Month: November 2020
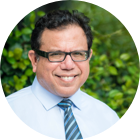
MMS • RSS
Article originally posted on Data Science Central. Visit Data Science Central
This blog was originally written in 2012, and am republishing it because with the increased use of black box AI / ML models to power key operational decisions, these human decision-making traps need to be thoroughly and holistically addressed during the analytics definition stage to avoid the dangers of unintended consequences.
Organizations are looking to integrate big data and advanced analytics into their business operations in order to become more analytics-driven in their decision-making. However, there are several challenges that need to be addressed in order to make that transformation successful. One of those challenges is the very nature of how humans make decisions, and how our genetic makeup works against us in analyzing data and making decisions.
Human Decision-Making Dilemma
The human brain is a poor decision-making tool. Human decision-making capabilities have evolved from millions of years of survival on the savanna. Humans became very good at pattern recognition: from “That looks just a harmless log behind that patch of grass,” to “Yum, that looks like an antelope!” to “YIKES, that’s actually a saber-toothed tiger!!” Necessity dictated that we become very good at recognizing patterns and making quick, instinctive survival decisions based upon those patterns.
Unfortunately, humans are lousy number crunchers (guess we didn’t need to crunch many numbers to know to spot that saber-toothed tiger). Consequently, humans have learned to rely upon heuristics, gut feel, rules of thumb, anecdotal information, and intuition as our decision guides. But these decision tricks are inherently flawed and fail us in a world of very large, widely varied, high velocity data sources.
Figure 1: Dilbert by Scott Adams
Awareness of these human decision-making flaws is important if we want to transform our organization, and our people, to become an analytics-driven business.
Human Decision-Making Traps
Let’s cover a few examples, or decision traps, where the human brain will lead us to suboptimal, incorrect, or even fatal decisions.
Trap #1: Over-confidence
We put a great deal of weight on whatever we happen to know, and assume that what we don’t know isn’t important. The casinos of Las Vegas were built on this human flaw (and why my son likes to say that “gambling is a tax on those who are bad at math”).
For example, hedge fund Long-Term Capital Management (LTCM), with two Nobel Prize winners on staff, returned ~40% per year from 1994 to 1998. Soon other traders copied their techniques. So LTCM looked for new markets where others might not be able to mimic them. LTCM made the fatal assumption that these new markets operated in the same way as the old markets. In 1998, the LTCM portfolio dropped from $100B to $0.6B in value and a consortium of investment banks had to take LTCM over to avoid a market crash[1].
Companies make similar mistakes by over-valuing their experience in an existing market when they move into a new market (e.g., AT&T with computers), or launching a new product into a different product category (e.g., Procter & Gamble with orange juice). Companies don’t do enough research and analysis to identify and model the business drivers, and the competitive and market risks of moving into a new market or product category.
Trap #2: Anchoring Bias
Anchoring is the subtle human tendency to glom onto one fact as a reference point for decisions, even though that reference point may have no logical relevance to the decision at hand. During normal decision-making, individuals anchor, or overly rely, on specific information and then adjust to that value to account for other elements of the circumstance. Usually once the anchor is set, there is a bias toward that information.
For example, humans struggle deciding when to sell a stock. If someone buys a stock at $20 and then sees it rise to $80, they have a hard time selling the stock when it starts to drop because we’ve been anchored by the $80 price. This was a fairly common occurrence during the dot.com bust, as people whose low-cost stock options rose to unimaginable highs, then rode their stock options (chased the tape) all the way to zero because they had set their anchor point to the high.
This anchoring bias tends to show up in organizations’ pricing, investment, and acquisition decisions.
Trap #3: Risk Aversion
Our tolerance for risk is highly inconsistent. Risk aversion is a manifestation of people’s general preference for certainty over uncertainty, and for minimizing the magnitude of the worst possible outcomes to which they are exposed. Risk aversion surfaces in the reluctance of a person to accept a bargain with an uncertain payoff rather than another bargain with a more certain, but possibly lower, expected payoff.
For example, a risk-averse investor might choose to put his or her money into a bank account with a low but guaranteed interest rate, rather than into a stock that may have high expected returns but also involves a chance of losing value.
