
- 12:48 It’s not when things go wrong that we should be asking people how they are; it’s when things are well, that can help prevent the problem from becoming a problem to begin with.
- 12:56 Shane: And that’s a pretty good segue, actually. What does well look like and feel like in a distributed team today?
- 13:04 Michelle: That’s a good question.
- 13:06 I’m going to ping that back at you guys. So when you’re well, what does well look like for you?
- 13:12 Shane: I feel productive. I feel engaged with the work. Now my work is remote. I’ve been working completely remote for the last three years, so for me, the transition that’s happened through COVID was not suddenly remote. I have a separate office space. I’ve got a quiet space, I can sit and get stuff done and so forth. So for me, it’s that engagement with the work and the regular contact with my colleagues. We, despite being a distributed team, I can certainly recognize some of the things you’re talking about in myself at the moment and in some of my colleagues.
- 13:52 Michelle: Shane, what you said about having regular contact with people, so when you have that regular contact and things are going well, what does that contact look like?
- 14:01 Shane: Because we’re a distributed team and we consciously know that, we actually put aside time for those sort of water cooler conversations you’re talking about.
- 14:08 We also have, as a distributed team, a daily 15 minute catch up. Now we’re globally distributed as well, so time zones become a bit of a challenge, but we consciously look out for that. We schedule in social time deliberately. We put aside every couple of weeks at least an hour where we’re going to just sit down across a zoom line and chat.
- 14:34 Michelle: And can I ask you, in terms of the outputs of your work and how you’re working together, what does that look like?
- 14:41 Shane: We’re, and this is something that I think we have noticed a bit through the COVID crisis, there have been ebbs and flows, which are normal, but they are exaggerated, in terms of how productive we feel.
- 14:55 Being an agile organization, we do a lot of pairing together, so we try and find those opportunities to work collaboratively.
- 15:04 Pairing and mobbing, and most of the time really look forward to those sessions. And I think when I’m feeling stressed, I don’t, and that’s possibly, you’re teasing some things out here.
- 15:14 Michelle: This is exactly what psychologists do. I’m going to get you to stop asking me questions and I’m going to turn the tables and be asking you all the questions, but I think you put your finger on something really important in terms of our willingness to collaborate when we’re feeding well.
- 15:29 When we’re feeling well, we see the world through a bright, positive lens. We give people benefit of the doubt. We think that people will help us, and that good things will happen.
- 15:39 When we’re feeling stressed and under pressure we start to put a lens on that kid ends up having negative thoughts about ourselves, other people, the future. And it can all of a sudden start to feel quite global.
- 15:52 So when things are working well and you come up against an obstacle, your thoughts will often be, well, if I collaborate and if I share this problem then we can work through it together.
- 16:02 Whereas when you’re not feeling in such a good space, I mean, it’s easy to start even feeling, quite cynical. People won’t be able to help me. People are out to get me. They want me to fail. And so that willingness to collaborate, I think is a real sign of the health of the team and being able to engage in that collaborative problem solving and sharing problems, essentially. Doug, what about you?
- 16:24 Douglas: I think I wanted to take us on to elements of broaching concerns as a manager with your staff. We talked about the fact that in Shane’s team there are pairing and there’s a specific time for social interaction, and I’ve seen that in quite a lot of tech teams recently as that they’ve got that 15 minutes even on a Friday drinks kind of time, or they’ve got the 15 minutes in the morning like your team, Michelle.
- 16:49 But it’s very hard to sometimes broach a very private or personal conversation about wellbeing with an individual in that situation. And if you don’t have a deliberate one-on-one set up every day because you’re not going to be able to bump into them in a COVID situation, what might be a nice way to broach the topic to be able to sit down with that person (in inverted commas) and have that conversation and get into a very potentially difficult space. Do you have any advice how you might be doing that now? I know you’ve recently taken on management of a team, Michelle, any thoughts about how you’re hoping to spot and broach those topics if it comes up?
- 17:27 Michelle: Well, I think one of the first things that you have to do is model vulnerability as well as modeling coping. So it has to come from you first of all.
- 17:37 I think sometimes as managers, we feel like we need to be seen as stoic and infallible, but actually it’s important to, you know, just as we know that failure is important and how we cope with failure and showing how to cope with failure is really important, it’s the same for vulnerability.
- 17:53 So although. I don’t want my team to perceive me as a mess every day. I do sometimes when we’re having our 15 minute calls, I’ll let people know if I’m not feeling great, if I’ve had a bit of a rubbish time and just talking a little bit about it.
- 18:07 I think also when we expect people to talk about their mental health, it’s a really big risk sometimes to talk about your mental health. There has been a lot of shame and stigma around mental health, so we can’t just expect people to start opening up. We have to work really hard at creating a culture that is safe and one of the most powerful ways to do that is by showing that vulnerability from on top.
- 18:33 The people who should make themselves vulnerable first are the people on top. Because you have to model again, that sense of safety.
- 18:41 One of the things that has taken up quite a lot in the UK is storytelling, so people sharing their stories of mental ill health. There’s the this is me campaign, the green ribbon campaign where people will record videos of themselves talking about their journey and experiences with mental health.
- 18:58 I think that goes a really long way to breaking down some of those barriers.
- 19:03 Sometimes we think that mental ill health is something that happens to other people, that it could never happen to people that we know where people that we work with or heaven forbid us, but when we see people that we admire and people that we respect talking about their mental health, all of a sudden we realize that mental health is something that we all have and that we all go through peaks and troughs, and that we’re all going to our own little battles every day, just trying to get by.
- 19:28 I would say in terms of how to broach, if you’re concerned about someone, how to get that conversation going, I would say, first of all, just make sure that you’re putting in the foundation of regularly checking in with people.
- 19:40 So asking, how are you? Shouldn’t come as a surprise.
- 19:43 Douglas: Is it okay to be able to just use WhatsApp or Slack or something, or do you think that that should be a face to face thing? Now that we’re relying on all these communication channels, I think there is a tendency, particularly in the tech industry and our teams, I’ve seen the introvert come out and people start doing everything via thousands of instant messages.
- 20:03 And sometimes the video conferencing starts to vanish away except for those very prescribed times. Any thoughts on communicating via those?
- 20:13 Michelle: I think different people will prefer different ways of communicating, and this is the other key trick of being a manager is that there’s no one size fits all for managing different people in your team.
- 20:24 You have to know individuals and know how they will respond. Some people might find video conferencing quite intrusive and actually feel much more comfortable with the bit of a barrier of instant messaging there. I personally try to do a little bit of a mixture of everything. You know, I’ll have some messages during the day.
- 20:40 I wouldn’t invalidate instant messaging. I think it can be a really great tool to just touch base and show someone that you’re holding them in mind. Not every conversation has to be deep and meaningful. It just has to show I’m thinking about you. And I think knowing that somebody is thinking about you can be really, really powerful in itself, but it’s knowing that that’s not a replacement for conversations and trying to get calls and video calls in can definitely be really, really helpful.