Another example is the reluctance of a business to cannibalize an incumbent product, even an aging or falling incumbent product, at the expense of up-and-coming product.
Trap #4: Don’t Understand Sunk Costs
Many companies often throw good money after bad investments because they don’t comprehend the concept of “sunk costs.” In economics, sunk costs are retrospective (past) costs that have already been incurred and cannot be recovered. Sunk costs are sometimes contrasted with prospective costs, which are future costs that may be incurred or changed if an action is taken. However, sunk costs need to be ignored when making going-forward decisions.
As an example, people will sit through a bad movie until the end even though they are not enjoying the movie. Why? Most of us would watch the rest of the movie since we paid for it, but the truth is, the price of the movie is a sunk cost.
As business examples, Coca Cola (with New Coke) and IBM (with OS/2) continued to throw good money at bad investment decisions because they had invested significant time and money (and emotional capital) into those products and wanted to try to recoup their investments, even at the cost of missing more lucrative business opportunities. We see this today with on-going marketing campaign spend, brand rationalization decisions, and decisions to exit poorly performing markets.
Trap #5: Framing
How a decision is stated or framed can impact what decision is made. Information, when presented in different formats, alters people’s decisions. Individuals tend to select inconsistent choices, depending on whether the question is framed to concentrate on losses or gains.
As an example, participants were offered two alternative solutions for 600 people affected by a hypothetical deadly disease:
- Option A saves 200 people’s lives
- Option B has a 33% chance of saving all 600 people and a 66% possibility of saving no one
These decisions have the same expected value of 200 lives saved, but option B is risky. 72% of participants chose option A, whereas only 28% of participants chose option B.
However, another group of participants were offered the same scenario with the same statistics, but described differently:
- If option C is taken, then 400 people die
- If option D is taken, then there is a 33% chance that no people will die and a 66% probability that all 600 will die
In this group, 78% of participants chose option D (equivalent to option B), whereas only 22% of participants chose option C (equivalent to option A).
The discrepancy in choice between these parallel options is the framing effect; the two groups favored different options because the options were expressed employing different language. In the first problem, a positive frame emphasizes lives gained; in the second, a negative frame emphasizes lives lost[2].
Other human decision-making traps include Herding (Safety in Numbers), Mental Accounting, Reluctance to Admit Mistakes (Revisionist History), Confusing Luck with Skill, Bias to the Relative, Don’t Respect Randomness and Over-emphasize the Dramatic.
What Can One Do?
The key is to guide, not stifle, human intuition (think guard rails, not railroad tracks). Here are some things that you can do to guide your decision-making as you make the transformation to an analytics-driven organization:
- Use analytic models to help decision makers understand and quantify the decision risks and returns. Leverage proven statistical tools and techniques to improve the understanding of probabilities. Employ a structured analytic discipline that captures and weighs both the risks and opportunities.
- Confirm and then reconfirm that you are using the appropriate metrics (think Moneyball). Just because a particular metric has always been the appropriate metric, don’t assume that it is the right one for this particular decision.
- Challenge your model’s assumptions. Test the vulnerability of the model and the model’s assumptions using Sensitivity Analysis and Monte Carlo techniques. For example, challenging the assumption that housing prices would never decline would have averted the recent mortgage market meltdown.
- Consult a wide variety of opinions when you vet a model. Avoid Group Think[3] (which is yet another decision-making flaw). Have someone play the contrarian (think Tom Hanks in the movie “Big”). Use facilitation techniques in the decision process to ensure that all voices are heard and all views are contemplated.
- Be careful how you frame decisions.
- Create business models that properly treat sunk costs. Ensure that the model and analysis only consider incremental costs. And be sure that your models also include opportunity costs.
- Use “after the decision” Review Boards and formal debriefs to capture what worked and what didn’t, and why.
- Beware of counter-intuitive compensation; humans are revenue optimization machines
Figure 2: Dilbert by Scott Adams
Making the transformation to an analytics-driven culture is a powerful business enabler, but more than technology needs to be considered in driving that transformation. Understanding, managing, and educating on common decision-making traps will help ensure a successful transformation.