- 21:06 But the other thing that I would say is if you are concerned about somebody, trying to put explicit time in the diary that you both agreed on to have those conversations is really important. I think we can notice something might be wrong and if that person is in the back of our mind, but if we don’t act, if we don’t do anything about just that’s when it can become a problem. So making sure that you schedule in a time just to have that conversation. You’re carving it out for both of you and they know that that’s time is about their wellbeing, again, can be incredibly powerful.
- 21:39 And then in terms of how do you get past the, I’m fine because I think we’re all guilty of saying I’m fine when we’re absolutely not fine.
- 21:47 I always find it quite useful to try to hook in to the things that you’ve noticed. So the fact that you’re organizing this conversation means that you’ve noticed something, and I think it can be really, really helpful to spot the things that you’ve noticed.
- 22:01 So it might be something as simple as saying, you haven’t seen like yourself.
- 22:05 That’s also quite a nice, safe, neutral one. If you’ve noticed you’re eating 10 doughnuts a day, it can feel a little bit safer. You haven’t been coming along to the games evenings online that we’ve been having, and that’s not quite like you, I’m just a bit worried.
- 22:18 And again, it’s that act of being seen. Somebody seeing your distress can be really, really powerful and can help open the door for a powerful conversation.
- 22:27 Douglas: That gives you kind of the in. In terms of they’re saying, Hey, I’m not in a great place. Any advice for what the manager should do then if they do open up a little bit, what’s our next steps?
- 22:38 Michelle: I think that this is one of the big things that people are scared of: what do I do now? And I would say probably 90% of the time when people are upset, they really don’t need anything at all other than a listening ear. So don’t be paralyzed by worrying about what to do.
- 22:55 Listening is doing something, providing validation and empathy. Again, it’s really soothing to the animal part of us. You know, when you think about we’re social animals and it’s our tribes that help us survive. So when somebody sees your distress, when somebody sees your pain, it’s sending a very powerful message to your brain that your tribe has got you , we’re around you, you’re safe, and that could be really powerful in itself.
- 23:23 I would also say that if you do think somebody needs support, first of all, ask them what would they like you to do? And they might say nothing at all and then it’s grand. You’ve just had the conversation. But there might be some practical things, especially as a line manager that you can do and it could be around managing their workload or even a greater control over a project.
- 23:42 There’s lots of kind of quite practical things. I would encourage line managers, if you’re concerned around stress within your team at the moment, to consider doing a stress risk assessment.
- 23:52 Within the UK, we have some legal regulations around stress, and if you go onto the HSE website, they have a stress management standard and quite a lot of useful tools for assessing and managing stress.
- 24:06 Ideally you’d want to be doing a stress risk assessment with people, and this is looking at things like the demands that are paced and people how much control they have over their job, the support that they have, and it’s really systematically going through these domains at work that you can actively influence.
- 24:24 And it’s going through it with the person and trying to identify the points at work that you can both directly influence. So again, if somebody is having a lot of conflict at home, you might not be able to do anything about that as a line manager, but if they’ve got a lot of conflict at home, but they’ve also got a really the stressful project, it might be the combination of those two things that might be making it especially difficult to cope.
- 24:46 So it’s about trying to think about what are the things that are within your domain of control as a line manager, rather than thinking that you need to have a magic wand where you can solve everyone’s problems.
- 24:56 Douglas: That’s an interesting lead in there to the fact that during this COVID crisis, a number of teams, particularly support teams with e-commerce or social large customer bases have had massively increased demand.
- 25:09 The retail sector and the eCommerce sector delivery sector, I worked at Ocado, which is a massive online supermarket, they got hundreds of times their normal Christmas demand. Teams have suddenly been put under huge amounts of almost 24 hour a day pressure. The NHS being a classic example in the UK and medical stuff around the world, and the people behind those providing all the IT support, their workload has gone through the roof, but there’s not been more resources.
- 25:35 Have you got thoughts on how managers should be watching for overload on their people, and obviously cognitive overload in tech is a big topic, and I think a regular state, even without a pandemic now.
- 25:47 Michelle: Pressure in itself is not a problem. Pressure only really becomes a problem, generally when it’s quite excessive or if it goes on for quite a long period of time.
- 25:57 So I think for starters, don’t underestimate the ability of people to adapt. I think lots of people thrive in a crisis, and although it’s not ideal, I think a lot of people will be rising up to these challenges and able to manage it quite well. And I think also, during these times where there’s so much instability, one of the most beneficial things that you can do is provide stability through the relationships and the team.
- 26:25 So we might not know what’s going to come through on a day to day basis, both at work and with the pandemic. However, if your team know what they can expect of you and what they can expect from each other. That can be incredibly containing. So I think it’s, again, trying to nurture the relational aspect of work.
- 26:46 In terms of, I suppose trying to manage the cognitive overload, everybody’s work is going to be completely different. So it’s hard to generalize. But one thing I’d like to point out is this myth around our ability to multitask. We’re not really designed to multitask. It’s a lie that they’ve been selling us.
- 27:04 Every time we think we’re multitasking, all we’re doing is rapidly switching our attention from one task to another, and that is a really inefficient way to work. So even though we’re incredibly busy, we want to try to still carve out time for deep thought. For starters it can be very protective of our mental health because it allows us to, not feel bombarded all the time, but it also is really important for productivity.
- 27:29 From my own personal experience, I’ve turned off the notifications on my Outlook because every time something pings off, it’s diverting my attention, and it’s making me feel like I should be doing something else.
- 27:39 Similarly, when I’m on a conference call I try to make sure that my settings are on do not disturb because if somebody messages me when I’m on a conference call, even if I don’t respond to them, my attention is completely taken away from the call and that makes me less productive.
- 27:54 Shane and Doug, have you guys found anything from your own personal work that has helped with managing that constant onslaught of notifications?
- 28:01 Shane: Personally, I try and use techniques like Pomodoro, the timer tomato, where you block aside 25 minutes at the time, literally you set the timer, you focus on one thing and at the end of that 25 minutes, then you look at the distractions and then you bring another one. I don’t succeed all the time, but it helps.
- 28:20 Michelle: And I think it’s also good to know when are your most productive hours? You know, some people work better early in the morning, some people work later in the evening, so trying to schedule those deep thought times for the times where naturally you’re more productive. I’m an early morning person, , if I have any big task to do, I try to do it first thing before the day starts going all crazy, and by three o’clock I’m like an absolute brain dead zombie, but some people then work in quite the opposite.
- 28:45 So it’s about knowing yourself.
- 28:47 Douglas: I found that things actually got worse, definitely with the remote working for me. When I was in an office space, I’d often be physically moving to a meeting and I found it much easier to focus on the conversation I was having with that person face to face. But now that every conversation I’m having with someone is, even if it’s video, face to face, is sitting on my laptop; every single notification mechanism, whether it’s JIRA or Trello or our CRCD pipeline, or you know, the tech community is full of these things that are trying to grab your attention. And, and I found it has definitely been a lot worse being on my laptop permanently now, and I’m having to try and figure out how to stop some of them coming at me, which has been a change for myself.
- 29:27 Michelle: Absolutely. And I think as a line manager, it’s important to give people permission to turn off some of these notifications sometimes and again, to model that, protecting time to do certain deep thought activities. I’ve had to explicitly tell my team that if you have some task that you want to do and you just want to be offline, I’m not assuming that if you’re offline, you’re not working, I’m assuming you’re doing some deep thinking.
- 29:51 But I think people often are feeling like they need to have an online presence all the time, otherwise, they’re not going to be seen to be working. So I think it’s really important to nip those thoughts in the bud because sometimes being seen to be working is some of the least productive work that we’re doing.
- 30:07 Douglas: Yeah. Great. Piece of advice. .
- 30:08 Shane: This is really powerful and interesting stuff. Michelle, thank you very much for taking the time to talk to us. If people do want to continue the conversation, where would they find you?
- 30:18 Michelle: You can connect with me on LinkedIn under DrMichelleOS, and I’m also on Twitter under drMichelleOS , so if anyone has any questions, feel free to ping me and get in touch and I’ll certainly do my best to support.
- 30:32 Shane: Doug, you have been facilitating a series of articles that Michelle is putting together for InfoQ, do you want to tell us a little bit about the series?
- 30:39 Douglas: we just wanted to break the problem down into three basic sets of information.
- 30:44 One is for managers and the concepts of how can they support their staff., we’ve focused on five key hints and tips and ideas. And we’ve provided a bunch of research links and links to things like the health and safety in the UK tools that Michelle mentioned.
- 31:01 The second article we’re focusing on what can you do for yourself and hints and tips and a series of key items on what should you be thinking about for yourself and your own mental health and wellbeing.
- 31:11 And the third one we’re looking at is what can you do for peers, whether that’s manager to manager or teammate to teammate, or even to your family and other people in your community around you. We wanted to look at what can you do for others in those situations that can take it a little bit away from the contractual relationship in a hierarchy.
- 31:39 Shane: Thank you both so much.
- 31:41 Michelle: Oh, thanks
Mentioned
More about our podcasts
You can keep up-to-date with the podcasts via our RSS Feed, and they are available via SoundCloud, Apple Podcasts, Spotify, Overcast and the Google Podcast. From this page you also have access to our recorded show notes. They all have clickable links that will take you directly to that part of the audio.
Previous podcasts
Presentation: Deep Learning at Scale: Distributed Training and Hyperparameter Search for Image Recognition Problems
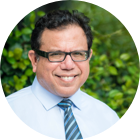
MMS • Alexandra Noonan
Article originally posted on InfoQ. Visit InfoQ
Transcript
Noonan: Choosing to move to a microservices architecture is something that almost every engineering team is seduced by at some point, no matter what size of company or product you have. There’s so much content out there warning you about the trade-offs, but your developer productivity is declining due to the fault isolation and modularity that come inherently with a monolith. However, if they are implemented incorrectly or used as a Band-Aid without addressing some of the root flaws in your system, you’ll find yourself no longer to make any new product development because you’ll be drowning in the complexity.
At Segment, we decided to break apart our monolith to microservices about a year after we launched. However, it failed to address some of the flaws in our systems. About three years of after continuing to add to our microservices, we were drowning in the complexity. In 2017, we took a step back and decided to move back to a monolith. The trade-offs that came with microservices caused more issues than they fixed. Moving back to a monolith is what allowed us to be able to fix some of the fundamental flaws in our system. My hope today is that you’ll understand and learn to deeply consider the trade-offs that come with microservices, and make the decision that is right for your team.
What we’re going to cover is I’ll go over what Segment does to help give you some context on the infrastructure decisions that we made. I’ll go over our basic monolithic architecture. Then why we moved to microservices, and why they worked for us in production for so long. Then I’ll cover what led us to hit the tipping point and inspired us to move back to a monolith, and how we did that. Then as with every big infrastructure decision, there are always trade-offs. I’ll cover the trade-offs that we dealt with moving back to a monolith and how we think about them moving forward.
What Does Segment Do?
What Does Segment do? I’m assuming most of you don’t know this, maybe some of you do. At Segment, we have a data pipeline. That data pipeline ingests hundreds of thousands of events per second. This is what a sample event looks like. It’s a JSON payload that contains basic information about users and their actions. These events are generated by the software that our customers built. A lot of people build really great software, and they want to understand how their customers are using that software. There are a ton of great tools out there that let you do that. For example, Google has one called Google Analytics. If you want to get up and started with Google Analytics, you have to go and add code in every single piece of your product to start sending data to Google Analytics’ API. Your marketing team maybe wants to use Mixpanel, your sales team wants to use Salesforce. Every time you want to add a new tool, you have to go in and write code in all the different sources of your product. Eventually, you could turn into something like this. It’s basically this big mesh of sources and tools that you’re sending your data to. Maybe one of them is leaking PII. The data is not consistent across tools. You want to try using a new tool, but you have to write code again in all of your sources to get started with that tool.
Segment’s goal is to make that easier. We provide a single API that you could send your data to. Then we’ll take care of sending your events to any end tool that you want. We have a single API. As a developer, you have to write significantly less code, because you just implement us once and then we handle sending that data for you. We provide tools along the way to help you do things such as strip any sensitive data or run any analysis on these tools. If you want to start using a new tool, it’s as simple as just going into our app, and enabling that tool. The piece of our infrastructure that I’m going to focus on today is these end tools and the forwarding of events to those tools. We refer to these tools as destinations.
In 2013, when we first launched our product, we launched with a monolith. We needed the low operational overhead that came with managing a monolith. It was just our fore-founders, and they needed whatever infrastructure was easiest for them to move and iterate on.
Original Architecture
This was the original architecture. We had a single API that ingested events and forwarded them to a distributed message queue. Then there was a single monolithic destination worker at the end that consumed from this queue. Each one of these destination APIs expects events to be in a specific format. The destination worker would consume an event from the queue, check customer managed settings to see which destination the event needed to go to. It would then transform the event to be compatible with that destination API. Send them a request over the internet to that destination. Wait for the response back. Then move on to the next destination.
Requests to destination actually fail pretty frequently. We categorize those errors into two different types. There’s a retryable error and a non-retryable error. A non-retryable error is something that we know will never be accepted by the destination API. This could be that you have invalid credentials or your event is missing a required field like a user ID, or an email. Then there are retryable errors. Retryable errors are something that we think could potentially be accepted later by the destination with no changes.
This is what retries looked like in our infrastructure. The destination worker would get a response back from the destination API. If it needed to be retried, it would put it back in the queue in line with everything else. We keep retrying events for a specific amount of time before giving up. It’s usually anywhere from about 2 to 10 attempts. What this caused, though, is something called head-of-line blocking. Head-of-line blocking is a performance issue where things are blocked by whatever is first in line. This is first-in, first-out design. If you zoom in on our queue, in particular, you’ll see we have the newest events in line with retry events across all our customers and all destinations.