[1] Trading Doctor – The Danger of Overconfidence (http://www.marketoracle.co.uk/Article4935.html)
[2] http://en.wikipedia.org/wiki/Framing_effect_%28psychology%29
[3] Groupthink is a psychological phenomenon that occurs within groups of people and happens when the desire for harmony in a decision-making group overrides a realistic appraisal of alternatives. The Enron scandal and the Bay of Pigs decisions are two such examples.
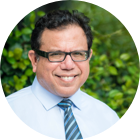
MMS • RSS
Article originally posted on Data Science Central. Visit Data Science Central
“Robotic process automation is not a physical [or] mechanical robot,” says Chris Huff, chief strategy officer at Kofax. In fact, there is no presence or involvement of any robots in the automation software, as its name says.
RPA or Robotic Process Automation is an amalgamation of three factors:
- Robotic – entities impersonating human activities and processes known as ‘Robots.’
- Process – multiple small activities that lead to one outcome or result.
- Automation – tasks done by machines and robots instead of humans.
Robotic Process Automation is a set of software robots that run on a virtual or physical machine. It automates most of the mundane and boring tasks of the business. RPA bots are capable of impersonating almost all human-computer interactions, which further tackles those business tasks, error-free. Besides, this technology works at a much faster pace and volume.
Robotic Process Automation (RPA) Market Revenues Worldwide from 2017 to 2023
Source: Statista
We believe the technology was built with the perspective to minimize the human efforts wasted in those mundane business tasks. Those repetitive tasks not only limited the progress of the humans but also delayed the project’s completion by many folds. Automation has simplified such tedious tasks, allowing us to be more productive within the given time.
Amazing Definitions of RPA by some experts:
“Robotic process automation is nothing but instructing a machine to execute mundane, repetitive manual tasks. If there is a logical step to performing a task, a bot will be able to replicate it.” – Vishnu KC, senior software analyst lead at ClaySys Technologies.
Source: Enterprisers Project
“Put simply, the role of RPA is to automate repetitive tasks that were previously handled by humans. The software is programmed to do repetitive tasks across applications and systems. The software is taught a workflow with multiple steps and applications.”– Antony Edwards, COO at Eggplant.
These definitions clearly mention how those mundane tasks can be executed better.
Four Phases (Stages) of RPA Implementation:
So, when you have decided that you don’t want to indulge your employees in those tedious business tasks. Also, you have decided to invest in this effective replacement called RPA. At this stage, you will have to go through these RPA life cycle stages, which are as follows:
Important note: Remember, that the different cultures of the organization and its bureaucracy decide how much time RPA will consume. Also, approximately 70% of the time is invested in the first two stages of these four stages of robotic process automation.
Now, moving ahead with explaining the four ultimate stages of robotic process automation, we have:
- Assess
- Approve
- Design
- Implement
Commencing with the first stage of RPA:
Source: k2 Partnering
1. Assess
So, the first stage of RPA implementation starts with assessing the entire process, its pros and cons, tasks that can be automated, and many other important questions. At this stage, you will have to figure out the answers to different questions, including:
- What will be the outcome of the implementation of RPA?
- Does your organization really need Robotic Process Automation?
- Does it really fit your organizational needs?
- How will it better the productivity of the employees?
When you start by answering all these questions, it becomes easier for you to know what the actual requirements of implementing Robotic Process Automation are in your organization.
Besides, evaluation of key criteria, including the KPIs and other important factors involved in the success of the project is also indispensable. Both these factors need proper understanding and evaluation before you finally move on to the next step.
Once the evaluation process is done, the stage will come to an end with the report that mentions what has been done if the stage, in detail. Also, the feasibility of the same will be mentioned in this assessment report when this RPA stage is completed.
2. Approve
Continuing with the next step, we have the ‘approval stage,’ of RPA, where the agreement about moving from the pilot processes to automation is decided.
Here, the decision is made regarding which tasks are to be automated, and which can’t be listed on the automation. A team will investigate the tasks mentioned in the list of automation, and scrutinize how the further process will be applied smoothly. The same is done with the proper documentation.
Once the documentation process is finished, a future process of how everything will be shifted to robots will be designed. Now, this stage will require you to compare both the task lists (the existing and the newly designed).
The process will proceed with the detailed comparison of both to analyze how exactly the changes will be adopted, and what will be the benefits experienced after this shift from human to automation.