What would happen is Salesforce, for example, is having a temporary outage. Every event sent to Salesforce fails and is put back in the queue to be sent again at a later time. We had auto-scaling at the time to add more workers to the pool to be able to handle this increase in queued up. This sudden flood would outpace our ability to scale up, which then resulted in delays across all our destinations for all of our customers. Customers rely on the timeliness of this data. We can’t afford these types of delays anywhere in our pipeline.
Now we’re at a point we had this monolith in production for about a year and the operational overhead is great. This environmental isolation between the destinations is really starting to bite us. About 10% of requests that we send out to destinations fail with a retryable error. This is something that we were constantly dealing with. It was a huge point of frustration. A customer that would not even be using Salesforce would be impacted by a Salesforce outage. This trade-off is what inspired us to move to microservices.
The Switch to Microservices
In 2014, we made the switch to microservices, because environmental isolation is something that comes naturally when you make the switch to microservices. This is what the new architecture looked like. Now we have the API that’s ingesting events, and forwarding them to a router process. This router process is responsible for fetching customer managed settings to see which destination the event needs to go to. Then it makes a copy of the event and distributes it to each destination-specific queue. Then at the end of each queue, there’s a destination specific worker that is responsible for handling messages.
Our microservice architecture allowed us to scale our platform like we needed to at the time for a few different reasons. The first was it was our attempt to solve that head-of-line blocking issue that we saw. If a destination was having issues, only its queue would back up and no other destinations would be impacted. It also allowed us to quickly add destinations to the platform. A common request that we would get is our sales team would come in, say, “We have this big, new customer, but they want to use Marketo. We don’t support Marketo. Can you guys add that in?” We’ll be like, “Sure.” We’d add another queue, and another destination worker to support Marketo. Now I can go off and build this Marketo destination worker without worrying about any of the other destinations.
In this situation, the majority of my time is actually spent understanding how Marketo’s API works and ironing out all the edge cases with their API. For example, each destination requires events to be in a specific format. For example, one destination would expect birthday as data of birth, our API accepts it as birthday. Here’s an example of a relatively basic transform that the destination worker would be responsible for. Some of them are pretty simple like this one, but some of them can be really complex. One of our destinations, for example, requires payloads to be in XML. Another thing that the worker is responsible for is not every destination sends responses back that are in a standard HTTP format. For example, this is one of our destinations, and it returns a 200. If you look, you’ll see it says success true. Then if you look at the results array, it was actually a not found error. This is the code that we have to write for this destination now, to parse every single response we’re getting back from that destination to understand if we need to retry the error or not.
Mono-repo vs. Micro-repo
When you move to microservices, something that comes up often is, do you keep everything in a mono-repo, or do you break it out into micro-repos and have one repo per service? When we first broke out everything into microservices, we actually kept everything in one repo. The destinations were broken out into their subdirectories. In these subdirectories lived all the custom code that we just went over for each destination, as well as any unit test that they had to verify this custom code.
Something that was causing a lot of frustration with the team was if I had to go in and make a change to Salesforce. Marketo’s tests were breaking. I’d have to spend time fixing the Marketo test to get out my change for Salesforce. In response to that, we broke out all the destinations into their own repos. This isolation allowed us to move quickly when maintaining destinations, but we’ll find out that this turned out to be a false advantage and came back to bite us.
The next and final benefit that we got from microservices, which is a little specific to Segment is we got really good visibility out of the box into our infrastructure. Specifically, the visibility that I’m talking about is program execution, like hot code paths and stack size. Most tools aggregated these types of metrics on the host, or the service level. You can see here, we had a memory leak. With all the destinations separated out, we knew exactly which destination was responsible for it.
Something we were constantly paged for was queue depth. Queue depth is generally a good indicator that something is wrong. With our one-to-one destination worker queue setup, we knew exactly which destination was having issues. The on-call engineer can then go check the logs for that service and understand relatively quickly what was happening. You definitely can get this type of visibility from a monolith. You don’t get it for free right away. It takes a bit of time and effort to implement.
2015, we only had about 10 engineers total. Our microservice setup is allowing us to scale our product like we needed to at the time. If we look at the trade-offs, this is what we see. We have really good environmental isolation. Now one destination having issues doesn’t impact anybody else. We have good, improved modularity. With all the destinations broken out into their own repos, failing tests don’t impact any of the other destinations. We had this good default visibility. The metrics and logging that come out of the box with microservices significantly cut down on our time having to spend debug whenever we got paged. The operation overhead story isn’t great, but it’s not a real problem for us yet, because we only have about 20 destinations at this time. However, with the way our microservices are set up, this specific trade-off will soon be our downfall.
Now we’re going to go into 2016. Our product is starting to gain some real traction. We’re entering that hyper-growth startup phase. Requests with the sales team were happening more. They would come in, “We had this other new customer that’s an even bigger deal. They want webhooks and we don’t support webhooks. Can you add that in?” Of course, why not? Spin up a new queue, new destination worker. Another thing we were seeing was we’re at the point where destinations were actually reaching out to us to be supported on our platform, which was really cool. In our microservice architecture, it was really easy to spin them up a new queue and a new worker. This keeps happening again over time.
In 2017, we’ve now added over 50 new destinations. I couldn’t quite fit 50 on here, but you get the idea. The thing is, though, with 50 new destinations, that meant 50 new repos. Each destination worker is responsible for transforming events to be compatible with the destination API. Our API will accept name in any of these formats. Either just name or first and last name, camelCase, first and last name, snake_cased. I want to get the name from this event. I have to check each of these cases in that destination code base. I’m already having to do a decent amount of context switching to understand the differences in destination APIs. Now all of our destination code bases also have specific code to get the name from Segment event. This made maintenance for us a bit of a headache. We wanted to decrease that customization across our repos as much as possible.
We wrote some shared libraries. Now for that name example, I could go into any of our destination code bases, call event.name. This is what would happen under the hood. The library would check for traits.name, that didn’t exist. It would go through and check for first name and last name, checking all the cases. These familiar methods made the code bases a lot more uniform, which made maintenance less of a headache. I could go into any code base and quickly understand what was going on.
What happens when there’s a bug in one of these shared libraries? Let’s say, for argument’s sake, that we forgot to check snake_case here. Any customer that is sending us an event.snake_case won’t have the name of their users in their end tools. I go into the shared library. Write a fix for it to start checking snake_case. I release a new version of it. All of our infrastructure at the time was hosted on AWS. We were using Terraform to manage the state of it. The state of our infrastructure lived in GitHub. This was the standard deploy process for just one of these services. If everything went perfectly smoothly, I could probably get this change out to one service in about an hour. We had over 50 new destinations now, which meant 50 new queues and 50 new services. If I wanted to get this fix out to just check snake_case on a name, I now had to test and deploy dozens of services. With over 50 destinations, that’s at least one week of work for me to get this change out, or the whole team is with me heads down in a room and we’re powering through these destinations.
Changes to our shared libraries began to require a ton of time and effort to maintain. Two things started happening because of that. One, we just stopped making changes to our shared libraries even when we desperately needed them. It started to cause a lot of friction in our code base. The second was, I would go in and just update the version in that specific destination code base. Because of that, eventually, the versions of our shared libraries started to diverge across these code bases. That amazing benefit we once had of reduced customization, completely reversed on us. Eventually, all of our destinations were using different versions of these shared libraries. We probably could have built tooling to help us with testing and automating the deploys of all of these services. Not only was our productivity suffering because of this customization, we were starting to run into some other issues with our microservices.
Destination Traffic
Something that we noticed was that some of our destinations were handling little to no traffic. For example, you have destination Y here, and they’re handling less than one event per second. One of our larger customers who is sending us thousands of events per second, sees destination Y, wants to try it out. They turn on destination Y. All of a sudden, destination Y’s queue is flooded with these new events, just because one customer enabled them. I’m getting paged to have to go in and scale up destination Y. Because similar to before, it outpaced our ability to scale up and handle this type of load. This happened very frequently.
At the time, we had one auto-scaling rule applied to all of these services. We would play around with it to try and master the configuration to help us with these load spikes. Each of these services also had a distinct amount of CPU and memory resources that they used. There wasn’t really any set of auto-scaling rules that worked. One solution to this could be to just overprovision and just have a bunch of minimum workers in the pool. That gets expensive. We’d also thought about having dedicated auto-scaling rules per destination worker, but we were already drowning in the complexity across the code bases. That wasn’t really an option for us.
As time goes on, we’re still adding to our microservice architecture, and we eventually hit a breaking point. We were rapidly adding new destinations to this platform. On average, it was about three per month. With our setup, our operational overhead was increasing linearly with each new destination added. Unless you’re adding more bodies to the problem, what tends to happen is your developer productivity will start to decrease as your operational overhead increases. Managing all of these services was a huge tax on our team. We were literally losing sleep over it, because it was so common for us to get paged to have to go in and scale up our smaller destinations. We’d actually gotten to a point where we’d become so numb to pages on issues that we weren’t responding to them as much anymore. People were reaching out to our CEO to be like, “What is going on over there”?
Not only did this operational overhead cause our productivity to decline, but it also halted any new product development. A really common feature request we got for our destinations was customers wanted to understand if their events were successfully sent to the destination or not. At the time, our product was a bit of a black box. We’d given customers visibility into whether their event had made it to our API, like I’m showing you here. Then if they wanted to know if their events had made it to their destination, they’d have to go into that destination and check for themselves. The solution for that in our microservice world would have been to create a shared library that all the destinations could use to easily publish metrics to a separate queue. We were already in that situation with our current shared libraries that were on different versions for each of these services. We knew that that was not going to be an option. If we ever want to make a change to that feature, we’d have to go through and test and deploy every single one of these services.
Microservices Trade-offs
If we take a look now at the trade-offs, the operational overhead is what is killing us. We’re struggling just to keep this system alive. This overhead of managing this infrastructure is no longer maintainable and was only getting worse as we added new destinations. Because of this, our productivity and velocity was quickly declining. The operational overhead was so great that we weren’t able to test and deploy these destinations like we needed to, to keep them in sync, which resulted in the complexity of our code bases exploding. Then new product development just didn’t happen on the platform anymore. Not only because we were drowning, just trying to keep the system alive, but because we knew that any additions that we made would make the complexity worse. When we started to really think about it, microservices didn’t actually solve that fundamental head-of-line blocking problem in our system, it really just decreased the blast radius of it.
If you look at our individual queue now, we still have new events in line with retry events. It’s 2017, we have some pretty large customers that are sending a ton of events. Then we also are now supporting some destinations, who can only handle about 10 requests a minute. Rate limiting was something we were constantly dealing with across all our destinations. Rate limiting is an error that we want to retry on. One customer’s rate limits would cause delays for all of our customers using that destination. We still have this head-of-line blocking issue. It’s now just isolated at the destination level.
The ideal setup to actually solve for that issue in our microservice world would have been one queue and one destination worker per customer per destination. Since we were already reaching our breaking point with microservices, that was not an option. I’m not talking about adding a couple hundred more microservices and queues. I’m talking tens of thousands of more microservices and queues.
It’s 2017 now, and we have officially reached the breaking point. At this point, we have over 140 services and queues and repos. The microservice setup that originally allowed us to scale is now completely crippling us. We brought on one of our most senior engineers to help us with the situation. This is the picture that he drew to depict what the situation was we’re in. In case you can’t tell, that’s a big hole in the ship, and there’s three engineers struggling to ship water out of it. It’s what it felt like.
Moving Back To a Monolith
The first thing that we knew we wanted to do was we wanted to move back to a monolith. The operational overhead of managing all these microservices was the root of all of our problems. If you look at the trade-offs, the burdens of this operational overhead was actually outweighing all of the benefits that microservices gave us. We’d already made the switch once before. This time, we actually knew that we had to really consider the trade-offs that were going to come with it, and think deeply about each one, and be comfortable with potentially losing some of the benefits that microservices gave us.
We knew we wanted to move everything back into a service, but the architecture at the time would have made that a bit difficult. If we’d put everything in one service but kept the queues, now this monolithic destination worker would have been responsible for checking each queue for work. That would have added a layer of complexity to the destinations with which we weren’t comfortable with. It also doesn’t solve that fundamental head-of-line blocking issue that we see. One customer’s rate limits can still impact everybody using that destination. Moving back to a single queue puts us back in the same situation we were in when we first launched, where now one customer’s retries impact all destinations and all customers.
This was the main inspiration for Centrifuge. Centrifuge would replace all of our queues and be responsible for sending events to this single monolithic worker. You could think of Centrifuge as providing a queue per customer per destination but without all the external complexity. It finally solved that head-of-line blocking issue that we’d experienced since we launched once and for all. Now the destinations team, there’s about 12 of us. We are dedicated to just building Centrifuge and this destination worker. After we’d designed the system, we were ready to start building. We knew that since we were going to go back to one worker, we wanted to move back to a mono-repo. This specific part of the project we called it the great mono-gration.
The destinations were divided up amongst each of us. We started porting them over back into a mono-repo. With this change, we saw an opportunity to fix two fundamental issues. One, we were going to put everything back on the same versions of our dependencies. At this point, we had 120 unique dependencies. We were committed to having one version of the shared dependency. As we moved destinations over, we’d update to the latest. Then we’d run the tests and fix anything that broke.
Building a Test Suite
The next part of the mono-gration, this is really the meat of it, was, we needed to build a test suite that we could quickly and easily run for all of our destinations. The original motivation for breaking destinations out into their own repos was these failing tests. This turned out to be a false advantage. Because these destination tests, were actually making outbound HTTP requests over the internet to destination APIs to verify that we’re handling the requests and responses properly. What would happen is I would go into Salesforce, which hadn’t been touched in six months, and need to make a quick update. Then the tests are failing because our test credentials are invalid.