The second or the ‘approval’ stage of robotic process automation ends when a business case is presented in front of the project’s sponsor. Besides the detailed comparison of both the existing and the plan, the total cost incurred by the project, and the expected ROI is also mentioned in the documentation.
3. Design
The third or the ‘design’ stage of the robotic automation process aka RPA decides which software vendor should be relied upon. Possibly, you would be selecting from a list of software vendors listed.
So, it is crucial to make them understand your business needs. Besides, the selection of software has to be done cautiously. Since the entire project, actions, and success of the project depends on how well the design has been prepared, it is essential to invest ample time and money in selecting the right software for your business.
Different software will deliver different results, hence the careful selection of the best one is a must. So, you will have to carefully scrutinize the requirements of your business to figure out which software best fits it. After the software is finalized, it is recommended to design the final process before proceeding.
Now, this stage of the robotic automation process aka RPA will be tested. The experts will analyze whether what has been planned and implemented until now really works, as per the expectations or not. Of course, glitches in the process will occur. But that has to be settled at this very RPA stage for further processes.
Activities that consume time without really adding any value to the organizational goals are tested using this new automated process. The glitches are fixed and the automation is increased to shift those mundane tasks to robotics.
Alterations in the processes are made until the robot is finely tuned. And, finally, when the robot completely and smoothly starts imitating the processes earlier done by the human, the orders for final release are made.
4. Implement
The last and final stage of the robotic automation process is the implementation. So, this being the last RPA stage, everything that has been researched and prepared will be implemented in the business processes now. This stage is quite enthusiastic, though. After the installation is done, the businesses will monitor whether the robot is capable enough to handle the expectations of the business or not.
In some cases, robots might not address a particular task or malfunction. That is when the robots are to be reprogrammed. For this, the IT team has to be on their toes until the robots begin to function as expected.
Once the processes are satisfactorily functioning, all four stages of RPA are fulfilled. However, there has to be a constant check on the functioning of the robotic process automation to figure out whether there is still any glitch to be fixed or everything is excellently managed by the robot.
So, these were the phases of robotic process automation, following which you can implement RPA in your business processes.
FAQs regarding Robotic Automation Process
Source: Google
Now, let’s throw some light on common questions, generally asked during the implementation of RPA.
1. Does RPA need coding?
Robotic Process Automation doesn’t require any specific programming skills. Any employee with knowledge of the subject can be trained to operate RPA right away. The robots take access to the user’s systems and manage the processes, which diminishes the requirement of any system programming or coding.
2. How do I get started with the RPA?
There are a few steps to get started with RPA implementation, which are as follows:
- Understand the vendors. Since the market is full of vendors, you will have to invest time in understanding which one best fits your requirements.
- Use the software, as many vendors will allow you to use the trial version of the software. That’s a great way to see how the product works, and will it be able to really match your business’s requirements.
- Experimenting is essential. Don’t abide by a single product or vendor. In case, one doesn’t work well, don’t hesitate to go with the other. So, if you have had a bad experience with one of those vendors, don’t assume that about everyone. Research the market and pick the best one that you believe will help you in making the project successful.
- Don’t hesitate to invest in good technology and a team, even if you need to outsource an experienced team for getting started with RPA. In the long run, it will turn out to be a good decision for your business, anyway.
3. When can RPA be used?
RPA or Robotic Process Automation should be highly used when the repetitive and mundane tasks have been amplified or increased in the business. When you can see that your employees are continuously engaged in completing tedious and repetitive tasks, it is the right time to invest in technology like RPA.
The main motive of implementing RPA in any organization is to ensure that humans are involved in much more productive work. And, these tedious tasks can be easily automated utilizing the technology known as RPA, which easily automates mundane tasks.
4. Which is the best tool for RPA in 2020?
Blue Prism is said to be one of the best tools for RPA in 2020. It is compatible with all the platforms with any type of application. The basic skills required to perform on this tool are programming skills. Also, it is a user-friendly tool for developers or programmers. Hence, Blue Prism is said to be the best tool for RPA in 2020.