I go into our shared tool and I try and find updated credentials, and of course they’re not there. Now I have to reach out to our partnerships’ team or Salesforce directly to get new test credentials, just so I can get out my small change to Salesforce. Something that should have taken me only a few hours is now taking me over a week of work because I don’t have valid test credentials. Stuff like that should never fail tests. With the destinations broken out into separate repos, there was very little motivation for us to go in and clean up these failing tests. This poor hygiene led to a constant source of frustrating technical debt. We also knew that some destination APIs are much slower than others. One destination test suite could take up to five minutes to run, waiting for the responses back from the destination. With over 140 destinations, that means our test suite could have taken up to an hour to run, which was not acceptable.
Traffic Recorder
We built something called traffic recorder. Traffic recorder was responsible for recording and saving all destination test traffic. On the first test run, what it would do is the requests and responses were recorded to a file like this. Then on the next run, the request and response in the file is played back instead of actually sending the request to the destination API. All these files are checked into a repo as well, so that they’re consistent with every change. That’s made our test suite significantly more resilient, which we knew was going to be a must-have moving back to a mono-repo. I remember running the tests for every destination for the first time. It only took milliseconds, when most tests before would take me a matter of minutes, and it just felt like magic.
We finished the great mono-gration in the summer of 2017. Then the team shifts to focus on building and scaling out Centrifuge. We’re slowly moving destinations over into the monolithic worker now as we’re learning to scale Centrifuge. We complete this migration at the beginning of 2018. Overall, it was a massive improvement. If we look back at some of the auto-scaling issues that we had before, some of our smaller destinations weren’t able to handle big increases in load. It was a constant source of pages for us. With every destination now living in one service, we had a good mix of CPU and memory intensive destinations, which made scaling a service to meet demand significantly easier. This large worker pool was able to absorb spikes in loads. We’re no longer getting paged for the destinations that only handle a small number of events. We could also add new destinations to this and it wouldn’t add anything to our operational overhead.
Next was our productivity. With every destination living in one service, our developer productivity substantially improved because we no longer had to deploy over 140 destinations to get a change out to one of our shared libraries. One engineer was able to deploy this service in a matter of minutes. All our destinations were using the same versions of the shared libraries as well, which significantly cut down on the complexity of the code bases. Our testing story was much better. We had traffic recorder now. One engineer could run the tests for every destination in under a minute, when making changes to the shared libraries. We also started building new products again. A few months after we finished Centrifuge and moved back to a monolith, myself and one other engineer were able to build out a delivery system for our destinations in only a matter of months.
Dealing With the Trade-offs
We’re building new products again, and we’re able to scale our platform without adding to our operational overhead. It wasn’t all sunshine and roses moving back to a monolith. There were some trade-offs. If you take a quick, high-level look at the trade-offs, it doesn’t look great. Our operational story had improved greatly. That was the root of all of our issues. We almost need a weight on here to signify how important each trade-off is. Traffic recorder had made us comfortable with the loss in improved modularity. Yes, if I had to go in and fix Salesforce and Marketo’s tests are failing, I would have to fix those tests to get the change out. It made us more resilient to those types of failures that we saw in the beginning where invalid credentials would fail tests. Centrifuge fixed the head-of-line blocking issue, which was one of the main causes of environmental isolation. We actually ran into another issue with that. Then there was the default visibility that we no longer had, this was the first thing we ran into.
A few months after we moved to a monolith, we started seeing these destination workers running out of memory and crashing. This is commonly referred to as OOMKills. It wasn’t happening in an alarming rate, but it was happening with enough frequency that we wanted to fix it. With our destination workers broken out into microservices before, we would have known exactly which destination was responsible, but now it was a bit more difficult.
OOM Debugging With Sysdig
To give you a sense of what we had to do now was we used this tool called Sysdig. Sysdig lets you capture and filter and decode system calls. We go on the host, and we run Sysdig monitoring these workers, and we think we find a connection link. We go in and we blindly make some updates to this service to fix this leak. Workers are still crashing. We didn’t fix it. It took a few more weeks of different attempts and trying to almost blindly debug this memory issue.
What ended up working was we used a package called node-heapdump. How that works is it synchronously writes a snapshot to a file on disk that you can later go and inspect with Google Chrome Dev tools. If you look really closely at the last two fields here, you’ll see that the issue is in our intercom destination. For some reason, there’s a 100 MB array. We know which destination it is. We’re able to go and debug from there. It took some time and effort to implement this visibility into the worker that we got for free with microservices. We probably could have implemented something similar in our original monolith but we didn’t have the resources on the team, like we did now.
Environmental isolation, this was the most frequent issue and unforeseen issue that we ran into moving back to a monolith. At any given time, we run about 2000 to 4000 of these workers in production. Something that we were constantly running into was, this worker is written in Node and we would constantly have uncaught exceptions. Uncaught exceptions are unfortunately common, and very easily can get into a code base undetected. For example, I remember, I was on-call one weekend and I happened to be in a different state with my whole family. It was my grandma’s 90th birthday. We’re all crammed into this hotel room. It’s 2:00 a.m. on a Friday, and I’m getting paged. I’m like, “What could this possibly be? It’s 2:00 a.m. on a Friday. Nobody is deploying code right now.” I go in and workers are crashing. I debug it down to this catch block here. I go and look at GitHub to see when this was committed, and it had been put in the code base two weeks ago. Only just now were events coming in triggering this uncaught exception, because the response here is not defined.
What happens with an uncaught exception in Node is that the process will exit, which causes one of these workers to exit. When one of them exits, this event is considered failed and Centrifuge will actually retry that event. When there are multiple events that are coming in and hitting this code path, we see this cascading failure because now new events are coming in causing these uncaught exceptions, causing these workers to exit. Then Centrifuge is retrying those events causing those workers to exit. We host these workers on AWS’s ECS platform. ECS will bring up a new worker whenever one exits, but not at a fast enough rate with how quickly they’re exiting here. We also have found that when workers have a really high turnover like this, ECS will actually stop scheduling new tasks to come up. Now we’re in the situation where our worker pool is quickly shrinking, but we’re sending it more traffic because of all the retries. Then that creates this back pressure situation where every customer and destination is impacted, which should sound a bit familiar.
What’s really interesting is that there was a new engineer on the team. One of the first suggestions that came from them was how about we break all these destinations into their own service? That way, if there’s an uncaught exception in one, now, it’s only reduced to that destination and those customers. There is some truth to that. It would isolate the destinations very nicely so that an uncaught exception would only impact that destination. We’d already been down that road. We’d already been burned by the operational overhead that came with microservices, all the destinations living in their own service. It doesn’t actually solve the root issue here. It really just decreases the blast radius.