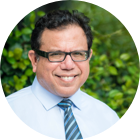
MMS • RSS
Article originally posted on Data Science Central. Visit Data Science Central
Diversity and inclusion (D&I) increasingly are becoming a focus area for businesses. And it is not just because it is the right thing to do, but it also makes excellent business sense. According to McKinsey, the top quarter of companies on the diversity list were 33 percent more likely to be among the most profitable in their industries. Also, in the world of startups, diverse founding teams earn 30 percent higher returns for their investors.
Why is D&I important?
This is because it is an essential part of creating a best-in-class work environment and workforce. Talent management professionals recognize that a diverse and inclusive workforce is able to bring about new ways of thinking and more innovation, by combining different mindsets and backgrounds.
What are the gains from D&I?
Here is what an organization stands to gain with strong D&I efforts:
- Unique cognitive attributes from diverse people fostering creativity, innovation, and problem-solving
- Better access to the required skill sets
- Minimal compliance requirements
- Improved reputation for the organization
- Better experiences for customers
When organizations step up their efforts in these areas, their ability to innovate rises by 83 percent, their customer responsiveness by 31 percent, and the effectiveness of their team collaboration by 42 percent, as per research by Deloitte. Companies that rank at the top of racial diversity scales earn 15 times the revenue of those at the bottom, according to the American Sociological Association. Inclusive cultures bring thrice as good performance, six times as much innovation, and eight times as much likelihood of achieving a better outcome for the business. And for 64 percent of candidates, their decision to take on a job offer was significantly determined by diversity.
How important is the D&I issue?
For talent management systems, the most important issue these days is making the workplace more diverse and inclusive. This requires a focus on evaluating candidates as per their capabilities and putting any sort of bias out of the picture. Toward this end, artificial intelligence (AI) and machine learning (ML) are extremely useful tools that can be employed for identifying, recruiting, and hiring candidates solely based on how capable they are, and staying clear of all bias triggers.
What gets in the way of better D&I?
Talent management professionals find a diverse and inclusive workplace easier to talk about than to actually create. Bias is the biggest issue, exerting its effect on every stage of finding the right candidate. From talent attraction and hiring to career development and performance appraisal and many other stages, bias is always a factor. It can be seen in a desire to work only with those from a similar background, or to hire people with only one profile.
How does bias affect D&I?
Conscious and unconscious biases – also known as explicit and implicit stereotyping – negatively affect daily work life and formal employment decision-making. The following are examples of bias:
- Masculine words in job advertisements for male-dominated fields, reducing their appeal to women
- Racist tones to selection or negative considerations for specially-abled candidates who are as productive as anyone else
- Lower promotion chances for women
- Poorer performance ratings for older workers and women
- Better compensation and settled job prospects for physically attractive candidates compared to those who are less attractive
- Microaggressions – verbal or non-verbal insults or slights
Why are traditional systems proving ineffective?
Traditional talent management systems place HR at the center of the workflow, not the candidate, and they rely on manual, siloed processes. Applicant tracking systems (ATSs) look less at candidate capabilities than at keywords in their profiles. Such systems, unfortunately, scale with the same biases and a reason for a success rate in hiring as low as 30 percent.
How can AI help to improve matters?
AI could be a great contributor to improving D&I efforts in hiring by companies around the world. This is how:
- Better job postings: Eliminating gendered language that otherwise discourages some applicants
- Finding diverse candidates faster: Identifying candidates with the most potential for the job at hand, irrespective of age, ethnicity, or other factors; also giving attention to often underrepresented groups
- More fair pay packages: More precise calculation of salary payouts after analyzing and parsing market data to prevent undue pay disparities
- Improved interview panels: Eliminating bias and boosting inclusiveness of the interview process, and attracting diverse candidates
- Minimized bias across the talent lifecycle: Detecting discrimination at every stage and addressing it accordingly
Is there anything to be concerned about?
For all its promise, AI in HR could increase or perpetuate bias in hiring and talent development, according to 23 percent of HR professionals responding to an IBM survey. This comes from the underlying data being collected by possibly biased humans, and the system perpetuating the same faults. Continuous testing and adjustment are the way ahead.
What are the most critical actions to take?