We thought about it a bit more. What we ended up doing was we created a wrapper. How this wrapper works is it’s a container runtime that acts as a middleware between the application and the infrastructure. A very simplified version of how it works in the uncaught exception situation is when an uncaught exception happens, it will catch it. Discard it. Then restart the worker. This is really just a workaround, because ECS can’t restart tasks quick enough with how quickly they exit. This also doesn’t solve the uncaught exception problem. Now at least our worker pool isn’t shrinking. We’re able to set up alerts and metrics when we have these uncaught exceptions, and then go in and fix them. If you look at the trade-offs again, we’ve actually made some improvements on our environmental isolation and visibility. We now have the resources and time to build this type of wrapper or to add heapdumps on these hosts.
The Migration Journey
As we’ve been running into new issues, it’s been really interesting to see that almost every time, that our gut reaction is we should break this apart into microservices. It never really solves the root of our issues. I think the biggest lessons that we learned as we moved from monolith to microservices, and then back to monolith is that it’s really all about trade-offs, and really understanding the issues that you’re dealing with, and making the decision that’s right for your team at the time. If we had started with microservices right away in 2013, there’s a chance we never would have made it off the ground because of the operational overhead that comes with them. Then we quickly ran into environmental isolation where destinations were impacting one another.
With microservices you get good environmental isolation. We moved to microservices. We had more resources on the team so the operational overhead we were willing to trade off a little bit. It isolated the destinations like we needed from one another at the time. We were having sales deals coming in saying, “You don’t support this destination. We need that. Otherwise we’re not signing with you.” I think when we moved to microservices this time we didn’t have a full understanding of what the root issue was. We didn’t understand that it was actually our queuing system that was causing a lot of our problems. Even if we did understand this and really took the time to think critically about it, we had only 10 engineers total at the time. I don’t know if we would have been able to build Centrifuge that actually solved that problem for us. Centrifuge took us a full year and two of our most senior engineers to get it into production. Microservices provided that isolation that we needed at the time to scale.
Then, after a few years, in 2017, we hit a tipping point and the operational overhead is too great for us. We lacked the proper tooling for testing and deploying these services, so our developer productivity was quickly declining. We were just trying to keep the system alive. The trade-offs were no longer worth it. We now had the resources to really think critically about this problem and fix the system. We moved back to a monolith. This time we made sure to think through every one of the trade-offs and have a good story around each. We built traffic recorder proactively since we knew we were going to lose some of that modularity. Centrifuge helped us solve some of those environmental isolation issues. As we iterate on this infrastructure and are continuing to encounter new issues, we’re doing a much better job of really thinking through these trade-offs and doing our best to attempt to solve the root issues that we’re dealing with.
Takeaways
If you’re going to take anything away from this talk, it’s that, there is no silver bullet. You really need to look at the trade-offs and you have to be comfortable with some of the sacrifices that you’re going to make. When we move to microservices, and whenever it’s suggested again, it’s never really solved the root of our problems. When we first moved, it only decreased the blast radius. Then those problems came back to bite us later, but we’re much bigger. Nothing can replace, really critically thinking about your problem and making sure you weigh the trade-offs and make the decision that is right for your team at the time. Other parts of our infrastructure, we actually still use microservices, and they work great. Our destinations are a perfect example of how this trend can end up hurting your productivity. The solution that worked for us was moving back to a monolith.
See more presentations with transcripts
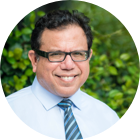
MMS • Anthony Alford
Article originally posted on InfoQ. Visit InfoQ
Google open-sourced Table Parser (TAPAS), a deep-learning system that can answer natural-language questions from tabular data. TAPAS was trained on 6.2 million tables extracted from Wikipedia and matches or exceeds state-of-the-art performance on several benchmarks.
By Anthony Alford
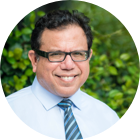
MMS • Jamie Dobson
Article originally posted on InfoQ. Visit InfoQ
Many business leaders are not skilled or experienced strategists, but those skills are more crucial than ever. Strategic patterns can speed the creation of new strategies, and give novice strategists the benefit of knowledge they haven’t had time to build on their own. Jamie Dobson of Container Solutions shows how strategic patterns can give you the planning tools you need now.
By Jamie Dobson
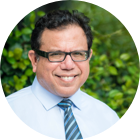
MMS • Uday Tatiraju
Article originally posted on InfoQ. Visit InfoQ
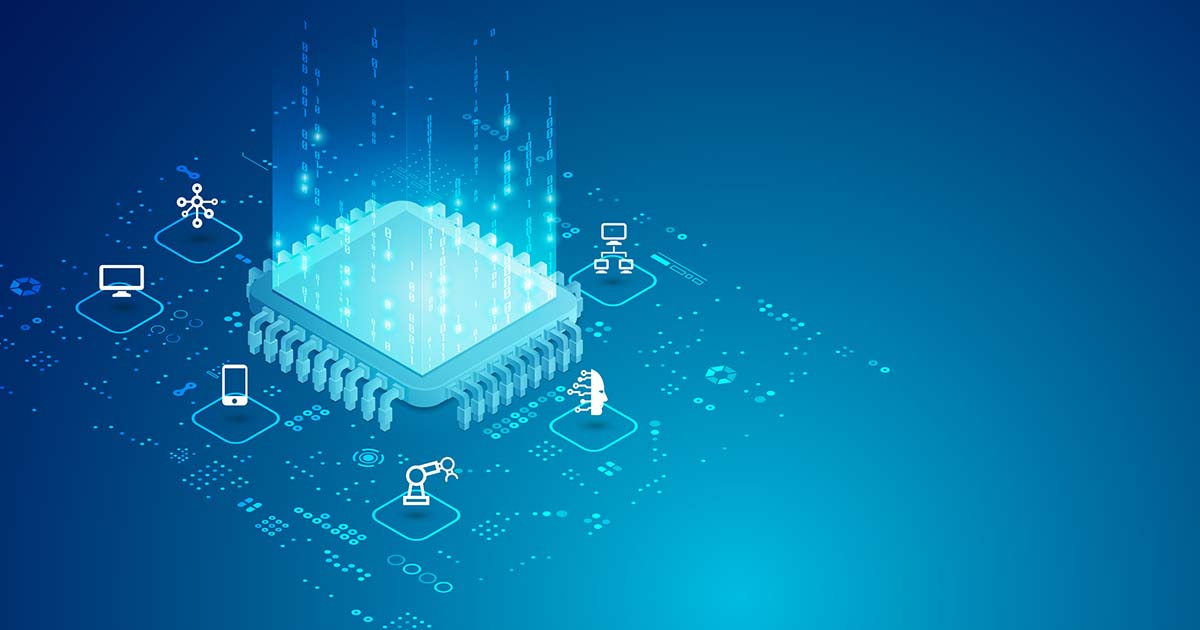
Concurnas is a new open source JVM programming language designed for building concurrent and distributed systems. Concurnas is a statically typed language with object oriented, functional, and reactive programming constructs.
With a concise syntax that hides multithreaded complexity, and native support for GPU computing, vectorization, and data structures like matrices, Concurnas allows for building machine learning applications and high performance parallel applications. In addition, Concurnas provides interoperability with other JVM languages like Java and Scala. Concurnas supports Oracle JDK and OpenJDK versions 1.8 through to the latest GA release 14.