For talent management professionals looking to boost D&I efforts, it is important to create systems leveraging the right expertise. Standardized competency models and job descriptions can eliminate personal subjectivity. Of course, the data fed in must be high-quality and bias-free.
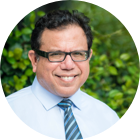
MMS • RSS
Article originally posted on Data Science Central. Visit Data Science Central
|
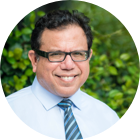
MMS • Steef-Jan Wiggers
Article originally posted on InfoQ. Visit InfoQ
AWS announces a new service called Amazon S3 Storage Lens, which can provide customers with organization-wide visibility into their object storage usage and activity trends. With the service, they can understand what happens across their S3 Object Storage installations.
By Steef-Jan Wiggers
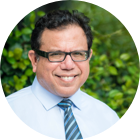
MMS • Ben Linders Arthur Yeung Dave Ulrich
Article originally posted on InfoQ. Visit InfoQ
The book Reinventing the Organization provides a framework of principles of practices that can help companies to deliver greater value in fast-moving markets. The authors explored some of today’s nimblest and fastest-growing large companies, looking at what goes on inside these companies and what’s outside: networks, partners, and the marketplace they want to dominate.
By Ben Linders, Arthur Yeung, Dave Ulrich
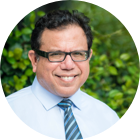
MMS • RSS
Article originally posted on Data Science Central. Visit Data Science Central
This article was written by Carlos E. Perez.
In this post I will explore further the characteristics of Artificial Intuition with the goal of describing a set of patterns that can aid us in formulating novel architectures for Deep Learning. In a previous post, I introduced the idea that there are two distinct cognitive mechanisms, one based on logical inference and another based on intuition. At least 6 decades have been spent exploring cognitive mechanisms based on logical inference without making much progress towards AGI. Deep Learning, a breakthrough discovered in 2012, revealed an alternative promising research approach based on the a different cognitive paradigm.
In the field of Psychology, Kahneman and Tversky researches the interplay of these two kinds of cognitive function in a book “Thinking, Fast and Slow”.
Kahneman’s book explores human cognitive biases and employs the dual cognitive processes as a root cause of these biases. In this post however, I will be exploring system 1 (i.e. intuition), more specifically artificial intuition and the mechanisms that give rise to it.
The origins of Deep Learning of course has had a long history. The approach originates from the Connectionist approach and derives much of its philosophy from ideas found in the Complexity sciences. In a nutshell, the idea is that emergent complex behavior can arise from simple mechanisms. Chaos and complexity are the two driving forces that exist in complex systems.
Our goal then is to either explain or better understand how emergent features arise through chaos and complexity. Here are some key features and some questions that require good answers.
To read the whole article, with questions and some of big conceptual leaps explained, click here.
DSC Ressources
- Free Book and Resources for DSC Members
- New Perspectives on Statistical Distributions and Deep Learning
- Deep Analytical Thinking and Data Science Wizardry
- Statistical Concepts Explained in Simple English
- Machine Learning Concepts Explained in One Picture
- Comprehensive Repository of Data Science and ML Resources
- Advanced Machine Learning with Basic Excel
- Difference between ML, Data Science, AI, Deep Learning, and Statistics
- Selected Business Analytics, Data Science and ML articles
- Hire a Data Scientist | Search DSC | Find a Job
- Post a Blog | Forum Questions
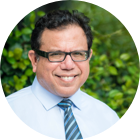
MMS • RSS
Article originally posted on Data Science Central. Visit Data Science Central
This article was written by Matthew Hughes.
CS 106A is Stanford University’s introductory programming course. The module – which is also available to view on YouTube – introduces the fundamentals of coding in an accessible way, and lays the foundations for future growth.
Throughout its existence, the course was taught using the Java programing language. Java is popular, certainly, but it’s also extremely clunky and syntactically bloated.
But a new version of the course, CS 106J is based on JavaScript.
According to the University website, “[CS 106J] covers the same material as CS 106A but does so using JavaScript, the most common language for implementing interactive web pages, instead of Java.”
The decision to ditch Java is a laudable one. While there’s a lot to like about it, Java is perhaps the harshest language you can learn as a beginner. In fact, in this respect, it’s straight-up awful.
To read the rest of the article, with illustrations, click here.
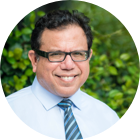
MMS • RSS
Article originally posted on Data Science Central. Visit Data Science Central
Why developers prefer Google’s Angular app development for their projects? Keep reading to find out!
Mobile app development is gaining popularity all over the world. Businesses are trying to incorporate this tech into their process in order to bridge the gap between the users and the complete process. Not only they assure convenience, but they also help in accelerating the revenue funnel. But do you know what plays the key factor in locking the success of the application?
Can you guess it???
Well, selecting the right framework is necessary to create a seamless app as per the requirement of your target user base. It can either make or break the reputation of your brand. Hence, it is necessary to have a detailed analysis of all the available choices before making a final decision. Today, in this blog we would be learning about one of the most popular framework choices for application development. We would be exploring the latest versions, the advantages of choosing this platform, and much more. But first, let us just introduce this popular framework.
A Brief Introduction To Angular!
It is used for building applications. With its ready to use libraries and thoroughly defined architecture, it presents the developer’s community with several options. Angular’s generic functionalities can help developing teams to speed up their work, and also helps developers to deal with low-level concerns that are common to all applications.
Latest Features Of Angular 10.1.0 You Have Been Waiting For!
- For static evaluation errors, more insights have been added in the new version.
- In order to boost performance in the compiler-cli, the new version has a fix for performance regressions that affect incremental program reuse.
- Multiple big fixes are being offered to parts of Angular, router, and core.
- In Angular 10.1.0, an option has been added for absolute URL HTTP support.
- Now modification of lifecycle hooks can be initiated anytime before bootstrap.
- PrioritizedGuardValue operator is being applied for router performance. This is being done to optimize CanLoad guards.
- In version 10.1.0 canparse() diagnostics are exposed.
- ProgramBasedEntryPointFinder will reuse EntryPointManifest in order to load entry-point dependencies. This will allow them to avoid having to parse them again on every invocation of logic.
- In the latest version a new message extraction tool is supported. This tool will be integrated into CLI.
- Here you can witness the performance improvements that are being made. For example- in the compiler CLI, router, the ngcc compiler compatibility tool, and forms.
- In this version, the support of TypeScript 4.0 has been added to the compiler.
- In Angular 10.1.0, LinkabablePackageInfo is being added to the ng-module rule, for Bazel build tool. This permits the linker to properly link ng_modult targets in Node.js runtime actions.
What You Can Create With Angular?
Here is the list of a variety of options that it offers-
- Mobile applications with attractive animations
- Single page web apps
- Progressive web apps
- E-commerce apps
- Enterprise apps
Here Is The List Of Angular’s Superpowers!
If you are wondering why you must create your next business app with Angular, then here is your answer. Take a look!
- Ecosystem- The ecosystem is very active and it can help your project. There is no need to rely on third-party codes. Another add-on benefit is that the documentation is regularly being improved.
- TypeScript- It saves time and decreases the number of errors.
- Official libraries- The professional team supports the most used libraries, which means fewer errors. It also helps in boosting security.
- Flexible- It delivers off-the-shelf solutions to multiple problems, which allows developers to diverge from mainstream Angular.
- Testing framework- With out-of-the-box testing tools developers can build faster.
In A Nutshell
These are the popular factors of Angular app development that everyone must know about. But this is not all, as there are several other important technicalities involved with it. If you are interested in shifting your brand on the digital platform, then you must consider this framework for the creation. Another factor that is crucial for the success of your app is a good team. So find a good angular app development company and discuss your project idea with them. Working with an experienced and talented team will help you to spread your dominance in the market.
The app industry can provide you with several opportunities to make your brand a global success. With the rise in technologies like Artificial Intelligence, Machine Learning, Augmented Reality, Blockchain, IoT, VR, etc chances of innovation have also increased.
Apart from Angular, there are multiple other efficient frameworks that you can choose from. For example, React Native, PWA, Native, Flutter, and much more. There are a lot of options that you can choose from. So what are you waiting for? Begin your digital journey today!
Feel free to reach out to us if you have any doubts, or drop your queries in the comment section. For more tech-based updates from around the world, stay tuned to this space.