InfoQ spoke to Jason Tatton, creator of Concurnas and the founder of Concurnas Ltd., about the language, some of its design decisions, and features.
InfoQ: What motivated you to create a new programming language?
Jason: During the first phase of my career I worked in investment banking running teams and building trading models and systems for high frequency trading. I saw that the engineering problems which we were solving on a day to day basis were mostly centered around building reliable scalable high performance distributed concurrent systems. I found that the current thread and lock with shared mutable state model of concurrency exposed within most popular programming languages (such as Java and C++) was just too difficult for even exceptionally talented world class engineers to get right. I thought to myself, “there has to be a better way of solving these sorts of concurrent problems”. So I quit my job in 2017 and set out to solve this problem and Concurnas as a programming language for making concurrent programming easier was born.
InfoQ: What motivated you to choose JVM as opposed to say LLVM?
Jason: There is much innovation to be made in the areas of both runtimes/virtual machines (such as LLVM or the JVM) and host languages. Building either a new language or virtual machine is a massive undertaking and realistically, especially when working with a small team and a tight deadline, one must choose to focus on one area or another.
With Concurnas I chose to focus upon the language and so this left the decision of which runtime to use. Performance wise LLVM and the JVM are similar. In the end the JVM was chosen for two reasons: 1). the JVM is the most popular and widely distributed virtual machine on the planet – most enterprises use Java and so have an established use case for the JVM, 2). There is a large body of existing enterprise scale code written in JVM languages such as Java, Scala and Kotlin which are crying out for leverage within a language that can provide a model of concurrency which is easier to understand and use. By implementing Concurnas on the JVM, users are afforded the capability to utilize all of their existing JVM language code. We also gain access to the Java standard library and so do not have to create one from scratch in support of the language.
InfoQ: How did you come up with the name “Concurnas”?
Jason: As Concurnas is designed primarily as a language to make concurrent programming easier for everyone we started to call it Concur, for “Concur-rent”. Later on the “-nas” was tagged on to make “Concurnas” as this sounded nicer.
InfoQ: The general trend has been to use C, Rust, and Go predominantly for systems programming while languages like Java, Python, and C# are being predominantly used for applications programming. Where does Concurnas fall on the spectrum?
Jason: Concurnas is designed for solving concurrent, parallel and distributed computing problems. To a large extent the sorts of problems Concurnas is good at solving exist within both the systems and application domains of programming. One thing we are researching now is adopting a more Rust like model of memory management in order to give users who need to do more low level memory management the opportunity to do this whilst having the existing functionality of automatic memory management with garbage collection in Concurnas to fall back on if they so wish.
InfoQ: Would you recommend Concurnas for writing machine learning algorithms?
Jason: Although the JVM and the Java language offers tremendous performance, it has not been widely adopted for ML applications, this is unfortunate. I believe this may be because of the verbosity of the Java language. Concurnas solves a lot of these problems so on that basis I would say that it’s an excellent candidate thanks to its model of concurrency and first class citizen support for GPU computing – things which are tremendously beneficial for ML applications.
Furthermore we’re looking at adding first class citizen support for automatic differentiation to the language. In this way Concurnas will become the second language, in addition to Apple Swift, to support this feature at the language level. This will be of tremendous benefit for implementing ML algorithms and those users working in finance for derivatives calculations.
InfoQ: Which feature of Concurnas that you built gave you the most satisfaction?
Jason: The GPU’s which are present in modern graphics cards can be leveraged for general purpose massively parallel computation. It is common for algorithms which leverage GPU’s to improve computational speed by up to 100x vs a conventional single core CPU based algorithm. Furthermore, per FLOP this computation is provided with a much reduced power consumption and hardware cost vs a CPU based implementation. Traditionally, users have had to learn C/C++ in order to leverage the GPU for general purpose computation, which for many presents a large barrier for entry. Concurnas has first class citizen support for GPU computing built within it. Users can write idiomatic, normal looking Concurnas code and have that code run directly upon the GPU without having to first learn C/C++.
Providing this feature was very satisfying for two reasons. 1). GPU computing can make a real difference in so far as reducing the environmental costs of computation is concerned. Providing language level support for this opens up the floodgates for developers to start leveraging GPU hardware and reaping the benefits. 2). On a technical level the GPU computing component of Concurnas is predominantly written in Concurnas language code itself! This presented some interesting technical challenges concerning bootstrapping the compilation of the Concurnas language compiler but it was important to do this in keeping with the “eat your own dog food” principle.
InfoQ: How does Concurnas provide concurrent computing? Does it use synchronization primitives and threads under the hood?
Jason: The core concurrency primitive exposed within Concurnas is the isolate. Isolates are light weight threads which can perform computation concurrently. All code in Concurnas is executed within isolates. Isolates cannot directly share memory between one another, dependent data is copied into isolates at the point of creation – which prevents accidental sharing of state that could otherwise lead to unscalable, non deterministic program behavior. Controlled communication of state between isolates is achieved via the use of special objects known as refs, support for which is provided by the type system of Concurnas itself.
Whereas with raw threads we are bounded by the restrictions of our JVM in terms of the number we may spawn, with isolates we are bounded only by the amount of memory our machine(s) have access to. In this way isolates scale much better than raw threads. In terms of execution, isolates are multiplexed and run in a cooperative manner as continuations. When an isolate hits a point where it is unable to continue computation due to waiting for data from another isolate (communicated via a ref) it will yield execution of its underlying raw thread such that that thread may execute a different isolate. Whether we are executing on a single core or a many core machine the fundamental model of concurrent execution remains the same.
A side effect of this model is that we are able to use refs in support of the reactive programming paradigm. We can create special isolates (via the `every` and `onchange` keywords or the relevant compact Concurnas syntax) which react and trigger on changes made to one or many input refs and optionally return refs themselves – thus creating for ourselves reactive graphs of calculation. This is a natural way of solving concurrent problems.
InfoQ: Is Concurnas production ready?
Jason: The build out of Concurnas was started in 2017 with the first production release as open source under the MIT license in December 2019. Concurnas is now production ready and at Concurnas Ltd. we are able to offer commercial support packages for all sizes of organization which require it. There is also a growing body of free community resources concerning the language available on the internet.
InfoQ: Where do you see Concurnas in the next five years?
Jason: Since the inception of Concurnas, three years ago, a lot has been achieved. It’s very exciting to imagine what we will be able to achieve as the community continues to grow in the next five, ten or thirty years! In the immediate future, as previously mentioned, we are looking at adding automatic differentiation to the language. In addition to this we are looking at improved support for off heap memory management for working with large data sets and an improved GPU computing interface. Finally we are looking at providing developer tool support in the form of IDE support for Jupyter notebook, VS Code, IntelliJ and Eclipse.
We are very much community focused, open to feedback and committed to satisfying the needs of our customers that are actively making use of the language. To this end we would love to hear from any readers on what they would like to see in the Concurnas programming language, please feel free to get in touch via one of the methods listed on the Concurnas website.